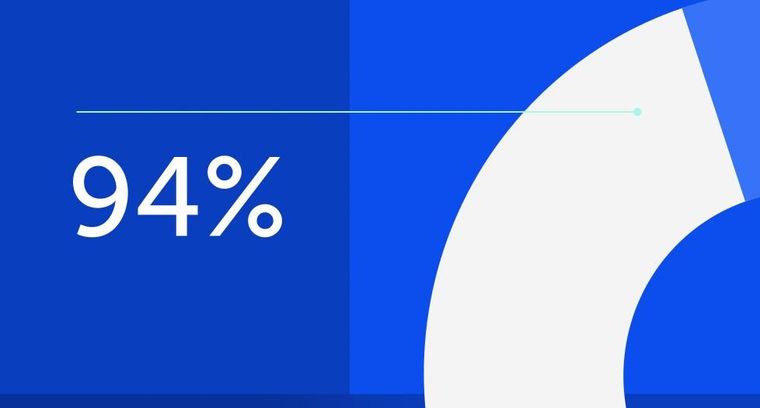
94% of researchers rate our articles as excellent or good
Learn more about the work of our research integrity team to safeguard the quality of each article we publish.
Find out more
HYPOTHESIS AND THEORY article
Front. Ecol. Evol., 14 February 2022
Sec. Evolutionary Ecology of Social Behaviour
Volume 10 - 2022 | https://doi.org/10.3389/fevo.2022.791104
This article is part of the Research TopicSocial Evolution and the What, When, Why and How of the Major Evolutionary Transitions in the History of LifeView all 11 articles
Species’ adaptation to their environments occurs via a range of mechanisms of adaptation. These include genetic adaptations as well as non-traditional inheritance mechanisms such as learned behaviors, niche construction, epigenetics, horizontal gene transfer, and alteration of the composition of a host’s associated microbiome. We propose to supplement these with another modality of eco-evolutionary dynamics: cases in which adaptation to the environment occurs via what may be called a “distributed adaptation,” in which the adaptation is not conferred via something carried by an individual of the adapted species (as with genes, behavior, or associated microbes), but by some structural or compositional aspect of the population. Put differently, the adaptively relevant information cannot be reduced to information possessed by a single individual, whether genetic or otherwise. Rather, the adaptively relevant information is distributed, and is found strictly at the population level. While human culture is presumably such a case, as may be cases found in social insects, we want to suggest that there are other cases that belong to this category and to explore its evolutionary implications. In particular, we discuss the factors that affect whether adaptive information is stored in a distributed way, to what degree, and what kinds of adaptive information are most likely to be found in this modality of adaptation.
Can a species be adapted to its environment in a certain respect, while no single individual carries the adaptation? Consider the following example: imagine a species in which individuals need to find suitable habitat to breed or dwell when they reach maturity. Adaptations that are carried by the individual that could accommodate this need are, for example, innate knowledge about the environmental cues that characterize a suitable site or learned knowledge about where such sites are to be found. These solutions consist of individual-level adaptive information. Alternatively, a distributed adaptation (DA), comprising population-level adaptive information, might accommodate the need as well: individuals could rely solely on searching for other individuals of the species, and joining them. Such a strategy is likely to be adaptive, since—by definition—locations in which multiple individuals are found are highly likely to be habitable (see, e.g., Bee, 2007; Fouquet et al., 2021). In this case, the information necessary for survival is encapsulated in the species’ demography as a whole, i.e., in the spatial distribution of the species’ individuals (coupled with their aggregating behavior).
Our discussion will proceed as follows. In section 2 we define and develop the concept of distributed adaptations. In section 3 we discuss the concept’s relation to other phenomena and paradigms, and in section 4 we offer a number of comments regarding its utility and some theoretical implications. Section 5 summarizes and offers a series of concluding remarks and open avenues for further exploration.
To discuss modalities of adaptation, the definition of an adaptation must be clear. As an intuitive and broad definition which does not pre-determine the level at which an adaptation is implemented, we will use the term adaptation to refer to any trait that facilitates survival and persistence in the environment, including over multi-generational timescales.
To illustrate the notion of a distributed adaptation we discuss two hypothetical cases. Let us consider first the example from above in more detail: a species of frogs living in a semi-arid region. Let us imagine that an individual must find a seasonal pond in which to survive and reproduce, but these ponds are scattered across inhospitable terrain (see, e.g., Goldber et al., 2009; Degani, 2016, 2015). Searching haphazardly for them is costly. A possible adaptation might involve some level of innate knowledge of the terrain or navigation skills, such as having preferences to go in certain directions. But this may not be possible if the location of ponds is highly irregular or changes too quickly (e.g., Vaira, 2005). An alternative adaptation involves learning the location of ponds from the mother, but this may not be possible in a species in which there is little or no parental care after hatching. A third possibility would involve some cognitive and sensory capabilities that improve navigation to water or to the individual’s specific natal environment. Such adaptations are possibly difficult to evolve or may be costly to maintain. A distributed adaptation to the problem of finding ponds may be simple: the frog listens to croaking sounds coming from conspecifics and navigates toward them. Since the sounds of several other individuals coming from the same location typically indicate hospitable locations, going in the direction of croaking will often lead in the direction of a pond. The sensory and behavioral adaptation is simple and may build on existing sensory abilities and behavioral preferences (Bee, 2007; Fouquet et al., 2021). Navigating toward sounds of multiple conspecifics thus constitutes an adaptation to a major challenge posed by the species’ environment.
This hypothetical case highlights the essence of what we term distributed adaptations: the information that the frog relies on to find the ponds is not “stored” in any individual. It is, for example, impossible for a single frog to learn from another where to find the ponds, nor does any frog remember the location of ponds across the terrain. The information about the location is honestly represented by the actual location of the frogs in the population, and croaking makes this distributed information accessible. The adaptive information can thus be said to be distributed information. A possible implication of such a scenario, for example, may be that the distributed adaptation is a potential source of difficulty in re-introduction programs for species that had gone locally extinct. Simply reintroducing enough individuals may not be sufficient, if they are not spread out in a way that realizes the appropriate distribution of the population in its specific terrain. It would be critical in such a case to realize that the adaptation to the environment is a distributed adaptation, to allow wildlife managers to plan reintroduction programs accordingly. Considering only the individual-level adaptations that allow the frogs to persist in their environment misses an important aspect of the adaptation.
This example illustrates how distributed adaptation differs from related theoretical notions, in particular division of labor (Robinson, 1992; Beshers and Fewell, 2001) and niche construction (Laland et al., 2000, 1999; Odling-Smee et al., 2003, 1996). There is no division of labor in the frog population, with some frogs better able to navigate than others, leading to group benefits. Our frog example can be viewed as a form of a collective memory. We suggest that collective memories that are important for a population’s persistence are a category of distributed adaptation (e.g., Kaczensky et al., 2011); however, the more interesting among them, and those in which the distributed adaptations’ perspective may be particularly useful, are cases in which different individuals carry different information and their sum is greater than its parts: those in which no single individual has the relevant information (Seeley et al., 2006). In particular, no individual has the “correct” or best information. The adaptive information arises from combining signals from multiple individuals.
This case is also not a case of niche construction. It is similar in so far that in niche construction multiple individuals contribute to the changes in the niche. Yet, in this case there are no persistent changes to the environment, on the one hand, and individuals make use of the distributed information, and integrate multiple pieces of information, on the other hand. These differences between distributed adaptation and related notions all lead to empirically testable predictions. For example, it is possible to empirically study whether different frogs have different phenotypes or behaviors (suggesting division of labor) and whether there are persistent changes to the environment that affect selection pressures or development (suggesting a niche construction explanation). Conversely, to test a distributed adaptation hypothesis it is possible to manipulate the information that is potentially aggregated, by experimentally producing croaking sounds and seeing how they affect the behavior of the frogs; manipulating the sound levels (e.g., by obstructing them); and so on; and seeing how these manipulations affect frogs’ navigation. It is also possible to construct mathematical models and simulations to study the effects of such manipulations theoretically.
