- 1Institutes for Systems Genetics, Frontiers Science Center for Disease-related Molecular Network, West China Hospital, Sichuan University, Chengdu, China
- 2Key Laboratory of Birth Defects and Related Diseases of Women and Children, Ministry of Education, West China Second University Hospital, Sichuan University, Chengdu, China
- 3Department of Pediatric Surgery, West China Hospital, Sichuan University, Chengdu, China
- 4Laboratory of Neurodegenerative Disorders, Department of Neurology, National Clinical Research Center for Geriatrics, West China Hospital, Sichuan University, Chengdu, China
- 5Key Lab of Transplant Engineering and Immunology, Ministry of Health, Regenerative Medicine Research Center, West China-Washington Mitochondria and Metabolism Research Center, West China Hospital, Sichuan University, Chengdu, China
- 6Department of Experimental Animal Center, West China Hospital, Sichuan University, Chengdu, China
Introduction: The microcephaly is a rare and severe disease probably under purifying selection due to the reduction of human brain-size. In contrast, the brain-size enlargement is most probably driven by positive selection, in light of this critical phenotypical innovation during primates and human evolution. Thus, microcephaly-related genes were extensively studied for signals of positive selection. However, whether the pathogenic variants of microcephaly-related genes could affect the regions of positive selection is still unclear.
Methods: Here, we conducted whole genome sequencing (WGS) and positive selection analysis.
Results: We identified novel compound heterozygous variants, p.Y613* and p.E1368K in TUBGCP6, related to microcephaly in a Chinese family. The genotyping and the sanger sequencing revealed the maternal and the paternal origin for the first and second variant, respectively. The p.Y613* occurred before the second and third domain of TUBGCP6 protein, while p.E1368K located within the linker region of the second and third domain. Interestingly, using multiple positive selection analyses, we revealed the potential impacts of these variants on the regions of positive selection of TUBGCP6. The truncating variant p.Y613* could lead to the deletions of two positively selected domains DUF5401 and Spc97_Spc98, while p.E1368K could impose a rare mutation burden on the linker region between these two domains.
Discussion: Our investigation expands the list of candidate pathogenic variants of TUBGCP6 that may cause microcephaly. Moreover, the study provides insights into the potential pathogenic effects of variants that truncate or distribute within the positively selected regions.
Introduction
Autosomal recessive primary microcephaly is a rare genetic disease, in which a baby’s head circumference is much smaller than expected. According to Centers for Disease Control and Prevention (CDC) of the United States, the microcephaly is defined as a head circumference measurement more than two standard deviations (SDs) below the average. In some cases, the head circumference was reported to be over four standard deviations lower than normal at birth (Puffenberger et al., 2012). As a birth defect, microcephaly is estimated to affect 1 in every 800–5,000 babies in United States. In western China, the prevalence was estimated to 3.3 (95% confidence interval 2.8–3.9) per 1,000 live births (Shen et al., 2021). In Europe, the prevalence of microcephaly was 1.53 (95% CI 1.16 to 1.96) per 10,000 births (Morris et al., 2016). The symptoms of microcephaly usually include global developmental delay, motor retardation, and intellectual disability, etc. (Arboleda et al., 2015; Abuduxikuer et al., 2020).
The human microcephaly has long been spotlighted, due to their self-evident importance in mirroring the phenotypic innovation of human as a species (Evans et al., 2004; Montgomery and Mundy, 2014). The rationale underlying these evolutionary interests roots in the brain expansions of primates, especially in human, which are exceptional among mammals. The enlargement of the brain-size is, arguably, the most distinguished hallmark of primate and human evolution. Indeed, compared with other mammals, primates have larger brains after adjusting allometric scaling with body mass (Martin, 1990). Indeed, compared with apes, human has more than 3 times larger brain-size emerged 0.2–0.4 MY ago, leading to high-order cognitions including language (Herculano-Houzel and Kaas, 2011). Strikingly, the brain phenotypes of microcephaly cases are comparable level of early hominids (Wood and Collard, 1999; Kumar et al., 2002). Multiple evolutionary studies have focused on the questions involving whether selection had shaped the evolution of genes related to microcephaly.
One of common procedures of identifying pathogenic variants emphasizes the importance of evolutionary conservation. Indeed, based on the ACMG guideline and numerous genetic studies on rare diseases, evolutionary conservation, which is indicative of purifying selection, is an important criterion for identifying causal variants (Richardson et al., 2020). This “rule of thumb” seems rational because the deep evolutionary conservation across species indicates the fundamental importance of, and thus the purifying selection on, viability- or fertility-related phenotypes. However, this common procedure may not be applicable for detecting pathogenic mutations of microcephaly. From the perspective of evolutionary biology, the brain size change in human is apparently a novel phenotype under positive Darwinian selection. Thus, the gene mutations accounting for the phenotypic innovation could be under positive selection in evolutionary past. Consistent with this idea, multiple previous studies have observed a pattern of positive selection on microcephaly genes, which indicates the elevated evolutionary rates or plasticity across species (Evans et al., 2004; Wang and Su, 2004; Montgomery et al., 2011; Montgomery and Mundy, 2012).
Although it is now known that positive Darwinian selection drives the evolution of microcephaly genes, it is still unclear about the relationship between positive selection and pathogenic variants. Whether the pathogenic mutations occur within positively selected regions or negatively selected regions? In this study, we conducted trio-based whole-genome sequencing for a 4-year-old microcephaly patient and his parents. We uncovered novel compound heterozygous variants, p.Y613* and p.E1368K of TUBGCP6, Tubulin Gamma Complex Associated Protein 6, related to the microcephaly. Interestingly, the variant p.Y613* deletes the positively selected domains of TUBGCP6 while p.E1368K occurs within the two domains. Our study revealed an interesting pattern that the pathogenic variants of TUBGCP6 may cause disruptive and sequence-altering changes in evolutionarily important regions under positive selection.
Results
The variant statistics revealed balanced whole genome sequencing depths
The intelligence assessment revealed that the proband’s intellectual development is delayed compared to kids in similar age (4-year-old). Aside the developmental delay, the most unusually phenotype is the head circumference of the proband. When compared to the reference standards for growth and development of children in China issued in 2009 by China Ministry of Health, the head circumference of the proband is 3 standard deviations (SDs) lower than the average level.
We conducted WGS on a family trio, of which a proband with microcephaly (Figure 1A). The DNA from throat swabs of all individuals were sequenced into depth of ∼20x on average (father: 19.95x, mother: ∼19.96, proband: ∼18.76). The reason for the WGS of this depth is based on conclusions of multiple sensitivity studies that the WGS depth of 15x is sufficient for achieving accurate single-nucleotide variants (SNVs) calling, while 30x is appropriate for insertions and deletions (indels) calling (<50 bp) (Zhao et al., 2020; Sun et al., 2021). Thus, the depths of WGS sequencing data in this study can empirically guarantee the high reliability of SNVs and some small indels.
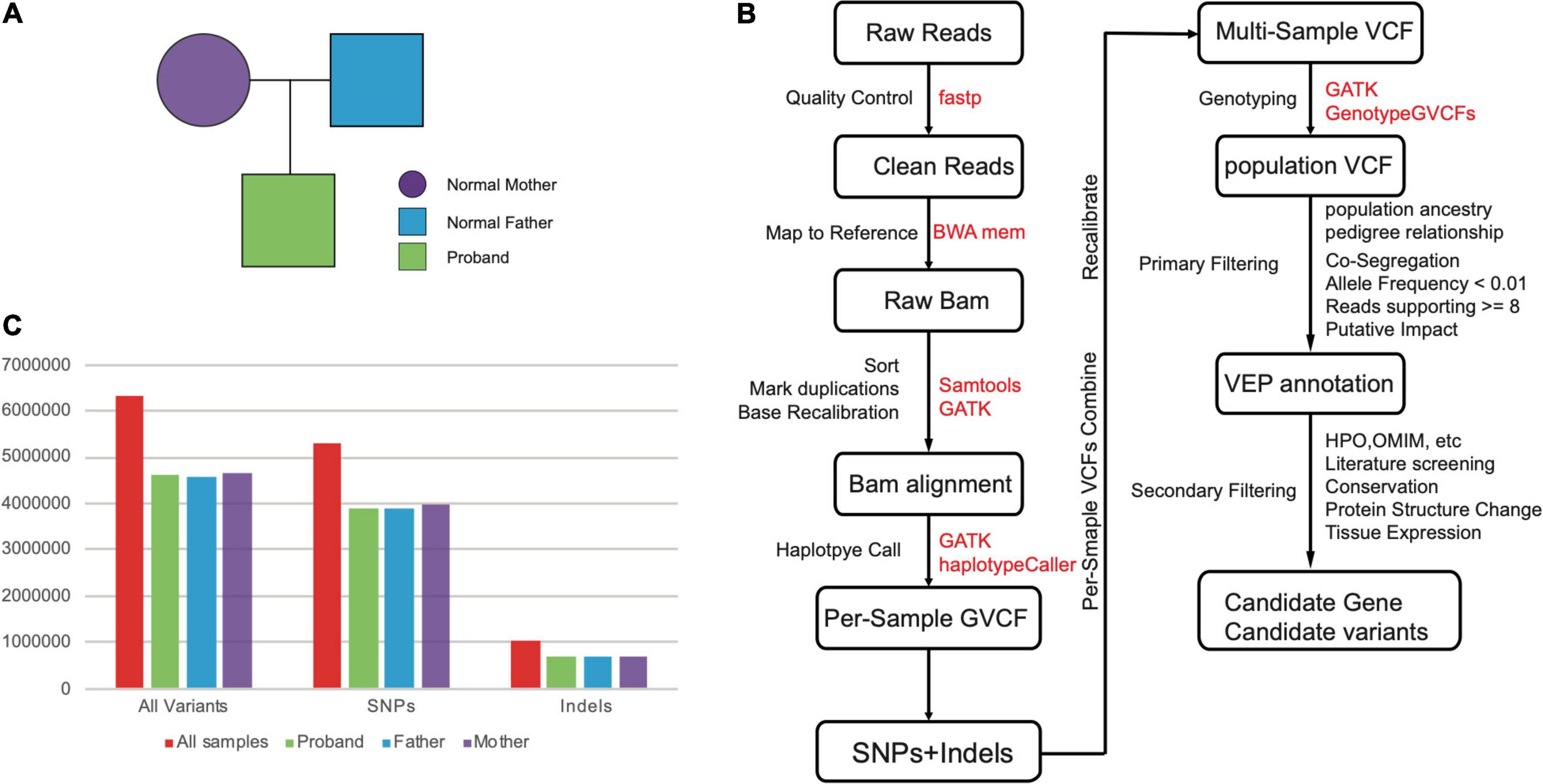
Figure 1. (A) The pedigree of our samples. (B) The pipeline for the computational identification for candidate gene and variants. (C) The numbers of variants (SNPs and Indels) for our whole-genome sequenced samples.
