- 1Key Laboratory of Wetland Ecology and Environment & Heilongjiang Xingkai Lake Wetland Ecosystem National Observation and Research Station, Northeast Institute of Geography and Agroecology, Chinese Academy of Sciences, Changchun, China
- 2College of Geographical Sciences, Changchun Normal University, Changchun, China
- 3Key Laboratory of Songliao Aquatic Environment, Ministry of Education, Jilin Jianzhu University, Changchun, China
Excessive accumulation of heavy metals in global lake sediments poses a serious threat to lake water quality and ecosystem security. However, there is still a knowledge gap in comparison of heavy metal variation and pollution in hydrologically connected lakes. In this study, concentrations of As, Cd, Cr, Cu, Hg, Pb, and Zn in sediments of two hydrologically connected lakes, Xingkai Lake and Xiaoxingkai Lake, were determined during the hydrologically connected periods (May and September) and disconnected period (January and July) in 2021. We found the range of As was 2.58∼14.35 mg/kg, Cd was 0.050∼0.21 mg/kg, Cr was 28.58∼262.3 mg/kg, Cu was 3.12∼28.05 mg/kg, Hg was 0.0030∼0.14 mg/kg, Pb was 10.87∼58.86 mg/kg, and Zn was 18.21∼90.73 mg/kg. Heavy metal concentrations were lower than grade I level in Chinese soil quality standards with significant spatial and temporal differences in the basin. Overall, most of the sampling sites in Xingkai Lake and Xiaoxingkai Lake were at the uncontaminated level and moderate ecological risk during the sampling period. Two lakes showed different heavy metal compositions, accompanied by higher contamination level and higher potential ecological risk in the small lake than those in the large lake based on analysis of the geo-accumulation index and potential ecological risk index. Besides, the contamination level and potential ecological risk in May and September were higher than those in January and July, mainly due to human activities and hydrological connectivity. The ecological risks were moderate for Cd and Hg, and low for As, Cr, Cu, Pb, and Zn. Correlation and PCA analyses showed that Cd mainly originated from anthropogenic sources, while other metals mainly came from natural sources. These findings elucidate the effects of agriculture and hydrological connectivity on heavy metals in sediments, and provide scientific basis for the reasonable management of lake ecosystem.
Introduction
Heavy metal pollution is one of the most concerning issues globally, and is considered as typical pollutant in the environment due to their high toxicity, environmental persistence, bioaccumulation, carcinogenicity, and complex mechanisms with multiple sources and pathways (Yu et al., 2021; Miranda et al., 2022). Heavy metals are discharged into lakes through multiple natural and anthropogenic pathways such as atmospheric deposition, rain wash, and surface runoff, and then deposit in the sediments (Yu et al., 2021), resulting in increasing heavy metal concentrations in global lakes from 1970s to 2010s (Zhou et al., 2020). Although many studies on heavy metal pollution in lakes have been carried out (e.g., Veeranam Lake in India, Suresh et al., 2012; Poyang Lake in China, Feng et al., 2019), it is still necessary to conduct relevant studies on individual lakes due to their differences in local economic development, pollution sources, and geographical conditions, particular for hydrologically connected lakes that characterized by complex hydrodynamics and landscape heterogeneity (Teng et al., 2020).
Lake sediment shows strong adsorption capacity for heavy metals and thus reduces the pollution level of overlying water (Xu et al., 2017). However, heavy metals are released into water under changing environmental conditions, including pH (Zhang Y. et al., 2018), dissolved oxygen, salinity, nitrogen, and phosphorus (Liu M. et al., 2019; Wang et al., 2021), and in turn cause secondary pollution to the water environment (Liu M. et al., 2019), influencing the structure and function of lake ecosystem (Xu et al., 2017). Moreover, heavy metal pollution in lakes has changed from single metal pollution to mixed metal pollution (Zhou et al., 2020), associated with complex interactions with clay, Fe-Mn oxides, and organic matter by means of ion exchange, complexation and chemical adsorption (Yuan et al., 2014; Yu et al., 2021). Sediment adsorption and desorption capacity play a key role in regulating the bioavailability and bioaccumulation of heavy metals along the food chain in lakes that ultimately endangers human health (Coelho et al., 2018). Therefore, it is crucial to understand heavy metal pollution and ecological risk and their interactions with environmental variables in sediments for the scientific management of lakes.
China is increasingly concerned about heavy metal risks and impacts, due to the rapid development of urbanization, industrialization, and agriculture that are considered as main sources of heavy metals in lakes (Xu et al., 2017; Han et al., 2020; Zhou et al., 2020). In particular, long-term irrational use of chemical fertilizer, pesticide, herbicide, and mulching film in agricultural activities can lead to severe heavy metal pollution in lake sediments (Li et al., 2017; Peng et al., 2020). Cd, Hg, As, Cu, Pb, Cr, and Zn show high pollution levels and potential ecological risk in 71% of provinces in China, and thus are considered as key management targets for relevant administrative agencies (Xu et al., 2017). Understanding the temporal and spatial variation of heavy metals and differentiating the source of the metal accumulation in surface sediment are critical for sediment quality assessment and lake ecosystem protection.
Xingkai (Khanka) Lake, a shallow transboundary lake shared by China and Russia, is the largest freshwater lake in northeast China. Lots of large farms have been built concomitant with rapid shrinkage and fragmentation of wetlands (Song et al., 2012; Yuan et al., 2018). Moreover, continuous use of fertilizer, pesticide, and herbicide in agriculture activities contributed to increased concentrations of nutrients and heavy metals and decreased water quality in the entire basin (Yuan et al., 2021). As a consequence, the water quality of Xingkai Lake and its hydrologically connected lake (Xiaoxingkai Lake) has changed from oligotrophic to eutrophic which may affect water quality in the Russian part of this lake (Piao and Wang, 2011). Nevertheless, little is known about the heavy metal pollution and ecological risk in Xingkai Lake and Xiaoxingkai Lake. Understanding the temporal and spatial variation, pollution and ecological risk of heavy metals in sediments of these two lakes is helpful to provide a scientific basis for ecological assessment of water quality and effective basin management.
The principal goal of our study was to investigate the temporal and spatial variation of different heavy metals in the surface sediment and to evaluate their potential ecological risks in two hydrologically connected lakes. We also examined the interactions between heavy metals and environmental variables to identify the primary driving factors. Our hypotheses were that: (1) heavy metals show significant temporal and spatial differences in sediments; and (2) different potential ecological risks of heavy metals in two lakes although they are hydrologically connected.
Materials and methods
Study area
Xingkai Lake basin (44° 30’ – 45° 30’ N, 132° 00’ – 132° 50’ E) (Figure 1) is an important breeding area and migration habitat for waterfowl, including Grus japonensis, Grus vipio, and Ciconia boyciana (Jin and Zhai, 2006), with an open water area of 4,190 km2 and a catchment area of 16,890 km2 (Sun et al., 2018). Xingkai Lake is a typical semi-enclosed shallow lake (mean water depth 3.5 m) and is characterized by frequent strong winds and low water transparency (Yuan et al., 2021). Xiaoxingkai Lake is an inland shallow lake (mean water depth 1.8 m) with a volume of 3.3 × 108 m3 water. These two lakes are formed in a Cenozoic tectonic depression and divided by a nature sandy ridge, and their hydrological connectivity is controlled by floodgates. Large amounts of natural silt minerals were brought into the lakes by inflow rivers, leading to the dominance of silt with a content ranging from 61.9 to 93.8% in sediments (Sun et al., 2018). The climate is characterized by a temperate continental monsoon, with below-freezing temperatures from October to April. The annual precipitation is 574 mm, up to 80% of which takes place from May to September (Song et al., 2012). The annual water inflow into the entire catchment is 55.68 × 108 m3, and the annual outflow is 17.2 × 108 m3. The main inflow coming from the Muling River contributes more than 70% of the total inflows of Xiaoxingkai Lake. The Song’acha River is the single outflow channel through which the water of Xingkai Lake flows into the Ussuri River (Jin and Zhai, 2006).
Sample collection and processing
According to the technical manual of lake ecology survey (Huang, 2000), combined with the characteristics of geography and surrounding environment in the study area, a total of 15 sampling sites were set up. Of all sampling sites, there were nine sites (XCZH, X25L, XXKL, XHX, XDH, XYZ, XKK, XEZ, and X31L) in Xiaoxingkai Lake and six sites (DBPZ, DLWZ, DXKL, DBYT, DYZ, and DEZ) in Xingkai Lake, as shown in Figure 1. Of these sites, X25L, X31L, XCZH, XDH, and XKK were at irrigation channels that connected with Xiaoxingkai Lake, XXKL was a tourism site with shipping activity, XYZ, XEZ, DLWZ, DYZ, DXKL, and DEZ were near flooding gates between Xingkai Lake and Xiaoxingkai Lake, DBPZ, and DBYT were fishing sites, while XHX was at the center of Xiaoxingkai Lake.
Surface sediment (0–10 cm) samples were collected using a grab sampler from each sampling site during the hydrologically connected periods (May and September) and disconnected period (January and July) in 2021 in Xingkai Lake and Xiaoxingkai Lake, and then stored in sealed bags at -18°C for subsequent analysis. The collected sediment samples were dried at 60°C, and then crushed and homogenized using a ceramic mortar and pestle. The ground samples were sieved through a 2-mm-mesh screen for soil organic matter (OM) content by loss-on-ignition method (Zhu et al., 2021) and through a 63-μm-mesh screen for determination of heavy metals, including Chromium (Cr), Copper (Cu), Zinc (Zn), Cadmium (Cd), Arsenic (As), Mercury (Hg), and Lead (Pb).
