- Center for Macroecology, Evolution and Climate, Globe Institute, University of Copenhagen, Copenhagen, Denmark
Billions of birds undertake long-distance migration and the complexity of schedules has only recently become clear. Such movements occur as a response to seasonality but the ultimate drivers of these changing distributions remain difficult to study directly. Modeling seasonal distributions based fundamentally on climate and vegetation without parameterizing with empirical data, we focus on the potential role of ambient temperature and available resources in shaping the migratory program. We simulate the complete annual cycle over the Afro-Palearctic region in a round-trip migration model allowing full variation in the extent and timing of movement, and multiple stopovers. The resultant simulated tracks and associated environmental metrics are interrogated: we evaluate the thermal and resource consequences of staying in Europe versus crossing the Sahara, and secondly identify the movement patterns optimizing exposure to green vegetation and local surpluses. There is a distinct thermal gain from crossing the Sahara and the pattern emerging of optimal seasonal vegetation resembles contemporary migration routes regarding Sahara crossing, loop structure and itinerancy. Thus, our first-principle simulations suggest that variations in migration patterns among species are caused by a complex trade-off between risks and rewards of staying versus moving, including innate physiological constraints and the resultant gain of the high-risk Sahara crossing.
Introduction
Migration enables birds to utilize habitats separated by thousands of kilometers (Alerstam et al., 2003; Somveille et al., 2015). Often migrants overfly otherwise ecologically inhospitable regions such as oceans, deserts and mountain ranges (Alerstam and Hedenström, 1998; Backman et al., 2017). Recent tracking studies have revealed a diversity and complexity of movements among species (Jonzén et al., 2011; McKinnon et al., 2013; Kays et al., 2015). While some populations use single breeding and non-breeding sites (Kristensen et al., 2013), others use multiple, discrete sites across the non-breeding period (Heckscher et al., 2011; Stach et al., 2012; Arlt et al., 2015; Hewson et al., 2016; Jacobsen et al., 2017), and loop migrations are common (Klaassen et al., 2010; McKinnon et al., 2013; Willemoes et al., 2014).
Understanding the drivers of migration has attracted substantial interest from theoretical and quantitative biology (Berthold, 1996; Alerstam and Hedenström, 1998; Shaw, 2016). Requirements during breeding are generally specific and differ to those of other key life history events, necessitating a potentially abrupt switch in desirable geographical regions (Alerstam et al., 2003). Furthermore, migration enables individuals to exploit the seasonal variation in the environment (Newton, 2010) but it is not clear how this is optimally solved given environmental and physiological constraints. There is direct evidence that seabirds take advantage of multiple areas in the non-breeding period to exploit Net Primary Productivity (Block et al., 2011) and raptors and geese track resource abundance (e.g., Trierweiler et al. (2013), Shariatinajafabadi et al. (2014)) but this is less clear in small insectivorous songbirds (Heckscher et al., 2011; Tøttrup et al., 2012b; Kristensen et al., 2013; Lemke et al., 2013; Renfrew et al., 2013; Willemoes et al., 2014; Bridge et al., 2016; Gersten and Hahn, 2016; Thorup et al., 2017).
Balancing potential gains in resources, migration incurs specific costs (Alerstam and Lindström, 1990; Ramenofsky and Wingfield, 2007). However, staying in the same area may also be constrained by seasonal physiologically-limiting conditions such as ambient temperature (Scanes, 2015). Even small songbirds can persist in extreme low temperatures under responsive endothermy, for example through supplementary thermogenesis (Irving and Krog, 1954; Calder, 1974; Eppley, 2008) which entails increased maintenance costs (Swanson, 2010); furthermore, adaptive traits enhance the ability to cope with low temperatures but the constraints of these is poorly known (Swanson and Vézina, 2015; Blix, 2016). Physiological adaptations to the thermal environment have been identified across the taxa (Swanson and Vézina, 2015), for example, birds that experience lower winter temperatures also have more insulation in the form of body feather mass (Osváth et al., 2018). Temperature itself has been proposed to be involved in driving the distributions of avian and mammalian species (Khaliq et al., 2014). We propose that obligate Afro-Palearctic migration could evolve at least partially as an adaptation to avoid the risk of low ambient temperatures while optimizing resource abundance.
Long-distance intra- and inter-continental flights invariably involve negotiating ecological barriers (Alerstam and Lindström, 1990). In addition to carrying an anticipated physiological cost of obligate endurance flight, ecological barriers are presumably limiting for some individuals, particularly in combination with adverse and unpredictable weather (Gill and Hays, 2015; Loonstra et al., 2019). Crossing deserts, seas and long-distance flights have been shown to account for the greatest mortality in migrating birds (Sillett and Holmes, 2002; Strandberg et al., 2010; Blackburn and Cresswell, 2016; Paxton et al., 2017; Senner et al., 2019; Snell and Thorup, 2019). However, tracking studies have revealed a remarkable capacity for even small songbirds to undertake extensive non-stop endurance flights (DeLuca et al., 2015; Snell et al., 2018). Overall, the risks and physiological costs of long-distance migration must be offset by the resultant conditions, and potentially those tracks that do not stop in the desert are rewarded with improved seasonal resource availability associated with vegetation conditions in sub-Saharan Africa.
While physiological constraints are easily quantified, quantifying resource availability is more challenging. NDVI (Normalized Difference Vegetation Index), a measure of vegetation greenness, has been used extensively as a proxy for food availability over ephemeral or stochastic variation in temperature or precipitation, for both herbivorous and insectivorous avian species (Pettorelli et al., 2011). Thorup et al. (2017) demonstrated that migration schedules were overall related to seasonal accumulation of vegetation greenness (NDVI) and to spatially local peaks (Surplus NDVI) in three species of long-distance insectivorous avian migrants. Primary productivity, a measure of net energy gain through, principally, the photosynthesis of green plants, has garnered much interest in biogeography studies, however to date, these typically have used a proxy (e.g., chlorophyll a or EVI: Block et al. (2011); La Sorte et al. (2014), Bridge et al. (2016)). These three metrics are distinct in their magnitudes both between resources and their seasonal variation. The temporal resolution of these, and global meteorological products, enables examination of the seasonal variation of ecological and climatic parameters in which predictable changes throughout the annual cycle are thought to underlie the seasonal movements of species (Kemp et al., 2012; Lisovski et al., 2017).
Migration routes result from a variety of factors acting in concert through the annual cycle across a seasonally changing landscape. Migrants need to balance the energetic demands of a number of life-history stages such as breeding, molting and migration while at the same time escaping predation and parasites. Our aim here is to explore the optimal strategies with regard to geographical constraints, thermal environment and resource availability. We investigate the spatiotemporal characteristics of simple, fundamental simulated migration tracks across a real-word landscape and explore the migration characteristics and patterns of environmental metrics encountered. Such bottom-up approaches in modeling emerging patterns (Connolly et al., 2017) holds great promise for identifying the underlying drivers of the evolution of complex migration routes. Because of the trade-offs birds have to balance and our simplified description of birds’ environments, we do not expect birds to strictly follow the identified individual routes but instead we focus on overall qualitative agreement of strategies. Fundamental simulations have been employed to investigate potential drivers between single breeding and wintering sites (e.g., Somveille et al., 2018; Somveille et al., 2019) but here we aim to simulate the full annual cycle (Bridge et al., 2016; Stutchbury et al., 2016; Thorup et al., 2017; Shaw, 2020) and account for the complex spatio-temporal strategies that have been revealed recently including multiple wintering sites and variation in timings of flights.
We investigate the potential factors underlying the conserved patterns in populations’ seasonally changing distribution in the Afro-Palearctic region in silico. We modeled the spatiotemporal regimes of a complete annual cycle of small migratory land-birds, with realistic constraints based on empirical patterns Thorup et al. (2017). We simulated individual bird tracks across the Palearctic region and Africa, incorporating the complexity of multiple non-breeding sites in a round-trip model generating individuals’ locations from breeding, throughout wintering and back.
