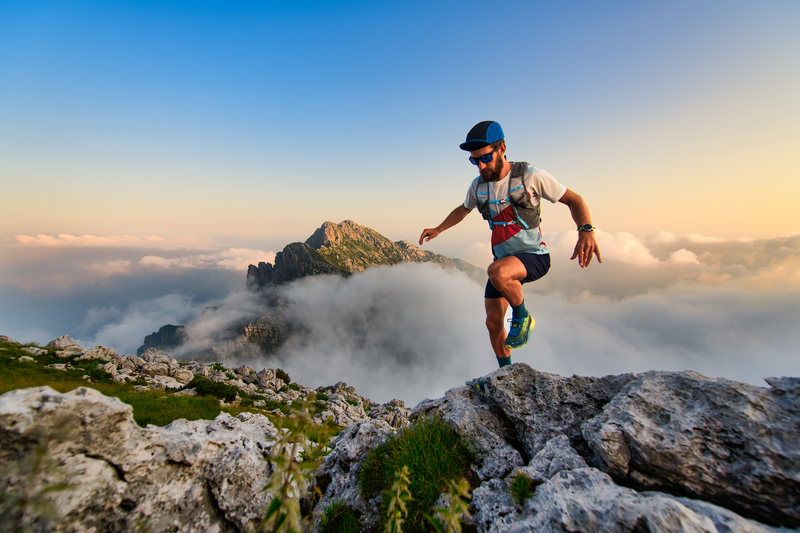
95% of researchers rate our articles as excellent or good
Learn more about the work of our research integrity team to safeguard the quality of each article we publish.
Find out more
ORIGINAL RESEARCH article
Front. Ecol. Evol. , 15 December 2022
Sec. Phylogenetics, Phylogenomics, and Systematics
Volume 10 - 2022 | https://doi.org/10.3389/fevo.2022.1008477
This article is part of the Research Topic Neotropical Dung Beetle Diversity: Ecological, Historical, and Anthropogenic Perspectives View all 11 articles
Introduction: Ecuador harbors an astounding number of ecosystems and species. However, anthropogenic land-use changes are the primary drivers of biodiversity loss in major taxonomic groups, especially insects. Among them, the Scarabaeinae subfamily containing dung beetles, is an excellent taxon for studying taxonomic and functional diversity, as they are relatively stable taxonomically and have a wide variety of ecological services. Their distribution is mainly influenced by biogeography and climate as their main ecological and environmental factors will allow us to quantify what aspects of diversity are being impacted under different circumstances and at different scales.
Methods: To understand the main of dung beetle distribution drivers, we analyzed a museum database from the National Institute of Biodiversity, Ecuador (INABIO) of over 5000 dung beetle specimens with 122 species collected throughout the country, we addressed the following questions: i) How does tribe distribution vary across climatic and elevational gradients? and ii) How does functional and taxonomic beta diversity vary across spatial scales? To address them, we focused on three main tribes: Canthonini, Coprini, and Phanaeini. We constructed GLM’s and niche-based models to estimate Ecuador’s distributions based on climate variables to explore potential predictor variables, using tree classification models, along with taxonomic and functional beta diversity across scales.
Results: The main variables influencing dung beetle distribution were elevation, and precipitation. The Phanaeini niche model is significantly better at predicting dung beetle presence throughout Ecuador than Canthonini and Coprini. We found high turnover in functional groups at larger scales, suggesting that dung beetles show high levels of habitat specialization, which associates to our findings where taxonomic beta diversity was higher in the Amazon basin compared to the coastal region. This may be due to the higher rate of dung production in Amazonia. Our findings also suggest that dung beetles are not found in areas above 2000m, mainly because dung beetles are well adapted to warmer and moist climatic regions. Precipitation and elevation are consistently essential variables for predicting Canthonini and Coprini presence, while temperature explains Phanaeini presence. Low levels of species turnover at the regional scale may be because the total species richness in Ecuador is different, where divergence in taxonomic beta diversity between the two regions is an artifact of such differences in richness in Amazonia versus the coast, the distinction is also due to nonrandomly low taxonomic beta diversity levels in the coastal region.
Conclusions: Our results provide an essential framework for evaluating potential dung beetle habitat and diversity at different scales; therefore, by identifying dung beetles’ diversity, combined with considerations of habitat fragmentation, human land-use alteration, and climate change, will be an important next step to inform better and prioritize dung beetle conservation efforts in other countries.
Ecuador is the smallest of the 17 megadiverse countries (Mittermeier et al., 1998) harboring an astounding number of ecosystems and species, with many endemic species occurring in small geographic ranges (Brooks et al., 2002, 2006; Bass et al., 2010). Unfortunately, the country has experienced profound changes to its natural habitats (Cuesta et al., 2017). In 2014, the country reported an estimated 1.83 million-hectare (14%) loss of forest area since 1990 (Sierra et al., 2021). The annual deforestation rate for 2008–2014 was – 0.37%, equivalent to an average annual loss of 47,000 hectares (Sierra et al., 2021). Due to these recent trends in habitat loss and extensive historical changes that occurred during the mid-20th century, only ~30% of the original natural vegetation remains in the coastal plains, 60% in the Andean region, and 88% in the Amazon lowlands. In this context, anthropogenic land-use changes are the primary drivers of global biodiversity loss in major taxonomic groups (Reid et al., 2005), and Ecuador is not the exception. Invertebrates are often particularly susceptible to landscape changes (Dunn, 2004; Samways, 2005), and because of the key roles they play in many ecosystem processes, their loss could produce cascading effects on the entire communities (Coleman and Hendrix, 2000). Despite this, our knowledge about the response of many insects to climate conditions and human activity is minimal (Nichols et al., 2008; Wagner, 2020). Understanding the response of insects to human activity and climate is necessary to support conservation policies and assess the functional consequences of human disturbance (Balmford and Bond, 2005; Halsch et al., 2021).
The order Coleoptera constitutes the richest group of species of any animal taxa, with more than 400,000 species described so far (McKenna et al., 2015; Zhang et al., 2018). Among this diverse order, dung beetles belong to the Scarabaeoidea superfamily, one of the largest superfamilies of beetles in the world, with approximately 150 families and 30,000 species (Carvajal et al., 2011). Scarabaeoidea is the most diverse group within the Coleoptera order in terms of its biology, ecology, and behavior (Woodruff, 1973). Dung beetles are an excellent taxon for studying both taxonomic and functional diversity. They are widely distributed, diverse, and abundant in tropical and warm temperate ecosystems. Additionally, the ecological roles of dung beetles are known (Hanski and Cambefort, 1991) and the group has a relatively stable taxonomy (Philips et al., 2004). Adults and larvae are part of a specialized group that feeds mainly on the feces of herbivorous mammals, thus playing an integral role in recycling nutrients, improving plant growth, reducing pests, bioturbation, pollination, and secondary seed dispersal (Nichols et al., 2008). In addition, this taxon has been proposed as an excellent bioindicator group of environmental processes, being very sensitive to anthropic disturbance events (Halffter and Favila, 1993, McGeoch et al., 2002, Spector, 2006, Otavo et al., 2013, Noriega et al., 2021).
