- 1Public Health Unit, Department of Primary Health Care, Faculty of Medicine and Health Sciences, Universiti Sains Islam Malaysia, Nilai, Malaysia
- 2Department of Social and Preventive Medicine, Faculty of Medicine, University of Malaya, Kuala Lumpur, Malaysia
- 3Division of Health Economic Research and Case-Mix, Department of Research, Development and Innovation, University Malaya Medical Center, Kuala Lumpur, Malaysia
Haze imposes a substantial disease burden on the human population especially in the Southeast Asia region due to the high frequency of haze episodes. The reduction of air quality levels by haze has resulted in a substantial disease burden and an increase in healthcare utilization (HU). This study aims to determine the association between haze and HU of haze-related respiratory illnesses with a focus given on the acute exacerbation of bronchial asthma (AEBA) and chronic obstructive pulmonary disease (AECOPD). A cross-sectional study was conducted through secondary data collection of haze/non-haze episodes as the study exposures and HU related to the exacerbation of bronchial asthma and COPD as the study outcomes. Data on haze/non-haze episodes and HU for four consecutive years (2012–2015) were retrieved from the Department of Environment and Ministry of Health Malaysia, respectively. In the four consecutive years, the percentage of haze episodes recorded in all stations was higher (67%) as compared to non-haze (33%) episodes. Means (SD) of patients diagnosed with AEBA and AECOPD were also significantly higher (p < 0.05) for inpatient 74 (62.1) and outpatient 320 (650.1) cases during haze episodes as compared to inpatient 34 (16.5) and outpatient 146 (170.5) cases during non-haze episodes. Findings from this study indicated that haze episodes incurred a significant healthcare burden due to an increase in HU. The evidence from this study will help the policymakers to prepare and allocate resources to control future implications of haze-related illnesses.
Introduction
Haze is a condition associated with the disruption of visibility, clarity and transparency in an area due to the presence of fine suspended particles (Cheng et al., 2013; Othman et al., 2014). Specifically, it is defined as “an aggregation in the atmosphere of very fine, widely dispersed, solid, or liquid particles, or both, giving the air an opalescent appearance that subdues colors” (Hyslop, 2009, p. 182). Over the recent decades, Malaysia’s transformation from an agricultural-based to a more industrial-based country has led to rapid urbanization and an increase in manufacturing and industrial processing activities (Awang et al., 2000; Abdullah et al., 2012). Migration to urban areas such as Klang Valley has resulted in a dramatic increase in population density and traffic congestion. Exhaust emissions from motor vehicles and pollutants from industrial activities, in that order, are the main domestic sources of haze in Malaysia (Afroz et al., 2003; Abdullah et al., 2012; Mohd Shahwahid, 2016). Transboundary air pollutants from neighboring countries also contribute significantly to the occurrence of haze episodes in Malaysia. Along with other countries in the Southeast Asian region, Malaysia has been affected several times by haze episodes due to open forest burning in Indonesia (Othman et al., 2014; Mohd Shahwahid, 2016).
Haze poses serious and recurring medical problems, especially among susceptible individuals such as those suffering from chronic diseases, children, the elderly and pregnant women. The burden of illness is directly proportionate to the intensity of haze due to higher healthcare utilization and related healthcare costs during the haze episode. The health impacts of air pollution are more pronounced on the respiratory system than on the cardiovascular system (CVS). Healthcare utilization related to CVS illnesses mainly involves inpatient rather than outpatient cases, as most of these conditions require patients to be admitted for further monitoring and assessment. In contrast, exposure to air pollution has an immediate impact on the respiratory system, which makes it easier for researchers to attribute the episode to air pollution (Adar et al., 2014; Sahani et al., 2014). The main reasons for respiratory-related outpatient visits and hospital admissions are acute exacerbation of bronchial asthma (AEBA), acute exacerbation of chronic obstructive pulmonary disease (AECOPD), acute bronchitis, pneumonia and bronchiolitis (in infants), with exacerbation of asthma and chronic obstructive pulmonary disease accounting for the majority of cases (Peacock et al., 2011; Anderson et al., 2012; Mehta et al., 2013; Laumbach and Kipen, 2014). Health complications due to haze-related illnesses are significantly associated with increased healthcare utilization and reduced productivity due to work absenteeism. Both transboundary and local sources that result in haze episodes have been found to play a significant role in the increased use of healthcare facilities (Brauer and Jamal, 1998; Othman et al., 2014) and, hence, increased healthcare cost and expenditure. Together with the loss of productivity due to complications from haze-related illnesses, the health impact of haze episodes produces a significant financial burden for both healthcare providers and patients (Kochi et al., 2010; Othman et al., 2014). The objective of this study is to determine the trends in haze incidence and healthcare utilization in Malaysia so that steps can be taken to combat haze and allocate appropriate resources to meet the healthcare demands associated with haze episodes.
Materials and Methods
Study Background
The study was conducted for 4 years. Data for the period 2012–2015 were collected from the Department of Environment (DOE), the Ministry of Health (MOH), and University Malaya Medical Center (UMMC) and represented the latest data available from these bodies. A period of 4 years was considered sufficient to ensure adequate variation in the data distribution. This study was conducted in Selangor, which is one of the most developed state but with the worst air quality level compared to other states in Malaysia. The total population of Selangor in the year 2019 was 6.48 million people with a total land area of 7,930 km2 (674 persons per km2). Selangor has nine districts, each of which has its own health district office and at least one public hospital. The state has 12 public hospitals and 57 health clinics.