It might be tempting to consider only the croaking and moving in the direction of croaking sounds to be the adaptation. However, this perspective would miss the fact that these are adaptive only in relation to properties of the population (i.e., the number and distribution of frogs in the terrain), properties of the environment (i.e., the difficulty of navigation, terrain features that block sounds, etc.), and the relation between these factors. This relation concerns population level properties. That said, a key set of questions prompted by the notion of distributed adaptation involves the degree to which individuals rely on distributed information as opposed to individual traits and individual learning, and whether and how this is shaped evolutionarily. Consider a second thought experiment. Imagine a larva that transforms into a beetle. The imaginary beetles have the genetic capacity to produce several pigment colors; however, during transformation an irrevocable choice of one of them must be made. The color of the beetle affects the success of camouflage and hence survival. The best camouflage color depends on the type of predator that is prevalent at a given moment: birds, spiders, and lizards have different eyesight, and imagine that each can spot certain colors more easily than others. One possibility is for the larvae to choose a color randomly, in frequencies that are optimized evolutionarily. Another possible adaptation is to sense the colors of beetles in the vicinity and preferentially choose the color of the majority. If survival depends on having the right color, the relative prevalence of colors among adults that were not predated upon is a reliable indication of which color is currently preferable. As in the previous example, the key point is that the information about “best” color is not known to any individual nor genetically determined, but rather is spread in the population. As in the previous example, the ability to sense the color of conspecifics and use it to choose which color to adopt may evolve from an existing ability to detect colors in the environment.
The ability of a population to acquire and utilize information transcends the ability of a single individual in many well-known cases (Sosna et al., 2019). Consider vultures’ foraging for carcasses to feed on: a single individual has a relatively low probability of finding food. However, by flying at a high altitude while keeping eye-contact with each other, groups of vultures jointly survey vast landscapes. When a vulture identifies a carcass it dives down, attracting others to dive in the same direction (Houston, 1974; Buckley, 1996; Jackson et al., 2008). This example illustrates that even over short periods of time distributed information may be crucial for survival [see also in this context the concept of emergent synergies discussed in Keenan and McShea (2021)].
One may wonder regarding the role of intentionality in DAs and about the relation between individual-level adaptation and DAs. Should a population-level adaptation be considered a DA only if individuals share their information with others in a way that seems intentional, as in a case of frogs’ croaking, or vice versa? Should it be viewed as a DA only if it is also adaptive to the individual? For example, in the short term it is likely that a vulture could benefit from making its descent toward a carcass go unnoticed, if this were possible, thus decreasing the competition that it will experience while feeding (see also Rendell et al., 2010). Perhaps a “true” DA is only one in which adaptive value is gained at the population level and not—in the short term—by the individual? We suggest that these considerations are important, with crucial implications for understanding and predicting evolutionary dynamics of each studied scenario, but should be viewed as orthogonal to the question of which of these cases is considered a DA. We suggest that a DA perspective can yield insights regarding each of these scenarios. Interestingly, these considerations also influence whether a DA is likely to evolve in the first place and whether it is likely to persist: if sharing of information is not in the best interest of individuals, it is unlikely to emerge, unless avoidance of information-sharing has an even greater cost. Thus, for example, it may be that the frogs’ calling, which attracts competing individuals, is maladaptive at the individual level in the short term but cannot be avoided because calling is also the frogs’ means of attracting mates. Full treatment of these factors’ roles in the evolution and maintenance of DAs is beyond the scope of the current study.
What distinguishes distributed adaptations from other forms of adaptation is that the population functions as a distributed information store. The information needed to produce the adaptive trait of an individual cannot be reduced to information found in a single genome or carried by a single individual’s microbiome, memory, or morphological phenotype but instead consists of interactions between multiple individuals in the population and their ecological interaction with the environment.
This observation paves the path to a generalization of the concept: it is reasonable to consider the population-environment complex, and not only the population in itself, as often providing the necessary information for an individual’s survival (Blanchet et al., 2010). This information may be embedded in the features of the population’s spatial distribution, its behavior, or its interactions with elements in the environment. This dependency on the environment may be, for example, the availability of other individuals of the same species (as in our original example), presence of individuals of other species, or various other aspects of the biotic or abiotic environment; these can be structural, nutritional, chemical, etc. We refer to complex cases of distributed adaptation, in which properties of the population are significant and especially when the phenotypes of individuals depend on population-level features and their relations to the environment are critical for understanding the system, as conformational adaptations.
Cultural adaptations may be viewed as distributed adaptations. In simple cases it is possible for an individual to learn from a single other individual, while the population may embody additional information, such as the frequencies of different behaviors and their payoffs, which organisms may evolve to utilize. In more complex cases, an individual acquires the information it requires by combining information from multiple other individuals. Often, the acquisition has to occur in a specific order. The availability and salience of information may affect the developmental trajectory of individuals.
Distributed adaptations depend on the population or ecosystem level; however, the claim is not that they are the result of group selection or multi-level selection (Smith, 1976; Wilson, 1983, 1975; Dugatkin and Reeve, 1994; Kerr and Godfrey-Smith, 2002; Okasha, 2006; Traulsen et al., 2008; Eldakar and Wilson, 2011). The notion of a distributed adaptation refers to the location of the adaptive information, and not to how this distribution of information and reliance on distributed information came about evolutionarily or its inheritance (cf. Charbonneau, 2014). Put differently, when we talk about a distributed adaptation, we are not attributing fitness to the population or group, and the cases we discuss in this paper are primarily concerned with distributed information that affects the fitness of individuals. Moreover, a population may manifest a distributed adaptation without being a replicator/reproducer (Lloyd, 2016). Indeed, information may even be acquired from different species (Stensland et al., 2003; Sridhar et al., 2009; Farine et al., 2012; Gil et al., 2018), though in this paper we focus primarily on cases involving the dynamics of a single population.
A wide variety of species rely on social information, including fish, birds, and mammals (Danchin et al., 2004; Galef and Laland, 2005; Valone, 2007; Blanchet et al., 2010; Rieucau and Giraldeau, 2011). The social information is used in foraging, in avoiding predators, assessing habitats, and so on [for a review see (Gil et al., 2018)].
Several theoretical notions that have been studied previously are possibly special cases of distributed adaptation. Among them division of labor (Robinson, 1992; Beshers and Fewell, 2001), the skill pool effect (Giraldeau, 1984), strategy choice (Kendal et al., 2009; Rendell et al., 2010), distributed cognition (Hutchins, 1991; Cole and Engeström, 1993), and collective memory (Couzin et al., 2002; De Luca et al., 2014). However, the analyses of these often assume that the information is stored by specific individuals and that it can be transmitted from one individual to another individual, whether through social learning or inheritance. The general case of distributed adaptation considers situations in which the adaptive information cannot be reduced to anything that is possessed by any single individual, whether in their brains or genes. Thus, not all cases of social information involve distributed information in the sense we highlight. Moreover, not all cases of distributed information are cases of distributed adaptation, that is, distributed information is not necessarily adaptive. Finally, distributed adaptations need not involve explicit transmission of information between individuals and may result from their ecological activities, spatial conformation and so on (see Figure 1).
Figure 1. The relations between the theoretical notions of social information, distributed information, and distributed adaptation. Social information does not always involve information that is distributed, in the sense of not being easily partitioned into information stored by specific individuals. Not all cases of distributed information in a population are cases of distributed adaptation. Note that the figure represents only distributed adaptations that involve explicit transmission of information between individuals (see text).
It is useful to consider a couple of these phenomena from a perspective of distributed adaptations. In conformist learning, individuals sample several models and pick what is most frequent to imitate (Henrich and Boyd, 1998; Aplin et al., 2017, 2015; Smaldino et al., 2018). Conformism depends on the prevalence of behaviors and the prevalence is not information possessed by any individual. Thus, it may be instructive in some cases to consider conformism as a specific case of distributed adaptation in which there are two pieces of information that play an adaptive role: the behavior and its prevalence. The information about prevalence is distributed and conformist learning is a sampling mechanism for (approximately) acquiring it. Making this distinction explicit may for example be productive in cases in which conformism interacts in interesting ways with population structure and ecological context.
Collective memory refers to cases in which the population as a whole remembers and is able to access information that exceeds the memory of individual members. A typical set of examples involves memories of older individuals, produced by longer experience or experience of different environmental conditions (Morales et al., 2010). It may be possible for the population to utilize such information, by imitating the older individuals, when an adverse condition reoccurs (e.g., Kaczensky et al., 2011). There are two questions that can help distinguish between cases of collective memory and distributed adaptations. (1) Can the information be acquired from a single individual (e.g., one older individual)? (2) Does the information persist in the population through chains of individuals, or does it involve interactions between multiple individuals or group level behaviors (e.g., flocking, collective grazing)? In practice, many cases of collective memory are to some extent also cases of a distributed adaptation. For example, consider a scenario in which the information about an adaptive destination of migration in a certain rare event is carried by individuals. However, to reach a decision to carry out such a migration, the group might need to make a quorum decision, requiring that several experienced individuals all support the unusual direction of migration (Conradt and Roper, 2005; Couzin et al., 2005; Harel et al., 2021).
