- Department of Biology, Colorado State University, Fort Collins, CO, United States
Cognitive variation is proposed to be the fundamental underlying factor that drives behavioral variation, yet it is still to be fully integrated with the observed variation at other phenotypic levels that has recently been unified under the common pace-of-life framework. This cognitive and the resulting behavioral diversity is especially significant in the context of a social group, the performance of which is a collective outcome of this diversity. In this review, we argue about the utility of classifying cognitive traits along a slow-fast continuum in the larger context of the pace-of-life framework. Using Tinbergen’s explanatory framework for different levels of analyses and drawing from the large body of knowledge about honeybee behavior, we discuss the observed interindividual variation in cognitive traits and slow-fast cognitive phenotypes from an adaptive, evolutionary, mechanistic and developmental perspective. We discuss the challenges in this endeavor and suggest possible next steps in terms of methodological, statistical and theoretical approaches to move the field forward for an integrative understanding of how slow-fast cognitive differences, by influencing collective behavior, impact social evolution.
Introduction
In the classic story of the tortoise and the hare, we learn about two distinctly different personalities and the lesson that speed does not always matter, and an individual can be as successful doing things slowly. Understanding questions regarding phenotypic variations such as why some animals are slow and others are fast is a fundamental question in biology that has a long history in terms of r and k selection and life history theory (MacArthur and Wilson, 1967; Pianka, 1970; Stearns, 1976). Such slow-fast differences in behavior, both between and within a species, have been modeled under the pace-of-life syndrome (POLS) framework (Ricklefs and Wikelski, 2002; Réale et al., 2007, 2010), which proposes a suite of contrasting phenotypic traits to characterize this slow-fast axis (Figure 1). In this framework, a slow pace-of-life, characterized by life history traits such as slow growth, delayed reproduction and high survival, and a fast pace-of-life, marked by fast growth, early reproduction and low survival, are functionally mediated by a large set of correlated physiological and behavioral traits.
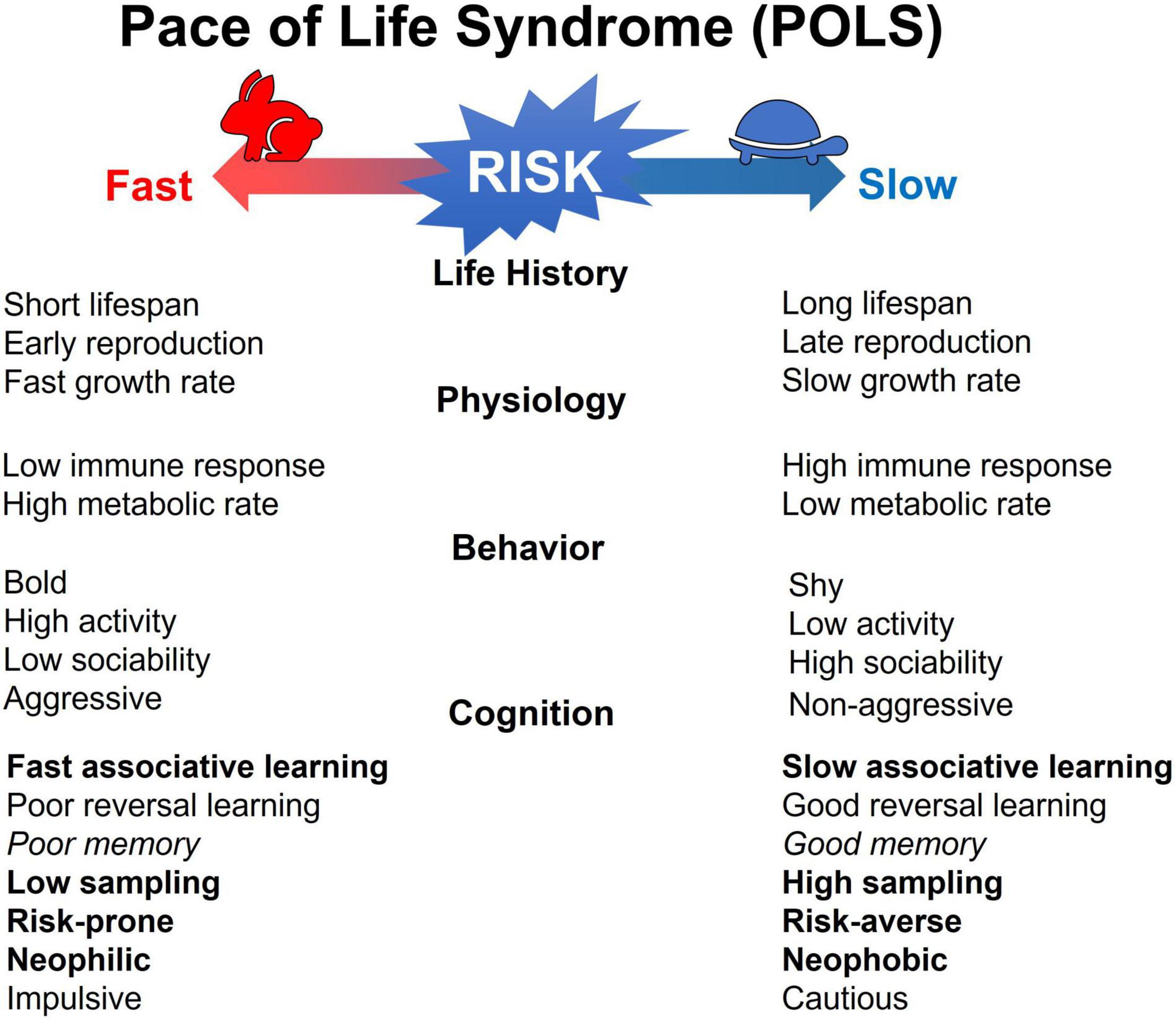
Figure 1. A summary of the predicted integration between the slow-fast pace-of-life axis at different phenotypic levels (life-history, physiology and behavior) and the slow-fast cognitive axis defined by the speed-accuracy trade-off, with examples of a few traits at each level (after Réale et al., 2010; Sih and Del Giudice, 2012). Specifically with respective to cognition, traits in bold letters are those for which the empirical data met theoretical predictions, those in italics did not meet predictions, and the remaining are those that were not tested (Tait and Naug, 2020).
Behavioral variation correlates to slow-fast life history differences through a risk-reward trade-off in which the higher expression of certain behaviors can bring more rewards, but at the cost of higher risk (Stamps, 2007; Wolf et al., 2007). Behaviorally, fast individuals are those who can engage in more risk-taking behaviors that allow them to gather resources more rapidly and thereby express the traits associated with a faster life history, compared to slow individuals. Since behavioral output is an outcome of underlying cognitive mechanisms, these slow-fast behavioral differences are proposed to be outcomes of a speed-accuracy trade-off in terms of decision-making (Chittka et al., 2009; Sih and Del Giudice, 2012; Jolles et al., 2020). Fast decisions made with little information are subject to higher inaccuracy but can result in greater and more immediate rewards while slower decisions made with more information are predicted to improve accuracy but come at the cost of immediacy. The cognitive axis resulting from this trade-off predicts fast individuals to broadly demonstrate rapid learning, lower sampling and poor retention of information, relative to slow individuals (Figure 1). Although a link between behavioral and cognitive variation has been demonstrated to some extent (Amy et al., 2012; Cole et al., 2012; Dougherty and Guillette, 2018; Tait and Naug, 2020), most of these studies are still limited in their scope and examining the covariation of multiple cognitive traits with other phenotypic traits remains a significant challenge.
This recent interest in a covariance between cognitive and behavioral variation is mostly focused at the between-individual level within a species (Carere and Locurto, 2011; Thornton et al., 2014; Griffin et al., 2015; Boogert et al., 2018). In contrast, the consequences of this individual level covariance structure have rarely been studied at the level of a group, even though the pace-of-life framework provides a convenient basis to understand this interplay (Dammhahn et al., 2018). Group living provides an opportunity to potentially relax the constraints placed upon the individual by the tight correlation among a set of traits that define the trade-offs in terms of risks or rewards and speed or accuracy (Figure 2). This extends the idea that at the collective level, any phenotypic variation allows the collective phenotype to show a greater range of response to a variable, complex and multivariate environment (Piersma and Drent, 2003; Woods, 2014). However, the final expression of the collective phenotype can be more complex than predicted because the different phenotypes in a social group may modulate the performance of each other (Webster and Ward, 2011; van den Bos et al., 2013). Negative frequency dependent processes can increase the performance of a phenotype when it is rare while positive frequency dependent processes can decrease the performance of a rare phenotype.
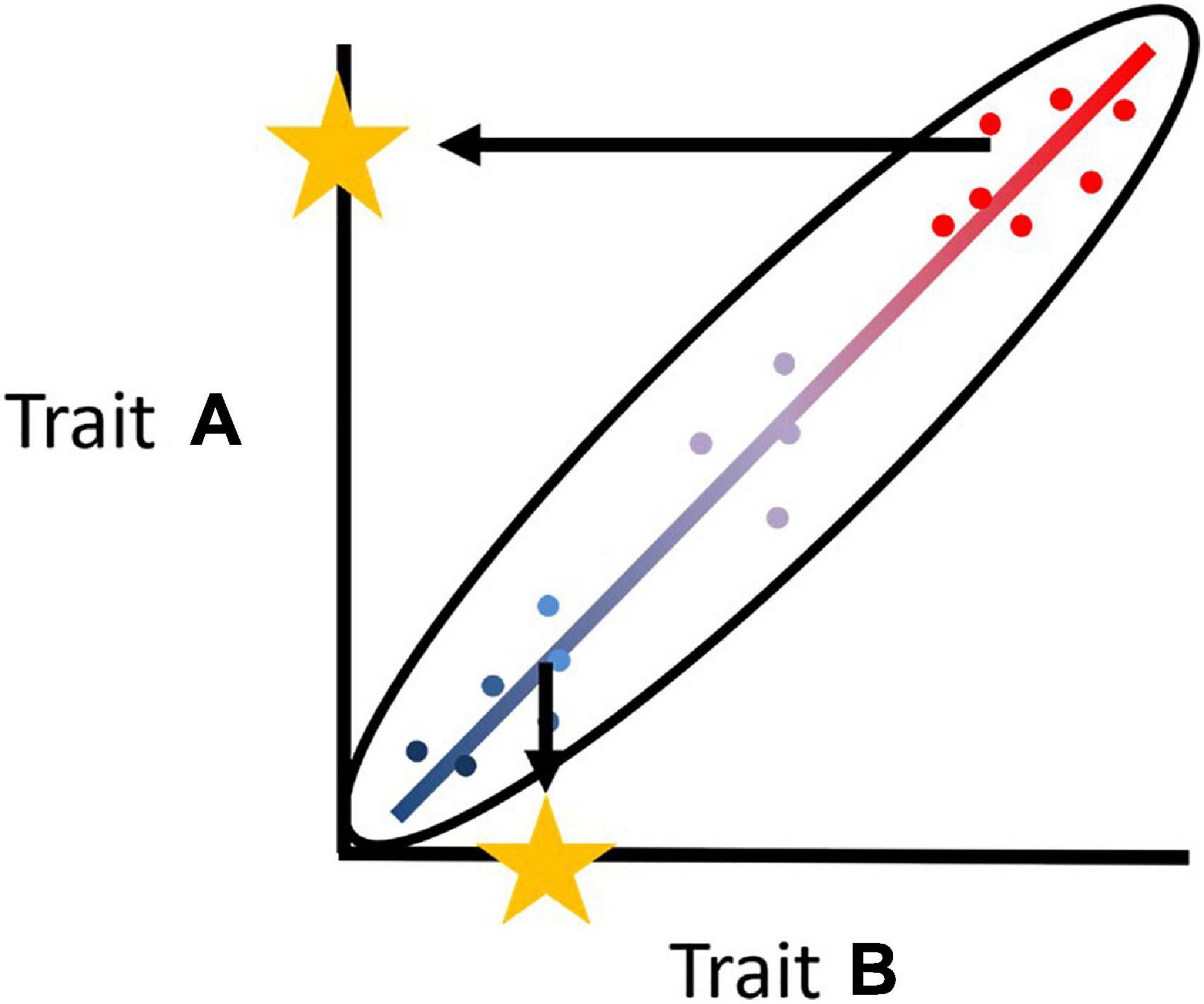
Figure 2. Hypothetical correlation between two traits, (A,B) at the individual level that leads to possible slow (blue) and fast (red) phenotypes. This can lead to an individual being constrained in its phenotypic expression, defined by its tightly associated trait values for (A,B). However, a group composed of both slow and fast phenotypes, by being able to express trait values at different points on the slow fast axis, is able to relax these constraints at the collective level.