Two principal ecological factors influence present tribal, genera, and species distribution patterns of Scarabaeinae dung beetles worldwide (Davis and Scholtz, 2001): suitable climate and the number of dung types. At the species and generic level, there is a strong correlation between dung beetle taxon richness and the area of suitable climate in each of the world’s biogeographical regions. However, at the tribal level, taxon richness and composition strongly correlate to both climatic area and the number of dung types. Dung type diversity also varies between biogeographical regions according to the evolutionary history of mammals (Davis et al., 2002). However, their relative influence differs according to the two main components of biodiversity: overall numerical richness and taxonomic identity. In the first instance, current distributional variance in taxon richness is often correlated strongly with current ecological or environmental factors (Francis and Currie, 1998; Davis and Scholtz, 2001; Moura et al., 2016). Scarabaeinae dung beetles also show strong spatial specializations stemming from their Gondwana land origin (Halffter and Matthews, 1966; Halffter, 1974; Silva and Hernández, 2014) centered on warmer, moister, climatic regions (Halffter, 1991; Davis et al., 2002; Birkett et al., 2018). At a regional scale, climate type exerts a significant influence on dung beetle diversity (Kirk and Ridsdill-Smith, 1986; Davis and Dewhurst, 1993; Davis, 1997; Duncan et al., 2009), which appears to be a general rule in the large-scale distribution of any taxonomic group (Currie et al., 1999; Clark et al., 2011). At a local scale, soil and vegetation types, influence dung beetle distribution (Nealis, 1977; Doube, 1983; Cambefort, 1991; Davis, 1996; Daniel et al., 2021); which, could strongly affect ecosystem functioning and disrupt all ecosystem services provided by this group of insects. Potential distribution and/or niche models for this group of species in Ecuador are scarce.
To quantify the effects of this habitat alteration and climate change, we need to understand which roles, functions, and ecological services exist on the landscape. Understanding where, when, and how species change in relation with the environment will allow us to quantify what aspects of diversity are being impacted under different circumstances and at different scales of land-use change (Barragán et al., 2011). These patterns allow for more effective management of natural areas to avoid biodiversity loss in critical regions (Hayes et al., 2009). In this context, beta diversity may be a handy metric for elucidating the processes underlying dung beetle community assembly at a regional scale. Beta diversity, which measures how species composition changes across space, can allow us to evaluate how community structure and assembly processes change as a function of the environment (McKnight et al., 2007; Buckley and Jetz, 2008; Lepori and Malmqvist, 2009). At local scales, high beta diversity measurements can reflect a decisive role of environmental filtering and species interactions in community organization (Fukami et al., 2005). At regional scales, high beta diversity can reflect a strong role in trait evolution, habitat specialization, and speciation (Graham and Fine, 2008). Taxonomic diversity, however, is often sensitive to stochastic effects such as genetic and ecological drift due to dispersal limitations (Baiser and Lockwood, 2011). And, furthermore, the presence of functionally redundant species limits the ability of taxonomic diversity to reflect environmental filtering (Swenson et al., 2011; Siefert et al., 2012). To tease apart these processes, one solution is to compare taxonomic and functional beta diversity. In comparison to taxonomic diversity, functional trait diversity is highly responsive to deterministic environmental processes but not to the stochasticity caused by ecological drift and dispersal limitation (Villéger et al., 2012; Myers et al., 2013). When functional beta diversity and taxonomic beta diversity respond similarly to spatial or temporal changes, communities are likely to assemble deterministically, while opposed responses suggest that stochastic effects may be causing mismatches in functional and taxonomic levels (Condit et al., 2002; Chase and Myers, 2011; Leibold and Chase, 2017). The dung beetle assemblages of Ecuador span a large and heterogeneous area, making it is critical to also consider regional habitat type, which can affect beta diversity (Swenson et al., 2011). By measuring the correlation between taxonomic and functional beta diversity, we hope to understand whether dung beetle assemblages in Ecuador are organized via habitat filtration and species interactions, which act at the functional level, or dispersal limitation and ecological drift, which operate at the species level.
To understand distribution and biodiversity patterns among Ecuador’s dung beetles, we conducted two analyses utilizing a database of over 5,000 dung beetle specimens collected throughout the country, addressing the following questions: (i) How does tribe distribution vary across climatic and elevational gradients? And (ii) How does taxonomic and functional beta diversity vary across spatial scales? We first examined the distribution of three widespread tribes that play distinct roles in ecosystem functioning (Canthonini, Coprini, and Phanaeini) using distribution models to evaluate which environmental and topographic variables are significant drivers of tribe presence. We hypothesized that elevation and temperature would be the most important drivers of these tribes’ distributions because of the dung beetle’s small body size and dependence on mammal populations, which likely vary according to vegetation assemblages driven by elevation and moisture. Second, to assess the relative importance of stochastic versus ecologically driven community assemblage processes throughout Ecuador, we compared the relationship between alpha and beta diversity at the taxonomic level (taxonomic alpha/beta diversity) and functional group level (functional alpha/beta diversity) using 20 different spatial scales. Spatial scale was represented here as 20 spatial grids which all covered the same extent of Ecuador, but which differed in grain size, ranging from a 4-bit grid to a 256-bit grid. Based on their sensitivity to climate and specialization to dung type, we hypothesized that dung beetle community assemblage processes are likely driven by ecological factors such as niche specialization and biotic interactions both at the local and regional levels. We thus expected functional beta diversity and taxonomic beta diversity to respond similarly to spatial scale and alpha richness. Finally, to assess the importance of habitat type on stochastic versus ecologically driven community assembly processes, we compared the response of taxonomic and functional beta diversities to scale in the northern coastal region to the northern Amazonian region of Ecuador. We hypothesized that highly productive and more environmentally stable regions, such as the Amazon basin, would show high beta diversity at a much finer scales than heterogeneous regions such as the northern Coast.
Data were analyzed from the Coleoptera Collection of the National Institute of Biodiversity, Ecuador. This database includes adult dung beetle collections with records from the 1970s, with more than 5,000 entries, encompassing 122 species from three natural regions (Coast, Andes, and Amazonia), 19 provinces, and 11 vegetative formations. All the collections belong to more than 200 locations across Ecuador. All analyses focused on the three main tribes: Canthonini (42 species), Coprini (42 species), and Phanaeini (17 species), as they belong to groups with broad distributions and adapted to high percentage of canopy cover. Ecuador is divided into four unique geographical regions; the Coast, which has seven provinces ranging from 0 – 900 m and limiting with the western Andes cordillera; the Sierra region (Andes) has 10 provinces all in high elevations ranging from 900 to 6,263 m, the Amazon has six provinces all located in the Amazon Basin from the Eastern Andes foothills at 800 m towards the evergreen forests at 200 m.
The Coleoptera Collection was trimmed to include only one entry per species per location to account for sampling bias. We analyzed the data in two ways. First, to understand how different tribes are distributed across the landscape, we fitted distribution models using several climate variables and elevation data (Supplementary Table S1). Second, we analyzed how grain size affects β-diversity using taxonomic and functional approaches, where the largest grain size divides the region (Amazonia or Coast) into fourths, each plot about 22,000 km2, and the smallest grain size divides the region into 256 plots each equal to 344 km2. All data analysis was conducted in R (R Core Team, 2022).
