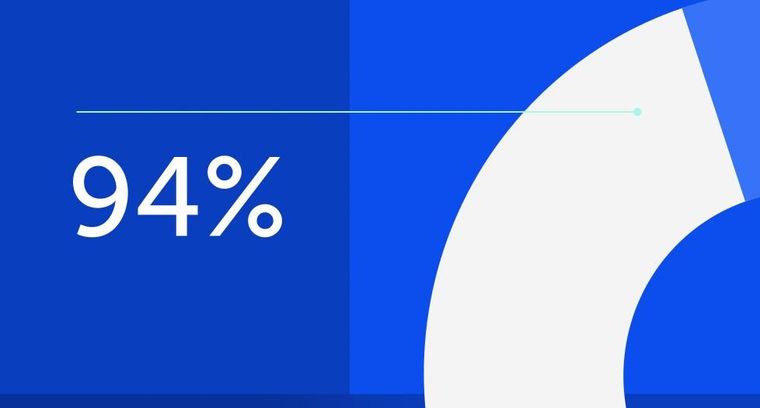
94% of researchers rate our articles as excellent or good
Learn more about the work of our research integrity team to safeguard the quality of each article we publish.
Find out more
ORIGINAL RESEARCH article
Front. Ecol. Evol., 29 March 2022
Sec. Phylogenetics, Phylogenomics, and Systematics
Volume 10 - 2022 | https://doi.org/10.3389/fevo.2022.854509
Systematics and taxonomy are the backbone of all components of biology and ecology, yet cryptic species present a major challenge for accurate species identification. This is especially problematic as they represent a substantial portion of undiscovered biodiversity, and have implications for not only species conservation, but even assaying potential risk of zoonotic spillover. Here, we use integrative approaches to delineate potential cryptic species in horseshoe bats (Rhinolophidae), evaluate the phenotypic disparities between cryptic species, and identify key traits for their identification. We tested the use of multispecies coalescent models (MSC) using Bayesian Phylogenetic and Phylogeography (BPP) and found that BPP was useful in delineating potential cryptic species, and consistent with acoustic traits. Our results show that around 40% of Asian rhinolophid species are potentially cryptic and have not been formally described. In order to avoid potential misidentification and allow species to be accurately identified, we identified quantitative noseleaf sella and acoustic characters as the most informative traits in delineating between potential cryptic species in Rhinolophidae. This highlights the physical differences between cryptic species that are apparent in noseleaf traits which often only qualitatively described but rarely measured. Each part of the noseleaf including the sella, lateral lappets, and lancet furrows, play roles in focusing acoustic beams and thus, provide useful characteristics to identify cryptic Rhinolophus species. Finally, species delimitation for cryptic species cannot rely on genetic data alone, but such data should be complemented by other evidence, including phenotypic, acoustic data, and geographic distributions to ensure accurate species identification and delineation.
Systematics and taxonomy are crucial foundations to all biological fields, as species are the fundamental unit for almost any analysis or management (Agnarsson and Kuntner, 2007; De Queiroz, 2007; Eberle et al., 2019). Numerous approaches for accurate species identification and delimitation have been developed in recent years, including various molecular approaches (Hebert et al., 2003; Hebert and Gregory, 2005; Ratnasingham and Hebert, 2007; Kekkonen and Hebert, 2014), integrative species delimitation by combining taxonomic, genetic, and phenotypic datasets (DeSalle et al., 2005; Will et al., 2005; Wortley and Scotland, 2006; Padial et al., 2010), and statistical model based-approach with multi-species coalescent models (Yang and Rannala, 2010). One of the challenges in current taxonomy is delimiting cryptic species, which, if left unidentified, may cause inaccurate estimates of species richness (over- or underestimating, or misidentifications) [definitions of what constitutes a cryptic species have been broadly discussed in previous studies (Bickford et al., 2007; Struck et al., 2018)]. With the increasing use of molecular approaches in recent decades, increasing numbers of cryptic species are being distinguished (i.e., Kingston et al., 2001; Jones and Barlow, 2004; Stuart et al., 2006; Sedlock and Weyandt, 2009; Salicini et al., 2011; Bogdanowicz et al., 2015; Tu et al., 2017). However, many such studies make little effort to measure phenotypic disparity in a species and thus empirically differentiate inter- and intra-specific variation. Genetic divergence, discrete and continuous morphological comparison and other forms of evolutionary traits of inter- and intra-species should be employed to assess whether candidate cryptic species can be statistically distinguished. Previous studies have evaluated morphological similarity in cryptic species subjectively, but rarely measured the degree of similarity of more cryptic traits which may be associated with evolutionary adaptations (Struck et al., 2018). This may lead to over-splitting in more variable groups and neglecting species in more cryptic ones, or ones for which trait selection has not been optimized.
A combination of phenotypic and genotypic datasets have been shown to yield higher resolution phylogenies (Wortley and Scotland, 2006), and better reflects the evolutionary processes which may relate to speciation to provide a more robust and standardized analysis in order to detect hidden species (Padial et al., 2010; Carstens et al., 2013; Struck et al., 2018). Cryptic species are common in bats, but frequently go undetected unless genetic and acoustic data are used to diagnose species identity (Taylor et al., 2018). Therefore, in this study we use bats as models to identify potential hidden species within one family, Rhinolophidae (horseshoe bats), and address above issues to detect potential cryptic species.
The horseshoe bats (Rhinolophidae), are of exceptional scientific interest, with 106 described species (18 new species described since 2000) encompassed in a single genus Rhinolophus (Simmons and Cirranello, 2021). In addition, rhinolophids are considered to be the natural reservoir hosts for various zoonotic viruses, including severe acute respiratory syndrome (SARS) coronavirus (Latinne et al., 2020; Zhou et al., 2020, 2021). External morphological evidence, even with skull and dental characters, may fail to distinguish potential cryptic species (Taylor et al., 2018). One important characteristics of Rhinolophidae species is the prominent shape of the noseleaf (Bogdanowicz, 1992; Francis, 2019). Noseleaf features in rhinolophids are involved in shaping the directionality of calls emission beam and served quantitatively different functions for different species (Zhang et al., 2009; Vanderelst et al., 2010, 2011, 2013). However, due to high structural similarity, many non-trained taxonomists can find it difficult to distinguish between species, which may lead to errors in species identification. In this study, we propose a framework to quantitatively measure the noseleaf traits from all aspects (i.e., posterior and anterior, lancet, and sella), and identify the most informative traits for species identification.
All species in Rhinolophidae emit constant frequency calls (CF) ranging from >25 to 103 kHz with relatively long duration commencing and ending with a brief frequency-modulated (FM) sweep (Grinnell, 2009). The call structure in Rhinolophidae is relatively simple, but they possess a sophisticated function and auditory specialization (Neuweiler, 1990). Rhinolophidae species emit high-duty cycles call through nasal passages, and the returning echoes are received with an acoustic fovea which provides high sensitivity in detecting small variations in call frequency (Neuweiler, 1990). Therefore, call frequency plays an essential part in mate recognition which could lead to reproductive isolation (Kingston et al., 2001). High variation in call frequency and genetic differences within species is also known in a number of studies (i.e., Sedlock and Weyandt, 2009; Francis et al., 2010; Mao et al., 2010; Taylor et al., 2018; Liu et al., 2019). Yet, few former studies on cryptic species used rigorous criteria to investigate the phenotypic disparities between known acoustically divergent taxa within the rhinolophids. The lack of such studies hinders our understanding of their taxonomy, ecology, and evolutionary significance (Struck et al., 2018). Accurate taxonomy lays a foundation for quantifying biodiversity and provides a basis for all other biological or ecological assessments. Misidentification of a potential species could lead to incorrect interpretation of other biological research, including diversity, species interactions and biogeography, and even potential zoonotic spillovers, as understanding community structure and species interactions requires knowledge of what species are present (Agnarsson and Kuntner, 2007). Therefore, correct species recognition and the adoption of interdisciplinary approaches are fundamental and will provide a sounder basis for policy making in management and conservation.
The utility of molecular data to uncover cryptic lineages has been demonstrated in numerous studies (Salicini et al., 2011; Patrick et al., 2013; Kekkonen and Hebert, 2014). Along with increasing use of molecular techniques, the statistical framework of species delimitation incorporating coalescent theory provides statistical support to identify cryptic and/or allopatric species (Fujita et al., 2012). However, growing use of multi-loci datasets means utilizing older data, or maximizing species coverage is increasingly challenging. The caveats of a single-locus approach have been discussed broadly (Knowles and Carstens, 2007), including potential discordance of species tree and gene trees due to various biological processes such as incomplete lineage sorting and interspecific hybridization (Funk and Omland, 2003; Mallo and Posada, 2016), however, single-locus data can provide useful information of species evolutionary history (Avise, 2009). Integrating a statistical framework in coalescent theory resolved the major caveats to a single locus approach (i.e., incomplete lineage sorting and interspecific hybridization) into the analysis and has been proven to produce reliable results, even with a single locus (Esselstyn et al., 2012; Fujisawa and Barraclough, 2013; Dowton et al., 2014; Kapli et al., 2017; Yang and Rannala, 2017). In this study we investigate how can we accurately delineate cryptic Rhinolophidae species by incorporating phenotypic and genotypic information, including morphological, acoustic and genetic data. Thus, the main objectives are: (1) to use multispecies coalescent model approaches to delimit potential cryptic species, (2) to investigate morphological and acoustic disparities between potential cryptic species, and (3) to identify informative traits to diagnose cryptic species morphologically and acoustically.
Bats were caught using harp traps and mist nets across caves and forest paths in South Chinese Karst, and other parts of Southeast Asia (Supplementary Table 1); each bat individual trapped was placed in the bat bags for measurements, photographs, and tissue samples. External characters were measured using Mitutoyo Absolute Series-500 (Mitutoyo Corporation, Japan) calliper with an accuracy of 0.01 mm, and body mass was measured with Pesola Spring Scale (Pesola® Präzisionswaagen AG, Switzerland) with a precision of 0.3%. Each bat individual was photographed using a FUJIFILM X100F camera (Fujifilm Corporation, Japan).1 Bats were positioned in front of the camera for noseleaf photographs, and the photographs were taken horizontally from the bats. Front, lateral, and sella surfaces were photographed for each individual with the scale for the noseleaf width and length. The noseleaf width and length used in the photographic scale were measured with a digital calliper for each individual when the photograph was taken, then used to calibrate measures on the photograph. The wing was photographed by holding the bat with its wing extended over the scale-board at a consistent angle (flat, with the tip clearly visible), photos were taken vertically from directly above, so the scale was visible. Detailed measurements are provided in Supplementary Figure 1.
Tissue samples were obtained from wing membranes of bats collected using 3 mm biopsy punch. No specimens were collected during this study and bats were released after tissue samples were collected and measurements, photographs, and echolocation calls were recorded. Tissue samples were stored in vials containing 99.7% alcohol. The Mammalian Genomic DNA Miniprep kit Sigma-Aldrich (Sigma-Aldrich Corporation, Hongkong SAR) was used for DNA extraction. Samples were amplified using the 12.5 ml PCR reaction mixes, consisted of 6.25 ml of 10% trehalose, 2 ml of ultrapure water, 1.25 ml of 10X PCR buffer, 0.625 ml of MgCl2 (50 mM), 0.125 ml of both primers, 0.0625 ml of dNTP mix (10 mM), 0.3125 U of Taq polymerase, and 2.0 ml of DNA. We amplified 658 bp fragment of mtDNA COI gene using a universal primer adapted from the “Bats of Southeast Asia in BOLD (Barcode of Life Data) Systems” project as follows:
fwd_seq: TGTAAAACGACGGCCAGTTCTCAACCAAC CACAAAGACATTGG,
rev_seq: CAGGAAACAGCTATGACTAGACTTCTGGG TGGCCAAAGAATCA.
