- 1Tiger Watch, Maa Farm, Sawai Madhopur, India
- 2Department of Wildlife Sciences, Navsari Agricultural University, Navsari, India
Gray wolves are capable of adapting to human-dominated landscapes by utilizing domestic prey as a source of food. Livestock depredation by wolves incurs a heavy economic loss to the villagers, resulting in negative attitudes toward the species and leading to increased conservation conflict. We used multi-state occupancy modeling on the interview data to assess the ecological factors governing livestock depredation by wolves. We also assessed the socio-demographic factors that may govern the attitude of villagers toward the wolf using ordinal regression. Over the past year, 64% of respondents reported a loss of livestock, in which goats (63%) comprised the major share, followed by sheep (22%) and cattle calves (15%). Wolves tend to hunt medium-sized domestic prey (sheep and goats) that commonly graze in open agricultural areas. The estimated livestock depredation probability of wolves was 0.84 (SD = ± 0.23). Depredation probability was influenced by habitat use by wolves, the extent of agricultural areas, scrubland area, and settlement size. Respondents with prior experience of livestock loss held more negative attitudes. Shepherds held more negative attitudes than other occupations. Increases in the respondent’s age and education level reflected a positive shift in attitudes toward the wolf. High economic loss caused by livestock depredation by wolves can lead to retaliatory persecution of wolves. Adequate compensation for livestock loss, along with better education and awareness can help lead to coexistence between wolves and humans in multi-use landscape of Kailadevi Wildlife Sanctuary, Rajasthan, India.
Introduction
Wildlife conservation and management in India is mostly limited to protected areas (Ghosal et al., 2013). Large carnivores due to their large home ranges are more likely to come in contact with humans, with most of the human-wildlife interactions occurring at the edge of the protected areas where carnivores, people, and livestock overlap (Nyhus and Tilson, 2004; Woodroffe et al., 2005). Therefore, large carnivores residing outside the protected areas in highly human-dominated landscapes require effective management for their conservation (Linnell et al., 2001). For instance, snow leopards (Panthera uncia) in the Himalayan region and leopards (Panthera pardus) in India and Pakistan tend to kill more livestock near the human habitation where the livestock is high in number and easily accessible (Dar et al., 2009; Aryal et al., 2014; Miller et al., 2015), whereas tigers (Panthera tigris) generally depredate livestock inside the forest, away from villages and human habitation (Wang and Macdonald, 2006; Miller et al., 2015). However, due to the degraded status of their habitats, wolves (Canis lupus pallipes) in India generally reside outside the protected areas where most of the interactions between wolves and humans occur (Mahajan and Khandal, 2021). Conservation of carnivores in shared landscapes is the major challenge for the persistence of large carnivores (Chapron et al., 2014; Majgaonkar et al., 2019), therefore achieving harmonious coexistence of humans with carnivores is the ultimate goal for the survival of the carnivores in these shared landscapes (Linnell et al., 2001; Carter and Linnell, 2016).
The social drivers of human–wildlife interactions also play an important role in achieving coexistence (Dickman, 2010). Considering the importance of livestock to the local economy, understanding people’s attitudes toward carnivores is also important for effective conservation planning (Bagchi and Mishra, 2006). It is thus crucial to identify and address both the ecological and social factors to understand the complex interactions between humans and wildlife (Gálvez et al., 2018; Lischka et al., 2018). People’s perceptions and attitudes toward a species are based on personal experiences, social and cultural norms, knowledge of their surroundings, and beliefs associated with the species (Dickman, 2010), which affect the level of tolerance toward a particular species. Therefore, to ensure long-term persistence of a carnivore in a human-dominated landscape, it is pertinent to assess the relative roles of potential ecological and social drivers of human-carnivore interactions to better understand the social carrying capacity of a carnivore in a landscape.
Livestock depredation by carnivores is a complex phenomenon governed by multiple factors (Inskip and Zimmermann, 2009). Areas predisposed to livestock depredation are influenced by ecological factors like the availability of wild prey (Gurung et al., 2008; Kaartinen et al., 2009; Sharma et al., 2015), density of livestock (Mech et al., 2000; Wang and Macdonald, 2006; Aryal et al., 2014; Carvalho, Zarco-Gonzalez et al., 2015; Suryawanshi et al., 2017), habitat characteristics (Treves et al., 2004; Kaartinen et al., 2009; Suryawanshi et al., 2013; Davie et al., 2014), and livestock husbandry management (Kolowski and Holekamp, 2006; Wang and Macdonald, 2006; Abade et al., 2014). Apart from these, social factors like gender (Ogra, 2009: Koziarski et al., 2016), education level (Mkonyi et al., 2017; Behmanesh et al., 2018), religion (Dickman et al., 2014; Arbieu et al., 2019), and economic importance of livestock to a community, shape the attitudes, perceptions, and belief systems of people toward a carnivore (Dickman, 2010) and govern the type and severity of human response toward them. Identifying relevant ecological and socio-economic factors associated with livestock depredation is crucial to understanding complex human-carnivore interactions and accordingly prioritizing conservation activities.
Wolves, due to their large home ranges and dietary requirements, are difficult to conserve in small protected areas of India, where the average size is 240 km2 (UNEP-WCMC, 2021). Moreover, most of these protected areas are surrounded by high densities of human settlements, and wolves in such human-dominated landscapes can utilize domestic prey as a source of food. Wolf predation on livestock severely affects the economy of the pastoral communities that barely manage to eke out a living from the highly overgrazed and degraded landscapes of semi-arid India (Mahajan and Khandal, 2019). This often brings them into conflict with wolves (Kumar and Rahmani, 2000; Krithivasan et al., 2009; Palei et al., 2013; Behmanesh et al., 2018), sometimes leading to retaliatory persecution of wolves by indirect means such as smoking out dens to kill pups (Shahi, 1982; Kumar and Rahmani, 2000; Singh and Kumara, 2006) and by using poison (Jhala, 2003; Mahajan and Khandal, 2019). For the small-scale households living near the protected areas, the loss arising due to livestock depredation poses a challenge to rural development and biodiversity conservation (Treves and Karanth, 2003; Khorozyan et al., 2015). This is particularly important for the conservation of wolves in India due to their persistence in human-dominated landscapes. Unlike tigers and leopards which commonly reside within the dedicated protected areas, wolf habitats do not come under the protected area network, and most human-wolf interactions occur due to the Wolf’s dietary dependence on livestock and lack of wild prey (Mahajan et al., 2021).
In present study, we used a socio-ecological framework for examining human-wolf interactions in the human-dominated landscape of Kailadevi Wildlife Sanctuary. The covariates used to model the attack on livestock by wolves were selected based on earlier studies conducted on human-wolf interactions (Agarwala and Kumar, 2009; Krithivasan et al., 2009; Majgaonkar et al., 2019; Srivathsa et al., 2019; Rehman et al., 2021). We hypothesized that attacks on livestock will be more frequent in areas or habitats that are used by wolves (Majgaonkar et al., 2019; Srivathsa et al., 2019). Earlier studies have shown that wolves use habitats where livestock is easily accessible (Agarwala and Kumar, 2009; Mahajan et al., 2021). Presence of water and scrubland are defined as important limiting factors for wolves (Jhala, 2003; Singh and Kumara, 2006), therefore, we predicted that these areas will have a positive influence on the probability of attack on livestock. High forest cover areas are generally avoided by wolves (Mahajan et al., 2021), therefore, we predicated those areas with high forest cover will have negative influence on the probability of livestock attack. Moreover, predation on livestock by wolves has also been linked to low densities of wild prey in Southern Europe (Meriggi and Lovari, 1996) and North America (Mech et al., 2000; but see Treves et al., 2004). In a few protected areas in India, where native ungulate wild prey species such as blackbuck (Antilope cervipera) and chinkara (Gazella bennettii) are readily available, wolves prefer wild prey over livestock (Kumar and Rahmani, 1997; Jhala, 2003). However, due to low availability of wild prey in the study area (Mahajan and Khandal, 2021), we hypothesized that wolf will switch to alternative prey i.e., livestock, which is easily available and assessable. We therefore, predicted that availability of wild prey will have the negative influence while domestic prey availability will have the positive influence on the probability of livestock attack. Moreover, we hypothesized that anthropogenic factors like large human settlements will have a negative influence on the probability of livestock attack, since more disturbance can deter the presence of wolves (Srivathsa et al., 2019). However, open agricultural areas are generally grazing grounds for medium sized livestock and thus provide an opportunity to wolves to hunt livestock in those areas (Majgaonkar et al., 2019; Mahajan et al., 2021), we therefore speculated that agricultural areas would aid wolves to attack livestock.
Certain socio-demographic factors were also selected to model the attitude of people toward wolves. Caste of the respondents, for example can negatively or positively influence perceptions toward animals based on cultural values associated among different caste systems (Dickman, 2010; Arbieu et al., 2019). Age is another important factor which influences the behavior of respondents toward wolf. Based on previous studies on wolves we hypothesized that older people would hold more negative attitudes than younger people (Kellert, 1985; Kaltenborn et al., 1999; Røskaft et al., 2007; Majić and Bath, 2010). Similarly, we predicted that people with more formal education would be more likely to respond positively toward wolf (Kellert, 1985; Majić and Bath, 2010; Mkonyi et al., 2017). Different types of occupation can also positively or negatively impact the attitude of people toward wolf conservation (Kellert, 1985; Kaltenborn et al., 1999; Williams et al., 2002; Carlson et al., 2020). We hypothesized that shepherds would hold more negative attitude than other occupations as they encounter wolves more frequently. Moreover, people with prior experience of livestock loss to wolves would hold a more negative attitude than respondents who have not suffered any livestock loss (Williams et al., 2002; Røskaft et al., 2007).
We combined both ecological and social aspects of human-carnivore interactions in a single coherent framework using the approach developed by Gálvez et al. (2018). We used interview surveys with local people which provide both cost-effective and reliable information for the conservation of species at large spatial scales (Zeller et al., 2011; Rich et al., 2013). We first collected ecological data on habitat use by wolves (Mahajan et al., 2021), and on the same spatial scale, we conducted socio-ecological interviews to determine the drivers of livestock depredation by wolves. We further used the interviews to assess the socio-demographic factors shaping the perceptions of the respondents toward human-wolf interactions. We used occupancy models to determine the suite of ecological and social factors that govern livestock depredation by wolves. Occupancy models are based on the detection and non-detection of species over multiple surveys to reduce the chances of “false negative” which helps in accounting for the detection probability (MacKenzie and Royle, 2005). Using occupancy models with interview data has been applied across multiple studies to understand human-wildlife interactions (Goswami et al., 2015; Srivathsa et al., 2019; Puri et al., 2020; Bista et al., 2021). Based on the results of our study we provided recommendations for conserving the highly neglected species of semi-arid landscapes of India that can aid the overall coexistence of carnivores and humans in shared landscapes.