We constructed a niche-based model to infer climatic processes driving the species spatial distribution. To characterize the niche climatic space, we used 9 bioclimatic variables (Supplementary Table S1) obtained from the WorldClim database (Fick and Hijmans, 2017), including global monthly climate data from 1970 to 2000 at approximately 1 km resolution. We selected climate variables related to temperature and precipitation. These raster data and elevational data were imported in the statistical software R (R Core Team, 2022) as a raster stack for spatial analysis and the models were generated using the “sf” (Pebesma, 2018), “dismo” (Hijmans et al., 2022) and “raster” (Hijmans, 2022) packages. Since elevation data were available as spatial polylines, the data were converted to a raster using nearest-neighbor interpolation with the same extent and resolution as the climate data for ease of analysis. Global climate data were cropped to Ecuador, then extracted to the location of each dung beetle specimen. Although vegetation data were also available, we did not use these data in distribution modeling because of the large number of different vegetation classes for the number of observations available, then we performed distribution models separately for each tribe.
Because our data were presence-only, we generated pseudo-absences by randomly sampling background locations across Ecuador to produce approximately equal sample sizes of presence/absence data, as recommended by Barbet-Massin et al. (2012). Climate and elevation data were again extracted to these background locations.
In order to select the most appropriate climate variables, we used a classification and regression tree model (CART) (Lewis, 2000, de Ona and de Ona, 2012) to identify potential predictor variables for determining tribe presence/absence. Classification trees partition the response recursively into hierarchical subsets that are increasingly homogeneous in presences/absences (Urban et al., 2002). The model uses single predictor variables for partitioning at each branch of the tree. Classification trees were ‘pruned’ using a cost-complexity parameter that minimized the relative misclassification rate from 10-fold cross-validation to avoid overfitting. To evaluate each reduced classification tree, we fit a model on a training subset (70% of the data), then predicted tribe presence/absence on the holdout dataset. Variables identified through each pruned classification tree were then used to model tribe distribution using generalized linear models using the package “rpart” (Therneau et al., 2015) in R (R Core Team, 2022). The final models specify the logit (ln(P/(1-P)) of presence probability, P, as a linear combination of the specified predictor variables and interactions between predictors.
We selected elevation, mean annual temperature, annual precipitation, precipitation of the wettest and driest months, precipitation seasonality, and precipitation of the warmest and coldest quarters for inclusion in our models based on a threshold Pearson’s correlation of 0.7 (Sedgwick, 2012). Classification tree models predicting tribe presence/absence allowed us to reduce the number of potential predictor variables for further consideration in logistic regression.
We began our analysis by exploring potential predictor variables for explaining the distribution of dung beetle tribes across Ecuador. We first plotted univariate histograms of the possible predictor variables (Supplementary Figure S1), the response variable, and tribe presence to understand the underlying distribution of each variable. We then explored a correlation and bivariate scatterplot matrix of all variables to understand the full scope of correlations between the potential predictors. After considering redundancy in several variables and visualizing possible important predictor variables graphically and through the CART model, we decided to explore elevation and all bioclimatic variables, as potential predictor variables in generalized linear models. Because the response variable, tribe presence/absence, is discrete binary count data bounded at zero, we specified a full generalized linear model (GLM) with a binomial distribution and logit-link function for each tribe. We then identified several reduced models predicting tribe presence/absence with predictor variables and determined the statistically significance with an ANOVA – chi-squared test in the entire model. The final models for each tribe were selected by comparing Akaike Information Criterions (AIC) and Bayesian Information Criterions (BIC) (Table 1), as well as their evaluation performance. Using the last models for each tribe (Table 2), we predicted the probability of occurrence across Ecuador based on the climate and elevation raster inputs.
Table 1. AIC and BIC data for model selection for each of the three tribe distribution models using binomial generalized linear regressions.
Table 2. Coefficients and significance statistics of final tribe distribution models using logistic generalized linear regression.
To assess whether a binomial GLM with a logit link function was appropriate for characterizing the distribution of different dung beetle tribes, we produced diagnostic plots for the model and performed ANOVA, chi-square analyses to compare its ability to explain variance in the data with the full binomial model. We assessed linearity by plotting the residuals versus the model’s fitted values and examining the normal quantile-quantile (Q-Q) plots. The residual versus fitted values plot was used to detect non-linearity, unequal error variances, and outliers. We used Cook’s distance and plotted hat values in an index plot to determine if any outliers were exerting undue influence on the model. We examined response, Pearson, and deviance residual plots for each final model to assess for normality. To determine the accuracy of our distribution models, we used a threshold-dependent metric. We took an initial random sample of 70% training data to fit the top distribution models. We then predicted dung beetle tribe presence/absence using the validation set on the training model. We performed this holdout model selection and validation five times to examine consistency in the top model’s covariates, parameter estimates, and accuracy, afterwards, we specified the threshold for each validation set around the prevalence of a tribe in the data and converted our predicted probabilities to presence/absence predictions, and produced a contingency table to gauge overall accuracy, false positives, and negatives. We also assessed the error rate in classification using the same threshold. The class agreement command produced the overall accuracy metric and the kappa coefficient, which measures the agreement between predicted and observed data beyond chance agreement between classes. Furthermore, we used receiver operating curves (ROC) based on the overall accuracy to calculate an area-under-curve statistic (AUC) given our specified threshold.
For our taxonomic approach, within the three tribes, we used the collection data of 100 species spread among 682 observation points throughout Ecuador. Afterwards, we identified 14 functional groups (Supplementary Table S2) conceptualized as non-phylogenetic, aggregated units of species sharing an important ecological characteristic and playing an equivalent role in the community (Orfanidis et al., 2011), based on their feeding strategy (coprophagous, necrophagous, generalist), nesting behavior (paracoprid – tunnellers and telecoprid – ball-rollers), and size (big, medium, and small). Using the sf package (Pebesma, 2018), we created 20 different spatial grids, dividing the area of the total spatial extent by (x2), where x equals two through 21 (Figure 1). The largest grid divided the total area of Ecuador into fourths, each 22,000 km2, while the smallest grid had 256 cells of area 343.9 km2. Alpha diversity was calculated as the number of species (taxonomic richness) and the number of functional groups (functional richness) present on each cell; while Beta diversity was calculated for each cell as the total number of species or functional groups present within the entire grid, divided by the species/functional group richness within a given cell, minus one (beta = gamma/richness – 1), following Whittaker (1960) approximation.
To compare the coastal and Amazonian regions, we subsetted the data from these two regions and repeated the above methodology for each using 15 different spatial grids with cell sizes from 5,264 km2 down to 82.3 km2. We then compared the coefficients from each linear model. To evaluate whether differences in beta diversity between the two regions was an artifact of differences in species richness between the two regions, we used a null model to compare the observed beta diversity to the expected beta diversity, given richness (Crist et al., 2003; Myers et al., 2013). The null model randomly sampled individuals from the regional species pool to fill each cell within the grid, while keeping the number of individuals sampled per cell equal to that observed and the number of records per species in the regional pool equal to that observed. We then compared the observed beta diversity values to the mean of 1000 iterations of the null model outputs and calculated p-values for each grid as the ratio of iterations with values less than the observed beta diversity value.
Figure 1. Dung beetle occurrence records (red) throughout Ecuador, fitted with a 64-bit grid to calculate taxonomic and functional beta diversity. Base map colors represent vegetation regimes.
