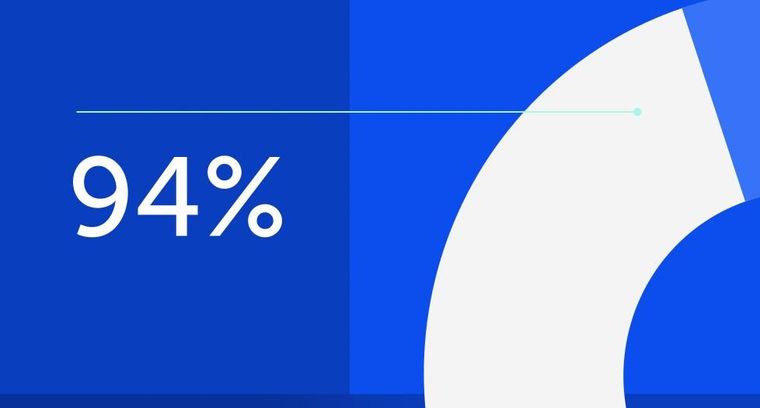
94% of researchers rate our articles as excellent or good
Learn more about the work of our research integrity team to safeguard the quality of each article we publish.
Find out more
ORIGINAL RESEARCH article
Front. Ecol. Evol., 21 November 2022
Sec. Urban Ecology
Volume 10 - 2022 | https://doi.org/10.3389/fevo.2022.1048585
This article is part of the Research TopicEcological Impacts of Domestic Cat Activity on WildlifeView all 6 articles
Free-roaming domestic cats (Felis catus) are known to pose threats to ecosystem health via transmission of zoonotic diseases and predation of native wildlife. Likewise, free-roaming cats are also susceptible to predation or disease transmission from native wildlife. Physical interactions are required for many of these risks to be manifested, necessitating spatial and temporal overlap between cats and wildlife species. Therefore, knowledge of the location and extent of shared habitat and activity periods would benefit management programs. We used data from a 3-year camera trap survey to model species-specific occupancy and identify landscape variables that contribute to the distribution of free-roaming domestic cats and eight native mammal species in Washington, DC. (USA). Our analysis includes five species that are common prey items of domestic cats, and three species that are potential disease vectors or are otherwise known to be a risk to cats. We then predicted the probability of occupancy and estimated the probability of spatial overlap between cats and each native wildlife species at multiple scales. We also used kernel density estimations to calculate temporal overlap between cats and each native wildlife species. Across spatial scales, occupancy for potential disease vector species was generally positively correlated with canopy cover and open water. Prey species were also generally positively correlated with canopy cover, but displayed negative associations with human population density and inconsistent associations with average per capita income. Domestic cat occupancy was negatively correlated with natural habitat characteristics and positively correlated with human population density. Predicted spatial overlap between domestic cats and native wildlife was greatest for potential disease vector species. Temporal overlap was high (>0.50) between cats and all but two native wildlife species, indicating that temporal overlap is probable wherever species overlap spatially. Our findings indicate that the risk to and from domestic cats varies across urban landscapes, but primarily arises from human activities. As such, humans are implicated in the negative outcomes that result from cats interacting with wildlife. Data-driven management to reduce such interactions can aid in cat population management, biodiversity conservation, and public health campaigns.
Free-roaming domestic cats (Felis catus; hereafter, “cats”) impact native wildlife through predation and the transmission of disease, posing serious threats to native wildlife (Fredebaugh et al., 2011; Loss et al., 2013; Loyd et al., 2013; Cove et al., 2018a; Dubey et al., 2020). Likewise, native wildlife can also pose risks to cats such as predation by native species like coyotes (Kays et al., 2015; Larson et al., 2020; Tan et al., 2020) and the transmission of rabies (Roseveare et al., 2009; Gehrt et al., 2013). These bidirectional risks are absent when cats are kept indoors, but manifest themselves when cats are allowed to roam freely outdoors.
The variation of risk across time and space is a fundamental concept in ecology (Brown et al., 1999; Mayor et al., 2009; Gaynor et al., 2019), and is applicable to free-roaming cat management since the bidirectional risks of cats are not uniform across the landscape. For instance, predation by cats is greatest near habitat edges (Kays and DeWan, 2004; Herrera et al., 2022; Pirie et al., 2022), and predation rates fluctuate based on the season and time of day (Thomas et al., 2012; Loyd et al., 2013; Seymour et al., 2020). Likewise, cats face less predation risk where native predators are scarce (Gehrt et al., 2013; Kays et al., 2015), and predation risk is presumably greater for cats whose diel patterns match those of native predators. Similarly, the risk of cat-wildlife disease transmission increases with heightened interactions between species (Beran and Frith, 1988; Theimer et al., 2015). Thus, cats and native wildlife must be in proximity to each other for these risks to be realized, and the risk of negative interactions between species will be greatest where species overlap in space and time (Holmala and Kauhala, 2009).
Location-based management policies that explicitly consider spatial variation in risk are helpful in managing wildlife populations (Stevens and Pfeiffer, 2016; Davis et al., 2021). While permanent removal through adoption or euthanasia ensures elimination of cat-wildlife risks, trap-neuter-return (TNR) remains a common free-roaming cat management strategy that is spatially explicit—cats are trapped, sterilized, and returned to the same location from which they were trapped (Longcore et al., 2009). However, this attention to location when releasing cats is primarily concerned with the individual cat’s familiarity with its surroundings (Alley Cat Allies [ACA], 2022), rather than to abate risks to or from cats. If the spatial variation in risk was an additional consideration in free-roaming cat management, cats might be spatially separated from areas in which high risk outcomes are likely. The degree to which cats and native wildlife overlap can thus be used to quantify the risk of negative outcomes between cats and native wildlife.
Here we assess the habitat use and diel patterns of cats and eight native mammal species in Washington, DC. We assessed landscape variables across multiple spatial scales to account for variations in species’ spatial scale of response (Rettie and Messier, 2000; Gallo et al., 2018; Moll et al., 2020). We also predicted the probability of spatial overlap of cats and each native species to assess apparent risk of being preyed upon by cats or serving as a disease or predation risk to cats. We asked the following research questions: (1) what natural and anthropogenic features of the urban environment most influence the distribution of cats and native mammal species in urban areas?, (2) what is the probability of spatial overlap between cats and native mammal species?, and (3) to what degree do activity patterns overlap between cats and native mammal species?
We hypothesized that the presence of native mammal species would exhibit a positive correlation with natural features of the urban environment (e.g., tree cover, open water), but would have a negative correlation with most anthropogenic features (e.g., human population density and traffic). Due to the luxury effect commonly seen in urban ecosystems, we also hypothesized that native mammals would exhibit a positive association with average per capita income (Magle et al., 2021). Conversely, we hypothesized that the presence of cats would be negatively influenced by natural features and positively influenced by anthropogenic factors, but would display a negative relationship with average per capita income (Gehrt et al., 2013; Cove et al., 2019, in press; Vanek et al., 2020). Because we hypothesize that the distribution of cats and native mammals would occupy different ends of an urban-natural continuum, we predicted that the degree of spatial overlap would be highest at the fringes of either species’ habitat requirements and thus allow for spatially explicit management policies. We further predicted that the known variation in cat diel activity would result in substantial activity overlap between cats and all native mammal species (Vanek et al., 2020; Bennett et al., 2021). Our findings identify areas with the greatest potential for bidirectional risk and can inform management policies that considers the wellbeing of cats and wildlife alike.
Washington, District of Columbia (hereafter “D.C.”) has a population of approximately 7,05,750 residents (∼4,466 people per km2; U.S. Census Bureau, 2019), and an estimated 7,296 free-roaming domestic cats (of which ∼35% have undergone TNR; Cove et al., 2019, in press). D.C. contains two major rivers and is situated at the ecotone of the coastal plains and Piedmont regions (Ossi et al., 2015). The climate is temperate/tropical with an average of 110 cm of annual precipitation and average annual temperatures ranging from 21.6 to 6.9°C (National Oceanic and Atmospheric Administration [NOAA], 2021). An extensive park system is situated within the densely developed city (Harnik et al., 2017), resulting in a heterogeneous landscape of developed gray space, landscaped greenspace, and semi-natural greenspace (e.g., forested parks). D.C. resides in the ancestral homeland of the Nacochtank (also called Anacostan) people, and later the Piscataway people (Tayac, 2009).