Other phenomena that partially overlap with distributed adaptations are cases of local enhancement and conspecific cuing (Muller et al., 1997; Greene and Stamps, 2001; Doligez et al., 2003; Donahue, 2006; Rendell et al., 2011; Galef, 2013; Arbilly and Laland, 2014), that consider dynamics in which individuals are attracted to localities in which conspecifics occur or engage in certain behaviors (feeding or mating for example). These cases often overlap with cases of distributed adaptation—as in the frog example provided above, which can be described in these terms. We suggest that the notion of distributed adaptations is useful even when a phenomenon is well-described by the concepts of local enhancement or conspecific cueing: the latter are typically invoked to explain behavior, to provide an account of a behavioral mechanism. One might debate, for example, whether chickadees learn to open milk bottles via individual trial and error coupled with local enhancement, or whether true imitation is involved in the process (Fisher and Hinde, 1949; Sherry and Galef, 1984; Aplin et al., 2013). Local enhancement and conspecific cueing are rarely invoked to describe an evolutionary adaptation of a species to its environment. In other words, the different terms invoke consideration of implications on different time scales and in different contexts. Local enhancement is only rarely invoked when planning reintroduction programs of a species into the wild, for example, while the concept of distributed adaptation lends itself naturally to such contexts, providing a useful framework for the explicit treatment of this important facet of population success (Reed and Dobson, 1993; Dobson and Poole, 1998; Ahlering and Faaborg, 2006; Halpern et al., 2007; James et al., 2015). More speculatively, this discussion suggests that local enhancement can lead to population-level results that may be studied using the notion of distributed adaptation.
In a similar vein, distributed adaptations have much in common, and in some cases overlap, with phenomena that are described and studied in complex systems’ research: self-organization, collective behavior, and emergent properties (Green, 1993; Lansing and Kremer, 1993; Parrish et al., 2002; Couzin and Krause, 2003; Goldstone and Gureckis, 2009; Lukeman et al., 2010). Here, too, we suggest that the different concepts implicitly invoke different perspectives: research in the field of complexity focuses on the explanation of observed behaviors of dynamical systems, such as patterns that emerge from the interaction of multiple individuals without explicit coordination. They are not readily translatable to discussion of a species’ robustness to environmental change or to risk assessments of bottlenecked populations. They are also not primarily concerned with the evolutionary dynamics of interchange between individual level and distributed adaptation. Due to limitations of scope, a full analysis of the relation between distributed adaptations and these many related notions will await future research.
Finally, there are many instances in which achievement of adaptive goals relies on joint action by multiple individuals, possibly involving distributed information or communication for successful coordination. These include, for example, coordinated attack of trees by bark beetles (Chiu et al., 2018; Toffin et al., 2018), or pack hunting among wolves (Mech, 2007). Phenomena such as these are often studied under the heading of collective action. It is debatable whether all these cases should be considered to be DAs. We put off this discussion for future work but offer the following preliminary observations. The key feature of DA is that there is something interesting in the way that the population and its structure are the substrate for the adaptation of the species to its environment. Cases in which the number or density of organisms each doing their own thing explain the observed behavior are probably best viewed as degenerate cases of DA. Distributed, heterogeneous, behaviors are better signs of a DA. Two characteristics in particular may suggest that the DA perspective would be useful in a certain context. First, that there is good reason to consider the phenomenon to be an adaptation to specific life challenges or to have evolved in such a context. Second, that there are specific, non-trivial, individual traits or a population structure that are necessary for the collective result. Whether the DA perspective is useful also depends on the question of interest. For example, group hunting of colobus monkeys by chimpanzees (Boesch and Boesch, 1989) may be analyzed in the context of social roles, in which case the populational perspective of DA is perhaps less useful. It would be insightful, however, if the question of interest is the population’s ability to recover from demographic bottlenecks, for example.
Many categories of adaptation that we propose to view as distributed adaptations are well-known. What, then, is the added value of introducing a new concept?
We propose that this view is useful in assessing an adaptation’s evolutionary stability and in predicting evolutionary dynamics. As noted earlier, distributed adaptations may readily arise, founded upon small adjustments of existing sensory and behavioral abilities of the species. However, due to the utter reliance on the environment and the population, distributed adaptations are highly sensitive to changes, and may readily fail. They can be viewed as a special category of niche specialization; it has been suggested that high niche specialization is prone to leading to evolutionary dead-ends (Haldane, 1951; Kelley and Farrell, 1998; Nosil and Mooers, 2005; Vamosi et al., 2014). Similarly, distributed adaptations are prone to collapse due to environmental instability. On the other hand, in certain cases distributed adaptations may be more robust than other adaptations, thanks to the redundancy provided by the population. In addition, when the behavior of organisms in the population dynamically adjusts to a changing or uncertain environment, the behavior at the population level may act as a “sensing device” that individuals can make use of as illustrated by the examples in section 2.
It is helpful to consider the evolutionary interaction between distributed and non-distributed adaptations to the same challenge: distributed adaptations can be viewed as cases in which crucial aspects of a species’ survival were “outsourced” to the environment, such as when a species loses innate knowledge about utilization of a food resource and comes to rely on social learning of the information. The opposite also occurs, of course: the Baldwin effect (Baldwin, 1896; Weber and Depew, 2003; Crispo, 2007; Scheiner, 2014), or other cases of genetic assimilation, are in some cases distributed adaptations that are being replaced by individual-level adaptive information (Simpson, 1953; Sznajder et al., 2012). These evolutionary dynamics may interact with long-term selection processes and with species’ demographic history to determine species’ evolutionary trajectories and provide insight, for example, on the conditions that may foster or inhibit the emergence of cultural adaptation, or the evolution of learning and teaching abilities.
To illustrate possible implications of distributed adaptations, we will make use of a simple model. Consider a case in which the phenotype of individual i, pi, depends on the number of individuals in the population that have a desired phenotype T0. The idea is that T0 is the phenotype that other individuals express allowing the focal individual to acquire the distributed information. In the example of the frogs navigating toward croaking conspecifics, T0 is the croaking when in the ponds while pi is effective navigation to ponds. The phenotype pi is suboptimal and the population declines unless the population size is larger than A, in which case enough individuals express the required phenotype T0. The phenotype becomes increasingly better, up to a point in which additional individuals are not required to “carry” the distributed adaptation. In other words, the population needs at least A individuals with the phenotype T0. We assume that the average phenotype and fitness depends on the number of individuals with the required phenotype, so that on average the number of offspring is below 1 until N≥A and increases asymptotically to the maximal reproductive success when N > A.
The Allee effect is used to describe cases in which the mean individual fitness increases with population size or density (Stephens and Sutherland, 1999; Stephens et al., 1999; Deredec and Courchamp, 2007). Different mechanisms have been proposed to drive Allee effects, but the causality is often unclear. Distributed adaption may explain Allee effects in some cases: in distributed adaptation, the ability of the population to “store” the adaptive information depends on the size of the population, since the information is stored in a distributed manner across it.
The toy model above illustrates a strong Allee effect driven by distributed adaptation. This is the simplest scenario, used for demonstration, and shows that interesting dynamics may emerge when adaptive information is distributed. The resulting population growth curve, under this model of a simple distributed adaptation, matches that of a typical Allee Effect (Figure 2).
Figure 2. An Allee effect produced because of distributed adaptation. When population size is smaller than A, not enough individuals express T0 and the fitness is below 1. When the population size is greater than A the distributed information is expressed by the population and the reproductive success of individuals increases asymptotically to the maximal reproductive success, in check by competition for resources.
The consequences of a population bottleneck depend on whether the new population size N′ is smaller or larger than the minimal required number A of individuals needed for the distributed adaption. Some environmental changes that lead to a population bottleneck may involve a new, smaller A′ (e.g., when the environment becomes less complex), while in general a population bottleneck does not change the required number of individuals, A. For illustration, consider two scenarios.
Here the population size is decreased, but the environment is otherwise unchanged, for example: N′ = N/2, while the carrying capacity k and A remain fixed.
In this scenario, the population bottleneck co-occurs with moving to an environment that is manageable with less outsourced information, and hence a smaller A. For example, a smaller niche, but with more resources per unit area, will have a denser population, thus increasing the probability of interactions between individuals, which reduces the number of individuals with the required phenotype that are needed to make acquisition from others robust. A second case in which A decreases is one in which the new environment is simpler in the sense that there is less information that needs to be stored by the population (e.g., more ponds, which are hence easier for the frogs to locate). In this kind of population bottleneck, for example, N′ = N/2, A′ = A/2, while k is unchanged.