The probability of presence for each tribe is a function of the predictors specified in Tables 2 and 3, being elevation the most significant predictor variable in all three models. Canthonini presence varied as a function of elevation, annual precipitation, and precipitation of the coldest quarter and based on the tree model, all the precipitation variables worked as important predictor variables, with a 21% error rate predicting on a holdout dataset; its probability decreased as elevation increased, with a much more pronounced negative trend as annual rainfall increased, indicating the importance of the timing of precipitation for this tribe (Figure S5). Coprini presence increased slightly as elevation increased, precipitation of the driest month, and precipitation seasonality, and its classification tree suggested precipitation and seasonality as potentially important variables, with a 24% error rate predicting on a holdout dataset (Figure S6). Finally, Phanaeini presence varied as a function of elevation and mean annual temperature. The probability of Phanaeini presence increased with mean annual temperature but decreased as elevation increased with the classification tree suggesting elevation, mean annual temperature, and precipitation of the wettest month as potentially important variables, with a 5% error rate predicting on a holdout dataset (Figure S7).
The distribution model for the Phanaeini tribe performed significantly better than the final distribution models for Canthonini and Coprini (Table 3). The average error rate from five trials of validating the last models on a holdout dataset was 31.02% for Canthonini, 30.88% for Coprini, and 9.28% for Phanaeini. These error rates are based on average threshold values for each tribe of 0.40 for Canthonini, 0.40 for Coprini, and 0.31 for Phanaeini. The threshold values used were optimized to maximize accuracy in each trial while minimizing false positives and negatives. To evaluate each model with a threshold-independent metric, we used the area under the receiver operating curve (AUC), representing the tradeoff between true positives and false positives. The average AUC for five holdout validation trials were 0.73, 0.76, and 0.97, respectively. Therefore, the Phanaeini distribution model is significantly better at predicting dung beetle presence throughout Ecuador compared to the final Canthonini and Coprini models (Figure 2).
Figure 2. Predicted tribe distribution maps from logistic generalized linear models for Canthonini (A), Coprini (B), and Phanaeini (C) in Ecuador. Colors represent presence probability. Plus, signs represent presence data points.
Over the scope of Ecuador, taxonomic beta diversity (species turnover) had an inverse relationship to taxonomic alpha diversity (species richness) (Figure 3A), such that at smaller spatial scales, the rate of species turnover among plots was much higher than the mean species richness of any individual plot. Functional beta diversity (functional turnover) also increased as plot size decreased (Figure 3B), however, unlike with taxonomic turnover, functional turnover was only marginally greater than functional richness at small spatial scales. In other words, even at small spatial scales, there was low variation of functional groups among plots.
Figure 3. Taxonomic (A) and functional (B) alpha (black circles) and beta (blue triangles) diversity of dung beetles as a function of grain size. Plot area is the size of each grain within a grid, transformed with log base ten for visual examination. 20 grids of decreasing grain size were used, ranging from a 4-bit grid with 22,000 sq km cells, to a 256-bit grid with 344 sq km cells.
In this dataset, 72 species of dung beetle from 287 observations were recorded in Amazonia, and 38 species from 291 observations were recorded in the coastal region. Out of the 14 identified functional groups, all 14 were represented in Amazonia and 12 were represented in the coastal region. Taxonomic beta diversity was higher in Amazonia compared to the coast (Figure 4A) while functional beta diversity did not differ between the two regions (Figure 4B). Taxonomic beta diversity levels in Amazonia were in line with what null model analysis predicted based on the region’s species richness: the higher levels of taxonomic turnover in Amazonia compared to the coast can be largely attributed to higher species richness in Amazonia (Figure S8A). However, taxonomic beta diversity levels from the coastal region were significantly lower than expected based on the null model in 9 out of the 15 grids and marginally lower in 11 out of 15 grids (Figure S8B). Thus, while some of the divergence in taxonomic beta diversity between the two regions is an artifact of differences in species richness in Amazonia versus the coast, the distinction is also due to nonrandomly low taxonomic beta diversity levels in the coastal region. Functional beta diversity did not significantly differ from the null expectation in either region.
Figure 4. Taxonomic (A) and functional (B) beta diversity as a function of log grain size. At smaller spatial scales, dung beetle beta diversity in Northern Ecuador is higher in the Amazon (closed circles) than in the coastal region (open circles). Functional beta diversity shows equal dependence on grain size in both regions.
Precipitation and elevation were consistently significant for predicting presence of Canthonini and Coprini, while the temperature was more important for predicting presence of Phanaeini in Ecuador. These climatic distribution patterns of dung beetle taxa reflect historical and current ecological influences, where tribes are correlated to a combination of tropical climate types (Davis et al., 2002). Despite broad limitations to dung beetle diversity associated with annual temperatures below 15°C, and annual rainfall below 250 mm (Halffter, 1991; Espinoza, 2018), taxa distribution models reveal taxa-specific climatic niches, with some taxa exhibiting narrower climatic preferences and consequently more distribution-limited than others. The probability of Canthonini presence decreased with increasing elevation, with a much more pronounced negative trend as annual rainfall increased. Despite these trends, the probability of Canthonini presence increased as the precipitation of the coldest quarter increased, indicating the importance of the timing of precipitation for this tribe. In contrast to Coprini presence increased slightly as elevation augmented, with a stronger positive trend as precipitation of the driest month increased. Furthermore, Coprini presence also increased as precipitation seasonality increased. Phanaeini had a much higher probability of occurrence across much of Ecuador, exhibiting a more generalized climatic niche. The probability of Phanaeini presence increased with mean annual temperature and decreased as elevation increased.
Furthermore, Coprini presence also increased as precipitation seasonality increased. Phanaeini had a much higher probability of occurrence across much of Ecuador, exhibiting a more generalized climatic niche. The probability of Phanaeini presence increased with mean annual temperature and decreased as elevation increased.
Our results suggest that dung beetles were not frequently found in areas above 2000 m, consistent with Halffter (1991) and Espinoza (2018), who emphasizes that dung beetles are well adapted to climatic regions which are warmer and moister than those found at high elevations (Figure 2). Specifically, dung beetles in Ecuador are unlikely to be found in the High Andes, where climatic conditions get colder and have low atmospheric pressure, where only few a species like Dichotomius cotopaxi, can tolerate elevations above 2,500 m (Villamarín-Cortez, 2010, 2013; Carvajal et al., 2011).
All tribes analyzed are well distributed throughout the Neotropics and are expected to be found in areas where environmental conditions are favorable to their survival, especially the Coast and Amazonia, consistent with our distribution predictions. These regions have an appropriate environment for dung beetles to accomplish their life cycle. For example, the Coast has the most fertile and productive land, along with a tropical climate and a mean temperature of 25°C (77°F). Amazonia, in contrast, has less productive soil but is comprised mainly of rainforest, with a mean temperature of 23–25°C. Despite known temperature limitations, tribes were most strongly correlated to both rainfall (Walter and Lieth, 1964; Noriega et al., 2015) and elevation (Espinoza, 2018), which may reflect past Cenozoic dispersal patterns into northern regions when their climates were warmer (Laporte and Zihlman, 1983; Parrish, 1987; Carvajal et al., 2011), these different climate conditions have helped dung beetles to evolve and survive since the megafauna extinction and Pleistocene climate oscillations (Maldaner et al., 2021). Nevertheless, these regions and ecosystems have changed drastically, due to the expansion of the agricultural border, such activities reflect deep modifications in dung beetle assemblage composition, mainly because they are a very sensitive group to even low-intensity habitat modification (Bicknell et al., 2014) While our results suggest differences in climatic niche breadth for the three taxa, it is important to recognize the limitations of the distribution models presented here, specifically, the lack of true absence data and the importance of species interactions and finer scale resource limitations in moderating habitat use and distributions. However, this broad scale understanding of taxa distribution and climatic preferences aids our understanding of potential climate change impacts and helps identify critical habitat for dung beetle diversity from Ecuador.