For the randomly generated simulated tracks, we first focus on evaluating the thermal (minimum temperature encountered) and resource (vegetation) consequences for bird tracks staying in Europe versus those crossing the Sahara. We then investigate in detail the locations in space and time that allow migrants to optimize resources across the annual cycle (based on the amount of green vegetation as well as surplus in green vegetation experienced over the year), by mapping the spatiotemporal characteristics of the tracks with the highest resources encountered. Furthermore, from exploring simulations testable hypotheses concerning the drivers of migration patterns can be generated.
Materials and Methods
Round-Trip Migration Simulations
Individual tracks were simulated as obligate round-trip migrations starting in the breeding area and ranging from Europe to the south of Africa. We restricted the extremely high number of possible annual spatiotemporal schedules to within realistic constraints of space and strategies. Thus, during migration from the breeding area only southward steps were allowed and during return migration only northward steps. Within each simulation of an annual cycle, a southern-most latitude was pre-defined and the out- and return-migrations were accomplished with a random number of migratory steps (two to eight) occurring at a random time intervals.
We generated the simulated tracks in R (R Core Team, 2017) restricting movement specifically within the Afro-Palearctic region (35°S to 55°N, 17°W to 57°E) and incorporating a stationary breeding season defined arbitrarily as eastern Denmark (55°N, 13°E) for the two months of June and July. The annual cycle was divided into 24 biweekly periods and the geographic area was gridded into cells of 2° latitude and longitude. Southern extents were distributed at 10° latitude intervals, resulting in the maximum number of latitudes crossed from 20° to 180° (summing both south and northbound movements). Individuals have free longitudinal scope and cells that are exclusively water, sand or ice filled are excluded. The number of steps (and therefore resultant non-breeding stopover sites) was pre-specified for each individual bird and ranged from a minimum of two up to 20 with unrestricted temporal movements during the non-breeding period; the model was run with sufficient iterations to represent the infinite possibilities of spatial and temporal movements available, while remaining within the boundaries of observed patterns for migratory passerines (constrained breeding dates, southwards followed but northwards route, duration of stopovers). We ran the model 100 times for each possible southern extent and all possible numbers of steps, generating periodic and geographic cell information for 11692 valid random round-trip migrations. Supplementary Figure 1 illustrates the simulation procedure and Supplementary Figure 2 illustrates examples of the simulation output.
Biotic and Abiotic Environmental Data
Remote-sensed environmental data was obtained for the years 2008 to 2015, where all products were available in a consistent format, and aggregated to determine expected values. Surface temperature and precipitable water were obtained from NCEP FNL re-analysis product (National Centers for Environmental Prediction/National Weather Service/NOAA/U.S. Department of Commerce, 2000; Kanamitsu et al., 2002). Data are available at 1° spatial resolution and 6 hourly temporal resolution. Data were aggregated to the same resolution using MATLAB, in three dimensions, i.e., the values for each 2° by 2°cell and biweekly temporal period, across all years, for absolute minimum temperature (°C) and mean precipitable water (kg m–2).
Normalized difference vegetation index is a spectrographic measure of chlorophyll and is proportional to canopy leaf density (Tucker, 1979); various metrics of NDVI have been demonstrated to be associated with migratory patterns of insectivores. NDVI was extracted from the MODIS satellite images with a resolution of 0.05° from a 16-day period (MOD13C1; Justice et al. (1998), MODIS (2016)), and Net Primary Productivity (NPP; gC m–2 day–1) was extracted at 0.1° for 8-day intervals (MOD17; Running et al. (2015), MODIS (2017)). Data were aggregated (areas with NDVI values commensurate with water, sand or ice are excluded to allow individual birds to use model cells that contain physical or biological islands) using the “raster” package in R (Hijmans, 2016; R Core Team, 2017). For each cell we calculated absolute NDVI and NPP as mean value in three dimensions (latitude, longitude and temporal period). Surplus NDVI is a metric of the ratio of mean NDVI for each temporal period for all years relative to the mean annual value for that cell (Thorup et al., 2017).
In order to minimize the number of possibilities for the model run, we used 10° intervals as the southern extent rather than each 2°cell of latitude, as such we inspected the latitudinal gradient of variables of interest to ensure that intermediate steps could be appropriately represented with the spatial resolution designated.
Evaluating the Sahara Crossing
To test the environmental parameters in relation to overflying a substantial ecological barrier crossing, we defined the Sahara Desert and Mediterranean Sea together as simply the latitudes between 15° and 33°N which correspond closely to less than the isohyet of 100 mm and an NDVI of consistently less than 0.2 (classed as non-vegetated). The number of generated tracks with a southern extent south of the Sahara that did not spend any periods in cells within the Sahara region were 1,220 (10.4%; excluded tracks include the 25% of all tracks where the southern extent was defined as within the desert region). The total number of tracks that did not spend any periods within the Sahara region (including those with a southern extent in Europe) was 2,520 (21.6%).
Where there was clear distinction in the overall values of abiotic variables experienced between tracks staying in Europe compared to those with a greater southern extent, we partitioned tracks according to this metric to investigate the conditions and consequences experienced temporally. For minimum temperature (Figures 1A,B) we partitioned tracks conservatively at −7°C (a threshold for no quantile overlap between tracks remaining in Europe compared to those crossing the Sahara) to investigate properties of tracks that maintained relatively warmer conditions. A similar pattern was observed for precipitable water, where tracks with a southern extent in Africa encountered 20.7% greater mean precipitation than those remaining at altitudes above 5°N. Again, there was no overlap in quartiles between the two regions. Therefore, to control for the interaction with precipitation (17.8% greater precipitation was associated with warm tracks), we partitioned tracks at 18 kg m–2. Over the course of the annual cycle, tracks grouped by mean precipitation profiles did not return periods without quartile overlap; there was also substantial overlap in all resource metrics between the high and low precipitation groups.
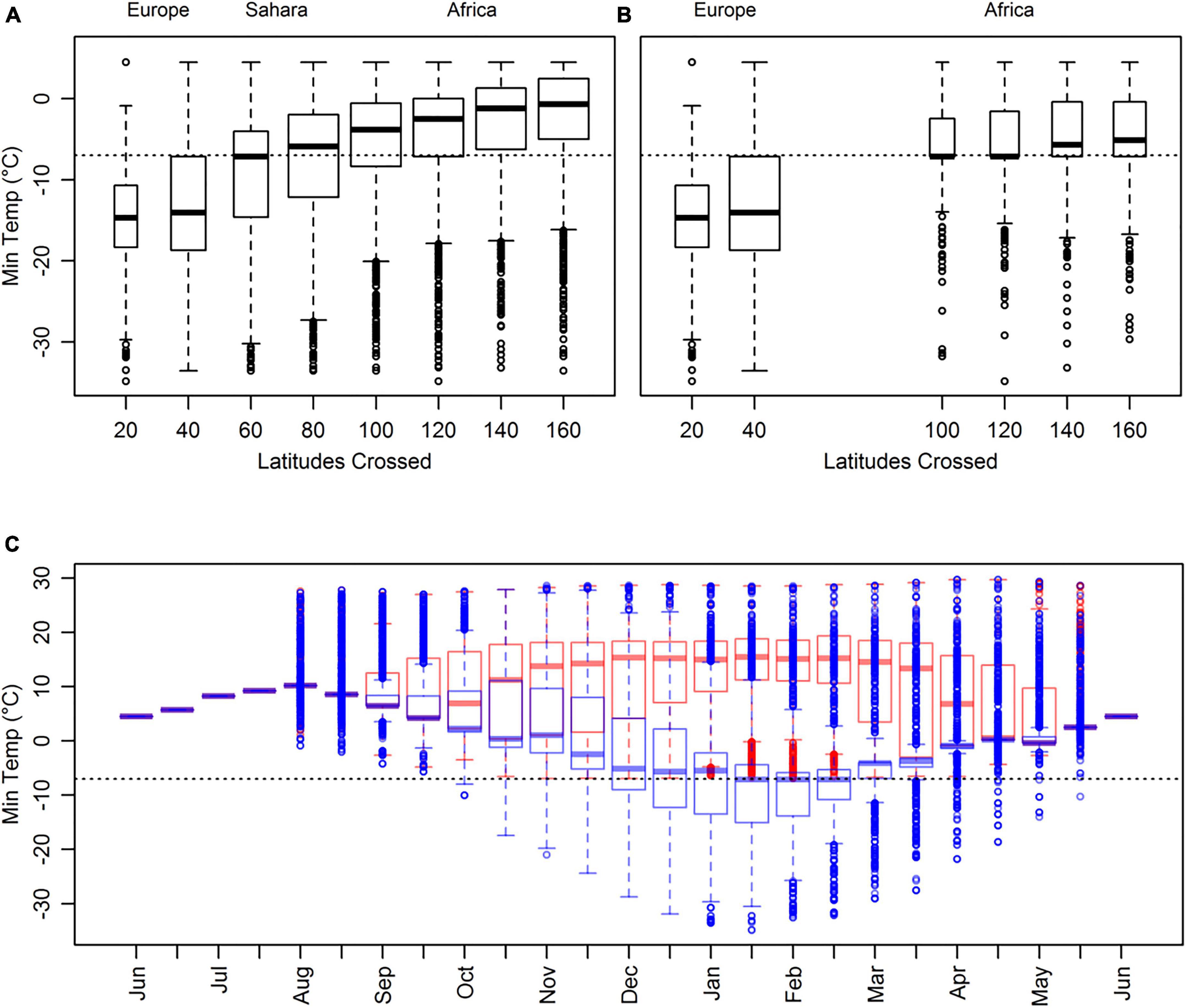
Figure 1. Absolute minimum temperature (°C) encountered over the complete round-trip for simulated tracks grouped by the total number of latitudes crossed for (A) all tracks n = 11692 and (B) only tracks that do not use the Sahara region n = 2520. The dotted line indicates the −7°C threshold used to partition tracks more likely to cross the Sahara Region from those in Europe. Latitudes crossed are the accumulated latitudes from the breeding site to the breeding site, i.e., a track crossing 20 latitudes had a southern extent 10° south of the breeding site. (C) Minimum temperature (°C) for tracks that did not use the Sahara region, for each temporal period. Tracks are partitioned by minimum temperature experienced: tracks maintaining temperatures above –7°C (n = 798) in red and those with at least one period with temperatures below this in blue (n = 1722). Whiskers represent the 95% confidence interval and outliers are shown by dots. Variable widths of boxplots represent the sample size.