Passive infrared-sensor camera traps (Reconyx HyperFire 2, Reconyx Inc., 3828 Creekside Ln, Ste 2, Holmen, WI 54636) were deployed across Washington, DC. between September-December 2018 (n = 192), April 2019–January 2020 (n = 867), and June 2020–October 2020 (n = 471). To select camera locations, a 64 ha grid was superimposed on the study area, and grid cells to be sampled were chosen based on the median household income and predominant landcover categories within each grid cell (see Herrera et al., 2021 and Flockhart et al., 2022 for details on the study design). Cameras were placed at a location (sampling site) within each chosen grid cell based on accessibility and permitting. Although this sampling grid informed the original selection of sampling sites, these social-environmental variables were not used for this analysis.
Camera locations included public greenspaces (n = 796), private residences and institutions (n = 441), alleys (n = 265), and sidewalks (n = 28). Each camera deployment consisted of a single camera aimed at a probable corridor of animal movement (e.g., game trails, fence lines, etc.) from a height of <0.5 m, and was affixed to a vertical substrate using a nylon strap and cable lock. Each time a camera was triggered, five consecutive photographs were taken with no delay period. At each sampling site, a camera was continuously active for 15 consecutive days and sites were not resampled (Herrera et al., 2021; Flockhart et al., 2022). Over the course of the study, 11 cameras were stolen, 8 deployments were tampered with such that they were no longer collecting data, and 3 cameras were damaged beyond repair after being struck by vehicles.
Photographs taken within 1 min of each other were considered a single observation. All photographs were separately reviewed by two individuals, and all animals within each sequence were identified to species. If species-level identification was not possible, animals were identified to the lowest taxonomic level afforded by the photographs. Observations without animals visible were coded as “blank.” All photographs were uploaded to the eMammal photo repository (McShea et al., 2016; see also http://emammal.si.edu).
Three grid arrays, each at different scales, were overlaid on our study area using ArcMap 10.8 (ESRI, 380 New York Street, Redlands, CA, USA). Each spatial scale was greater than the average domestic cat home range in urban areas (0.036 ± 0.056 km2; Kays et al., 2020) to achieve spatial independence, and included: 400 × 400 m (0.16 km2, hereafter “small”), 800 × 800 m (0.64 km2, hereafter “medium”), and 1 × 1 km2 (1 km2, hereafter, “large”) cell sizes (Figure 1). Grid cells were only included in our analysis if at least half of the cell’s area was within D.C.’s administrative boundary (Figure 1). At each spatial scale, observations from all cameras (sampling sites) located together within a respective grid cell were combined and considered a single sampling unit (Figure 1). Respectively, 50, 71, and 77% of the total small, medium, and large grid cells were sampled.
Figure 1. (A) All camera locations (sampling sites) in Washington, DC. (B–D) Sampling grids for each spatial scale. Data from all cameras within each sampling cell were pooled together. Sampling block colors indicate the number of cameras deployed within each sampling cell.
At each spatial scale, observation data from all cameras located within each grid cell were pooled to create daily detection histories (detected = 1, not detected = 0, not surveyed = NA) for the respective cell using the package “CamtrapR” in R version 4.1.2 (Niedballa et al., 2016; RStudio Team, 2019; R Core Team, 2020). Species included in this analysis were domestic cat due to its centrality to the research questions, and eastern chipmunk (Tamias striatus), eastern cottontail (Sylvilagus floridanus), eastern gray squirrel (Sciurus carolinensis), groundhog (Marmota monax), and white-footed mouse (Peromyscus leucopus) because they are native species known to be depredated by domestic cats (George, 1974; Loyd et al., 2013; Paul and Friend, 2020; Herrera et al., 2022). Raccoon (Procyon lotor), red fox (Vulpes vulpes), and Virginia opossum (Didelphis virginiana) were also included because they are disease vectors or are otherwise known to be a risk to cats through direct conflict or interference competition (Carey and McLean, 1983; Jenkins et al., 1988; Sogliani and Mori, 2019; Zecca et al., 2020; Hennessy and Hild, 2021). Although the dynamics between coyotes (Canis latrans) and cats is a topic of growing ecological interest (e.g., Larson et al., 2020), coyote observations (n = 43) from our study area were too sparse to sufficiently analyze.
To estimate and predict the spatial distribution of each species we fitted single-season occupancy models (MacKenzie et al., 2002) for each species at each spatial scale. Occupancy models estimate the probability of a species occurring at a location, while accounting for imperfect detection (MacKenzie et al., 2002, 2017).
We broadly hypothesized that forested habitat (Bozek et al., 2007; Fidino et al., 2016; Lombardi et al., 2017), water sources (e.g., Fidino et al., 2016; Lombardi et al., 2017; Wait and Ahlers, 2020), human population density (e.g., Luck, 2007; Lombardi et al., 2017), vehicular traffic (e.g., Goodwin and Shriver, 2011; Chen and Koprowski, 2015), and per capita income (e.g., Hope et al., 2003; Clarke et al., 2013; but see also Magle et al., 2021) would influence the probability of presence for each species. We initially considered 23 variables that represented these broader categories and calculated them for each grid cell at each spatial scale (see Supplementary Appendix 1 for a list of variables and methods for calculating each variable). We calculated the variance inflation factor for each mean-centered variable at each scale using the package “USDM” in R (Naimi et al., 2014). Variables with a high degree of collinearity (variance inflation factor > 3) at any scale were removed from the overall analysis such that each spatial scale contained the same set of variables. A single variable was then selected for each category, with preference given to those that were considered more directly interpretable or deemed to have greater ecological significance. The final variables included in our models were percent canopy cover (forested habitat), percent open water, human population density, average daily traffic volume of roads within the sampling cell (vehicular traffic), and average per capita income (neighborhood income). All variables were scaled and centered to have a mean of 0 and standard deviation (SD) of 1.
To account for imperfect detection, we considered the number of cameras active in each grid cell, daily temperature, daily precipitation, the presence of fog, and moon phase on detection probability. The number of cameras active in each grid cell per day was calculated using CamtrapR (Niedballa et al., 2016). Daily precipitation (cm), presence of fog (yes = 1, no = 0), and daily maximum temperature (°C) data were obtained from local weather stations [National Oceanic and Atmospheric Administration (NOAA), 2021]. Precipitation and temperature data were averaged if readings were available from multiple stations. The percent visible moon for the Eastern time zone was calculated for each sampling day using SKYCAL (National Aeronautics and Space Administration [NASA], 2021) based on the time passed since the last new (0) or full (1) moon and did not consider nightly cloud cover. Environmental detection variables were unique to each sampling day rather than the sampling cell, while the number of active cameras was unique to each sampling cell. All variables were scaled and centered to have mean of 0 and SD of 1.
We fitted single-season Bayesian occupancy models (MacKenzie et al., 2017) using the “ubms” package in R (Kellner et al., 2021), which uses “rstan” for MCMC computation (Stan Development Team, 2020). A single fully parameterized model was fit for each species at each spatial scale. All parameters including intercepts were given vague Normal priors with mean 0 and SD 1 (Northrup and Gerber, 2018). MCMC chains were constructed using the No-U-Turn Sampler, an extension of the Hamiltonian Monte Carlo algorithm (Hoffman and Gelman, 2014). Four chains were constructed for each parameter, where each chain consisted of 100,000 iterations and a burn-in of 50,000 iterations. Model convergence was assessed by visually inspecting each chain’s trace plot, ensuring the Gelman–Rubin statistics were <1.1, and ensuring that all effective sample sizes were greater than 127,000. We considered any variable to have evidence of an effect if the 95% Bayesian credible intervals did not overlap zero.