Taxonomic beta diversity (species turnover) was more sensitive to spatial scale than functional beta diversity (functional group turnover) in assemblages of dung beetles throughout Ecuador (Figure 3). When plot size was decreased, species turnover increased at a greater rate compared to functional group turnover. In other words, plots were more likely to contain distinct species of beetle, but not necessarily distinct functional groups. This pattern signifies that a few key functional groups are present in most habitats, but that these functional roles are often filled by unique species. The presence of many functionally redundant species suggests that dispersal limitation may structure dung beetle communities in Ecuador. When species are dispersal limited, communities often become spatially heterogeneous through ecological or evolutionary drift (Baur, 2014), which creates high taxonomic beta diversity. However, it is also possible that the functional groups that we have delineated, which are based on size, feeding strategy, and nesting behavior, do not capture critical traits that distinguish a species’ ability to survive in a particular habitat or community. Additionally, while this analysis considered an array of spatial scales, all were above 80 km2. Taxonomic and functional beta diversities may perform differently at smaller scales, where dispersal is likely to be less limiting.
According to our findings, taxonomic and functional beta diversity was higher in the Amazon basin compared to the coastal region. This may be due to the higher rate of dung production in Amazonia, which is known to influence dung beetles’ abundance and diversity (Campos and Hernández, 2013, da Silva and Hernandez, 2014, Silva and Hernández, 2015, Silva and Hernández, 2016). The physical structure of the forest floor, which is influenced by leaf litter, can affect the nesting activities of certain dung beetles’ guilds such as rollers (Nichols et al., 2008). Roller dung beetles roll food resources several meters away from their source until they find an adequate place to bury them. This behavior helps them escape high competition on ephemeral resources such as dung and carrion. In contrast, we see lower levels of species heterogeneity in the Coast. We expected the drier climate in this region to reduce the diversity of dung beetle nesting behaviors found in Amazonia. However, surprisingly, the two regions contained a similar number of functional groups (12 in the coast and 14 in Amazonia) and, furthermore, functional beta diversity responded to spatial scale equally in both regions (Figure 4B). This suggests that ecological drift and dispersal limitation, affect taxonomic diversity more than functional diversity, may play large roles in maintaining high species richness in the Amazonian region. While deterministic factors such as niche partitioning certainly must also be at play, the similarity of functional beta diversity between the coast and Amazonia suggests that stochastic processes are more responsible for the maintenance of species diversity in the Amazon.
In the context of threats due to land use, our results demonstrate the necessity of conserving landscapes across a wide variety of environmental gradients to respond to beetle diversity. Dung beetle communities can be sensitive to habitat change and it is common to see the reduction in diversity and compositional changes in degraded habitats (Nichols et al., 2007; Solar et al., 2016). Our results suggest that habitat changes primarily drive these responses to the microclimate and vegetation composition and structure. The high levels of taxonomic beta diversity at small spatial scales suggests that the amazon maintains a high species richness by having higher species turnover, especially because vegetation is well preserved, maintaining good soil conditions, augmenting successful breeding and manure burial (Maldonado et al., 2019), which are essential for dung beetle survival.
Our results provide an essential framework for evaluating potential dung beetle habitat and diversity at different scales. Overall, our results are consistent with the knowledge of dung beetle biological and ecological requirements, and provides a country-wide analysis of Canthonini, Coprini, and Phanaeini dung beetle tribes distribution and diversity. Therefore, by identifying dung beetles’ diversity, combined with considerations of habitat fragmentation, human land-use alteration, and climate change, will be an important next step to inform better and prioritize dung beetle conservation efforts in other countries.
The raw data supporting the conclusions of this article will be made available by the authors, without undue reservation.
SV-C: study concept and design. SV-C, LH, SC, and JM: analysis, interpretation of data, and statistical analysis. SV-C, LH, SC, JM, and JN: drafting of the manuscript. All authors contributed to the article and approved the submitted version.
We are grateful to the University of Nevada, Reno for putting this work to test; Kevin Shoemaker for his valuable comments and to the National Institute of Biodiversity of Ecuador for letting us use their labs and collections.
The authors declare that the research was conducted in the absence of any commercial or financial relationships that could be construed as a potential conflict of interest.
All claims expressed in this article are solely those of the authors and do not necessarily represent those of their affiliated organizations, or those of the publisher, the editors and the reviewers. Any product that may be evaluated in this article, or claim that may be made by its manufacturer, is not guaranteed or endorsed by the publisher.
The Supplementary material for this article can be found online at: https://www.frontiersin.org/articles/10.3389/fevo.2022.1008477/full#supplementary-material
Baiser, B., and Lockwood, J. L. (2011). The relationship between functional and taxonomic homogenization. Glob. Ecol. Biogeogr. 20, 134–144. doi: 10.1111/j.1466-8238.2010.00583.x
Balmford, A., and Bond, W. (2005). Trends in the state of nature and their implications for human well-being. Ecol. Lett. 8, 1218–1234. doi: 10.1111/j.1461-0248.2005.00814.x
Barbet-Massin, M., Jiguet, F., Albert, C., and Thuiller, W. (2012). Selecting pseudo-absences for species distribution models: how, where and how many? Methods Ecol. Evol. 3, 327–338. doi: 10.1111/j.2041-210X.2011.00172.x
Barragán, F., Moreno, C., Escobar, F., Halfter, G., and Navarrete, D. (2011). Negative impacts of human land use on dung beetle functional diversity. PLoS One 6:e17976. doi: 10.1371/journal.pone.0017976
Baselga, A., Bonthoux,, and Balent, (2015). Temporal beta diversity of bird assemblages in agricultural landscapes: land cover change vs stochastic processes. PLoS One 10:e0127913. doi: 10.1371/journal.pone.0127913
Bass, M. S., Finer, M., and Jenkins, C. N. (2010). Global conservation significance of Ecuador’s Yasuní National Park. PLoS One 5:e8767. doi: 10.1371/journal.pone.0008767
Baur, B. (2014). Dispersal-limited species -a challenge for ecological restoration. Basic Appl. Ecol. 15, 559–564.