Materials and Methods
Study Area
Kailadevi Wildlife Sanctuary (KWLS) forms the northern boundary of Ranthambhore Tiger Reserve (RTR) (26°13′40.05″N–26°15′17.42″N, 76°35′52.68″E–77°13′52.45″E). It is located in the Karauli district of the western Indian state of Rajasthan (Figure 1). The sanctuary covers an area of 673 Km2 and for its effective management the sanctuary is further divided into four administrative ranges (Figure 1) namely Kailadevi, Karanpur, Mandrail, and Nainyaki. The KWLS falls within the semi-arid climatic zone. It experiences three distinct seasons, monsoon, winter, and summer. More than 90% of the annual precipitation occurs during monsoon (July–September) with an average of 750–800 mm of rainfall. October is a transition period between monsoon and winter. The two major rivers Chambal and Banas form the southern and western boundaries of the sanctuary, respectively. The parallel running ridges forming deep gorges are an important geographical feature of the sanctuary and are locally known as “Khoh.”
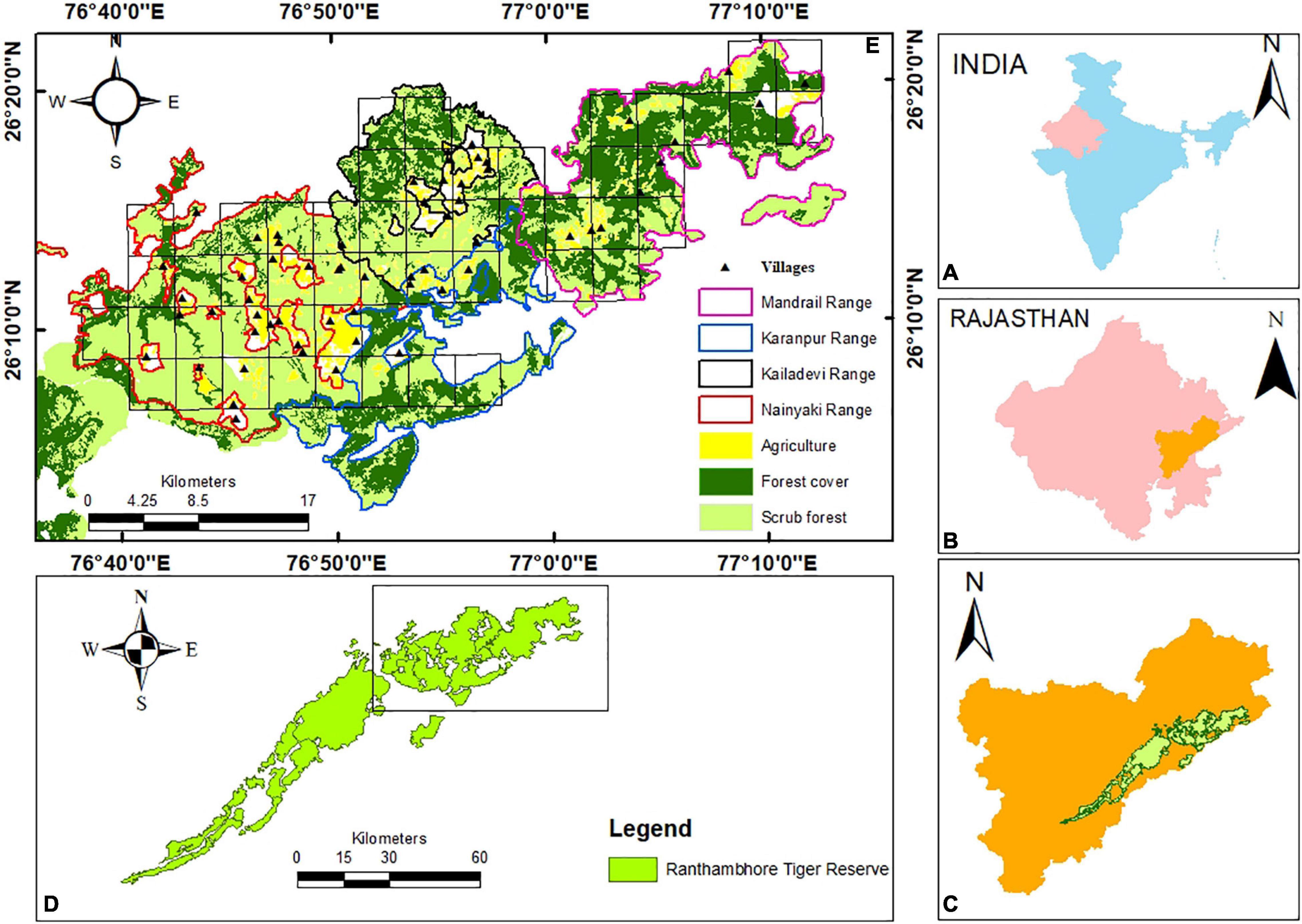
Figure 1. Study area showing the grids with villages inside the Kailadevi Wildlife Sanctuary, Rajasthan, India. (A) The state of Rajasthan, India. (B) District in Rajasthan where the study area is located. (C) Location of study area within the district. (D) Ranthambhore Tiger Reserve, where the marked block represents the KWLS. (E) Kailadevi Wildlife Sanctuary, Rajasthan, India.
The forests of the KWLS are mainly composed of Northern Tropical Dry Deciduous forests, Zizyphus scrub, dry deciduous scrub, and dry Grass lands (Champion and Seth, 1968). Dhonk (Anogeissus pendula) is the dominant tree species in the sanctuary constituting nearly 80% of the vegetation cover. The KWLS supports a rich diversity of mammalian species such as the Tiger, Leopard, Indian Gray Wolf, Golden Jackal (Canis aureus), Sloth Bear (Melursus ursinus), Indian Striped Hyena (Hyaena Hyaena), Indian Fox (Vulpes bengalensis), Indian crested Porcupine (Hystrix indica), Wild-pig (Sus scrofa), Honey badger (Mellivora capensis), Jungle cat (Felis chaus), Caracal (Caracal caracal), Rusty Spotted Cat (Prionailurus rubiginosus), Common Civet (Paradoxurus hermaphroditus), Small Indian civet (Viverricula indica), Sambar (Rusa unicolor), Cheetal (Axis axis), Bluebull (Boselaphus tragocamelus), and Chinkara (Gazella bennettii).
KWLS is a human-dominated landscape and is home to several agro-pastoralist communities that are substantially dependent on its resources for their livelihood. Currently, there are 60 villages inside the KWLS with a total of 4,773 households and 18,344 people (Mahajan and Khandal, 2019). Most of the villages have a multi-caste composition among which Meenas and the Gurjars are the most predominant communities. Villages inside the forest and in its peripheries exert immense pressure on the forest for resources like timber, fodder, etc.
Field Surveys
To record the presence of livestock depredation by wolves, we conducted semi-structured questionnaire surveys from October 2018 to December 2018 in grids of 14.4 km2 each, across the KWLS for the events pertaining to the previous year. We collected both presences of indirect signs of the wolf (Mahajan et al., 2021) and the presence of any depredation event at the same spatial scale (Zeller et al., 2011; Gálvez et al., 2018; Srivathsa et al., 2019). The presence of indirect signs was observed in each grid by searching for indirect signs near roads and trails. Each kilometer walked inside a grid was considered as a single spatial replicate. The presence/absence data thus generated was used to estimate the habitat-use of wolves with the suite of ecological and anthropological covariates using a single-season correlated detection occupancy modeling approach (Mahajan et al., 2021). Using the same grids as our sampling units, we conducted socio-ecological interview surveys to know the presence of livestock attack by wolves in KWLS. Out of 48 grids, villages were present in 29 grids and we, therefore, conducted interviews in those grids only. We selected local residents of the KWLS to conduct questionnaire interviews. Prior to the survey, all these volunteers were trained on how to conduct interviews and to avoid any ambiguity generated through the respondent’s answers. These volunteers held more than 10 years of experience in conducting ecological surveys (Mahajan and Khandal, 2019). To avoid any false positives, respondents were shown pictures of different species found in the study area and were asked to identify wolves. Upon correct identification, the respondents were asked if they have encountered or seen the wolves in and around their village and their responses were validated through the presence of indirect signs if the encounter was recent (not more than 5 days). They were also asked about incidents of livestock depredation by wolves for the last 12 months (Puri et al., 2020). Respondents that were unable to correctly identify wolves from photographs were discarded and were considered as “missing observations” for the occupancy analysis. We further collected information on the respondent’s family demographics which included the number of males and females in the family, age of different family members, education level, caste, and occupation of the respondent. Other information like number of different livestock holdings, various measures to mitigate livestock depredation, and compensation claimed for the loss of livestock by wolves was also collected. To understand the attitude and perceptions of respondents toward livestock depredation by we asked specific questions like their opinion on what should be done regarding the loss of livestock caused by wolves and what they believe regarding wolf conservation in KWLS. We conducted a total of 442 interviews across the KWLS to assess the effect of socio-demographic factors on people’s attitudes toward the wolves. Out of multiple respondents in grids, each respondent was considered a survey-specific replicate that allows estimation of detection probability (MacKenzie et al., 2006). Out of 442 we only considered 271 (maximum of 10 replicates in each grid) interviews to avoid correlations among multiple replicates within a grid. Also, we assumed that due to high detection probability of livestock depredation by wolves, fewer replicates would be enough to model detection probability (MacKenzie and Royle, 2005).
Model Covariates for Livestock Depredation and Human Attitudes Toward Wolf
Values of wolf habitat use, availability of water, scrubland, forest cover, wild prey availability, domestic prey availability, anthropogenic disturbance, and presence of agricultural land in the grids were taken from another study on habitat-use by wolves (for information on how these values for each grid were generated; see Mahajan et al., 2021). The area of human settlements in each grid was calculated by digitizing human settlements using Google Earth imagery (2017–2018). Boundaries of settlements were hand drawn to form polygons and the area of human settlements in each grid was computed in QGIS (Version 2.18.25- Pisa, QGIS Development Team, 2018). All covariates were standardized by calculating z-scores (y-ȳ)/SDy. Prior to developing any model, Pearson’s correlation values were calculated to minimize the effect of correlations among covariates and only one variable among the highly correlated pairs (r > 0.7) was retained (Dormann et al., 2013).