All sediment samples were analyzed in the Analysis and Measurement Center of Northeast Institute of Geography and Agroecology, Chinese Academy of Sciences. Cd and Pb were measured using an Inductively Coupled Plasma Mass Spectrometer (ICP-MS, ICAP Qc, Thermo Fisher, Germany). Concentrations of Cr, Cu, and Zn were determined by Inductively Coupled Plasma Atomic Emission Spectroscopy (ICP-AES, Perkin-Elmer Corp., Norwalk, United States). As and Hg were measured using Atomic Fluorescence Spectrometry (AFS, AFS-9750, Haiguang Instrument Co., China). Soil total nitrogen (TN) was determined using the modified Kjeldahl method (Kjeltec Auto Analyzer, Behr Labor Technik, Germany), and soil total phosphorus (TP) was measured by alkali fusion–Mo-Sb Anti spectrophotometric method with a UV–Vis spectrophotometer (Lambda750, PerkinElmer, United States).
Assessment and homology analysis of heavy metal pollution
The ecological risk and detected heavy metal contamination were quantified in this study. The Geo-accumulation index (GI) and Hakanson’s potential ecological risk index (PERI) were used to spatially quantify the pollution status and potential threats caused by heavy metal concentrations. Pearson correlation coefficient and principal component analysis were used to deduce the statistical relation of heavy metal homology.
Geo-accumulation index
Geo-accumulation index was proposed by Muller (1969), which has been widely used to assess the pollution degree of heavy metals in sediments and to determine the impact of human activities on the environment. Therefore, it is considered to be an important parameter to distinguish the concentration level of heavy metals induced by human influence and nature (Zhang P. et al., 2018; Liu et al., 2022). The index expression is shown as Equation 1:
In the equation, GI is the Geo-accumulation index of heavy metals, Ci is the measured concentrations of heavy metals in sediments, in mg⋅kg–1. Bi is the geochemical background value measured in the deposition of heavy metals, and the background values of soil heavy metals in Heilongjiang Province are adopted. 1.5 is a constant that allows for variations in background values possibly caused by diagenesis and weathering (Loska et al., 2004). According to the GI value, heavy metal pollution is divided into seven levels, ranging from practically uncontaminated to extremely contaminated, as shown in Table 1.
Potential ecological risk index
The index of potential ecological risk is proposed by Hakanson (1980), which simultaneously regards the heavy metal concentrations in sediments, type of pollutant, level of toxicity, and sensitivity of water bodies to heavy metal pollution (Zhang P. et al., 2018), and thus is usually used to comprehensively assess the potential impact of heavy metals on lake ecosystems. Equation 2 is used to calculate the ecological risk value of individual heavy metals in sediments:
Equation 3 calculates the comprehensive ecological risk of heavy metals in sediments:
where RI is the comprehensive potential ecological risk index, Ei is the individual potential ecological risk index of heavy metal i. Ti is the toxicity coefficient of heavy metal i (Cr = 2, Cu = 5, Zn = 1, As = 10, Cd = 30, Hg = 40, Pb = 5) (Yi et al., 2011). Ci is the measured value of heavy metal i in the sediment. Bi is the background value of heavy metal i in study area. Potential ecological risk of heavy metals in sediments are divided into five levels, and the specific classification is shown in Table 2.

Table 2. Classification of potential ecological risks according to Ei and RI values (Hakanson, 1980; Yi et al., 2011; Liu et al., 2021).
Inverse distance weighted
Inverse distance weighted (IDW) method was applied to simulate and predict heavy metal content based on the clear spatial distribution of heavy metals in the surface sediment as an exact interpolator, according to the consideration of data characteristics, sampling site setting and literature data (Chen et al., 2018). IDW interpolation uses a linearly weighted combination of a set of sampling points to determine pixel values for unsampled points (Haldar et al., 2020). Moreover, IDW is a deterministic method for multivariate interpolation of a known scattered set of points (Song et al., 2015), and can process extreme values (outliers) in a data set to make a comprehensive prediction of values in an unknown region (Wu et al., 2021). The general IDW interpolation formula is shown in Equations 4, 5:
where Z(x) is the predicted value at an interpolated point x, Zi is a known point, n is the total number of known points used in interpolation, di is the distance between point i and the prediction point, and wi is the weight assigned to point i. Greater weighting values are assigned to values closer to the interpolated point. Considering the distance increases with decreasing the weight (Shepard, 1968; Chen et al., 2018), u is the weighting power that determines how the weight decreases as the distance increases.
Statistical analysis
The patterns of heavy metal composition in sediments are visualized using non-metric multidimensional scaling (NMDS) based on Bray-Curtis distance. Similar metal compositions were grouped together on a two-dimensional NMDS diagram. Stress values are used to determine the number of dimensions. A stress value less than 0.1 is considered a good ordination of the data (Stauffer et al., 2014; Yuan et al., 2018).
Principal component analysis (PCA) is widely applied to identify the underlying factors and further explain their structure and internal relationships through transforming and rearranging correlated variables into a reduced set of new independent, orthogonal components, which are called principal components (PCs) (Gao et al., 2016). In this study, PCA of all metals with the varimax rotation of the standardized component loadings was conducted to distinguish the sources of possible pollutions. The Kaiser–Meyer–Olkin test and Bartlett’s test were performed to evaluate the validity of PCA, which required that the Kaiser–Meyer–Olkin (KMO) values should be more than 0.5 and Bartlett’s test be significant (<0.001). The loadings in PCA illustrated the extent to which each original variable contributes to the PCs. The association coefficients between variables and factors reflect the degree of proximity between them. Such proximity increases with the absolute value (loading) of a coefficient.
Data-processing software Excel 2016, SPSS 26, and R 4.1.0 were used to conduct statistical analysis of data. IDW interpolation method in Arcgis 10.2 was used to analyze spatial differences of heavy metals across the study area. Pearson correlation analysis was used to explore the relationships between the physicochemical properties and heavy metals, and one-way ANOVA and two-way ANOVA were applied to identify significant spatial and seasonal differences of heavy metals in sediments. Means ± standard errors of heavy metal concentrations and environmental variables are reported further. Significant results are reported at the 95% confidence level (p < 0.05).
Results
Characteristics of heavy metals in the sediment
The characteristics of heavy metals, TN, TP, and OM in the sediment of Xingkai Lake and Xiaoxingkai Lake were shown in Table 3. Average concentrations of metals in the surface sediments were ranked by Cr > Zn > Pb > Cu > As > Cd > Hg. Regarding to the coefficient of variation, the dispersion degree of Zn was the smallest with a low CV value of 0.26, while Hg showed the largest dispersion degree with a high CV value of 0.73. By contrast, the dispersion degrees of TN, TP, and OM were relatively higher (one-way ANOVA, p < 0.05) compared with those of heavy metals.
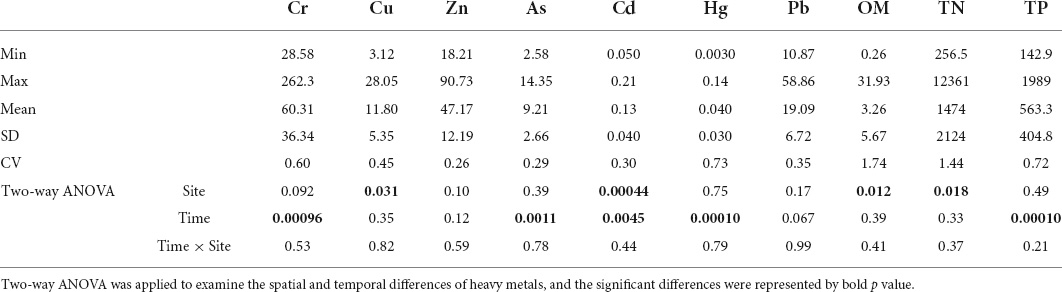
Table 3. Characteristics of heavy metals (mg/kg), TN (mg/kg), TP (mg/kg), and OM (%) in sediments of Xingkai Lake and Xiaoxingkai Lake.
Cd, Cu, OM and TN showed significant spatial differences (two-way ANOVA, p < 0.05) at all sampling sites, and the significant temporal differences (two-way ANOVA, p < 0.01) were observed in As, Cd, Cr, Hg, and TP from January to September. However, the interaction of site and sampling time had no effects on heavy metals and nutrients.
IDW spatial interpolation model was used to predict the spatial distribution of heavy metals in sediments (Figure 2). Taking the spatial distribution of metals as a whole, we found that there were two different distribution trends of seven heavy metals in two lakes. The spatial distribution trends of As, Cr, Cu, and Pb were similar, showing low-high-low concentrations distribution pattern from the western region to the eastern region. By contrast, heavy metals of Cd, Hg, and Zn showed higher concentrations in the western region compared to those in the eastern region (one-way ANOVA, p < 0.05), especially Cd (one-way ANOVA, p < 0.01). Besides, heavy metals in Xingkai Lake showed significant difference compared with those in Xiaoxingkai Lake (one-way ANOVA, p < 0.01), except Cu and Zn.