Bicknell, J. E., Phelps, S. P., Davies, R. G., Mann, D. J., Struebig, M. J., and Davies, Z. G. (2014). Dung beetles as indicators for rapid impact assessments: evaluating best practice forestry in the neotropics. Ecol. Indic. 43, 154–161. doi: 10.1016/j.ecolind.2014.02.030
Birkett, A. J., Blackburn, G. A., and Menéndez, R. (2018). Linking species thermal tolerance to elevational range shifts in upland dung beetles. Ecography 41, 1510–1519. doi: 10.1111/ecog.03458
Brooks, T. M., Mittermeier, R. A., and da Fonseca, G. A. B. (2006). Global biodiversity conservation priorities. Science 313, 58–61. doi: 10.1126/science.1127609
Brooks, T. M., Mittermeier, R. A., and Mittermeier, C. G. (2002). Habitat loss and extinction in the hotspots of biodiversity. Conserv. Biol. 16, 909–923. doi: 10.1046/j.1523-1739.2002.00530.x
Buckley, L. B., and Jetz, W. (2008). Linking global turnover of species and environments. PNAS 105, 17836–17841. doi: 10.1073/pnas.0803524105
Cambefort, Y. (1991). “Biogeography and evolution” in Dung beetle ecology. eds. I. Hanski and Y. Cambefort (Princeton: Princeton University Press), 51–67. doi: 10.1515/9781400862092.51
Campos, R. C., and Hernández, M. I. M. (2013). Dung beetle assemblages (Coleoptera, Scarabaeinae) in Atlantic forest fragments in southern Brazil. Revista Brasileira de Entomologia 57, 47–54. doi: 10.1590/S0085-56262013000100008
Cardoso, P., Rigal, F., Carvalho, J. C., Fortelius, M., Borges, P. A. V., Podani, J., et al. (2014). Partitioning taxon, phylogenetic and functional beta diversity into replacement and richness difference components. J. Biogeogr. 41, 749–761. doi: 10.1111/jbi.12239
Carvajal, V., Villamarín-Cortez, S., and Ortega, A. M. (2011). Escarabajos del Ecuador. Principales géneros. Instituto de Ciencias Biológicas, Escuela Politécnica Nacional, Serie Entomología, No. 1. Quito, Ecuador. xviii + 350 pp.
Chase, J. M., and Myers, J. A. (2011). Disentangling the importance of ecological niches from stochastic processes across scales. Philos. Trans. R. Soc. Lond. B. Biol. Sci. 366, 2351–2363. doi: 10.1098/rstb.2011.0063
Clark, J. S., Bell, D. M., Hersh, M. H., and Nichols, L. (2011). Climate change vulnerability of forest biodiversity: climate and competition tracking of demographic rates. Glob. Chang. Biol. 17, 1834–1849. doi: 10.1111/j.1365-2486.2010.02380.x
Coleman, D. C., and Hendrix, P. F. (eds.) (2000). Invertebrates as webmasters in ecosystems. CABI Publishing, Wallingford, doi: 10.1079/9780851993942.0000.
Condit, R., Pitman, N., Leigh, E. G., Chave, J., Terborgh, J., Foster, R. B., et al. (2002). Beta-diversity in tropical forest trees. Science 295, 666–669. doi: 10.1126/science.1066854
Crist, T. O., Veech, J. A., Gering, J. C., and Summerville, K. S. (2003). Partitioning species diversity across landscapes and regions: a hierarchical analysis of alpha, beta, and gamma diversity. Am Nat. 162, 734–743. doi: 10.1086/378901
Cuesta, F., Peralvo, M., Merino-Viteri, A., Bustamante, M., Baquero, F., Freile, J. F., et al. (2017). Priority areas for biodiversity conservation in mainland Ecuador. Neotro. Biodiv. 3, 93–106. doi: 10.1080/23766808.2017.1295705
Currie, D. J., Francis, A. P., and Kerr, J. T. (1999). Some general propositions about the study of spatial patterns of species richness. Ecoscience 6, 392–399. doi: 10.1080/11956860.1999.11682541
Daniel, G. M., Noriega, J. A., da Silva, P. G., Deschodt, C. M., Sole, C. L., Scholtz, C. H., et al. (2021). Soil type, vegetation cover and temperature determinants of the diversity and structure of dung beetle assemblages in a south African open woodland and closed canopy mosaic. Austral Ecol. 47, 79–91. doi: 10.1111/aec.13138
Davis, A. L. V. (1996). Habitat associations in a south African, summer rainfall, dung beetle community(Coleoptera: Scarabaeidae, Aphodiidae, Staphylinidae, Histeridae, Hydrophilidae). Pedobiologia 40, 260–280.
Davis, A. L. V. (1997). Climatic and biogeographical associations of southern African dung beetles (Coleoptera: Scarabaeidae s. str.). Afr. J. Ecol. 35, 10–38.
Davis, A. L. V., and Dewhurst, C. F. (1993). Climatic and biogeographical associations of Kenyan and north Tanzanian dung beetles (Coleoptera: Scarabaeidae). Afr. J. Ecol. 31, 290–305. doi: 10.1111/j.1365-2028.1993.tb00543.x
Davis, A. L. V., and Scholtz, C. H. (2001). Historical versus ecological factors influencing global patterns of scarabaeine dung beetle diversity. Divers. Distrib. 7, 161–174. doi: 10.1111/j.1472-4642.2001.00102.x
Davis, A. L. V., Scholtz, C. H., and Philips, T. K. (2002). Historical biogeography of scarabaeine dung beetles. J. Biogeogr. 29:1217. doi: 10.1046/j.1365-2699.2002.00776.x
De Ona, J., De Ona, R., and Calvo, F. J. (2012). A classification tree approach to identify key factors of transit service quality. Expert Syst. Appl. 39, 11164–11171.
Doube, B. M. (1983). The habitat preference of some bovine dung beetles (Coleoptera: Scarabaeidae) in Hluhluwe game reserve, South Africa. Bull. Entomol. Res. 73, 357–371. doi: 10.1017/S0007485300008968
Duncan, R. P., Cassey, P., and Blackburn, T. M. (2009). Do climate envelope models transfer? A manipulative test using dung beetle introductions. Proc. Nat. Acad. Sci. U. S. A. 276, 1449–1457. doi: 10.1098/rspb.2008.1801
Espinoza, V., and Noriega, Y. J. (2018). Diversity of the dung beetles (Coleoptera: Scarabaeinae) in an altitudinal gradient in the east slope of los Andes, Napo province, Ecuador. Neotro. Biodiv. 4, 145–151. doi: 10.1080/23766808.2018.1512199
Fick, S. E., and Hijmans, R. J. (2017). Worldclim 2: new 1-km spatial resolution climate surfaces for global land areas. Int. J. Climatol. 37, 4302–4315. doi: 10.1002/joc.5086
Francis, A. P., and Currie, D. J. (1998). Global patterns of tree species richness in moist forests: another look. Oikos 81, 598–602. doi: 10.2307/3546780
Fukami, T., Bezemer, T. M., Mortimer, S. R., and Putten, W. H. (2005). Species divergence and trait convergence in experimental plant community assembly. Ecol. Lett. 8, 1283–1290. doi: 10.1111/j/1461-0248.2005.00829.x
Graham, C. H., and Fine, P. V. (2008). Phylogenetic beta diversity: linking ecological and evolutionary processes across space in time. Ecol. Lett. 11, 1265–1277. doi: 10.1111/j.1461-0248.2008.01256.x
Halffter, G. (1974). Element anciens de l’entomofaune néotropical: ses implications biogéographiques. Quaestiones Entomologicae 10, 223–262.
Halffter, G. (1991). Historical and ecological factors determining the geographical distribution of dung beetles (Coleoptera: Scarabaeidae: Scarabaeinae). Folia Entomológica Mexicana 82, 195–238.