For modeling the attitudes, socio demographic factors were selected from the interview data. The predictor variables were age, caste, education, occupation and previous attack on livestock. Age was the only continuous variable while other factors were categorical. Caste was categorized into 3 groups where “1,” “2,” and “3” represents Gurjar, Meena, and others, respectively. Similarly, level of education was also categorized into 3 groups where “1” represents respondents whose education level was up to 5th standard or below, “2” represents respondents whose education level was from 6th to 10th standard and “3” represents respondents who were educated above 10th standard. The occupation was categorized into four groups in which “1” represents laborer, “2” represents farmer, “3” represents shepherd and “4” represents respondents belonging to other occupations. Respondents who had experienced wolf attack on livestock were labeled as “1” while those who did not experience any attack by wolves were labeled as “0.”
Estimating Determinants of Livestock Depredation
We used a multi-state occupancy model to assess the pattern of livestock depredation by wolves (MacKenzie et al., 2009). The data generated from the interview surveys were classified as “0,” “1,” and “2” in the design matrix, where “0” represents the absence of wolf in a grid, “1” represents the presence of wolves in a grid but without depredation, and “2” represents depredation by wolves in a grid. The following parameters were estimated: Ψp—probability of wolf presence in a grid (without depredation); Ψd—probability of depredation by wolves in a grid; ppp—probability of detecting wolf presence in a grid; pdd—probability of detecting depredation in a grid; ppd —probability of detecting only presence although there may be depredation in the grid. The probability of depredation in a site was modeled as a function of covariates using the multinomial-logit link function (MacKenzie et al., 2009). To estimate detection probability we used the number of interviews in a grid (i.e., survey effort) as a covariate (Srivathsa et al., 2019; Puri et al., 2020). Since our parameter of interest was the probability of depredation (Ψd), the probability of wolf presence (Ψp) was retained as intercept-only to avoid issues of overfitting and to maintain parsimony. We ran a set of 10 plausible models with different covariates for modeling the probability of depredation (Supplementary Table 1). We also ran a null model without covariates. We used Akaike’s Information Criterion (AIC) to rank and select the best models. Akaike weights (w) were also computed for the models in the candidate set to compare the weight of evidence (Burnham and Anderson, 2002). Since no model stood out as the best based on AIC values, averaging of models with values ΔAIC < 2 was used to derive estimates of depredation probability at the site level (Burnham and Anderson, 2002). We selected the five best models out of 10 using AIC with the lowest AIC value and Akaike weights (cumulative wi of 0.99, ΔAIC < 2). β-coefficients and associated Standard Errors (SE) of selected models were assessed to know the effect of the covariates on the probability of depredation. Analyses were performed using the single-season multi-state model in program PRESENCE (Version 2.12.22, Hines, 2006). A spatially explicit map depicting the grids with high and low model-average probabilities of depredation by wolves across the different sites was generated using QGIS (Figure 2).
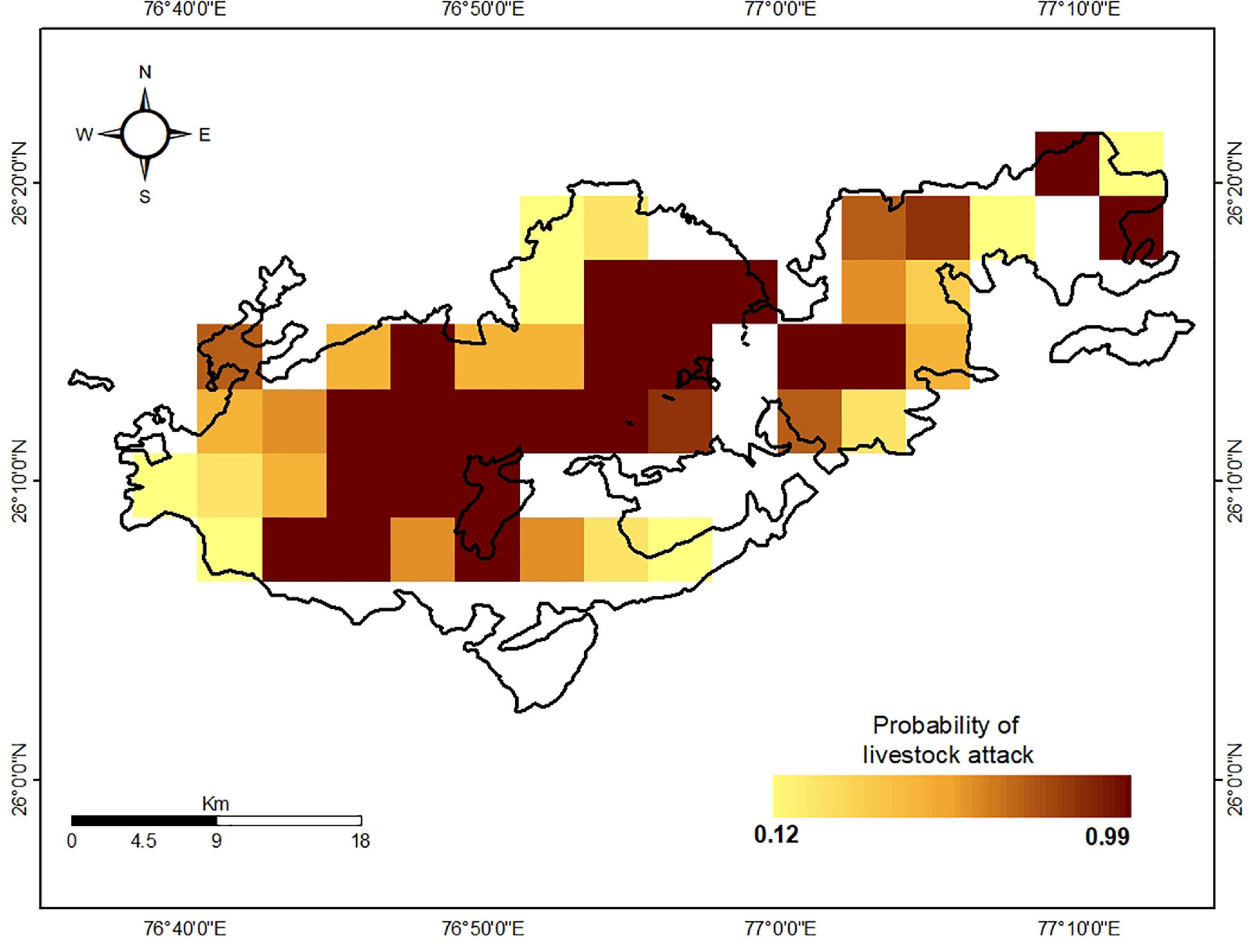
Figure 2. Map showing estimated probability of livestock attack by wolves based on interview surveys across the Kailadevi Wildlife Sanctuary, Rajasthan, India.
Patterns of Livestock Depredation and Socio-Demographic Determinants Associated With Attitudes of Respondents Toward the Wolf
We used descriptive statistics to characterize patterns of livestock depredation by wolves. The Chi-square goodness of fit test was used to examine (i) the association between the range of KWLS and the frequencies of livestock killed by wolves and (ii) the difference in the frequencies of different livestock killed. Ordinal logistic regression was used to determine the effects of socio-demographic factors on people’s attitudes toward the wolves. Ordinal attitudinal scores were entered in the model as response variables, and socio-demographic factors including age, caste, education level, occupation, and previous attack on livestock were considered as predictor variables. AICc scores were used to rank and select the models that accounted for a small sample size. Prior to the analysis, we performed a Spearman’s Rank correlation matrix to confirm that none of the variables were inter-correlated using Spearman’s rho (rs)> 0.7 as a criterion for exclusion. The probability graphs of respondent attitudes toward wolves with the effect of different socio-demographic variables as predicted by the ordinal logistic regression were produced to know the importance of the variables. The importance of response variables ranges from 0 to 1, with importance values of 1 indicating that the variable made a strong contribution to the model. We examined the relationship of each predictor variable to predict the probability of respondent attitude. All the statistical analyses were carried out using R (v.3.5.0, R Development Core Team, 2021).
Results
We conducted questionnaire interviews across 58 villages inside the KWLS (Table 1). We surveyed 15% of the total households present in each village. Respondents were primarily men (99%), of which 6% were educated till the 8th grade. The remaining respondents (94%) had no formal education or had dropped out of formal education before the 8th grade. The majority of the respondents were from the Gurjar community (63%), which are either pastoralists or agriculturists. The majority of the households (92%) were engaged in livestock rearing, of which goat (73%) formed the major livestock, followed by cattle (15%), sheep (11.5%), and donkey (0.5%).
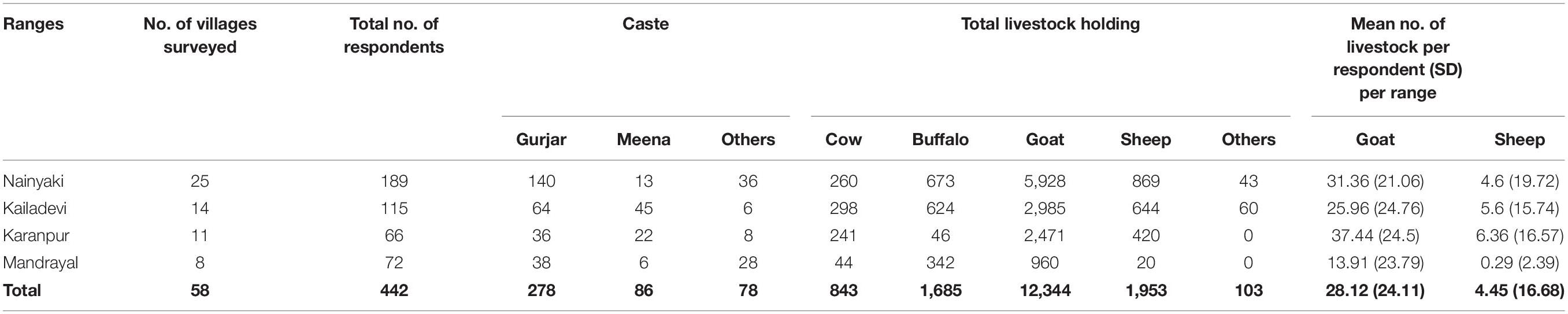
Table 1. Demographic characteristics of surveyed villages and livestock holdings across the ranges of Kailadevi Wildlife Sanctuary, Rajasthan, India.