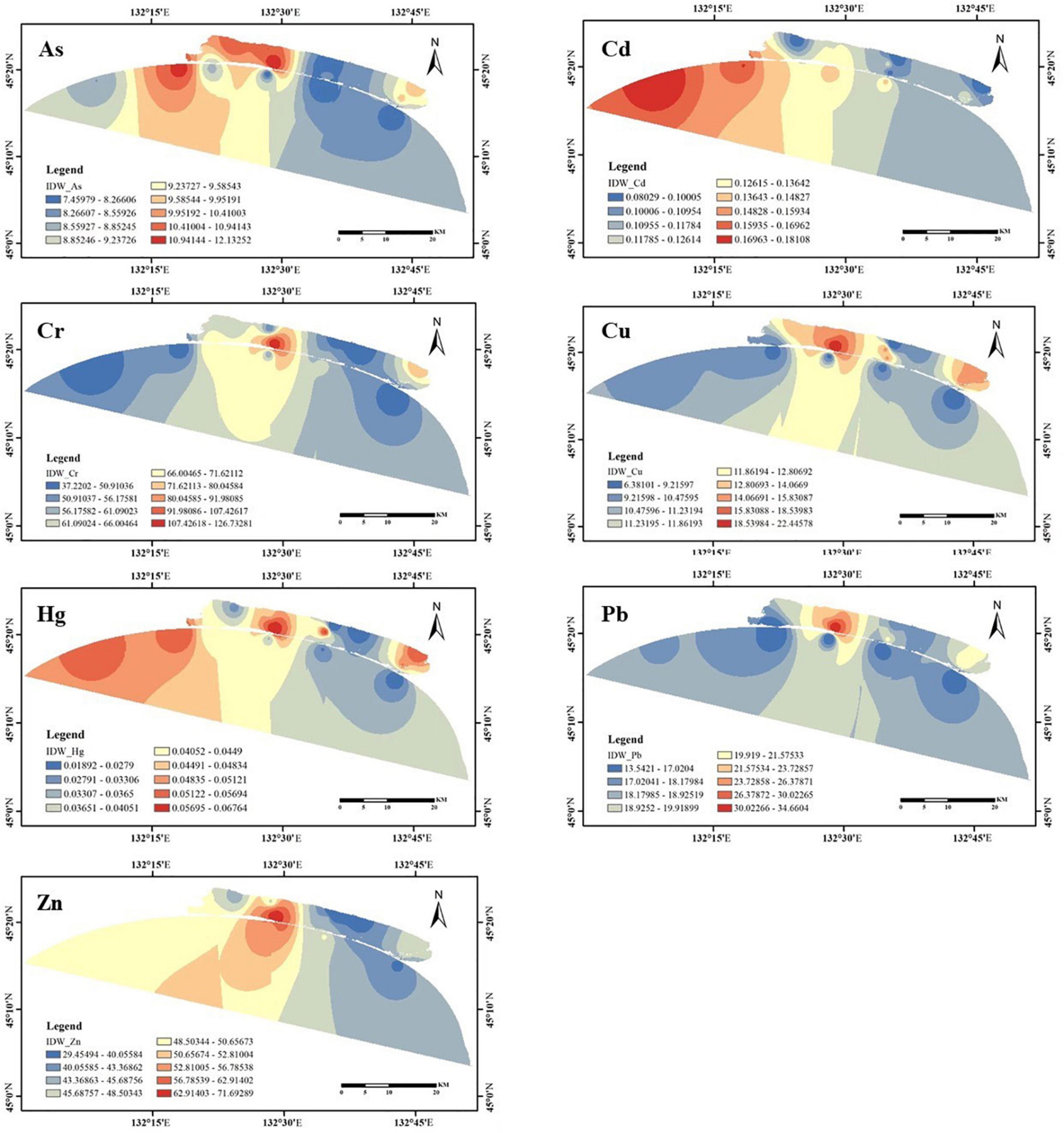
Figure 2. Spatial distribution of heavy metals in sediments in two lakes using IDW spatial interpolation model.
Heavy metal compositions in the sediment
The compositions of heavy metals in two lakes were further explored using NMDS (Figure 3). In Xingkai Lake, heavy metals in different months were mainly distributed in the left lower quadrant. In contrast, heavy metals in Xiaoxingkai Lake were mainly distributed in the right upper quadrant. Thus, heavy metal compositions were divided into two groups with low similarity between two lakes. Furthermore, total heavy metal concentration in XXKL was relatively higher than that in other sites (one-way ANOVA, p < 0.01, Figure 4).
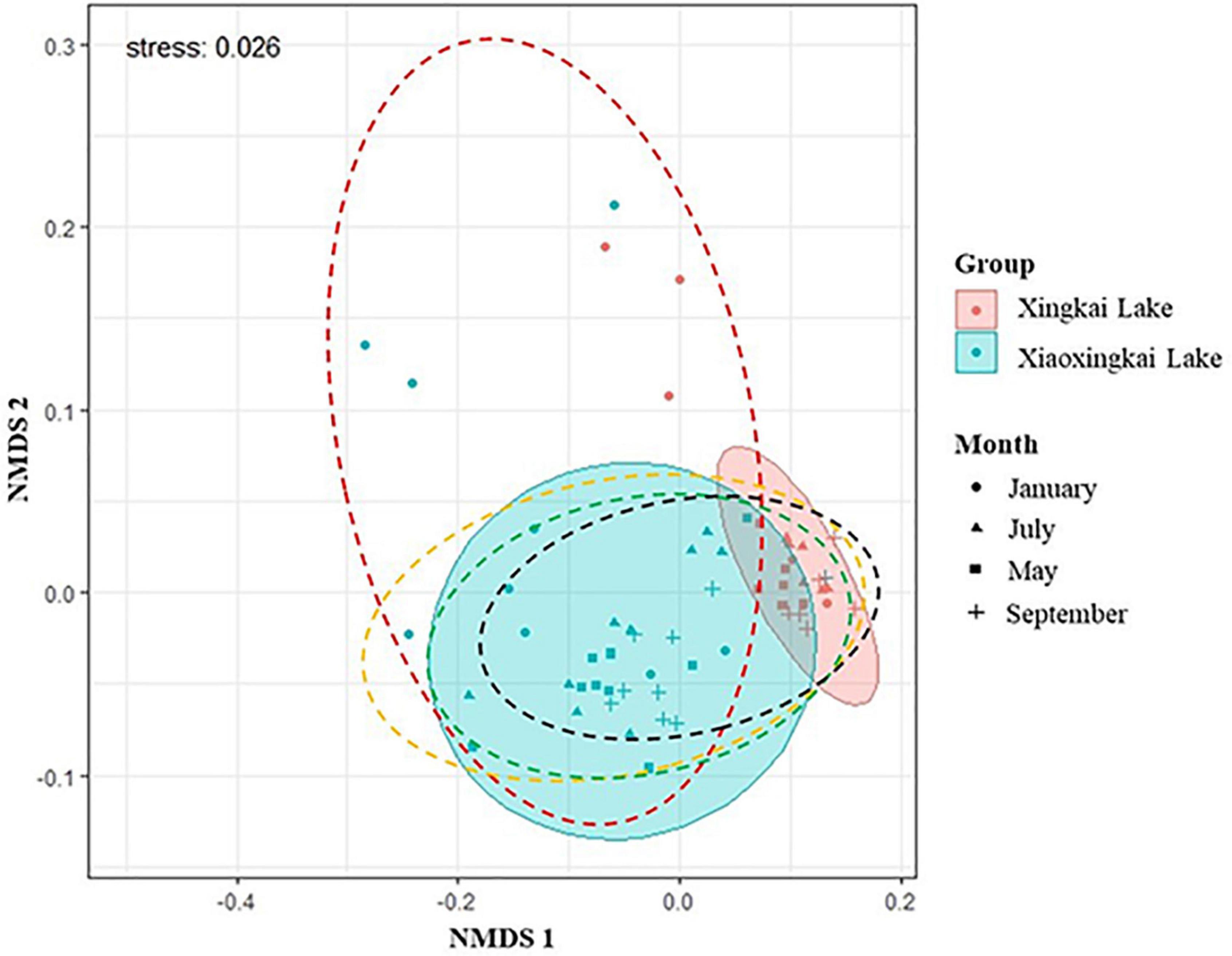
Figure 3. Ordination diagrams for non-metric multidimensional scaling of heavy metals in Xingkai Lake and Xiaoxingkai Lake in 4 months. The distance between points represents the similarity degree in heavy metal composition. Metals in January, May, July, and September are enclosed by red, orange, green, and black dotted lines. Metals in Xingkai Lake and Xiaoxingkai Lake are enclosed by pink and cyan circles. Stress = 0.026.