The variant calling with WGS data was majorly based on the GATK pipelines include data cleaning, mapping, duplications marking, base recalibration, initial calling with haplotypeCaller, and genotyping with multiple samples (Figure 1B). In total, we obtained 6,340,999 variants including 5,291,379 SNVs, and 1,049,620 Indels, respectively (Supplementary Table 1 and Figure 1C). We observed similar level of variant count distributions for three individuals, suggesting our WGS data are effectively balanced to guarantee equally optimal calling results (Figure 1C).
Population genetic analyses confirmed pedigree relationship and ethnic population
The Mendelian diseases can be rapidly exposed in consanguineous marriage, which can facilitate the detection of deleterious and rare homozygosity (Wright et al., 2018). To confirm the orally described genetic relationship between these individuals, we tried to explore whether there are potential hidden consanguineous signals, by estimating empirical kinship based on identity-by-state (IBS) model. As expected, IBS distances between parent and proband were lower than distance between mother and father, which confirmed the orally description of familial relationship (Figure 2A). This genetic relationship was further confirmed with kinship analysis (Manichaikul et al., 2010). The inferred kinship coefficient between father and proband (0.217) was nearly equal to that between mother and proband (0.212), while the father and mother were unrelated with each other (−0.02). This result confirmed that both father and mother were the first-degree relatives to proband.
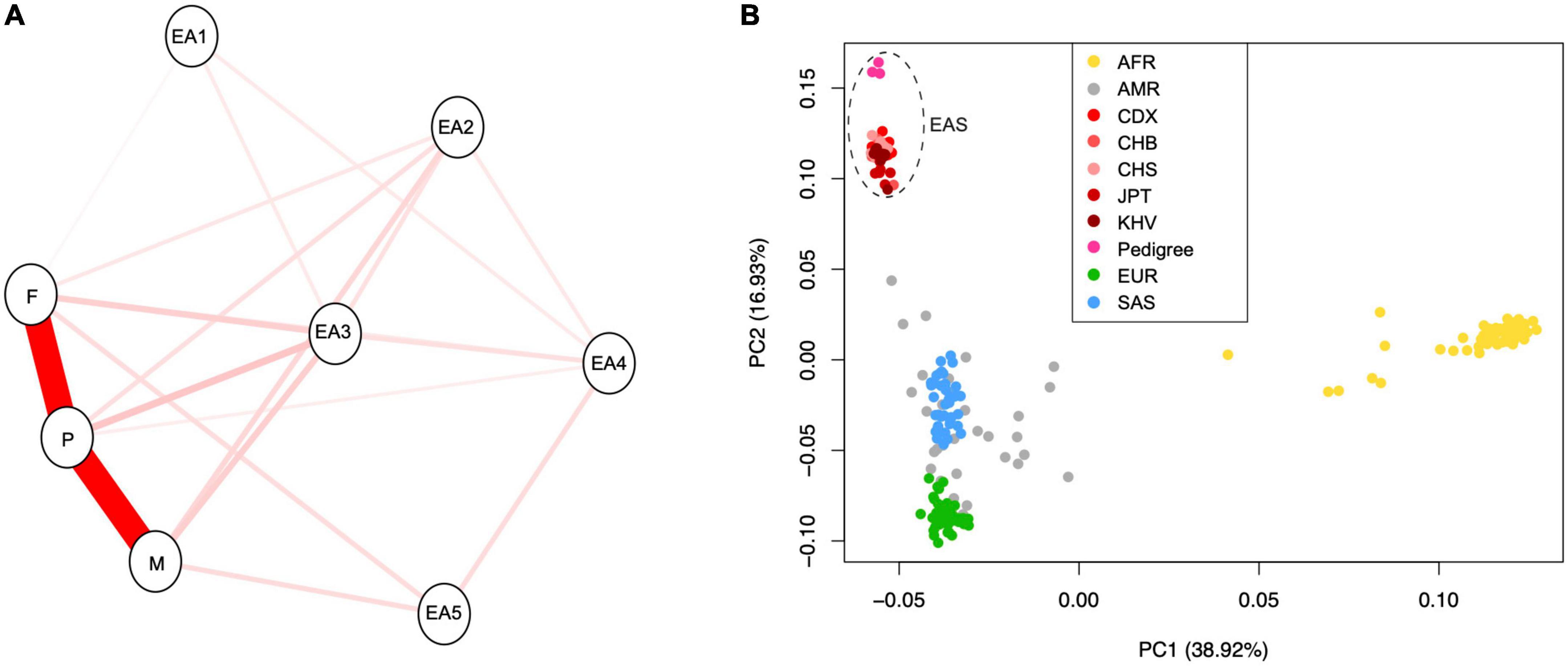
Figure 2. (A) The identity-by-state (IBS) relationship between newly sequenced pedigree samples and randomly chosen individuals with East Asian ancestry from “1,000 genome” project. (B) The principal component analysis (PCA) for three samples in this study and 208 publicly available individuals from 26 population of the 1,000 genome project. The “EA” indicates “East Asian population.” The populations AFR, AMR, CDX, CHB, CHS, JPT, KHV, EUR, and SAS represent African, Admixed American, Chinese Dai in Xishuangbanna, Han Chinese in Beijing, Southern Han Chinese, Japanese in Tokyo, Kinh in Ho Chi Minh City, European, and South Asian, respectively.
Based on knowledge from population genomics, allele frequencies are usually different among ethnic populations. New rare variants during evolution may introduce population heterogeneity in disease penetrance (McClellan and King, 2010). Thus, we analyzed the population ancestry of individuals in this study using the commonly used PCA method. We revealed that all newly sequenced individuals should belong to the ancestry of East Asia (Figure 2B).
The compound heterozygosity was detected in TUBGCP6
To identify the related gene(s) for microcephaly in proband of our sampled family, we performed screening based on the principle of ACMG guidance (Richards et al., 2015). Firstly, due to the larger effects of rare variants on disease phenotypes (Carss et al., 2017; Wright et al., 2018), we filtered out the non-rare variants with frequency over 0.01 which can be found in normal population in gnomAD database (v3.1). Among the 6,181,562 biallelic variants, 8.58% were found to be rare, while 4.16%, and 87.27% were less common (0.01 < Allele frequency < 0.05) and common (Allele frequency > 0.05) variants, respectively. Among rare variants, mutational burdens were estimated using protein-disruption mutations (“HIGH” annotated in SnpEff) as a proxy. Subsequently, we filtered out sites with low-quality mapping, where the numbers of supporting reads in alignment were less than 8 reads/variant. We further focused on co-segregated variants with disease-control status to guarantee the proper inheritance models. The microcephaly is generally reported to be the autosomal recessive disease (Kaindl et al., 2010). Some reports also support the X-linked recessive mode of this rare disease (Deshaies et al., 1979). Thus, both modes were used for screening. For X-linked recessive pattern, we only analyzed the variants within non-pseudoautosomal regions (non-PAR) of X chromosome, ranging from 10,001 bp to 2,781,479 bp (PAR1) and from 155,701,383 bp to 156,030,895 bp (PAR2). The variants were then predicted with the Ensembl Variant Effect Predictor (VEP) and only the variants with MAF < 0.01 in all non-disease populations of genomAD and 1,000 genome dataset were kept. To identify whether there are compound heterozygous variants, we focused on two or more heterozygous rare variants that were detected in one gene but showed different parental origins for the proband.