For a comprehensive understanding of any biological question, it is important to take an integrative approach that encompasses both proximate (how?) and ultimate (why?) levels of analyses. In this review, we therefore use “Tinbergen’s four questions” approach (Tinbergen, 1963) to address cognitive variation at four different levels—functional value, evolution, causation and development. Using this background, we argue that social insects, especially honeybees, provide ideal model systems to pursue integrative studies that span these different levels of inquiries at different levels of biological organization, using the pace-of-life as the central framework to understand the importance of slow-fast differences in cognition in the social context. We review our current understanding regarding cognitive variation in honeybees and identify existing gaps in knowledge, offering suggestions regarding potential methodological and statistical approaches that can help close these gaps.
Interindividual Variation in Cognitive Traits and Cognitive Phenotypes in Social Insects
Social insect colonies are comprised of significant morphological, physiological and behavioral variation among individuals, differences which underlie the observed division of labor that is widely considered to be at the heart of their extraordinary ecological success (Oster and Wilson, 1978; Beshers and Fewell, 2001). Although variation in cognitive traits is less studied, one of the best examples of such interindividual variation that is known to be correlated across multiple phenotypic levels comes from the extensive study of low and high strains of pollen hoarding honeybees (Pankiw and Page, 2000; Page et al., 2012). The two strains differ in their sensorimotor and learning abilities such that the high strain bees have higher sensitivity to sugar and water, a higher performance on odor- and tactile based associative learning tasks as well as an earlier age of first foraging, higher levels of vitellogenin and ovary development, a suite of traits referred to as the foraging syndrome (Page et al., 1998, 2006). The strong association among several learning traits and their correlation with other behavioral and life history traits are linked through a common genetic architecture of overlapping quantitative trait loci (QTLs) that show broad epistatic and pleiotropic effects (Page et al., 2012). These QTLs are also associated with insulin-insulin like signaling (IIS) and target of rapamycin (TOR) pathways, which have broad effects in nutritional signaling and regulation of behavior across a wide range of taxa, indicating the fundamental integration of cognition with a variety of traits at different levels of the phenotype. These observations, however, bring up the important question about the nature of causal relationships among these multitude of traits and it has been shown that differences in learning ability are largely explained by differences in sucrose sensitivity—a physiological trait (Scheiner et al., 2005; Roussel et al., 2009). Interindividual variation in cognitive traits has also been documented in other bees (Spaethe et al., 2007; Raine and Chittka, 2008; Muller and Chittka, 2012; Klein et al., 2017) and it is important to ask if this variation is similarly correlated to differences at other phenotypic levels as what is observed in the honeybees.
Although simple associative learning, due to the relative ease with which it can be measured, is the most commonly used trait to describe the cognitive phenotype of an individual, it is somewhat limited in its scope and one has to go beyond it if we are to understand the functional relevance of cognitive variation (Giurfa, 2015). Honeybees do show variation in other learning traits such as reversal learning (Bhagavan et al., 1994; Carr-Markell and Robinson, 2014; Cook et al., 2019) and aversive learning (Junca et al., 2019), and these different types of learning measures are correlated at the individual level such that there is a negative relationship between associative and reversal learning, and between appetitive and aversive learning abilities. Apart from these learning traits, little is known about similar variation with respect to other cognitive traits other than some limited work showing interindividual variability in terms of risk-sensitivity (Mayack and Naug, 2011), sampling and novelty preference (Katz and Naug, 2015), all of which are connected to energetic state. Using this as a background, it was more recently shown that several of these cognitive traits covary in a manner that largely meets the predictions of the speed-accuracy trade-off and results in slow and fast cognitive phenotypes such that fast bees are described by high associative learning and high preferences for novelty and risk, compared to slow bees (Tait and Naug, 2020). These cognitive differences also translated to functional differences in behavior and life history traits—fast bees transitioning to a forager role at an earlier age than slow bees. These findings suggest that slow-fast cognitive phenotypes describe broad differences among individuals in a variety of cognitive traits and provide a window into how cognitive variation fits into the pace-of-life framework.
There are two distinct foraging phenotypes in honeybees—scouts that gather new information about the resource environment and recruits which use that information (Seeley, 1983; Biesmeijer and De Vries, 2001). These two phenotypes can therefore be considered functionally equivalent to producers and scroungers (Katz and Naug, 2016), which allows us to consider how different cognitive phenotypes might occupy distinct social roles in a broader ecological framework (Katsnelson et al., 2011). The ability of producers to acquire information through personal experience should be reflected in their high performance on an individual learning task while the ability of scroungers to do the same by observing conspecifics should be reflected in their high performance on a social learning task. Given that individual learning should require higher levels of sampling (Kurvers et al., 2010; Rosa et al., 2012), one would predict that scouts represent the slow cognitive phenotype which spends more time gathering information. While this seems to be substantiated by the slower learning seen in scouts (Cook et al., 2019), it stands in contrast with their observed preference for novelty (Liang et al., 2012), which aligns more with what is expected of the fast cognitive phenotype (Sih and Del Giudice, 2012). Such inconsistencies highlight the fact that the original predictions of the slow-fast cognitive axis might not be as universal, an issue that we feel is also partly related to the challenge of clearly defining the cognitive traits themselves, an issue which we discuss later in more detail. In addition, the scout/recruit behavioral axis might also be plastic over the foraging lifespan of an individual bee, subject to its developmental, social and resource environment, which can lead to inconsistencies in the cognitive traits measured in these two phenotypes.
Cognitive Diversity: Adaptive Value of Slow-Fast Cognitive Phenotypes in a Group
The historic and continued fascination with social insects is primarily to do with the fact that they are considered superorganisms due to their collective performance based on the tight integration among colony members (Wheeler, 1911; Wilson, 1971; Kennedy et al., 2017). This view of insect societies has been addressed in terms of several phenotypic traits (Lumsden, 1982; Southwick, 1983; Behmer, 2009; Gillooly et al., 2010) including cognition (Franks, 1989; Seeley, 1989; Couzin, 2009; Menzel, 2012; Feinerman and Korman, 2017). Phenotypic diversity among colony members (Jeanne, 1988), which allows flexibility and resilience to environmental heterogeneity and perturbations, promotes group productivity and fitness (Jones et al., 2004; Mattila and Seeley, 2007; Oldroyd and Fewell, 2007; Wray et al., 2011; Modlmeier et al., 2012) although there are studies which suggest that the effects of diversity could be more complex (Moritz and Page, 1999; Arathi and Spivak, 2001; Baer and Schmid-Hempel, 2001; Mugel and Naug, in press). Models of division of labor and task allocation addressing how interindividual behavioral variability affects group performance (Beshers and Fewell, 2001), have, however, included relatively little on the specific role of cognitive variation on collective performance. Even the recent interest in understanding the significance of interindividual variability within a social insect colony (Pinter-Wollman, 2012; Bengston and Jandt, 2014; Jandt et al., 2014; Jeanson and Weidenmuller, 2014; LeBoeuf and Grozinger, 2014; Jandt and Gordon, 2016) has not explicitly addressed the role of cognitive differences among individuals and its significance for social evolution.
The observed interindividual variation in cognitive traits at the colony level (Page et al., 2006; Muller and Chittka, 2012; Junca et al., 2014; Smith and Raine, 2014; Tait et al., 2019) can be described in terms of a speed-accuracy trade-off (Chittka et al., 2003). The functional consequences of this trade-off are, however, ambiguous—while one study suggested that fast and inaccurate foragers, which are more indiscriminate in their choice between rewarding and non-rewarding flowers, can collect resources at a higher rate (Burns, 2005), another showed that such foragers end up with a lower lifetime collection (Evans et al., 2017). Although the results of these two studies are not easily comparable given their different experimental designs, they suggest that slow and fast cognitive strategies might have different costs and benefits associated with them. Colonies that maintain a cognitively diverse workforce therefore might be able to show an overall higher efficiency in resource acquisition (Burns and Dyer, 2008).
The positive influence of cognitive diversity on collective foraging is most likely mediated by the resource landscape, which has a strong influence on foraging dynamics (Waddington et al., 1994; Steffan-Dewenter and Kuhn, 2003; Couvillon et al., 2015). Since slow and fast cognitive phenotypes differ with respect to how they gather and use information, a more challenging or scarce resource environment can enhance the value of producing new information, while a more rich or clumped resource environment can reduce its value and provide an advantage for scrounging. In a social foraging context, the collective performance of the group can therefore be viewed in the framework of a Genotype × Environment interaction, where the genotype specifies the relative frequency of each cognitive phenotype in the group and the environment refers to the spatial and temporal distribution of resources. Despite the long history of theoretical work on this topic (Caraco and Giraldea, 1991; Vickery et al., 1991; Luttbeg and Sih, 2010), actual empirical tests of how the performance of different cognitive strategies is influenced by the resource environment are extremely rare, largely because of their challenging nature (King et al., 2009).