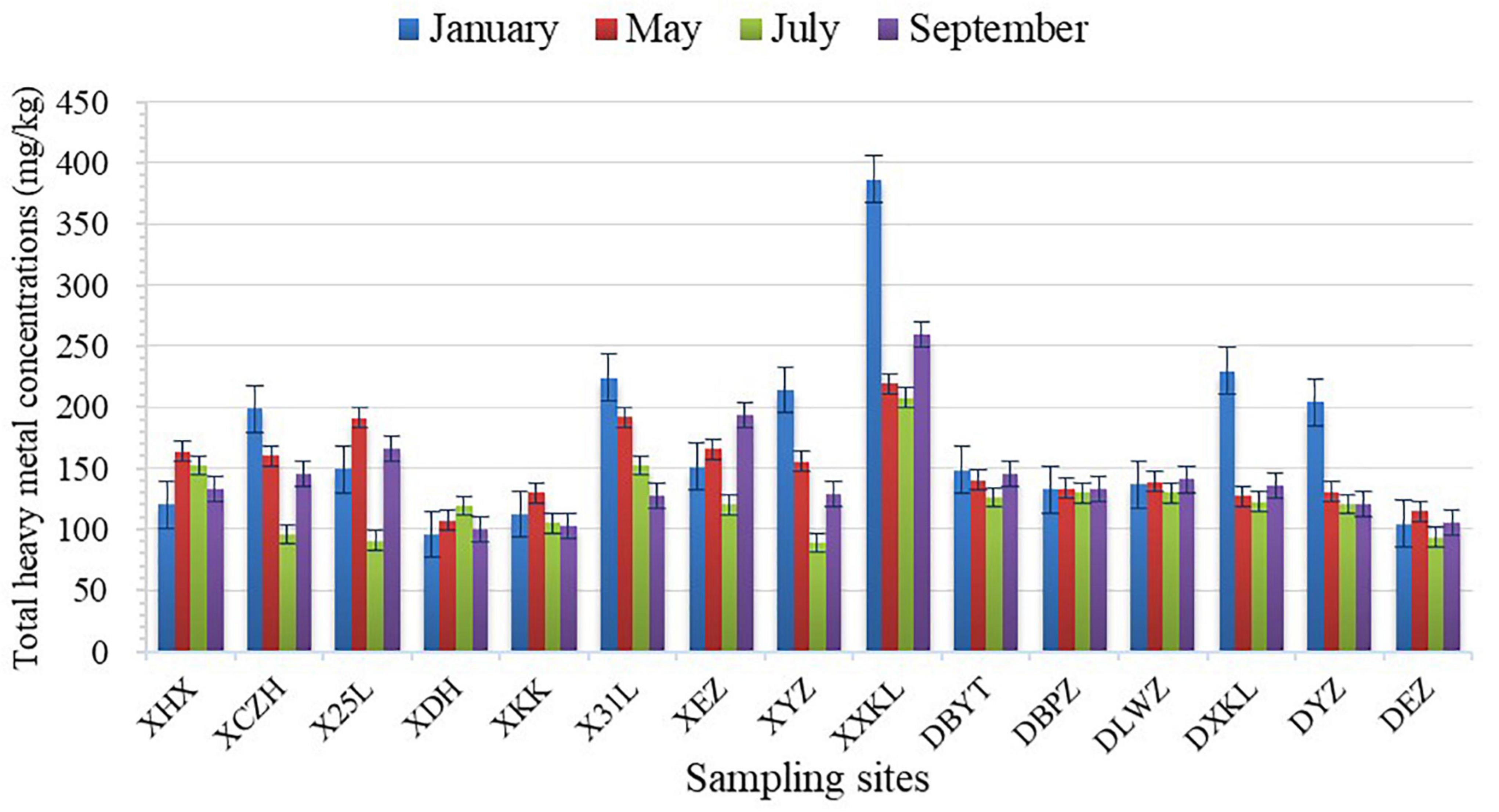
Figure 4. Total heavy metal concentrations in all sampling sites in January, May, July, and September.
Meanwhile, heavy metal compositions were similar in May, July, and September, whereas heavy metal composition in January showed low similarity with other three months. Comparing total heavy metal concentrations in four months (Figure 4), we found that the total heavy metal concentration was lower in July than that in other months (one-way ANOVA, p < 0.05). Among the four months, the standard deviation of total heavy metal concentration was higher in January (one-way ANOVA, p < 0.05), and the CV value further calculated in January was about twice that of other months (CV value of January was 0.41, May 0.20, July 0.24, and September 0.27, respectively), indicating high spatial variation of heavy metals in January.
Pollution and risk assessment of heavy metals in sediments
Geo-accumulation index
The GI values of heavy metals in the sediment of each sampling site are shown in Table 4. According to the spatial variation of GI value, only the heavy metal Cr in XXKL was in uncontaminated to moderately contaminated level. By contrast, other sites were subject to uncontaminated levels. Overall, the contamination level of heavy metals in Xiaoxingkai was higher than that in Xingkai Lake (p < 0.05), except Pb. From the perspective of temporal variation of GI value, the GI values of Cr in January (0.059), Cd in May (0.004) and September (0.208), and Hg in September (0.483) were determined to be uncontaminated to moderately contaminated level, whereas other heavy metals As, Cu, Pb, and Zn were at uncontaminated level during the sampling time. In total, the contamination level of heavy metals in September was higher than that in other months (p < 0.05).
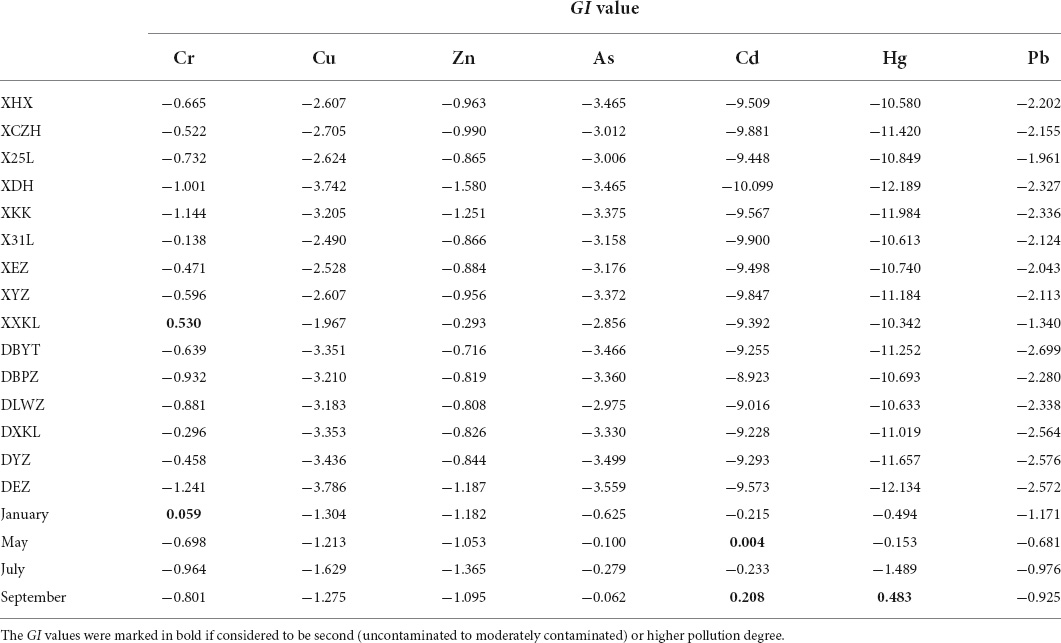
Table 4. The spatial and temporal variations of Geo-accumulation index (GI) value for heavy metals in sediments.
Potential ecological risk index
The PERIs of heavy metals showed significant spatial (one-way ANOVA, p < 0.05) and temporal differences (one-way ANOVA, p < 0.05) in the sediment of the study area (Table 5). The temporal variation of individual potential ecological risk and comprehensive potential ecological risk of Cd and Hg showed that they were at moderate to considerable ecological risk levels in May and September, and low ecological risk level in January and July. While other metals were at low ecological risk level during the sampling period. According to the variation of Ei value, Cd and Hg were considered at moderate ecological risk in most of the sampling sites, whereas other heavy metals As, Cr, Cu, Pb, and Zn showed low ecological risks in all sampling sites. In terms of RI value, most of the sampling sites were considered at moderate risk (Table 5). The RI value was particularly higher in XXKL with considerable ecological risk (one-way ANOVA, p < 0.01), while DEZ, XDH, and XKK showed lower RI values with low ecological risk in sediments compared to other sites (one-way ANOVA, p < 0.01).
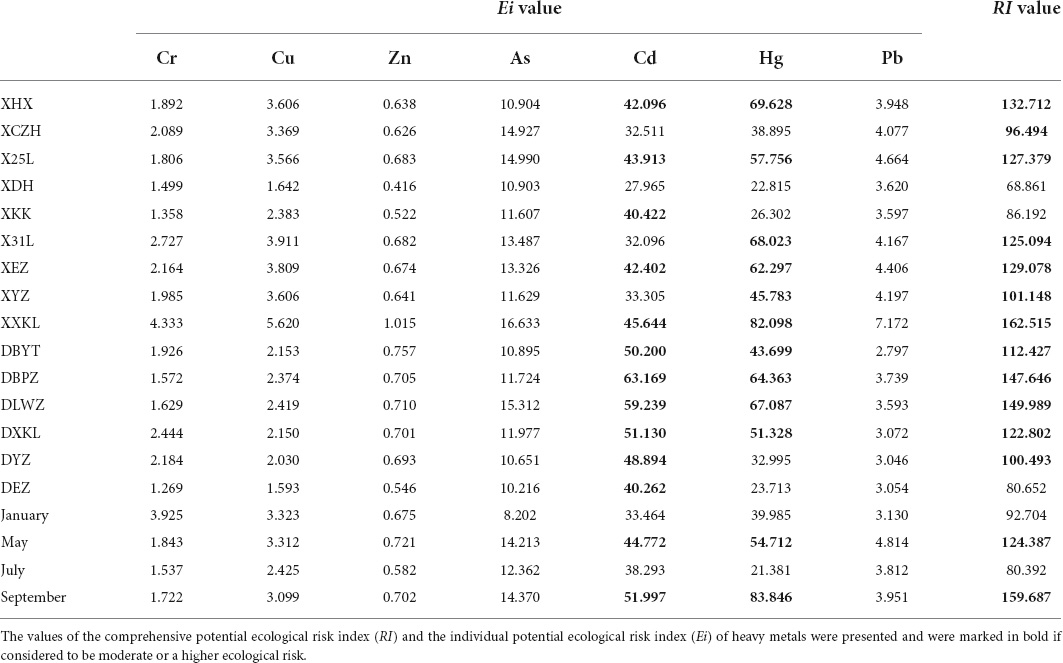
Table 5. Potential ecological risk index (PERI) of heavy metals in sediments at different sampling months and sites.