The PCR thermal conditions were: initial denaturation 95°C for 30 s, 35 cycles of denaturation (95°C) for 5 s, annealing at 60°C for 34 s and final extension at 72°C for 10 m. PCR products were then purified and sequenced using Sanger ABI 3730 DNA analyzers in South China DNA Barcoding Center, Kunming Institute of Zoology, Chinese Academy of Sciences.
Additional sequences from GenBank were selected carefully, and only sequences with a length longer than 650 bp were selected and included in the analysis, COI has been extensively sampled for bats across Southeast Asia as part of the BOLD initiative and therefore sequenced in bats captured in this analysis. Accession numbers, geographic coordinates, and references are provided in Supplementary Table 2. We selected sequences which were also recorded in China (all available high quality data was used from elsewhere in Asia). For most samples GPS coordinate information was included, but also some sequences from China that listed localities were included (Supplementary Figure 2).
A total 26 of Rhinolophus species sensu lato in the Asian lineage were included for genetic analysis, including R. JLE [undescribed species (Soisook et al., 2015); the acronym from Francis et al. (2010) denoting Judith L. Eger] (n = 3, n = total number of sequences), R. sedulus (n = 1), R. luctus (n = 10), R. trifoliatus (n = 10), R. chiewkweeae (n = 3), R. pearsonii (n = 69), R. coelophylus (n = 5), R. shameli (n = 18), R. creaghi (n = 2), R. stheno (n = 53), R. affinis/R. andamanensis (n = 141), R. rouxi (n = 1), R. indorouxi (n = 1), R. sinicus (n = 132), R. acuminatus (n = 22), R. malayanus (n = 50), R. chaseni (n = 20), R. robinsoni (n = 1), R. philippinensis (n = 7), R. borneensis (n = 11), R. lepidus (n = 5), R. pusillus (n = 105), R. macrotis (n = 86), R. marshalli (n = 19), and R. rex (n = 81). A total of 10 species sensu lato were used for multispecies coalescent models, including R. luctus, R. pearsonii, R. shameli, R. stheno, R. affinis, R. sinicus, R. malayanus, R. pusillus, R. macrotis, and R. rex. We did not include R. marshalli in the species delimitation model due to the small sample sizes of individuals with morphological and acoustic measures recorded. Phenotypic disparity analysis between potential cryptic species was conducted when a sufficient sample size of morphological and acoustic data was available, including R. macrotis (n = 29, n = number of individuals with morphology and acoustic data), R. affinis (n = 16), R. sinicus (n = 51), R. pusillus (n = 34). Although based on genetic data R. luctus (n = 3, n = number of individuals with morphology and acoustic data), R. pearsonii (n = 5), R. shameli (n = 0), R. stheno (n = 13), R. malayanus (n = 16), and R. rex (n = 12) contains multiple potential cryptic species, these species do not have enough samples with complete morphological and acoustic data to use as comparisons between potential cryptic species within species sensu lato, thus cannot be used to assess phenotypic disparities.
We obtained tissue samples from 205 individuals comprising 13 putative species (11 species of Rhinolophidae and two species of Hipposideridae as the outgroup) collected across South China karst and nearby regions (GenBank accession numbers: OK483366; OK483495–OK483509; OK562850–OK563036; OK563109; and OK563727). The details of accession number and isolate numbers information are provided in Supplementary Table 1), the sequences will be made publicly available upon publication). The details of sampling procedures and laboratory work are provided in Supplementary Material). In order to obtain a rigorous comparison for Rhinolophidae species in the region, we added 655 sequences from NCBI GenBank2 and The Barcode of Life Data Systems (BOLD)3 bringing to 860 (681 bp) total sequences in the analysis (Supplementary Table 2, distribution maps of samples are provided in Supplementary Figure 2). Multiple sequences alignments were performed in MAFFT (Multiple Alignment for Amino Acid or Nucleotide Sequences) Version 7.467 (Katoh et al., 2002) using pairwise alignment and iterative refinements method or G-INS-i strategies.
A Maximum-Likelihood (ML) phylogeny was constructed using IQ-TREE 24 (Nguyen et al., 2015). Best-fit substitution model determined using ModelFinderPlus -MFP (Kalyaanamoorthy et al., 2017) with -cmax option and a thorough full tree search per model using –mtree option. The best-fit substitution model TIM2 + F + I + G4 was chosen based on the lowest Bayesian Inference Criterion (BIC) (BICw = 0.907, AICw = 0.696). We use –B 1000 ultrafast bootstrap (UFBoot) replicates (Minh et al., 2013; Hoang et al., 2018) and SH-aLRT (approximate likelihood ratio test) using –alrt 1000 to assess branch support. A hill-climbing Nearest Neighbor Interchange (NNI) search -bnni option was set to minimize the risk of overestimating branch support.
We constructed Bayesian Inference trees in MrBayes 3.2.7a (Ronquist and Huelsenbeck, 2003) with GTR (General Time Reverse) DNA substitution model. The posterior probability of trees was estimated using Metropolis-coupled Markov Chain Monte Carlo (MCMC). We performed MCMC analysis with five chains (four heated and one cold chains) with temperature 0.5. The chains were run for 50,000,000 generations with two independent analysis runs simultaneously and trees were sampled every 1,000 generations. The first 10% of initial trees (500,000 trees) were discarded. The sampled parameter values were summarized using sump. The two runs converged at 50,000,000 million generations and showed no trend in the likelihood values plot. The 95% Highest Posterior Density (HPD) interval for all parameters showed the convergence diagnostic of estimated samples sizes (ESS) values are all above 500 which indicates that all chains mixed well. The Potential Scale Reduction Factor (PSRF) value is 1 for all parameters, indicating a good sample from the posterior probability. The statistical summary of trees was produced using sumt, and the final trees were inspected in FigTree v1.4.4 (Rambaut, 2016). The tree topologies and branch support of ML and BI trees were inspected, strongly support clades were collapsed together and each clade within complex species was encoded with abbreviated species code and numbers. The genetic divergence between and within species was analyzed using MEGA X5 based on Kimura 2-parameter model (Kumar et al., 2018).
We used Bayesian statistics to identify potential cryptic species with a multispecies coalescent model (MSC) in Bayesian phylogenetic and phylogeography program, BPP v 4.3.8 (Yang, 2015). The analysis was conducted using a single locus of mtDNA COI genes as it has the best coverage of all studied species groups in the region. We implemented A10 analysis (species delimitation using guided tree) in BPP using species trees generated in BEAST. The analysis was subset into smaller species groups, and focused on species with supporting morphological and acoustic data from a primary dataset collected in South China karst and Southeast Asia (Figure 1A: clade in dark shades, 10 species complex). Analysis was conducted subsequently per “putative” species sensu lato including R. luctus, R. pearsonii, R. stheno, R. affinis, R. sinicus, R. malayanus, R. macrotis, R. rex, and R. pusillus (Figure 2). We explored a range of parameters to test the sensitivity of population size parameter (θs) priors and the divergence time at the root at species tree (τ0) prior toward the species delimitation models [prior A = θ (3 0.004), τ (3 0.03); prior B = θ (3 0.002), τ (3 0.03); prior C = θ (3 0.04), τ(3 0.003); prior D = θ (3 0.02), τ (3 0.003), prior E = θ (3 0.004), τ (3 0.003); prior F = θ (3 0.04), τ (3 0.03); prior G = θ (3 0.0004), τ (3 0.0003)]. The priors were set to inverse-gamma distribution IG (α, β) and mean m = β/(α − 1). All priors were set to diffuse priors due to little information available for θs and τ0, and we explore the possible influence when the time divergence older [τ = IG (3 0.03), with mean 0.03/(3 − 1) = 0.015 or 1.5% of mean sequence divergence between the root of the species tree and the present time. Following the assumption of mutation rates 10–9 mutation/sites/year (Flouri et al., 2020) then it transcribed time divergence estimate at 15 Ma] and younger [τ = IG (3 0.0003), with the mean at 0.0003/(3 − 1) = 0.00015, translated the time of divergence to 0.15 Ma. Species model priors were assigned as 1 for equal probabilities of rooted trees and two independent runs were applied to algorithm0 and algorithm1 (Yang and Rannala, 2010) (total four independent runs for each priors setting)]. The substitution model was set to GTR, and each independent run was run for 25,000 burn-in rjMCMC steps and 100,000 MCMC samples, sampling at every five steps. The chain convergences were assessed by comparing the consistency of posterior probability produced for each run and among two algorithms. Each species group was separately analyzed to minimize the computational burden for each prior and algorithm. The posterior probability for species delimitation model, pp. > 0.95 are considered to be split well and supported, 0.90 ≤ pp. < 0.95 were considered moderately supported, and when pp. < 0.9 were considered weakly supported.
Figure 1. (A) Phylogenetic gene tree constructed based on COI gene inferred using maximum likelihood and Bayesian inferences, the value in the branch presenting UFBootstrap/sh-alrt (above) for Maximum Likelihood and posterior probability for Bayesian Inference tree (below). The branches support only shows for main branches between sister lineages. Dark shades in the collapsed branches indicated individuals with both morphological measurements and acoustic data; white shades in collapsed branches indicated samples collected from GenBank without morphological and acoustic data; (B) morphological cluster using Ward’s method in involving acoustic and morphological (external, wing, and noseleaf) parameters. Abbreviation in species name rex, R. rex; luc, R. luctus; pea, R. pearsonii; aff, R. affinis; sth, R. stheno; sin, R. sinicus; mar, R. marshalli; mac, R. macrotis; mal, R. malayanus; pus, R. pusillus.
Figure 2. Summary of species delimitation model based on BPP per each potential cryptic species, the tree was based on BEAST as guided tree, each node represents the posterior probability of ancestor nodal support (τ = 0, absence/lumped; τ = 1, presence/split). (A) R. pearsonii represents 12 well supported candidate cryptic species; (B) R. affinis represents five supported candidate cryptic species (A1 = R. andamensis); (C) R. sinicus represents six supported candidate cryptic species; (D) R. pusillus represents six supported candidate cryptic species (P1 = R. cf lepidus); (E) R. luctus (L1 and L2); (F) R. stheno (ST1 and ST2), R. shameli (SH); (G) R. malayanus; (H) R. macrotis; (I) R. rex. (*J = R. JLE, S = R. sedulus, T = R. trifoliatus, CR = R. creaghi, CO = R. coelophylus).
In total, 190 Rhinolophus individuals were sampled from South China and Vietnam. Each individual was measured morphologically, acoustically recorded, had tissue samples collected, and photographs were taken before release. Field identification was based on the Mammals of South East Asia (Francis, 2019). Each individual captured was measured, photographed, calls were recorded on release and a tissue sample was collected from wing membrane and sequenced for genetic analysis (please see supplemental methods). A total of 56 characters were measured from the same genetically sequenced individuals, including seven external characters, 31 noseleaf characters, 11 wing characters, and six acoustic parameters (see details of method in Supplementary Figure 1). The measurements for external characters were based on standard measurement following Francis (2019) using digital callipers Mitutoyo Absolute Series-500 (accuracy 0.01 mm). Noseleaf and wing characters were measured from standardized photographs (see above) using ImageJ version1.52o (National Insitutes of Health, Bethesda, Maryland, United States) software. Each noseleaf photograph was scaled using known distance (in mm) prior measurement, which was generated from noseleaf width and length for noseleaf characters and scale bar for wing characters. Only adults and non-pregnant adult females were included in the data analysis. Maturity was determined by examining each metacarpal/phalangeal joint, interphalangeal joints that had a fused epiphyses which could be seen when bat wing is trans illuminated were adults. Sub-adult bats have visible joints because cartilaginous epiphyseal (growth) areas are lighter than fused epiphyses. Sex was determined by examine the genitalia, and female’s reproduction status was determined by palpating abdomen (for pregnancy) and nipples for signs of lactation based on Simmons and Voss (2009). We obtained echolocation calls from hand-released bats recorded using a Pettersson M500-384 (Pettersson Electronic AB, Uppsala, Sweden),6 acoustic calls were then analyzed using BatSound ver4 (Pettersson electronic AB, Uppsala, Sweden) at a sampling rate of 44.1 kHz, and spectrograms were set at 1,024 sampling site in Fast Fourier Transform (FFT) in conjunction with a Hanning window. We measured 5–10 search-pulses in each call sequence and the following parameters for call measurement were taken from the highest quality calls in a sequence: FMAX, FMIN, FMAXE, bandwidth, duration, and inter-pulse interval (Vaughan et al., 1997).