Comparative Cognition: Evolution of Slow-Fast Cognitive Phenotypes
Our understanding of the functional relevance of cognitive variation can strongly benefit from studies at the interspecific level (Chittka et al., 2012; Rosati, 2017; Wenseleers and van Zweden, 2017). Although the initial studies that placed behavioral and life history traits along a slow-fast axis made use of interspecific comparisons (Promislow and Harvey, 1990; Wiersma et al., 2007), the same approach has not been used as much to understand similar variation in cognitive traits across species. Since interspecific differences in cognition represent adaptations to differences in ecological factors (Healy et al., 2009; Sheehan and Tibbetts, 2011; Cauchoix and Chaine, 2016), understanding such differences in terms of a slow-fast axis would be an important step toward identifying its evolutionary significance. In the context of a social group, we know little regarding whether the link between individual and collective cognition is modulated by ecological factors.
However, interspecific comparisons of cognitive traits can be confounded by the fact that any observed differences across species could be attributed to assays or experimental conditions that are not equally relevant for each species. One therefore needs to exercise good judgment in terms of the experimental design and the species that are chosen for such studies. It has also been pointed out that seemingly similar cognitive capacities might be mediated by entirely different neural mechanisms and therefore behavioral studies must be complemented with research at a proximate level (Chittka et al., 2012). Neuroecology, the comparative study of mechanisms that underlie cognitive capacity, has provided robust support for the adaptive nature of cognitive differences in social insects in terms of brain evolution (Kamhi et al., 2016; Godfrey and Gronenberg, 2019). Based on these findings that support the classic idea that social evolution is one of the strongest drivers of brain evolution (Dunbar, 1998; Dunbar and Shultz, 2007), social insects are particularly suited to understanding how cognitive trait evolution is related to sociality.
Although honeybees have served as a classic model of cognition research (Menzel, 2012; Giurfa, 2015), our extensive knowledge regarding their cognitive capacity is largely derived from studies with Apis mellifera, with little known about the cognitive traits of other honeybee species. Comparative studies of cognition across these different species offer an excellent, yet untapped, experimental opportunity that can give insights into the role of ecological factors on cognitive variation at both the individual and the collective levels. The two cavity nesting species, A. mellifera and A. cerana, have been described as “fast” due to their shorter lifespan, higher metabolic rate and faster behavioral “tempo” compared to the two open nesting species, A dorsata and A. florea, described as “slow” (Seeley, 1985; Dyer and Seeley, 1991). In fact, Seeley (1985) speculated about clusters of functionally related traits that could identify causal relationships between ecology and social organization and how such a goal could be realized with a comparative trait-oriented approach across the four species. The slow-fast phenotypic axis offers exactly that opportunity in terms of a unifying framework that can be used to measure a large set of traits at different phenotypic levels and systematically test if the slow-fast differences among these species also extend at the level of cognition and if the covariance among traits is shaped by the ecological differences among these species.
A comparative approach inspired by this framework was recently used to test if the observed slow-fast cognitive differences among A. mellifera individuals (Tait and Naug, 2020) are consistent across the other honeybee species and if there are slow-fast cognitive differences among these species that match differences in their behavior, life history and ecology (Tait et al., 2021). The results suggest some consistency in the traits that define the slow-fast cognitive axis within each of the four species—specifically, individuals which are fast learners also show higher preference for novelty compared to those who are slow. However, interspecific differences in cognitive traits did not correlate to slow-fast differences in life history and nesting ecology as it was A. florea (slow “tempo”) and A. cerana (fast “tempo”) which were found to cluster together as a distinct group, characterized by their lower associative learning and higher risk preference than A. dorsata (slow “tempo”) and A. mellifera (fast “tempo”) which formed a separate cluster. Instead, it was found that these interspecific cognitive differences correlate to differences in absolute brain size—A. dorsata and A. mellifera, the two species with higher associative learning also have significantly larger brains than A. cerana and A. florea (Gowda and Gronenberg, 2019; Tait et al., 2021). Although the analysis of this observed relationship is somewhat rudimentary, these results highlight the importance of integrating measurements from multiple phenotypic levels to understand the basis of cognitive differences across species. The two species with larger brains, A. dorsata and A. mellifera, also have both a larger colony size and a more complex foraging niche in terms of its spatiotemporal complexity, compared to A. florea and A. cerana (Seeley, 1985; Dyer and Seeley, 1991). It is therefore worth asking if the evolution of slow-fast cognitive traits is related to social and environmental complexity, which are widely recognized as important drivers of cognitive evolution (Roth and Pravosudov, 2009; Roth et al., 2010), which includes evidence from social insects (Farris and Schulmeister, 2011; Farris, 2016; Kamhi et al., 2016).
Energetics of Cognition: Mechanistic Basis of Slow-Fast Cognitive Phenotypes
If brain size is an important determinant of cognitive capacity (Chittka and Niven, 2009), it becomes important to understand the neural mechanisms that shape slow-fast differences in cognitive traits. There are substantial energetic costs associated with neural processing and differences in cognitive capacity are predicted to be fundamentally derived from variation in brain metabolic activity (Laughlin et al., 1998). This mechanistic connection between cognitive capacity and energy use allows us to test if slow-fast differences in cognitive traits are shaped by differences in metabolic rate at both the intra- and interspecific levels. Combined with the knowledge that behavioral traits such as aggression are also correlated to metabolic activity in the brain (Rittschof et al., 2018), this will help connect the slow-fast cognitive axis to the common energetic link that characterizes the broader pace-of-life axis (Careau et al., 2008; Mugel and Naug, 2020).
In honeybees, energetic availability can drive differences in associative learning (Jaumann et al., 2013), risk sensitivity (Mayack and Naug, 2011), exploration-exploitation tendency (Katz and Naug, 2015, 2016) and impulsivity (Mayack and Naug, 2015), each of which is part of the slow-fast cognitive axis. A growing body of work shows that differences in associative learning and memory are robustly related to metabolic activity in the brain, as measured with cytochrome oxidase (COX), a metabolic marker of neuronal activity (Déglise et al., 2003). The well-known link between cognitive differences and levels of various neurotransmitters (Mercer and Menzel, 1982; Giurfa, 2006; Cook et al., 2019) could also be reflective of such differences in brain metabolic activity since energy use is regulated through the same signaling pathways (Roeder, 2020). By combining measurements of variation in both whole-body metabolic rate and brain ATP capacity with performance on cognitive assays, one can test whether the slow-fast differences in cognitive phenotypes are fundamentally related to a difference in metabolic capacity.
A link between cognitive capacity and energy use suggests that the collective cognitive capacity of a social group would be fundamentally influenced by the variation in metabolic rate within the colony. However, such a relationship can be complicated by the fact that collective cognition is proposed as a mechanism for relaxing the energetic constraints on cognition at the individual level (Lihoreau et al., 2012; Feinerman and Traniello, 2016; Feinerman and Korman, 2017; Coto and Traniello, 2021). In social insects, increasing social complexity is correlated to a decrease in brain size at the individual level and it is argued that distributed cognition can allow for investment in functionally specialized brain regions, ultimately lowering brain metabolic costs (O’Donnell et al., 2015). In ants, socially complex species have larger brains but a lower energetic activity in the higher order processing centers such as mushroom bodies (Kamhi et al., 2016). These results suggest that individual energetic constraints related to learning and other slow-fast cognitive differences may not be reflected as easily in social insects and emphasizes the importance of identifying the mechanisms that link individual cognitive capacity to collective cognition.
Cognitive Plasticity: Developmental Analysis of Slow-Fast Cognitive Phenotypes
What adds to the difficulty of understanding and defining the cognitive capacity of an individual is its plasticity, subject to influences from both the current and the developmental environment (Thornton and Lukas, 2012; Buchanan et al., 2013; van den Bos et al., 2013; Davidson et al., 2018; Cauchoix et al., 2020). These include influences of the nutritional, physical and social components of the environment, which acting through various epigenetic modifications and signaling pathways, can shape brain development, function and neural plasticity (Murphy et al., 2014). Environmental variability plays a major role in the covariance among different traits (Sgrò and Hoffmann, 2004; Wright et al., 2019) and it is proposed that the predicted slow-fast trait correlations are more likely to be observed in unfavorable environments (Hämäläinen et al., 2021). Such developmental effects on the adult phenotype can be either plastic (permanent environment effects) or flexible in the short term (reversible plasticity). Developmental effects may be adaptive if they result in a phenotype that is better fitted to the environment the individual is likely to experience as an adult—the so called Predictive Adaptive Response (PAR) hypothesis, according to which a match between the developmental and the adult environments leads to positive effects and a mismatch leads to adverse effects on various phenotypic traits (Gluckman and Hanson, 2004). Despite the strong support for such developmental effects on various phenotypic traits, including some work on honeybees (Wang et al., 2016), studies focusing on the patterns of slow-fast cognitive traits in an environmental context are rare.
Developmental effects are likely to be particularly relevant in social insects because the age-based division of labor is a developmental process that is both plastic and is accompanied by several important changes in cognitive traits (Ben-Shahar et al., 2000; Cabirol et al., 2018). In honeybees, several studies show the influence of birth weight and early social experience on sucrose responsiveness and associative learning (Pankiw et al., 2004; Scheiner, 2012; Arenas et al., 2013; Mortensen and Ellis, 2018; Tsvetkov et al., 2019) but we lack the knowledge about similar effects on other cognitive traits. The use of social information can be shaped by resource unpredictability during development, pre-natal stress leading to copying behavior in adulthood and post-natal stress leading to the opposite effect (Boogert et al., 2013). Since social information use is one of the key parameters that defines the scout-recruit behavioral axis or the slow-fast cognitive axis, these results suggest promising research avenues to understand the inconsistencies that are sometimes seen in the expression of these cognitive phenotypes. Developmental effects on cognitive traits are especially relevant in the current context of the influence of anthropogenic changes and other stressors on behavior (Decourtye et al., 2005; Gómez-Moracho et al., 2017).
Challenges and Solutions: A Roadmap for Future Work
Methodological Approaches
One of the most challenging aspects of establishing a detailed and robust cognitive axis is the measurement of multiple cognitive traits in multiple contexts, made even more difficult in social insects due to the relatively short worker lifespan. Perhaps one of the most appealing aspects of using honeybees as a model system for the study of cognitive variation is the ability of the well-established laboratory-based Proboscis Extension Reflex (PER) assay to measure several cognitive traits in a large number of individuals with a high throughput. The PER assay consists of presenting a bee with an odor, a conditioned stimulus (CS), followed by a sucrose reward, an unconditioned stimulus (US), in a series of trials and measures learning ability as the number of conditioned responses (CR), instances when the bee extends its proboscis to the CS prior to the US delivery (Bitterman et al., 1983). While this assay is conventionally used for measuring various types of learning, including discrimination learning (Smith et al., 1991) and reversal learning (Chandra et al., 2000), it can also be adapted to measure a variety of more complex cognitive traits such as risk-sensitivity (Shafir et al., 1999; Mayack and Naug, 2011), sampling and novelty preference (Katz and Naug, 2015; Tait and Naug, 2020). These assays have also been adapted for use in the other honeybee species (Ali et al., 2021; Tait et al., 2021), an important consideration if we are to extend the comparative study of cognitive phenotypes in honeybees. The appetitive PER assay, and the closely similar, aversive Sting Extension Reflex (SER) assay (Vergoz et al., 2007), also allow us to control for differences in motivational state that might otherwise confound the measurement of cognitive traits in field-based assays.