Multiple techniques were used to assess model fit. Model residuals were compared against predicted values (both occupancy and detection) to assess the variation of deviance within each model, where the distribution of residuals was visually assessed for skew or clustering along the distribution of predicted values (Gelman et al., 2000; Broms et al., 2016; Wright et al., 2019). Posterior post-predictive values were obtained by using the model to simulate 100 datasets and comparing the proportion of zeros in these datasets against the proportion of zeros present in the observed dataset at each spatial scale, with matching proportions indicating good model fit (Broms et al., 2016; Wright et al., 2019). Additionally, the Pareto k diagnostic values were calculated for each model, where values less than 0.5 indicated good model fit (Vehtari et al., 2017). Finally, the MacKenzie–Bailey chi-squared statistic and resulting Bayesian p-value were also calculated for each model, where the Bayesian p-value is interpreted similarly to a frequentist p-value (MacKenzie and Bailey, 2004; but see also Wright et al., 2016). Diagnostic tests were conducted using the “ubms” package (Kellner et al., 2021), and indicated good model fit for all models (Supplementary Appendix 2).
To assess the spatial overlap of each native wildlife species with cats, we calculated the percent canopy cover, percent open water, human population density, average daily traffic volume, and average per capita income for all grid cells across the study area, and used our occupancy models to predict the probability of occupancy for each species in each grid cell (including unsampled cells) at each spatial scale. The probability of spatial overlap between cats and each native wildlife species was calculated for each grid cell by multiplying the posterior distribution of predicted occupancy probabilities for cats by the posterior distributions of predicted occupancy probabilities for each wildlife species. We derived this parameter for all grid cells at all three spatial scales. When predicting occupancy probabilities across the landscape, each detection variable was held constant at its respective mean value.
We considered each species’ 95% kernel activity isopleth, the smallest duration of time in which 95% of observations occur, to be each species’ active period (Oliveira-Santos et al., 2013). Prior to calculation, observation timestamps were converted from a linear timescale to a circular timescale by equating each timestamp to its respective value between 0 and 1 (e.g., noon = 0.5, etc.), then multiplying each value by 2π to arrive at radians (Ridout and Linkie, 2009). The “circular” package was then used to calculate a 95% kernel isopleth with a smoothing parameter of 5 (Oliveira-Santos et al., 2013; Agostinelli and Lund, 2022). The smoothing parameter accounts for inordinate peaks in activity which might arise from different criteria to bin multiple observations into a single observation (Oliveira-Santos et al., 2013). Mean observation times were also calculated, then back-transformed to a linear timescale for ease of interpretation.
Activity overlap was calculated using circular time via the “overlap” package in R. A smoothing parameter of 3 was used per Ridout and Linkie (2009). The estimate of overlap between cats and each species () consists of the proportion of the larger activity curve that is shared by the smaller activity curve. The overlap estimate is reported as a value between 0 and 1, where 0 indicates no overlap and 1 indicates identical activity patterns (Lashley et al., 2018). Cumulative species-level activity estimates were assumed to be representative of individual activity patterns (Rowcliffe et al., 2014).
Over 22,268 trap nights, we detected 22 mammal species in Washington, DC. We collected 33,134 observations of domestic cat; 31,966 of eastern gray squirrel; 8,067 of raccoon, 2,785 of red fox, 2,407 of Virginia opossum, 1,044 of eastern cottontail, 980 of eastern chipmunk, 717 of groundhog, and 448 of white-footed mouse.
At the largest scale (1 km × 1 km), the 95% credible interval for each variable overlapped zero, indicating no strong effect of the variables that we analyzed at the largest spatial scale (Figure 2 and Supplementary Appendix 2). At the medium scale (800 m × 800 m), open water had the greatest negative influence on cat occupancy (βM = −1.68, 95% BCI = −2.66 to −0.76) followed by canopy cover (βM = −0.78, 95% BCI = −1.25 to −0.33). Cats were 46.4% less likely to occur (odds ratio (OR) = 0.55, 95% BCI = 0.26–0.83) at sites with a 1 SD increase from the mean percent open water, and 18.9% less likely to occur (OR = 0.81, 95% BCI = 0.67–0.92) with a one SD increase from the mean canopy cover. No variables had a positive effect on cat occupancy at the medium scale (Figure 3).
Figure 2. Posterior distributions of model coefficients for (A) domestic cat, (B) groundhog, (C) eastern gray squirrel, (D) red fox, (E) white-footed mouse, (F) raccoon, (G) eastern cottontail, (H), Virginia opossum, and (I) eastern chipmunk. Solid blue lines indicate the full posterior distribution and shaded regions represent the 95% Bayesian credible interval. Vertical blue lines denote the median posterior value. Distributions colored in light blue indicate that the 95% credible interval overlapped zero.
Figure 3. Occupancy probabilities for domestic cats across Washington, DC, at three spatial scales using the mean posterior value for each parameter. Cooler colors signify lower occupancy probability, while warmer colors signify higher occupancy probability. Initials below each map denote the variables whose 95% credible interval did not overlap zero, in their order of magnitude (W = water, C = canopy cover, P = human population density, I = per capita income, T = traffic). A positive sign preceding each initial signifies a positive relationship, while a negative sign signifies a negative relationship. Maps show predicted occupancy using the median value for each parameter. Maps depicting predicted occupancy at the upper and lower limits of the 95% credible interval are available in Supplementary Appendix 4.
Open water also had the greatest negative effect on cat occupancy at the smallest scale (400 m × 400 m; βS = −1.30, 95% BCI = −1.86 to −0.80), followed by canopy cover (βS = −0.68, 95% BCI = −0.94 to −0.44), and per capita income (βS = −0.40, 95% BCI = −0.68 to −0.14). An increase of 1 SD from the mean in canopy cover decreased cat occupancy by 24.4% (OR = 0.76, 95% BCI = 0.66–0.85). Cat occupancy would also decrease 46.9% (OR = 0.53, 95% BCI = 0.34–0.73) with a one SD increase from the mean of open water and 14.1% (OR = 0.86%, 95% BCI = 0.76–0.95) with a one SD increase from the mean per capita income. Human population density had the greatest positive effect on cat occupancy at the smallest scale (βS = 0.83, 95% BCI = 0.48–1.23). Cats were 23% more likely to occur (OR = 1.23, 95% BCI = 1.14–1.35) at sites with an increase of one SD from the mean human population density. The mean probability of domestic cat occupancy across the entire study area was 0.66 (0.28 SD) at the small spatial scale, 0.75 (0.26 SD) at the medium spatial scale, and 0.81 (0.21 SD) at the large spatial scale (Table 1 and Supplementary Appendix 3).
Table 1. Mean predicted occupancy across Washington, DC, for each species, and mean predicted overlap between cats and native mammal species.
At the largest scale, percent canopy cover had a positive effect on red fox (βL = 1.0, 95% BCI = 0.22–2.05) and raccoon (βL = 0.96, 95% BCI = 0.12–1.91) occupancy, and per capita income had a negative effect on Virginia opossum occupancy (βL = −0.76, 95% BCI = −1.32 to −0.24). All other variables’ 95% credible intervals overlapped zero (Figure 2 and Supplementary Appendix 3).
Canopy cover also had a positive effect on raccoon occupancy at the medium scale (βM = 1.10, 95% BCI = 0.38–1.96) and per capita income had a negative effect on Virginia opossum occupancy (βM = −0.95, 95% BCI = −1.44 to −0.50; Figure 2). Red fox occupancy at the medium scale was positively correlated with canopy cover (βM = 1.82, 95% BCI = 1.07–2.63), open water (βM = 1.63, 95% BCI = 0.32–3.03), and daily traffic volume (βM = 1.12, 95% BCI = 0.10–2.17), but negatively correlated with human population density (βM = −0.95, 95% BCI = −1.88 to −0.02; Figure 2).
At the smallest scale, canopy cover had a positive effect on red fox (βS = 1.75, 95% BCI = 1.33–2.21), raccoon (βS = 1.42, 95% BCI = 1.05–1.83), and Virginia opossum (βS = 0.41, 95% BCI = 0.19–0.63) occupancy, and open water had a positive effect on red fox (βS = 0.74, 95% BCI = 0.20–1.31) and raccoon (βS = 0.55, 95% BCI = 0.08–1.07) occupancy (Figure 2). Human population density had a negative effect on the presence of red fox (βS = −0.70, 95% BCI = −1.16 to −0.34) and raccoon (βS = −0.38, 95% BCI = −0.69 to −0.09), and per capita income had a negative effect on raccoon (βS = −0.31, 95% BCI = −0.58 to −0.05) and Virginia opossum (βS = −0.87, 95% BCI = −1.14 to −0.62) occupancy (Figure 2 and Table 1). ORs for each species and each variable at 1 SD from the mean are available in Supplementary Table 2 and Supplementary Appendix 3.