Optimizing Seasonal Vegetation
Thorup et al. (2017) reported that tracked long-distance avian migrants experienced overall NDVI (common cuckoos Cuculus canorus) and Surplus NDVI (thrush nightingales Luscinia luscinia and red-backed shrikes Lanius collurio) within the upper 5% of simulated tracks with a representative Southern Extent. Mean overall values were 0.610 NDVI in cuckoos and 1.180 Surplus NDVI in red-backed shrike (lower than thrush nightingale for the same latitudinal range, hence used in this study). The top 5% performing simulated track data for all southern extents is equivalent to 0.619 and 1.182, respectively. As such, we conservatively used the values presented for the tracked birds which represent 6.3% and 5.4% of all simulated tracks from our model run.
Results
The minimum temperatures experienced were lowest for tracks with southern limits in Europe (North of 33°N), and higher for those that travelled into Africa (Figures 1A,B). Tracks remaining in Europe experienced a lower median minimum temperature of −14.4°C compared to the −6.2°C for the tracks with southern extents in sub-Saharan Africa that did not stop in the desert (Figure 1B; no overlap of upper- and lower-quartiles, respectively). A minimum temperature of −7.2°C separated the upper quartile of tracks remaining in Europe and the lower quartile of tracks extending into sub-Saharan Africa (Figure 1B). Tracks that maintained temperatures above −7°C experienced the highest minimum temperatures from December to end-March (Figure 1C) and improved vegetation conditions for the same period (Supplementary Figure 3).
Tracks with a southern extent south of 40°N experienced very low minimum NDVI (Figure 2A). For tracks crossing the desert non-stop, minimum NDVI increased (Figure 2B). The highest NDVI was experienced during breeding (Figure 2C). As the season progressed there was overlap in resources experienced for all tracks that did not stop within the desert region, regardless of whether they continued into the African subcontinent or remained in Europe (Figure 2C), although for half the non-breeding periods median values were greater than those of tracks remaining north of 35°N.
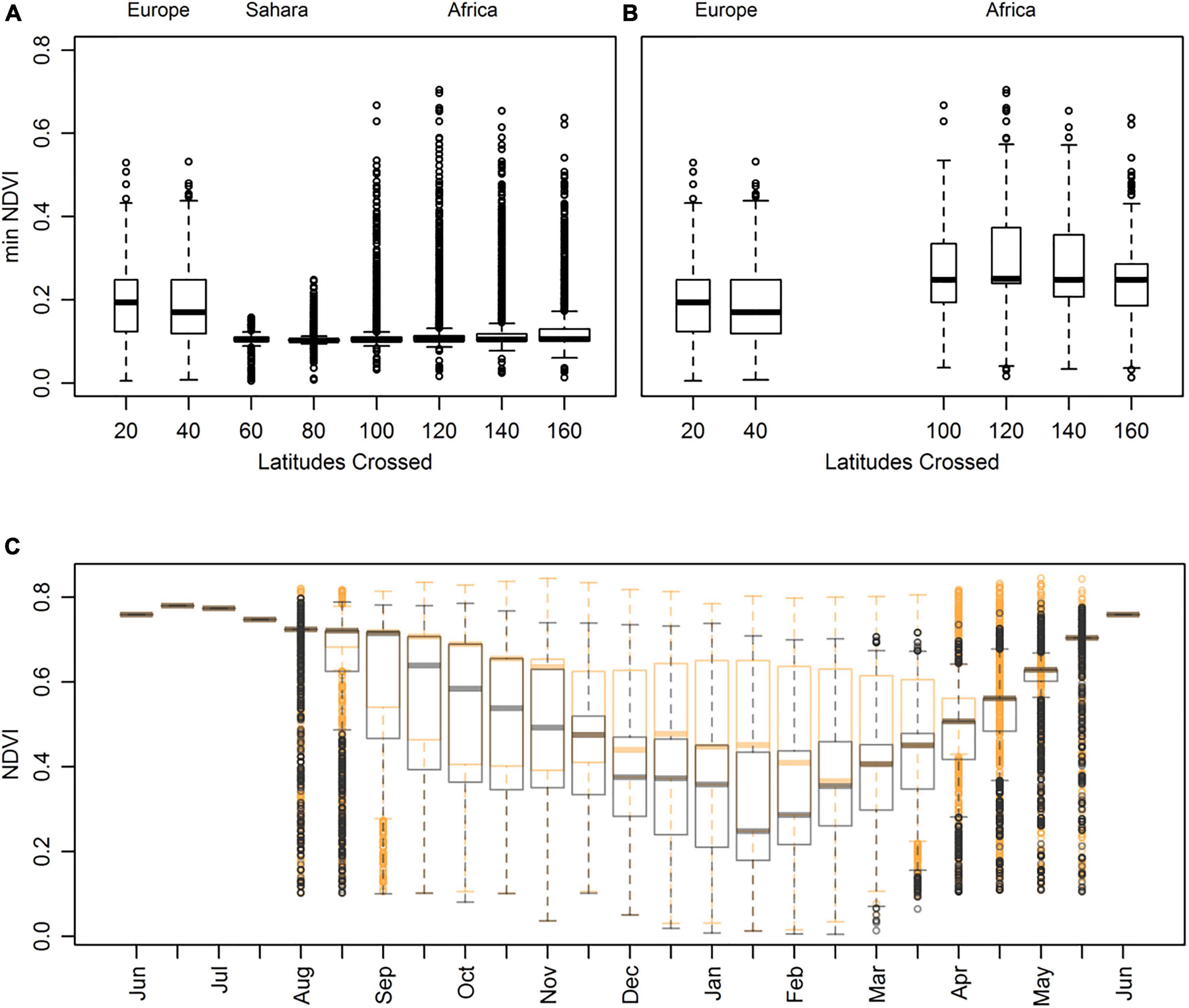
Figure 2. Absolute minimum NDVI encountered over the complete round-trip for simulated tracks grouped by the total number of latitudes crossed for (A) all tracks n = 11692 and (B) only tracks that do not use the Sahara region n = 2520. Latitudes crossed are the accumulated latitudes from the breeding site to the breeding site, i.e., a track crossing 20 latitudes had a southern extent 10° south of the breeding site. Median mean NDVI for all tracks south of 40°N was 0.06 lower than tracks in Europe. (C) NDVI for tracks that did not use the Sahara region, for each temporal period. Sampled cells within Europe generally return decreasing resource values (NDVI, NPP and Surplus NDVI) with latitude (median values of 0.72, 3.39 gC m–2 day–1 & 1.27 at the breeding site respectively and 0.39, 1.07 gC m–2 day–1 & 1.06 respectively at 35°N). Latitudes with highest values NDVI and NPP during migration were located at cells between 15°S to 5°N and 15°S to 1°N in central Africa (between 0.60-0.72 and 1.61-2.32 gC m–2 day–1, respectively). Tracks are partitioned by those with a southern extent in sub-Saharan Africa in orange (n = 1220) and those that remained ≥ 35°N in grey (n = 1300). The boxplots represent the median value and upper and lower quartiles. Whiskers represent the 95% confidence interval and outliers are shown by dots.