The variation of phenotypic traits was assessed in order to observe whether genetic data is consistent with morphology and acoustic traits between potentially cryptic species and assessed from the same individuals. In total 10 focal species were included in the analysis, species identifications were based on Francis (2019) and Simmons and Cirranello (2021). R. luctus, R. pearsonii, R. stheno, R. affinis, R. sinicus, R. malayanus, R. pusillus, R. macrotis, R. marshalli, and R. rex. We ran sexual dimorphism analysis by comparing the mean parameter values of each sex within potential cryptic species using a Welch t-test in R and no characters showed sexual dimorphism. Shapiro-Wilk test and F-test were performed prior to the analysis and visual inspection using qqplot to ensure data were normally distributed and the heterodascity of variance between the groups. Multivariate statistical analysis was used to visualize the variation within variables, and data were scaled prior analysis to standardize the variable with different units. Principal Component Analysis (PCA) was used to visualize the trends between traits across species using R package “factoextra version 1.0.7” (Kassambara, 2016) and “FactoMineR version 2.4” (Lê et al., 2008). The PCA biplot was later visualized using R package “ggplot2 version 3.3.3” (Wickham, 2016) and “ggpubr 0.4.0” (Kassambara, 2020), the coloration in the plot used palette in R package “RColorBrewer version 1.1-2” (Neuwirth and Maindonald, 2014) and “ggsci version 2.9” (Xiao and Li, 2018). A simple linear regression using the lm function in R was conducted to statistically examine the interaction between traits between quadrants in a PCA.
We ran separate PCAs for each character set (external, noseleaf, sella, wing and acoustic). We then selected the parameters that contributed most to PC1 and PC2 for each PCA and plotted the characters among the potential cryptic species using boxplot to visualize traits that differed between potential cryptic species. The characters contribution to PCs (PC1 and PC2) was calculated using fviz_contrib() function in R package “factoextra version 1.0.7” (Kassambara, 2016). A Welch t-test was performed to further test the significance of traits using the parameters which contributed most in PCA between each pair of potential cryptic species. The potential cryptic species were assigned based on the combination of genetic analysis (phylogenetic, genetic distance, and BPP), thus, the identifier for each candidate was assigned as: (1) species complex name, followed by (2) the number from the clade with ancestral nodes supported by BPP to indicate different groups. R. affinis with two potential cryptic species (aff1: R. affinis clade11; aff34: R. affinis in clade3 and clade4 was lumped due to low posterior probability in ancestral nodes in BPP analysis), R. macrotis (mac3A vs mac3B; both groups are from the same R. macrotis clade3), and R. pusillus (pusS vs pusM/pusillus with S = sequences, morphological and acoustic data available, M = morphological data only).
We performed ANOVA to compare the characters within potential cryptic species in R. sinicus (denoted as sin2: R. sinicus clade2, sin34: R. sinicus clade3 and clade4 lumped due to low posterior probability in ancestral nodes in BPP analysis, sin89: R. sinicus clade8 and 9 were also lumped together due to low support in ancestral nodes), and a Tukey test for post hoc analysis using a pairwise comparison for the traits that were shown to be statistically significant in ANOVA. A hierarchical cluster analysis using Ward’s D hierarchical agglomerative clustering method (Murtagh and Legendre, 2014) was analyzed with hclust function in R program. The dendrograms were visualized and colored using R package “dendextend version 1.15.1” (Galili, 2015) and further edited in Adobe Illustrator.
Our results show that Maximum Likelihood and Bayesian trees provide a consistent tree topology for Rhinolophus species lineages in COI gene trees. Rhinolophus species in Asia were confirmed to be a monophyletic lineage, with R. JLE, R. sedulus, R. luctus, and R. trifoliatus forming the sister clade of the remaining species (Figure 1A). A polytomy was present in COI gene tree, but the removal of the newly described species R. xinanzhongguensis from China and R. ferrumequinum (Supplementary Figure 3) resolved this polytomy without altering the tree topology, and remaining analysis was rerun without these species. In addition, we identified some possible mislabeled or misidentified sequences in GenBank and BOLD databases in COI for several species complexes that require further revision (i.e., R. affinis/R. cf. ferrumequinum/R. sinicus; R. pusillus/R. lepidus) (Supplementary Table 3). The majority of species relationships were well resolved in the COI gene tree, and therefore this tree was used for further analysis.
In total eight clades were recovered from the final COI tree, and the majority of nodes were supported with high bootstrap values (UFBoot ≥ 95% and SH-aLRT ≥ 80%) and high posterior probabilities for each node (Figure 1A). The eight clades are: (clade 1) consists of R. JLE, R. sedulus, R. luctus, and R. trifoliatus (bootstrap/alrt/posterior probability = 100/99/1), (clade 2) R. chiewkweeae, and R. pearsonii (100/99/1), (clade 3), R. coelophylus, R. shameli, R. creaghi, R. stheno, R. andamanensis, and R. affinis (100/99/1), (clade 4), R. rouxi, R. indorouxi, and R. sinicus (89/78/0.98), (clade 5), R. acuminatus, R. malayanus, and R. chaseni (92/88/0.99), (clade 6), R. robinsoni, R. philippinensis, and R. borneensis (91/93/1), (clade 7), R. lepidus, R. pusillus (89/88/1) and (clade 8), R. macrotis, R. marshalli, and R. rex (100/99/1). We identified potential cryptic species from the trees within species sensu lato, thus, branches with well-supported bootstrap and posterior probabilities were collapsed together (Figure 1A). The interspecific genetic divergence was analyzed between species and within species complexes (inter-clade). The genetic divergence between sister species ranged from 1.82% (R. shameli vs R. coelophyllus) to 15.89% (R. malayanus vs R. acuminatus). Closely related species R. macrotis, R. rex, and R. marshalli have 3.43% genetic divergence (2.94–4.08%) (Supplementary Table 4). Although genetic divergence between some closely related species (sensu-lato) was low (<4%), their recognition as separate species was clearly distinguished based on morphology and acoustic traits with reference to existing field guides (Figures 1B, 3D). For instance, R. rex can be easily distinguished from other two species (R. macrotis and R. marshalli) from large ear size and longer sella and short lancet. R. marshalli differs from R. macrotis by having an additional internarial cup extending behind sella but this is absent in R. macrotis (Figure 1B, acoustic differences between species in Figure 3C). As the lowest genetic divergence between morphologically distinct species was 1.82% in the COI gene, it was used as the threshold for candidates of distinct species and potential cryptic species in prior settings. These divergence values were based on those applied in similar regional bat phylogenetic analysis (i.e., Francis et al., 2010); and used it as prior information in multispecies coalescent model (BPP).
Figure 3. Boxplot of selected characters per potential cryptic species. The statistically significant characters were noted with “*” based on Welch t-test and “***” based on ANOVA. Species listed in x-axis were detailed in methods section.
In total 59 (Supplementary Table 3 and Supplementary Figure 5) potential cryptic species from 11 species sensu lato were recognized according to phylogenetic analysis, or approximately >50% unrecognized species within species complexes (Supplementary Table 5). The intraspecific genetic variation within species ranging from 1.29 to 3.43% within R. macrotis and 2.39, 4.84, 2.2–3.6% within R. stheno, R. luctus, and R. malayanus, respectively. A wide range of genetic divergences between clades within the R. affinis complex was documented, ranging from 0.65 to 5.46%, which indicated some clades formed several incipient species within the group. High genetic divergence in the R. affinis complex were identified from clade1 (see Figure 1) which now recognized as R. andamanensis (Srinivasulu et al., 2019; Figure 1A). However, R. andamanensis is still not listed in the global bats taxonomy database as separate species, but as subspecies of R. affinis7 (Simmons and Cirranello, 2021). Similarly, the R. sinicus complex showed wide genetic divergences ranging from 0.54 to 6.15% indicating a number of potential cryptic species in the group. High genetic divergence was recorded among R. pusillus/R. cf. lepidus species group, with genetic divergences of 9.38–11.43% between R. cf. lepidus (pus1/clade1) and the other clades. This indicated R. cf. lepidus is a distinct species genetically, and R. cf. lepidus individuals currently listed in the other clades may be misidentified specimens, and further taxonomic assignment is needed to evaluate their status (Supplementary Tables 2, 3). However, here we emphasize that genetic data alone should not be the standard in delineating cryptic species in the absence of additional data, especially if using a single gene. Therefore, splitting and collapsing clades in the phylogenetic tree was used as prior information for delineating potential cryptic species or incipient species by associating it with the species delimitation model in BPP.
Bayesian Phylogenetic and Phylogeography (BPP) supported the splitting of the ancestral node for species with 2–3 candidate cryptic species (posterior probability 0.98–1.00) in all prior settings and between two algorithms. Therefore, we identified a total of 14 candidate cryptic species, within each putative species: R. luctus (2), R. stheno (2), R. malayanus (3), R. macrotis (3), R. marshalli (1), and R. rex (3). In contrast, different prior settings greatly impacted the posterior probability of species complexes with more than five potential cryptic species i.e., R. pearsonii, R. affinis, R. pusillus, and R. sinicus (Supplementary Table 6). Posterior probability was relatively low under low θ setting [θ = IG (3 0.04), mean = 0.04/(3 − 1) = 0.02 or two mutations of two random sequences taken per 100 bp] in R. pearsonii (pp = 0.97–0.98), but results remained consistent with different τs prior settings (estimation of divergence time for the root of the tree -as described in the Materials and Methods). The summary of presence and absence of ancestral nodes in R. pearsonii, R. affinis, R. sinicus, and R. pusillus (Figure 2) brings the total number of species in the group to 28 candidates cryptic or incipient species [R. pearsonii (12), R. affinis (5), R. sinicus (5), and R. pusillus (6)]. Our BPP analysis reduces the estimated number of potential cryptic species, initially from phylogenetic and genetic divergence analysis from estimated 59 potential cryptic species and BPP summarized around 44 total of potential cryptic species within 11 species sensu lato (Supplementary Table 3).
To complement species delimitation, we incorporated morphological and acoustic data (detailed measurements are listed in Supplementary Figure 1). Only individuals with complete morphological and acoustic data were included in the analyses, and were given identifiers based on the lineages from species delimitation model analysis. Multivariate Statistical Analysis was conducted for each trait separately. The selected traits from the most important characters contributed in external PCA (Figure 4B), acoustic PCA (Figure 4C), sella PCA (Figure 4D), noseleaf PCA and wing PCA (Figures 4E,F) were taken to reduce the variable dimensions and an all-characters PCA (Figure 4A) was analyzed.
Figure 4. Principal Component Analysis (PCA) for five important characters, the first two axes were retained for each PCA (n = 190; number of potential cryptic species = 15) (The abbreviation of characters were detailed in Supplementary Appendix 1: Supplementary Figure 1). Sella parameters and ears are shown as better predictors for acoustic echolocation calls, meanwhile body size and other external characters including wing are not/weak correlated with echolocation calls parameters.