At the large spatial scale, canopy cover had a positive effect on eastern chipmunk occupancy (βL = 1.20, 95% BCI = 0.59–1.89), per capita income had a negative effect on groundhog occupancy (βL = −1.53, 95% BCI = −2.26 to −0.88), and human population density had a negative effect on white-footed mouse occupancy (βL = −1.33, 95% BCI = −2.56 to −0.19). Each variable’s 95% credible interval overlapped zero at the large scale for both eastern cottontails and eastern gray squirrels (Figure 2, Supplementary Table 1, and Supplementary Appendix 3).
At the medium scale, per capita income had a positive effect on eastern cottontail (βM = 0.42, 95% BCI = 0.03–0.83) and eastern chipmunk (βM = 1.19, 95% BCI = 0.62–1.80) occupancy (Figure 2), but had a negative effect on groundhog occupancy (βM = −1.48, 95% BCI = −2.09 to −094). Human population density (βM = −0.87, 95% BCI = −1.57 to −0.21) and traffic volume (βM = −0.84, 95% BCI = −1.80 to −0.04) also had negative effects on groundhog occupancy (Figure 2). Canopy cover had a positive effect on both eastern chipmunk (βM = 1.50, 95% BCI = 0.92–2.14) and white-footed mouse (βM = 0.84, 95% BCI = 0.32–1.43) occupancy (Figure 2). Similar to the larger scale results, each variable’s 95% credible interval overlapped zero for eastern gray squirrels (Figure 2, Supplementary Table 1, and Supplementary Appendix 3).
At the smallest scale, canopy cover had a positive effect on occupancy for eastern chipmunk (βS = 0.87, 95% BCI = 0.57–1.18), white-footed mouse (βS = 0.62, 95% BCI = 0.29–0.97), and eastern gray squirrel (βS = 1.10, 95% BCI = 0.51–1.75). Per capita income had a positive effect on occupancy for eastern cottontail (βS = 0.40, 95% BCI = 0.11–0.71) and eastern chipmunk (βS = 1.25, 95% BCI = 0.81–1.73), but had a negative effect on groundhog occupancy (βS = −1.46, 95% BCI = −1.92 to −1.06; Figure 1). Human population density also had a negative effect on groundhog occupancy (βS = −0.55, 95% BCI = −0.89 to −0.20; Figure 1). ORs for each parameter can be found in Supplementary Table 2 and Supplementary Appendix 3.
Predicted overlap of cats and wildlife species varied by species and scale. Vector species displayed greater predicted overlap with cats than did prey species (Table 1). Across species, predicted overlap was greatest at the largest spatial scale (Figure 4 and Table 1). The species with the highest probability of overlap with cats was raccoon, with a mean probability of overlap of 0.72 at the largest spatial scale (Table 1). Eastern chipmunk has the lowest probability of overlap with cats, with a mean probability of overlap of 0.06 at the smallest spatial scale (Table 1).
Figure 4. Predicted occupancy probabilities and probability of spatial overlap with cats for (A) groundhogs, (B) eastern gray squirrels, (C) red fox, (D) white-footed mice, (E) raccoon, (F) eastern cottontail, (G), Virginia opossum, and (H) eastern chipmunk across Washington, DC. Occupancy color gradient is identical to Figure 2. For each species, the top row depicts the median occupancy probability across the study area. The second row for each species depicts the median probability of spatial overlap. Maps depicting predicted occupancy and overlap probabilities at the upper and lower limits of the 95% credible interval are available in Supplementary Appendix 4.
Cats exhibited a cathemeral activity pattern, with a 95% activity isopleth–the smallest duration of time that contains 95% of observations (hereafter, “isopleth”) – of 22.45 h with a mean activity time of approximately midnight. Red fox, raccoon, and Virginia opossum adhered to nocturnal activity patterns, with an isopleths of 17.77, 13.56, and 13.19 h and mean activity times of approximately 00:15, 01:30, and 00:45, respectively. Eastern cottontail and white-footed mouse were the only prey species to also exhibit a nocturnal activity pattern, displaying isopleths of 18.32 and 15.60 h with a mean activity time of 01:20 and 00:00, respectively. Groundhog, chipmunk, and eastern gray squirrel all adhered to diurnal activity patterns, and displayed isopleths of 15.17, 13.86, 14.20 h and mean activity times of 13:30, 12:00, and 13:15, respectively.
The coefficient of overlap () between cats and wildlife was greatest in nocturnal species. Red fox had the highest degree of overlap of the vector species ( = 0.73), while overlap between cats and raccoons ( = 0.56) and Virginia opossums ( = 0.54) was lower. Eastern cottontail displayed the greatest temporal overlap with cats of the prey species ( = 0.78), followed by white-footed mouse ( = 0.69). Diurnal prey species—i.e., eastern gray squirrel ( = 0.45), groundhog ( = 0.52) and eastern chipmunk ( = 0.45)—displayed less activity overlap with cats than did nocturnal prey species but still exhibited temporal overlap near or greater than 50% (Figure 5 and Supplementary Appendix 5).
Figure 5. Activity patterns and temporal overlap plotted on a 24 h clock for panel (A) cats, (B) groundhogs, (C) eastern gray squirrels, (D) red fox, (E) white-footed mice, (F) raccoon, (G) eastern cottontail, (H), Virginia opossum, and (I) eastern chipmunk. Plots depict cat activity density and mean activity time using a dark blue line, while the light blue polygon and white line are used for the species of interest. Activity overlap (area beneath both curves) is colored in dark blue. Note that plot (A) utilizes a different scale than plots (B–I).
Our analysis suggests that cats and native mammal species overlap both spatially and temporally across Washington, DC. Since temporal overlap was high (>0.50) between cats and all native species except eastern gray squirrel and eastern chipmunk, our analyses suggest that species are likely to share active hours wherever they overlap spatially. Because cat-wildlife activity overlap is plausible across the diel period, the risk of negative outcomes such as predation and rabies transmission is more greatly mediated by spatial rather than temporal overlap. We used single-season occupancy models at three spatial scales to identify species-habitat relationships of free-roaming domestic cats and eight native mammal species common in urban areas. We then used our model results to estimate the probability of spatial overlap between native mammals and domestic cats based on the most influential landscape characteristics for each species (Figure 4).
Our findings largely supported our first and second hypotheses that native mammal occupancy is correlated with natural variables, while cat occupancy is correlated with anthropogenic variables. We also used kernel density functions to estimate diel patterns and activity overlap between cats and each species (Figure 5). The results from this analysis supported our third hypothesis that temporal overlap is substantial between cats and native mammals. These findings suggest that species interactions are not likely to be mediated by conflicting diel patterns, but rather that temporal overlap is possible wherever there is spatial overlap. Given the inherent risks presented by interactions among cats and native mammals, excluding cats from areas with high probabilities of spatial overlap with native species would preserve the safety and welfare of both cats and native wildlife.
Our analysis found considerable temporal overlap between cats and most mammal species. High temporal overlap at the species level is likely due to the cathemeral activity pattern displayed by cats allowing for temporal overlap with other species across the entire diel period regardless of those individual species’ diel patterns. Individual cats are not continuously active, but the collective cathemeral pattern is achieved by staggered active periods among individuals (Oliveira-Santos et al., 2013). Although our study examined the collective activity pattern of cats, management efforts may benefit from future research which examines how these activity patterns differ across the urban landscape and correlate with landscape characteristics (Gallo et al., 2022). Because the species-level opportunity for temporal overlap is relatively indiscriminate, opposing diel periods between cats and other mammal species cannot be relied upon to predict interactions between cats and wildlife. Rather, spatial overlap may prove more insightful since cats (as a species) can be considered as constantly active wherever they occur across the urban landscape.