Simulated tracks with high overall NDVI were concentrated in the tropical rainforest of Central Africa (most time spent; Figures 3Ai,ii). Only a small proportion of tracks had a southern extent within the Sahara or Sahel zones (1.5%) or remained north of the desert (11.3%; Figure 3Aii). Tracks with high Surplus NDVI were more widely distributed, with main utilization areas across the Mediterranean and the northernmost region of South Africa (tropical shrub-land to moist deciduous forest; Figures 3Bi,ii) and had a tendency to extend further south than tracks with high NDVI. For each southern extent, there was little overlap in resource metrics encountered between high performance NDVI tracks and high performance Surplus NDVI (Supplementary Figures 4, 5). The greatest differences occurred for tracks reaching the Sahara and Sahel region (Supplementary Figure 4).
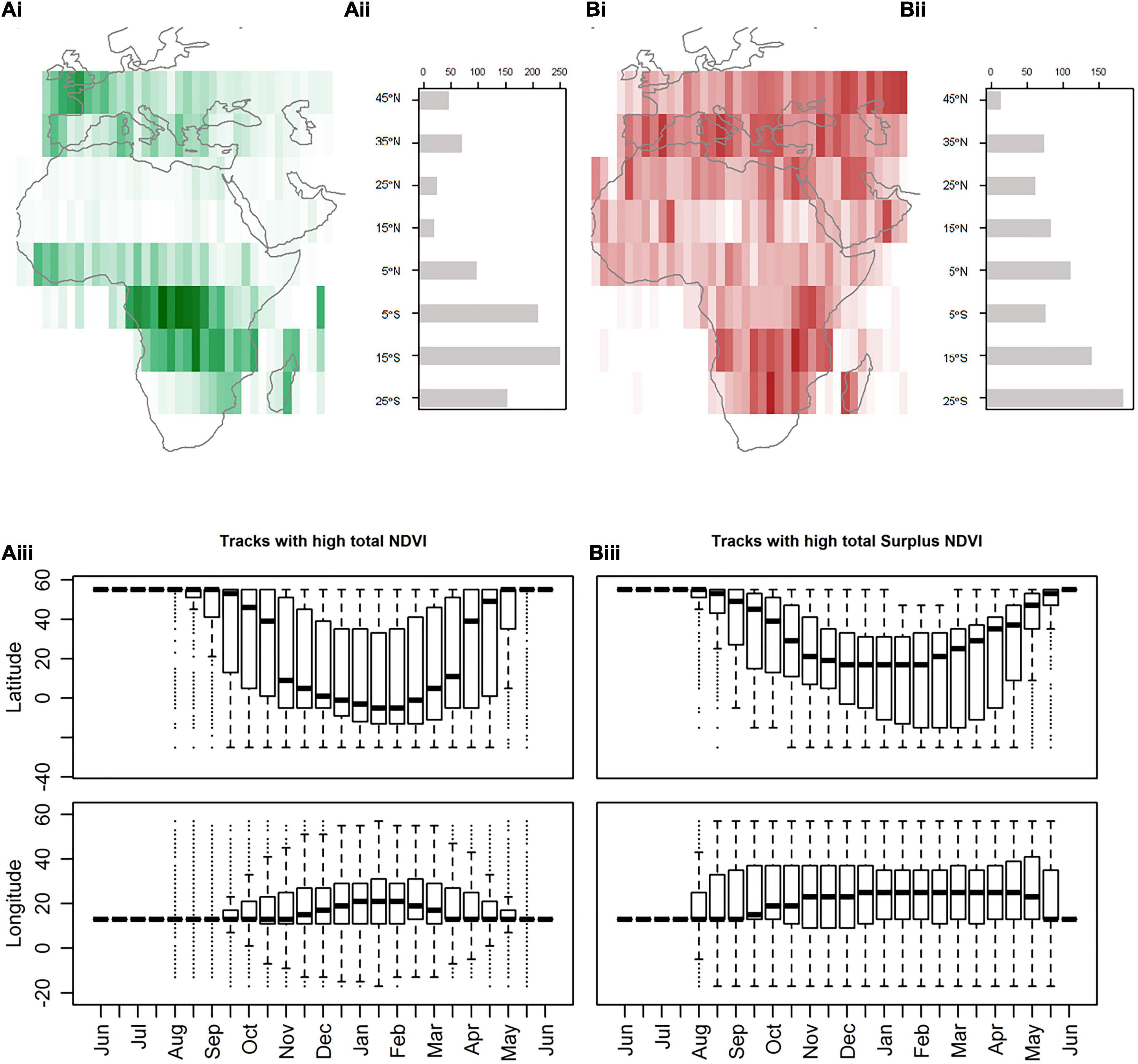
Figure 3. Space use of highest NDVI and Surplus NDVI tracks across the annual cycle. (A) Tracks with high total NDVI of all those generated (n = 740) and (B) total Surplus NDVI (n = 640). Count of cell use for high performance tracks aggregated every 10° of latitude south of the breeding site (i). Breeding sites are excluded from counts. Histogram (ii) of count of the southern extent of the same high performance tracks (from 45°N to 25°S). Latitudinal and longitudinal profiles (iii) for each temporal period. The boxplots represent the median value and upper and lower quartiles. Whiskers represent the 95% confidence interval and outliers are shown by dots.
Tracks with overall high NDVI had a tendency to depart the breeding grounds later and be more synchronized (early November; Figure 3Aiii) and arrive earlier (end April), compared to high Surplus NDVI tracks (early September and mid-May; Figure 3Biii). Tracks with high Surplus NDVI (Figure 3Biii) had a larger temporal and spatial longitudinal spread compared to a narrower, more directed range of cells used by tracks with high NDVI (Figure 3Aiii).
A greater number of stationary sites (Supplementary Figure 5C) were used by individual tracks with high Surplus NDVI compared to tracks with high NDVI where 42% of tracks used a single non-breeding site in central Africa, generally used between November and April (Figure 4A). High Surplus NDVI tracks with 2-11 stationary periods were equally represented (Supplementary Figure 5C). Monthly distributions (Figure 4) indicate high-use fall stopover sites (August/September) in coastal Western Europe and Eastern Europe for high NDVI tracks, and a broad area ranging from Continental to Steppic Europe north of 45°N in the fall (August/September) and north of 35°N in spring (April/May) for high Surplus NDVI tracks. Overall, tracks with high NDVI were more westerly distributed than those with high Surplus NDVI (which also moved further in winter). In particular, tracks were highly concentrated in western sub-Saharan Africa in fall and spring, compared to the broad space use of high surplus NDVI tracks. High NDVI tracks showed earlier northbound migration than high Surplus NDVI tracks. During northbound spring migration high surplus NDVI tracks were concentrated along the east coast of East Africa.