Finally, we identified two candidate pathogenic variants in exon 10 and 15 of TUBGCP6, following the inheritance mode of compound heterozygosity (Figure 3 and Table 1). Based on HGVS recommended nomenclature system, these two variants are denoted as NP_065194.2:p.(Y613*) and NP_065194.2:p.(E1368K). The variant NP_065194.2:p.(Y613*) is absent in the gnomAD, a public database with 76,156 human genomes of diverse population ancestries. It is also absent in the Exome Aggregation Consortium (ExAC) dataset of over 60,000 exomes (Karczewski et al., 2016), suggesting the rarity of this disruptive variant in population level. The variant NP_065194.2:p.(E1368K), also the variant rs184425523, is comparatively higher in allele frequency (AF). Based on gnomAD estimation, NP_065194.2:p.(E1368K) has the global AF 0.000079 (12/152254) and East Asian AF 0.0023 (12/5198).
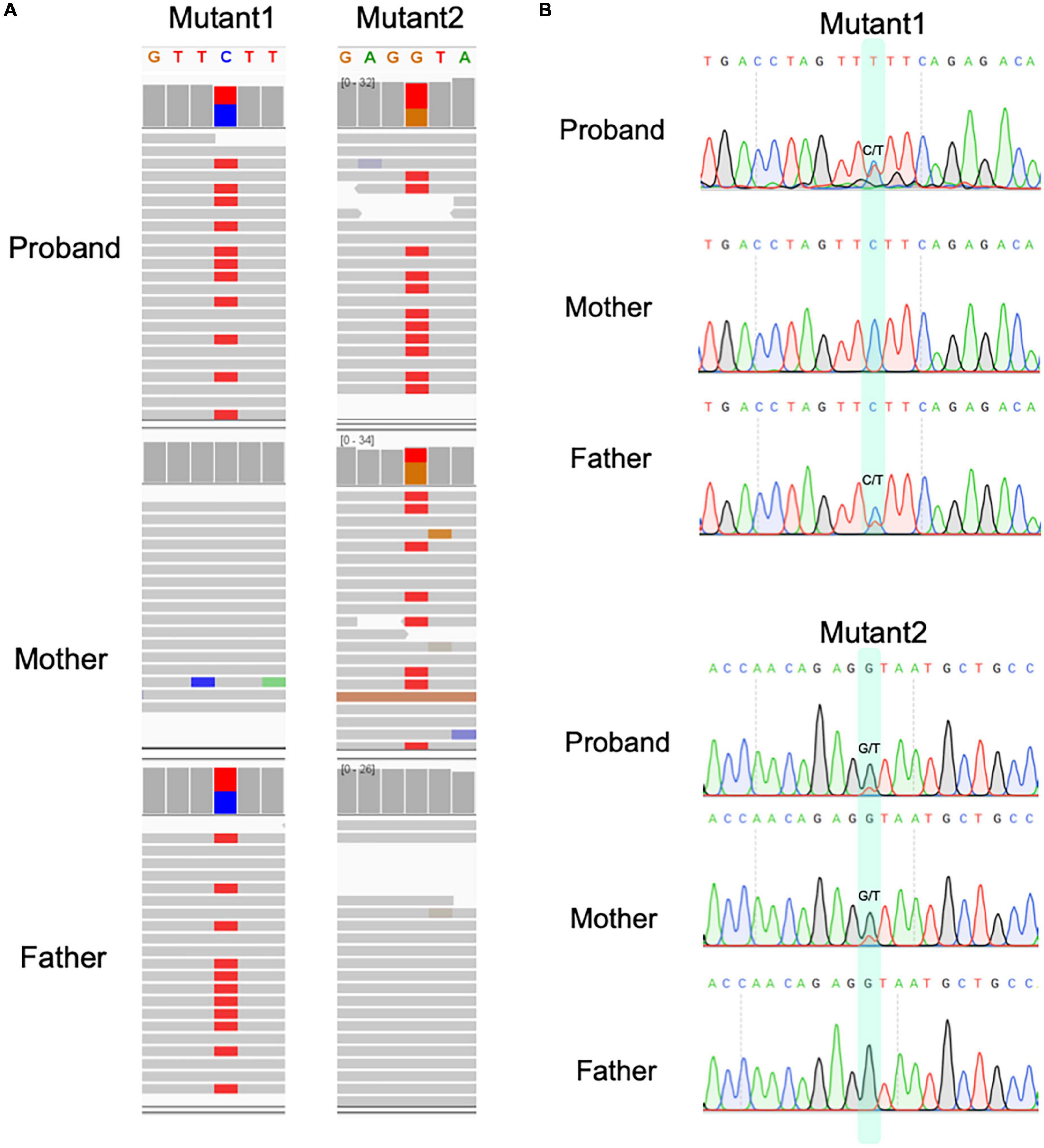
Figure 3. The compound heterozygous variants identified from proband of microcephaly in this study. (A) The compound heterozygous variants in proband and their parental origin based on genomic sequencing and IGV. (B) The Sanger sequencing for two variants in three individuals.

Table 1. Variants’ locations, genotype, numbers of supporting reads, and allele frequencies (AF) in global population and East Asian population (EA) for TUBGCP6.
These variants were supported by at least 18 sequencing reads, indicating the high quality of variants. It is considerably interesting to know the parental origin of these two variants. By manually checking the mapping file (“bam” format) using IGV software and performing the Sanger sequencing for three individuals, we determined the parental origin of heterozygous variants (Figure 3B). We found that the C > T variant was inherited from father, while G > T variant from mother. Based on annotation of gene structure, RNA transcriptional machinery of the gene TUBGCP6 reads from the reverse strand (Figure 4A). Thus, the maternal inherited G > T is the leading variant relative to paternal inherited variant C > T. The maternal origin variant NP_065194.2:p.(Y613*) results in premature termination in site 613 of protein NP_065194.2. The paternal inherited C > T variant, NP_065194.2:p.(E1368K), is the subsequent variant that result in a missense variant from Glutamic acid (E) to Lysine (K). Compared to orthologous genes in other species, we found that the maternal disruptive variant NP_065194.2:p.(Y613*) locates within a conserved region while the paternal variant in highly diverged region across species (Figure 4B). Based on the tertiary structure of TUBGCP6 from Alphafold2 prediction, the leading variant NP_065194.2:p.(Y613*) occurred in a α-helix region (confidence score > 85.04) while the second variant NP_065194.2:p.(E1368K) occurred within a random coil region with very low modeling confidence (confidence score = 21.73).
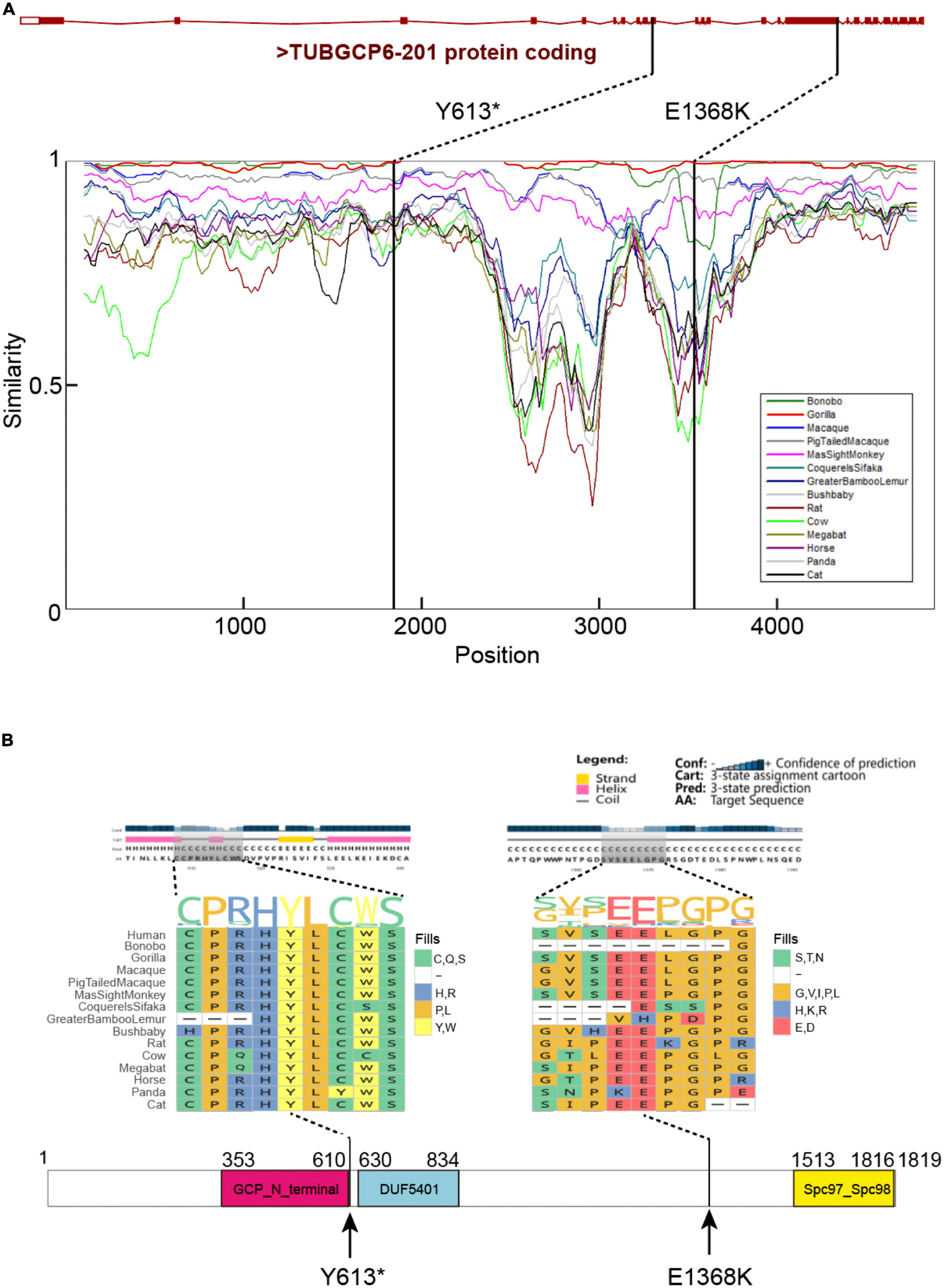
Figure 4. (A) The gene structure of TUBGCP6, locations of the identified two variants, and the regional similarities across mammalian species. The similarity percentages were inferred with SimPlot V3.5.1 (Lole et al., 1999). (B) The protein secondary structure and multiple species protein alignment, with a focus on the two variants (Y613* and E1368K). The protein domain were inferred with PSIPRED (Jones, 1999).