Experimental evolution and artificial selection approaches can be powerful tools in uncovering how a phenotypic trait might be shaped by specific selection pressures. Studies in cognitive variation have, however, lagged in this regard probably due to the large number of traits that are required to define the cognitive axis, their plasticity and the likely small heritability component in these traits due to the complex genetic architecture of cognition. The honeybee, being the only social insect that has been successfully bred for specific traits, allows extraordinary opportunities to select and breed for specific phenotypic traits using instrumental insemination techniques. Using the heritable variation in associative learning (Brandes, 1988, 1991; Laloi and Pham-Delegue, 2010), genetic lines with differences in their associative learning or reversal learning ability have been bred (Brandes and Menzel, 1990; Bhagavan et al., 1994; Chandra et al., 2000; Ferguson et al., 2001). Such cognitive lines can allow us to rigorously test the nature of the covariance structure among different cognitive traits and partition it into genetic and environmental effects. These lines can enable us to test the functional influence of various cognitive traits at the individual level as well as allow the possibility of creating experimental mixes of different cognitive phenotypes to test how cognitive differences scale up from the individual to the collective level to influence group-level performance and life history (Cook et al., 2020).
Statistical Approaches
Cognitive phenotypes, defined as consistent individual differences in several cognitive traits, also pose a challenge in terms of statistical analysis. Since these phenotypes are described by multiple traits that covary with each other, the complexity of the multivariate cognitive trait space needs to be captured by data reduction techniques such as principal component analysis (PCA), which can be used to identify specific cognitive traits that explain the largest differences among different cognitive phenotypes (Keagy et al., 2011; Mazza et al., 2018; Tait and Naug, 2020). PCAs, used to study interindividual variation within a species, however, are not appropriate when comparing the variation between species because individuals of a species are more similar to each other than those from other species. A related technique, canonical variate analysis (CVA), which maximizes the separation of a priori defined groups, rather than the individuals within each of them, is more appropriate for such interspecific comparisons (Campbell and Atchley, 1981; Carter and Feeney, 2012; Tait et al., 2021). Such statistical non-independence arising from phylogenetic inertia (Harvey and Purvis, 1991; Sherry, 2006) could also be addressed by using a phylogenetically corrected analysis such as the phylogenetic generalized least squares (PGLS) method (Székely et al., 2013). With the measurement of multiple cognitive traits, it also becomes important to understand the causal relationships among these different traits and the use of Structural Equation Models (SEM) or path analysis can allow us to extract such relationships. Studies on cognitive phenotypes, which mostly rely on simple covariance analyses, need to see a wider adoption of these more sophisticated statistical approaches.
In terms of collective performance, groups are generally compared using statistical models that are focused on the parameters of mean or variance. Such analyses are, however, unable to offer any insights into the possible mechanisms that mediate the effects of phenotypic diversity. In this context, a rarely used technique based on the Price equation, which identifies the different mechanisms that underlie the effects of diversity more specifically, can be used to analyze the influence of diversity on performance (Loreau and Hector, 2001; Takahashi et al., 2018). In this approach, the effect of inter-individual variation or heterogeneity on group performance is quantified as a diversity effect, which is then further partitioned into a complementarity effect, the influence of interactions between different phenotypes, and a selection effect, the disproportionate influence of a phenotype. A recent study used this technique to explore the influence of metabolic diversity in honeybees to find that the effects of diversity on collective performance can be complex (Mugel and Naug, in press) and it remains an exciting prospect to use a similar approach in studies of cognitive diversity.
Modeling Approaches
The speed-accuracy trade-off, which is considered as the underlying basis for the slow-fast cognitive axis, is a classic paradigm that addresses how animals manage these two constraints at the same time in a manner that maximizes the benefit to cost ratio of a decision. Numerous models describing such decision-making processes show a parallel between individual and collective decision-making where populations of neurons or individuals accumulate evidence for alternative choices and a decision is made for a specific alternative once the population reaches a threshold for that alternative (Bogacz, 2007; Marshall et al., 2009; Pelé and Sueur, 2013). These decision-making models are therefore more fundamentally tied to models of optimal sampling and learning, which predict that an animal should sample more and therefore learn more slowly, but more accurately, if the cost of making a wrong decision is large or if the cost of waiting to make a decision is low, both of which in turn are tied to the variability in the environment (Stephens, 1987, 1989, 1991). Sampling and the statistical property of the central limit theorem (CLT), which posits that the estimate of the true mean (accuracy) improves with sample (group) size, is also what explains the improved performance of a collective unit as against an individual. However, it is important to note that this outcome of CLT is based on the assumption of a random sample, which in this context would refer to a random assortment of cognitive phenotypes. Using specific distributions to model cognitive heterogeneity within a group, it would be instructive to generate testable predictions about how diversity of slow-fast cognitive phenotypes would influence the sampling process and how that in turn would affect the collective cognitive performance of the group.
Since the basis of collective cognition lies in a group of heterogeneous individuals interacting together, it has frequency-dependent outcomes that can be modeled using a game-theoretic approach, which allows us to predict the performance of each morph based on its relative frequency and how that in turn shapes the performance of the entire group. While the inclination to learn can be strongly influenced by the frequency of learners and non-learners in a group, only a handful of studies has examined the evolution of learning or any other cognitive parameter and its consequence on collective performance in a game-theoretic context (Giraldeau, 1984; Dubois et al., 2010; Katsnelson et al., 2012; Aplin and Morand-Ferron, 2017). Modeling approaches like these can be productively combined with statistical approaches that can analyze the details of the diversity effects in empirical data as described above. The scarcity of studies in this framework probably stems from the fact that we largely lack the ability to create experimental groups with specific compositions of different cognitive phenotypes, an endeavor which can be accomplished by the ability to generate selection lines as discussed earlier.
Finally, we feel that a major, but mostly overlooked, problem in the field of animal personality and animal cognition in general, is a lack of coherent and consistent definitions of different traits. Overlapping definitions sometimes lead to the same traits being measured in different ways, which means that they are not statistically independent of each other and can lead to inconsistent patterns about how these traits covary. For example, many studies measure exploration, activity and neophilia as three independent “eco-cognitive behavioral traits,” but unless defined and assayed carefully, the magnitude of these three traits could interdependently follow from each other. A lower preference for novelty could also be expressed as a lower level of sampling, which in turn may also lead to poorer learning, a covariance structure that is not only non-independent but also one that does not exactly match both model predictions (Sih and Del Giudice, 2012) and empirical data (Tait and Naug, 2020) about slow-fast cognitive phenotypes. Similarly, “reaction to a novel object” is frequently used as a definition of exploration, but it is also used as a measure for boldness even though the latter might be more specifically to do with an individual’s reaction to a risky situation. Risk itself is defined in terms of tendency of an individual to expose itself to a predator (Ferrari, 2014) or a preference for variability (Tait and Naug, 2020; Tait et al., 2021). The biological mechanisms driving these diverse behaviors could be very different and might lead to very different predictions about how they might co-vary with each other. This problematic issue is partly a consequence of the fact that cognitive processes are not directly measurable and can only be inferred by assessing a change in behavior (Barron et al., 2015; Griffin et al., 2015; Mazza et al., 2018). In this setting, process-based models can be informative about how these traits might be linked to each other, allowing us to see if these numerous traits are outcomes of a single fundamental cognitive process, such as sampling, or if they can vary independently of each other.
These obfuscations about how different cognitive traits are defined and measured point to a larger problem of how a set of cognitive traits is predicted to covary with each other to define different cognitive phenotypes. Models of the speed-accuracy trade-off, which is used as the major framework to conceptualize decision-making and the existence of slow but accurate and fast but inaccurate cognitive phenotypes (Sih and Del Giudice, 2012), have not considered broader and influential ideas regarding trade-offs. According to life history theory, trade-offs are a combined outcome of differences in both resource acquisition and allocation such that negative correlations between traits are produced only when individuals vary mainly in their allocation while positive correlations between the same traits can be seen when individuals vary mainly in their acquisition (Van Noordwijk and De Jong, 1986; Reznick et al., 2000). Since information can be considered as a resource, the same framework also applies in the context of decision making (Figure 3). Therefore, if individuals vary mainly in their ability to acquire information, as it is likely to be if it is correlated to traits such as metabolic rate and energetic capacity as might be expected from the pace-of-life hypothesis, it can produce a positive correlation between speed and accuracy, which might explain the seemingly contradictory absence of a trade-off between speed and accuracy during learning in some studies (Raine and Chittka, 2012; Mamuneas et al., 2015; Chang et al., 2017). Only when individuals similar in their information acquisition ability vary in how they allocate this information, should one see the more commonly expected trade-off between speed and accuracy. Since differences in acquisition are more likely to be manifested in some environments than others (Reznick et al., 2000), it means that the expression of the speed-accuracy tradeoff could be restricted to specific types of information environments. This also implies that the nature of covariation among different cognitive traits could be driven by Gene x Environment interactions, leading to possible polymorphisms in terms of different suites of covarying cognitive traits.