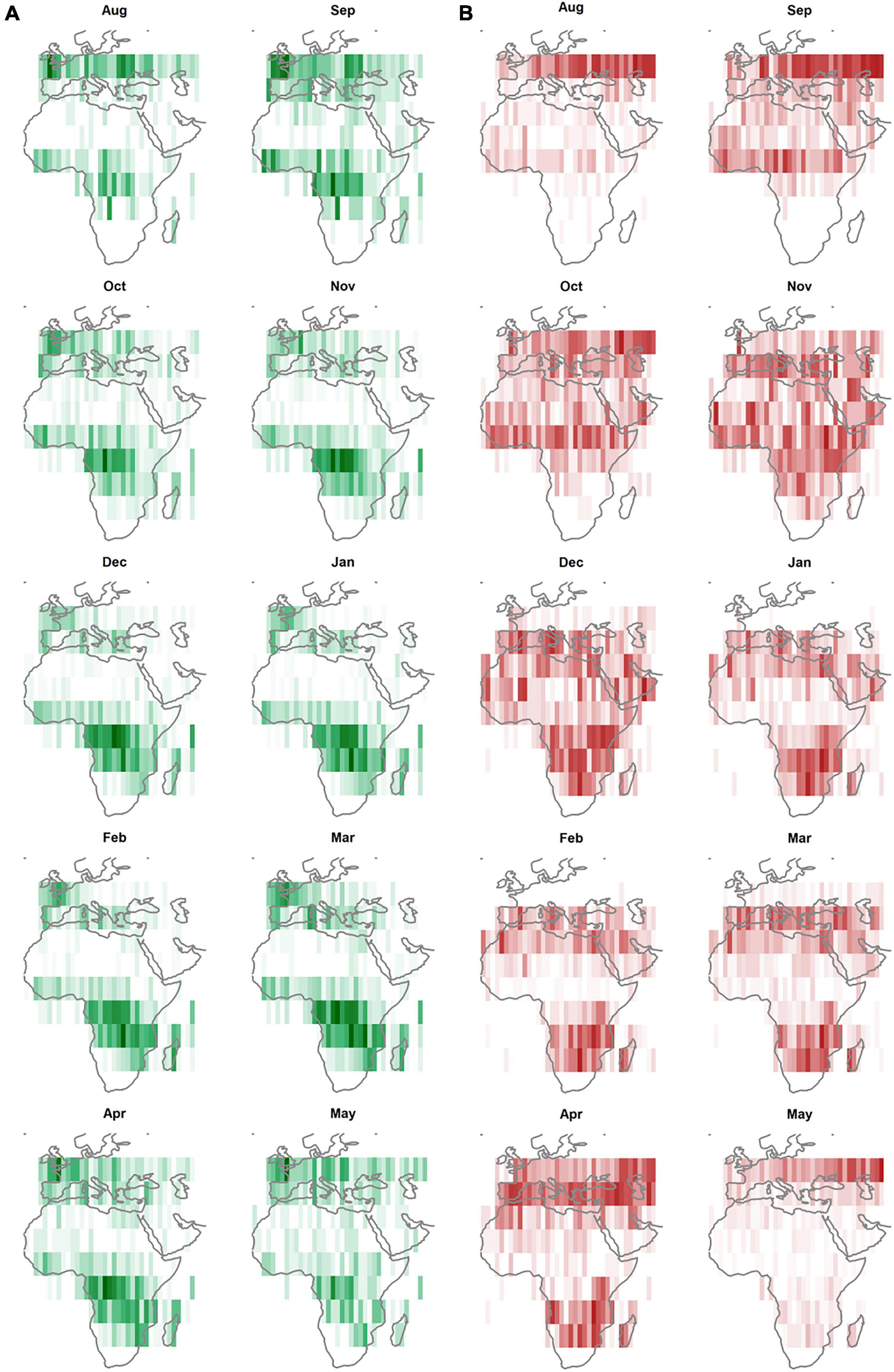
Figure 4. Seasonal space use of highest NDVI and Surplus NDVI tracks. Count of cell use for high performance tracks aggregated every 10° of latitude south of the breeding site per month outside the defined breeding period: beginning June – end July. (A) Tracks with highest total NDVI of all those generated (n = 740) and (B) total Surplus NDVI (n = 640). Breeding sites are excluded from counts.
Discussion
Temperature Drives Sub-Saharan Migration
Our fundamental conceptual simulation framework based on physiological constraints and resource availability suggests that the movement patterns of free-flying avian migrants reflect different responses to trade-offs in these parameters. We show a distinct temperature advantage from wintering in sub-Saharan Africa which might be associated with reduced physiological cost. The thermal advantage of crossing the ecological barrier from Europe into sub-Saharan Africa coupled with no obvious cost differential in resources, is evidence that ambient temperature could be an ultimate driver of obligate long-distance avian migration. Staying in areas with cold temperatures in Europe entail costs associated with a facultative or endogenous acclimatization response (Swanson, 1991; Eppley, 2008; Swanson and Vézina, 2015; Yahav, 2015). Metabolic or structural evolutionary adaptations are also likely to have occurred in response to temperature (Swanson and Vézina, 2015; Yahav, 2015; Blix, 2016; Nord et al., 2021). For example, a European robin has almost twice the ventral feather mass compared to a thrush nightingale (0.058 and 0.032 g cm–2, respectively; Osváth et al., 2018). In this case, the looser plumage in the long-distance migrant thrush nightingales could enhance heat loss in the warmer wintering environment that they experience. Thus, the thermal environment and phenotypic constraints or associated metabolic costs may drive species-specific adaptations to stay or leave.
Optimizing Seasonal Vegetation Resources
Our high-performing simulated tracks replicated much of the complexity of migration patterns observed in free-flying avian migrants in nature (Tøttrup et al., 2006), and indicate distinct differences between tracks optimized for high NDVI or high Surplus NDVI.
Tracks with high overall NDVI spend around an additional three months at the breeding site outside the defined two-month breeding period in June and July and generally used a single distinct area of Central Africa from September until April. This region of tropical rainforest represents the greatest ecological diversity within the continent (Jetz and Rahbek, 2002; Somveille et al., 2018), and simulations revealed an accurate conserved spatiotemporal wintering site (between October and February) for tracked migratory species (Gersten and Hahn, 2016; Jacobsen et al., 2017; Thorup et al., 2017). Furthermore, we find much overlap with a temporally explicit species distribution model (SDM) for common cuckoos (Williams et al., 2017): in summary, a conserved wintering region, long breeding residency and multiple within-year movements. High NDVI was generally available along a western/central route with wintering in the rainforest zone in the Congo Basin, similar to migration routes observed in common swifts, common cuckoos and European nightjars (Åkesson et al., 2012; Catry et al., 2014; Evens et al., 2017; Jacobsen et al., 2017). Also, the temporal schedule of numerous sub-Saharan migratory birds conform to high NDVI tracks (European nightjars Caprimulgus europaeus, European rollers Coracias garrulous, common redstarts Phoenicurus phoenicurus and European honey buzzards Pernis apivorus: Hake et al. (2003), Åkesson et al. (2012), Kristensen et al. (2013), Catry et al. (2014), Evens et al. (2017), Jacobsen et al. (2017)).
Tracks with high Surplus NDVI use more widely distributed areas, with a higher frequency of cell use across continental Europe, the Sahara and Sahel region and in southern East- and eastern South Africa, compared to high NDVI tracks. High Surplus NDVI tracks favor tropical shrubland, dry forest and moist deciduous forest, and multiple wintering sites were used in October and November before birds continued further south in December. This was equivalent to recorded wintering sites of insectivorous songbirds, with a comparable over-wintering duration within this region (Briedis et al., 2016; Thorup et al., 2017). In particular, these species travel further south than others tracked from Southern Scandinavia (Jacobsen et al., 2017). The multiple, consecutive, intra-continental movements and migratory detours observed primarily in high surplus NDVI simulated tracks also reflect the complex strategy of birds using multiple sites outside the breeding grounds (Jonzén et al., 2011; Stach et al., 2012; Tøttrup et al., 2012b; Thorup et al., 2017). Tracks of songbirds associated with high surplus vegetation follow the trends of the modeled tracks utilizing stopover sites in southern rather than northern continental Europe during fall migration (Stach et al., 2012; Tøttrup et al., 2012a). This is likely to have an advantage for migratory fattening in readiness for crossing over the Mediterranean Sea and the extensive Sahara Desert, as well as reducing the overall distance of the ecological barrier. Additionally, both Surplus NDVI optimizing tracks and tracked birds tend to move south earlier than NDVI optimized tracks, departing from the breeding site in August (Thorup et al., 2017). But the majority reach southern regions considerably later than NDVI specialists. Surplus NDVI was available along an eastern route with wintering in southern Africa. This route coincided well with migratory tracks of thrush nightingales and red-backed shrikes (Thorup et al., 2017). The stopovers before or after the barrier observed in most tracked migrants were less obviously resource-rich but, in general, timing and location of observed stopovers coincided with the highest local resource availability for regions in the periphery of the barrier.