Momentary Loss and Patterns of Livestock Depredation by Wolves
In the last 1 year, 283 (64%) respondents reported livestock losses due to wolf attacks. Respondents reported a total loss of 943 livestock due to wolf depredation (Table 2). Depredation of goats (63%) by wolves was significantly higher than sheep (22%), cow calves (14%) and buffalo calves (1%) (χ2 = 799.52, d.f. = 3, p < 0.01). Frequency of livestock depredation was reported significantly more from villagers in the Nainyaki range (44%), followed by Kailadevi (31%), Karanpur (17%), and Mandrail (8%) ranges (χ2 = 81.72, d.f. = 3, p < 0.01). The majority of the attacks occurred during the day time (96%) while the shepherds were herding their livestock and few instances of livestock depredation occurred during the nighttime (4%).

Table 2. Frequency of livestock depredation by wolves across the ranges of Kailadevi Wildlife Sanctuary, Rajasthan, India.
Out of 283 reported cases of livestock depredation, 265 (94%) reportedly did not file for compensation, while 18 (6%) respondents filed claims for the year 2017–1018. Out of the 18 who filed for compensation, 15 individuals received compensation, while 3 individuals did not receive any compensation. The majority of the respondents who didn’t file for compensation stated that they were unaware of any compensation scheme for livestock loss caused by wolves (93%), while the remaining respondents stated other reasons, such as the non-receipt of compensation in a previous case, a greater investment of resources in the application than the compensation amount, and unavailability of the forest department officers when applying for compensation. Livestock depredation in terms of monetary loss was estimated using average local prices of livestock in 2017–2018. The value of each livestock type was calculated according to the species and age. The average amount of annual loss for households that reported livestock loss (n = 283) by wolves was valued to be around 230 USD. The total amount of compensation received by the 15 people who had filed for compensation was 761 USD for a total of 60 livestock heads comprising 40 goats, 8 sheep, 11 cow calves, and 1 buffalo calf. Based on the total number of livestock holdings of the respondents (Table 1), the percentage of goat and sheep loss over a year due to wolves was 5 and 1%, respectively.
Based on interview surveys, we established that livestock in the Kailadevi Wildlife Sanctuary is pastured from early morning (approximately 2–3 h after sunrise) to 1.5–2 h before sunset (Mahajan and Khandal, 2019). Respondents revealed that cattle were usually unguarded, but for goats and sheep at least one person remained on guard while the animals were grazing. The majority (67%) of the respondents stated that they stayed with their livestock and made loud noises during herding in order to protect their livestock from wolves, while other 18% of the respondents stated that they did nothing to protect their herd against the wolves. Only 7% of the respondents stated that they keep their livestock in a fenced enclosure during the night time, while 8% stated that they used vigilance both during the day and night time to prevent livestock from wolf attack and used fencing in the night time to guard their livestock. The mesh pens used to guard the livestock are not very well constructed and cannot keep the wolves away from attacking livestock (Krithivasan et al., 2009). Most of the livestock guarding techniques used by shepherds in KWLS is not very effective to prevent livestock depredation.
Determinants of Livestock Depredation and Attitudes of Respondents Toward Wolves
The probability of depredation across the sites in KWLS was modeled using the data from 271 interviews conducted with the villagers. The naïve probability (proportion of grids in which livestock depredation was reported without taking detection probability into account) of livestock depredation was 0.58 in KWLS during the survey. There was a high correlation between the availability of agricultural land and the habitat use of wolves (r = 0.73) and also between the domestic prey and availability of agricultural land (r = 0.77). Therefore, these two pairs were not included together while building the models. The estimated depredation probability by wolves after model averaging was estimated at 0.84 (SD = ± 0.23). Based on the ΔAIC and Akaike weight, the habitats frequently used by wolves, the presence of agricultural areas, the presence of scrubland, and the area of settlements were the most reliable in explaining the probability of livestock depredation at a site (Table 3). β-coefficient values indicated that the depredation probability at a site was positively influenced by the habitat-use probability by wolves, extent of agricultural area, scrubland, and settlement size depending on the model (Table 4). The direction of slopes for all covariates except the settlement size were consistent with our a priori predictions. Therefore, the areas which had a high intensity of usage by wolves, the presence of agriculture, scrubland, and human settlements had a higher probability of livestock attack by wolves
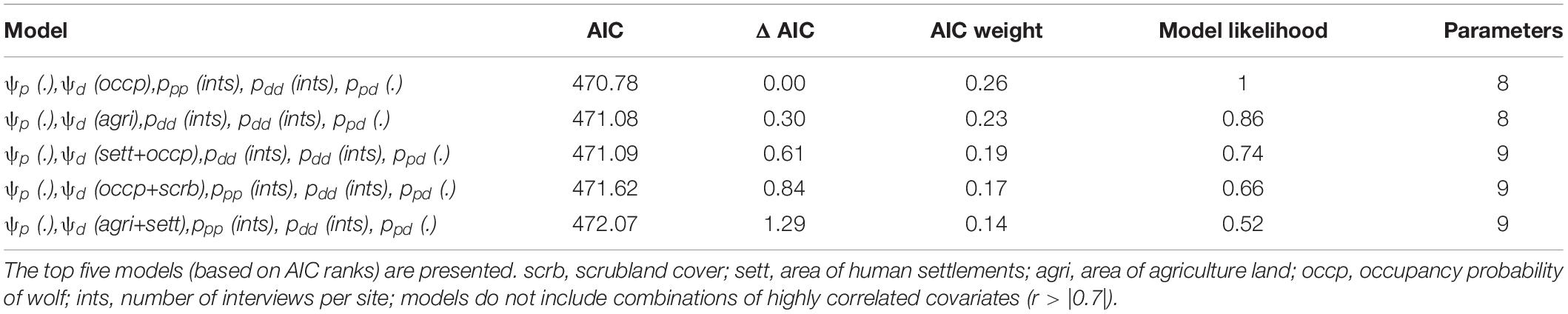
Table 3. Model comparisons for probability of presence-only without depredation (ψp), probability of depredation (ψd), and associated detection probabilities (ppp, pdd, ppd) in the Kailadevi Wildlife Sanctuary, Rajasthan, India, 2018.

Table 4. β-coefficients (± SE) from most-supported models used to assess the effect of variables on Ψd (Depredation probability) of wolves in Kailadevi Wildlife Sanctuary, Rajasthan, India.
When asked if wolves should be conserved or not, 66% of the respondents said that they had no opinion (Neutral) about conserving wolves, 18% responded positively toward wolf conservation, while the remaining 16% (Negative) believed that wolves should not be conserved and should be either removed or exterminated from the area near their villages. In response to the question “what should be done to reduce losses due to wolves?,” a majority of 54% of respondents believed that nothing could be done as wolves are wild animals who need food for survival and thus, will continue to kill their livestock, whereas 29% of the respondents believed that wolves should be entirely removed from the area with another 7% of the opinion that wolves should be killed to reduce livestock losses. Conversely, 10% of the surveyed people were tolerant of the losses caused by wolves (Figure 3). The majority of respondents (94%) either had no formal education or had dropped out before the 8th grade, out of which 63% held neutral attitudes toward wolves while 17 and 14% held positive and negative attitudes, respectively. Most of the respondents held neutral attitudes toward wolves irrespective of whether they had suffered from livestock losses or not.
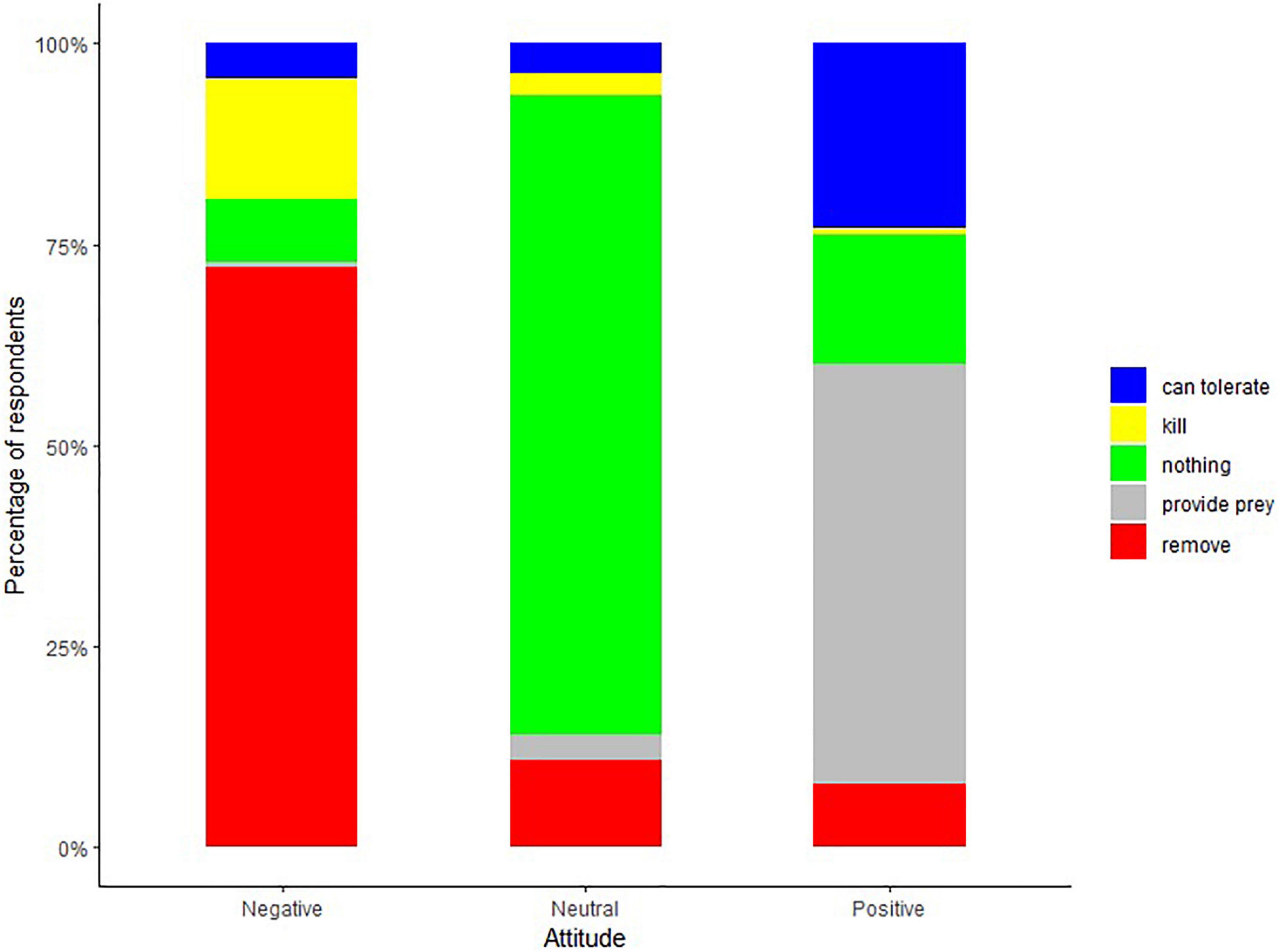
Figure 3. Comparison of attitudes of respondents toward the wolves, for the question “what should be done to reduce the loss caused by wolves”.