Halffter, G., and Favila, M. E. (1993). The Scarabaeidae (Insecta: Coleoptera) an animal group for analysing, inventoryng and monitoring biodiversity in tropical rainforest and modified landscapes. Biol. Int. 27, 1–21.
Halffter, G., and Matthews, E. G. (1966). The natural history of dung beetles of the subfamily Scarabaeinae (Coleoptera, Scarabaeidae). Folia Entomológica Mexicana 12–14, 1–312.
Halsch, C. A., Shapiro, A. M., Fordyce, J. A., Nice, C. C., Thorne, J. H., Waetjen, D. P., et al. (2021). Insects and recent climate change Proc. Natl. Acad. Sci. U. S. A. 118, 1–9. doi: 10.1073/PNAS.2002543117
Hanski, I., and Cambefort, Y. (1991). “Species richness pp” in Dung beetle ecology. eds. I. Hanski and Y. Cambefort (Princeton, New Jersey: Princeton University Press), 350–365. doi: 10.1515/9781400862092.350
Hayes, L., Mann, D., Monastyrskii, A., and Lewis, O. (2009). Rapid assessments of tropical dung beetle and butterfly assemblages: contrasting trends along a forest disturbance gradient. Insect. Cons. Div. 2, 194–203. doi: 10.1111/j.1752-4598.2009.00058.x
Hijmans, R. (2022). Raster: Geographic data analysis and modeling. R package version 3, 5–29 https://CRAN.R-project.org/package=raster.
Hijmans, R. J., Phillips, S., Leathwick, J., and Elith, J. (2022). Dismo: Species distribution modeling. R package version 1, 3–8 https://CRAN.R-project.org/package=dismo.
Kirk, A. A., and Ridsdill-Smith, T. J. (1986). Dung beetle distribution patterns in the Iberian Peninsula. Entomophaga 31, 183–190. doi: 10.1007/BF02372369
Laporte, L. F., and Zihlman, A. L. (1983). Plates, climate and hominoid evolution. S. Afr. J. Sci. 79, 96–110.
Leibold, M. A., and Chase, J. M. (2017). “Metacommunity ecology: functional traits in metacommunities” in Monographs in population biology, vol. 59 (New Jersey, USA: Princeton University Press) doi: 10.2307/j.ctt1wf4d24
Lepori, F., and Malmqvist, B. (2009). Deterministic control on community assembly peaks at intermediate levels of disturbance. Oikos 118, 471–479. doi: 10.1111/j.1600-0706.2008.16989.x
Lewis, R. J. (2000). An introduction to classification and regression tree (CART) analysis, 2000 annual meeting of the Society for Academic Emergency Medicine. Available at: http://www.saem.org/download/lewis1.pdf. San Francisco, California.
Maldaner, M. E., Sobral-Souza, T., Prasniewski, V. M., and Vaz-de-Mello, F. Z. (2021). Effects of climate change on the distribution of key native dung beetles in south American grasslands. Agronomy 11:2033. doi: 10.3390/agronomy11102033
Maldonado, M. B., Aranibar, J. N., Serrano, A. M., Chacoff, N. P., and Vázquez, D. P. (2019). Dung beetles and nutrient cycling in a dryland environment. Catena 179, 66–73.
McGeoch, M. A., Van Rensburg, B. T., and Botes, A. (2002). The verification and application of bioindicators: a case study of dung beetles in a savanna ecosystem. J. Appl. Ecol. 39, 661–672. doi: 10.1046/j.1365-2664.2002.00743.x
Mckenna, D. D., Wild, A. L., Kanda, K., Bellamy, C. L., Beutel, R. G., Caterino, M. S., et al. (2015). The beetle tree of life reveals that Coleoptera survived end-Permian mass extinction to diversify during the Cretaceous terrestrial revolution. Syst. Entomol. 40, 835–880.
McKnight, M. W., White, P. S., McDonald, R. I., Lamoreux, J. F., Sechrest, W., Ridgely, R. S., et al. (2007). Putting beta-diversity on the map: broad-scale congruence and coincidence in the extremes. PLOS Biology 5:e272. doi: 10.1371/journal.pbio.0050272
Mittermeier, R. A., Myers, N., and Thomsen, J. B. (1998). Biodiversity hotspots and major tropical wilderness areas: approaches to setting conservation priorities. Conserv. Biol. 12, 12, 516–520. doi: 10.1046/j.1523-1739.1998.012003516.x
Moura, M. R., Villalobos, F., Costa, G. C., and Garcia, P. C. A. (2016). Disentangling the role of climate, topography and vegetation in species richness gradients. PLoS One 11:e0152468. doi: 10.1371/journal.pone.0152468
Myers, J. A., Chase, J. M., Jiménez, I., Jørgensen, P. M., Araujo-Murakami, A., Paniagua-Zambrana, N., et al. (2013). Beta-diversity in temperate and tropical forests reflects disimilar mechanisms of community assembly. Ecol. Lett. 16, 151–157. doi: 10.1111/ele.12021
Nealis, V. G. (1977). Habitat associations and community analysis of South Texas dung beetles (Coleoptera: Scarabaeinae). Can. J. Zool. 55, 138–147. doi: 10.1139/z77-017
Nichols, E., Larsen, T. H., Spector, S. H., Davis, A. J., Escobar, F., Favila, M., et al. (2007). Globaervl dung beetle response to tropical forest modification and fragmentation: a quantitative literature review and meta-analysis. Biol. Conserv. 137, 1–19. doi: 10.1016/j.biocon.2007.01.023
Nichols, E., Spector, S., Louzada, J., Larsen, T., Amesquita, S., Favila, M. E., et al. (2008). Ecological functions and ecosystem services provided by Scarabaeinae dung beetles. Biol. Conserv. 141, 1461–1474.
Noriega, J. A., Camero, E., Arias-Buriticá, J., Locarno, L. C. P., Montes, J. M., Acevedo, A., et al. (2015). Grado de cobertura del muestreo de escarabajos coprófagos (Coleoptera: Scarabaeidae: Scarabaeinae) en Colombia. Int. J. Trop. Biol. Conserv. 63, 97–125. doi: 10.15517/rbt.v63i1.13323
Noriega, J. A., Santos, A., Calatayud, J., Chozas, S., and Hortal, J. (2021). Short-and long-term temporal changes in the assemblage structure of Amazonian dung beetles. Oecologia 195, 719–736.
Orfanidis, S., Panayotidis, P., and Ugland, K. (2011). Ecological evaluation index continuous formula (EEI-c) application: a step forward for functional groups, the formula and reference condition values. Mediterr. Mar. Sci. 12, 199–232.
Otavo, S., Parrado-Rosselli, A., and Noriega, J. A. (2013). Superfamilia Scarabaeoidea (Insecta: Coleoptera) como elemento bioindicador de perturbaci on antropog enica en un parque nacional amaz onico. Rev. Biol. Trop. 61, 735–752.
Parrish, J. T. (1987). “Global palaeogeography and palaeoclimate of the late Cretaceous and early Tertiary,” in The Origins of Angiosperms and their Biological Consequences. eds. E. M. Friis, W. G. Chaloner, and P. R. Crane (New York: Cambridge University Press), 51–73.