For prior analysis, all data were scaled due to differences in units applied (e.g., mm, kHz) from the dataset (see supplemental code for details), so the magnitude of range variation needed to be comparable. In total 35 characters were included, and 66.81% total variance was explained by the first two axes, 46.61% on axis 1 (PC1) and 20.20% on axis 2 (PC2) (Figure 4A). The characters contribute to each PC are provided in Supplementary Table 7. Wing aspect ratio (AR) and ratio between hand-wing and arm-wing area (TS) were the less influential variables among all the characters. External characters were positively associated with wing characters (e.g., forearm/FA length with wingspan, y = 11.43 + 0.1179, r2 = 0.732, p << 0.001) and noseleaf width, y = 22.08 + 2.699x, r2 = 0.622, p << 0.001), while sella length was positively and highly correlated with ear length (y = 10.99 + 2.389x, r2 = 0.744, p << 0.001). In contrast, acoustic call parameters such as FmaxE were negatively correlated with ears (y = 152.7 − 3.725x, r2 = 0.77, p << 0.001) and sella length (y = 116.4 – 10.1x, r2 = 0.738, p << 0.001) (generally, larger ears and wider and taller sella correlated to lower acoustic calls). We also note that there is no/weak correlation between acoustic call parameters (FMaxE) with external traits and wing traits (i.e., forearm length, y = 165.55 – 1.91x, r2 = 0.322, p << 0.001; head-body length, y = 142.07 – 1.32x, r2 = 0.24, p << 0.001; body mass, y = 86.42 − 0.93x, r2 = 0.069, p < 0.001); aspect ratio, y = 90.1 – 1.957x, r2 = 0.00828, p = 0.212; wing loading, y = 76.38 + 0.1602x, r2 = 0.000246, p = 0.83; wing span, y = 142 – 0.219x, r2 = 0.224, p << 0.001; lancet and noseleaf width, y = 153.4 – 8.52, r2 = 0.548, p << 0.001; and noseleaf length, y = 146.2 – 5.365x, r2 = 0.489, p << 0.001; Supplementary Figure 4 and Supplementary Table 8). Here we arbitrarily used a threshold of r2 > 0.5 (more than 50% of variance found in the response variable can be explained by predictor variables) to show stronger relationships between predictor and response variables, and no/weak relationship if r2 < 0.5. Significant p-values indicated there is relationship between our predictors and response variables (except aspect ratio and wing loading) as also shown in PCA Figure 4A. However, even if r2 –values were low, the observed relationships were statistically significant in most cases, suggesting that analyzed regression models need more attention. The results showed a weak correlation between most of body size characters such as forearm length, head-body length and body mass against call frequencies. This result may be due to allometric deviation in some species, for instance, small body size with lower calls of R. rex, R. macrotis, and R. marshalli. However, the best predictors which correlated with acoustic call frequencies were ears and sella traits (r2 = 0.77, r2 = 0.738, respectively), indicating the importance of sella to consider for identification traits as it is highly correlated with call emissions. The internarial cups width (INCW) was also negatively related to the frequency of maximum energy in echolocation calls (y = 99.15 – 6.978x, r2 = 0.698, p << 0.001) indicating the character may play an important role in call emission (Figure 4A and Supplementary Figure 4). The PCA for all characters shows the pattern of species and potential cryptic species interacted with measured morphological traits and acoustic in morphospace based on PCA analysis.
We then separated four Principal Component Analyses (acoustic, noseleaf, sella and wing characters), and retained the first two dimensions (Figures 4B–D and Supplementary Table 7). External, sella and acoustic traits provide a good character sets for grouping potential cryptic species; however, noseleaf traits (posterior and anterior) and wing traits do not show as good traits in grouping the potential cryptic species. A dendrogram from agglomerative hierarchical cluster analysis using Ward’s algorithm method showed that the pattern of all measured traits corresponds to each observed individual measurement (Figure 1B). The tips with minimum variance were clustered together and significant dissimilarity were coalesced at the higher branch height. Ward’s hierarchical cluster showed the value of using multiple traits in species identification (combination of external, acoustic, noseleaf, sella and wing traits). The individuals were accurately assigned to correct species group in the same cluster when all traits were included, but showed lower accuracy in clustering when one or more traits were not included in the analysis (Figures not provided). The results also showed a well-clustered of potential cryptic species within R. macrotis and R. pusillus groups indicating that candidate species can be distinguished with morphological and acoustic data. In contrast, morphologically indistinguishable candidate cryptic species were shown in R. sinicus and R. affinis indicating highly cryptic species within some species groups.
A simple statistical analysis was conducted to examine highly significant characters between potential cryptic species. The characters that are significantly different between two potential cryptic species are presented in Supplementary Table 9 and Supplementary Figures 5A–D, and selected trait were shown in boxplot (Figures 3A–F). A Welch t-test was used to assess significant traits to differentiate between potential cryptic species within R. affinis, R. macrotis, and R. pusillus. An ANOVA and Tukey test were conducted for R. sinicus. Acoustic differentiation between potential cryptic species showed significantly differences between FMAXE of R. affinis (aff11 = 76.45 kHz, aff34 = 84.46 kHz, p-value = 3.43e-05), R. macrotis (mac3A = 75.97 kHz, max3B = 59.4 kHz, p-value = 2.3e-16), R. pusillus (pusM = 91.44, pusS = 103, p-value = 0.0004) and R. sinicus (sin2 = 83.03 kHz, sin34 = 86.49 kHz, sin89 = 82.95 kHz). Acoustic differentiation cannot be used in isolation for species identification (though it can signal potential cryptic species in need of further study), but accompanied with other morphological traits it can provide useful support. Although R. affinis showed two significantly distinct phonic types, but had highly similar morphological measurements (i.e., forearm length, aff11 = 51.98 mm and aff34 = 52.16 mm, p-value = 0.87). In contrast, in R. sinicus (sin3 and sin 89) are not different acoustically, but showed distinct morphological characteristics (forearm length; sin2 = 44.56 mm, sin89 = 51.62 mm; ear length: sin2 = 14.28 mm, sin89 = 19.17 mm, and including other morphological traits (Supplementary Table 9).
Species relationships based on phylogenetic analysis using COI gene were generally consistent with previous publications in this taxonomic group. The phylogenetic relationship is in concordance with morphological identification of species group, for instance, R. luctus, R. sedulus, and R. trifoliatus are grouped together due to similarity in noseleaf structure by the presence of lateral lappets in the base of sella and long woolly hair. R. JLE in the same clade, closer to newly described species R. francisi (not included in this study), and which appears to have similar morphological appearance to R. luctus, although further taxonomic assessment is still needed (Soisook et al., 2015). The results also showed the strength of COI gene for validation of species identification, which has been highlighted in other studies (Francis et al., 2010; Kekkonen and Hebert, 2014; Srinivasulu et al., 2019). The placement of R. luctus, R. JLEsp, R. sedulus, and R. trifoliatus clades as sister to all other Asian Rhinolophidae, is in broad agreement with other publications, even using different genetic markers, including nuclear introns and partial mtDNA (e.g., Francis et al., 2010; Stoffberg et al., 2010; Foley et al., 2015; Dool et al., 2016). Joint maximum likelihood and Bayesian tree inferences were able to infer better support for most of the inter-species relationships using COI, but multifurcating branches/polytomies still occur within younger species groups (e.g., Francis et al., 2010). The polytomy in main branch was resolved when two species were removed from the analysis, R. ferrumequinum and R. xinanzhongguensis, which are closely related to Afro-Palearctic lineages (Zhou et al., 2009). The presence of polytomies in the tree may be due to multiple divergence events simultaneously between the species groups (Lin et al., 2011), insufficient information from a single gene to resolve relationships between clades, or rapid speciation between these clades. Thus, we agree with previous publications that multiloci approaches can yield more robust phylogenetic information (Posada and Crandall, 1998; Baker and Bradley, 2006; Salicini et al., 2011; Yang and Rannala, 2014; Dool et al., 2016). Phylogenies using only mitochondrial genes may be susceptible to mitochondrial introgression or incomplete lineage sorting, thus requires evaluation against nuclear phylogenies to fully validate. Previous studies have reported the mito-nuclear discordances in Rhinolophidae with mtDNA introgression (Dool et al., 2016; Demos et al., 2019; Mao and Rossiter, 2020); however, these studies typically have limited taxonomic coverage and extended geographical sampling is still needed. Dool et al. (2016), used nuclear introns, but could not resolve the more internal relationships in the families. Additionally, Demos et al. (2019), also found conflicting signals between nuclear and mitochondrial phylogenies, and suggested expanding geographic sampling to resolve these uncertainties. The aforementioned studies focused on Afrotropical clades with limited taxonomic sampling from Asia lineages, highlighting the need for further research.
However, these approaches come with challenges, whilst concept and theory are one thing, obtaining sufficient data can be challenging (Blomberg et al., 2003). Our findings are supported by analysis limited to particular species groups, for instance R. macrotis (Zhang et al., 2018; Liu et al., 2019) and R. sinicus (Mao et al., 2019), these studies sampled different populations in China but included few samples from the rest of Southeast Asia. Zhang et al. (2018) used mito-nuclear genes to assess species boundaries in the R. macrotis group and concluded that R. cf. siamensis, R. huananus, and R. cf. macrotis should be lumped together because there was insufficient evidence to support delineating between these putative species. Hence, they suggest revising the taxonomy of R. huananus as a synonym of R. cf. siamensis and R. cf. macrotis. Analysis based on multilocus data (Zhang et al., 2018) agrees with our results, as the result placed R. macrotis, R. cf. siamensis, and R. huananus in one clade [R. macrotis identifies with mac3 (see Supplementary Table 3)], even though we are using single gene analysis. Regardless number of genes and loci used in phylogenetic studies, we emphasize the importance on using integrative taxonomic approaches (Dayrat, 2005; Will et al., 2005; Agnarsson and Kuntner, 2007; Vogler and Monaghan, 2007; Francis et al., 2010; Padial et al., 2010; Fujita et al., 2012; Taylor et al., 2018) in delineating species by combining phenotypic and genotypic data. Furthermore, the identification of key characters to reliably identify these species in the field are essential to ensure that species can be accurately identified, and thereby to survey their distribution more easily.
The informativeness of intra- and interspecific genetic differences varies between species groups, and the species can be categorized in the “gray zone of speciation” when the genetic divergences were lower than 2% (Roux et al., 2016). Low levels of genetic divergence between allopatric populations may result from frequent gene flow in the past, and low genetic divergence within sympatric populations may be due to hybridization or genetic introgression. Low genetic divergence in extant taxa may be the result of hybridization, cross-species gene flow, mitochondrial introgression and imperfect reproductive isolation which may occur with secondary contact during ancestral range expansion (Huang, 2020; Smith and Carstens, 2020; Çoraman et al., 2020; Jiao and Yang, 2021). In contrast, high levels of intraspecific genetic divergences between allopatric populations indicated substantial geographic variation in COI gene, nevertheless, it also shows a high genetic divergence within some sympatric lineages (Francis et al., 2010).
Multispecies coalescent models (MSC) have proved to be statistically efficient with more intensive computation in assigning cryptic species using single locus data even though it contains less phylogenetic information than those using more genetic information (Yang and Rannala, 2017; Leaché et al., 2019). However, more reliable results may be obtainable by increasing the number of loci analyzed, especially for the case of incipient species with a high probability of gene flow between populations. Different combinations of priors were used, due to the lack of empirical data for certain parameters such as population size (θs) and the divergence time at the root of species tree (τ0) of Rhinolophidae which was measured by the expected number of mutations per site, to project the possibility of different substitution rates between species (Nabholz et al., 2007, 2009). Across all the prior settings, BPP shows no influence of prior combinations for species with 2–3 groups, but affects the posterior probability of species model for species with >5 groups, hence should be used with caution. The computational constraint increases when large numbers of groups are analyzed, which may affect the species delimitation model chosen for different prior combinations.