Using ΔAIC and Akaike weight (wi of 0.98, ΔAIC < 2) the ordinal regression revealed that the occupation of respondents had the greatest influence on the attitude toward wolf (Table 5). Shepherds held more negative views toward the wolf than farmers and other occupations. Also, with an increase in a respondent’s age, the attitude shifted more toward positive, although respondents with prior experience of livestock loss held more negative attitudes. Moreover, shepherds with previous experience of livestock loss were more likely to hold negative attitudes than shepherds who didn’t have any experience of livestock loss. Education did not have a significant effect on the attitude of respondents toward the wolf.

Table 5. Model comparison for socio-demographic factors (Occupation, Attack, Age, Caste, Education) affecting attitudes of respondents toward the wolf in Kailadevi Wildlife Sanctuary, Rajasthan, India.
Discussion
Patterns and Determinants of Livestock Depredation by Wolves
Our study examined the relevant social and anthropogenic factors that govern the attack on livestock by wolves. The attack on livestock in a site was majorly influenced by four factors which included, the probability of habitat used by the wolf in a site, the extent of agricultural area, settlement size, and availability of scrubland. As predicted, the probability of an attack on livestock was higher in those sites which are used more by wolves and have higher availability of scrubland and agricultural area. However, contrary to our prediction the size of settlements positively influenced the attack on livestock. Our results show that goats and sheep were the major livestock depredated by wolves. Previous studies have also shown that goats and sheep form the major prey of wolves outside the protected areas (Kumar and Rahmani, 2000; Behmanesh et al., 2018; Srivathsa et al., 2019; Khan et al., 2020; Rehman et al., 2021). Depredation of sheeps and goats may be related to higher vulnerability associated with their easy handling due to medium body size and absence of anti-predator behavior. Moreover, in our study area due to the low availability of wild prey (Jhala et al., 2020; Mahajan and Khandal, 2021), wolves might be dependent upon livestock. Livestock depredation by carnivores is also related to high carnivore density in an area (Kolowski and Holekamp, 2006). Our results reveal that the frequency of depredation was greater in the Nainyaki range than in other ranges, which might be attributed to the high density of wolves in the Nainyaki range (Mahajan and Khandal, 2021) and also due to greater availability of sheep and goats which might attract the wolves toward them due to large livestock holdings (Mech et al., 2000; Palei et al., 2013; Srivathsa et al., 2019). In our study, most of the livestock depredation by wolves occurred during the day, which was consistent with the other studies (Kumar and Rahmani, 2000; Palei et al., 2013) and might be due to an overlap of wolf activity pattern with peak livestock grazing activity away from the human settlements during the day. However, Krithivasan et al. (2009) recorded that among the nomadic shepherds, most of the attacks on the livestock occurred during the night.
The predictions of our factors were in accordance with the other studies conducted on human–wolf interactions. For instance, in Mongolia, Davie et al. (2014), also found that the risk of livestock depredation reflects the patterns of space use by wolves. Mahajan et al. (2021) and Majgaonkar et al. (2019) in Rajasthan and Maharashtra, respectively, found that harvested agricultural plots attract wolves since these areas are grazed by livestock after crops are harvested, providing an opportunity for the wolves to hunt sheeps and goats. Past and existing destruction of wolf habitats can cause wolves a future ecological loss known as extinction debt (Tilman et al., 1994). As a strategy to escape the extinction debt, wolves have adapted to living in agricultural lands through their dependence on livestock (Agarwala and Kumar, 2009). Moreover, in a study in Western Iran, Behdarvand et al. (2014) found that areas away from human settlements and a higher proportion of dry farms increased the probability of livestock depredation by wolves. However, where wolves depend on wild prey, the presence of wolves in agricultural lands is mostly due to the use of agricultural lands by wild prey (Chavez and Gese, 2006). In contrast to other studies (Behdarvand et al., 2014; Majgaonkar et al., 2019; Srivathsa et al., 2019), we found that the size of the human settlements was positively related to the livestock depredation by wolves in a site. This may be due to the fact that larger settlements have larger livestock holdings, and thus attracting wolves. However, it is important to demarcate the threshold at which the probability will be positively influenced by livestock holdings, beyond which it would either become stable or would exert a negative influence (Sharma et al., 2015). Our results do not reflect the presence of wild prey on the probability of livestock depredation, which may be an artifact of low wild prey density in the study area (Mahajan and Khandal, 2021).
Socio-Demographic Factors Govern the Attitude Toward the Wolf
The perception of a conflict can be quite different from the actual scale of a problem (Suryawanshi et al., 2013). The attitude toward a species can therefore misrepresent the scale of conflict causing people to take retributive action. Even though wolves in KWLS cause substantially high economic loss, the villagers are quite tolerant of the species. People were generally accepting of wolves in their surroundings but did not want them to depredate their livestock as they are heavily dependent upon their livestock, and having more livestock is a symbol of wealth. Therefore, if no immediate mitigation actions are taken to resolve livestock depredation by wolves, the attitudes of people can shift negatively, which can be detrimental to the conservation of wolves in KWLS.
The direction of the slope of people’s attitude was consistent with our prior hypothesis for most of our selected socio-demographic factors (Figure 4). Many studies on carnivores have found that older people hold more negative attitudes than the younger population (Røskaft et al., 2007; Majić and Bath, 2010; Liu et al., 2011). Deep rooted cultural beliefs and traditions mostly shape the attitude of older people toward wolves. In contrast, older people’s positive attitudes may result from personal experiences related to their prolonged residency and exposure to wolves (Mkonyi et al., 2017). Our results similarly suggest that older people in KWLS hold less negative attitude, although the results were not significant. On the contrary, younger people had more negative attitudes toward wolves, which may be attributed to their occupation. Most of the younger population in KWLS comprises shepherds, an occupation that entails greater encounters with wolves, and livestock depredation (Mahajan and Khandal, 2021). Such negative experiences, sometimes very frequent, often manifest as negative attitudes in the younger generation especially among shepherds who hold more negative attitudes than those in other occupations. Our results were consistent with other studies that have also found people with large livestock holdings or occupations related to the rearing of livestock, hold more negative attitudes than any other occupations (Kaltenborn et al., 1999; Williams et al., 2002; Liu et al., 2011; Carlson et al., 2020). Our results suggest that prior experience of losing livestock to a carnivore can also generate negative attitudes among villagers. Shepherds having prior experience of livestock loss hold more negative attitudes than people who did not experience any loss. In China, Liu et al. (2011) observed that individuals who did not suffer damage due to the Asiatic black bear (Ursus thibetanus) held 4.8 times more positive attitudes than the people who have experienced loss to bears. Dickman et al. (2014), in Tanzania’s Ruaha Landscape, also reported that people hold more negative attitudes if they have prior experience of livestock depredation.
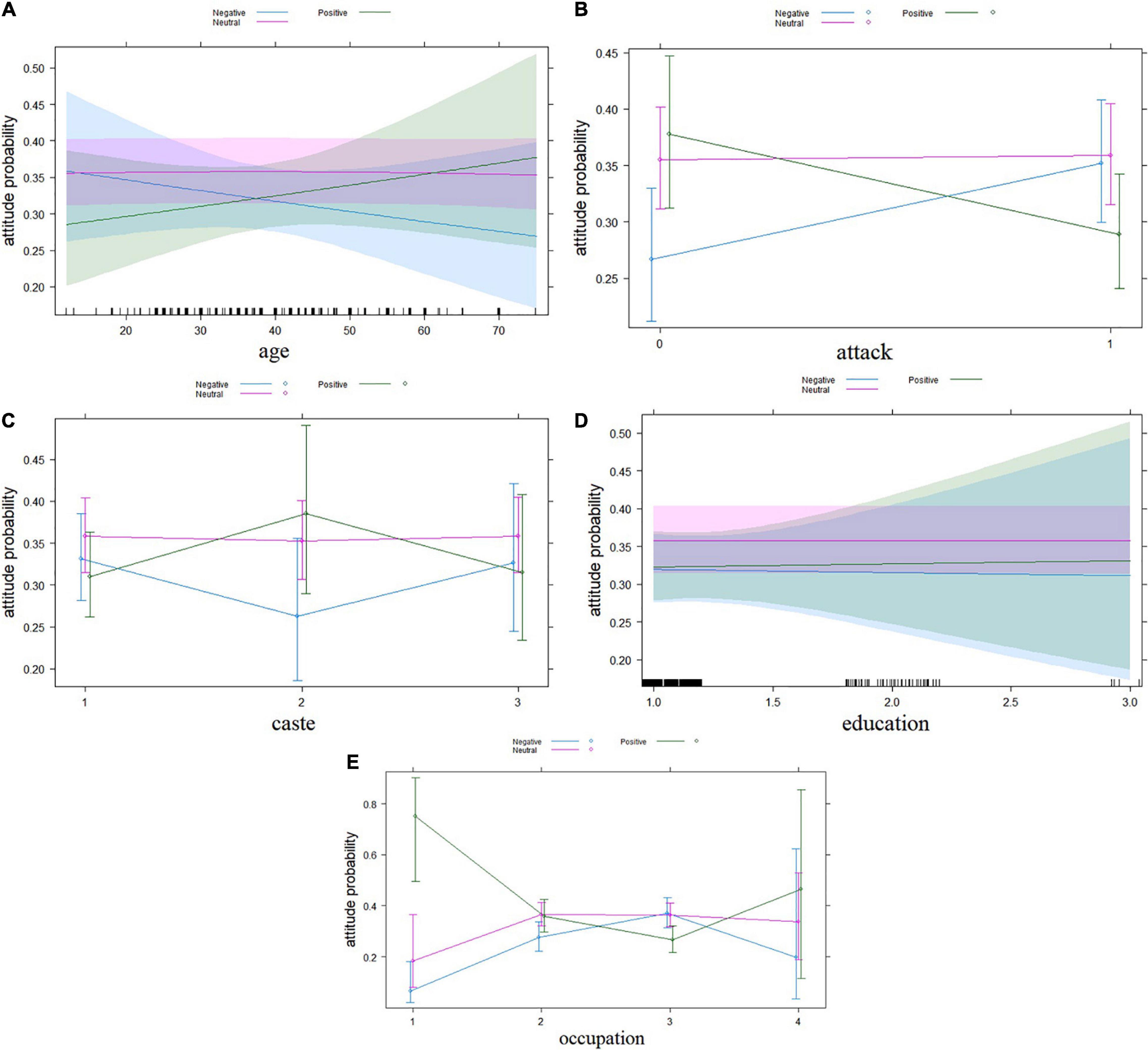
Figure 4. Probability of attitude responses in ordinal models for predicting attitudes toward wolves. (A) Effect of Age. (B) Effect of livestock attacked by wolves (0 = No attack, 1 = attack). (C) Effect of caste (1 = Gurjar, 2 = Meena, 3 = Others). (D) Effect of Education (1 = 5th and below, 2 = 6th-10th standard, 3 = 10th and above. (E) Effect of Occupation (1 = Laborer, 2 = Farmer, 3 = Shepherd, 4 = Others).