When taking all sampling sites apart into two lakes, Xiaoxingkai Lake faced higher ecological risks compared with Xingkai Lake in September (one-way ANOVA, p < 0.05). In addition, the overall ecological risk in Xingkai Lake and Xiaoxingkai Lake was lower in January and July than that in May and September (one-way ANOVA, p < 0.05).
Pollution source identification
Correlations between metals and nutrition elements
The correlations between heavy metals and nutrition elements in sediments were visually expressed in Figure 5. Most of heavy metals showed positive relationships with each other. Cr showed strongly positive correlations with As (r = 0.578, p < 0.05), Cu (r = 0.779, p < 0.001), Hg (r = 0.607, p < 0.05), Pb (r = 0.772, p < 0.001), and Zn (r = 0.818, p < 0.001). Cu was strong positively correlated with As (r = 0.699, p < 0.01), Hg (r = 0.724, p < 0.01), Pb (r = 0.901, p < 0.001), and Zn (r = 0.658, p < 0.01). As was positively correlated with Hg (r = 0.606, p < 0.05) and Pb (r = 0.736, p < 0.01). Zn showed positive relationships with As (r = 0.585, p < 0.05), Hg (r = 0.748, p < 0.01), and Pb (r = 0.624, p < 0.05). Meanwhile, Hg and Pb had a strong positive correlation (r = 0.601, p < 0.05). However, Cd showed no significant relationship with other metals or nutrients.
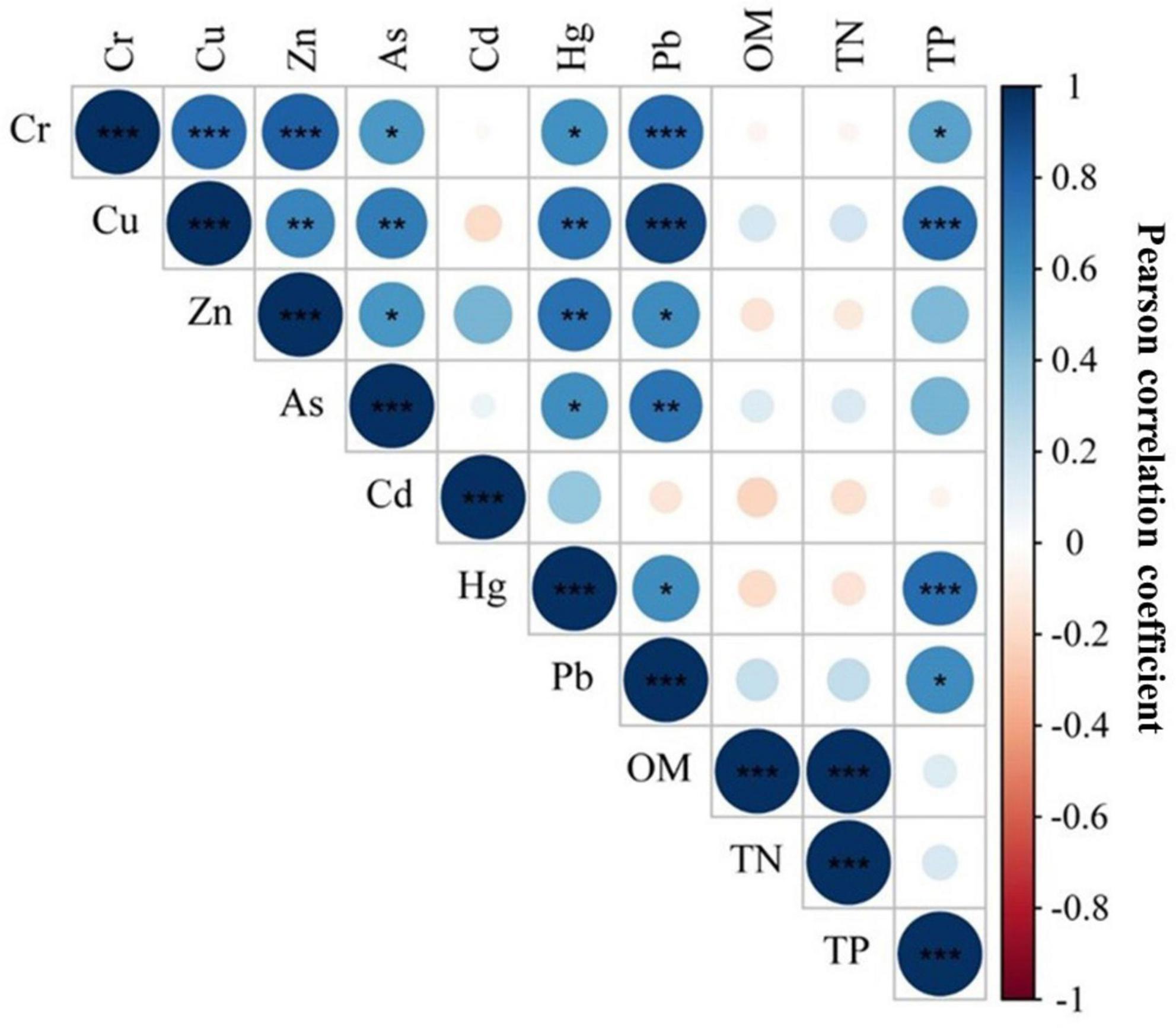
Figure 5. Pearson correlations between heavy metals and nutrition elements in sediments. The color and size of all circles indicate the strength of the correlations. The significant differences were represented by *p < 0.05, **p < 0.01, and ***p < 0.001.
Of all nutrition elements, OM was only strong positively correlated with TN (r = 0.997, p < 0.001), whereas OM and TN were not significantly correlated with any metals. By contrast, TP showed strong positive correlations with heavy metals, including Cr (r = 0.538, p < 0.05), Cu (r = 0.767, p < 0.001), Hg (r = 0.755, p < 0.001), and Pb (r = 0.624, p < 0.05).
Principal component analysis of heavy metals
The results of PCA of heavy metal contents in sediments are listed in Table 6. Firstly, the applicability of PCA was tested by Kaiser-Meyer-Olkin (KMO) to verify the adequacy of the sample and Barlett tests to examine the independence of each variable, with the results that KMO of 0.611 (>0.5) and Barlett test value of 0 (<0.05), indicating that the data is suitable for PCA.
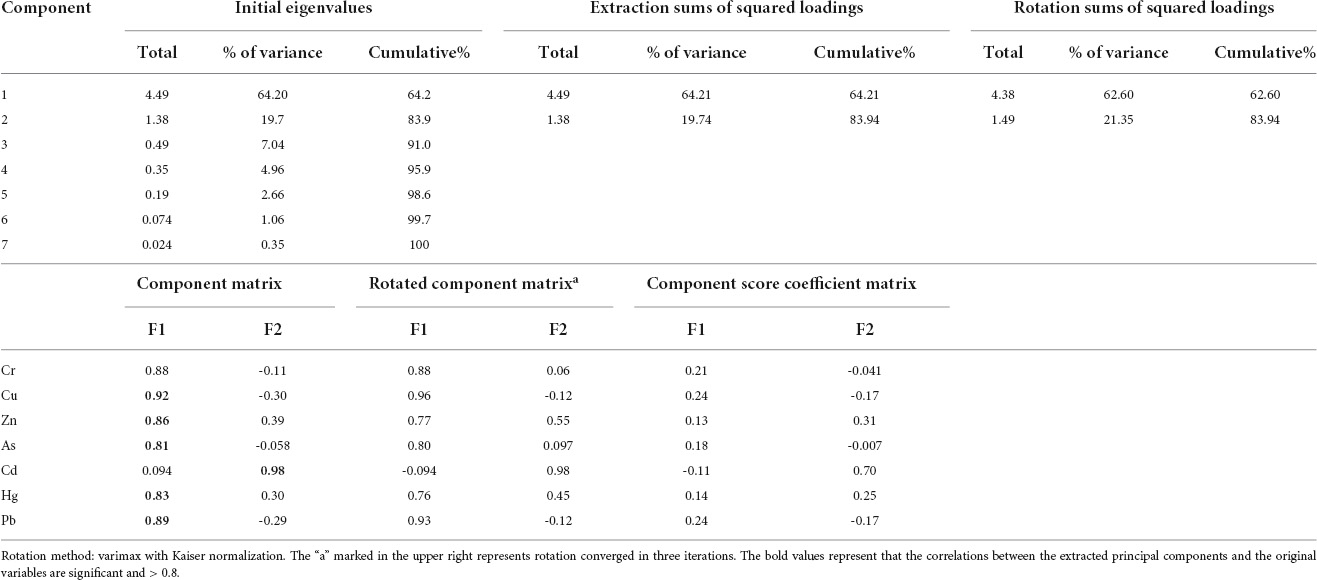
Table 6. Principal component analysis to distinguish the sources of possible pollutions with total variance explanation and component matrices of heavy metal concentrations in the sediment of Xingkai Lake and Xiaoxingkai Lake.
Based on the PCA result, the concentrations of As, Cd, Cr, Cu, Hg, Pb, and Zn could be divided into a two-component model, which accounted for 83.94% of all of the data variation. The initial component matrix (F1) showed that As, Cr, Cu, Hg, Pb, and Zn had strong positive loads and their values were greater than 0.80 with a cumulative contribution rate of 64.21%, suggesting these metals were possibly derived from similar sources. In the second component (F2, a contribution rate of 21.35%), Cd showed much higher values than other heavy metals (p < 0.05), indicating a notably different source for Cd compared with other metals.