The brain-tissue expression and frequent protein-protein interaction
The anatomical position(s) of gene expression may indicate the affected organs/tissues of patients. The RNAseq and proteomics data have been piled up to facilitate our understanding on normal function of human genes. TUBGCP6 was found to be widely expressed based on both GTEx1 and HPA2 databases. Notably, the GTEx is a large-scale gene expression resource based on 54 tissues, allowing for gene expression analysis at population level (The GTEx Consortium et al., 2015). GTEx data revealed that TUBGCP6 is expressed highest in brain tissues (median TPM, transcript per million, 124.7) and lowest in heart (median TPM 9.628). In addition, the expression levels in reproduction-related organs of female (ovary and uterus) are higher than that of male (testis and prostate).
Based on the single-cell RNAseq from HPA, although the expression of TUBGCP6 has relatively low cell type specificity and brain regional specificity, the highest expression was found in the astrocytes and excitatory neurons of brain (Supplementary Figure 1A). Interestingly, it is well-known that the differentiation of astrocytes is related to brain size (Kang et al., 2020). In addition, the Zika virus can result in the disruption of astrocytic proteins, thereby leading to the featuring symptom of microcephaly (Sher et al., 2019). The excitatory neurons make up the majority (80%) of neurons in the cerebral cortex. The microcephaly gene was validated to be essential for progenitors of cortical glutamatergic neurons (Venkataramanappa et al., 2021). TUBGCP6 also expressed in cerebellum, cerebral cortex, olfactory region, hippocampal formation, amygdala, basal ganglia, thalamus, hypothalamus, midbrain, and pons and medulla (Supplementary Figure 1B). This broad expression of TUBGCP6 in different brain regions suggest the critical role of its protein in normal brain development and function.
To understand whether the gene expression in brain is common for microcephaly-related genes, we focused on gene annotation of primary microcephaly from HPO and the population-level RNA-seq expression from GTEx. Among the 38 genes related to microcephaly based on HPO annotation (Supplementary Table 2), 42.11% (16/38) of genes, which are PSAT1, AFF3, EOMES, MECP2, NSF, RELN, TELO2, TRIO, TSEN54, VPS4A, GRIN2A, TUBB3, SLC1A4, NDE1, PHGDH, and ZEB2, shows the highest expression in brain. Interestingly, apart from brain, testis is also the preferred organ for microcephaly-related genes to show the highest expression. The highest testis expression pattern covers 23.68% (9/38) of genes, which are CLPB, LMNB2, NUP188, RBBP8, RNF2, SMC3, SPATA5, TRAPPC12, and TRMT10A. The high testis expression, which could be due sexual selection (Montgomery et al., 2011), has been used to interpret the rapid evolution of some microcephaly-related genes.
We further analyzed the protein-protein interaction (PPI) network of TUBGCP6 based on the STRING database v11.5 (Jensen et al., 2008). Among 20 genes with significant protein interactions with TUBGCP6, we found half of the genes (10) are disease genes for microcephaly (local clustering coefficient: 0.915; PPI enrichment p-value < 1.0e–16 Supplementary Figure 1C). The functional enrichments for these genes revealed the microtubule and mitosis-related functions for the top five significant biological processes, including microtubule nucleation (GO:0007020; False discovery rate, FDR = 8.96e–17), microtubule cytoskeleton organization (GO:0000226; FDR = 1.56e–14), microtubule nucleation by microtubule organizing center (GO:0051418; FDR = 2.63e–14), spindle organization (GO:0007051; FDR = 5.03e–13), and mitotic cell cycle (GO:0000278; FDR = 8.64e–13). Disease-gene associations revealed significant enrichment of congenital nervous system abnormality (FDR = 2.62e–12), microcephaly (FDR = 3.65e–11), and monogenic disease (FDR = 0.00022).
The positive selection on TUBGCP6
Previous reports have revealed multiple microcephaly-related genes are under positive selection along lineages leading to human. For example, the microcephalin gene has signals of accelerated evolution or positive selection in the common ancestor of great apes (Evans et al., 2004). In addition, some sites of microcephalin have unexpectedly higher frequency of derived alleles during human evolution, which is inconsistent with genetic drift under neutral evolution (Wang and Su, 2004). Other microcephaly-related genes including the ASPM, CDK5RAP2, and CENPJ have also been found to undergo positive selection, suggesting a general propensity for them to be positively selected in primates (Montgomery et al., 2011; Montgomery and Mundy, 2012). These studies connote the importance of positive selection on brain enlargement during human and primate evolution. However, probably due to insufficient number of identified pathogenic variants before the advent and application of WES (Whole exome sequencing) or WGS (Whole genome sequencing), these evolutionary studies did not reveal whether the pathogenic variants may impact the positively selected regions or not. It is highly expected that the pathogenic variants could influence positively selected regions more frequently than the unselected regions, under the assumption that historical positive selection had driven the molecular evolution of microcephaly-related genes to facilitate the enlargement of brain size.
We performed gene selection analyses based on multiple complementary methods, including the MK (McDonald–Kreitman) test, the CODEML branch-site model test, CODEML branch model test, CODEML site model test, the Hudson–Kreitman–Agaude (HKA) test, and MEME method in HyPhy package (Figure 5). The MK test can be used to detect the violation of the neutral expectation, in which the ratio of non-synonymous and synonymous polymorphism (pN/pS) should be similar to the ratio of non-synonymous and synonymous divergence (dN/dS) (McDonald and Kreitman, 1991). Based on genomic analyses covering 26 chimpanzees (Fair et al., 2020; García-Pérez et al., 2021), 1,000 human individuals (Clarke et al., 2012), and the annotation of variants from gnomAD database (Gudmundsson et al., 2021), the MK test revealed that the divergence ratio (dN/dS = 1/5) is not significantly higher than the polymorphism ratio (pN/pS = 2/3, maf > 0.05) (p = 0.39). This result suggests multiple testable possibilities, including very weak positive selection in human protein of TUBGCP6, selection in regulatory regions instead of protein regions, selection in evolutionary past instead of modern human branch.