Our models suggest that among all the socio-demographic factors, education has the least effect on attitudes of people toward wolves, although education has often been suggested as the preferred management tool for reducing conflict (Johansson et al., 2016). Previous studies have shown that the role of education is the most important factor that influences the perception about carnivores (Mkonyi et al., 2017; Behmanesh et al., 2018; Arbieu et al., 2019). Moreover, only 5% of the respondents were educated up to 10th grade or above, therefore there was not much variation in the data to generate the differences in the attitude of the respondents. Education when combined with other interventions like the use of a compensation scheme and involvement of local people in management as a means of developing trust, can be helpful in reducing negative attitudes toward carnivores (Johansson et al., 2016). Therefore, further studies should focus on the assessment of attitudes post successful implementation of educational programs to better address human–wolf interactions (Baruch-Mordo et al., 2011).
The data collected on livestock depredation through interviews with the locals might have several limitations. Overestimation of loss by livestock owners is a common source of error in interview-based studies (Amit et al., 2013; Boast et al., 2016). Our estimates, therefore, represent a probable upper limit for our reported figures. Nonetheless, these interviews serve as an important source of information to understand the determinants of livestock depredation and the attitudes of villagers, which is crucial for the management of conservation conflict (Redpath et al., 2015).
Compensation Scheme as a Tool for Co-existence
In our study, we found that wolves caused a relatively high annual economic loss (180 USD) per household as compared to other studies where wolves depredated sheep and goats. For instance, in Maharashtra, the economic loss caused by wolves per person annually by depredating on sheep and goats was 60 USD (Agarwala et al., 2010), and in eastern India annual livestock (sheep and goats) loss per household was 125 USD (Palei et al., 2013). In Pakistan, at two different sites, the livestock (sheep and goats) loss per household annually was 95 USD (Ali et al., 2016) and 78 USD (Khan et al., 2020), respectively. In areas such as Wisconsin where wolves depredate on larger livestock such as cattle calf, the annual loss reached high as 602 USD per person (Agarwala et al., 2010).
Many studies in India have recommended adequate compensation schemes to ensure wolf conservation (Kumar and Rahmani, 2000; Jhala, 2003). Currently, in India, compensation schemes are variable across states and are usually prioritized for more charismatic mega fauna, including the tiger, leopard, lion, elephant, and sloth bear (Madhusudhan, 2003; Johnson et al., 2018). The State of Maharashtra has a good compensation policy scheme for ensuring wolf conservation (Agarwala et al., 2010). In the KWLS, the forest department of Rajasthan also started providing compensation for the loss of livestock due to wolves. However, only a few people are aware of the program, as they believe that the compensation scheme is primarily applicable for livestock loss by tigers and leopards. Even though wolves cause high economic loss in the KWLS, in our survey we found that only 6% of the victims filed for compensation against livestock depredation by wolves. To claim compensation, it is necessary to produce photographic documentation of the kill. Since it is difficult to locate the kill made by the wolf, claiming compensation is rather difficult (Behdarvand et al., 2014). Also, the process of receiving compensation is often delayed due to bureaucratic issues (Barua et al., 2013) which usually involve a veterinarian’s report, and cost, time, and effort of the applicant. As a result, the value of the compensation received is often considerably less than the market value of the animal killed (Krithivasan et al., 2009). Such a discrepancy in losses vs. compensation likely instills greater negative attitudes among people of KWLS. Therefore, greater awareness among the people of the KWLS is required through education and outreach about the compensation program for livestock depredation by wolves. There should be an effective and efficient compensation scheme to reduce negative perceptions among villagers. Moreover, the procedure for applying the compensations should be easy to follow with minimal requirement of documentation. In our study area, we recommend that a trained team with veterinarians and forest department personnel should be deployed in each range to report and file the compensation as soon as depredation is reported.
Conclusion
Our study highlights the importance of both ecological and social determinants of livestock depredation by Indian wolves in the semi-arid landscape of western India. Previous studies were mostly based on characterizing losses caused by wolves but did not highlight the factors behind the depredation. This study demonstrates that the combined use of ecological and social factors can help generate a better understanding of the complex human-wolf interactions. Our spatial risk map identifies the areas with a high probability of depredation, which can help the managers to prioritize and mitigate conflict in those areas. It is always better to prevent wolf attacks on livestock, however, given the current scenario, it seems inevitable to avoid much of the interaction between humans and wolves. We suggest that better livestock management can help minimizing the rates of depredation. During the day, shepherds should be more vigilant and graze their livestock closer to villages. We found that only a small proportion of respondents use pens to safeguard their livestock from wolves and most of these pens are poorly constructed, made of dry thorny scrub branches (Supplementary Figure 1) or soft metal wire mesh (Supplementary Figure 2) which are not strong enough to keep wolves away. More emphasis should be placed on using appropriate husbandry methods including better construction of pens, to prevent future depredation events. A compensation program, as suggested above, is the most efficient method to resolve human-wolf interactions and reduce negative attitudes among the villagers toward wolves. More awareness should be created regarding the compensation program through education and outreach. The present study can be replicated in other wolf-occupied regions of India where they cause high economic loss to the marginalized communities through livestock depredation.
Data Availability Statement
The original contributions presented in this study are included in the article/Supplementary Material, further inquiries can be directed to the corresponding author.
Ethics Statement
Verbal consent was gained from all participants before the interviews, but written informed consent and ethical review and approval was not required for this study in accordance with the national legislation and the institutional requirements.
Author Contributions
PM and DK conceived the study. PM designed, performed the data collection, analyzed, and drafted the manuscript. RC conducted the data analysis. AK revised the manuscript. All authors reviewed and approved the final draft.
Funding
This study was financially supported by the Ranthambhore Tiger Conservation Foundation (RTCF), Government of Rajasthan, India [F(05)016/07/03/09/rtcf/580 date15-02-2018].
Conflict of Interest
The authors declare that the research was conducted in the absence of any commercial or financial relationships that could be construed as a potential conflict of interest.
Publisher’s Note
All claims expressed in this article are solely those of the authors and do not necessarily represent those of their affiliated organizations, or those of the publisher, the editors and the reviewers. Any product that may be evaluated in this article, or claim that may be made by its manufacturer, is not guaranteed or endorsed by the publisher.
Acknowledgments
We would like to thank all members of Tiger Watch, Ranthambhore, for their continuous support. We would also like to thank the Rajasthan Forest Department at RTR for permissions and for facilitating this work. We especially thank our Village Wildlife Volunteers, Harimohan Gurjar, Lakhan Gurjar, and Battilal Gurjar for collecting and assisting in data collection for the interview surveys.
Supplementary Material
The Supplementary Material for this article can be found online at: https://www.frontiersin.org/articles/10.3389/fevo.2022.855084/full#supplementary-material
References
Abade, L., Macdonald, D. W., and Dickamn, A. J. (2014). Assessing the relative importance of landscape and husbandry factors in determining the large carnivore depredation risk in Tanzania’s Ruaha landscape. Biol. Conserv. 180, 241–248. doi: 10.1016/j.biocon.2014.10.005
Agarwala, M., and Kumar, S. (2009). Wolves in agricultural landscapes in Western India. Trop Resources Bull. 28, 48–53.
Agarwala, M., Kumar, S., Treves, A., and Naughton-Treves, L. (2010). Paying for wolves in Solapur, India and Wisconsin, USA: comparing compensation rules and practice to understand the goals and politics of wolf conservation. Biol. Conserv. 143, 2945–2955. doi: 10.1016/j.biocon.2010.05.003
Ali, U., Minhas, R. A., Awan, M. S., Ahmed, K. B., Qamar, Q. Z., and Dar, N. I. (2016). Human-grey Wolf (Canis lupus Linnaeus, 1758) conflict in Shounther valley, district Neelum, Azad Jammu and Kashmir, Pakistan. Pak. J. Zool. 48, 861–868.