Similarities between the modeled tracks under expected biotic and abiotic conditions and known migration strategies allow us to elucidate mechanistic components that potentially shape the diversity and complexity of long-distance migratory patterns. Closely related spatiotemporal patterns between model-generated tracks and free-flying birds support niche-following strategies in species apparently utilizing either NDVI or Surplus NDVI. Yet temporal schedules, principally in spring, suggest optimizing resources could compete with hastening arrival at the breeding sites or an advantage of shorter flights especially before and after the barrier crossings. Interestingly, a strategy of long winter residence time in the northern Sahel and the Savanna zone (e.g., in common redstarts and pied flycatchers Ficedula hypoleuca) is not characteristic of either of the optimal resource strategies identified here (Willemoes et al., 2013; Ouwehand et al., 2016). Potentially, for these species, avoiding the low temperatures in Europe is an important driver of sub-Saharan refuge migration rather than tracking high resource availability of importance for the longer-distance migrant species.
Data Availability Statement
The original contributions presented in this study are included in the article/Supplementary Material, further inquiries can be directed to the corresponding author/s.
Author Contributions
KT wrote the modeling script. KS developed the analysis, collated environmental data and wrote the first version of the manuscript. Both authors revised the text and designed the study.
Funding
The Danish Council for Independent Research supported the MATCH project (1323-00048B) and the Danish National Research Foundation supported the Center for Macroecology, Evolution and Climate (DNRF96). KS is also supported by a Marie Skłodowska-Curie Fellowship (TesSEH – 892006).
Conflict of Interest
The authors declare that the research was conducted in the absence of any commercial or financial relationships that could be construed as a potential conflict of interest.
Publisher’s Note
All claims expressed in this article are solely those of the authors and do not necessarily represent those of their affiliated organizations, or those of the publisher, the editors and the reviewers. Any product that may be evaluated in this article, or claim that may be made by its manufacturer, is not guaranteed or endorsed by the publisher.
Acknowledgments
We thank Stephanie Snyder and Simeon Lisovski for constructive discussions on modeling approach and environmental data handling.
Supplementary Material
The Supplementary Material for this article can be found online at: https://www.frontiersin.org/articles/10.3389/fevo.2022.824641/full#supplementary-material
References
Åkesson, S., Klaassen, R., Holmgren, J., Fox, J. W., and Hedenstrom, A. (2012). Migration routes and strategies in a highly aerial migrant, the common swift Apus apus, revealed by light-level geolocators. PLoS One 7:e41195. doi: 10.1371/journal.pone.0041195
Alerstam, T., and Hedenström, A. (1998). The development of bird migration theory. J. Avian Biol. 29, 343–369. doi: 10.2307/3677155
Alerstam, T., Hedenström, A., and Åkesson, S. (2003). Long-distance migration: evolution and determinants. Oikos 103, 247–260. doi: 10.1034/j.1600-0706.2003.12559.x
Alerstam, T., and Lindström, Å (1990). “Optimal bird migration: the relative importance of time, energy, and safety,” in Bird Migration, ed. E. Gwinner (Berlin, Heidelberg: Springer), 331–351. doi: 10.1007/978-3-642-74542-3_22
Arlt, D., Olsson, P., Fox, J. W., Low, M., and Pärt, T. (2015). Prolonged stopover duration characterises migration strategy and constraints of a long-distance migrant songbird. Anim. Migr. 2, 47–62. doi: 10.1515/ami-2015-0002
Backman, J., Andersson, A., Pedersen, L., Sjoberg, S., Tottrup, A. P., and Alerstam, T. (2017). Actogram analysis of free-flying migratory birds: new perspectives based on acceleration logging. J. Comp. Physiol. A 203, 543–564. doi: 10.1007/s00359-017-1165-9
Blackburn, E., and Cresswell, W. (2016). High within-winter and annual survival rates in a declining Afro-Palaearctic migratory bird suggest that wintering conditions do not limit populations. Ibis 158, 92–105. doi: 10.1111/ibi.12319
Blix, A. S. (2016). Adaptations to polar life in mammals and birds. J. Exp. Biol. 219, 1093–1105. doi: 10.1242/jeb.120477
Block, B. A. I, Jonsen, D., Jorgensen, S. J., Winship, A. J., Shaffer, S. A., Bograd, S. J., et al. (2011). Tracking apex marine predator movements in a dynamic ocean. Nature 475, 86–90. doi: 10.1038/nature10082
Bridge, E. S., Ross, J. D., Contina, A. J., and Kelly, J. F. (2016). Do molt-migrant songbirds optimize migration routes based on primary productivity? Behav. Ecol. 27, 784–792. doi: 10.1093/beheco/arv199
Briedis, M., Träff, J., Hahn, S., Ilieva, M., Král, M., Peev, S., et al. (2016). Year-round spatiotemporal distribution of the enigmatic Semi-collared Flycatcher Ficedula semitorquata. J. Ornithol. 157, 895–900. doi: 10.1007/s10336-016-1334-6
Calder, W. (1974). “Thermal and caloric relations of birds,” in Avian Biology, eds D. Farner and J. King (New York: Academic Press). doi: 10.3382/ps/pez176
Catry, I., Catry, T., Granadeiro, J. P., Franco, A. M. A., and Moreira, F. (2014). Unravelling migration routes and wintering grounds of European rollers using light-level geolocators. J. Ornithol. 155, 1071–1075. doi: 10.1007/s10336-014-1097-x
Connolly, S. R., Keith, S. A., Colwell, R. K., and Rahbek, C. (2017). Process, Mechanism, and Modeling in Macroecology. Trends Ecol. Evol. 32, 835–844. doi: 10.1016/j.tree.2017.08.011
DeLuca, W. V., Woodworth, B. K., Rimmer, C. C., Marra, P. P., Taylor, P. D., McFarland, K. P., et al. (2015). Transoceanic migration by a 12 g songbird. Biol. Lett. 11:20141045. doi: 10.1098/rsbl.2014.1045
Eppley, Z. A. (2008). “Development of thermoregulation in birds: physiology, interspecific variation and adaptation to climate,” in Animals and temperature: phenotypic and evolutionary adaptation, eds I. A. Johnston and A. F. Bennett (Cambridge: Cambridge University Press), 313–346. doi: 10.1017/CBO9780511721854.014
Evens, R., Conway, G. J., Henderson, I. G., Cresswell, B., Jiguet, F., Moussy, C., et al. (2017). Migratory pathways, stopover zones and wintering destinations of Western European nightjars Caprimulgus europaeus. Ibis 159, 680–686. doi: 10.1111/ibi.12469
Gersten, A., and Hahn, S. (2016). Timing of migration in Common Redstarts (Phoenicurus phoenicurus) in relation to the vegetation phenology at residence sites. J. Ornithol. 157, 1029–1036. doi: 10.1007/s10336-016-1359-x
Gill, J. A., and Hays, G. (2015). Encountering extreme weather during migration: individual strategies and their consequences. J. Anim. Ecol. 84, 1141–1143. doi: 10.1111/1365-2656.12412
Hake, M., Kjellén, N., and Alerstam, T. (2003). Age-dependent migration strategy in honey buzzards Pernis apivorus tracked by satellite. Oikos 103, 385–396. doi: 10.1034/j.1600-0706.2003.12145.x
Heckscher, C. M., Taylor, S. M., Fox, J. W., and Afanasyev, V. (2011). Veery (Catharus fuscescens) Wintering Locations, Migratory Connectivity, and a Revision of its Winter Range Using Geolocator Technology. Auk 128, 531–542. doi: 10.1525/auk.2011.10280
Hewson, C. M., Thorup, K., Pearce-Higgins, J. W., and Atkinson, P. W. (2016). Population decline is linked to migration route in the Common Cuckoo. Nat. Commun. 7:12296. doi: 10.1038/ncomms12296
Hijmans, R. J. (2016). raster: Geographic Data Analysis and Modeling. R package version 2.5-8. Available Online at:https://CRAN.R-project.org/package=raster (accessed July 26, 2017).