The spatial distribution of cats was not associated with any variables at the large spatial scale, indicating that the distribution of cats is primarily influenced by smaller-scale landscape features. At the medium and small spatial scales, cats demonstrated negative associations with open water and canopy cover (natural features) but had positive associations with high human population density and low-income areas (anthropogenic features). These findings are consistent with previous research on outdoor cats that have found free-roaming cats tend to stay within approximately 100 m of their home and spend most of their time (>90%) in built environments (Gehrt et al., 2013; Doherty et al., 2015; Cove et al., 2018b, in press; Kays et al., 2020). Our results further support the notion that humans influence where cats are found in urban areas rather than habitat availability alone (Doherty et al., 2015).
Since humans heavily influence the spatial distribution of cats, humans also determine the risks faced by cats and the risks that cats impose on native wildlife–signifying that actions can be taken to mitigate these bidirectional risks. Prohibiting the release (e.g., TNR) or subsidization (e.g., feeding) of cats in areas where spatial overlap with vector species is high may reduce the risk of disease transmission (Gerhold and Jessup, 2013; Theimer et al., 2015). Likewise, limiting cats from areas of high spatial overlap with native prey species would likely reduce mortality from cats (Seymour et al., 2020; Herrera et al., 2022). Environmental characteristics which correlate with species-specific habitat use can offer insight regarding potential spatial overlap, and thus inform management practices which reduce cat-wildlife interactions.
We found that in most cases, native mammal species exhibited the opposite habitat requirements compared to cats. While cats had a negative association with canopy cover and water at the small and medium scales, all native species except groundhog and white-footed mouse exhibited positive responses to canopy cover, and red fox and racoon had a positive response to water at the same scales. Conversely, cats had a higher probability of occupancy in areas of high human population density at the smallest spatial scale, yet groundhog, red fox, and raccoon had a negative response to human population density at that same scale. These divergent habitat relationships suggest that cats and native mammals can be spatially separated in the urban environment if cat population management is considerate of these differences and prohibits cats near favorable wildlife habitat.
The risk of disease transmission between cats and wildlife is still possible regardless of spatial (e.g., transmission of toxoplasmosis to other ecosystems via runoff; Conrad et al., 2005) or temporal (e.g., transmission of toxoplasmosis via fecal oocytes; Davis et al., 2018) overlap, but is most apparent when both spatial and temporal overlap are achieved (Holmala and Kauhala, 2009). Unlike toxoplasmosis, rabies requires physical interaction to be transmitted (Beran and Frith, 1988). Of the three vector species included in this analysis, raccoon and red fox are the most likely to transmit rabies to cats (Carey, 1982; Jenkins et al., 1988; Blanton et al., 2010; Ma et al., 2021). The mean probabilities of spatial overlap between domestic cats and either raccoon or red fox were substantial (Figure 4). The notable degree of spatial overlap between cats and these species may facilitate routes of transmission between the species, and subsequently to humans (Roseveare et al., 2009; Keesing and Ostfeld, 2021), bearing troubling implications for both public health and animal welfare.
Vaccination of animals significantly reduces the risk of disease transmission throughout a community (Roebling et al., 2014; Pieracci et al., 2019), but is not a reliable means of stopping transmission among free-roaming cats. Cat population control efforts, such as TNR, often vaccinate cats prior to their release (e.g., Kreisler et al., 2019). However, cats enrolled in TNR programs are rarely trapped more than once, allowing some vaccines to expire within their lifetime (Roebling et al., 2014). Furthermore, herd immunity cannot be achieved if the transmission rate exceeds the vaccination rate, which is often the case for TNR programs (Roebling et al., 2014). Since vaccination of free-roaming cats does not reliably inhibit disease transmission, mitigation strategies must rely on the disruption of transmission via restricting contact between cats and common vectors (Beran and Frith, 1988; Roebling et al., 2014). Management practices that restrict free-roaming cats from areas where such contact is probable (areas of spatial overlap) can achieve such disruption (Roebling et al., 2014).
The risk of zoonotic disease transmission is further amplified by biodiversity loss (Jones et al., 2008; Johnson et al., 2020; Keesing and Ostfeld, 2021), such as that attributed to free-roaming cats (Loss et al., 2013; Loyd et al., 2013; Doherty et al., 2016; Loss and Marra, 2017). Thus, native biodiversity would benefit from management that simultaneously seeks to preserve habitat and remove non-native predators such as free-roaming cats from habitat patches. Yet, these efforts could be thwarted by cats living outside of, but still able to access, those habitat patches. Our study found that spatial overlap between cats and prey was relatively limited due to divergent habitat associations. However, cats are known to have higher rates of predation at forest edges (Kays and DeWan, 2004; Herrera et al., 2022; Pirie et al., 2022). Location-specific cat management policies that minimize free-roaming cats based on the probability of species overlap might address this concern by limiting cat populations in habitat-adjacent areas where the probability of overlap is still relatively high.
Our study identifies landscape variables that contribute to the occupancy of several native mammal species in urban areas. Our findings vary by species and scale, but broadly indicate that wildlife species are primarily driven by habitat availability (canopy cover and access to water), while free-roaming domestic cats display inverse associations with habitat availability and respond positively to anthropogenic variables (human population density and low socioeconomics). Furthermore, our study finds that cats are active, at the population level, across the entire diel period. Thus, if a wildlife species overlaps with cats spatially, it also overlaps with cats temporally to some degree. Free-roaming cat management programs and public health programs alike would benefit from the explicit consideration of potential species overlap across the landscape. Since temporal overlap is ubiquitous, management strategies should focus on spatially separating cats from wildlife, and thereby reduce risks to both populations. While such risks can be avoided altogether by keeping cats indoors—until that goal is realized—removing or excluding cats from areas with high probabilities of spatial overlap with various wildlife species would provide an intermediate solution that simultaneously protects biodiversity and the wellbeing of domestic cats.
Observation data is publicly available via the eMammal repository at https://emammal.si.edu/analysis/data-download with the Project Name ‘D.C. Wildlife Project.’ Data associated with occupancy and detection variables are also publicly available, and their sources are cited within this manuscript. Further inquiries can be directed to the corresponding author.
Ethical review and approval was not required for the animal study because of the non-invasive nature of camera traps.
TF, WM, and MC designed the initial sampling protocol. DH, SM, MC, and SD collected and processed the data. DH and TG analyzed the data and wrote the original manuscript draft. All authors contributed revisions to the manuscript.
Major financial support was provided by the PetSmart Charities, American Society for the Prevention of Cruelty to Animals, The Humane Society of the United States, Humane Rescue Alliance, Winn Feline Foundation, Maddie’s Fund, Cat Depot, and B. Von Gontard.
We thank Lauren Lipsey and Erin Robinson for their administrative assistance, Justin Belsley, Claire Bresnan, Elly Grant, Neha Singh, and Sam Newkirk for their assistance in data collection, and Jen Zhao, Haydee Hernandez-Yanez, Helen Bontrager, Ashley Whipple, Johnny Stutzman, Ben Ranelli, Emily Renkey, and Jamie Fetherolf for their assistance with data processing and storage. We also wish to thank the facilities and support staff at the Humane Rescue Alliance and George Mason University for providing a safe and functional working environment.
Author TF was employed by Flockhart Consulting.
The remaining authors declare that the research was conducted in the absence of any commercial or financial relationships that could be construed as a potential conflict of interest.
All claims expressed in this article are solely those of the authors and do not necessarily represent those of their affiliated organizations, or those of the publisher, the editors and the reviewers. Any product that may be evaluated in this article, or claim that may be made by its manufacturer, is not guaranteed or endorsed by the publisher.
The Supplementary Material for this article can be found online at: https://www.frontiersin.org/articles/10.3389/fevo.2022.1048585/full#supplementary-material
Agostinelli, C., and Lund, U. (2022). R package ‘circular’: Circular statistics (version 0.4-95). Available online at: https://r-forge.r-project.org/projects/circular/ (accessed August 1, 2022).
Alley Cat Allies [ACA] (2022). Feral cats safe relocation tips [Internet]. Bethesda, MD: Alley Cat Allies.