Amit, R., Gordillo-Chávez, E. J., and Bone, R. (2013). Jaguar and puma attacks on livestock in Costa Rica. Hum. Wildl Interact. 7, 77–84. doi: 10.15517/rbt.v62i4.13199
Arbieu, U., Mehring, M., Bunnefeld, N., Kaczensky, P., Reinhardt, I., Ansorge, H., et al. (2019). Attitudes towards returning wolves (Canis lupus) in Germany: exposure, information sources and trust matter. Biol. Conserv. 234, 202–210. doi: 10.1016/j.biocon.2019.03.027
Aryal, A., Brunton, D., Ji, W., Barraclough, R. K., and Raubenheimer, D. (2014). Human-carnivore conflict: ecological and economical sustainability of predation on livestock by snow leopard and other carnivores in the Himalaya. Sustain. Sci. 9, 321–329. doi: 10.1007/s11625-014-0246-8
Bagchi, S., and Mishra, C. (2006). Living with large carnivores: predation on livestock by the snow leopard (Uncia uncia). J. Zool. 268, 217–224. doi: 10.1111/j.1469-7998.2005.00030.x
Barua, M., Bhagwat, S. A., and Jadhav, S. (2013). The hidden dimensions of human- wildlife conflict: health impacts, opportunity and transaction costs. Biol. Conserv. 157, 309–316. doi: 10.1016/j.biocon.2012.07.014
Baruch-Mordo, S., Breck, S. W., Eilson, K. R., and Broderick, J. (2011). The carrot or the stick? Evaluation of education and enforcement as management tools for human-wildlife conflicts. PLoS One 6:e15681. doi: 10.1371/journal.pone.0015681
Behdarvand, N., Kaboli, M., Ahmadi, M., Nourani, E., Mahini, A. S., and Aghbolaghi, M. A. (2014). Spatial risk model and mitigation implications for wolf–human conflict in a highly modified agroecosystem in Western Iran. Biol. Conserv. 177, 156–164. doi: 10.1016/j.biocon.2014.06.024
Behmanesh, M., Malekian, M., Hemami, M. R., and Fakheran, S. (2018). Patterns and determinants of human-carnivore conflicts in Central Iran: realities and perceptions behind the conflict. Hum. Dimens. Wildl. 24, 14–30. doi: 10.1080/10871209.2018.1531182
Bista, A., Chanchani, P., Subedi, N., and Bajracharya, S. B. (2021). The peri-urban leopards of Kathmandu: assessing determinants of presence and predation on domestic animals. Oryx 56, 91–100. doi: 10.1017/S0030605320000423
Boast, L., Houser, A., Horgan, J., Reeves, H., Phale, P., and Klein, R. (2016). Prey preferences of free-ranging cheetahs on farmland: scat analysis versus farmers’ perceptions. Afr. J. Ecol. 54, 424–433. doi: 10.1111/aje.12296
Burnham, K. P., and Anderson, D. R. (2002). Model Selection and Multi-Model Inference: A Practical Information-Theoretic Approach, 2nd Edn. New York, NY: Springer-Verlag.
Carlson, S. C., Dietsch, A. M., Slagle, K. M., and Bruskotter, J. T. (2020). The VIPs of wolf conservation: how values, identity, and place shape attitudes toward wolves in the United States. Front. Ecol. Evol. 8:6. doi: 10.3389/fevo.2020.00006
Carter, N. H., and Linnell, J. D. C. (2016). Co-adaptation is key to coexisting with large carnivores. Trends Ecol. Evol. 31, 575–578. doi: 10.1016/j.tree.2016.05.006
Carvalho, E. A. R., Zarco-Gonzalez, M. M., Monroy-Vilchis, O., and Morato, R. G. (2015). Modelling the risk of livestock depredation by jaguar along the Transamazon highway, Brazil. Basic Appl. Ecol. 16, 413–419. doi: 10.1016/j.baae.2015.03.005
Champion, H. G., and Seth, S. K. (1968). A Revised Survey of the Forest Types of India. New Delhi: Government of India Press, 404.
Chapron, G., Kaczensky, P., Linnell, J. D. C., von Arx, M., Huber, D., Andrén, H., et al. (2014). Recovery of large carnivores in Europe’s modern human-dominated landscapes. Science 346, 1517–1519. doi: 10.1126/science.1257553
Chavez, A. S., and Gese, E. M. (2006). Landscape use and movements of wolves in relation to livestock in a wildland-agriculture matrix. J. Wildl. Manag. 70, 1079–1086. doi: 10.2193/0022-541X(2006)70[1079:LUAMOW]2.0.CO;2
Dar, N. I., Minhas, R. A., Zaman, Q., and Linkie, M. (2009). Predicting the patterns, perceptions and causes of human-carnivore conflict in and around Machiara National Park, Pakistan. Biol. Conserv. 142, 2076–2082. doi: 10.1016/j.biocon.2009.04.003
Davie, H. S., Murdoch, J. D., Lhagvasuren, A., and Reading, R. P. (2014). Measuring and mapping the influence of landscape factors on livestock predation by wolves in Mongolia. J. Arid Environ. 103, 85–91. doi: 10.1016/j.jaridenv.2014.01.008
Dickman, A. J. (2010). Complexities of conflict: the importance of considering social factors for effectively resolving human-wildlife conflict. Anim. Conserv. 13, 458–466. doi: 10.1111/j.1469-1795.2010.00368.x
Dickman, A. M., Hazzah, L., Carbone, C., and Durant, S. M. (2014). Carnivores, culture and ‘contagious conflict’: multiple factors influence perceived problems with carnivores in Tanzania’s Ruaha landscape. Biol. Conserv. 178, 19–27. doi: 10.1016/j.biocon.2014.07.011
Dormann, C. F., Elith, J., Bacher, S., Buchmann, C., Carl, G., Carré, G., et al. (2013). Collinearity: a review of methods to deal with it and a simulation study evaluating their performance. Ecography 36, 27–46.
Gálvez, N., Freya, G. G., John, A. V. S., Macdonald, D. W., and Davies, Z. G. (2018). A spatially integrated framework for assessing socioecological drivers of carnivore decline. J. Appl. Ecol. 55, 1393–1405. doi: 10.1111/1365-2664.13072
Ghosal, S., Athreya, V. R., Linnell, J. D. C., and Vedeld, P. O. (2013). An ontological crisis? A review of large felid conservation in India. Biodivers. Conserv. 22, 2665–2681. doi: 10.1007/s10531-013-0549-6
Goswami, V. R., Medhi, K., Nichols, J. D., and Oli, M. K. (2015). Mechanistic understanding of human–wildlife conflict through a novel application of dynamic occupancy models. Conserv. Biol. 29, 1100–1110. doi: 10.1111/cobi.12475
Gurung, B., Smith, J. L. D., McDougal, C., Karki, J. B., and Barlow, A. (2008). Factors associated with human-killing tigers in Chitwan National Park, Nepal. Biol. Conserv. 141, 3069–3078. doi: 10.1016/j.biocon.2008.09.013
Hines, J. E. (2006). PRESENCE2 – Software to Estimate Patch Occupancy and Related Parameters. Version 2.4. Reston, VA: USGS-PWRC.
Inskip, C., and Zimmermann, A. (2009). Human-felid conflict: a review of patterns and priorities worldwide. Oryx 43, 18–34. doi: 10.1017/S003060530899030X
Jhala, Y. V. (2003). Status, ecology and conservation of the Indian wolf Canis lupus pallipes sykes. J. Bombay Nat. Hist. Soc. 79, 409–411.
Jhala, Y. V., Qureshi, Q., and Nayak, A. K. (2020). Status of Tigers, co-Predators and Prey In India, 2018. New Delhi: National Tiger Conservation Authority, Government of India.
Johansson, M., Ferreira, I. A., Støen, O. G., Frank, J., and Flykt, A. (2016). Targeting human fear of large carnivores—many ideas but few known effects. Biol. Conserv. 201, 261–269. doi: 10.1016/S0140-6736(16)00619-X
Johnson, M. F., Karanth, K. K., and Weinthal, E. (2018). Compensation as a policy for mitigating human-wildlife conflict around four protected areas in Rajasthan, India. Conserv. Soc. 16, 305–319. doi: 10.4103/cs.cs_17_1
Kaartinen, S., Luoto, M., and Kojola, I. (2009). Carnivore-livestock conflicts: determinants of wolf (Canis lupus) depredation on sheep farms in Finland. Biodivers. Conserv. 18, 3503–3517. doi: 10.1007/s10531-009-9657-8
Kaltenborn, B. P., Bjerke, T., and Vitterso, J. (1999). Attitudes toward large carnivores among sheep farmers, wildlife managers, and research biologists in Norway. Hum. Dimens. Wildl. 4, 57–73. doi: 10.1080/10871209909359157
Kellert, S. R. (1985). Public perceptions of predators, particularly the wolf and Coyote. Biol. Conserv. 31, 167–189. doi: 10.1016/0006-3207(85)90047-3
Khan, M. R., Mahmood, T., Fatima, H., Akrim, F., Andleeb, S., and Hamid, A. (2020). Distribution, diet menu and human conflict of grey wolf Canis lupus in Mahoodand Valley, Swat District, Pakistan. Pak. J. Zool. 52:179. doi: 10.17582/journal.pjz/2020.52.1.179.191
Khorozyan, I., Ghoddousi, A., Soofi, M., and Waltert, M. (2015). Big cats kill more livestock when wild prey reaches a minimum threshold. Biol. Conserv. 192, 268–275. doi: 10.1016/j.biocon.2015.09.031
Kolowski, J. M., and Holekamp, K. E. (2006). Spatial, temporal, and physical characteristics of livestock depredation by large carnivores along a Kenyan reserve border. Biol. Conser. 128, 529–541. doi: 10.1016/j.biocon.2005.10.021
Koziarski, A., Kissui, B., and Kiffner, C. (2016). Patterns and correlates of perceived conflict between humans and large carnivores in Northern Tanzania. Biol. Conserv. 199, 41–50. doi: 10.1016/j.biocon.2016.04.029
Krithivasan, R., Athreya, V., and Odden, M. (2009). Human-Wolf Conflict in Human Dominated Landscapes of Ahmednagar District, Maharashtra and Possible Mitigation Measures. London: Rufford Foundation, 1–53.
Kumar, S., and Rahmani, A. (1997). Status of Indian grey wolf Canis lupus pallipes and its conservation in marginal areas of Solapur district, Maharashtra. J. Bombay Nat. Hist. Soc. 94, 466–472.
Kumar, S., and Rahmani, A. (2000). Livestock depredation by wolves in the great Indian Bustard Sanctuary, Nannaj (Maharastra), India. J. Bombay Nat. Hist. Soc. 97, 340–348.
Linnell, J. D. C., Swenson, J. E., and Andersen, R. (2001). Predators and people: conservation of large carnivores is possible at high human densities if management policy is favourable. Anim. Conserv. 4, 345–349. doi: 10.1017/S1367943001001408
Lischka, S. A., Teel, T. L., Johnson, H. E., Reed, S. E., Breck, S., Carlos, A. D., et al. (2018). A conceptual model for the integration of social and ecological information to understand human-wildlife interactions. Biol. Conser. 225, 80–87.