Current BPP methods generally ignores gene flow, which implies the algorithm does not consider the migration rate in the current generation, or in ancestral populations (Yang and Rannala, 2010, 2017; Leaché et al., 2019; Flouri et al., 2020). Ignoring gene flow may cause the algorithm to detect populations and sub-populations rather than cryptic species (Sukumaran and Knowles, 2017). Thus other algorithms must be utilized to reflect gene flow to resolve these issues, for instance using genealogical divergence index (gdi) (although this is challenging to meaningfully analyze without nuclear DNA) (Jackson et al., 2017; Long and Kubatko, 2018; Eberle et al., 2019; Leaché et al., 2019; Chan et al., 2020; Jiao and Yang, 2021). Therefore, in the absence of gene flow, we assumed the result from BPP in our analysis may be over-split due to the high mobility of most species of bat, although it should be noted that species-specific ecomorphology means some species may still be unlikely to move through open landscapes. Although each candidate does not necessarily represent new species, they represent separate maternal lineages with long histories of evolutionary independence. Despite lacking a migration rate in its algorithm, BPP utilizes a more robust framework based on multispecies coalescent models, which are computationally effective compare to other species delimitation methods (i.e., general mixed Yule coalescent model (GMYC) (Fujisawa and Barraclough, 2013), Poisson Tree Processes (PTP, m-PTP) (Zhang et al., 2013; Kapli et al., 2017; Luo et al., 2018) and significantly improves single-locus species delimitation (Yang and Rannala, 2017). A “next-generation barcoding” using a single gene and incorporating a multispecies coalescent model has been proven to improve the standard barcoding framework (Dowton et al., 2014; Yang and Rannala, 2017). Thus when used in conjunction with phenotypic data this approach provides a low-cost tool to achieve a reliable result when limited genes are available. However, incorporating multiple genes as a standard would enhance our ability to better understand species diversity and their evolutionary history, and standard collection efforts should ensure that such data is shared. Furthermore, systematic work should not rely on genetic data alone, but potential species should require validation with multiple data types such as phenotypic, geographical distribution, behavioral and ecological information (Sukumaran and Knowles, 2017; Luo et al., 2018). Therefore, it is crucial to integrate other taxonomic data such as morphology and acoustics, to determine whether species are cryptic or incipient. The importance of using such approaches to reduce the risk of false-positives (type II error), which could artificially inflate the number of potential species should not be understated.
Our analysis shows incongruence between genetic and morphological data in some instances when genetic divergence is low. We identified two sister R. macrotis lineages, but they are morphologically and acoustically distinct. In contrast, conserved morphology with little variation was present in R. affinis but there was high divergence in genetic and acoustic traits. Theoretically, genotype and phenotype are expected to be congruent (Pavey et al., 2010); however, incongruence between genotype and phenotype is possible (Jacobs et al., 2013, 2016; Schumer et al., 2015), in most cases in widespread species with high mobility and unidentified reproductive isolation barriers. In addition, for recent divergences in the early stages of speciation, the morphological disparities may be not have accumulated yet. Although morphological characters are conserved, some selective traits are advantageous in evolution such as acoustics have the potential to form a pre-mating barrier and thus prevent gene-flow even in the absence of physical barriers (either geographic, or functional such as bacular differences). The multifunctional use of calls in the Rhinolophidae may act as pre-mating barrier, subject to pressures of climate, landscape structure, dietary availability, and community structure. Yet whilst these factors and acoustic partitioning between conspecific species, the presence of an acoustic fovea means that short time periods in allopatry may enable the formation of pre-mating barriers in the absence of morphological change. Acoustic fovea in Rhinolophidae act to compensates for the Doppler shift effect, and the returning echoes must fall within the frequency range of acoustic fovea (Schuller and Pollak, 1979; Neuweiler, 1990). This fine-tuned function is closely related to acoustic traits, the emitter and the receiver (Kingston et al., 2001). Thus, only morphological characters that play an important role in emitting and receiving acoustic calls that may varies when call frequency are different. This is in agreement with our result in general as sella and lancet (as the emitter) and ears (as the receiver) are correlated with acoustic calls (FMAXE).
Our acoustic data from bat populations in other parts of Southeast Asia was limited (as molecular and acoustic data had to be paired); however, the results based on available data is consistent with BPP analysis. For instance, R. affinis shows two phonic types with acoustic differences of 8 kHz [aff34 = 84.46 kHz (83.3–85.88 kHz) and aff11 = 76.45 kHz (76–77.04 kHz)], in concordance with BPP analysis. In BPP, we merged R. affinis clade 3 and 4 because of the low support of splitting in ancestral nodes between them (thus, aff34), but high support of split to R. affinis clade 11 (Figures 1, 2). The two phonic types of R. affinis are genetically and acoustically different, yet morphologically very similar. Acoustic differentiation in R. affinis is also reported to be geographically diverse, ranging from 68.9 (Borneo) to 82.3 (Java, Indonesia) (Ith et al., 2015). The acoustic divergence in this species may result from periods of allopatry where populations were subjected to different pressures (diet, habitat structure, and community composition) driving differences in the call frequency of maximum energy (FMAXE) of each population and forming a pre-mating barrier. Little morphological variation also found R. pusillus, although the call frequency between two potential cryptic species is significantly different (91.44 and 103 kHz). Nevertheless, high variations between potential cryptic species are found within R. macrotis (18 characters) and R. sinicus (19 characters) (Supplementary Figure 5). Wing characters such as wingspan and aspect ratio are significantly different between the two phonic types of R. macrotis. Meanwhile, wing loading and wing-tip index are significantly different in three R. sinicus potential cryptic species. The differences in wing shape between potential cryptic species may correlate with niche partitioning strategy by partitioning foraging space, or foraging method and to facilitate species coexistence in sympatry, or occupy slightly different habitats with different degrees of forest density.
Multiple hypotheses have been proposed to understand the ecology and the evolutionary significance of acoustic differences in morphologically conserved species. First, acoustic differentiation can be the consequence of genetic drift and or founder effects in isolated populations, or selection within communities (Jones, 1997). The isolation process has potential to form a pre-mating barrier when the population becomes sympatric with other populations of that the same species due to a lack of con-specific recognition. Second, the “acoustic resource partitioning” hypothesis, suggests acoustic divergences may occur to partition dietary niches and thus assist foraging efficiency (Stoffberg et al., 2011). Third, “social selection models” hypothesis, which hypothesized that acoustic divergence can occur when acoustic calls are used for intraspecific communication and to reduce competition with conspecifics (Guillen et al., 2000). The first hypothesis is more likely applicable to allopatric populations. The second and third hypotheses may be applicable in sympatric populations, and acoustic divergences may also evolve as the result of interactions with other environmental factors such open and closed landscape, community structure, resource availability, and other ecological drivers. As Rhinolophidae emit the echolocation calls through nasal passages, and acoustic differences may align with phenotypic disparities expressed as noseleaf characters.
The majority of the characters that are significantly different between potential cryptic species which also differ acoustically are sella characters. Sella characters were shown to be significantly correlated with acoustic traits, this result is in contrast with general allometric hypothesis. In allometric hypothesis, acoustic call frequency decreases as body size increases (Jacobs and Barclay, 2007), however, our result showed that body characters such as forearm and body mass have a weaker relationship with acoustic calls than sella characters. Furthermore, our results highlight the importance of sella shape in distinguishing potential cryptic species as it exhibits a strong relationship with ear morphology and acoustic calls frequency. Species with lower frequency acoustic calls tend to have larger ears, and wider-taller sellas. For instance, R. rex, R. luctus, and R. marshalli have relatively lower frequency calls and larger ears and sellas relative to body size. In contrast, R. sinicus, R. pusillus, R. affinis, and R. stheno have relatively smaller ears and narrow-smaller sella. Thus, we identify sella traits to be some of the most important traits to identify cryptic species. This result agrees with previous experimental studies which stated that noseleaf and ear pinnae play important roles in acoustic and the variation are driven by a trade-off between sensitivity and accuracy (Reijniers et al., 2010).
The acoustic function associated with sella length is to forming baffles for the echolocation ultrasound beam. Species with larger sella produce narrower acoustic beams, thus produce low call frequencies, which is consistent with basic physical principles: the larger an aperture is relative to wavelength, the narrower a beam it is capable of producing (Zhang et al., 2009). In R. rex (25 kHz) for example, the use of low call frequencies and focus beams suffer atmospheric sound absorption (Zhang et al., 2009), thus wider coverage can only be achieved by scanning which is time-consuming and energetically costly. In addition, the furrows in the lancet have been shown to act as resonance cavities to widen biosonar/echolocation beam in other species (Zhuang and Müller, 2006), which are absent and less developed in R. rex but well developed in other species with higher frequencies such as R. affinis. The acoustic function of lancet furrows which may widen the acoustic beam may be inferred as a strategy for monitoring the ground to maintain a constant weight and avoid ground collision, as some rhinolophid bats are known to fly close to the ground (i.e., R. rouxi) (Neuweiler, 1990; Zhuang and Müller, 2006). Therefore, furrows in the lancet also assist directional sensitivity to enable foraging and navigation. When species have a basal lappet (i.e., R. luctus), and well-developed lancet furrows, these two structures decrease the ambiguity in selecting relevant targets for ranging by focusing emission beams in the FM component of the calls (Vanderelst et al., 2013). Other than Rhinolophidae, another nasal-emitting family is Phyllostomidae which also develop distinctive noseleaf structures. In Phyllostomidae (New-World leaf-nosed bats), long lancet-shaped processes are related to nasal echolocators and have significantly narrower nostrils than species which rely on oral echolocation (e.g., Vespertilionidae). However, the variety of noseleaf structures in animal-eating Phyllostomidae bats (subfamily Phyllostominae) coincided with variation in diet, but not with variety in echolocation calls (Bogdanowicz et al., 1997). Nasal echolocators with narrow nostrils may be due to a trade-off between sonar pulse emission and stereo-olfaction (Brokaw and Smotherman, 2020). For instance, some insectivorous bats, such as Molossidae, may rely on stereo-olfaction more than expected which may hypothesized similarly in other insectivorous bats (Brokaw and Smotherman, 2020), although olfactory tracking in insectivorous Rhinolophidae remains under-studied. Further studies investigating the evolutionary trade-offs of echolocation of nasal-emitting bats with morphological, physiological and behavioral studies may improve our understanding on the function of various noseleaf features in Rhinolophidae. In this study, we highlight the importance of noseleaf features in shaping acoustic emission, and each part of the noseleaf has a significant function in emission acoustic calls and are not mere decorations, thus their value as traits in cryptic species identification should not be underestimated.