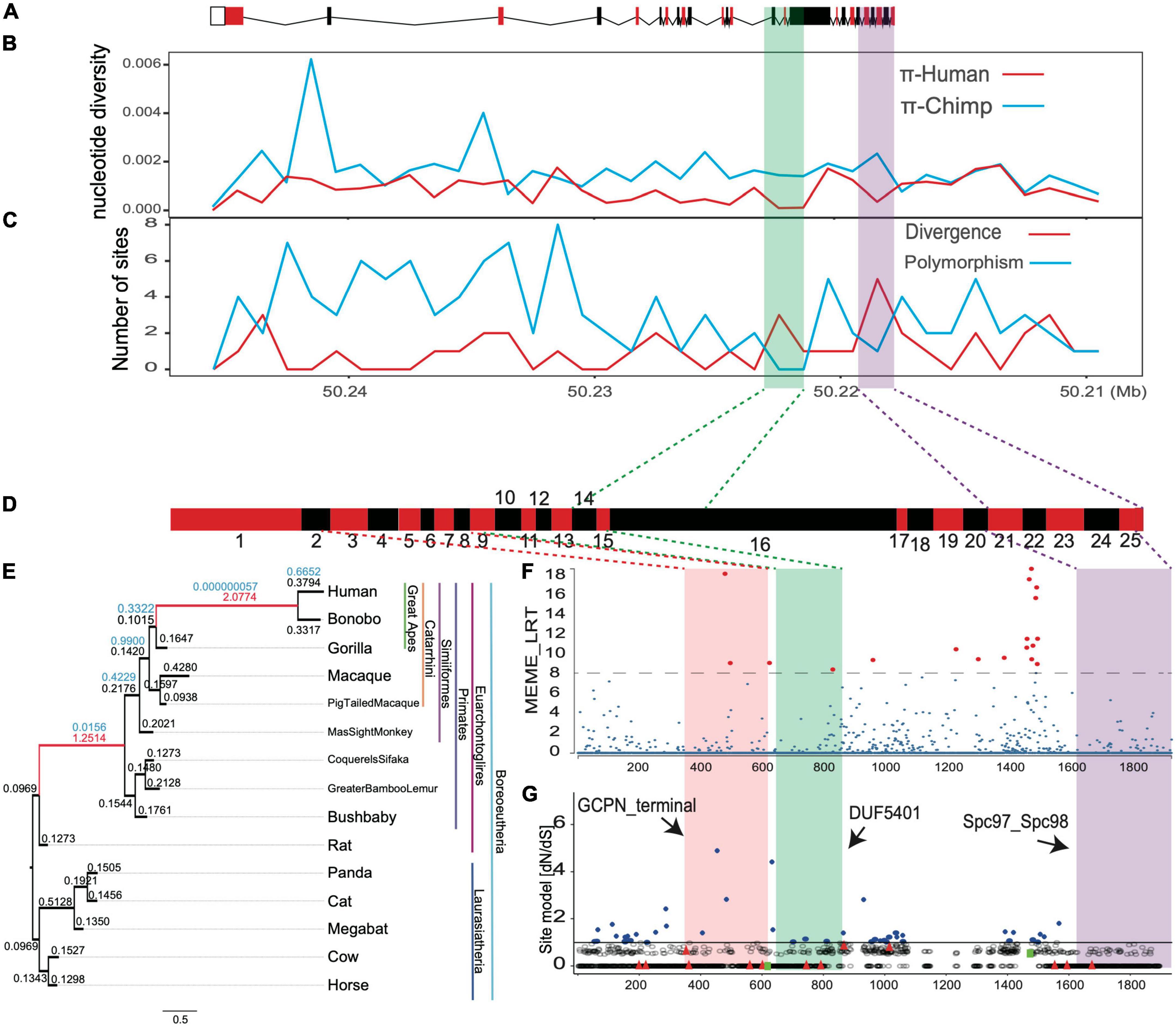
Figure 5. The positive selection signals for TUBGCP6 based on multiple methods. (A) The gene structure of TUBGCP6, including exons (boxes) and introns (lines). (B–C) The combined methods of nucleotide diversity (π) and the HKA test. (D) The exons (red and black boxes) of TUBGCP6. (E) The branch model test results based on the comparisons between two-ratio models and one-ratio model. All branches in the phylogeny were used as foreground lineage in an iterative way. The numbers above the ancestral branches showed the dN/dS and significance level. Only the significant branches and dN/dS were shown in red. (F) The MEME method in HyPhy package. The significance level was shown with dotted line and with the consideration of multiple test correction (p < 0.01). The red points showed the inferred positively selected sites. (G) The potentially positively selected sites (blue, dN/dS > 1) based on the site model test of CODEML (M7 vs. M8). The two green squares represent two variants (Y613* and E1368K). The black arrows indicate the three domains.
To understand whether the regulatory region of human TUBGCP6 might be under selection, we used two methods: (1) comparing nucleotide diversities (π) between human and chimpanzee sequences (Supplementary Table 3); (2) comparing the number of divergence sites to that of polymorphic sites across the gene (also the HKA test). Hallmarks of positive selection in DNA sequence would demonstrate the reduction of regional nucleotide diversity (π) and the faster depletion of polymorphic sites than divergent sites (Hudson et al., 1987; Booker and Keightley, 2018). We found that the shared positive selection signals from these two methods converged into two DNA regions, which are the region within exon 14 and exon 16 and the region within exon 21 and exon 25 (Figures 5A–C), supporting the selection at regulatory regions of human TUBGCP6.
We further tried to examine whether the positive selection occurred in evolutionary past using the branch model of CODEML. Based on the iterative comparisons between two-ratio models and one-ratio model for all external and internal branches, we found the signals of positive selection with dN/dS > 1 on two ancestral branches, the common ancestor of apes (Hominin) and the common ancestor of primates (chi-squared test, p < 0.01, Figures 5D,E). This result echoes the finding of the MK test and suggests that the positive selection on protein sequence of TUBGCP6 could be significantly stronger in the evolutionary past than the extant branch of human.
We also examined the site-level selection signals for the protein domains of TUBGCP6 based on MEME method in HyPhy package and the site model of CODEML (M7 vs. M8). These two methods showed consistently the potential positive selection on a few sites within the first two domains (GCPN terminal and DUF5401, Figures 5F,G) and the linker region between the second and the third domain (DUF5401 and Spc97_Spc98). The second and third domain were also supported by the combined methods of nucleotide diversity (π) and the HKA test (Figures 5A–C). Because the first variant (Y613*) occurred just before the positively selected domains DUF5401 and Spc97_Spc98, these two domains could be affected and deleted by this truncating mutation. In addition, the second variant (E1368K) was found within the linker section between DUF5401 and Spc97_Spc98, suggesting the rare mutation burden on this positively selected region. Nevertheless, we noted that, due to the reliability problem in the site model prediction (Nozawa et al., 2009), further studies based on experimental validations could be more critical and decisive for phenotypical importance of the two domains of TUBGCP6.
Discussion
In cellular biology, a salient feature of neurons is that neuron proliferation is largely complete by mid-gestation (Spalding et al., 2005) and nearly none of cerebral cortex neurons are generated after birth (Stiles and Jernigan, 2010). Although the brain volume grows until adulthood, the increase is limited to only the neuropil, which are cell connections including glial processes, axons, dendrites, etc. Moreover, the ratios of brain volumes to neuron numbers across primates are almost constant, suggesting a isometrical function between the brain volume and the number of pre-existed neurons (Herculano-Houzel et al., 2007). Thus, children with developmental microcephaly is ultimately due to neuron deficiency rooted in genetic defects (Gilmore and Walsh, 2013).
The microcephaly has been generalized as a cell cycle disease (Doobin et al., 2017). It is now known that the cell cycle-dependent nuclear machinery and activities are powered by microtubule motor proteins. Multiple microcephaly-related genes, for example, ASPM, CDK5RAP2, PLK4, Microcephalin, STIL, WDR62, CEP152, and CENPJ, are involved in microtubule or centrosomal-related activities, mitotic cell division, and DNA damage pathways (Bond and Woods, 2006; Cox et al., 2006; Fish et al., 2006; Kumar et al., 2009; Bilgüvar et al., 2010; Buchman et al., 2010; Press et al., 2019). As a direct phosphorylation target of the PLK4 kinase, TUBGCP6 has also been found in multiple unrelated microcephaly cases of Caucasian, the Middle East, and South American ancestry (Puffenberger et al., 2012; Martin et al., 2014). The molecular mechanism of TUBGCP6 may be involved in the shared cellular pathway with PLK4 to cause the microcephaly (Martin et al., 2014). The in vitro experiment revealed that TUBGCP6 is the substrate of PLK4-induced centriole overduplication and required for centriole duplication (Bahtz et al., 2012). Altogether, current knowledge collectively support the indispensable role of evolutionary changes of genes related to cell division in contributing a bigger brain during human evolution (Fish et al., 2008; Matsuzaki and Shitamukai, 2015).
Here, we expanded the list of candidate pathogenic variants contributing to the microcephaly, based on trio sequencing and rigorous medical bioinformatics analyses. We found the compound heterozygous variants (Y613* and E1368K) in a previously known gene TUBGCP6 as the most probable pathogenic variants. Literature review revealed the evidence of the causative relationship between TUBGCP6 defects and microcephaly (Table 2). A homozygous variant resulting in read-through of TUBGCP6 (X1820G) was identified in six Pennsylvania Mennonite patients with microcephaly and chorioretinopathy (Puffenberger et al., 2012). In another study, based on four patients from three families with microcephaly, compound heterozygous mutations in the TUBGCP6 gene were identified (Martin et al., 2014). Interestingly, a shared feature between Martin et al. (2014) and this study is the involvement of heterozygous mutations leading to premature stop codon.
Evolutionary methodologies have been applied extensively to address questions involving the molecular evolution of critical genes and the phenotypical novelty of brain-size enlargement (Montgomery et al., 2011; Montgomery and Mundy, 2012). It’s known that the mammalian evolution leading to human lineage accompanies a long-term, gradual, and complicated process of brain-size enlargement (Seyfarth and Cheney, 2002; Herculano-Houzel et al., 2007). Thus, it is conceivable that the positive natural selection of microcephaly genes could contribute to the phenotypical innovation of human species (Evans et al., 2004; Wang and Su, 2004; Montgomery et al., 2011; Montgomery and Mundy, 2012). Until now, however, the knowledge gap between the pathogenic mutations and positive selection still exists. In this study, we provided evidence that the compound heterozygous variants could strongly impact the positively selected regions of TUBGCP6. We focused on the fine-scale structures and selection signals for TUBGCP6 at both gene- and protein-level. We found evidence of positive selection on the two domains (DUF5401 and Spc97_Spc98) and the liner region between these domains. Although currently there is no function studies on DUF5401, Spc97_Spc98 belongs to a family of spindle pole body (SBP) component, which is functional in microtubule cytoskeleton organization (Chen et al., 2017). Interestingly, based on the mapping of candidate pathogenic variants, these variants would influence the regions of positive selection. The truncating mutation (Y613*) occurred before the two positively selected domains, which could cause deletions of the two domains. The second missense variant (E1368K) would influence the structure or stability of the linker region, which was also found to be under positive selection.