If N′ > k′ the population will collapse to k′. However, if N′ < k′ the fate of the population depends on the relation between N′ and A′: if N′ > A′ the population will increase to k′ while if N′ < A′ the population will decrease to 0. The key to the dynamics is whether A decreases in line with the decrease in N. The scenarios above suggest two cases in which this may happen: when the new environment is simpler or when increased population density makes the distributed information storage more efficient (thus requiring less individuals) than in the original environment. Both cases depend on the specific nature of the information outsourced and the properties of the organism that affect acquisition.
This analysis shows why a small population (e.g., a species introduced to nature) may benefit from an artificial environment that is enriched, or simpler (thus, lower A), until the population increases enough in size to be introduced to an environment which requires a larger minimal population. However, that may not be enough: if the population needs to organize to capture/represent the adaptive distributed information, it may be necessary to support the population for an interim period, until this is achieved. Note that these two things would have to follow each other: a population that is too small will not be able to capture the required information even if supported exogenously.
Distributed adaptation also suggests a possible explanation of a gradual adaptation to the environment in invasive species. Many species are characterized by an invasion time lag: a long period of limited success between the time point of establishment and until they become invasive, spreading rapidly and driving significant changes to the ecosystem (Crooks, 2005; Coutts et al., 2018). There are different explanations to what causes this waiting period and what ends it; the notion of distributed adaptations adds the possibility that it takes time for the distributed information to emerge or build up, possibly requiring the invasive population to be large enough. This is not unlike niche construction.
The model discussed so far illustrated the effects of a DA on the success of a population in various scenarios. We now turn to the evolutionary interaction between distributed and non-distributed adaptations to the same challenge. We can think of an organism evolving to use external adaptive information from the population or resulting from the activities of the population, instead of relying on genetic information, as externalizing the information. Conversely, moving to rely on genetic information instead of distributed information may be viewed as internalizing the adaptive information. Internalizing and externalizing both have potential costs and benefits. Relying on a DA may lead to fragility and is sensitive to changes in population size and bottlenecks, as illustrated above. Its benefits include the advantages of redundancy and fault-tolerance more broadly, since the organism does not rely on one copy of the information (i.e., its own genome) but rather on multiple individuals in the population, which would typically mean not relying on any specific individual. Externalizing may in some cases allow the organism to reduce the size of its genome or to save the cost of development and maintenance of morphological or neural substrates that would have otherwise been necessary. Thus, for example, if the non-DA solution requires complicated behaviors or trial-and-error learning, the DA may allow reduction of the size and complexity of the nervous system. In other cases, the non-DA solution may necessitate a specialized sensing apparatus and neural substrate that supports its operation, whose maintenance can be spared if the DA relies on other substrates that must be maintained anyway (the frog and the nymph examples illustrate how this could work). Two important potential benefits of DAs are that they reduce the cost of exploration for the individual, since exploration costs are divided across the population, and that DA may change more quickly in response to environmental changes, in comparison to genetic changes (i.e., mutation and selection) or individual learning.
The costs and benefits of internalization are the mirror image of this. Relying on genetically endowed abilities makes the individual less sensitive to what others are doing, to evolutionary cheating, to conflicts of interest, and so on. Internalization is also a way to decrease the time and resources needed to collect information or cues from the population. This may have an advantage for the individual as illustrated in the discussion of reintroduction and of invasive species above. It may also be advantageous at the population level, increasing in some cases its chances of responding to changes and recovering from bottlenecks.
One situation that may lead to externalization is relaxed selection (Lahti et al., 2009; Hunt et al., 2011; Schrader et al., 2021). Consider as an example the frog scenario and assume that there are two genes: gene A contributing to the ability of the frog to navigate the terrain in search of ponds (think of this as the non-DA solution) and gene B that allows the frogs to go in the direction of croaking (this gene may for example be involved in mating). If the population is large enough such that croaking is a reliable signal for the location of ponds, differences in the navigation abilities (i.e., in gene A) would not significantly affect the individual frogs’ fitness. This relaxed selection on A may lead the gene to accumulate mutations and potentially cease being functional. Thus, the frogs would end up relying exclusively on going in the direction of croaking sounds to find ponds. The individual level adaptation represented by gene A is thus replaced by a DA.
The opposite may also happen. For example, if at some point during the evolutionary scenario just described the population dwindles, making the croaking sounds less useful for navigation, selection in favor of better navigation skills (gene A) would become stronger and an individual-level adaptation may spread and fix. Assume further that the terrain is stable enough that a navigation strategy is clearly superior to others and that relying on sounds may lead to mistakes, perhaps because a related species makes similar croaking sounds but inhabits drier areas. In such a case there would be selection against relying on the external information.
A variety of properties of the population may affect phenotypes that are influenced by outsourced information. In the examples above we illustrated the role of population size. Roughly put, if relevant information is somehow encoded in the composition, structure, or dynamics of the population, the size of the population may be too small to carry the amount of information needed for the adaptation, while a large population may possibly encode information more robustly than small ones (e.g., by sheer redundancy). Demographic collapse may lead distributed information to be lost, which cannot simply be recreated when population size increases. The following may all be affected by population size: the stability of the traits over the lifetime of individuals; the heritability of traits; the quality of the trait (e.g., larger population produce better distributed adaptation); whether a population maintains a stable population level distributed adaptation, and in consequence maintains a viable population size. We focus here on population size per se for clarity, however, additional population-level features may have related and partially overlapping effects. These include population structure and networks of interaction, on a broad range of timescales, from day-to-day interactions to rare events of inter-population migration that occur once in a generation, for example. Similarly, life history and demographic structure may influence distributed adaptations, and distributed adaptations may even influence selection on these traits in certain conditions. Thus, for example, the robustness of a distributed adaptation may be strongly influenced if individuals at different ages contribute to the dynamics related to it differently.
Whether an equilibrium between relying on individual level or distributed adaptation exists and in what contexts natural selection can fine-tune the distribution of an adaptation are currently open questions. Table 1 summarizes the factors discussed in this section.
The notion of distributed adaptations introduced in this paper complements and generalizes other evolutionary notions, including social information, niche construction and developmental niche construction, distributed skills and the skill pool effect, and work on strategy choice and conformism. It directs our theoretical focus to the role of information that is distributed in the population and its ecological conformation. Finally, we suggest that distributed adaptations have implications for conservation biology and in particular for re-introduction efforts.
We illustrated the notion of distributed adaptation with two simplified but realistic examples: frogs navigating an arid terrain and nymphs color choice. The first adaptation makes use of the spatial distribution of the population and the second makes use of the population as a way to sense the frequency of predators. Crucially, in both cases no single individual has the adaptive information, and cannot store it, transmit it to others etc. Yet in both these cases the evolutionary beneficiary is the individual and it is adaptive for individuals to make use of the distributed information.
By taking seriously the consequences of evolutionary relevant information that transcends an individual brain or genome, and that is encoded in interaction networks and environmental conformation, a coevolutionary approach to distributed adaptations offers explanatory traction regarding the effects of population size and dynamics, environmental stability, and properties of information acquisition, in particular its reliability. This suggests general answers as to why and when information may be distributed as well as concerning the natural history of distributed adaptations. Our informal examples suggested an important role for sensory pre-adaptations that allow individuals to profit from being sensitive to the conditions of the population. This perspective may also help identify the factors that explain the variety of distributed information storage phenomena.
More generally, a formal framework of distributed adaptations connects population level and individual properties to derive specific predictions about the cost/benefits that affect internalization and externalization of information, and the resulting evolutionary processes (see Table 1). It sheds light on dynamics that exist in various evolutionary models of social and cultural evolution, but that so far have not been addressed under a unified framework.
An important theoretical insight stemming from the notion of distributed adaptations is that evolutionary relevant information that is distributed in the population can spontaneously emerge once individuals can utilize such information. This need not require selection between groups. We illustrated this by showing that when information is available as a result of the typical activities of members of the population it can be evolutionarily beneficial for individuals to make use of them in favor of more costly, individual-level adaptations. Distributed adaptations may lead to coevolutionary dynamics between traits that rely on outsourced information and traits that improve the ability of a population to serve as a distributed information store. One significant example in human evolution may be coevolutionary dynamics between emotional control, which facilitates social learning, and individual learning: Better emotional control, within well-functioning groups, will reduce the selection pressure on individual problem-solving, thereby in return increasing the selection pressure for emotional control.