Our study demonstrates that the use of available datasets in conjunction with testing multispecies coalescent models offers a promising approach to the delineation of species in cryptic Rhinolophidae. The initial 11 Rhinolophus species are actually species complexes which potentially harbor a large number of cryptic species with a total of 44 candidate cryptic species (i.e., R. luctus, R. pearsonii, R. shameli, R. stheno, R. affinis, R. sinicus, R. malayanus, R. pusillus, R. macrotis, R. marshalli, and R. rex) in Southeast Asia and South China. Within them, we estimate 15 potential cryptic species from South China Karst alone using a combination of phenotypic and genotypic approaches. This result highlights the probable underestimation of richness during biodiversity assessments. However, due to limitations in this study (only CO1 has been collected from bats in most of Asia), we recommend using multiple loci and genes for future studies, and highlights the value of incorporating complete morphological and acoustic data. The value of these approaches to identify and delineate cryptic species is further supported by other studies in specific clades (Zhang et al., 2018, 2021; Liu et al., 2021), and further data would bolster patterns shown here. In addition, validation using measurements to identify the phenotypic disparities in various characteristics to support the likelihood of separate species, in particular for noseleaf and wing characters, are often neglected in phylogenetic analysis. Experimental and computational studies investigating the relationship between acoustic emissions and noseleaf structure highlight the importance of noseleaf traits in Rhinolophidae. We propose noseleaf metrics should be adopted as basic measures in bat monitoring, as invasive approaches cannot be applied whilst in the field, and as bats are long-lived and have slow reproduction rate, thus obviously non-destructive measures are needed. Other traits such as dental, cranial, mandibular and bacular characters are frequently used to identify species in rodents and various bat families such as Miniopteridae and Vespertillionidae (Francis et al., 2007; Goodman et al., 2009). Yet insufficient data exists to understand which traits are relevant, and may not recognize cryptic diversity at fine scales (Taylor et al., 2018). Selecting characters at fine scales to identify cryptic species may be different in each bat family, and further studies are needed to optimize trait selection to accurately identify species for other bat families. Voucher specimens provide important information for taxonomic studies, however, a careful consideration of sample size and other biological information is needed (i.e., life expectancy, population growth rates, and threats). Additional information on morphology can be obtained with standardized photographs, which can be deposited digitally for wider access and greater ease of comparison with other specimens. As key traits such as noseleaf traits and other soft tissue may deform or change color in alcohol or formaldehyde solution in museum specimens, thus digital specimens taken from live individuals with scaled-photographs provides an alternative to use for long term research and are less destructive.
Our study highlights the importance of using molecular species delimitation methods in concert with an integrative taxonomic framework to avoid false positives that would potentially mislead the identification of cryptic species. The use of MSC models in BPP analysis in Rhinolophidae proved to be a valuable approach to recognize hidden species within a putative species complex, however, using a range of different prior settings (the ratio of population size to divergence times) are crucial to ensure accurate validation of species delimitation models. Morphologically and acoustically, our results show deviation of general rules of the allometric hypothesis, suggesting that body size is weakly correlated with acoustic calls in Rhinolophidae. However, ears, sella traits and internarial cups are the better predictors of potential cryptic species, and are highly correlated with acoustic call frequencies. Each part of noseleaf plays an important role in call emission and acoustic beam formation which supports the importance of sella and lancet measures as diagnostic traits for potential cryptic species. We demonstrate that detailed measurements of sella characters significantly contribute to our ability to delineate cryptic species, yet these characters are generally overlooked in assessments. We identified 44 potential cryptic rhinolophids species within 11 species of Rhinolophus sensu lato in Southeast Asia and South China, highlighting the need for further work to describe species across the region and better understand their cryptic diversity, and underscoring the unappreciated cryptic diversity in the group. Increasing sampling size for systematic and geographic coverage with multilocus information, supplemented with morphological and acoustic data from the region would facilitate assessment of the diversity, ecology and evolutionary of rhinolophids regionally and globally.
The datasets presented in this study can be found in online repositories. The names of the repository/repositories and accession number(s) can be found in the article/Supplementary Material.
The animal study was reviewed and approved by Xishuangbanna Tropical Botanical Garden Ethics Committee.
AH and AC conceived the idea, edited, and revised the draft of the manuscript. AC analyzed the raw data, conducted the analysis, and drafted the initial draft of the manuscript. AH, AC, and JL conducted the fieldwork, reviewed, and approved the final submission.
This project was supported by the Chinese National Natural Science Foundation (Grant No. U1602265, Mapping Karst Biodiversity in Yunnan), the Strategic Priority Research Program of the Chinese Academy of Sciences (Grant No. XDA20050202), and the Chinese Academy of Sciences Southeast Asia Biodiversity Research Center fund (Grant No. Y4ZK111B01).
The authors declare that the research was conducted in the absence of any commercial or financial relationships that could be construed as a potential conflict of interest.
All claims expressed in this article are solely those of the authors and do not necessarily represent those of their affiliated organizations, or those of the publisher, the editors and the reviewers. Any product that may be evaluated in this article, or claim that may be made by its manufacturer, is not guaranteed or endorsed by the publisher.
The Supplementary Material for this article can be found online at: https://www.frontiersin.org/articles/10.3389/fevo.2022.854509/full#supplementary-material
Agnarsson, I., and Kuntner, M. (2007). Taxonomy in a changing world: seeking solutions for a science in crisis. Syst. Biol. 56, 531–539. doi: 10.1080/10635150701424546
Avise, J. C. (2009). Phylogeography: retrospect and prospect. J. Biogeogr. 36, 3–15. doi: 10.1111/j.1365-2699.2008.02032.x
Baker, R. J., and Bradley, R. D. (2006). Speciation in mammals and genetic species concept. J. Mammal 87, 643–662.
Bickford, D., Lohman, D. J., Sodhi, N. S., Ng, P. K. L., Meier, R., Winker, K., et al. (2007). Cryptic species as a window on diversity and conservation. Trends Ecol. Evol. 22, 148–155. doi: 10.1016/j.tree.2006.11.004
Blomberg, S. P., Garland, T., and Ives, A. R. (2003). Testing for phylogenetic signal in comparative data: behavioral traits are more labile. Evolution 57, 717–745. doi: 10.1111/j.0014-3820.2003.tb00285.x
Bogdanowicz, W. (1992). Phenetic relationship among bats of the family Rhinolophidae. Acta Theriol. 37, 213–240. doi: 10.4098/AT.ARCH.92-22
Bogdanowicz, W., Csada, R. D., and Fenton, B. M. (1997). Structure of noseleaf, echolocation and foraging behaviour in the Phyllostomidae (Chiroptera). J. Mammal 78, 942–953.
Bogdanowicz, W., Hulva, P., Bolfíková, B. C., Buś, M. M., Rychlicka, E., Sztencel-Jabłonka, A., et al. (2015). Cryptic diversity of Italian bats and the role of the Apennine refugium in the phylogeography of the western Palearctic. Zool. J. Linn. Soc. Lond. 174, 635–648. doi: 10.1111/zoj.12248
Brokaw, A. F., and Smotherman, M. (2020). Role of ecology in shaping external nasal morphology in bats and implications for olfactory tracking. PLoS One 15:e0226689. doi: 10.1371/journal.pone.0226689
Carstens, B. C., Pelletier, T. A., Reid, N. M., and Satler, J. D. (2013). How to fail at species delimitation. Mol. Ecol. 22, 4369–4383. doi: 10.1111/mec.12413
Chan, K. O., Hutter, C. R., Wood, P. L., Grismer, L. L., Das, I., and Brown, R. M. (2020). Gene flow creates a mirage of cryptic species in a Southeast Asian spotted stream frog complex. Mol. Ecol. 29, 3970–3987. doi: 10.1111/mec.15603
Çoraman, E., Dundarova, H., Dietz, C., and Mayer, F. (2020). Patterns of mtDNA introgression suggest population replacement in Palaearctic whiskered bat species: population replacement in whiskered bats. R. Soc. Open Sci. 7:191805. doi: 10.1098/rsos.191805rsos191805
Dayrat, B. (2005). Towards integrative taxonomy. Biol. J. Linn. Soc. 85, 407–415. doi: 10.1111/j.1095-8312.2005.00503.x
De Queiroz, K. (2007). Species concepts and species delimitation. Syst. Biol. 56, 879–886. doi: 10.1080/10635150701701083
Demos, T. C., Webala, P. W., Goodman, S. M., Peterhans, J. C. K., Bartonjo, M., and Patterson, B. D. (2019). Molecular phylogenetics of the African horseshoes bats (Chiroptera: Rhinolophidae): expanded geographic and taxonomic sampling of the Afrotropics. BMC Evol. Biol. 19:166. doi: 10.1186/s12862-019-1485-1
DeSalle, R., Egan, M. G., and Siddall, M. (2005). The unholy trinity: taxonomy, species delimitation and DNA barcoding. Philos. Trans. R. Soc. B Biol. Sci. 360, 1905–1916. doi: 10.1098/rstb.2005.1722
Dool, S. E., Puechmaille, S. J., Foley, N. M., Allegrini, B., Bastian, A., Mutumi, G. L., et al. (2016). Nuclear introns outperform mitochondrial DNA in inter-specific phylogenetic reconstruction: lessons from horseshoe bats (Rhinolophidae: Chiroptera). Mol. Phylogenet. Evol. 97, 196–212. doi: 10.1016/j.ympev.2016.01.003
Dowton, M., Meiklejohn, K., Cameron, S. L., and Wallman, J. (2014). A preliminary framework for DNA barcoding, incorporating the multispecies coalescent. Syst. Biol. 63, 639–644. doi: 10.1093/sysbio/syu028
Eberle, J., Bazzato, E., Fabrizi, S., Rossini, M., Colomba, M., Cillo, D., et al. (2019). Sex-biased dispersal obscures species boundaries in integrative species delimitation approaches. Syst. Biol. 68, 441–459. doi: 10.1093/sysbio/syy072
Esselstyn, J. A., Evans, B. J., Sedlock, J. L., Ali, F., Khan, A., and Heaney, L. R. (2012). Single-locus species delimitation: a test of the mixed Yule – coalescent model, with an empirical application to Philippine round-leaf bats. Proc. R. Soc. B 279, v3678–v3686. doi: 10.1098/rspb.2012.0705
Flouri, T., Rannala, B., and Yang, Z. (2020). “Chapter no.5.6 – A tutorial on the use of BPP for species tree estimation and species delimitation,” in Phylogenetic in the Genomic Era, eds C. Scornavacca, F. Delsuc, and N. Galtier 5.6:1–5.6:16. Available online at: https://hal.inria.fr/PGE
Foley, N. M., Thong, V. D., Soisook, P., Goodman, S. M., Armstrong, K. N., Jacobs, D. S., et al. (2015). How and why overcome the impediments to resolution: lessons from rhinolophid and hipposiderid bats. Mol. Biol. Evol. 32, 313–333. doi: 10.1093/molbev/msu329
Francis, C. M. (2019). Field Guide to the Mammals of South-East Asia, 2nd Edn. London: Bloomsbury Wildlife.
Francis, C. M., Borisenko, A. V., Ivanova, N. V., Eger, J. L., Lim, B. K., Guillén-Servent, A., et al. (2010). The role of DNA barcodes in understanding and conservation of mammal diversity in Southeast Asia. PLoS One 5:e12575. doi: 10.1371/journal.pone.0012575
Francis, C. M., Kingston, T., and Zubaid, A. (2007). A new species of Kerivoula (Chiroptera: Vespertilionidae) from peninsular Malaysia. Acta Chiropterol. 9, 1–12.
Fujisawa, T., and Barraclough, T. G. (2013). Delimiting species using single-locus data and the generalized mixed yule coalescent approach: a revised method and evaluation on simulated data sets. Syst. Biol. 62, 707–724. doi: 10.1093/sysbio/syt033
Fujita, M. K., Leaché, A. D., Burbrink, F. T., McGuire, J. A., and Moritz, C. (2012). Coalescent-based species delimitation in an integrative taxonomy. Trends Ecol. Evol. 27, 480–488. doi: 10.1016/j.tree.2012.04.012
Funk, D. J., and Omland, K. E. (2003). Species-level paraphyly and polyphyly: frequency, causes and consequences, with insights from animal mitochondrial DNA. Annu. Rev. Ecol. Syst. 34, 397–423. doi: 10.1146/annurev.ecolsys.34.011802.132421
Galili, T. (2015). ‘dendextend’: an R package for visualizing, adjusting and comparing trees of hierarchical clustering. Bioinformatics 31, 3718–3720. doi: 10.1093/bioinformatics/btv428
Goodman, S. M., Drive, S., Maminirina, C. P., Weyeneth, N., Ruedi, M., Bradman, H. M., et al. (2009). The use of molecular and morphological characters to resolve the taxonomic identity of cryptic species: the case of Miniopterus manavi (Chiroptera, Miniopteridae). Zool. Scr. 38, 339–363. doi: 10.1111/j.1463-6409.2008.00377.x
Grinnell, A. D. (2009). “Echolocation I: behavior,” in Encyclopedia of Neuroscience, ed. L. R. Squire (Cambridge, MA: Academic Press).