Bennett, K. F. P., Evans, B. S., Clark, J. A., and Marra, P. P. (2021). Domestic cat abundance and activity across a residential land use gradient. Front. Ecol. Evol. 9:e643845. doi: 10.3389/fevo.2021.643845
Beran, G. W., and Frith, M. (1988). Domestic animal rabies control: An overview. Rev. Infect. Dis. 10, 672–677. doi: 10.1093/clinids/10.supplement_4.s672
Blanton, J. D., Palmer, D., and Rupprecht, C. E. (2010). Rabies surveillance in the United States during 2009. J. Am. Vet. Med. Assoc. 237, 646–657. doi: 10.2460/javma.237.6.646
Bozek, C. K., Prange, S., and Gehrt, S. D. (2007). The influence of anthropogenic resources on multi-scale habitat selection by raccoons. Urban Ecosyst. 10, 413–425. doi: 10.1007/s11252-007-0033-8
Broms, K. M., Hooten, M. B., and Fitzpatrick, R. M. (2016). Model selection and assessment for multi-species occupancy models. Ecology 97, 1759–1770. doi: 10.1890/15-1471.1
Brown, J. S., Laundré, J. W., and Gurung, M. (1999). The ecology of fear: Optimal foraging, game theory, and tropic interactions. J. Mammal. 80, 385–399. doi: 10.2307/1383287
Carey, A. B. (1982). The ecology of red foxes, gray foxes, and rabies in the eastern United States. Wildl. Soc. Bull. 10, 18–26.
Carey, A. B., and McLean, R. G. (1983). The ecology of rabies: Evidence of co-adaptation. J. Appl. Ecol. 20, 777–800. doi: 10.2307/2403126
Chen, H. L., and Koprowski, J. L. (2015). Animal occurrence and space use change in the landscape of anthropogenic noise. Biol. Conserv. 192, 315–322. doi: 10.1016/j.biocon.2015.10.003
Clarke, L. W., Jenerette, G. D., and Davila, A. (2013). The luxury of vegetation and the legacy of tree biodiversity in Los Angeles, CA. Landsc. Urban Plan. 116, 48–59. doi: 10.1016/j.landurbplan.2013.04.006
Conrad, P. A., Miller, M. A., Kreuder, C., James, E. R., Mazet, J., Dabritz, H., et al. (2005). Transmission of Toxoplasma: Clues from the study of sea otters as sentinels of Toxoplasma gondii flow into the marine environment. Int. J. Parasitol. 35, 1155–1168. doi: 10.1016/j.ijpara.2005.07.002
Cove, M. V., Fergus, C., Lacher, I., Akre, T., and McShea, W. J. (2019). Projecting mammal distributions in response to future alternative landscapes in a rapidly transitioning region. Remote Sens. 11:e2482. doi: 10.3390/rs11212482
Cove, M. V., Gardner, B., Simons, T. R., Kays, R., and O’Connell, A. F. (2018a). Free-ranging domestic cats (Felis catus) on public lands: Estimating density, activity, and diet in the Florida Keys. Biol. Invasions 20, 333–344. doi: 10.1007/s10530-017-1534-x
Cove, M. V., Gardner, B., Simons, T. R., and O’Connell, A. F. (2018b). Co-occurrence dynamics of endangered lower keys marsh rabbits and free-ranging domestic cats: Prey responses to an exotic predator removal program. Ecol. Evol. 8, 4042–4052. doi: 10.1002/ece3.3954
Cove, M. V., Herrmann, V., Herrera, D. J., Augustine, B. C., Flockhart, D. T. T., and McShea, W. J. (in press). Counting the capital’s cats: Estimating the drivers of occupancy and abundance of free-roaming domestic cats (Felis catus) in an urban mosaic. Ecol. Appl.
Davis, A. A., Lepczyk, C. A., Haman, K. H., Morden, C. W., Crow, S. E., Jensen, N., et al. (2018). Toxoplasma gondii detection in fecal samples from domestic cats (Felis catus) in Hawai‘i. Pac. Sci. 72, 501–511. doi: 10.2984/72.4.9
Davis, A. J., Kirby, J. D., Chipman, R. B., Nelson, K. M., and Gilbert, A. T. (2021). Data-driven management – a dynamic occupancy approach to enhanced rabies surveillance prioritization. Viruses 13:e1795. doi: 10.3390/v13091795
Doherty, T. S., Bengsen, A. J., and Davis, R. A. (2015). A critical review of habitat use by feral cats and key directions for future research and management. Wildl. Res. 41, 435–446. doi: 10.1071/WR14159
Doherty, T. S., Glen, A. S., Nimmo, D. G., Ritchie, E. G., and Dickman, C. R. (2016). Invasive predators and global biodiversity loss. Proc. Natl. Acad. Sci. U.S.A. 113, 11261–11265. doi: 10.1073/pnas.1602480113
Dubey, J. P., Cerqueira-Cézar, C. K., Murata, F. H. A., Kwok, O. C. H., Yang, Y. R., and Su, C. (2020). All about toxoplasmosis in cats: The last decade. Vet. Parasitol. 283:e109145. doi: 10.1016/j.vetpar.2020.109145
Fidino, M. A., Lehrer, E. W., and Magle, S. B. (2016). Habitat dynamics of the Virginia opossum in a highly urban landscape. Am. Midl. Nat. 175, 155–167. doi: 10.1674/003-0031-175.2.155
Flockhart, D. T. T., Lipsey, L., Herrera, D. J., Belsley, J., Decker, S. T., Moore, S. M., et al. (2022). Estimates of domestic cats in urban areas using interdisciplinary science: The Washington DC cat count. Soc. Anim. 2022, 1–18. doi: 10.1163/15685306-BJA10097
Fredebaugh, S. L., Mateus-Pinilla, N. E., McAllister, M., Warner, R. E., and Weng, H. Y. (2011). Prevalence of antibody to Toxoplasma gondii in terrestrial wildlife in a natural area. J. Wildl. Dis. 47, 381–392. doi: 10.7589/0090-3558-47.2.381
Gallo, T., Fidino, M., Gerber, B., Ahlers, A. A., Angstmann, J. L., Amaya, M., et al. (2022). Mammals adjust diel activity across gradients of urbanization. eLife 11:e74756. doi: 10.7554/eLife.74756
Gallo, T., Lehrer, E. W., Fidino, M., Kilgour, R. J., Wolff, P. J., and Magle, S. B. (2018). Need for multiscale planning for conservation of urban bats. Conserv. Biol. 32, 638–647. doi: 10.1111/cobi.13047
Gaynor, K. M., Brown, J. S., Middleton, A. D., Power, M. E., and Brashares, J. S. (2019). Landscapes of fear: Spatial patterns of risk perception and response. Trends Ecol. Evol. 34, 355–368. doi: 10.1016/j.tree.2019.01.004
Gehrt, S. D., Wilson, E. C., Brown, J. L., and Anchor, C. (2013). Population ecology of free-roaming cats and interference competition by coyotes in urban parks. PLoS One 8:e75718. doi: 10.1371/journal.pone.0075718
Gelman, A., Goegebeur, Y., Tuerlinckx, F., and Van Mechelen, I. (2000). Diagnostic checks for discrete data regression models using posterior predictive simulations. Appl. Stat. 49, 247–268.
George, W. G. (1974). Domestic cats as predators and factors in winter shortages of raptor prey. Wilson Bull. 86, 384–396.
Gerhold, R. W., and Jessup, D. A. (2013). Zoonotic diseases associated with free-roaming cats. Zoonoses Public Health 60, 189–195. doi: 10.1111/j.1863-2378.2012.01522.x
Goodwin, S. E., and Shriver, W. G. (2011). Effects of traffic noise on occupancy patterns of forest birds. Conserv. Biol. 25, 406–411. doi: 10.1111/j.1523-1739.2010.01602.x
Harnik, P., McCabe, C., and Hiple, A. (2017). 2017 City park facts. San Francisco, CA: The Trust for Public Land.