Conclusion
The findings in this study could shed light on a growing knowledge base and a new paradigm for the investigation of human-specific traits and the gene evolution. The evolutionary conservation, which is maintained by the force of purifying selection, may have served as the cornerstone for the identification of pathogenic variants in medical genetics. In contrast, the novel DNA changes and the subsequent positive selection are even more important for phenotypical innovation and speciation. Our study reconciles the two evolutionary forces in medical genetics and layouts a useful potential for further studies on the pathogenic disruption of positively selected regions for species-specific traits and diseases.
Materials and methods
Patient background
The proband was diagnosed to be developmental, intelligence, and motor delays in multiple hospitals including the West China Second University Hospital (also the Women and Children hospital in Chengdu, China). The intelligence assessment was conducted and the proband’s intellectual development was compared to kids in similar age (4-year-old) by professional clinicians. For the phenotype of head circumference of the proband, the official release of the reference standards for growth and development of children under 7 years of age in China issued in 2009 by China Ministry of Health was used for calculating the standard deviations (SDs) from average level. Informed consent was obtained from the participating parent. The study was approved by the Ethics Committee of West China Hospital (Registration number: 2021389).
Whole-genome sequencing, variants calling, and genotyping
Deoxyribonucleic acid was extracted from throat swabs of all individuals. The WGS of 150 bp paired-end reads with an insert-size 350 bp was conducted for three samples using DNBSEQ-T7 sequencer developed by MGI. The raw reads were treated with the tool fastp for quality control, adapter trimming, and quality filtering cleaning (Chen et al., 2018). The clean reads were then aligned to the human reference genome (hg38) retrieved from GATK file bundle using the Burrows-Wheeler Aligner mem algorithm (Li, 2013). The subsequent processes including alignment sorting, duplicates marking, and base quality scores recalibration were performed by following the Best Practice protocol of GATK v4.1 (McKenna et al., 2010; Van der Auwera and O’Connor, 2020). The dataset covering human variants from the “1,000 genomes” project and 26 chimpanzee genomic variants were also composed for the positive selection analysis.
Family genetic relationship network and population ancestry
We tried to use genomic information to answer questions involving genetic background. The super-population ancestry was estimated using the principal component analysis (PCA) with FlashPCA2 (Abraham et al., 2017), after performing the linkage disequilibrium filtering (–indep 50 5 2) with PLINK (Purcell et al., 2007). The relationship between familial members was evaluated with identity by state (IBS) using PLINK with MAF threshold 0.05. IBS was visualized with R package qgraph (Epskamp et al., 2012). KING software was used to estimate kingship (Manichaikul et al., 2010). The threshold of kinship value range > 0.354, (0.177, 0.354), (0.0884, 0.177), and (0.0442, 0.0884) was translated into duplicate/MZ twin, 1st-degree, 2nd-degree, and 3rd-degree relationship, respectively.
The identification of variants related to microcephaly
An in-house pipeline was used to perform disease-related variants identification following the guidance of ACMG (Richards et al., 2015). The major procedures include: (1) allele frequency filtering (Minor allele frequency, MAF < 0.01); (2) the number of supporting reads in alignment (≥ 8 reads/variant); (3) focusing on variants of co-segregated with disease status, following two inheritance modes: the autosomal recessive and X-linked recessive patterns. For X-linked recessive pattern, only the variants within non-pseudoautosomal regions (non-PAR) of X chromosome were used. The variants were then predicted with the Ensembl Variant Effect Predictor (VEP). Only the variants with MAF < 0.01 in all populations based on genomAD and mutational impact predicted with “HIGH/MODERATE” were kept. The compound heterozygous variants were identified by focusing on two or more heterozygous variants within a single gene but with different parental origin.
Notably, the threshold of MAF < 0.01 can indicate the rarity of the variant in common population and thus suggest the potential purifying selection. The variant impact prediction was based on SIFT (Ng and Henikoff, 2003), PolyPhen (Adzhubei et al., 2013), and LoFtools (Fadista et al., 2017). The “HIGH” impact on protein comprises disruptive and knock-out effects on genes, including the chromosome number variation, exon loss, frameshift, rare amino acid, splice acceptor, splice donor, start codon losses, stop codon gains and losses, and transcript ablation. The “MODERATE” comprised inframe insertions, disruptive inframe insertions, inframe deletions, disruptive inframe deletions, missense variants, splice region variants, 3 prime UTR truncations, and 5 prime UTR truncations, all of which may result in non-disruptive but effectiveness-alteration consequences on the protein.
The protein structure prediction, RNAseq expression, and protein-protein interaction network
PSIPRED4.0 (McGuffin et al., 2000) and SCANSITE4.0 (Obenauer et al., 2003) were used for predicting secondary structure for the protein of the identified gene. The impacts of variants on tertiary structure were based on Alphafold2 prediction. The orthologous gene alignment was performed based on MAFFT (Katoh et al., 2002) with visualized with SimPlot V3.5.1 (Lole et al., 1999). The protein-protein interaction (PPI) network was analyzed based the STRING database (Szklarczyk et al., 2019). The population-level RNAseq expression and single-cell RNA expression pattern were retrieved from GTEx (Thul and Lindskog, 2018) and HPA (The GTEx Consortium et al., 2015).
The evolutionary selective pressure analyses
Since human brain-size has been changed extremely during primate evolution, we tried to understand whether the microcephaly-related gene should be under positive selection in primate or human lineage. We estimated dN/dS ratios using CODEML package of PAML 4.9 (Yang, 2007) for maximum likelihood analysis. For branch model, we estimated dN/dS ratios for different lineages with confident orthologous genes retrieved from Ensembl. The statistical significance was estimated by following a chi-square distribution, with two times the difference in log-likelihood values and freedom degree as the difference in number of parameters for the two models. The MK test was based on the variants datasets covering 26 chimpanzees (Fair et al., 2020; García-Pérez et al., 2021), 1,000 human individuals (Clarke et al., 2012), and the annotation of variants from gnomAD database (Gudmundsson et al., 2021). HKA test and nucleotide diversity (π) based on human and chimpanzee genomic data were also performed along the genic coordinates (Hudson et al., 1987; Booker and Keightley, 2018). The MEME method (Murrell et al., 2012) and the site model of CODEML (Yang, 2007) were used for screening potential sites under positive selection.
Data availability statement
The datasets for this article are not publicly available due to concerns regarding participant/patient anonymity. Requests to access the datasets should be directed to the corresponding author.
Ethics statement
The studies involving human participants were reviewed and approved by the Ethics Committee of West China Hospital (Registration number: 2021389). Written informed consent to participate in this study was provided by the participants’ legal guardian/next of kin. Written informed consent was obtained from the individual(s), and minor(s)’ legal guardian/next of kin, for the publication of any potentially identifiable images or data included in this article.
Author contributions
JC, LY, YJ, HY, CL, GY, and LZ designed the research. JC wrote the manuscript. All authors helped to improve the manuscript.
Funding
This study was supported by the Fifth Batch of Technological Innovation Research Projects in Chengdu (2021-YF05-01331-SN), Postdoctoral Research and Development Fund of West China Hospital (2020HXBH087), and the Short-Term Expert Fund of West China Hospital (139190032).
Acknowledgments
We acknowledged the computing supports from the West China Biomedical Big Data Center and the Med-X Center for Informatics of Sichuan University.
Conflict of interest
The authors declare that the research was conducted in the absence of any commercial or financial relationships that could be construed as a potential conflict of interest.
Publisher’s note
All claims expressed in this article are solely those of the authors and do not necessarily represent those of their affiliated organizations, or those of the publisher, the editors and the reviewers. Any product that may be evaluated in this article, or claim that may be made by its manufacturer, is not guaranteed or endorsed by the publisher.
Supplementary material
The Supplementary Material for this article can be found online at: https://www.frontiersin.org/articles/10.3389/fevo.2022.1059477/full#supplementary-material
Supplementary Figure 1. The gene expression of single-cell RNAseq in multiple tissues (A) and brain subregions (B), were retrieved from HPA database. The red arrow shows the tissue with highest expression. (C) The protein-protein interaction network of TUBGCP6, based on the STRING database. The red genes are related to the mitosis processes and functions.
Footnotes
References
Abraham, G., Qiu, Y., and Inouye, M. (2017). FlashPCA2: principal component analysis of biobank-scale genotype datasets. Bioinformatics 33, 2776–2778. doi: 10.1093/bioinformatics/btx299
Abuduxikuer, K., Zou, L., Wang, L., Chen, L., and Wang, J.-S. (2020). Novel NGLY1 gene variants in Chinese children with global developmental delay, microcephaly, hypotonia, hypertransaminasemia, alacrimia, and feeding difficulty. J. Hum. Genet. 65, 387–396. doi: 10.1038/s10038-019-0719-9
Adzhubei, I., Jordan, D. M., and Sunyaev, S. R. (2013). Predicting functional effect of human missense mutations using PolyPhen-2. Curr. Protocols Hum. Genet. Chapter 7:Unit7.20.