Guillen, A., Juste, J., and Ibanez, C. (2000). Variation in the frequency of the echolocation calls of Hipposideros ruber in the Gulf of Guinea: an exploration of the adaptive meaning of the constant frequency value in rhinolophoid CF bats. J. Evol. Biol. 13, 70–80. doi: 10.1046/j.1420-9101.2000.00155.x
Hebert, P. D. N., and Gregory, T. R. (2005). The promise of DNA barcoding for taxonomy. Syst. Biol. 54, 852–859. doi: 10.1080/10635150500354886
Hebert, P. D. N., Ratnasingham, S., and Jeremy, R. (2003). Barcoding animal life: cytochrome c oxidase subunit 1 divergences among closely related species. Proc. R. Soc. Lond. B 270, (Suppl. 1), S96–S99. doi: 10.1098/rsbl.2003.0025
Hoang, D. T., Chernomor, O., Von Haeseler, A., Minh, B. Q., and Vinh, L. S. (2018). UFBoot2: improving the ultrafast bootstrap approximation. Mol. Biol. Evol. 35, 518–522. doi: 10.1093/molbev/msx281
Huang, J. P. (2020). Is population subdivision different from speciation? From phylogeography to species delimitation. Ecol. Evol. 10, 6890–6896. doi: 10.1002/ece3.6524
Ith, S., Bumrungsri, S., Furey, N. M., Bates, P. J., Wonglapsuwan, M., Anwarali Khan, F. A., et al. (2015). Taxonomic implications of geographical variation in Rhinolophus affinis (Chiroptera: Rhinolophidae) in mainland Southeast Asia. Zool. Stud. 54:e31. doi: 10.1186/s40555-015-0109-8
Jackson, N. D., Carstens, B. C., Morales, A. E., and O’Meara, B. C. (2017). Species delimitation with gene flow. Syst. Biol. 66, 799–812. doi: 10.1093/sysbio/syw117
Jacobs, D. S., and Barclay, R. M. R. (2007). The allometry of echolocation call frequencies of insectivorous bats: why do some species deviate from the pattern? Oecologia 152, 583–594. doi: 10.1007/s00442-007-0679-1
Jacobs, D. S., Babiker, H., Bastian, A., Kearney, T., van Eeden, R., and Bishop, J. M. (2013). Phenotypic convergence in genetically distinct lineages of a Rhinolophus species complex (Mammalia, Chiroptera). PLoS One 8:e82614. doi: 10.1371/journal.pone.0082614
Jacobs, D. S., Mutumi, G. L., Maluleke, T., and Webala, P. W. (2016). “Convergence as an evolutionary trade-off in the evolution of acoustic signal: echolocation in horsehoe bats as a case study,” in Evolutionary Biology, ed. P. Pontarotti (Switzerland: Springer International Publishing), 89–103.
Jiao, X., and Yang, Z. (2021). Defining species when there is gene flow. Syst. Biol. 70, 108–119. doi: 10.1093/sysbio/syaa052
Jones, G. (1997). Acoustic signals and speciation: the roles of natural and sexual selection in the evolution of cryptic species. Adv. Study Behav. 26, 317–354. doi: 10.1016/S0065-3454(08)60383-6
Jones, G., and Barlow, K. (2004). “Cryptic species of echolocating bats,” in Echolocation in Bats and Dolphins, eds J. Thomas, C. Moss, and M. Vater (Chicago, IL: Chicago University Press), 345–349.
Kalyaanamoorthy, S., Minh, B. Q., Wong, T. K. F., Von Haeseler, A., and Jermiin, L. S. (2017). ModelFinder: fast model selection for accurate phylogenetic estimates. Nat. Methods 14, 587–589. doi: 10.1038/nmeth.4285
Kapli, P., Lutteropp, S., Zhang, J., Kobert, K., Pavlidis, P., Stamatakis, A., et al. (2017). Multi-rate Poisson tree processes for single-locus species delimitation under maximum likelihood and Markov chain Monte Carlo. Bioinformatics 33, 1630–1638. doi: 10.1093/bioinformatics/btx025
Kassambara, A. (2016). Package ‘factoextra’: Extract and Visualize the Result of Multivariate Data Analysis. Available online at: https://cran.r-project.org/web/packages/factoextra/index.html (accessed August 17, 2021).
Kassambara, A. (2020). ggpubr: ‘ggplot2’ Based Publication Ready Plots. Available online at: https://cran.r-project.org/package=ggpubr (accessed August 17, 2021).
Katoh, K., Misawa, K., Kuma, K. I., and Miyata, T. (2002). MAFFT: a novel method for rapid multiple sequence alignment based on fast Fourier transform. Nucleic Acids Res. 30, 3059–3066. doi: 10.1093/nar/gkf436
Kekkonen, M., and Hebert, P. D. N. (2014). DNA barcode-based delineation of putative species: efficient start for taxonomic workflows. Mol. Ecol. Resour. 14, 706–715. doi: 10.1111/1755-0998.12233
Kingston, T., Lara, M. C., Jones, G., Akbar, Z., Kunz, T. H., and Schneider, C. J. (2001). Acoustic divergence in two cryptic Hipposideros species: a role for social selection? Proc. R. Soc. London 268, 1381–1386. doi: 10.1098/rspb.2001.1630
Knowles, L. L., and Carstens, B. C. (2007). Delimiting species without monophyletic gene trees. Syst. Biol. 56, 887–895. doi: 10.1080/10635150701701091
Kumar, S., Stecher, G., Li, M., Knyaz, C., and Tamura, K. (2018). MEGA X: molecular evolutionary genetics analysis across computing platforms. Mol. Biol. Evol. 35, 1547–1549. doi: 10.1093/molbev/msy096
Latinne, A., Hu, B., Olival, K. J., Zhu, G., Zhang, L., Li, H., et al. (2020). Origin and cross-species transmission of bat coronaviruses in China. Nat. Commun. 11:4235. doi: 10.1038/s41467-020-17687-3
Lê, S., Josse, J., and Husson, F. (2008). FactoMineR: an R package for multivariate analysis. J. Stat. Softw. 25, 1–18.
Leaché, A. D., Zhu, T., Rannala, B., and Yang, Z. (2019). The spectre of too many species. Syst. Biol. 68, 168–181. doi: 10.1093/sysbio/syy051
Lin, G. N., Zhang, C., and Xu, D. (2011). Polytomy identification in microbial phylogenetic reconstruction. BMC Syst. Biol. 5, (Suppl. 3):S2. doi: 10.1186/1752-0509-5-S3-S2
Liu, T., Sun, K., Csorba, G., Zhang, K., Zhang, L., Zhao, H., et al. (2019). Species delimitation and evolutionary reconstruction within an integrative taxonomic framework: a case study on Rhinolophus macrotis complex (Chiroptera: Rhinolophidae). Mol. Phylogenet. Evol. 139:106544. doi: 10.1016/j.ympev.2019.106544
Liu, T., Zhang, K., Dai, W., Jin, L., Sun, K., and Feng, J. (2021). Evolutionary insights into Rhinolophus episcopus (Chiroptera, Rhinolophidae) in China: isolation by distance, environment, or sensory system? J. Zool. Syst. Evol. Res. 59, 294–310. doi: 10.1111/jzs.12394
Long, C., and Kubatko, L. (2018). The effect of gene flow on coalescent-based species-tree inference. Syst. Biol. 67, 770–785. doi: 10.1093/sysbio/syy020
Luo, A., Ling, C., Ho, S. Y. W., and Zhu, C. D. (2018). Comparison of methods for molecular species delimitation across a range of speciation scenarios. Syst. Biol. 67, 830–846. doi: 10.1093/sysbio/syy011
Mallo, D., and Posada, D. (2016). Multilocus inference of species trees and DNA barcoding. Philos. Trans. R. Soc. B Biol. Sci. 371:20150335. doi: 10.1098/rstb.2015.0335
Mao, X. G., and Rossiter, S. J. (2020). Genome-wide data reveal discordant mitonuclear introgression in the intermediate horseshoe bat (Rhinolophus affinis). Mol. Phylogenet. Evol. 150:106886. doi: 10.1016/j.ympev.2020.106886
Mao, X. G., Zhu, G. J., Zhang, S., and Rossiter, S. J. (2010). Pleistocene climatic cycling drives intra-specific diversification in the intermediate horseshoe bat (Rhinolophus affinis) in Southern China. Mol. Ecol. 19, 2754–2769. doi: 10.1111/j.1365-294X.2010.04704.x
Mao, X., Tsagkogeorga, G., Thong, V. D., and Rossiter, S. J. (2019). Resolving evolutionary relationships among six closely related taxa of the horseshoe bats (Rhinolophus) with targeted resequencing data. Mol. Phylogenet. Evol. 139:106551. doi: 10.1016/j.ympev.2019.106551
Minh, B. Q., Nguyen, M. A. T., and Von Haeseler, A. (2013). Ultrafast approximation for phylogenetic bootstrap. Mol. Biol. Evol. 30, 1188–1195. doi: 10.1093/molbev/mst024
Murtagh, F., and Legendre, P. (2014). Ward’s hierarchichal agglomerative clustering method: which algorithms implement ward’s criterion. J. Classif. 31, 274–295. doi: 10.1007/s00357-014-9161-z
Nabholz, B., Glemin, S., and Galtier, N. (2007). Strong variations of mitochondrial mutation rate across mammals–the longevity hypothesis. Mol. Biol. Evol. 25, 120–130. doi: 10.1093/molbev/msm248
Nabholz, B., Glémin, S., and Galtier, N. (2009). The erratic mitochondrial clock: Variations of mutation rate, not population size, affect mtDNA diversity across birds and mammals. BMC Evol. Biol. 9:54. doi: 10.1186/1471-2148-9-54
Neuweiler, G. (1990). Auditory adaptations for prey capture in echolocating. Physiol. Rev. 70, 615–641. doi: 10.1152/physrev.1990.70.3.615
Neuwirth, E., and Maindonald, J. (2014). Package ‘RColorBrewer’: ColorBrewer Palettes. Available online at: http://www.colorbrewer.org (accessed August 17, 2021).
Nguyen, L. T., Schmidt, H. A., Von Haeseler, A., and Minh, B. Q. (2015). IQ-TREE: a fast and effective stochastic algorithm for estimating maximum-likelihood phylogenies. Mol. Biol. Evol. 32, 268–274. doi: 10.1093/molbev/msu300
Padial, J. M., Miralles, A., De la Riva, I., and Vences, M. (2010). The integrative future of taxonomy. Front. Zool. 7:16. doi: 10.1186/1742-9994-7-16
Patrick, L. E., Mcculloch, E. S., and Ruedas, L. A. (2013). Systematics and biogeography of the arcuate horseshoe bat species complex (Chiroptera, Rhinolophidae). Zool. Scr. 42, 553–590. doi: 10.1111/zsc.12026
Pavey, S. A., Collin, H., Nosil, P., and Rogers, S. M. (2010). The role of gene expression in ecological speciation. Ann. N.Y. Acad. Sci. 1206, 110–129. doi: 10.1111/j.1749-6632.2010.05765.x
Rambaut, A. (2016). Figtree.v.1.4.4. Edinburgh: Institute of Evolutionary Biology, University of Edinburgh.