The analysis of distributed adaptation suggests that in addition to the types of evolutionary relevant individual information that have been discussed, specifically the genetic, epigenetic, behavioral and symbol information systems (Jablonka and Lamb, 2014), we should pay attention to ways population-level information systems evolve. This study may benefit from ideas developed by computer scientists studying distributed systems (Lynch, 1996). The notion of a memory hierarchy has been developed to classify computer storage systems according to their characteristics (primarily, response time), with the design goal of storing data using the lowest-cost components, that still allow the system to satisfy requirements (Hennessy and Patterson, 2011). Mutatis mutandis, similar things may happen in evolution: when considering costs, we should take into account the time and selection required to achieve an individual adaptation compared to the alternative distributed adaptation, the robustness or fragility of the individual versus the distributed adaptation, and so on. Genome size and genomic architecture may in some cases be constraints, for example, increasing the likelihood of the evolution or stability of one type of adaptation or the other, e.g., by facilitating the acquisition of new functions by existing mechanisms or hindering it. Similarly, selection pressures on the size or complexity of the brain may favor either the individual or the distributed route (Table 1).
The notion of DA highlights that information can be distributed in various ways (in individuals, in the entire population, in different population structures). The costs and benefits change, and the question is how and whether this distribution is subject to evolutionary change, in particular tuning by natural selection. e.g., tradeoffs between genome size or central nervous system complexity and population size.
Moreover, the notion of DA will hopefully inspire more biologists to consider theoretical constraints on what distributed systems with failure can do. For example, a classic result shows that a system may not reach consensus if more than a third of the agents give conflicting information to different agents (see Lynch, 1996). While a biological population should not typically be considered a “system” in the sense used in computer science, distribution of information and the need to integrate distributed information raises similar issues and the notion of DA may bring these issues to the attention of biologists.
The notion of niche construction was introduced to highlight that the fit between an organism and the environment stems not only from information flowing through selection “into” the genome of the organism but also from information that flows from the organism to the environment (Odling-Smee et al., 2003). The notion of developmental niche construction was meant to capture the fact that the constructed niche affects not only the fitness of individuals but also their development (Stotz, 2017). The notions suggested here extend these discussions. Distributed adaptations are cases in which the developmental trajectory of individuals is affected by the exhibited phenotypes in the population, which may change over time, by the distribution of phenotypes, as well by as the conformation of the population in the environment. However, as noted earlier, in the cases we highlighted there are no persistent changes to the environment, as found in niche construction, that indirectly affect the selection pressure affecting individuals in the population. In contrast, in the cases we highlighted individuals make direct use of the distributed information and integrate multiple pieces of information.
Major Transitions in Evolution were characterized by Maynard Smith and Szathmáry as transitions creating a new level of individuality and new kinds of information systems, that is, new ways of storing, transmitting, and interpreting information (Jablonka and Lamb, 2006; Robin et al., 2021). Our paper focuses on the latter. While it was suggested that these two dimensions should be treated separately, one assumption in particular remained prevalent in most subsequent work. Namely, the view that information is at the level of individuals. In other words, the information that is primarily tracked in such studies, whether theoretical or empirical, is information that can be stored and transmitted by and between individuals. This assumption may explain why it is tempting in studies of evolutionary transitions in individuality to wonder if new kinds of individuals are coupled with new kinds of information. Be that as it may, our discussion emphasizes and characterizes a supplementary perspective: cases in which adaptive information strictly transcends the level of individuals. It may even be thought of as a level of individuality, though one that is distinct from the biological individuality of individual organisms.
Two aspects of our characterization of DA may have implications for thinking about evolutionary transitions. DAs are cases in which (1) the adaptive information is distributed, and hence strictly the property of a population and (2) the information may arise from the ecological conformation of the population. We discussed the evolutionary implications of DA in section 4. As regards evolutionary transitions in particular, two possibilities are worth considering. First, DAs may contribute to the irreversibility of transitions. We noted that relying on a DA may affect selection pressures, causing relaxed selection on traits that allow the individual to be self-sufficient, possibly leading to the external information becoming increasingly necessary and the reliance on it mandatory. Change along this continuum may make the possibility of reverting to prior organization less likely. Second, since distributed information may arise spontaneously and may provide ecological information that may be otherwise hard to acquire, they may be an enabling factor that facilitates a transition or in some cases a necessary step, that is, they may be part of what Robin et al. (2021) call a Facilitating Evolutionary Transition.
An early example of a transition from distributed to non-distributed information may be what Carl Woese referred to as the Darwinian Threshold in the evolution of the cell (Woese, 2002). In this scenario, proto-cells relied heavily on horizontal gene transfer, but as the complexity of cells increased this became too unreliable, and vertical transmission became dominant. Woese referred to this as a phase transition. While this scenario has many unique features, not found in other cases, the evolutionary dynamics suggested by Woese are not entirely dissimilar from what we describe.
Two other transitions are worth mentioning here. The first, not included in Maynard Smith and Szathmáry’s original list, is the evolution of a nervous system (Jablonka and Lamb, 2006). The existence of a nervous system and sensory perception greatly increased the possibility of relying on distributed information, whether intentionally shared or not. The second is the emergence of symbolic language. Language obviously radically increases the opportunities to learn from others and is fundamental for cumulative culture in humans, aspects of which are arguably DAs. While it is beyond our scope to discuss language and its evolution (but see Lamm, 2014; Kolodny and Edelman, 2018), it is worth noting that recent work has identified relations between group size and properties of language, as would be expected from our discussion of DA (Lupyan and Dale, 2010; Atkinson et al., 2018; Raviv et al., 2019). An intriguing aspect of language as an information system is that it transcends a particular group and several groups may share languages while individuals may be multi-lingual (Evans, 2017). By acquiring a language one does not only increase the opportunities to communicate but also acquires information that is implicit in the structure of the language itself, such as categories of objects or events. This is arguably an additional way in which information distribution occurs in humans.
Robin et al. (2021) characterize five levels of information in the context of evolutionary transitions. This characterization allows to differentiate several kinds of DA. The frog example which we discussed throughout the paper belongs to what they call Level III. This level refers to learned information, collected during the lifetime of individuals. Other cases of DA would more naturally fall under Level IV, which refers to inscribed or iconic information. The information at this level is transmitted using somewhat persistent physical marks, as in scent marks in wolves or pheromone trails in ants. This level also includes instructional information, of potentially boundless capacity, that is primarily found in humans. These cases are forms of distributed information or can be used to create distributed information, in the sense developed here. A key aspect of the Level IV phenomena as DA is that the objects that carry the information may be themselves studied as a population of entities, whose dynamics are coupled with those of the population of organisms that create, use, and possibly destroy them.
To conclude, distributed adaptations may be a stage in transitions in individuality, possibly because of the fragility of the distributed adaptation vis-à-vis the environment (Szathmary and Smith, 1995; Smith and Szathmary, 1997; Michod, 2000; Jablonka and Lamb, 2006; Calcott and Sterelny, 2011; Szathmáry, 2015). A distributed adaptation means that multiple individuals gain shared benefits from maintaining the distributed information. Given appropriate conditions, this may lead to the evolution of stronger ties and mechanisms to decrease the fragility of the distributed adaption, possibly leading to a transition.
The original contributions presented in the study are included in the article/supplementary material, further inquiries can be directed to the corresponding author/s.
Both authors contributed to the study equally, conceptualizing the paradigm and writing the manuscript together.
OK was supported by the US–Israel Binational Science Foundation (BSF) and the Israel Science Foundation (ISF; grant 1826/20).
The authors declare that the research was conducted in the absence of any commercial or financial relationships that could be construed as a potential conflict of interest.
All claims expressed in this article are solely those of the authors and do not necessarily represent those of their affiliated organizations, or those of the publisher, the editors and the reviewers. Any product that may be evaluated in this article, or claim that may be made by its manufacturer, is not guaranteed or endorsed by the publisher.
We would like to thank the organizers and participants of the Batsheva De Rothschild interdisciplinary workshop “Is humanity undergoing an evolutionary transition?” (September 2019, Israel), and particularly Ayelet Shavit and Yohay Carmel, for inspiration and helpful comments.