Hennessy, C., and Hild, K. (2021). Are Virginia opossums really ecological traps for ticks? Groundtruthing laboratory observations. Ticks Tick Borne Dis. 12:e101780. doi: 10.1016/j.ttbdis.2021.101780
Herrera, D. J., Cove, M. V., McShea, W. J., Flockhart, D. T., Decker, S., Moore, S. M., et al. (2022). Prey selection and predation behavior of free-roaming domestic cats (Felis catus) in an urban ecosystem: Implications for urban can management. Biol. Conserv. 268:e109503. doi: 10.1016/j.biocon.2022.109503
Herrera, D. J., Moore, S. M., Flockhart, D. T. T., McShea, W. J., and Cove, M. V. (2021). Thinking outside the park: Recommendations for camera trapping mammal communities in the urban matrix. J. Urban Ecol. 7:juaa036. doi: 10.1093/jue/juaa036
Hoffman, M. D., and Gelman, A. (2014). The no-u-turn sampler: Adaptively setting path lengths in Hamiltonian Monte Carlo. J. Mach. Learn. Res. 15, 1593–1623.
Holmala, K., and Kauhala, K. (2009). Habitat use of medium-sized carnivores in southeast Finland – key habitats for rabies spread? Ann. Zool. Fenn. 46, 233–246. doi: 10.5735/086.046.0401
Hope, D., Gries, C., Zhu, W., Fagan, W. F., Redman, C. L., Grimm, N. B., et al. (2003). Socioeconomics drive urban plant diversity. Proc. Natl. Acad. Sci. U.S.A. 100, 8788–8792. doi: 10.1073/pnas.1537557100
Jenkins, S. R., Perry, B. D., and Winkler, W. G. (1988). Ecology and epidemiology of raccoon rabies. Rev. Infect. Dis. 10, 620–625.
Johnson, C. K., Hitchens, P. L., Pandit, P. S., Rushmore, J., Smiley Evans, T., Young, C. C. W., et al. (2020). Global shifts in mammalian population trends reveal key predictors of virus spillover risk. Proc. R. Soc. B 287:e20192736. doi: 10.1098/rspb.2019.2736
Jones, K. E., Patel, N. G., Levy, M. A., Storeygard, A., Balk, D., Gittleman, J. L., et al. (2008). Global trends in emerging infectious diseases. Nature 451, 990–993. doi: 10.1038/nature06536
Kays, R., Costello, R., Forrester, T., Baker, M. C., Parsons, A. W., Kalies, E. L., et al. (2015). Cats are rare where coyotes roam. J. Mammal. 96, 981–987. doi: 10.1093/jmammal/gyv100
Kays, R., and DeWan, A. A. (2004). Ecological impact of inside/outside house cats around a suburban nature preserve. Anim. Conserv. 7, 273–283. doi: 10.1017/S1367943004001489
Kays, R., Dunn, R. R., Parsons, A. W., Mcdonald, B., Perkins, T., Powers, S. A., et al. (2020). The small home ranges and large local ecological impacts of pet cats. Anim. Conserv. 23, 516–523. doi: 10.1111/acv.12563
Keesing, F., and Ostfeld, R. S. (2021). Impacts of biodiversity and biodiversity loss on zoonotic diseases. Proc. Natl. Acad. Sci. U.S.A. 118:e2023540118. doi: 10.1073/pnas.2023540118
Kellner, K. F., Fowler, N. L., Petroelje, T. R., Kautz, T. M., Beyer, D. E., and Belant, J. L. (2021). ubms: An R package for fitting hierarchical occupancy and N-mixture abundance models in a Bayesian framework. Methods Ecol. Evol. 13, 577–584. doi: 10.1111/2041-210X.13777
Kreisler, R. E., Cornell, H. N., and Levy, J. K. (2019). Decrease in population and increase in welfare of community cats in a twenty-three year trap-neuter-return program in Key Largo, FL: The ORCAT program. Front. Vet. Sci. 6:7. doi: 10.3389/fvets.2019.00007
Larson, R. N., Brown, J. L., Karels, T., and Riley, S. P. D. (2020). Effects of urbanization on resource use and individual specialization in coyotes (Canis latrans) in southern California. PLoS One 15:e0228881. doi: 10.1371/journal.pone.0228881
Lashley, M. A., Cove, M. V., Chitwood, M. C., Penido, G., Gardner, B., DePerno, C. S., et al. (2018). Estimating wildlife activity curves: Comparison of methods and sample size. Sci. Rep. 8, e4173. doi: 10.1038/s41598-018-22638-6
Lombardi, J. V., Comer, C. E., Scognamillo, D. G., and Conway, W. C. (2017). Coyote, fox, and bobcat response to anthropogenic and natural landscape features in a small urban area. Urban Ecosyst. 20, 1239–1248. doi: 10.1007/s11252-017-0676-z
Longcore, T., Rich, C., and Sullivan, L. M. (2009). Critical assessment of claims regarding management of feral cats by trap-neuter-return. Conserv. Biol. 23, 887–894. doi: 10.1111/j.1523-1739.2009.01174.x
Loss, S. R., and Marra, P. P. (2017). Population impacts of free-ranging domestic cats on mainland vertebrates. Front. Ecol. Environ. 15:502–509. doi: 10.1002/fee.1633
Loss, S. R., Will, T., and Marra, P. P. (2013). The impact of free-ranging domestic cats on wildlife of the United States. Nat. Commun. 4:e1396. doi: 10.1038/ncomms2380
Loyd, K. A. T., Hernandez, S. M., Carroll, J. P., Abernathy, K. J., and Marshall, G. J. (2013). Quantifying free-roaming domestic cat predation using animal-borne video cameras. Biol. Conserv. 160, 183–189. doi: 10.1016/j.biocon.2013.01/008
Luck, G. W. (2007). A review of the relationship between human population density and biodiversity. Biol. Rev. 82, 607–645. doi: 10.1111/j.1469-185X.2007.00028.x
Ma, X., Monroe, B. P., Wallace, R. M., Orciari, L. A., Gigante, C. M., Kirby, J. D., et al. (2021). Rabies surveillance in the United States during 2019. J. Am. Vet. Med. Assoc. 258, 1205–1220. doi: 10.2460/javma.258.11.1205
MacKenzie, D. I., and Bailey, L. L. (2004). Assessing the fit of site-occupancy models. J. Agric. Biol. Environ. Stat. 9, 300–318. doi: 10.1198/108571104x3361
MacKenzie, D. I., James, D. N., Royle, J. A., Pollock, K. H., Bailey, L. L., and Hines, J. E. (2017). Occupancy estimation and modeling, 2nd Edn. Cambridge, MA: Academic Press.
MacKenzie, D. I., Nichols, J. D., Lachman, G. B., Droege, S., Royale, A., and Langtimm, C. A. (2002). Estimating site occupancy rates when detection probabilities are less than one. Ecology 83, 2248–2255.
Magle, S. B., Fidino, M., Sander, H. A., Rohnke, A. T., Larson, K. L., Gallo, T., et al. (2021). Wealth and urbanization shape medium and large terrestrial mammal communities. Glob. Change Biol. 27, 5446–5459. doi: 10.1111/gcb.15800
Mayor, S. J., Schneider, D. C., Schaefer, J. A., and Mahoney, S. P. (2009). Habitat selection at multiple scales. Ecoscience 16, 238–247. doi: 10.2980/16-2-3238
McShea, W. J., Forrester, T., Costello, R., He, Z., and Kays, R. (2016). Volunteer-run cameras as distributed sensors for macrosystem mammal research. Landsc. Ecol. 31, 55–66. doi: 10.1007/s10980-015-0262-9
Moll, R. J., Cepek, J. D., Lorch, P. D., Dennis, P. M., Robison, T., and Montgomery, R. A. (2020). At what spatial scale(s) do mammals respond to urbanization? Ecography 43, 171–183. doi: 10.1111/ecog.04762
Naimi, B., Hamm, N. A. S., Groen, T. A., Skidmore, A. K., and Toxopeus, A. G. (2014). Where is positional uncertainty a problem for species distribution modelling? Ecography 37, 191–203. doi: 10.1111/j.1600-0587.2013.00205.x
National Aeronautics and Space Administration [NASA] (2021). SKYCAL – Sky events calendar [Internet]. Available online at: https://eclipse.gsfc.nasa.gov/SKYCAL/SKYCAL.html?cal=2020#skycal (accessed January 11, 2021).
National Oceanic and Atmospheric Administration [NOAA] (2021). Climate data online, daily summaries: Washington 5.1 NW, DC US; Washington 3.1 NNE, DC US; Washington 2.0 SSW, DC US. Available online at: https://www.ncdc.noaa.gov/cdo-web/datasets (accessed January 11, 2021).