Pebesma, E. (2018). Simple features for R: standardized support for spatial vector data. R J. 10, 439–446. doi: 10.32614/RJ-2018-009
Philips, K., Pretorius, E., and Scholtz, C. (2004). A phylogenetic analysis of dung beetles (Scarabaeinae: Scarabaeidae): unrolling an evolutionary history. Invertebr. Syst. 18, 53–88. doi: 10.1071/IS03030
R Core Team (2022). R: A language and environment for statistical computing. R Foundation for Statistical Computing, Vienna, Austria. Available at: https://www.R-project.org/.
Reid, W., Mooney, H. A., Cropper, A., Capistrano, D., Carpenter, S. R., Chopra, K., et al. (2005). Millennium ecosystem assessment synthesis. eds. J. Sarukhán and A. Whyte (United Nations), 1–219.
Samways, M. J. (2005). Insect diversity conservation. Cambridge University Press.Cambridge, doi: 10.1017/CBO9780511614163.
Siefert, A., Ravenscroft, C., Weiser, M., and Swenson, N. (2012). Patterns of functional beta diversity reveal deterministic assembly processes in North American tree communities. Glob. Ecol. Biogeogr. 22, 682–691.
Sierra, R., Calva, O., and Guevara, A. (2021). La Deforestación en el Ecuador, 1990-2018. Factores promotores y tendencias recientes. Ministerio de Ambiente y Agua del Ecuador, Ministerio de Agricultura del Ecuador, en el marco de la implementación del Programa Integral Amazónico de Conservación de Bosques y Producción Sostenible. Quito, Ecuador. 216 pp.
Silva, P. G. D., and Hernández, M. I. M. (2014). Local and regional effects on community structure of dung beetles in a mainland-island scenario. PLoS One 9:e111883. doi: 10.1371/journal.pone.0111883
Silva, P. G. D., and Hernandez, M. I. M. (2014). Local and regional effects on community structure of dung beetles in a mainland-island scenario. PLoS One 9:e111883
Silva, P. G. D., and Hernández, M. I. M. (2015). Scale-dependence of processes structuring dung beetle metacommunities using functional diversity and community deconstruction approaches. PLoS One 10:e0123030. doi: 10.1371/journal.pone.0123030
Silva, P. G. D., and Hernández, M. I. M. (2016). Spatial variation of dung beetle assemblages associated with forest structure in remnants of southern Brazilian Atlantic Forest. Revista Brasileira de Entomologia 60, 73–81. doi: 10.1016/j.rbe.2015.11.001
Socolar, J. B., Gilroy, J. J., Kunin, W. E., and Edwards, D. P. (2016). How should beta-diversity inform biodiversity conservation? Trends Ecol. Evol. 31, 67–80. doi: 10.1016/j.tree.2015.11.005
Sokol, E. R., Benfield, E. F., Belden, L. K., and Valett, H. M. (2011). The assembly of ecological communities inferred from taxonomic and functional composition. Am. Nat. 177, 630–644.
Solar, R. R., Barlow, J., Andersen, A. N., Schoereder, J. H., Berenguer, E., Ferreira, J. N., et al. (2016). Biodiversity consequences of land-use change and forest disturbance in the Amazon: a multi-scale assessment using ant communities. Biol. Conserv. 197, 98–107. doi: 10.1016/j.biocon.2016.03.005
Spector, S. (2006). Scarabaeine dung beetles (Coleoptera: Scarabaeidae: Scarabaeinae): an invertebrate focal taxon for biodiversity research and conservation. Coleopterist Bull. Monograph 5, 71–83.
Swenson, N. G., Anglada-Cordero, P., and Barone, J. A. (2011). Deterministic tropical tree community turnover: evidence from patterns of functional beta diversity along an elevational gradient. Proc. of the R Soc. B: Bio. Sci. 278, 877–884. doi: 10.1098/rspb.2010.1369
Therneau, T., Atkinson, B., and Ripley, B. (2015). rpart: Recursive partitioning and regression trees. R package version. 4, 1–9.
Urban, D., Goslee, S., Pierce, K., and Lookingbill, T. (2002). Extending community ecology to landscapes. Ecoscience 9, 200–212.
Villamarín-Cortez, S. (2010). Escarabajos estercoleros (Coleoptera: Scarabaeinae) de El Goaltal, provincia de Carchi, Ecuador: lista anotada de especies y ecología. Avances en Ciencias e Ingenierías 2, B98–B103.
Villamarín-Cortez, S. (2013). Caracterización bioecológica de escarabajos peloteros del proyecto Coca Codo Sinclair. eds. J. Ayala, M. Yanez-Muñoz and C. Ceron (Publicación Técnico-Divulgativa de la Empresa Pública Estratégica Hidroeléctrica Coca Codo Sinclair, Quito).
Villamarin-Cortez, S. (2014). Influencia de asentamientos humanos en la diversidad functional de escarabajos estercoleros (Scarabaeidae: Scarabaeinae) de la Reserva de Biosfera Sumaco. M.Sc. thesis, Facultad de Ciencias Exactas y Naturales, Pontificia Universidad Católica del Ecuador, Quito, Ecuador. 78 pp.
Villéger, S., Miranda, J. R., Hernandez, D. F., and Mouillot, D. (2012). Low funcional beta-diversity despite high taxonomic beta-diversity among tropical estuarine fish communities. PLOS ONE 7:e40679. doi: 10.1371/journal.pone.0040679
Wagner, D. L. (2020). Insect declines in the Anthropocene. Annu. Rev. Entomol. 65, 457–480. doi: 10.1146/annurev-ento-011019-025151
Whittaker, R. H. (1960). Vegetation of the siskiyou mountains, Oregon and California. Ecol. Monogr. 30, 279–338.
Woodruff, R. E. (1973). “The scarab beetles of Florida,” in Arthropods of Florida . Volume 8. Florida Department of Agriculture and Consumer Services, Gainesville, Florida, USA.
Keywords: Amazonia, biodiversity, distribution modeling, ecological niche modeling, functional beta diversity, Neotropics, Scarabaeidae, species turnover
Citation: Villamarin-Cortez S, Hankin L, Coronado S, Macdonald J and Noriega JA (2022) Diversity and distribution patterns of Ecuador’s dung beetles (Coleoptera: Scarabaeinae). Front. Ecol. Evol. 10:1008477. doi: 10.3389/fevo.2022.1008477
Received: 31 July 2022; Accepted: 02 November 2022;
Published: 15 December 2022.
Edited by:
Hui Zhang, Institute of Oceanology (CAS), ChinaReviewed by:
Dana Price, Salisbury University, United StatesCopyright © 2022 Villamarin-Cortez, Hankin, Coronado, Macdonald and Noriega. This is an open-access article distributed under the terms of the Creative Commons Attribution License (CC BY). The use, distribution or reproduction in other forums is permitted, provided the original author(s) and the copyright owner(s) are credited and that the original publication in this journal is cited, in accordance with accepted academic practice. No use, distribution or reproduction is permitted which does not comply with these terms.
*Correspondence: Santiago Villamarin-Cortez, c2FuYmlvbEBnbWFpbC5jb20=
Disclaimer: All claims expressed in this article are solely those of the authors and do not necessarily represent those of their affiliated organizations, or those of the publisher, the editors and the reviewers. Any product that may be evaluated in this article or claim that may be made by its manufacturer is not guaranteed or endorsed by the publisher.
Research integrity at Frontiers
Learn more about the work of our research integrity team to safeguard the quality of each article we publish.