Ahlering, M. A., and Faaborg, J. (2006). Avian habitat management meets conspecific attraction: if you build it, will they come? Auk 123, 301–312. doi: 10.1093/auk/123.2.301
Aplin, L. M., Farine, D. R., Morand-Ferron, J., Cockburn, A., Thornton, A., and Sheldon, B. C. (2015). Experimentally induced innovations lead to persistent culture via conformity in wild birds. Nature 518, 538–541. doi: 10.1038/nature13998
Aplin, L. M., Sheldon, B. C., and McElreath, R. (2017). Conformity does not perpetuate suboptimal traditions in a wild population of songbirds. Proc. Natl. Acad. Sci. U.S.A. 114, 7830–7837. doi: 10.1073/pnas.1621067114
Aplin, L. M., Sheldon, B. C., and Morand-Ferron, J. (2013). Milk bottles revisited: social learning and individual variation in the blue tit, Cyanistes caeruleus. Anim. Behav. 85, 1225–1232. doi: 10.1016/j.anbehav.2013.03.009
Arbilly, M., and Laland, K. N. (2014). The local enhancement conundrum: in search of the adaptive value of a social learning mechanism. Theor. Popul. Biol. 91, 50–57. doi: 10.1016/j.tpb.2013.09.006
Atkinson, M., Smith, K., and Kirby, S. (2018). Adult learning and language simplification. Cogn. Sci. 42, 2818–2854. doi: 10.1111/cogs.12686
Bee, M. A. (2007). Selective phonotaxis by male wood frogs (Rana sylvatica) to the sound of a chorus. Behav. Ecol. Sociobiol. 61, 955–966. doi: 10.1007/s00265-006-0324-8
Beshers, S. N., and Fewell, J. H. (2001). Models of division of labor in social insects. Annu. Rev. Entomol. 46, 413–440. doi: 10.1146/annurev.ento.46.1.413
Blanchet, S., Clobert, J., and Danchin, E. (2010). The role of public information in ecology and conservation: an emphasis on inadvertent social information. Ann. N. Y. Acad. Sci. 1195, 149–168. doi: 10.1111/j.1749-6632.2010.05477.x
Boesch, C., and Boesch, H. (1989). Hunting behavior of wild chimpanzees in the Tai National Park. Am. J. Phys. Anthropol. 78, 547–573. doi: 10.1002/ajpa.1330780410
Buckley, N. J. (1996). Food finding and the influence of information, local enhancement, and communal roosting on foraging success of North American vultures. Auk 113, 473–488. doi: 10.2307/4088913
Calcott, B., and Sterelny, K. (2011). The Major Transitions in Evolution Revisited. Cambridge, MA: MIT Press. doi: 10.7551/mitpress/9780262015240.001.0001
Charbonneau, M. (2014). Populations without reproduction. Philos. Sci. 81, 727–740. doi: 10.1086/677203
Chiu, C. C., Keeling, C. I., and Bohlmann, J. (2018). Monoterpenyl esters in juvenile mountain pine beetle and sex-specific release of the aggregation pheromone trans-verbenol. Proc. Natl. Acad. Sci. U.S.A. 115, 3652–3657. doi: 10.1073/pnas.1722380115
Cole, M., and Engeström, Y. (1993). “A cultural-historical approach to distributed cognition,” in Distributed cognitions: Psychological and Educational Considerations, ed. G. Salomon (New York, NY: Cambridge University Press), 1–46.
Conradt, L., and Roper, T. J. (2005). Consensus decision making in animals. Trends Ecol. Evol. 20, 449–456. doi: 10.1016/j.tree.2005.05.008
Coutts, S. R., Helmstedt, K. J., and Bennett, J. R. (2018). Invasion lags: the stories we tell ourselves and our inability to infer process from pattern. Divers. Distrib. 24, 244–251. doi: 10.1111/ddi.12669
Couzin, I. D., and Krause, J. (2003). Self-organization and collective behavior in vertebrates. Adv. Study Behav. 32, 1–75. doi: 10.1016/S0065-3454(03)01001-5
Couzin, I. D., Krause, J., Franks, N. R., and Levin, S. A. (2005). Effective leadership and decision-making in animal groups on the move. Nature 433, 513–516. doi: 10.1038/nature03236
Couzin, I. D., Krause, J., James, R., Ruxton, G. D., and Franks, N. R. (2002). Collective memory and spatial sorting in animal groups. J. Theor. Biol. 218, 1–11. doi: 10.1006/jtbi.2002.3065
Crispo, E. (2007). The Baldwin effect and genetic assimilation: revisiting two mechanisms of evolutionary change mediated by phenotypic plasticity. Evol. Int. J. Org. Evol. 61, 2469–2479. doi: 10.1111/j.1558-5646.2007.00203.x
Crooks, J. A. (2005). Lag times and exotic species: the ecology and management of biological invasions in slow-motion1. Ecoscience 12, 316–329. doi: 10.2980/i1195-6860-12-3-316.1
Danchin, E., Giraldeau, L.-A., Valone, T. J., and Wagner, R. H. (2004). Public information: from nosy neighbors to cultural evolution. Science 305, 487–491. doi: 10.1126/science.1098254
De Luca, G., Mariani, P., MacKenzie, B. R., and Marsili, M. (2014). Fishing out collective memory of migratory schools. J. R. Soc. Interface 11:20140043. doi: 10.1098/rsif.2014.0043
Degani, G. (2015). The habitats, burrowing behavior, physiology adaptation and life cycle of Spadefoot toads (Pelobates syriacus, Boettger, 1869) at the southern limit of its distribution in Israel. Open J. Anim. Sci. 5:249. doi: 10.4236/ojas.2015.53029
Degani, G. (2016). Life cycle of tree frogs (Hyla savygnyi) in semi-arid habitats in northern Israel. Int. J. Biol. 8:17. doi: 10.5539/ijb.v8n1p17
Deredec, A., and Courchamp, F. (2007). Importance of the Allee effect for reintroductions. Ecoscience 14, 440–451. doi: 10.2980/1195-6860(2007)14[440:IOTAEF]2.0.CO;2
Dobson, A., and Poole, J. (1998). “Conspecific aggregation and conservation biology,” in Behavioral Ecology and Conservation Biology, ed. T. Caro (Oxford: Oxford University Press), 193–208.
Doligez, B., Cadet, C., Danchin, E., and Boulinier, T. (2003). When to use public information for breeding habitat selection? The role of environmental predictability and density dependence. Anim. Behav. 66, 973–988. doi: 10.1006/anbe.2002.2270
Donahue, M. J. (2006). Allee effects and conspecific cueing jointly lead to conspecific attraction. Oecologia 149, 33–43. doi: 10.1007/s00442-006-0419-y
Dugatkin, L. A., and Reeve, H. K. (1994). Behavioral ecology and levels of selection: dissolving the group selection controversy. Adv. Study Behav. 23, 101–133. doi: 10.1016/S0065-3454(08)60352-6
Eldakar, O. T., and Wilson, D. S. (2011). Eight criticisms not to make about group selection. Evol. Int. J. Org. Evol. 65, 1523–1526. doi: 10.1111/j.1558-5646.2011.01290.x
Evans, N. (2017). Did language evolve in multilingual settings? Biol. Philos. 32, 905–933. doi: 10.1007/s10539-018-9609-3
Farine, D. R., Garroway, C. J., and Sheldon, B. C. (2012). Social network analysis of mixed-species flocks: exploring the structure and evolution of interspecific social behaviour. Anim. Behav. 84, 1271–1277. doi: 10.1016/j.anbehav.2012.08.008
Fisher, J. B., and Hinde, R. A. (1949). The opening of milk bottles by birds. Br. Birds 42, 347–357.
Fouquet, A., Tilly, T., Pašukonis, A., Courtois, E. A., Gaucher, P., Sebastian Ulloa, J., et al. (2021). Simulated chorus attracts conspecific and heterospecific Amazonian explosive breeding frogs. Biotropica 53, 63–73. doi: 10.1111/btp.12845
Galef, B. G. (2013). Imitation and local enhancement: detrimental effects of consensus definitions on analyses of social learning in animals. Behav. Processes 100, 123–130. doi: 10.1016/j.beproc.2013.07.026
Galef, B. G., and Laland, K. N. (2005). Social learning in animals: empirical studies and theoretical models. Bioscience 55, 489–499. doi: 10.1641/0006-3568(2005)055[0489:SLIAES]2.0.CO;2
Gil, M. A., Hein, A. M., Spiegel, O., Baskett, M. L., and Sih, A. (2018). Social information links individual behavior to population and community dynamics. Trends Ecol. Evol. 33, 535–548. doi: 10.1016/j.tree.2018.04.010
Giraldeau, L.-A. (1984). Group foraging: the skill pool effect and frequency-dependent learning. Am. Nat. 124, 72–79. doi: 10.1086/284252
Goldber, T., Nevo, E., and Degani, G. (2009). Breeding site selection according to suitability for amphibian larval growth under various ecological conditions in the semi-arid zone of northern Israel. Ecol. Mediterr. 35, 65–74. doi: 10.3406/ecmed.2009.1389
Goldstone, R. L., and Gureckis, T. M. (2009). Collective behavior. Top. Cogn. Sci. 1, 412–438. doi: 10.1111/j.1756-8765.2009.01038.x
Greene, C. M., and Stamps, J. A. (2001). Habitat selection at low population densities. Ecology 82, 2091–2100. doi: 10.1890/0012-9658(2001)082[2091:HSALPD]2.0.CO;2
Halpern, B. S., Silliman, B. R., Olden, J. D., Bruno, J. P., and Bertness, M. D. (2007). Incorporating positive interactions in aquatic restoration and conservation. Front. Ecol. Environ. 5:153–160. doi: 10.1890/1540-9295(2007)5[153:IPIIAR]2.0.CO;2
Harel, R., Loftus, J. C., and Crofoot, M. C. (2021). Locomotor compromises maintain group cohesion in baboon troops on the move. Proc. R. Soc. B 288:20210839. doi: 10.1098/rspb.2021.0839
Hennessy, J. L., and Patterson, D. A. (2011). Computer Architecture: A Quantitative Approach. Amsterdam: Elsevier.