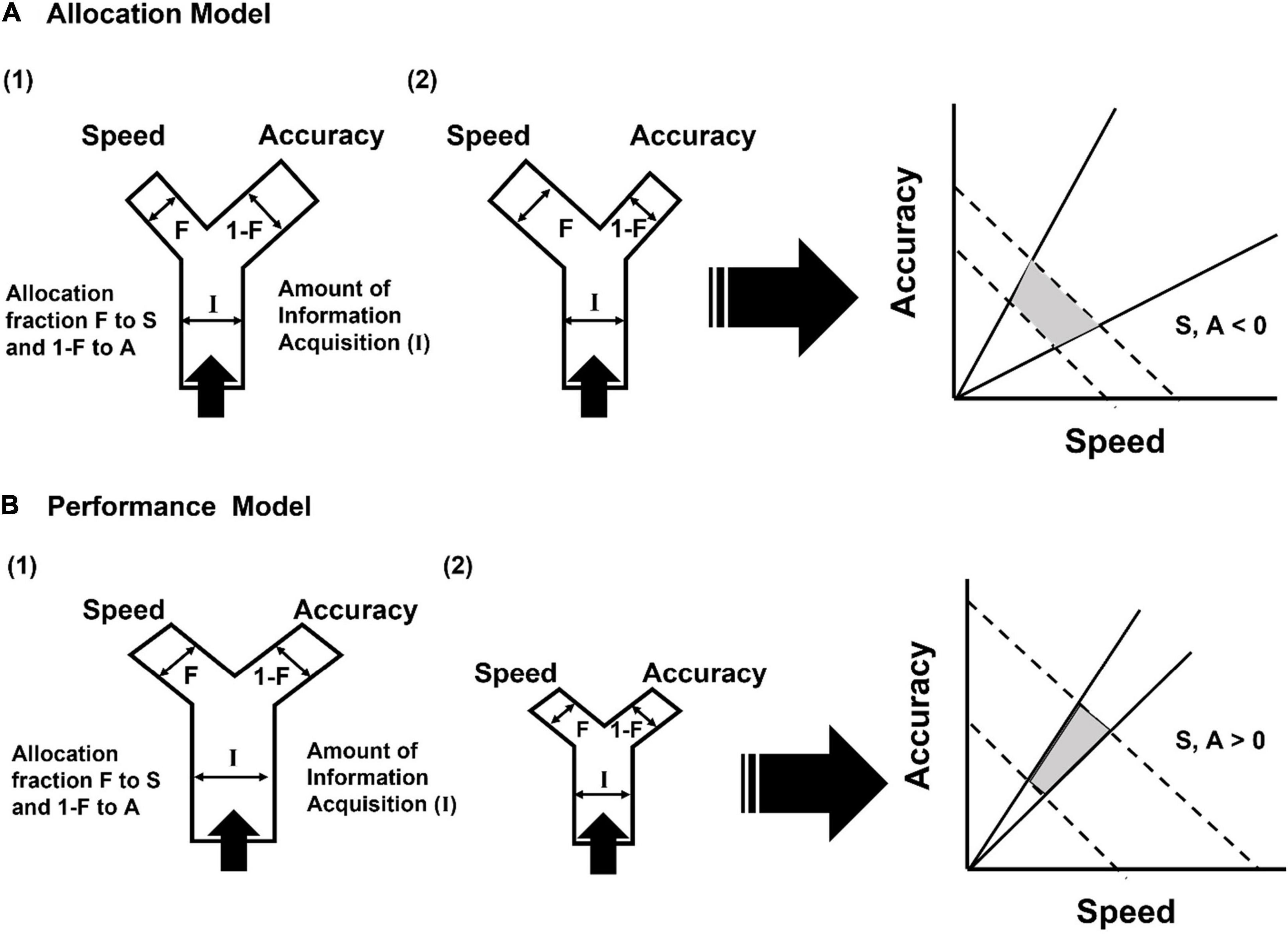
Figure 3. Possible alternative Y models of information (I) acquisition and its allocation to speed (S) and accuracy (A) in terms of either (A) an allocation model in which there is low inter-individual variation in information acquisition but high variation in its allocation, resulting in a scenario where (1) individuals invest more in accuracy at the expense of speed, or (2) vice versa, resulting in a negative relationship between speed and accuracy, or (B) a performance model in which there is high inter-individual variation in information acquisition but low variation in its allocation, resulting in a scenario where (1) individuals have a greater total amount of information that can be allocated to speed and accuracy, or (2) individuals have a lower total amount of information that can be allocated to speed and accuracy, resulting in a positive relationship between speed and accuracy.
Conclusion
Collective cognition has long been a topic of major interest to biologists and information scientists and a large body of both theoretical and empirical work has firmly established that groups can generally acquire and process information more efficiently and accurately than it is possible for single individuals. These findings about collective cognition closely parallel what is seen for collective traits at other phenotypic levels such as behavior and physiology so that it is now an established fact that collective action generally results in more robust outputs that are more stable to perturbations. Given this background, we feel that it is time now to move beyond this and take the next major step toward integrating these findings to a broader theoretical framework that can connect cognition to other levels of phenotypic variation at multiple levels of biological organization. The pace-of-life framework, which aims to place suites of phenotypic traits including cognition on a common slow-fast axis, seems particularly suited to this enterprise, allowing us to understand both the mechanistic and functional integration across these traits, which is important if we are to understand the role of cognition in collective behavior and social evolution. Applying the framework at the group level allows us to understand how the constraints posed upon the individual by the covariance among different cognitive traits might be relaxed by collective action. Social insects, especially honeybees, due to their wide-ranging experimental amenability, provide ideal model systems to apply this framework in testing how the slow-fast cognitive composition of a group shapes the emergent collective cognitive phenotype to influence colony behavior and life history.
Author Contributions
DN and CT conceived and wrote the manuscript together. Both authors contributed to the article and approved the submitted version.
Conflict of Interest
The authors declare that the research was conducted in the absence of any commercial or financial relationships that could be construed as a potential conflict of interest.
Publisher’s Note
All claims expressed in this article are solely those of the authors and do not necessarily represent those of their affiliated organizations, or those of the publisher, the editors and the reviewers. Any product that may be evaluated in this article, or claim that may be made by its manufacturer, is not guaranteed or endorsed by the publisher.
Acknowledgments
We would like to thank the funding from National Science Foundation, United States and Foundation for Food and Agricultural Research, United States for supporting the research and the effort working on the ideas expressed here. We also thank Stephen Mugel, who was a critical element in the vigorous discussions that generated some of these ideas, and the referees whose comments made a significant contribution to the final version of this manuscript.
References
Ali, H., Iqbal, J., Raweh, H. S., and Alqarni, A. S. (2021). Proboscis behavioral response of four honey bee Apis species towards different concentrations of sucrose, glucose, and fructose. Saudi J. Biol. Sci. 28, 3275–3283. doi: 10.1016/j.sjbs.2021.02.069
Amy, M., Van Oers, K., and Naguib, M. (2012). Worms under cover: relationships between performance in learning tasks and personality in great tits (Parus major). Anim. Cogn. 15, 763–770. doi: 10.1007/s10071-012-0500-3
Aplin, L. M., and Morand-Ferron, J. (2017). Stable producer–scrounger dynamics in wild birds: sociability and learning speed covary with scrounging behaviour. Proc. R. Soc. B 284:20162872. doi: 10.1098/rspb.2016.2872
Arathi, H. S., and Spivak, M. (2001). Influence of colony genotypic composition on the performance of hygienic behaviour in the honeybee, Apis mellifera L. Anim. Behav. 62, 57–66. doi: 10.1006/anbe.2000.1731
Arenas, A., Ramírez, G., Balbuena, M., and Farina, W. (2013). Behavioral and neural plasticity caused by early social experiences: the case of the honeybee. Front. Physiol. 4:41. doi: 10.3389/fphys.2013.00041
Baer, B., and Schmid-Hempel, P. (2001). Unexpected consequences of polyandry for parasitism and fitness in the bumblebee, Bombus terrestris. Evolution 55, 1639–1643. doi: 10.1111/j.0014-3820.2001.tb00683.x
Barron, A. B., Hebets, E. A., Cleland, T. A., Fitzpatrick, C. L., Hauber, M. E., and Stevens, J. R. (2015). Embracing multiple definitions of learning. Trends Neurosci. 38, 405–407. doi: 10.1016/j.tins.2015.04.008
Behmer, S. T. (2009). Animal behaviour: feeding the superorganism. Curr. Biol. 19, R366–R368. doi: 10.1016/j.cub.2009.03.033
Bengston, S. E., and Jandt, J. M. (2014). The development of collective personality: the ontogenetic drivers of behavioral variation across groups. Front. Ecol. Evol. 2:81. doi: 10.3389/fevo.2014.00081
Ben-Shahar, Y., Thompson, C. K., Hartz, S. M., Smith, B. H., and Robinson, G. E. (2000). Differences in performance on a reversal learning test and division of labor in honey bee colonies. Anim. Cogn. 3, 119–125.
Beshers, S. N., and Fewell, J. H. (2001). Models of division of labor in social insects. Annu. Rev. Entomol. 46, 413–440. doi: 10.1146/annurev.ento.46.1.413
Bhagavan, S., Benatar, S., Cobey, S., and Smith, B. H. (1994). Effect of genotype but not of age or caste on olfactory learning performance in the honey bee, Apis mellifera. Anim. Behav. 48, 1357–1369.
Biesmeijer, J. C., and De Vries, H. (2001). Exploration and exploitation of food sources by social insect colonies: a revision of the scout-recruit concept. Behav. Ecol. Sociobiol. 49, 89–99.
Bitterman, M. E., Menzel, R., Fietz, A., and Schäfer, S. (1983). Classical conditioning of proboscis extension in honeybees (Apis mellifera). J. Comp. Psychol. 97, 107–119. doi: 10.1037/0735-7036.97.2.107
Bogacz, R. (2007). Optimal decision-making theories: linking neurobiology with behaviour. Trends Cogn. Sci. 11, 118–125. doi: 10.1016/j.tics.2006.12.006
Boogert, N. J., Madden, J. R., Morand-Ferron, J., and Thornton, A. (2018). Measuring and understanding individual differences in cognition. Philos. Trans. R. Soc. B 373:20170280. doi: 10.1098/rstb.2017.0280
Boogert, N. J., Zimmer, C., and Spencer, K. A. (2013). Pre- and post-natal stress have opposing effects on social information use. Biol. Lett. 9:20121088. doi: 10.1098/rsbl.2012.1088
Brandes, C. (1988). Estimation of heritability of learning behavior in honeybees (Apis mellifera capensis). Behav. Genet. 18, 119–132. doi: 10.1007/BF01067081
Brandes, C. (1991). Genetic differences in learning behavior in honeybees (Apis mellifera capensis). Behav. Genet. 21, 271–294. doi: 10.1007/BF01065820
Brandes, C., and Menzel, R. (1990). Common mechanisms in proboscis extension conditioning and visual learning revealed by genetic selection in honeybees (Apis mellifera capensis). J. Comp. Physiol. A 166, 545–552.
Buchanan, K. L., Grindstaff, J. L., and Pravosudov, V. V. (2013). Condition dependence, developmental plasticity, and cognition: implications for ecology and evolution. Trends Ecol. Evol. 28, 290–296. doi: 10.1016/j.tree.2013.02.004
Burns, J. G. (2005). Impulsive bees forage better: the advantage of quick, sometimes inaccurate foraging decisions. Anim. Behav. 70, e1–e5.
Burns, J. G., and Dyer, A. G. (2008). Diversity of speed-accuracy strategies benefits social insects. Curr. Biol. 18, R953–R954. doi: 10.1016/j.cub.2008.08.028
Cabirol, A., Cope, A. J., Barron, A. B., and Devaud, J.-M. (2018). Relationship between brain plasticity, learning and foraging performance in honey bees. PLoS One 13:e0196749. doi: 10.1371/journal.pone.0196749
Campbell, N. A., and Atchley, W. R. (1981). The geometry of canonical variate analysis. Syst. Zool. 30, 268–280. doi: 10.1093/sysbio/30.3.268
Caraco, T., and Giraldea, L.-A. (1991). Social foraging: producing and scrounging in a stochastic environment. J. Theor. Biol. 153, 559–583. doi: 10.1016/s0022-5193(05)80156-0
Careau, V., Thomas, D., Humphries, M. M., and Réale, D. (2008). Energy metabolism and animal personality. Oikos 117, 641–653. doi: 10.1016/j.physbeh.2011.04.017
Carere, C., and Locurto, C. (2011). Interaction between animal personality and animal cognition. Curr. Zool. 57, 491–498.