Niedballa, J., Sollmann, R., Courtiol, A., and Wilting, A. (2016). camtrapR: An R package for efficient camera trap data management. Methods Ecol. Evol. 7, 1457–1462. doi: 10.1111/2041-210X.12600
Northrup, J. M., and Gerber, B. D. (2018). A comment on priors for Bayesian occupancy models. PLoS One 13:e0192819. doi: 10.1371/journal.pone.0192819
Oliveira-Santos, L. G. R., Zucco, C. A., and Agostinelli, C. (2013). Using conditional circular kernel density functions to test hypotheses on animal circadian activity. Anim. Behav. 85, 269–280. doi: 10.1016/j.anbehav.2012.09.033
Ossi, D., Rauch, D., Rohrbaugh, L., and Spencer, S. (2015). District of Columbia wildlife action plan: A conservation strategy for Washington, D.C., 2015 update. Washington, DC: Government of the District of Columbia, Department of Energy and Environment.
Paul, G. C., and Friend, D. G. (2020). Body weight as an indicator of vulnerability to domestic cat predation for juveniles of three species of cottontail rabbits (Sylvilagus spp.) in Colorado, USA: Implications for release criteria. J. Wildl. Dis. 56, 965–967. doi: 10.7589/JWD-D-20-00036
Pieracci, E. G., Pearson, C. M., Wallace, R. M., Blanton, J. D., Whitehouse, E. R., Ma, X., et al. (2019). Vital signs: Trends in human rabies deaths and exposures – United States, 1938-2018. Morb. Mortal. Wkly. Rep. 68, 424–528. doi: 10.15585/mmwr.mm6823e1
Pirie, T. J., Thomas, R. L., and Fellowes, M. D. E. (2022). Pet cats (Felis catus) from urban boundaries use different habitats, have larger home ranges and kill more prey than cats from the suburbs. Landsc. Urban Plan. 220:e104338. doi: 10.1016/j.landurbplan.2021.104338
R Core Team (2020). R: A language and environment for statistical computing. Vienna: R Foundation for Statistical Computing.
Rettie, W. J., and Messier, F. (2000). Hierarchical habitat selection by woodland caribou: Its relationship to limiting factors. Ecography 23, 466–478.
Ridout, M. S., and Linkie, M. (2009). Estimating overlap of daily activity patterns from camera trap data. J. Agric. Biol. Environ. Stat. 14, 322–337. doi: 10.1198/jabes.2009.08038
Roebling, A. D., Johnson, D., Blanton, J. D., Levin, M., Slate, D., Fenwick, G., et al. (2014). Rabies prevention and management of cats in the context of trap-neuter-vaccinate-release programmes. Zoonoses Public Health 61, 290–296. doi: 10.1111/zph.12070
Roseveare, C. W., Goolsby, W. D., and Foppa, I. M. (2009). Potential and actual terrestrial rabies exposures in people and domestic animals, upstate South Carolina, 1994-2004: A surveillance study. BMC Public Health 9:e65. doi: 10.1186/1471-2458-9-65
Rowcliffe, J. M., Kays, R., Kranstauber, B., Carbone, C., and Jansen, P. A. (2014). Quantifying levels of animal activity using camera trap data. Methods Ecol. Evol. 5, 1170–1179. doi: 10.1111/2041/210X.12278
Seymour, C. L., Simmons, R. E., Morling, F., George, S. T., Peters, K., and O’Riain, M. J. (2020). Caught on camera: The impacts of urban domestic cats on wild prey in an African city and neighboring protected areas. Glob. Ecol. Conserv. 23:e01198. doi: 10.1016/j.gecco.2020.e01198
Sogliani, D., and Mori, E. (2019). “The fox and the cat”: Sometimes they do not agree. Mammal. Biol. 95, 150–154. doi: 10.1016/j.mambio.2018.07.003
Stan Development Team (2020). Stan modeling language users guide and reference manual, 2.30. Available online at: https://mc-stan.org (accessed November 1, 2021).
Stevens, K. B., and Pfeiffer, D. U. (2016). “Chapter 25–The role of spatial analysis in risk-based animal disease management,” in Handbook of spatial epidemiology, eds A. B. Lawson, S. Banerjee, R. P. Haining, and M. D. Ugarte (Boca Raton, FL: Chapman & Hall/CRC Press), 449–475.
Tan, S. M. L., Stellato, A. C., and Niel, L. (2020). Uncontrolled outdoor access for cats: An assessment of risks and benefits. Animals 10:e258. doi: 10.3390/ani10020258
Tayac, G. (2009). “Chp. 4 – Allies of the land,” in The land has memory: Indigenous knowledge, native landscapes, and the National Museum of the American Indian, eds D. B. Spruce and T. Thrasher (Chapel Hill, NC: University of North Carolina Press), 49–59.
Theimer, T. C., Clayton, A. C., Martinez, A., Peterson, D. L., and Bergman, D. L. (2015). Visitation rate and behavior of urban mesocarnivores differs in the presence of two common anthropogenic food sources. Urban Ecosyst. 18, 895–906. doi: 10.1007/s11252-015-0436-x
Thomas, R. L., Fellowes, M. D. E., and Baker, P. J. (2012). Spatio-temporal variation in predation by urban domestic cats (Felis catus) and the acceptability of possible management actions in the UK. PLoS One 7:e49369. doi: 10.1371/journal.pone.0049369
Vanek, J. P., Rutter, T. S., Preuss, T. S., Jones, H. P., and Glowacki, G. A. (2020). Anthropogenic factors influence the occupancy of an invasive carnivore in a suburban preserve system. Urban Ecosyst. 24, 113–126. doi: 10.1007/s11252-020-01026-x
Vehtari, A., Gelman, A., and Gabry, J. (2017). Practical Bayesian model evaluation using leave-one-out cross-validation and WAIC. Stat. Comput. 27, 1413–1432. doi: 10.1007/s11222-016-9696-4
Wait, K. R., and Ahlers, A. A. (2020). Virginia opossum distributions are influenced by human-modified landscapes and water availability in tallgrass prairies. J. Mammal. 101, 216–225. doi: 10.1093/jmammal/gyz176
Wright, W. J., Irvine, K. M., and Higgs, M. D. (2019). Identifying occupancy model inadequacies: Can residuals separately assess detection and presence? Ecology 100:e02703. doi: 10.1002/ecy.2703
Wright, W. J., Irvine, K. M., and Rodhouse, T. J. (2016). A goodness-of-fit test for occupancy models with correlated within-season revisits. Ecol. Evol. 6, 5404–5415. doi: 10.1002/ece3.2292
Keywords: occupancy, activity, predation, zoonotic disease, feral cats, management, one health, public health
Citation: Herrera DJ, Cove MV, McShea WJ, Decker S, Flockhart DTT, Moore SM and Gallo T (2022) Spatial and temporal overlap of domestic cats (Felis catus) and native urban wildlife. Front. Ecol. Evol. 10:1048585. doi: 10.3389/fevo.2022.1048585
Received: 19 September 2022; Accepted: 28 October 2022;
Published: 21 November 2022.
Edited by:
Mathew Samuel Crowther, The University of Sydney, AustraliaReviewed by:
Ben T. Hirsch, James Cook University, AustraliaCopyright © 2022 Herrera, Cove, McShea, Decker, Flockhart, Moore and Gallo. This is an open-access article distributed under the terms of the Creative Commons Attribution License (CC BY). The use, distribution or reproduction in other forums is permitted, provided the original author(s) and the copyright owner(s) are credited and that the original publication in this journal is cited, in accordance with accepted academic practice. No use, distribution or reproduction is permitted which does not comply with these terms.
*Correspondence: Daniel J. Herrera, ZGhlcnJlcjNAdW1kLmVkdQ==
Disclaimer: All claims expressed in this article are solely those of the authors and do not necessarily represent those of their affiliated organizations, or those of the publisher, the editors and the reviewers. Any product that may be evaluated in this article or claim that may be made by its manufacturer is not guaranteed or endorsed by the publisher.
Research integrity at Frontiers
Learn more about the work of our research integrity team to safeguard the quality of each article we publish.