Arboleda, V. A., Lee, H., Dorrani, N., Zadeh, N., Willis, M., Macmurdo, C. F., et al. (2015). De novo nonsense mutations in KAT6A, a lysine acetyl-transferase gene, cause a syndrome including microcephaly and global developmental delay. Am. J. Hum. Genet. 96, 498–506. doi: 10.1016/j.ajhg.2015.01.017
Bahtz, R., Seidler, J., Arnold, M., Haselmann-Weiss, U., Antony, C., Lehmann, W. D., et al. (2012). GCP6 is a substrate of Plk4 and required for centriole duplication. J. Cell Sci. 125, 486–496. doi: 10.1242/jcs.093930
Bilgüvar, K., Öztürk, A. K., Louvi, A., Kwan, K. Y., Choi, M., Tatlı, B., et al. (2010). Whole-exome sequencing identifies recessive WDR62 mutations in severe brain malformations. Nature 467, 207–210. doi: 10.1038/nature09327
Bond, J., and Woods, C. G. (2006). Cytoskeletal genes regulating brain size. Curr. Opin. Cell Biol. 18, 95–101.
Booker, T. R., and Keightley, P. D. (2018). Understanding the factors that shape patterns of nucleotide diversity in the house mouse genome. Mol. Biol. Evol. 35, 2971–2988. doi: 10.1093/molbev/msy188
Buchman, J. J., Tseng, H.-C., Zhou, Y., Frank, C. L., Xie, Z., and Tsai, L.-H. (2010). Cdk5rap2 interacts with pericentrin to maintain the neural progenitor pool in the developing neocortex. Neuron 66, 386–402. doi: 10.1016/j.neuron.2010.03.036
Carss, K. J., Arno, G., Erwood, M., Stephens, J., Sanchis-Juan, A., Hull, S., et al. (2017). Comprehensive rare variant analysis via whole-genome sequencing to determine the molecular pathology of inherited retinal disease. Am. J. Hum. Genet. 100, 75–90.
Chen, S., Zhou, Y., Chen, Y., and Gu, J. (2018). fastp: an ultra-fast all-in-one FASTQ preprocessor. Bioinformatics 34, i884–i890. doi: 10.1093/bioinformatics/bty560
Chen, Y., Bi, H., Li, X., Zhang, Z., Yue, H., Weng, S., et al. (2017). Wsv023 interacted with Litopenaeus vannamei γ-tubulin complex associated proteins 2, and decreased the formation of microtubules. R. Soc. Open Sci. 4:160379. doi: 10.1098/rsos.160379
Clarke, L., Zheng-Bradley, X., Smith, R., Kulesha, E., Xiao, C., Toneva, I., et al. (2012). The 1000 genomes project: data management and community access. Nat. Methods 9, 459–462. doi: 10.1038/nmeth.1974
Cox, J., Jackson, A. P., Bond, J., and Woods, C. G. (2006). What primary microcephaly can tell us about brain growth. Trends Mol. Med. 12, 358–366. doi: 10.1016/j.molmed.2006.06.006
Deshaies, Y., Rott, H., Wissmuller, H., Schwanitz, G., Le Marec, B., and Koch, G. (1979). Recessive microencephaly linked to the X chromosome. J. Genetique Hum. 27, 221–236.
Doobin, D. J., Dantas, T. J., and Vallee, R. B. (2017). Microcephaly as a cell cycle disease. Cell Cycle 16, 247–248. doi: 10.1080/15384101.2016.1252591
Epskamp, S., Cramer, A. O., Waldorp, L. J., Schmittmann, V. D., and Borsboom, D. (2012). qgraph: network visualizations of relationships in psychometric data. J. Statist. Software 48, 1–18.
Evans, P. D., Anderson, J. R., Vallender, E. J., Gilbert, S. L., Malcom, C. M., Dorus, S., et al. (2004). Adaptive evolution of ASPM, a major determinant of cerebral cortical size in humans. Hum. Mol. Genet. 13, 489–494. doi: 10.1093/hmg/ddh055
Fadista, J., Oskolkov, N., Hansson, O., and Groop, L. (2017). LoFtool: a gene intolerance score based on loss-of-function variants in 60 706 individuals. Bioinformatics 33, 471–474. doi: 10.1093/bioinformatics/btv602
Fair, B. J., Blake, L. E., Sarkar, A., Pavlovic, B. J., Cuevas, C., and Gilad, Y. (2020). Gene expression variability in human and chimpanzee populations share common determinants. eLife 9:e59929. doi: 10.7554/eLife.59929
Fish, J. L., Dehay, C., Kennedy, H., and Huttner, W. B. (2008). Making bigger brains–the evolution of neural-progenitor-cell division. J. Cell Sci. 121, 2783–2793. doi: 10.1242/jcs.023465
Fish, J. L., Kosodo, Y., Enard, W., Pääbo, S., and Huttner, W. B. (2006). Aspm specifically maintains symmetric proliferative divisions of neuroepithelial cells. Proc. Natl. Acad. Sci. U S A. 103, 10438–10443. doi: 10.1073/pnas.0604066103
García-Pérez, R., Esteller-Cucala, P., Mas, G., Lobón, I., Di Carlo, V., Riera, M., et al. (2021). Epigenomic profiling of primate lymphoblastoid cell lines reveals the evolutionary patterns of epigenetic activities in gene regulatory architectures. Nat. Commun. 12:3116. doi: 10.1038/s41467-021-23397-1
Gilmore, E. C., and Walsh, C. A. (2013). Genetic causes of microcephaly and lessons for neuronal development. WIREs Dev. Biol. 2, 461–478. doi: 10.1002/wdev.89
Gudmundsson, S., Singer-Berk, M., Watts, N. A., Phu, W., Goodrich, J. K., and Solomonson, M. (2021). Variant interpretation using population databases: lessons from gnomAD. Hum. Mutation 43, 1012–1030.
Herculano-Houzel, S., Collins, C. E., Wong, P., and Kaas, J. H. (2007). Cellular scaling rules for primate brains. Proc. Natl. Acad. Sci. U S A. 104, 3562–3567. doi: 10.1073/pnas.0611396104
Herculano-Houzel, S., and Kaas, J. H. (2011). Gorilla and orangutan brains conform to the primate cellular scaling rules: implications for human evolution. Brain Behav. Evol. 77, 33–44. doi: 10.1159/000322729
Hudson, R. R., Kreitman, M., and Aguadé, M. (1987). A test of neutral molecular evolution based on nucleotide data. Genetics 116, 153–159. doi: 10.1093/genetics/116.1.153
Jensen, L. J., Kuhn, M., Stark, M., Chaffron, S., Creevey, C., Muller, J., et al. (2008). STRING 8—a global view on proteins and their functional interactions in 630 organisms. Nucleic Acids Res. 37(Suppl._1), D412–D416. doi: 10.1093/nar/gkn760
Jones, D. T. (1999). Protein secondary structure prediction based on position-specific scoring matrices11Edited by G. Von Heijne. J. Mol. Biol. 292, 195–202. doi: 10.1006/jmbi.1999.3091
Kaindl, A. M., Passemard, S., Kumar, P., Kraemer, N., Issa, L., Zwirner, A., et al. (2010). Many roads lead to primary autosomal recessive microcephaly. Prog. Neurobiol. 90, 363–383.
Kang, D., Shin, W., Yoo, H., Kim, S., Lee, S., and Rhee, K. (2020). Cep215 is essential for morphological differentiation of astrocytes. Sci. Rep. 10:17000. doi: 10.1038/s41598-020-72728-7
Karczewski, K. J., Weisburd, B., Thomas, B., Solomonson, M., Ruderfer, D. M., Kavanagh, D., et al. (2016). The ExAC browser: displaying reference data information from over 60 000 exomes. Nucleic Acids Res. 45, D840–D845. doi: 10.1093/nar/gkw971
Katoh, K., Misawa, K., Kuma, Ki, and Miyata, T. (2002). MAFFT: a novel method for rapid multiple sequence alignment based on fast fourier transform. Nucleic Acids Res. 30, 3059–3066. doi: 10.1093/nar/gkf436
Kumar, A., Girimaji, S. C., Duvvari, M. R., and Blanton, S. H. (2009). Mutations in STIL encoding a pericentriolar and centrosomal protein, cause primary microcephaly. Am. J. Hum. Genet. 84, 286–290. doi: 10.1016/j.ajhg.2009.01.017
Kumar, A., Markandaya, M., and Girimaji, S. C. (2002). Primary microcephaly:microcephalin and ASPM determine the size of the human brain. J. Biosci. 27, 629–632. doi: 10.1007/BF02708369
Li, H. (2013). Aligning sequence reads, clone sequences and assembly contigs with BWA-MEM. arXiv [preprint] doi: 10.48550/arXiv.1303.3997
Lole, K. S., Bollinger, R. C., Paranjape, R. S., Gadkari, D., Kulkarni, S. S., Novak, N. G., et al. (1999). Full-Length Human Immunodeficiency Virus Type 1 genomes from subtype C-Infected seroconverters in India, with evidence of intersubtype recombination. J. Virol. 73, 152–160. doi: 10.1128/JVI.73.1.152-160.1999
Manichaikul, A., Mychaleckyj, J. C., Rich, S. S., Daly, K., Sale, M., and Chen, W.-M. (2010). Robust relationship inference in genome-wide association studies. Bioinformatics 26, 2867–2873.