Carr-Markell, M. K., and Robinson, G. E. (2014). Comparing reversal-learning abilities, sucrose responsiveness, and foraging experience between scout and non-scout honey bee (Apis mellifera) foragers. J. Insect Behav. 27, 736–752. doi: 10.1007/s10905-014-9465-1
Carter, A. J., and Feeney, W. E. (2012). Taking a comparative approach: analysing personality as a multivariate behavioural response across species. PLoS One 7:e42440. doi: 10.1371/journal.pone.0042440
Cauchoix, M., and Chaine, A. S. (2016). How can we study the evolution of animal minds? Front. Psychol. 7:358. doi: 10.3389/fpsyg.2016.00358
Cauchoix, M., Chaine, A. S., and Barragan-Jason, G. (2020). Cognition in context: plasticity in cognitive performance in response to ongoing environmental variables. Front. Ecol. Evol. 8:106. doi: 10.3389/fevo.2020.00106
Chandra, S. B. C., Hosler, J. S., and Smith, B. H. (2000). Heritable variation for latent inhibition and its correlation with reversal learning in honeybees (Apis mellifera). J. Comp. Psychol. 114, 86–97. doi: 10.1037/0735-7036.114.1.86
Chang, C.-C., Ng, P. J., and Li, D. (2017). Aggressive jumping spiders make quicker decisions for preferred prey but not at the cost of accuracy. Behav. Ecol. 28, 479–484.
Chittka, L., and Niven, J. (2009). Are bigger brains better? Curr. Biol. 19, R995–R1008. doi: 10.1016/j.cub.2009.08.023
Chittka, L., Dyer, A. G., Bock, F., and Dornhaus, A. (2003). Bees trade off foraging speed for accuracy. Nature 424:388. doi: 10.1038/424388a
Chittka, L., Rossiter, S. J., Skorupski, P., and Fernando, C. (2012). What is comparable in comparative cognition? Philos. Trans. R. Soc. B 367, 2677–2685. doi: 10.1098/rstb.2012.0215
Chittka, L., Skorupski, P., and Raine, N. E. (2009). Speed–accuracy tradeoffs in animal decision making. Trends Ecol. Evol. 24, 400–407. doi: 10.1016/j.tree.2009.02.010
Cole, E., Morand-Ferron, J., Hinks, A., and Quinn, J. (2012). Cognitive ability influences reproductive life history variation in the wild. Curr. Biol. 22, 1808–1812. doi: 10.1016/j.cub.2012.07.051
Cook, C. N., Lemanski, N. J., Mosqueiro, T., Ozturk, C., Gadau, J., Pinter-Wollman, N., et al. (2020). Individual learning phenotypes drive collective behavior. Proc. Natl. Acad. Sci. U.S.A. 117, 17949–17956. doi: 10.1073/pnas.1920554117
Cook, C. N., Mosqueiro, T., Brent, C. S., Ozturk, C., Gadau, J., Pinter-Wollman, N., et al. (2019). Individual differences in learning and biogenic amine levels influence the behavioural division between foraging honeybee scouts and recruits. J. Anim. Ecol. 88, 236–246. doi: 10.1111/1365-2656.12911
Coto, Z. N., and Traniello, J. F. A. (2021). Brain size, metabolism, and social evolution. Front. Physiol. 12:612865. doi: 10.3389/fphys.2021.612865
Couvillon, M. J., Riddell Pearce, F. C., Accleton, C., Fensome, K. A., Quah, S. K. L., Taylor, E. L., et al. (2015). Honey bee foraging distance depends on month and forage type. Apidologie 46, 61–70. doi: 10.1007/s13592-014-0302-5
Couzin, I. D. (2009). Collective cognition in animal groups. Trends Cogn. Sci. 13, 36–43. doi: 10.1016/j.tics.2008.10.002
Dammhahn, M., Dingemanse, N. J., Niemelä, P. T., and Réale, D. (2018). Pace-of-life syndromes: a framework for the adaptive integration of behaviour, physiology and life history. Behav. Ecol. Sociobiol. 72:62. doi: 10.1111/ele.13187
Davidson, G. L., Cooke, A. C., Johnson, C. N., and Quinn, J. L. (2018). The gut microbiome as a driver of individual variation in cognition and functional behaviour. Philos. Trans. R. Soc. B 373:20170286. doi: 10.1098/rstb.2017.0286
Decourtye, A., Devillers, J., Genecque, E., Menach, K. L., Budzinski, H., Cluzeau, S., et al. (2005). Comparative sublethal toxicity of nine pesticides on olfactory learning performances of the honeybee Apis mellifera. Arch. Environ. Contam. Toxicol. 48, 242–250. doi: 10.1007/s00244-003-0262-7
Déglise, P., Dacher, M., Dion, E., Gauthier, M., and Armengaud, C. (2003). Regional brain variations of cytochrome oxidase staining during olfactory learning in the honeybee (Apis mellifera). Behav. Neurosci. 117, 540–547. doi: 10.1037/0735-7044.117.3.540
Dougherty, L. R., and Guillette, L. M. (2018). Linking personality and cognition: a meta-analysis. Philos. Trans. R. Soc. B 373:20170282. doi: 10.1098/rstb.2017.0282
Dubois, F., Morand-Ferron, J., and Giraldeau, L.-A. (2010). Learning in a game context: strategy choice by some keeps learning from evolving in others. Proc. R. Soc. B 277, 3609–3616. doi: 10.1098/rspb.2010.0857
Dyer, F. C., and Seeley, T. D. (1991). Nesting behavior and the evolution of worker tempo in four honey bee species. Ecology 72, 156–170. doi: 10.2307/1938911
Evans, L. J., Smith, K. E., and Raine, N. E. (2017). Fast learning in free-foraging bumble bees is negatively correlated with lifetime resource collection. Sci. Rep. 7:496. doi: 10.1038/s41598-017-00389-0
Farris, S. M. (2016). Insect societies and the social brain. Curr. Opin. Insect Sci. 15, 1–8. doi: 10.1016/j.cois.2016.01.010
Farris, S. M., and Schulmeister, S. (2011). Parasitoidism, not sociality, is associated with the evolution of elaborate mushroom bodies in the brains of hymenopteran insects. Proc. R. Soc. B 278, 940–951. doi: 10.1098/rspb.2010.2161
Feinerman, O., and Korman, A. (2017). Individual versus collective cognition in social insects. J. Exp. Biol. 220, 73–82. doi: 10.1242/jeb.143891
Feinerman, O., and Traniello, J. F. A. (2016). Social complexity, diet, and brain evolution: modeling the effects of colony size, worker size, brain size, and foraging behavior on colony fitness in ants. Behav. Ecol. Sociobiol. 70, 1063–1074. doi: 10.1007/s00265-015-2035-5
Ferguson, H. J., Cobey, S., and Smith, B. H. (2001). Sensitivity to a change in reward is heritable in the honeybee, Apis mellifera. Anim. Behav. 61, 527–534.
Ferrari, M. C. O. (2014). Short-term environmental variation in predation risk leads to differential performance in predation-related cognitive function. Anim. Behav. 95, 9–14. doi: 10.1016/j.anbehav.2014.06.001
Gillooly, J. F., Hou, C., and Kaspari, M. (2010). Eusocial insects as superorganisms. Commun. Integr. Biol. 3, 360–362. doi: 10.4161/cib.3.4.11887
Giraldeau, L.-A. (1984). Group foraging: the skill pool effect and frequency-dependent learning. Am. Nat. 124, 72–79. doi: 10.1086/284252
Giurfa, M. (2006). Associative learning: the instructive function of biogenic amines. Curr. Biol. 16, R892–R895. doi: 10.1016/j.cub.2006.09.021
Giurfa, M. (2015). Learning and cognition in insects. Wiley Interdiscip. Rev. 6, 383–395. doi: 10.1002/wcs.1348
Gluckman, P. D., and Hanson, M. A. (2004). Developmental origins of disease paradigm: a mechanistic and evolutionary perspective. Pediatr. Res. 56, 311–317. doi: 10.1203/01.PDR.0000135998.08025.FB
Godfrey, R. K., and Gronenberg, W. (2019). Brain evolution in social insects: advocating for the comparative approach. J. Comp. Physiol. A 205, 13–32. doi: 10.1007/s00359-019-01315-7
Gómez-Moracho, T., Heeb, P., and Lihoreau, M. (2017). Effects of parasites and pathogens on bee cognition. Ecol. Entomol. 42, 51–64.
Gowda, V., and Gronenberg, W. (2019). Brain composition and scaling in social bee species differing in body size. Apidologie 50, 779–792.
Griffin, A. S., Guillette, L. M., and Healy, S. D. (2015). Cognition and personality: an analysis of an emerging field. Trends Ecol. Evol. 30, 207–214. doi: 10.1016/j.tree.2015.01.012
Hämäläinen, A. M., Guenther, A., Patrick, S. C., and Schuett, W. (2021). Environmental effects on the covariation among pace-of-life traits. Ethology 127, 32–44.
Harvey, P. H., and Purvis, A. (1991). Comparative methods for explaining adaptations. Nature 351, 619–624. doi: 10.1038/351619a0
Healy, S. D., Bacon, I. E., Haggis, O., Harris, A. P., and Kelley, L. A. (2009). Explanations for variation in cognitive ability: behavioural ecology meets comparative cognition. Behav. Process. 80, 288–294. doi: 10.1016/j.beproc.2008.10.002
Jandt, J. M., and Gordon, D. M. (2016). The behavioral ecology of variation in social insects. Curr. Opin. Insect Sci. 15, 40–44. doi: 10.1016/j.cois.2016.02.012
Jandt, J. M., Bengston, S., Pinter-Wollman, N., Pruitt, J. N., Raine, N. E., Dornhaus, A., et al. (2014). Behavioural syndromes and social insects: personality at multiple levels. Biol. Rev. 89, 48–67. doi: 10.1111/brv.12042
Jaumann, S., Scudelari, R., and Naug, D. (2013). Energetic cost of learning and memory can cause cognitive impairment in honeybees. Biol. Lett. 9:20130149. doi: 10.1098/rsbl.2013.0149
R. L. Jeanne (ed.) (1988). Interindividual Behavioral Variability in Social Insects. Boulder, CO: Westview Press.
Jeanson, R., and Weidenmuller, A. (2014). Interindividual variability in social insects – proximate causes and ultimate consequences. Biol. Rev. 89, 671–687. doi: 10.1111/brv.12074
Jolles, J. W., King, A. J., and Killen, S. S. (2020). The role of individual heterogeneity in collective animal behaviour. Trends Ecol. Evol. 35, 278–291. doi: 10.1016/j.tree.2019.11.001
Jones, J. C., Myerscough, M. R., Graham, S., and Oldroyd, B. P. (2004). Honey bee nest thermoregulation: diversity promotes stability. Science 305, 402–404. doi: 10.1126/science.1096340
Junca, P., Carcaud, J., Moulin, S., Garnery, L., and Sandoz, J.-C. (2014). Genotypic influence on aversive conditioning in honeybees, using a novel thermal reinforcement procedure. PLoS One 9:e97333. doi: 10.1371/journal.pone.0097333
Junca, P., Garnery, L., and Sandoz, J.-C. (2019). Genotypic trade-off between appetitive and aversive capacities in honeybees. Sci. Rep. 9:10313. doi: 10.1038/s41598-019-46482-4
Kamhi, J. F., Gronenberg, W., Robson, S. K. A., and Traniello, J. F. A. (2016). Social complexity influences brain investment and neural operation costs in ants. Proc. R. Soc. B 283:20161949. doi: 10.1098/rspb.2016.1949
Katsnelson, E., Motro, U., Feldman, M. W., and Lotem, A. (2011). Individual-learning ability predicts social-foraging strategy in house sparrows. Proc. R. Soc. B 278, 582–589. doi: 10.1098/rspb.2010.1151
Katsnelson, E., Motro, U., Feldman, M. W., and Lotem, A. (2012). Evolution of learned strategy choice in a frequency-dependent game. Proc. R. Soc. B 279, 1176–1184. doi: 10.1098/rspb.2011.1734
Katz, K., and Naug, D. (2015). Energetic state regulates the exploration–exploitation trade-off in honeybees. Behav. Ecol. 26, 1045–1050.