Discussion
Spatial and temporal distribution of heavy metals
Consistent with a previous study in Taihu Lake (Rajeshkumar et al., 2018), the significant seasonal difference was observed in most of heavy metals, including As, Cd, Cr, and Hg, which were selected as the priority control heavy metals, due to their prevalently high concentrations in lake sediments (Xu et al., 2017). On the one hand, the distinct hydrodynamics in different seasons causes great difference in sediment resuspension process, thus transforming metal speciation from stable to labile fractions and changing the content of heavy metals in sediments (Huang et al., 2012; Tang et al., 2020). In fact, Xingkai Lake basin is freezing with weak water fluidity under the ice in January, whereas Xingkai Lake and Xiaoxingkai Lake showed strong hydrological connectivity with high water flow velocity in May, July, and September. On the other hand, consistent with Sun et al. (2019) that the highest mean concentration of total heavy metals occurred in the freezing period, resulting from the contribution of ice cover to the accumulation of chemical substances in sediments, such as anoxic condition and less bioturbation at the water-sediment interface (Jansen et al., 2021). Moreover, the NMDS result of heavy metal composition visibly distinguished January from other months, which further confirmed the accumulation characteristics of heavy metals in sediments in January.
As expected, heavy metals in Xingkai Lake showed significant difference compared with those in Xiaoxingkai Lake, with low similarity between two lakes. This may be caused by their different sources of heavy metals, Xingkai Lake is a transboundary lake receiving metal source from both China and Russia, whereas Xiaoxingkai Lake is separated from Xingkai Lake by a natural sandy ridge with main metal source coming from surrounding farmland. Based on the IDW spatial interpolation model, heavy metals showed noticeably different variations in eastern, central, and western regions in two lakes. Compared with the central and western regions, heavy metal concentrations in the eastern region were relatively lower, attributing to a large area of natural lacustrine marsh around the eastern region with respect to pollutant retention function compared to the great contribution of pollutant source from large area of farmland in the western region. Particularly, total heavy metal concentrations at a unique tourism site (XXKL) were significantly higher (one-way ANOVA, p < 0.05) than those in other sites in the Xingkai Lake basin, mainly due to heavy fuel oil burning in the ship emissions during navigation process (Wen et al., 2018).
Concentrations of As, Cr, and Zn were higher in lakes compared with marsh, dry farmland and paddy in the Xingkai Lake basin (Shi and Tian, 2020), indicating an enrichment effect of these three metals via surface runoff. In comparison, Cu showed lower concentrations in lakes, which may due to bio-utilization and complex physicochemical processes along the transmission pathway. Besides, Cd and Pb were relatively stable in different land use types in the Xingkai Lake basin.
The chemical forms and mobility of heavy metals are controlled by environmental variables, such as pH, redox potential and nutrients (Kang et al., 2017). We found positive correlations between most metals and TP, suggesting that the sediment nutrients had a great influence on the metal concentrations in the Xingkai Lake basin, which was consistent with studies in other lakes (Zhang H. et al., 2018), may due to high TP in the sediment in Xingkai Lake basin can reduce the mobility of metals by forming new materials such as low-solubility phosphates (Li et al., 2015). However, consistent with previous studies (Li et al., 2015), the concentrations of most metals showed weak correlations with OM and TN, indicating that the variations in OM and TN did not play significant roles in metal enrichment. Moreover, due to the dominance of silt with low OM content in Xingkai Lake and Xiaoxingkai Lake, heavy metals are less likely to be combined by coarse-grained particles in the sediment (Maslennikova et al., 2012; Kang et al., 2017).
Heavy metals pollution and potential risk assessment
Compared with most lacustrine sediments, such as Dianchi Lake in southwest, Taihu Lake in eastern, and Jingbo Lake in northeast China (Guo et al., 2015; Xu et al., 2017; Li et al., 2022), heavy metal concentrations in Xingkai Lake and Xiaoxingkai Lake were relatively lower, even lower than grade I level in Chinese soil quality standards. In recent years, the implementation of protection project along the lakeshore, for instance, surrounding wetlands restoration and sewage interception construction, can effectively intercept pollutants from the process of migration and reduce the risk of metal dispersal (Xu et al., 2017), and thus leading to considerable heavy metal removal from exogenous inflows in the Xingkai Lake basin. According to the GI and PERI analysis, sampling sites in the Xingkai Lake basin were at uncontaminated level and moderate ecological risk, except for XXKL with a notably high GI value of Cr and the highest RI value, indicating a considerable ecological risk in this tourism site because of human tourism behavior.
The overall heavy metal contamination level and ecological risk in Xiaoxingkai were higher than those in Xingkai Lake based on their GI and PERI values, mainly caused by the direct discharge of domestic sewage from nearby villages and agricultural wastewater from surrounding farmland to Xiaoxingkai Lake, and then flow into Xingkai Lake after reduction of heavy metal concentrations through adsorption, precipitation and other processes. Furthermore, the PERI value notably decreased with increasing lake surface area (Li et al., 2022), indicating a relatively low ecological risk level in Xingkai Lake which had a large area.
In terms of the temporal variations of GI and RI, the contamination level and ecological risk of heavy metals in both Xingkai Lake and Xiaoxingkai Lake in May and September were higher than those in other months, because the intensive agricultural activities and strong hydrological connectivity throughout the whole basin carrying a large amount of mud and sand in these two months that could elevate heavy metals via sediment deposition. Hydrological connectivity coupled with transport and transfer of heavy metals lead to distinctly spatial differences of heavy metal distribution. Previous study has found that Cd and Cr concentrations are higher in lakes connected to the Yangtze River, whereas concentrations of Cu, Zn, Pb, and As are higher in lakes disconnected with the Yangtze River, resulting in enriched metal pollutants in connected lakes, downstream and multi-branch (Luo et al., 2021). In addition to the direct transfer of heavy metals, hydrological connectivity also has indirect effects on heavy metals. For instance, hypoxic effect and high concentrations of nitrogen and phosphorus caused by hydrological connectivity can improve the bioavailability and accelerate the biogeochemical cycling of Cd and Zn (Liu M. et al., 2019; Niu et al., 2021). Particularly, Cd and Hg concentrations in the sediment were generally high with relatively high ecological risk levels due to agricultural exploration and farmland irrigation system construction in May and September, indicating an increase of the Cd and Hg input flux into water in agricultural seasons and may in turn leading a serious issue for human health by bioaccumulation and biomagnification via the food chain (Wang et al., 2016).
Source apportionment of heavy metals
High correlations between specific heavy metals in the sediment may reflect similar levels of contamination and/or release from the same sources of pollution (Yi et al., 2011). According to PCA result, the elements, As, Cr, Cu, Hg, Pb, and Zn were grouped together with strong positive loads indicating that the anthropogenic sources of these heavy metals were closely related in the sediment in the Xingkai Lake basin. By contrast, Cd was separated from other heavy metals, indicating a notably different source for Cd. Moreover, compared with previous study in Xingkai Lake (Guo et al., 2015), concentrations of Cr, Cu, Pb, and Zn synchronously decreased in sediments associated with low ecological risk level, but Cd concentration was remained at a high level associated with relatively high GI value, indicating a high potential eco-risk and high contaminated level of Cd now and in the future that strongly correlated with the long-term fertilizer application in this area (Shi and Tian, 2020), and further confirmed the distinct sources for Cd and other metals.
Heavy metals in sediments mainly come from natural sources (for example, soil erosion and rock weathering) and anthropogenic sources (for instance, industry, agriculture, transportation and domestic sewage) (Xu et al., 2017; Zhou et al., 2020). Metals of As, Cd, Cu, Pb, and Zn have been widely used in phosphate fertilizer, pesticides, or fungicides to control pests and diseases and promote agricultural production in China (Yu et al., 2021; Liu et al., 2022). Cr belongs to the iron group elements, which are easily combined with soil oxides and is closely related to soil parent materials (Sun et al., 2019). More than 80% of Hg originates from industrial activities such as coal burning and petrochemical production in China (Li et al., 2022). Cd is always considered as the marker of unreasonable agricultural management, and can be fixed and deposited into the sediment in the form of carbonate or hydroxide complex at an alkaline condition (Liu et al., 2022; Miranda et al., 2022). Meanwhile, tourism may increase heavy metal accumulation in sediments via heavy fuel oil burning during the ship navigation process, particularly in Cd (Wen et al., 2018). Notably, the study area is located in an economically undeveloped region characterized by tourism and agriculture but simple road networks. The industry in the Xingkai Lake basin is dominated by coal mining, which is mainly distributed in distant cities and their surroundings, contributing negligibly to sediment metal accumulation via atmospheric dust loading in the Xingkai Lake basin (Sun et al., 2018). In addition, the measured concentrations of Cd in this study were slightly higher than the background values in soil in Heilongjiang Province, probably attributing to the total emissions of Cd from anthropogenic sources. On the contrary, other metals were comparable to or lower than the background values in soil in Heilongjiang Province, which could be derived from natural sources. Therefore, the mixed sources of agricultural and shipping activities had a great influence on the accumulation of Cd, while As, Cr, Cu, Hg, Pb and Zn mainly came from natural sources.