Henrich, J., and Boyd, R. (1998). The Evolution of Conformist Transmission and the Emergence of Between-Group Differences. Evol. Hum. Behav. 19, 215–241. doi: 10.1016/S1090-5138(98)00018-X
Houston, D. C. (1974). Food searching in griffon vultures. Afr. J. Ecol. 12, 63–77. doi: 10.1111/j.1365-2028.1974.tb00107.x
Hunt, B. G., Ometto, L., Wurm, Y., Shoemaker, D., Soojin, V. Y., Keller, L., et al. (2011). Relaxed selection is a precursor to the evolution of phenotypic plasticity. Proc. Natl. Acad. Sci. U.S.A. 108, 15936–15941. doi: 10.1073/pnas.1104825108
Hutchins, E. (1991). “The social organization of distributed cognition,” in Perspectives on Socially Shared Cognition, eds L. B. Resnick, J. M. Levine, and S. D. Teasley (Washington, DC: American Psychological Association), 283–307. doi: 10.1037/10096-012
Jablonka, E., and Lamb, M. J. (2006). The evolution of information in the major transitions. J. Theor. Biol. 239, 236–246. doi: 10.1016/j.jtbi.2005.08.038
Jablonka, E., and Lamb, M. J. (2014). Evolution in Four Dimensions, Revised Edition: Genetic, Epigenetic, Behavioral, and Symbolic Variation in the History Of Life. Cambridge, MA: MIT Press. doi: 10.7551/mitpress/9689.001.0001
Jackson, A. L., Ruxton, G. D., and Houston, D. C. (2008). The effect of social facilitation on foraging success in vultures: a modelling study. Biol. Lett. 4, 311–313. doi: 10.1098/rsbl.2008.0038
James, M. S., Stockwell, M. P., Clulow, J., Clulow, S., and Mahony, M. J. (2015). Investigating behaviour for conservation goals: conspecific call playback can be used to alter amphibian distributions within ponds. Biol. Conserv. 192, 287–293. doi: 10.1016/j.biocon.2015.10.001
Kaczensky, P., Ganbataar, O., Altansukh, N., Enkhsaikhan, N., Stauffer, C., and Walzer, C. (2011). The danger of having all your eggs in one basket—winter crash of the re-introduced Przewalski’s horses in the Mongolian Gobi. PLoS One 6:e28057. doi: 10.1371/journal.pone.0028057
Keenan, J. P., and McShea, D. W. (2021). Synergies among behaviors drive the discovery of productive interactions. Front. Ecol. Evol. (in review).
Kelley, S. T., and Farrell, B. D. (1998). Is specialization a dead end? The phylogeny of host use in Dendroctonus bark beetles (Scolytidae). Evolution (N. Y) 52, 1731–1743. doi: 10.2307/2411346
Kendal, J., Giraldeau, L. A., and Laland, K. (2009). The evolution of social learning rules: payoff-biased and frequency-dependent biased transmission. J. Theor. Biol. 260, 210–219. doi: 10.1016/j.jtbi.2009.05.029
Kerr, B., and Godfrey-Smith, P. (2002). Individualist and multi-level perspectives on selection in structured populations. Biol. Philos. 17, 477–517. doi: 10.1023/A:1020504900646
Kolodny, O., and Edelman, S. (2018). The evolution of the capacity for language: the ecological context and adaptive value of a process of cognitive hijacking. Philos. Trans. R. Soc. B Biol. Sci. 373:20170052. doi: 10.1098/rstb.2017.0052
Lahti, D. C., Johnson, N. A., Ajie, B. C., Otto, S. P., Hendry, A. P., Blumstein, D. T., et al. (2009). Relaxed selection in the wild. Trends Ecol. Evol. 24, 487–496. doi: 10.1016/j.tree.2009.03.010
Laland, K. N., Odling-Smee, F. J., and Feldman, M. W. (1999). Evolutionary consequences of niche construction and their implications for ecology. Proc. Natl. Acad. Sci. U.S.A. 96, 10242–10247. doi: 10.1073/pnas.96.18.10242
Laland, K. N., Odling-Smee, J., and Feldman, M. W. (2000). Niche construction, biological evolution, and cultural change. Behav. Brain Sci. 23, 131–146. doi: 10.1017/S0140525X00002417
Lamm, E. (2014). “Forever united: the co-evolution of language and normativity,” in The Social Origins of Language: Studies in the Evolution of Language, eds D. Dor, C. Knight, and J. Lewis (Oxford: Oxford University Press), 267–283. doi: 10.1093/acprof:oso/9780199665327.003.0019
Lansing, J. S., and Kremer, J. N. (1993). Emergent properties of Balinese water temple networks: coadaptation on a rugged fitness landscape. Am. Anthropol. 95, 97–114. doi: 10.1525/aa.1993.95.1.02a00050
Lloyd, E. A. (2016). “Holobionts as units of selection: holobionts as interactors, reproducers, and manifestors of adaptation short title: holobionts as units of selection,” in Landscapes of Collectivity in the Life Sciences. Vienna Series in Theoretical Biology, eds S. B. Gissis, E. Lamm, and A. Shavit (Cambridge, MA: MIT Press).
Lukeman, R., Li, Y.-X., and Edelstein-Keshet, L. (2010). Inferring individual rules from collective behavior. Proc. Natl. Acad. Sci. U.S.A. 107, 12576–12580. doi: 10.1073/pnas.1001763107
Lupyan, G., and Dale, R. (2010). Language structure is partly determined by social structure. PLoS One 5:e8559. doi: 10.1371/journal.pone.0008559
Mech, L. D. (2007). Possible use of foresight, understanding, and planning by wolves hunting muskoxen. Arctic 60, 145–149. doi: 10.14430/arctic239
Michod, R. E. (2000). Darwinian Dynamics: Evolutionary Transitions in Fitness and Individuality. Princeton, NJ: Princeton University Press.
Morales, J. M., Moorcroft, P. R., Matthiopoulos, J., Frair, J. L., Kie, J. G., Powell, R. A., et al. (2010). Building the bridge between animal movement and population dynamics. Philos. Trans. R. Soc. B Biol. Sci. 365, 2289–2301. doi: 10.1098/rstb.2010.0082
Muller, K. L., Stamps, J. A., Krishnan, V. V., and Willits, N. H. (1997). The effects of conspecific attraction and habitat quality on habitat selection in territorial birds (Troglodytes aedon). Am. Nat. 150, 650–661. doi: 10.1086/286087
Nosil, P., and Mooers, A. Ø (2005). Testing hypotheses about ecological specialization using phylogenetic trees. Evolution (N. Y) 59, 2256–2263. doi: 10.1111/j.0014-3820.2005.tb00933.x
Odling-Smee, F. J., Laland, K. N., and Feldman, M. W. (1996). Niche construction. Am. Nat. 147, 641–648. doi: 10.1086/285870
Odling-Smee, F. J., Laland, K. N., and Feldman, M. W. (2003). Niche Construction: The Neglected Process in Evolution. Princeton, NJ: Princeton University Press.