Liu, F., McShea, W. J., Garshelis, D. L., Zhu, X., Wang, D., and Shao, L. (2011). Human-wildlife conflicts influence attitudes but not necessarily behaviors: factors driving the poaching of bears in China. Biol. Conser. 144, 538–547.
MacKenzie, D. I, Nichols, J. D., Royle, J. A., Pollock, K. H., Bailey, L. L., and Hines, J. E. (2006). Occupancy Estimation and Modeling: Inferring Patterns and Dynamics of Species Occurrence. San Diego, CA: Elsevier Academic Press.
MacKenzie, D. I., Nichols, J. D., Seamans, M. E., and Gutiérrez, R. J. (2009). Modeling species occurrence dynamics with multiple states and imperfect detection. Ecology 90, 823–835.
MacKenzie, D. I., and Royle, J. A. (2005). Designing efficient occupancy studies: general advice and tips on allocation of survey effort. J. Appl. Ecol. 42, 1105–1114. doi: 10.1111/j.1365-2664.2005.01098.x
Madhusudhan, M. D. (2003). Living Amidst large wildlife: livestock and crop depredation by large mammals in the interior villages of Bhadra tiger reserve, South India. Environ. Mangage. 31, 466–475. doi: 10.1007/s00267-002-2790-8
Mahajan, P., and Khandal, D. (2019). Assessing the ecological status of Indian grey wolf with a focus on anthropogenic interactions in Kailadevi Wildlife sanctuary. Tiger Watch: A report submitted to Forest Department. Tiger Watch, Sherpur Khiljipur, Rajasthan, India.
Mahajan, P., and Khandal, D. (2021). Preliminary status of the Indian grey wolf in Kailadevi Wildlife Sanctuary. Canid. Biol. Conserv. 23, 8–14.
Mahajan, P., Khandal, D., and Chandrawal, K. (2021). Factors Influencing habitat-use of Indian grey wolf in the semiarid landscape of western India. Mammal Study. 47, 1–15. doi: 10.3106/ms2021-0029
Majgaonkar, I., Vaidyanathan, S., and Srivathsa, A. (2019). Land-sharing potential of large carnivores in human-modified landscapes of western India. Conserv. Sci. Pract. 1:e34. doi: 10.1111/csp2.34
Majić, A., and Bath, A. J. (2010). Changes in attitudes toward wolves in Croatia. Biol Conserv. 143, 255–260. doi: 10.1016/j.biocon.2009.09.010
Mech, L. D., Harper, E. K., Meier, T. J., and Paul, W. J. (2000). Assessing factors that may predispose Minnesota farms to wolf depredation on cattle. Wildl. Soc. Bul. 28, 623–629.
Meriggi, A., and Lovari, S. (1996). A review of wolf predation in southern Europe: does the wolf prefer wild prey to livestock? J. Appl. Ecol. 33, 1561–1571.
Miller, J. R. B., Jhala, Y. V., Jena, J., and Schmitz, O. J. (2015). Landscape-scale accessibility of livestock to tigers: implications of spatial grain for modeling predation risk to mitigate human-carnivore conflict. Ecol. Evol. 5, 1354–1367. doi: 10.1002/ece3.1440
Mkonyi, F. J., Estes, A. B., Msuha, M. J., Lichtenfeld, L. L., and Durant, S. M. (2017). Local attitudes and perceptions toward large carnivores in a human-dominated landscape of northern Tanzania. Hum. Dimens. Wildl. 22, 314–330. doi: 10.1080/10871209.2017.1323356
Nyhus, P. J., and Tilson, R. (2004). Characterizing human-tiger conflict in Sumatra, Indonesia: implications for conservation. Oryx 38, 68–74.
Ogra, M. (2009). Attitudes toward resolution of human-wildlife conflict among forest-dependent agriculturalists near Rajaji National Park, India. Hum. Ecol. 37, 161–177.
Palei, H. S., Debata, S., Mohapatra, P. P., and Sahu, H. K. (2013). Livestock predation by endangered Indian wolf (Canis lupus) in hadagarh wildlife sanctuary, Eastern India. Indian For. 139, 895–898.
Puri, M., Srivathsa, A., Karanth, K. K., Patel, I., and Kumar, N. S. (2020). The balancing act: maintaining leopard-wild prey equilibrium could offer economic benefits to people in a shared forest landscape of central India. Ecol. Indic. 110:105931. doi: 10.1016/j.ecolind.2019
QGIS Development Team (2018). QGIS Geographic Information System. Open Source Geospatial Foundation Project. Austin, TX: QGIS Development Team.
R Development Core Team (2021). R: A Language and Environment for Statistical Computing. Version 3.5.0. R Foundation for Statistical Computing: Vienna.
Redpath, S. M., Gutiérrez, R. J., Wood, K. A., Sidaway, R., and Young, J. C. (2015). “An introduction to conservation conflicts,” in Conflicts in Conservation. Navigating Towards Solutions, eds S. M. Redpath, R. J. Gutierrez, K. Wood, and J. Young (Cambridge: Cambridge University Press).
Rehman, E. U., Din, J. U., Ahmad, S., Hameed, S., Shah, K. A., Mehmood, T., et al. (2021). Insight into occupancy determinants and conflict dynamics of grey wolf (Canis lupus) in the dry temperate zone of Hindukush Range. Glob. Ecol. Conserv. 25:e01402. doi: 10.1016/j.gecco.2020.e01402
Rich, L. N., Russell, R. E., Glenn, E. M., Mitchell, M. S., Gude, J. A., Po-druzny, K. M., et al. (2013). Estimating occupancy and predicting numbers of grey wolf packs in Montana using hunter surveys. J. Wildl. Manag. 77, 1280–1289. doi: 10.1002/jwmg.562
Røskaft, E., Händel, B., Bjerke, T., and Kaltenborn, B. P. (2007). Human attitudes towards large carnivores in Norway. Wildlife Biol. 13, 172–185. doi: 10.2981/0909-6396(2007)13[172:HATLCI]2.0.CO;2
Shahi, S. P. (1982). Status of grey wolf (Canis lupus pallipes) in India: a preliminary survey. J. Bombay Nat. Hist. Soc. 79, 493–502.
Sharma, R. K., Bhatnagar, Y. V., and Mishra, C. (2015). Does livestock benefit or harm snow leopards? Biol. Conserv. 190, 8–13. doi: 10.1016/j.biocon.2015.04.026
Singh, M., and Kumara, H. N. (2006). Distribution, status and conservation of Indian grey wolf (Canis lupus pallipes) in Karnataka. India. J Zool. 270, 164–169. doi: 10.1111/j.1469-7998.2006.00103.x
Srivathsa, A., Puri, M., Karanth, K. K., Patel, I., and Samba Kumar, N. (2019). Examining human - Carnivore interactions using a socio-ecological framework: sympatric wild canids in India as a case study. R. Soc. Open Sci. 6:182008. doi: 10.1098/rsos.182008
Suryawanshi, K. R., Bhatnagar, Y. V., Redpath, S., and Mishra, C. (2013). People, predators and perceptions: patterns of livestock depredation by snow leopards and wolves. J. Appl. Ecol. 50, 550–560. doi: 10.1111/1365-2664.12061
Suryawanshi, K. R., Redpath, S. M., Bhatnagar, Y. V., Ramakrishnan, U., Chaturvedi, V., Smout, S. C., et al. (2017). Impact of wild prey availability on livestock predation by snow leopards. R. Soc. Open Sci. 4:170026. doi: 10.1098/rsos.170026
Tilman, D., May, R. M., Lehman, C. L., and Nowak, M. A. (1994). Habitat destruction and the extinction debt. Nature 371, 65–66. doi: 10.1038/371065a0
Treves, A., and Karanth, K. U. (2003). Human-carnivore conflict and perspectives on carnivore management worldwide. Conserv. Biol. 17, 1491–1499. doi: 10.1111/j.1523-1739.2003.00059.x
Treves, A., Treves, L. N., Harper, E. K., Mladenoff, D. J., Rose, R. A., Sickley, T. A., et al. (2004). Predicting human-carnivore conflict: a spatial model derived from 25 years of data on wolf predation on livestock. Conserv. Biol. 18, 114–125. doi: 10.1111/j.1523-1739.2004.00189.x
UNEP-WCMC (2021). Protected Area Profile for India From the World Database of Protected Areas. Cambridge: UNEP-WCMC.
Wang, S. W., and Macdonald, D. W. (2006). Livestock predation by carnivores in Jigme Singye Wangchuck National Park. Bhutan. Biol. Conserv. 129, 558–565. doi: 10.1016/j.biocon.2005.11.024
Williams, C. K., Ericsson, G., and Heberlein, T. A. (2002). A quantitative summary of attitudes toward wolves and their reintroduction (1972-2000). Wildl. Soc. Bull. 30, 575–584.
Woodroffe, R., Lindsey, P., Romanach, S., Stein, A., and ole Ranah, S. M. (2005). Livestock predation by endangered African wild dogs (Lycaon pictus) in northern Kenya. Biol. Conserv. 124, 225–234. doi: 10.1016/j.biocon.2005.01.028
Keywords: coexistence, human-wolf interactions, Indian grey wolf, interview surveys, multi-state occupancy modeling, spatial predation risk
Citation: Mahajan P, Chaudhary R, Kazi A and Khandal D (2022) Spatial Determinants of Livestock Depredation and Human Attitude Toward Wolves in Kailadevi Wildlife Sanctuary, Rajasthan, India. Front. Ecol. Evol. 10:855084. doi: 10.3389/fevo.2022.855084
Received: 14 January 2022; Accepted: 20 June 2022;
Published: 20 July 2022.
Edited by:
Camilla Wikenros, Swedish University of Agricultural Sciences, SwedenReviewed by:
Richard Patrick Reading, Butterfly Pavilion, United StatesMuhammad Ali Nawaz, Quaid-i-Azam University, Pakistan
Copyright © 2022 Mahajan, Chaudhary, Kazi and Khandal. This is an open-access article distributed under the terms of the Creative Commons Attribution License (CC BY). The use, distribution or reproduction in other forums is permitted, provided the original author(s) and the copyright owner(s) are credited and that the original publication in this journal is cited, in accordance with accepted academic practice. No use, distribution or reproduction is permitted which does not comply with these terms.
*Correspondence: Prashant Mahajan, Prashant_mahajan@rocketmail.com
†Present address: Prashant Mahajan, Department of Wildlife Sciences, Aligarh Muslim University, Aligarh, India