Irving, L., and Krog, J. (1954). Body temperatures of arctic and subarctic birds and mammals. J. Appl. Physiol. 6, 667–680. doi: 10.1152/jappl.1954.6.11.667
Jacobsen, L. B., Jensen, N. O., Willemoes, M., Hansen, L., Desholm, M., Fox, A. D., et al. (2017). Annual spatiotemporal migration schedules in three larger insectivorous birds: european nightjar, common swift and common cuckoo. Anim. Biotelemetry 5:4. doi: 10.1186/s40317-017-0119-x
Jetz, W., and Rahbek, C. (2002). Geographic range size and determinants of avian species richness. Science 297, 1548–1551. doi: 10.1126/science.1072779
Jonzén, N., Knudsen, E., Holt, R. D., and Sæther, B.-E. (2011). “Uncertainty and predictability: the niches of migrants and nomads,” in Animal migration: a synthesis, eds E. J. Milner-Gulland, J. M. Fryxell, and A. R. E. Sinclair (Oxford: Oxford University Press), 91–109. doi: 10.1093/acprof:oso/9780199568994.003.0007
Justice, C. O., Vermote, E., Townshend, J. R., Defries, R., Roy, D. P., Hall, D. K., et al. (1998). The Moderate Resolution Imaging Spectroradiometer (MODIS): land remote sensing for global change research. IEEE Trans. Geosci. Remote Sens. 36, 1228–1249. doi: 10.1109/36.701075
Kanamitsu, M., Ebisuzaki, W., Woollen, J., Yang, S.-K., Hnilo, J. J., Fiorino, M., et al. (2002). NCEP–DOE AMIP-II Reanalysis (R-2). Bull. Am. Meteorol. Soc. 83, 1631–1643. doi: 10.1175/BAMS-83-11-1631
Kays, R., Crofoot, M. C., Jetz, W., and Wikelski, M. (2015). Terrestrial animal tracking as an eye on life and planet. Science 348:aaa2478. doi: 10.1126/science.aaa2478
Kemp, M. U., Emiel van Loon, E., Shamoun-Baranes, J., and Bouten, W. (2012). RNCEP: global weather and climate data at your fingertips. Methods Ecol. Evol. 3, 65–70. doi: 10.1111/j.2041-210X.2011.00138.x
Khaliq, I., Hof, C., Prinzinger, R., Bohning-Gaese, K., and Pfenninger, M. (2014). Global variation in thermal tolerances and vulnerability of endotherms to climate change. Proc. R. Soc. Lond. Ser. B Biol. Sci. 281:20141097. doi: 10.1098/rspb.2014.1097
Klaassen, R. H. G., Strandberg, R., Hake, M., Olofsson, P., Tøttrup, A. P., and Alerstam, T. (2010). Loop migration in adult marsh harriersCircus aeruginosus,as revealed by satellite telemetry. J. Avian Biol. 41, 200–207. doi: 10.1111/j.1600-048X.2010.05058.x
Kristensen, M. W., Tøttrup, A. P., and Thorup, K. (2013). Migration of the Common Redstart (Phoenicurus phoenicurus). Auk 130, 258–264.
La Sorte, F. A., Fink, D., Hochachka, W. M., DeLong, J. P., and Kelling, S. (2014). Spring phenology of ecological productivity contributes to the use of looped migration strategies by birds. Proc. Biol. Sci. 281:20140984. doi: 10.1098/rspb.2014.0984
Lemke, H. W., Tarka, M., Klaassen, R. H., Akesson, M., Bensch, S., Hasselquist, D., et al. (2013). Annual cycle and migration strategies of a trans-Saharan migratory songbird: a geolocator study in the great reed warbler. PLoS One 8:e79209. doi: 10.1371/journal.pone.0079209
Lisovski, S., Ramenofsky, M., and Wingfield, J. C. (2017). Defining the Degree of Seasonality and its Significance for Future Research. Integr. Comp. Biol. 57, 934–942. doi: 10.1093/icb/icx040
Loonstra, A. J., Verhoeven, M. A., Senner, N. R., Both, C., and Piersma, T. (2019). Adverse wind conditions during northward Sahara crossings increase the in-flight mortality of Black-tailed Godwits. Ecol. Lett. 22, 2060–2066. doi: 10.1111/ele.13387
McKinnon, E. A., Fraser, K. C., and Stutchbury, B. J. M. (2013). New discoveries in landbird migration using geolocators, and a flight plan for the future. Auk 130, 211–222. doi: 10.1525/auk.2013.12226
MODIS (2016). MOD13C1 - NDVI. NASA EOSDIS Land Processes DAAC. Sioux Falls, South Dakota: USGS Earth Resources Observation and Science (EROS) Center.
MODIS, (2017). MOD17A2_E_PSN - NPP. Available Online at: https://modis-land.gsfc.nasa.gov/npp.html (accessed 26 July, 2017).
National Centers for Environmental Prediction/National Weather Service/NOAA/U.S. Department of Commerce (2000). NCEP FNL Operational Model Global Tropospheric Analyses, continuing from July 1999. Boulder, CO: Research Data Archive at the National Center for Atmospheric Research, Computational and Information Systems Laboratory.
Nord, A., Metcalfe, N. B., Page, J. L., Huxtable, A., McCafferty, D. J., and Dawson, N. J. (2021). Avian red blood cell mitochondria produce more heat in winter than in autumn. FASEB J. 35:e21490. doi: 10.1096/fj.202100107R
Osváth, G., Daubner, T., Dyke, G., Fuisz, T. I., Nord, A., Pénzes, J., et al. (2018). How feathered are birds? Environment predicts both the mass and density of body feathers. Funct. Ecol. 32, 701–712. doi: 10.1007/s00442-010-1849-0
Ouwehand, J., Ahola, M. P., Ausems, A. N. M. A., Bridge, E. S., Burgess, M., Hahn, S., et al. (2016). Light-level geolocators reveal migratory connectivity in European populations of Pied Flycatchers Ficedula hypoleuca. J. Avian Biol. 47, 69–83. doi: 10.1111/jav.00721
Paxton, E. H., Durst, S. L., Sogge, M. K., Koronkiewicz, T. J., and Paxton, K. L. (2017). Survivorship across the annual cycle of a migratory passerine, the willow flycatcher. J. Avian Biol. 48, 1126–1131. doi: 10.1111/jav.01371
Pettorelli, N., Ryan, S., Mueller, T., Bunnefeld, N., Jedrzejewska, B., Lima, M., et al. (2011). The Normalized Difference Vegetation Index (NDVI): unforeseen successes in animal ecology. Clim. Res. 46, 15–27. doi: 10.3354/cr00936
R Core Team (2017). R: A Language and Environment for Statistical Computing. Vienna, Austria: R Foundation for Statistical Computing.
Ramenofsky, M., and Wingfield, J. C. (2007). Regulation of migration. Bioscience 57, 135–143. doi: 10.1641/B570208
Renfrew, R. B., Kim, D., Perlut, N., Smith, J., Fox, J., Marra, P. P., et al. (2013). Phenological matching across hemispheres in a long-distance migratory bird. Divers. Distrib. 19, 1008–1019. doi: 10.1111/ddi.12080
Running, S., Mu, Q., and Zhao, M. (2015). MOD17A3H MODIS/Terra Net Primary Production Yearly L4 Global 500m SIN Grid V006 [Data set]. NASA EOSDIS Land Processes DAAC. Available online at: https://doi.org/10.5067/MODIS/MOD17A3H.006 (accessed July 26, 2017).