Katz, K., and Naug, D. (2016). Dancers and followers in a honeybee colony differently prioritize individual and colony nutritional needs. Anim. Behav. 119, 69–74.
Keagy, J., Savard, J.-F., and Borgia, G. (2011). Complex relationship between multiple measures of cognitive ability and male mating success in satin bowerbirds, Ptilonorhynchus violaceus. Anim. Behav. 81, 1063–1070.
Kennedy, P., Baron, G., Qiu, B., Freitak, D., Helanterä, H., Hunt, E. R., et al. (2017). Deconstructing superorganisms and societies to address big questions in biology. Trends Ecol. Evol. 32, 861–872. doi: 10.1016/j.tree.2017.08.004
King, A. J., Isaac, N. J. B., and Cowlishaw, G. (2009). Ecological, social, and reproductive factors shape producer–scrounger dynamics in baboons. Behav. Ecol. 20, 1039–1049.
Klein, S., Pasquaretta, C., Barron, A. B., Devaud, J.-M., and Lihoreau, M. (2017). Inter-individual variability in the foraging behaviour of traplining bumblebees. Sci. Rep. 7:4561. doi: 10.1038/s41598-017-04919-8
Kurvers, R., Van Oers, K., Nolet, B. A., Jonker, R. M., Van Wieren, S. E., Prins, H. H. T., et al. (2010). Personality predicts the use of social information. Ecol. Lett. 13, 829–837. doi: 10.1111/j.1461-0248.2010.01473.x
Laloi, D., and Pham-Delegue, M.-H. (2010). Patriline-level variability in olfactory learning in the honey bee. Apidologie 41, 436–442. doi: 10.1051/apido/2009080
Laughlin, S. B., De Ruyter Van Steveninck, R. R., and Anderson, J. C. (1998). The metabolic cost of neural information. Nat. Neurosci. 1, 36–41. doi: 10.1038/236
LeBoeuf, A. C., and Grozinger, C. M. (2014). Me and we: the interplay between individual and group behavioral variation in social collectives. Curr. Opin. Insect Sci. 5, 16–24. doi: 10.1016/j.cois.2014.09.010
Liang, Z. Z. S., Nguyen, T., Mattila, H. R., Rodriguez-Zas, S. L., Seeley, T. D., and Robinson, G. E. (2012). Molecular determinants of scouting behavior in honey bees. Science 335, 1225–1228. doi: 10.1126/science.1213962
Lihoreau, M., Latty, T., and Chittka, L. (2012). An exploration of the social brain hypothesis in insects. Front. Physiol. 3:442. doi: 10.3389/fphys.2012.00442
Loreau, M., and Hector, A. (2001). Partitioning selection and complementarity in biodiversity experiments. Nature 412, 72–76. doi: 10.1038/35083573
Lumsden, C. J. (1982). The social regulation of physical caste: the superorganism revived. J. Theor. Biol. 95, 749–781. doi: 10.1016/0022-5193(82)90352-6
Luttbeg, B., and Sih, A. (2010). Risk, resources and state-dependent adaptive behavioural syndromes. Philos. Trans. R. Soc. B 365, 3977–3990. doi: 10.1098/rstb.2010.0207
MacArthur, R. H., and Wilson, E. O. (1967). The Theory of Island Biogeography. Princeton, NJ: Princeton University Press.
Mamuneas, D., Spence, A. J., Manica, A., and King, A. J. (2015). Bolder stickleback fish make faster decisions, but they are not less accurate. Behav. Ecol. 26, 91–96.
Marshall, J. A. R., Bogacz, R., Dornhaus, A., Planqué, R., Kovacs, T., and Franks, N. R. (2009). On optimal decision-making in brains and social insect colonies. J. R. Soc. Interface 6, 1065–1074. doi: 10.1098/rsif.2008.0511
Mattila, H. R., and Seeley, T. D. (2007). Genetic diversity in honey bee colonies enhances productivity and fitness. Science 317, 362–364. doi: 10.1126/science.1143046
Mayack, C., and Naug, D. (2011). A changing but not an absolute energy budget dictates risk-sensitive behaviour in the honeybee. Anim. Behav. 82, 595–600. doi: 10.1016/j.anbehav.2011.06.022
Mayack, C., and Naug, D. (2015). Starving honeybees lose self-control. Biol. Lett. 11:20140820. doi: 10.1098/rsbl.2014.0820
Mazza, V., Eccard, J. A., Zaccaroni, M., Jacob, J., and Dammhahn, M. (2018). The fast and the flexible: cognitive style drives individual variation in cognition in a small mammal. Anim. Behav. 137, 119–132. doi: 10.1016/j.anbehav.2018.01.011
Menzel, R. (2012). The honeybee as a model for understanding the basis of cognition. Nat. Rev. Neurosci. 13, 758–768. doi: 10.1038/nrn3357
Mercer, A. R., and Menzel, R. (1982). The effects of biogenic amines on conditioned and unconditioned responses to olfactory stimuli in the honeybee Apis mellifera. J. Comp. Physiol. A Sens. Neural Behav. Physiol. 145, 363–368. doi: 10.1007/bf00619340
Modlmeier, A. P., Liebmann, J. E., and Foitzik, S. (2012). Diverse societies are more productive: a lesson from ants. Proc. R. Soc. B 279, 2142–2150. doi: 10.1098/rspb.2011.2376
Moritz, R. F. B., and Page, R. E. Jr. (1999). “Behavioral threshold variability: costs and benefits in insect societies,” in Information Processing in Social Insects, eds C. Detrain, J. Deneubourg, and J. M. Pasteels (Basel: Birkhäuser Verlag), 203–215.
Mortensen, A. N., and Ellis, J. D. (2018). The effects of artificial rearing environment on the behavior of adult honey bees, Apis mellifera L. Behav. Ecol. Sociobiol. 72:92.
Mugel, S. G., and Naug, D. (2020). Metabolic rate shapes phenotypic covariance among physiological, behavioral, and life-history traits in honeybees. Behav. Ecol. Sociobiol. 74:129.
Mugel, S., and Naug, D. (in press). Metabolic rate diversity influences group-level performance in honeybees. Am. Nat.
Muller, H., and Chittka, L. (2012). Consistent interindividual differences in discrimination performance by bumblebees in colour, shape and odour learning tasks (Hymenoptera: Apidea: Bombus terrestris). Entomol. Gen. 34, 1–8. doi: 10.1127/entom.gen/34/2012/1
Murphy, T., Dias, G. P., and Thuret, S. (2014). Effects of diet on brain plasticity in animal and human studies: mind the gap. Neural Plast. 2014:563160. doi: 10.1155/2014/563160
O’Donnell, S., Bulova, S. J., Deleon, S., Khodak, P., Miller, S., and Sulger, E. (2015). Distributed cognition and social brains: reductions in mushroom body investment accompanied the origins of sociality in wasps (Hymenoptera: Vespidae). Proc. R. Soc. B 282:20150791. doi: 10.1098/rspb.2015.0791
Oldroyd, B. P., and Fewell, J. H. (2007). Genetic diversity promotes homeostasis in insect colonies. Trends Ecol. Evol. 22, 408–413. doi: 10.1016/j.tree.2007.06.001
Oster, G. F., and Wilson, E. O. (1978). Caste and Ecology in the Social Insects. Princeton, NJ: Princeton University Press.
Page, R. E. Jr., Erber, J., and Fondrk, M. K. (1998). The effect of genotype on response thresholds to sucrose and foraging behavior of honey bees (Apis mellifera L.). J. Comp. Physiol. A 182, 489–500. doi: 10.1007/s003590050196
Page, R. E., Rueppell, O., and Amdam, G. V. (2012). Genetics of reproduction and regulation of honeybee (Apis mellifera L.) social behavior. Annu. Rev. Genet. 46, 97–119. doi: 10.1146/annurev-genet-110711-155610
Page, R. E., Scheiner, R., Erber, J., Amdam, G. V., and Gerald, P. S. (2006). The development and evolution of division of labor and foraging specialization in a social insect (Apis mellifera L.). Curr. Top. Dev. Biol. 74, 253–286. doi: 10.1016/S0070-2153(06)74008-X
Pankiw, T., and Page, R. E. Jr. (2000). Response thresholds to sucrose predict foraging division of labor in honeybees. Behav. Ecol. Sociobiol. 47, 265–267. doi: 10.1007/s002650050664
Pankiw, T., Nelson, M., Page, R. E., and Fondrk, M. K. (2004). The communal crop: modulation of sucrose response thresholds of pre-foraging honey bees with incoming nectar quality. Behav. Ecol. Sociobiol. 55, 286–292.
Pelé, M., and Sueur, C. (2013). Decision-making theories: linking the disparate research areas of individual and collective cognition. Anim. Cogn. 16, 543–556. doi: 10.1007/s10071-013-0631-1
Piersma, T., and Drent, J. (2003). Phenotypic flexibility and the evolution of organismal design. Trends Ecol. Evol. 18, 228–233. doi: 10.1016/s0169-5347(03)00036-3
Pinter-Wollman, N. (2012). Personality in social insects: how does worker personality determine colony personality? Curr. Zool. 58, 580–588. doi: 10.1093/czoolo/58.4.580
Promislow, D. E. L., and Harvey, P. H. (1990). Living fast and dying young: a comparative analysis of life-history variation among mammals. J. Zool. 220, 417–437.