Conclusion
Our study presented an explicit analysis of spatial distribution and temporal variation of heavy metals (As, Cd, Cr, Cu, Hg, Pb, and Zn) in sediments of two hydrologically connected lakes in the Xingkai Lake basin. Nutritional components of TN, TP, and OM were also measured to examine the influencing variables on heavy metals. Pollution and ecological risk level and sources of metals were determined based on multiple analysis methods. We found significant spatial and temporal variations of heavy metals in relation to human activities and hydrological connectivity in the basin. Xingkai Lake and Xiaoxingkai Lake showed different heavy metal compositions, and the distribution of each metal varied from eastern to western regions in two lakes. Overall, the contamination level and potential ecological risk of heavy metals in two lakes were low, but relatively higher in the small lake than those in the large lake based on the geo-accumulation index and potential ecological risk index, mainly caused by agricultural activities. In particular, the only tourism site showed relatively a higher pollution level than other sites, mainly due to heavy fuel oil burning in the ship emissions during the navigation process. Besides, the contamination level and potential ecological risk in May and September were higher than those in January and July. Most of metals showed strong relationships with TP in sediments, but not TN and OM. Of all metals, Cd exhibited a high potential eco-risk and high contaminated level, mainly coming from agricultural and shipping activities. By contrast, other metals with low concentrations mainly came from natural sources. These findings are in accordance with our hypotheses, and provide a clearer understanding of heavy metal variation and pollution in hydrologically connected lakes. Therefore, government regulators should make efforts to reduce the use of heavy metal products (e.g., phosphate fertilizer, pesticides, and fungicides) and regulate ship voyage to maintain lake ecosystem sustainability.
Data availability statement
The original contributions presented in this study are included in the article/supplementary material, further inquiries can be directed to the corresponding author.
Author contributions
YY and XZ designed the study. MJ, QW, XT, XD, ZW, and YY performed field investigation and preliminary sample treatment. MJ and QW contributed to quality control of samples testing. YY, XZ, XT, and MJ conducted data collection, analysis, interpretation, and drafted of the manuscript. All authors worked together to interpret results, provided feedback on the manuscript, and approved its final version.
Funding
This research was financially supported by the National Natural Science Foundation of China (Nos. 42101071 and 42171107); Strategic Priority Research Program of the Chinese Academy of Sciences (Nos. XDA28100304 and XDA28100103); Young Scientist Group Project of Northeast Institute of Geography and Agroecology, Chinese Academy of Sciences (2022QNXZ01); Jilin Province Education Department Science and Technology Research Project (No. JJKH20210289KJ); and Natural Science Foundation of Jilin Province (YDZJ202201ZYTS480).
Acknowledgments
The authors thank Yongzheng Lu for his help in the field work.
Conflict of interest
The authors declare that the research was conducted in the absence of any commercial or financial relationships that could be construed as a potential conflict of interest.
Publisher’s note
All claims expressed in this article are solely those of the authors and do not necessarily represent those of their affiliated organizations, or those of the publisher, the editors and the reviewers. Any product that may be evaluated in this article, or claim that may be made by its manufacturer, is not guaranteed or endorsed by the publisher.
References
Chen, Y., Jiang, X., Wang, Y., and Zhuang, D. (2018). Spatial characteristics of heavy metal pollution and the potential ecological risk of a typical mining area: a case study in China. Process Saf. Environ. Prot. 113, 204–219. doi: 10.1016/j.psep.2017.10.008
Coelho, C., Foret, C., Bazin, C., Leduc, L., Hammada, M., Inácio, M., and Bedell, J. P. (2018). Bioavailability and bioaccumulation of heavy metals of several soils and sediments (from industrialized urban areas) for Eisenia fetida. Sci. Total Environ. 635, 1317–1330. doi: 10.1016/j.scitotenv.2018.04.213
Feng, Y., Chenglin, L., and Bowen, W. (2019). Evaluation of heavy metal pollution in the sediment of Poyang Lake based on stochastic geo-accumulation model (SGM). Sci. Total Environ. 659, 1–6. doi: 10.1016/j.scitotenv.2018.12.311
Gao, Q., Li, Y., Cheng, Q., Yu, M., Hu, B., Wang, Z., et al. (2016). Analysis and assessment of the nutrients, biochemical indexes and heavy metals in the Three Gorges Reservoir, China, from 2008 to 2013. Water Res. 92, 262–274. doi: 10.1016/j.watres.2015.12.055
Guo, W., Huo, S., Xi, B., Zhang, J., and Wu, F. (2015). Heavy metal contamination in sediments from typical lakes in the five geographic regions of China: Distribution, bioavailability, and risk. Ecol. Engineer. 81, 243–255. doi: 10.1016/j.ecoleng.2015.04.047
Hakanson, L. (1980). An ecological risk index for aquatic pollution control a sedimentological approach. Water Res. 14, 975–1001. doi: 10.1016/0043-1354(80)90143-8
Haldar, K., Kujawa-Roeleveld, K., Dey, P., Bosu, S., Datta, D. K., and Rijnaarts, H. H. M. (2020). Spatio-temporal variations in chemical-physical water quality parameters influencing water reuse for irrigated agriculture in tropical urbanized deltas. Sci. Total Environ. 708:134559. doi: 10.1016/j.scitotenv.2019.134559
Han, R., Zhou, B., Huang, Y., Lu, X., Li, S., and Li, N. (2020). Bibliometric overview of research trends on heavy metal health risks and impacts in 1989-2018. J. Clean. Product. 276:123249. doi: 10.1016/j.jclepro.2020.123249
Huang, J., Ge, X., and Wang, D. (2012). Distribution of heavy metals in the water column, suspended particulate matters and the sediment under hydrodynamic conditions using an annular flume. J. Environ. Sci. 24, 2051–2059. doi: 10.1016/S1001-0742(11)61042-5
Huang, X. (2000). Survey, observation and analysis of lake ecology. Beijing: Standards press of China.
Jansen, J., MacIntyre, S., Barrett, D. C., Chin, Y. P., Cortés, A., Forrest, A. L., et al. (2021). Winter limnology: How do hydrodynamics and biogeochemistry shape ecosystems under ice? J. Geophys. Res. 126:e2020JG006237. doi: 10.1029/2020JG006237
Jin, X., and Zhai, P. (2006). Lake Xingkai/Khanka. Experience and Lessons Learned Brief. San Francisco, CA: Scopus.
Kang, X., Song, J., Yuan, H., Duan, L., Li, X., Li, N., et al. (2017). Speciation of heavy metals in different grain sizes of Jiaozhou Bay sediments: bioavailability, ecological risk assessment and source analysis on a centennial timescale. Ecotoxicol. Environ. Saf. 143, 296–306. doi: 10.1016/j.ecoenv.2017.05.036
Li, D., Yu, R., Chen, J., Leng, X., Zhao, D., Jia, H., et al. (2022). Ecological risk of heavy metals in lake sediments of China: a national-scale integrated analysis. J. Clean. Product. 334:130206. doi: 10.1016/j.jclepro.2021.130206
Li, J., Pu, L., Liao, Q., Zhu, M., Dai, X., Xu, Y., et al. (2015). How anthropogenic activities affect soil heavy metal concentration on a broad scale: a geochemistry survey in Yangtze River Delta, Eastern China. Environ. Earth Sci. 73, 1823–1835. doi: 10.1007/s12665-014-3536-7
Li, N., Tian, Y., Zhang, J., Zuo, W., Zhan, W., and Zhang, J. (2017). Heavy metal contamination status and source apportionment in sediments of Songhua River Harbin region, Northeast China. Environ. Sci. Pollut. Res. 24, 3214–3225. doi: 10.1007/s11356-016-7132-0
Liu, B., Dong, D., Hua, X., Dong, W., and Li, M. (2021). Spatial distribution and ecological risk assessment of heavy metals in surface sediment of Songhua River, Northeast China. Chin. Geogr. Sci. 31, 223–233. doi: 10.1007/s11769-021-1186-8
Liu, J. J., Diao, Z. H., Xu, X. R., and Xie, Q. (2019). Effects of dissolved oxygen, salinity, nitrogen and phosphorus on the release of heavy metals from coastal sediments. Sci. Total Environ. 666, 894–901. doi: 10.1016/j.scitotenv.2019.02.288
Liu, M., Chen, J., Sun, X., Hu, Z., and Fan, D. (2019). Accumulation and transformation of heavy metals in surface sediments from the Yangtze River estuary to the East China Sea shelf. Environ. Pollut. 245, 111–121. doi: 10.1016/j.envpol.2018.10.128
Liu, P., Wu, Q., Wang, X., Hu, W., Liu, X., Tian, K., et al. (2022). Spatiotemporal variation and sources of soil heavy metals along the lower reaches of Yangtze River, China. Chemosphere 291:132768. doi: 10.1016/j.chemosphere.2021.132768
Loska, K., Wiechuła, D., and Korus, I. (2004). Metal contamination of farming soils affected by industry. Environ. Int. 30, 159–165. doi: 10.1016/S0160-4120(03)00157-0
Luo, M., Yu, H., Liu, Q., Lan, W., Ye, Q., Niu, Y., et al. (2021). Effect of river-lake connectivity on heavy metal diffusion and source identification of heavy metals in the middle and lower reaches of the Yangtze River. J. Hazard. Mater. 416:125818. doi: 10.1016/j.jhazmat.2021.125818
Maslennikova, S., Larina, N., and Larin, S. (2012). The effect of sediment grain size on heavy metal content. Lakes Reserv. Ponds 6, 43–54.