Posada, D., and Crandall, K. A. (1998). ModelTest: testing the model of DNA substitution. Bioinformatics 14, 817–818. doi: 10.1093/bioinformatics/14.9.817
Ratnasingham, S., and Hebert, P. D. N. (2007). BOLD: the barcode of life data system: barcoding. Mol. Ecol. Notes 7, 355–364. doi: 10.1111/j.1471-8286.2007.01678.x
Reijniers, J., Vanderelst, D., and Peremans, H. (2010). Morphology-induced information transfer in bat sonar. Phys. Rev. Lett. 105:148701. doi: 10.1103/PhysRevLett.105.148701
Ronquist, F., and Huelsenbeck, J. P. (2003). MrBayes 3: bayesian phylogenetic inference under mixed models. Bioinformatics 19, 1572–1574. doi: 10.1093/bioinformatics/btg180
Roux, C., Fraïsse, C., Romiguier, J., Anciaux, Y., Galtier, N., and Bierne, N. (2016). Shedding light on the grey zone of speciation along a continuum of genomic divergence. PLoS Biol. 14:e2000234. doi: 10.1371/journal.pbio.2000234
Salicini, I., Ibáñez, C., and Juste, J. (2011). Multilocus phylogeny and species delimitation within the Natterer’s bat species complex in the Western Palearctic. Mol. Phylogenet. Evol. 61, 888–898. doi: 10.1016/j.ympev.2011.08.010
Schuller, G., and Pollak, G. (1979). Disproportionate frequency representation in the inferior colliculus of Doppler-compensating greater horseshoe bats: evidence for an acoustic fovea. J. Comp. Physiol. 132, 47–54. doi: 10.1007/BF00617731
Schumer, M., Cui, R., Rosenthal, G. G., and Andolfatto, P. (2015). Reproductive isolation of hybrid populations driven by genetic incompatibilities. PLoS Genet. 11:e1005041. doi: 10.1371/journal.pgen.1005041
Sedlock, J. L., and Weyandt, S. E. (2009). Genetic divergence between morphologically and acoustically cryptic bats: novel niche partitioning or recent contact? J. Zool. 279, 388–395. doi: 10.1111/j.1469-7998.2009.00634.x
Simmons, N. B., and Cirranello, A. L. (2021). Bat Species of the World: A Taxonomic and Geographic Database. Available online at: https://batnames.org (accessed September 14, 2021).
Simmons, N. B., and Voss, R. S. (2009). “Collection, preparation, and fixation of specimens and tissues,” in Ecological and Behavioural Methods for the Study of Bats, 2nd Edn, eds T. H. Kunz and S. Parson (Baltimore, MD: John Hopkins University Press).
Smith, M. L., and Carstens, B. C. (2020). Process-based species delimitation leads to identification of more biologically relevant species*. Evolution 74, 216–229. doi: 10.1111/evo.13878
Soisook, P., Struebig, M. J., Noerfahmy, S., Bernard, H., Maryanto, I., Chen, S. F., et al. (2015). Description of a new species of the Rhinolophus trifoliatus-group (Chiroptera: Rhinolophidae) from Southeast Asia. Acta Chiropterol. 17, 21–36. doi: 10.3161/15081109ACC2015.17.1.002
Srinivasulu, C., Srinivasulu, A., Srinivasulu, B., and Jones, G. (2019). Integrated approaches to identifying cryptic bat species in areas of high endemism: the case of Rhinolophus andamanensis in the Andaman Islands. PLoS One 14:e0213562. doi: 10.1371/journal.pone.0213562
Stoffberg, S., Jacobs, D. S., and Matthee, C. A. (2011). The divergence of echolocation frequency in horseshoe bats: moth hearing, body size or habitat? J. Mamm. Evol. 18, 117–129. doi: 10.1007/s10914-011-9158-x
Stoffberg, S., Jacobs, D. S., Mackie, I. J., and Matthee, C. A. (2010). Molecular phylogenetics and historical biogeography of Rhinolophus bats. Mol. Phylogenet. Evol. 54, 1–9. doi: 10.1016/j.ympev.2009.09.021
Struck, T. H., Feder, J. L., Bendiksby, M., Birkeland, S., Cerca, J., Gusarov, V. I., et al. (2018). Finding evolutionary processes hidden in cryptic species. Trends Ecol. Evol. 33, 153–163. doi: 10.1016/j.tree.2017.11.007
Stuart, B. L., Inger, R. F., and Voris, H. K. (2006). High level of cryptic species diversity revealed by sympatric lineages of Southeast Asian forest frogs. Biol. Lett. 2, 470–474. doi: 10.1098/rsbl.2006.0505
Sukumaran, J., and Knowles, L. L. (2017). Multispecies coalescent delimits structure, not species. Proc. Natl. Acad. Sci. U.S.A. 114, 1607–1611. doi: 10.1073/pnas.1607921114
Taylor, P. J., MacDonald, A., Goodman, S. M., Kearney, T., Cotterill, F. P. D., Stoffberg, S., et al. (2018). Integrative taxonomy resolves three new cryptic species of small southern African horseshoe bats (Rhinolophus). Zool. J. Linn. Soc. 184, 1249–1276. doi: 10.1093/zoolinnean/zlz030
Tu, V. T., Hassanin, A., Görföl, T., Arai, S., Fukui, D., Thanh, H. T., et al. (2017). Integrative taxonomy of the Rhinolophus macrotis complex (Chiroptera, Rhinolophidae) in Vietnam and nearby regions. J. Zool. Syst. Evol. Res. 55, 177–198. doi: 10.1111/jzs.12169
Vanderelst, D., de Mey, F., Peremans, H., Geipel, I., Kalko, E., and Firzlaff, U. (2010). What noseleaves do for FM bats depends on their degree of sensorial specialization. PLoS One 5:e11893. doi: 10.1371/journal.pone.0011893
Vanderelst, D., Lee, Y. F., Geipel, I., Kalko, E. K. V., Kuo, Y. M., and Peremans, H. (2013). The noseleaf of Rhinolophus formosae focuses the frequency modulated (FM) component of the calls. Front. Physiol. 4:191. doi: 10.3389/fphys.2013.00191
Vanderelst, D., Reijniers, J., Steckel, J., and Peremans, H. (2011). Information generated by the moving pinnae of Rhinolophus rouxi: tuning of the morphology at different harmonics. PLoS One 6:e20627. doi: 10.1371/journal.pone.0020627
Vaughan, N., Jones, G., and Harris, S. (1997). Identification of Brithis bat species by multivariate analysis of echolocation call parameter. Bioacoustics 7, 189–207. doi: 10.1080/09524622.1997.9753331
Vogler, A. P., and Monaghan, M. T. (2007). Recent advances in DNA taxonomy. J. Zool. Syst. Evol. Res. 45, 1–10. doi: 10.1111/j.1439-0469.2006.00384.x
Will, K. W., Mishler, B. D., and Wheeler, Q. D. (2005). The perils of DNA barcoding and the need for integrative taxonomy. Syst. Biol. 54, 844–851. doi: 10.1080/10635150500354878
Wortley, A. H., and Scotland, R. W. (2006). The effect of combining molecular and morphological data in published phylogenetic analyses. Syst. Biol. 55, 677–685. doi: 10.1080/10635150600899798
Xiao, N., and Li, M. (2018). ggsci: Scientific Journal and Sci-Fi Themed Color Palettes for ‘ggplot2’. Available online at: https://cran.r-project.org/package=ggsci (accessed August 17, 2021).
Yang, Z. (2015). The BPP program for species tree estimation and species delimitation. Curr. Zool. 61, 854–865. doi: 10.1093/czoolo/61.5.854
Yang, Z., and Rannala, B. (2010). Bayesian species delimitation using multilocus sequence data. Proc. Natl. Acad. Sci. U.S.A. 107, 9264–9269. doi: 10.1073/pnas.0913022107
Yang, Z., and Rannala, B. (2014). Unguided species delimitation using DNA sequence data from multiple loci. Mol. Biol. Evol. 31, 3125–3135. doi: 10.1093/molbev/msu279
Yang, Z., and Rannala, B. (2017). Bayesian species identification under the multispecies coalescent provides significant improvements to DNA barcoding analyses. Mol. Ecol. 26, 3028–3036. doi: 10.1111/mec.14093
Zhang, J., Kapli, P., Pavlidis, P., and Stamatakis, A. (2013). A general species delimitation method with applications to phylogenetic placements. Bioinformatics 29, 2869–2876. doi: 10.1093/bioinformatics/btt499
Zhang, L., Sun, K., Csorba, G., Hughes, A. C., Jin, L., Xiao, Y., et al. (2021). Complete mitochondrial genomes reveal robust phylogenetic signals and evidence of positive selection in horseshoe bats. BMC Ecol. Evol. 21:199. doi: 10.1186/s12862-021-01926-2
Zhang, L., Sun, K., Liu, T., Zhao, H., Csorba, G., Jin, L., et al. (2018). Multilocus phylogeny and species delimitation within the philippinensis group (Chiroptera: Rhinolophidae). Zool. Scr. 47, 655–672. doi: 10.1111/zsc.12308
Zhang, Z., Truong, S. N., and Müller, R. (2009). Acoustic effects accurately predict an extreme case of biological morphology. Phys. Rev. Lett. 103:038701. doi: 10.1103/PhysRevLett.103.038701
Zhou, H., Chen, X., Hu, T., Li, J., Song, H., Liu, Y., et al. (2020). A novel bat coronavirus closely related to SARS – CoV – 2 contains natural insertions at the S1/S2 cleavage site of the spike protein. Curr. Biol. 30, 2196– 2203.e3.
Zhou, H., Ji, J., Chen, X., Bi, Y., Li, J., Hu, T., et al. (2021). Identification of novel bat coronaviruses sheds light on the evolutionary origins of SARS-CoV-2 and related viruses. Cell 184, 4380–4391.e14. doi: 10.1016/j.cell.2021.06.008
Zhou, Z. M., Guillén-Servent, A., Lim, B. K., Eger, J. L., Wang, Y. X., and Jiang, X. L. (2009). A new species from Southwestern China in the Afro-Palearctic lineage of the horseshoe bats (Rhinolophus). J. Mammal 90, 57–73. doi: 10.1644/08-MAMM-A-048.1
Keywords: acoustic, integrative taxonomy, morphological disparities, noseleaf, phylogenetic, sella
Citation: Chornelia A, Lu J and Hughes AC (2022) How to Accurately Delineate Morphologically Conserved Taxa and Diagnose Their Phenotypic Disparities: Species Delimitation in Cryptic Rhinolophidae (Chiroptera). Front. Ecol. Evol. 10:854509. doi: 10.3389/fevo.2022.854509
Received: 14 January 2022; Accepted: 28 February 2022;
Published: 29 March 2022.
Edited by:
Diego Baldo, CONICET Institute of Subtropical Biology (IBS), ArgentinaReviewed by:
Terrence C. Demos, Field Museum of Natural History, United StatesCopyright © 2022 Chornelia, Lu and Hughes. This is an open-access article distributed under the terms of the Creative Commons Attribution License (CC BY). The use, distribution or reproduction in other forums is permitted, provided the original author(s) and the copyright owner(s) are credited and that the original publication in this journal is cited, in accordance with accepted academic practice. No use, distribution or reproduction is permitted which does not comply with these terms.
*Correspondence: Alice Catherine Hughes, YWNoX2NvbnNlcnZhdGlvbjJAaG90bWFpbC5jb20=
Disclaimer: All claims expressed in this article are solely those of the authors and do not necessarily represent those of their affiliated organizations, or those of the publisher, the editors and the reviewers. Any product that may be evaluated in this article or claim that may be made by its manufacturer is not guaranteed or endorsed by the publisher.
Research integrity at Frontiers
Learn more about the work of our research integrity team to safeguard the quality of each article we publish.