Raine, N. E., and Chittka, L. (2008). The correlation of learning speed and natural foraging success in bumble-bees. Proc. R. Soc. B 275, 803–808. doi: 10.1098/rspb.2007.1652
Raine, N. E., and Chittka, L. (2012). No trade-off between learning speed and associative flexibility in bumblebees: a reversal learning test with multiple colonies. PLoS One 7:e45096. doi: 10.1371/journal.pone.0045096
Réale, D., Garant, D., Humphries, M. M., Bergeron, P., Careau, V., and Montiglio, P.-O. (2010). Personality and the emergence of the pace-of-life syndrome concept at the population level. Philos. Trans. R. Soc. B 365, 4051–4063. doi: 10.1098/rstb.2010.0208
Réale, D., Reader, S. M., Sol, D., Mcdougall, P. T., and Dingemanse, N. J. (2007). Integrating animal temperament within ecology and evolution. Biol. Rev. 82, 291–318. doi: 10.1111/j.1469-185X.2007.00010.x
Reznick, D., Nunney, L., and Tessier, A. (2000). Big houses, big cars, superfleas and the costs of reproduction. Trends Ecol. Evol. 15, 421–425. doi: 10.1016/s0169-5347(00)01941-8
Ricklefs, R. E., and Wikelski, M. (2002). The physiology/life-history nexus. Trends Ecol. Evol. 17, 462–468. doi: 10.1016/s0169-5347(02)02578-8
Rittschof, C. C., Vekaria, H. J., Palmer, J. H., and Sullivan, P. G. (2018). Brain mitochondrial bioenergetics change with rapid and prolonged shifts in aggression in the honey bee, Apis mellifera. J. Exp. Biol. 221(Pt 8):jeb176917. doi: 10.1242/jeb.176917
Roeder, T. (2020). The control of metabolic traits by octopamine and tyramine in invertebrates. J. Exp. Biol. 223:194282. doi: 10.1242/jeb.194282
Rosa, P., Nguyen, V., and Dubois, F. (2012). Individual differences in sampling behaviour predict social information use in zebra finches. Behav. Ecol. Sociobiol. 66, 1259–1265.
Rosati, A. G. (2017). Foraging cognition: reviving the ecological intelligence hypothesis. Trends Cogn. Sci. 21, 691–702. doi: 10.1016/j.tics.2017.05.011
Roth, T. C., and Pravosudov, V. V. (2009). Tough times call for bigger brains. Commun. Integr. Biol. 2, 236–238. doi: 10.4161/cib.2.3.8099
Roth, T. C., Ladage, L. D., and Pravosudov, V. V. (2010). Learning capabilities enhanced in harsh environments: a common garden approach. Proc. R. Soc. B 277, 3187–3193. doi: 10.1098/rspb.2010.0630
Roussel, E., Carcaud, J., Sandoz, J.-C., and Giurfa, M. (2009). Reappraising social insect behavior through aversive responsiveness and learning. PLoS One 4:e4197. doi: 10.1371/journal.pone.0004197
Scheiner, R. (2012). Birth weight and sucrose responsiveness predict cognitive skills of honeybee foragers. Anim. Behav. 84, 305–308. doi: 10.1016/j.anbehav.2012.05.011
Scheiner, R., Kuritz-Kaiser, A., Menzel, R., and Erber, J. (2005). Sensory responsiveness and the effects of equal subjective rewards on tactile learning and memory of honeybees. Learn. Memory 12, 626–635. doi: 10.1101/lm.98105
Seeley, T. D. (1983). Division of labor between scouts and recruits in honeybee foraging. Behav. Ecol. Sociobiol. 12, 253–259.
Sgrò, C. M., and Hoffmann, A. A. (2004). Genetic correlations, tradeoffs and environmental variation. Heredity 93, 241–248. doi: 10.1038/sj.hdy.6800532
Shafir, S., Wiegmann, D. D., Smith, B. H., and Real, L. A. (1999). Risk-sensitive foraging: choice behaviours of honeybees in response to variability in volume of reward. Anim. Behav. 57, 1055–1061. doi: 10.1006/anbe.1998.1078
Sheehan, M. J., and Tibbetts, E. A. (2011). Specialized face learning is associated with individual recognition in paper wasps. Science 334, 1272–1275. doi: 10.1126/science.1211334
Sih, A., and Del Giudice, M. (2012). Linking behavioural syndromes and cognition: a behavioural ecology perspective. Philos. Trans. R. Soc. B 367, 2762–2772. doi: 10.1098/rstb.2012.0216
Smith, B. H., Abramson, C. I., and Tobin, T. R. (1991). Conditional withholding of proboscis extension in honeybees (Apis mellifera) during discriminative punishment. J. Comp. Psychol. 105, 345–356. doi: 10.1037/0735-7036.105.4.345
Smith, K. E., and Raine, N. E. (2014). A comparison of visual and olfactory learning performance in the bumblebee Bombus terrestris. Behav. Ecol. Sociobiol. 68, 1549–1559.
Southwick, E. E. (1983). The honey bee cluster as a homeothermic superorganism. Comp. Biochem. Physiol. A 75, 641–645.
Spaethe, J., Brockmann, A., Halbig, C., and Tautz, J. (2007). Size determines antennal sensitivity and behavioral threshold to odors in bumblebee workers. Naturwissenschaften 94, 733–739. doi: 10.1007/s00114-007-0251-1
Stamps, J. A. (2007). Growth-mortality tradeoffs and ‘personality traits’ in animals. Ecol. Lett. 10, 355–363. doi: 10.1111/j.1461-0248.2007.01034.x
Stearns, S. C. (1976). Life-history tactics: a review of the ideas. Q. Rev. Biol. 51, 3–47. doi: 10.1086/409052
Steffan-Dewenter, I., and Kuhn, A. (2003). Honeybee foraging in differentially structured landscapes. Proc. R. Soc. B 270, 569–575. doi: 10.1098/rspb.2002.2292
Stephens, D. W. (1987). On economically tracking a variable environment. Theor. Popul. Biol. 32, 15–25.
Stephens, D. W. (1989). Variance and the value of information. Am. Nat. 134, 128–140. doi: 10.1086/284969
Stephens, D. W. (1991). Change, regularity, and value in the evolution of animal learning. Behav. Ecol. 2, 77–89.
Székely, T., Remeš, V., Freckleton, R. P., and Liker, A. (2013). Why care? Inferring the evolution of complex social behaviour. J. Evol. Biol. 26, 1381–1391. doi: 10.1111/jeb.12148
Tait, C., and Naug, D. (2020). Cognitive phenotypes and their functional differences in the honey bee, Apis mellifera. Anim. Behav. 165, 117–122. doi: 10.1016/j.anbehav.2020.04.023
Tait, C., Brockmann, A., and Naug, D. (2021). Nesting ecology does not explain slow–fast cognitive differences among honeybee species. Anim. Cogn. 24, 1227–1235. doi: 10.1007/s10071-021-01515-2
Tait, C., Mattise-Lorenzen, A., Lark, A., and Naug, D. (2019). Interindividual variation in learning ability in honeybees. Behav. Process. 167:103918. doi: 10.1016/j.beproc.2019.103918
Takahashi, Y., Tanaka, R., Yamamoto, D., Noriyuki, S., and Kawata, M. (2018). Balanced genetic diversity improves population fitness. Proc. R. Soc. B 285:20172045. doi: 10.1098/rspb.2017.2045
Thornton, A., and Lukas, D. (2012). Individual variation in cognitive performance: developmental and evolutionary perspectives. Philos. Trans. R. Soc. B 367, 2773–2783. doi: 10.1098/rstb.2012.0214
Thornton, A., Isden, J., and Madden, J. R. (2014). Toward wild psychometrics: linking individual cognitive differences to fitness. Behav. Ecol. 25, 1299–1301.
Tsvetkov, N., Cook, C. N., and Zayed, A. (2019). Effects of group size on learning and memory in the honey bee Apis mellifera. J. Exp. Biol. 222:193888. doi: 10.1242/jeb.193888
van den Bos, R., Jolles, J., and Homberg, J. (2013). Social modulation of decision-making: a cross-species review. Front. Hum. Neurosci. 7:301. doi: 10.3389/fnhum.2013.00301
Van Noordwijk, A. J., and De Jong, G. (1986). Acquisition and allocation of resources: their influence on variation in life history tactics. Am. Nat. 128, 137–142. doi: 10.1086/284547
Vergoz, V., Roussel, E., Sandoz, J.-C., and Giurfa, M. (2007). Aversive learning in honeybees revealed by the olfactory conditioning of the sting extension reflex. PLoS One 2:e288. doi: 10.1371/journal.pone.0000288
Vickery, W. L., Giraldeau, L.-A., Templeton, J. J., Kramer, D. L., and Chapman, C. A. (1991). Producers, scroungers, and group foraging. Am. Nat. 137, 847–863. doi: 10.1098/rspb.2016.0114
Waddington, K. D., Herbert, T. J., Visscher, P. K., and Richter, M. R. (1994). Comparisons of forager distributions from matched honey bee colonies in suburban environments. Behav. Ecol. Sociobiol. 35, 423–429.
Wang, Y., Kaftanoglu, O., Brent, C. S., Page, R. E., and Amdam, G. V. (2016). Starvation stress during larval development facilitates an adaptive response in adult worker honey bees (Apis mellifera L.). J. Exp. Biol. 219, 949–959. doi: 10.1242/jeb.130435
Wenseleers, T., and van Zweden, J. S. (2017). Sensory and cognitive adaptations to social living in insect societies. Proc. Natl. Acad. Sci. U.S.A. 114, 6424–6426. doi: 10.1073/pnas.1707141114
Wiersma, P., Muñoz-Garcia, A., Walker, A., and Williams, J. B. (2007). Tropical birds have a slow pace of life. Proc. Natl. Acad. Sci. U.S.A. 104, 9340–9345.
Wolf, M., Van Doorn, G. S., Leimar, O., and Weissing, F. J. (2007). Life-history trade-offs favour the evolution of animal personalities. Nature 447, 581–584. doi: 10.1038/nature05835
Woods, H. A. (2014). Mosaic physiology from developmental noise: within-organism physiological diversity as an alternative to phenotypic plasticity and phenotypic flexibility. J. Exp. Biol. 217, 35–45. doi: 10.1242/jeb.089698
Wray, M. K., Mattila, H. R., and Seeley, T. D. (2011). Collective personalities in honeybee colonies are linked to colony fitness. Anim. Behav. 81, 559–568.
Keywords: cognitive phenotypes, pace of life, speed-accuracy tradeoff, social behavior, honeybees
Citation: Naug D and Tait C (2021) Slow-Fast Cognitive Phenotypes and Their Significance for Social Behavior: What Can We Learn From Honeybees? Front. Ecol. Evol. 9:766414. doi: 10.3389/fevo.2021.766414
Received: 29 August 2021; Accepted: 03 December 2021;
Published: 21 December 2021.
Edited by:
Sara Arganda Carreras, Rey Juan Carlos University, SpainReviewed by:
Jean-Christophe Sandoz, Centre National de la Recherche Scientifique (CNRS), FranceAurore Avargues-Weber, UMR 5169 Centre de Recherches sur la Cognition Animale (CRCA), France
Copyright © 2021 Naug and Tait. This is an open-access article distributed under the terms of the Creative Commons Attribution License (CC BY). The use, distribution or reproduction in other forums is permitted, provided the original author(s) and the copyright owner(s) are credited and that the original publication in this journal is cited, in accordance with accepted academic practice. No use, distribution or reproduction is permitted which does not comply with these terms.
*Correspondence: Dhruba Naug, ZGhydWJhQGNvbG9zdGF0ZS5lZHU=