Senner, N. R., Verhoeven, M. A., Abad-Gómez, J. M., Alves, J. A., Hooijmeijer, J. C., and Howison, R. A. (2019). High migratory survival and highly variable migratory behavior in black-tailed godwits. Front. Ecol. Evol. 7:96. doi: 10.3389/fevo.2019.00096
Shariatinajafabadi, M., Wang, T., Skidmore, A. K., Toxopeus, A. G., Kolzsch, A., Nolet, B. A., et al. (2014). Migratory herbivorous waterfowl track satellite-derived green wave index. PLoS One 9:e108331. doi: 10.1371/journal.pone.0108331
Shaw, A. K. (2016). Drivers of animal migration and implications in changing environments. Evol. Ecol. 30, 991–1007. doi: 10.1007/s10682-016-9860-5
Shaw, A. K. (2020). Causes and consequences of individual variation in animal movement. Mov. Ecol. 8:12. doi: 10.1186/s40462-020-0197-x
Sillett, T. S., and Holmes, R. T. (2002). Variation in survivorship of a migratory songbird throughout its annual cycle. J. Anim. Ecol. 71, 296–308. doi: 10.1046/j.1365-2656.2002.00599.x
Snell, K. R., Stokke, B. G., Moksnes, A., Thorup, K., and Fossøy, F. (2018). From Svalbard to Siberia: passerines breeding in the High Arctic also endure the extreme cold of the Western Steppe. PLoS One 13:e0202114. doi: 10.1371/journal.pone.0202114
Snell, K. R. S., and Thorup, K. (2019). Experience and survival in migratory European Robins Erithacus rubecula and Song Thrushes Turdus philomelos negotiating the Baltic Sea. Bird Study 66, 83–91. doi: 10.1080/00063657.2019.1617233
Somveille, M., Manica, A., and Rodrigues, A. S. (2019). Where the wild birds go: explaining the differences in migratory destinations across terrestrial bird species. Ecography 42, 225–236. doi: 10.1111/ecog.03531
Somveille, M., Rodrigues, A. S., and Manica, A. (2018). Energy efficiency drives the global seasonal distribution of birds. Nat. Ecol. Evol. 2, 962–969. doi: 10.1038/s41559-018-0556-9
Somveille, M., Rodrigues, A. S. L., and Manica, A. (2015). Why do birds migrate? A macroecological perspective. Glob. Ecol. Biogeogr. 24, 664–674. doi: 10.1111/geb.12298
Stach, R., Jakobsson, S., Kullberg, C., and Fransson, T. (2012). Geolocators reveal three consecutive wintering areas in the thrush nightingale. Anim. Migr. 1, 1–7. doi: 10.2478/ami-2012-0001
Strandberg, R., Klaassen, R. H., Hake, M., and Alerstam, T. (2010). How hazardous is the Sahara Desert crossing for migratory birds? Indications from satellite tracking of raptors. Biol. Lett. 6, 297–300. doi: 10.1098/rsbl.2009.0785
Stutchbury, B. J., Siddiqui, R., Applegate, K., Hvenegaard, G. T., Mammenga, P., Mickle, N., et al. (2016). Ecological Causes and Consequences of Intratropical Migration in Temperate-Breeding Migratory Birds. Am. Nat. 188, S28–S40. doi: 10.1086/687531
Swanson, D. L. (1991). Seasonal adjustments in metabolism and insulation in the dark-eyed junco. Condor 93, 538–545. doi: 10.2307/1368185
Swanson, D. L. (2010). Seasonal Metabolic Variation in Birds: functional and Mechanistic Correlates. Curr. Ornithol. 17, 75–129. doi: 10.1007/978-1-4419-6421-2_3
Swanson, D. L., and Vézina, F. (2015). Environmental, ecological and mechanistic drivers of avian seasonal metabolic flexibility in response to cold winters. J. Ornithol. 156, 377–388. doi: 10.1007/s10336-015-1192-7
Thorup, K., Tøttrup, A. P., Willemoes, M., Klaassen, R. H., Strandberg, R., Vega, M. L., et al. (2017). Resource tracking within and across continents in long-distance bird migrants. Sci. Adv. 3:e1601360. doi: 10.1126/sciadv.1601360
Tøttrup, A. P., Klaassen, R. H., Strandberg, R., Thorup, K., Kristensen, M. W., Jørgensen, P. S., et al. (2012b). The annual cycle of a trans-equatorial Eurasian–African passerine migrant: different spatio-temporal strategies for autumn and spring migration. Proc. R. Soc. Lond. Ser. B Biol. Sci. 279, 1008–1016. doi: 10.1098/rspb.2011.1323
Tøttrup, A. P., Klaassen, R. H., Kristensen, M. W., Strandberg, R., Vardanis, Y., Lindstrom, A., et al. (2012a). Drought in Africa caused delayed arrival of European songbirds. Science 338:1307. doi: 10.1126/science.1227548
Tøttrup, A. P., Thorup, K., and Rahbek, C. (2006). Patterns of change in timing of spring migration in North European songbird populations. J. Avian Biol. 37, 84–92. doi: 10.1093/icb/icq028
Trierweiler, C., Mullie, W. C., Drent, R. H., Exo, K. M., Komdeur, J., Bairlein, F., et al. (2013). A Palaearctic migratory raptor species tracks shifting prey availability within its wintering range in the Sahel. J. Anim. Ecol. 82, 107–120. doi: 10.1111/j.1365-2656.2012.02036.x
Tucker, C. J. (1979). Red and photographic infrared linear combinations for monitoring vegetation. Remote Sens. Environ. 8, 127–150. doi: 10.1016/0034-4257(79)90013-0
Willemoes, M., Strandberg, R., Klaassen, R. H., Tottrup, A. P., Vardanis, Y., Howey, P. W., et al. (2014). Narrow-front loop migration in a population of the common cuckoo Cuculus canorus, as revealed by satellite telemetry. PLoS One 9:e83515. doi: 10.1371/journal.pone.0083515
Willemoes, M., Tøttrup, A. P., and Thorup, K. (2013). Migration of the Common Redstart (Phoenicurus phoenicurus): a Eurasian songbird wintering in highly seasonal conditions in the West African Sahel. Auk 130, 258–264. doi: 10.1525/auk.2013.13001
Williams, H. M., Willemoes, M., and Thorup, K. (2017). A temporally explicit species distribution model for a long distance avian migrant, the common cuckoo. J. Avian Biol. 48, 1624–1636. doi: 10.1111/jav.01476
Keywords: simulations, Afro-Palearctic, NDVI, movement ecology, ambient temperature
Citation: Snell KRS and Thorup K (2022) Modeling Complex Seasonal Avian Migration: Predictions From the Thermal Environment and Resource Availability. Front. Ecol. Evol. 10:824641. doi: 10.3389/fevo.2022.824641
Received: 29 November 2021; Accepted: 16 May 2022;
Published: 06 June 2022.
Edited by:
Eyal Shochat, Ben-Gurion University of the Negev, IsraelReviewed by:
Nathan R. Senner, University of South Carolina, United StatesChristine Howard, Durham University, United Kingdom
Adi Domer, Ben-Gurion University of the Negev, Israel
Copyright © 2022 Snell and Thorup. This is an open-access article distributed under the terms of the Creative Commons Attribution License (CC BY). The use, distribution or reproduction in other forums is permitted, provided the original author(s) and the copyright owner(s) are credited and that the original publication in this journal is cited, in accordance with accepted academic practice. No use, distribution or reproduction is permitted which does not comply with these terms.
*Correspondence: Katherine R. S. Snell, a3NuZWxsQGFiLm1wZy5kZQ==; Kasper Thorup, a2FzcGVyLnRob3J1cEBzdW5kLmt1LmRr
†ORCID: Katherine R. S. Snell, orcid.org/0000-0003-3720-4579; Kasper Thorup, orcid.org/0000-0002-0320-0601
‡Present address: Katherine R. S. Snell, Max-Planck Institute of Animal Behavior, Radolfzell, Germany