Okasha, S. (2006). Evolution and the Levels of Selection. Oxford: Oxford University Press. doi: 10.1093/acprof:oso/9780199267972.001.0001
Parrish, J. K., Viscido, S. V., and Grunbaum, D. (2002). Self-organized fish schools: an examination of emergent properties. Biol. Bull. 202, 296–305. doi: 10.2307/1543482
Raviv, L., Meyer, A., and Lev-Ari, S. (2019). Larger communities create more systematic languages. Proc. R. Soc. B 286:20191262. doi: 10.1098/rspb.2019.1262
Reed, J. M., and Dobson, A. P. (1993). Behavioural constraints and conservation biology: conspecific attraction and recruitment. Trends Ecol. Evol. 8, 253–256. doi: 10.1016/0169-5347(93)90201-Y
Rendell, L., Boyd, R., Cownden, D., Enquist, M., Eriksson, K., Feldman, M. W., et al. (2010). Why copy others? Insights from the social learning strategies tournament. Science 328, 208–213. doi: 10.1126/science.1184719
Rendell, L., Fogarty, L., Hoppitt, W. J. E., Morgan, T. J. H., Webster, M. M., and Laland, K. N. (2011). Cognitive culture: theoretical and empirical insights into social learning strategies. Trends Cogn. Sci. 15, 68–76. doi: 10.1016/j.tics.2010.12.002
Rieucau, G., and Giraldeau, L.-A. (2011). Exploring the costs and benefits of social information use: an appraisal of current experimental evidence. Philos. Trans. R. Soc. B Biol. Sci. 366, 949–957. doi: 10.1098/rstb.2010.0325
Robin, A. N., Denton, K. K., Horna Lowell, E. S., Dulay, T., Ebrahimi, S., Johnson, G. C., et al. (2021). Major evolutionary transitions and the roles of facilitation and information in ecosystem transformations. Front. Ecol. Evol. 9:711556. doi: 10.3389/fevo.2021.711556
Robinson, G. E. (1992). Regulation of division of labor in insect societies. Annu. Rev. Entomol. 37, 637–665. doi: 10.1146/annurev.en.37.010192.003225
Scheiner, S. M. (2014). The baldwin effect: neglected and misunderstood. Am. Nat. 184, ii–iii. doi: 10.1086/677944
Schrader, L., Pan, H., Bollazzi, M., Schiøtt, M., Larabee, F. J., Bi, X., et al. (2021). Relaxed selection underlies genome erosion in socially parasitic ant species. Nat. Commun. 12:2918. doi: 10.1038/s41467-021-23178-w
Seeley, T. D., Visscher, P. K., and Passino, K. M. (2006). Group decision making in honey bee swarms: when 10,000 bees go house hunting, how do they cooperatively choose their new nesting site? Am. Sci. 94, 220–229. doi: 10.1511/2006.59.220
Sherry, D. F., and Galef, B. G. (1984). Cultural transmission without imitation: milk bottle opening by birds. Anim. Behav. 32, 937–938. doi: 10.1016/S0003-3472(84)80185-2
Simpson, G. G. (1953). The baldwin effect. Evolution (N. Y) 7, 110–117. doi: 10.1111/j.1558-5646.1953.tb00069.x
Smaldino, P. E., Aplin, L. M., and Farine, D. R. (2018). Sigmoidal acquisition curves are good indicators of conformist transmission. Sci. Rep. 8:14015. doi: 10.1038/s41598-018-30248-5
Smith, J. M., and Szathmary, E. (1997). The Major Transitions in Evolution. Oxford: Oxford University Press. doi: 10.1093/oso/9780198502944.001.0001
Sosna, M. M. G., Twomey, C. R., Bak-Coleman, J., Poel, W., Daniels, B. C., Romanczuk, P., et al. (2019). Individual and collective encoding of risk in animal groups. Proc. Natl. Acad. Sci. U.S.A. 116, 20556–20561. doi: 10.1073/pnas.1905585116
Sridhar, H., Beauchamp, G., and Shanker, K. (2009). Why do birds participate in mixed-species foraging flocks? A large-scale synthesis. Anim. Behav. 78, 337–347. doi: 10.1016/j.anbehav.2009.05.008
Stensland, E. V. A., Angerbjörn, A., and Berggren, P. E. R. (2003). Mixed species groups in mammals. Mamm. Rev. 33, 205–223. doi: 10.1046/j.1365-2907.2003.00022.x
Stephens, P. A., and Sutherland, W. J. (1999). Consequences of the Allee effect for behaviour, ecology and conservation. Trends Ecol. Evol. 14, 401–405. doi: 10.1016/S0169-5347(99)01684-5
Stephens, P. A., Sutherland, W. J., and Freckleton, R. P. (1999). What is the Allee effect? Oikos 87, 85–190. doi: 10.2307/3547011
Stotz, K. (2017). Why developmental niche construction is not selective niche construction: and why it matters. Interface Focus 7:20160157. doi: 10.1098/rsfs.2016.0157
Szathmáry, E. (2015). Toward major evolutionary transitions theory 2.0. Proc. Natl. Acad. Sci. U.S.A. 112, 10104–10111. doi: 10.1073/pnas.1421398112
Szathmary, E., and Smith, J. M. (1995). The major evolutionary transitions. Nature 374:227. doi: 10.1038/374227a0
Sznajder, B., Sabelis, M. W., and Egas, M. (2012). How adaptive learning affects evolution: reviewing theory on the Baldwin effect. Evol. Biol. 39, 301–310. doi: 10.1007/s11692-011-9155-2
Toffin, E., Gabriel, E., Louis, M., Deneubourg, J.-L., and Grégoire, J.-C. (2018). Colonization of weakened trees by mass-attacking bark beetles: no penalty for pioneers, scattered initial distributions and final regular patterns. R. Soc. Open Sci. 5:170454. doi: 10.1098/rsos.170454
Traulsen, A., Shoresh, N., and Nowak, M. A. (2008). Analytical results for individual and group selection of any intensity. Bull. Math. Biol. 70, 1410–1424. doi: 10.1007/s11538-008-9305-6
Vaira, M. (2005). Annual variation of breeding patterns of the toad, Melanophryniscus rubriventris (Vellard, 1947). Amphib. Reptilia 26, 193–199. doi: 10.1163/1568538054253519
Valone, T. J. (2007). From eavesdropping on performance to copying the behavior of others: a review of public information use. Behav. Ecol. Sociobiol. 62, 1–14. doi: 10.1007/s00265-007-0439-6
Vamosi, J. C., Armbruster, W. S., and Renner, S. S. (2014). Evolutionary ecology of specialization: insights from phylogenetic analysis. Proc. Biol. Sci. 281:20142004. doi: 10.1098/rspb.2014.2004
Weber, B. H., and Depew, D. J. (2003). Evolution and Learning: The Baldwin Effect Reconsidered. Cambridge, MA: MIT Press.
Wilson, D. S. (1975). A theory of group selection. Proc. Natl. Acad. Sci. U.S.A. 72, 143–146. doi: 10.1073/pnas.72.1.143
Wilson, D. S. (1983). The group selection controversy: history and current status. Annu. Rev. Ecol. Syst. 14, 159–187. doi: 10.1146/annurev.es.14.110183.001111
Keywords: adaptation, major transitions, cultural evolution, social learning, collective behavior, collective memory, collective decision making, information theory
Citation: Lamm E and Kolodny O (2022) Distributed Adaptations: Can a Species Be Adapted While No Single Individual Carries the Adaptation? Front. Ecol. Evol. 10:791104. doi: 10.3389/fevo.2022.791104
Received: 07 October 2021; Accepted: 05 January 2022;
Published: 14 February 2022.
Edited by:
Peter Nonacs, University of California, Los Angeles, United StatesReviewed by:
Daniel Priego Espinosa, University of Kentucky, United StatesCopyright © 2022 Lamm and Kolodny. This is an open-access article distributed under the terms of the Creative Commons Attribution License (CC BY). The use, distribution or reproduction in other forums is permitted, provided the original author(s) and the copyright owner(s) are credited and that the original publication in this journal is cited, in accordance with accepted academic practice. No use, distribution or reproduction is permitted which does not comply with these terms.
*Correspondence: Ehud Lamm, ZWh1ZGxhbW1AcG9zdC50YXUuYWMuaWw=; Oren Kolodny, b3Jlbi5rb2xvZG55QG1haWwuaHVqaS5hYy5pbA==
†These authors have contributed equally to this work
Disclaimer: All claims expressed in this article are solely those of the authors and do not necessarily represent those of their affiliated organizations, or those of the publisher, the editors and the reviewers. Any product that may be evaluated in this article or claim that may be made by its manufacturer is not guaranteed or endorsed by the publisher.
Research integrity at Frontiers
Learn more about the work of our research integrity team to safeguard the quality of each article we publish.