Martin, C.-A., Ahmad, I., Klingseisen, A., Hussain, M. S., Bicknell, L. S., Leitch, A., et al. (2014). Mutations in PLK4, encoding a master regulator of centriole biogenesis, cause microcephaly, growth failure and retinopathy. Nat. Genet. 46, 1283–1292. doi: 10.1038/ng.3122
Matsuzaki, F., and Shitamukai, A. (2015). Cell division modes and cleavage planes of neural progenitors during mammalian cortical development. Cold Spring Harb. Perspect. Biol. 7:a015719. doi: 10.1101/cshperspect.a015719
McClellan, J., and King, M.-C. (2010). Genetic heterogeneity in human disease. Cell 141, 210–217. doi: 10.1016/j.cell.2010.03.032
McDonald, J. H., and Kreitman, M. (1991). Adaptive protein evolution at the Adh locus in Drosophila. Nature 351, 652–654. doi: 10.1038/351652a0
McGuffin, L. J., Bryson, K., and Jones, D. T. (2000). The PSIPRED protein structure prediction server. Bioinformatics 16, 404–405. doi: 10.1093/bioinformatics/16.4.404
McKenna, A., Hanna, M., Banks, E., Sivachenko, A., Cibulskis, K., Kernytsky, A., et al. (2010). The genome analysis toolkit: a mapreduce framework for analyzing next-generation DNA sequencing data. Genome Res. 20, 1297–1303. doi: 10.1101/gr.107524.110
Montgomery, S. H., Capellini, I., Venditti, C., Barton, R. A., and Mundy, N. I. (2011). Adaptive evolution of four microcephaly genes and the evolution of brain size in anthropoid primates. Mol. Biol. Evol. 28, 625–638.
Montgomery, S. H., and Mundy, N. I. (2012). Evolution of ASPM is associated with both increases and decreases in brain size in primates. Evolution 66, 927–932.
Montgomery, S. H., and Mundy, N. I. (2014). Microcephaly genes evolved adaptively throughout the evolution of eutherian mammals. BMC Evol. Biol. 14:120. doi: 10.1186/1471-2148-14-120
Morris, J. K., Rankin, J., Garne, E., Loane, M., Greenlees, R., Addor, M.-C., et al. (2016). Prevalence of microcephaly in Europe: population based study. BMJ 354:i4721.
Murrell, B., Wertheim, J. O., Moola, S., Weighill, T., Scheffler, K., and Kosakovsky Pond, S. L. (2012). Detecting individual sites subject to episodic diversifying selection. PLoS Genet. 8:e1002764. doi: 10.1371/journal.pgen.1002764
Ng, P. C., and Henikoff, S. (2003). SIFT: predicting amino acid changes that affect protein function. Nucleic Acids Res. 31, 3812–3814.
Nozawa, M., Suzuki, Y., and Nei, M. (2009). Reliabilities of identifying positive selection by the branch-site and the site-prediction methods. Proc. Natl. Acad. Sci. U S A. 106, 6700–6705. doi: 10.1073/pnas.0901855106
Obenauer, J. C., Cantley, L. C., and Yaffe, M. B. (2003). Scansite 2.0: proteome-wide prediction of cell signaling interactions using short sequence motifs. Nucleic Acids Res. 31, 3635–3641. doi: 10.1093/nar/gkg584
Press, M. F., Xie, B., Davenport, S., Zhou, Y., Guzman, R., Nolan, G. P., et al. (2019). Role for polo-like kinase 4 in mediation of cytokinesis. Proc. Natl. Acad. Sci. U S A. 116, 11309–11318. doi: 10.1073/pnas.1818820116
Puffenberger, E. G., Jinks, R. N., Sougnez, C., Cibulskis, K., Willert, R. A., Achilly, N. P., et al. (2012). Genetic mapping and exome sequencing identify variants associated with five novel diseases. PLoS One 7:e28936. doi: 10.1371/journal.pone.0028936
Purcell, S., Neale, B., Todd-Brown, K., Thomas, L., Ferreira, M. A., Bender, D., et al. (2007). PLINK: a tool set for whole-genome association and population-based linkage analyses. Am. J. Hum. Genet. 81, 559–575. doi: 10.1086/519795
Richards, S., Aziz, N., Bale, S., Bick, D., Das, S., Gastier-Foster, J., et al. (2015). Standards and guidelines for the interpretation of sequence variants: a joint consensus recommendation of the American College of Medical Genetics and Genomics and the Association for Molecular Pathology. Genet. Med. 17, 405–423.
Richardson, T. G., Sanderson, E., Palmer, T. M., Ala-Korpela, M., Ference, B. A., Davey Smith, G., et al. (2020). Evaluating the relationship between circulating lipoprotein lipids and apolipoproteins with risk of coronary heart disease: a multivariable Mendelian randomisation analysis. PLoS Med. 17:e1003062. doi: 10.1371/journal.pmed.1003062
Seyfarth, R. M., and Cheney, D. L. (2002). What are big brains for? Proc. Natl. Acad. Sci U S A. 99, 4141–4142. doi: 10.1073/pnas.082105099
Shen, S., Xiao, W., Zhang, L., Lu, J., Funk, A., He, J., et al. (2021). Prevalence of congenital microcephaly and its risk factors in an area at risk of Zika outbreaks. BMC Pregnancy Childbirth 21:214. doi: 10.1186/s12884-021-03705-9
Sher, A. A., Glover, K. K. M., and Coombs, K. M. (2019). Zika virus infection disrupts astrocytic proteins involved in synapse control and axon guidance. Front. Microbiol. 10:596. doi: 10.3389/fmicb.2019.00596
Spalding, K. L., Bhardwaj, R. D., Buchholz, B. A., Druid, H., and Frisén, J. (2005). Retrospective birth dating of cells in humans. Cell 122, 133–143. doi: 10.1016/j.cell.2005.04.028
Stiles, J., and Jernigan, T. L. (2010). The basics of brain development. Neuropsychol. Rev. 20, 327–348. doi: 10.1007/s11065-010-9148-4
Sun, Y., Liu, F., Fan, C., Wang, Y., Song, L., Fang, Z., et al. (2021). Characterizing sensitivity and coverage of clinical WGS as a diagnostic test for genetic disorders. BMC Med. Genom. 14:102. doi: 10.1186/s12920-021-00948-5
Szklarczyk, D., Gable, A. L., Lyon, D., Junge, A., Wyder, S., Huerta-Cepas, J., et al. (2019). STRING v11: protein-protein association networks with increased coverage, supporting functional discovery in genome-wide experimental datasets. Nucleic Acids Res. 47, D607–D613. doi: 10.1093/nar/gky1131
The GTEx Consortium, Ardlie, K. G., Deluca, D. S., Segrè, A. V., Sullivan, T. J., Young, T. R., et al. (2015). The Genotype-Tissue Expression (GTEx) pilot analysis: multitissue gene regulation in humans. Science 348, 648–660.
Thul, P. J., and Lindskog, C. (2018). The human protein atlas: a spatial map of the human proteome. Protein Sci. 27, 233–244. doi: 10.1002/pro.3307
Van der Auwera, G. A., and O’Connor, B. D. (2020). Genomics in the Cloud: Using Docker, GATK, and WDL in Terra. Sebastopol, CA: O’Reilly Media.
Venkataramanappa, S., Schütz, D., Saaber, F., Kumar, P. A., Abe, P., Schulz, S., et al. (2021). The microcephaly gene Donson is essential for progenitors of cortical glutamatergic and GABAergic neurons. PLoS Genet. 17:e1009441. doi: 10.1371/journal.pgen.1009441
Wang, Y.-Q., and Su, B. (2004). Molecular evolution of microcephalin, a gene determining human brain size. Hum. Mol. Genet. 13, 1131–1137. doi: 10.1093/hmg/ddh127
Wood, B., and Collard, M. (1999). The human genus. Science 284, 65–71. doi: 10.1126/science.284.5411.65
Wright, C. F., FitzPatrick, D. R., and Firth, H. V. (2018). Paediatric genomics: diagnosing rare disease in children. Nat. Rev. Genet. 19, 253–268. doi: 10.1038/nrg.2017.116
Yang, Z. (2007). PAML 4: phylogenetic analysis by maximum likelihood. Mol. Biol. Evol. 24, 1586–1591. doi: 10.1093/molbev/msm088
Keywords: positive selection, rare disease, microcephaly, human-specific traits, protein domains
Citation: Chen J, Ying L, Zeng L, Li C, Jia Y, Yang H and Yang G (2022) The novel compound heterozygous rare variants may impact positively selected regions of TUBGCP6, a microcephaly associated gene. Front. Ecol. Evol. 10:1059477. doi: 10.3389/fevo.2022.1059477
Received: 01 October 2022; Accepted: 17 November 2022;
Published: 02 December 2022.
Edited by:
Andrea Domenico Praticò, University of Catania, ItalyCopyright © 2022 Chen, Ying, Zeng, Li, Jia, Yang and Yang. This is an open-access article distributed under the terms of the Creative Commons Attribution License (CC BY). The use, distribution or reproduction in other forums is permitted, provided the original author(s) and the copyright owner(s) are credited and that the original publication in this journal is cited, in accordance with accepted academic practice. No use, distribution or reproduction is permitted which does not comply with these terms.
*Correspondence: Guang Yang, eWFuZ2d1YW5nQHdjaHNjdS5jbg==