Miranda, L. S., Ayoko, G. A., Egodawatta, P., and Goonetilleke, A. (2022). Adsorption-desorption behavior of heavy metals in aquatic environments: influence of sediment, water and metal ionic properties. J. Hazard. Mater. 421:126743. doi: 10.1016/j.jhazmat.2021.126743
Muller, G. (1969). Index of geoaccumulation in sediments of the Rhine River. Geojournal 2, 109–118. doi: 10.1016/j.envint.2020.106032
Niu, L., Cai, H., Jia, L., Luo, X., Tao, W., Dong, Y., et al. (2021). Metal pollution in the pearl river estuary and implications for estuary management: the influence of hydrological connectivity associated with estuarine mixing. Ecotoxicol. Environ. Saf. 225:112747. doi: 10.1016/j.ecoenv.2021.112747
Peng, M., Zhao, C., Ma, H., Yang, Z., Yang, K., Liu, F., et al. (2020). Heavy metal and Pb isotopic compositions of soil and maize from a major agricultural area in Northeast China: contamination assessment and source apportionment. J. Geochem. Explor. 208:106403. doi: 10.1016/j.gexplo.2019.106403
Piao, D., and Wang, F. (2011). Environmental conditions and the protection countermeasures for waters of Xingkai Lake (in Chinese). J. Lake Sci. 23, 196–202.
Rajeshkumar, S., Liu, Y., Zhang, X., Ravikumar, B., Bai, G., and Li, X. (2018). Studies on seasonal pollution of heavy metals in water, sediment, fish and oyster from the Meiliang Bay of Taihu Lake in China. Chemosphere 191, 626–638. doi: 10.1016/j.chemosphere.2017.10.078
Shepard, D. (1968). “A two-dimensional interpolation function for irregularly-spaced data,” in Proceedings of the 1968 23rd ACM National Conference, New York, NY, doi: 10.1145/800186.810616
Shi, L., and Tian, X. (2020). Pollution and ecological risk assessment of heavy metals in soils under different land use patterns in Xingkai Lake, Heilongjiang Province (in Chinese). Jiangsu Agric. Sci. 48, 282–286. doi: 10.15889/j.issn.1002-1302.2020.09.053
Song, K., Wang, Z., Li, L., Tedesco, L., Li, F., Jin, C., et al. (2012). Wetlands shrinkage, fragmentation and their links to agriculture in the Muleng-Xingkai Plain, China. J. Environ. Manage. 111, 120–132. doi: 10.1016/j.jenvman.2012.06.038
Song, L., Jian, J., Tan, D.-J., Xie, H.-B., Luo, Z.-F., and Gao, B. (2015). Estimate of heavy metals in soil and streams using combined geochemistry and field spectroscopy in Wan-sheng mining area, Chongqing, China. Int. J. Appl. Earth Observ. Geoinf. 34, 1–9. doi: 10.1016/j.jag.2014.06.013
Stauffer, B. A., Goes, J. I., McKee, K. T., do Rosario Gomes, H., and Stabeno, P. J. (2014). Comparison of spring-time phytoplankton community composition in two cold years from the western Gulf of Alaska into the southeastern Bering Sea. Deep Sea Res. II 109, 57–70. doi: 10.1016/j.dsr2.2014.03.007
Sun, C., Zhang, Z., Cao, H., Xu, M., and Xu, L. (2019). Concentrations, speciation, and ecological risk of heavy metals in the sediment of the Songhua River in an urban area with petrochemical industries. Chemosphere 219, 538–545. doi: 10.1016/j.chemosphere.2018.12.040
Sun, W., Zhang, E., Liu, E., Chang, J., and Shen, J. (2018). Linkage between Lake Xingkai sediment geochemistry and Asian summer monsoon since the last interglacial period. Palaeogeogr. Palaeoclimatol. Palaeoecol. 512, 71–79. doi: 10.1016/j.palaeo.2018.06.026
Suresh, G., Sutharsan, P., Ramasamy, V., and Venkatachalapathy, R. (2012). Assessment of spatial distribution and potential ecological risk of the heavy metals in relation to granulometric contents of Veeranam lake sediments, India. Ecotoxicol. Environ. Saf. 84, 117–124. doi: 10.1016/j.ecoenv.2012.06.027
Tang, C., Li, Y., He, C., and Acharya, K. (2020). Dynamic behavior of sediment resuspension and nutrients release in the shallow and wind-exposed Meiliang Bay of Lake Taihu. Sci. Total Environ. 708:135131. doi: 10.1016/j.scitotenv.2019.135131
Teng, J., Xia, S., Liu, Y., Cui, P., Chen, J., Si, W., et al. (2020). Differences of regulative flexibility between hydrological isolated and connected lakes in a large floodplain: insight from inundation dynamics and landscape heterogeneity. Water 12:991. doi: 10.3390/w12040991
Wang, F., Ouyang, W., Hao, F., Jiao, W., Shan, Y., and Lin, C. (2016). Role of freeze-thaw cycles and chlorpyrifos insecticide use on diffuse Cd loss and sediment accumulation. Sci. Rep. 6:27302. doi: 10.1038/srep27302
Wang, Y., Ouyang, W., Wang, A., Liu, L., Lin, C., and He, M. (2021). Synergetic loss of heavy metal and phosphorus: evidence from geochemical fraction and estuary sedimentation. J. Hazard. Mater. 416:125710. doi: 10.1016/j.jhazmat.2021.125710
Wen, J., Wang, X., Zhang, Y., Zhu, H., Chen, Q., Tian, Y., et al. (2018). PM2. 5 source profiles and relative heavy metal risk of ship emissions: source samples from diverse ships, engines, and navigation processes. Atmosph. Environ. 191, 55–63. doi: 10.1016/j.atmosenv.2018.07.038
Wu, H., Xu, C., Wang, J., Xiang, Y., Ren, M., Qie, H., et al. (2021). Health risk assessment based on source identification of heavy metals: a case study of Beiyun River, China. Ecotoxicol. Environ. Saf. 213:112046. doi: 10.1016/j.ecoenv.2021.112046
Xu, Y., Wu, Y., Han, J., and Li, P. (2017). The current status of heavy metal in lake sediments from China: pollution and ecological risk assessment. Ecol. Evol. 7, 5454–5466. doi: 10.1002/ece3.3124
Yi, Y., Yang, Z., and Zhang, S. (2011). Ecological risk assessment of heavy metals in sediment and human health risk assessment of heavy metals in fishes in the middle and lower reaches of the Yangtze River basin. Environ. Pollut. 159, 2575–2585. doi: 10.1016/j.envpol.2011.06.011
Yu, Z., Liu, E., Lin, Q., Zhang, E., Yang, F., Wei, C., et al. (2021). Comprehensive assessment of heavy metal pollution and ecological risk in lake sediment by combining total concentration and chemical partitioning. Environ. Pollut. 269:116212. doi: 10.1016/j.envpol.2020.116212
Yuan, X., Zhang, L., Li, J., Wang, C., and Ji, J. (2014). Sediment properties and heavy metal pollution assessment in the river, estuary and lake environments of a fluvial plain, China. Catena 119, 52–60. doi: 10.1016/j.catena.2014.03.008
Yuan, Y., Jiang, M., Liu, X., Yu, H., Otte, M. L., Ma, C., et al. (2018). Environmental variables influencing phytoplankton communities in hydrologically connected aquatic habitats in the Lake Xingkai basin. Ecol. Indic. 91, 1–12. doi: 10.1016/j.ecolind.2018.03.085
Yuan, Y., Jiang, M., Zhu, X., Yu, H., and Otte, M. L. (2021). Interactions between Fe and light strongly affect phytoplankton communities in a eutrophic lake. Ecol. Indic. 126:107664. doi: 10.1016/j.ecolind.2021.107664
Zhang, H., Huo, S., Yeager, K. M., Xi, B., Zhang, J., He, Z., et al. (2018). Accumulation of arsenic, mercury and heavy metals in lacustrine sediment in relation to eutrophication: impacts of sources and climate change. Ecol. Indic. 93, 771–780. doi: 10.1016/j.ecolind.2018.05.059
Zhang, P., Qin, C., Hong, X., Kang, G., Qin, M., Yang, D., et al. (2018). Risk assessment and source analysis of soil heavy metal pollution from lower reaches of Yellow River irrigation in China. Sci. Total Environ. 633, 1136–1147. doi: 10.1016/j.scitotenv.2018.03.228
Zhang, Y., Zhang, H., Zhang, Z., Liu, C., Sun, C., Zhang, W., et al. (2018). pH effect on heavy metal release from a polluted sediment. J. Chem. 2018:7597640. doi: 10.1155/2018/7597640
Zhou, Q., Yang, N., Li, Y., Ren, B., Ding, X., Bian, H., et al. (2020). Total concentrations and sources of heavy metal pollution in global river and lake water bodies from 1972 to 2017. Glob. Ecol. Conserv. 22:e00925. doi: 10.1016/j.gecco.2020.e00925
Keywords: heavy metals, ecological risk, Xingkai Lake, hydrological connectivity, agriculture
Citation: Jiang M, Wang Q, Tian X, Zhu X, Dong X, Wu Z and Yuan Y (2022) Spatiotemporal variation and ecological risk assessment of sediment heavy metals in two hydrologically connected lakes. Front. Ecol. Evol. 10:1005194. doi: 10.3389/fevo.2022.1005194
Received: 28 July 2022; Accepted: 26 August 2022;
Published: 14 September 2022.
Edited by:
He Yixin, Chengdu Institute of Biology (CAS), ChinaReviewed by:
Jiusheng Ren, East China University of Technology, ChinaChengxue Ma, Northeast Forestry University, China
Copyright © 2022 Jiang, Wang, Tian, Zhu, Dong, Wu and Yuan. This is an open-access article distributed under the terms of the Creative Commons Attribution License (CC BY). The use, distribution or reproduction in other forums is permitted, provided the original author(s) and the copyright owner(s) are credited and that the original publication in this journal is cited, in accordance with accepted academic practice. No use, distribution or reproduction is permitted which does not comply with these terms.
*Correspondence: Yuxiang Yuan, yuanyuxiang@iga.ac.cn
†These authors have contributed equally to this work and share first authorship