Study Population and Sampling Method
A universal sampling method was used in this study. First, districts with a Continuous Air Quality Monitoring (CAQM) station in Selangor were identified. These four districts are Petaling, Klang, Kuala Selangor, and Kuala Langat (Figure 1). All four districts were then included in the study. Districts without CAQM stations were excluded since it was difficult to identify a proxy measure of exposure levels to air pollutants. Data on the level of air pollutants and ecological factors recorded by the respective CAQM stations in each district were retrieved from DOE. To ensure the information on healthcare utilization corresponded to the data on the level of air pollutants and ecological factors in the identified area, patients who were diagnosed with AEBA or AECOPD and were admitted to public hospitals or visited emergency departments or health clinics in Petaling, Klang, Kuala Selangor, and Kuala Langat districts were identified and included as the study population. Their information was collected from cumulative monthly record data for every included hospital and health district office.
Exposure and Outcome
In this study, the definition of haze episode was based on World Health Organization (WHO) Air Quality Guideline (AQG), mean readings with 24 h PM10 value of ≥ 51 μg/m3 were considered as a haze episode and readings with 24 h PM10 value of ≤ 50 μg/m3 were considered as a non-haze episode (Krzyzanowski and Cohen, 2008). Haze and non-haze episodes were used as the exposure variable while the outcome was the utilization of healthcare facilities (treatment episodes of admissions and number of visits to the emergency department of selected public hospitals and health clinics) by patients diagnosed with AEBA and AECOPD during the defined events. CO, O3, NO2, SO2, temperature, wind speed, and humidity were treated as the covariates, based on previous literature (Dominick et al., 2012; Othman et al., 2014).
Data Collection
All data used in this study were from secondary sources. The process of data collection had two components: a collection of data on air pollutants and ecological factors; and collection of data on admissions (inpatients) and visits to public healthcare facilities (outpatients) for patients diagnosed with AEBA or AECOPD. Data on air pollutants and ecological factors were taken from CAQM stations in Selangor. Each station monitors five air pollutants and three ecological parameters, namely, PM10, CO, O3, NO2, SO2, ambient temperature, wind speed, and relative humidity. These data were collected since they are the two major components of haze, which are the particles and gaseous pollutants. Particles are formed by suspended liquid or solid elements and are commonly referred to as particulate matter (PM) (Hyslop, 2009). The other component, gaseous pollutants are made up of carbon monoxide (CO), sulfur dioxide (SO2), nitrogen dioxide (NO2), and ozone (O3). NO2 is one of the compounds of the family of nitrogen oxides (NOx), along with nitric oxide (NO), nitrogen trioxide, nitrogen tetroxide, and di-nitrogen pentoxide. Fossil fuel combustion results in the formation of atmospheric nitrogen (N2). The oxidation process of atmospheric N2 produces NO and subsequently NO2. CO is produced through incomplete combustion of carbonic materials and is a good indicator of the presence of pollution associated with combustion. SO2 can combine with water to produce sulfurous acid, which causes considerable irritation, especially in the eyes, skin and mucous membrane. The main source of SO2 is the combustion and roasting of sulfur-containing materials such as metal sulfide ores. Finally, O3 is formed in the troposphere through the reaction between reactive hydrocarbon (from combustion), UV light and NO2 (Brook et al., 2004). Data on the ambient temperature, wind speed, and relative humidity levels were also collected since these factors might also have a significant influence on the air pollutants level.
Except for PM10 (where the unit of measurement is in μg/m3), parts per million (ppm) is used as the unit of measurement for other air pollutants. For the ecological parameters, the unit of measurement is in Celsius (C) for temperature, kilometers per hour (km/h) for wind speed and percentage (%) for humidity (Rahman et al., 2015). These parameters were collected in the form of daily data. It was then converted into monthly data since there was a possibility of lag effect (2–4 weeks) from the time of exposure with the onset of respiratory symptoms. This was done to match and determine the association of air pollution with healthcare utilization. Details on the trend and factors associated with haze were presented in another manuscript (Jaafar, 2019).
For healthcare utilization, the data were retrieved from the health information center (HIC) under MOH and the UMMC records unit. As described earlier, all public healthcare facilities located in districts with a CAQM station (Petaling, Klang, Kuala Selangor, and Banting) in Selangor were included. Data from the CAQM stations were matched with healthcare utilization data for the public healthcare facilities located in their respective districts. The public healthcare utilization data needed were the numbers of inpatient and outpatient cases diagnosed with AEBA and AECOPD at the respective healthcare facilities. The diagnosis used was based on the discharge diagnosis and followed the WHO International Classification of Diseases (ICD 10). These are the standard codes used by all healthcare facilities under MOH Malaysia and were also used in the HIC recording system. The ICD code for asthma is ICD-10:J45-J46 and ICD-10:J44 for COPD (WHO, 2016).
Data Analysis
Descriptive analyses were done to determine healthcare utilization in public healthcare facilities during haze and non-haze episodes. Continuous variables were presented as mean and standard deviation (median and inter-quartile range for data that were not normally distributed). These include monthly data for CO, O3, NO2, SO2, temperature, wind speed, and humidity.
For the categorical variable (haze episode), the data were presented as the frequency with percentage. Healthcare utilization that was in the form of discrete data (also known as a Poisson distribution) was also presented as frequency and percentage.
Bivariate analyses were done to determine any association between haze episode (as the exposure) and healthcare utilization (as the outcome). Associations between exposure and covariates were also included in the analyses to identify any interaction between those variables. For bivariate analysis, the level of significance was pre-set at 0.05. For bivariate analyses, Independent T-test (Mann-Whitney for non-normally distributed data) was used to identify a significant association between exposure (haze episodes) and outcomes (healthcare utilization). For the covariates (CO, O3, NO2, SO2, temperature, wind speed, and humidity), since all the data were continuous variables, Pearson’s (Spearman’s for non-normally distributed data) correlation test was used instead. Associations between exposure and covariates were also analyzed using Independent T-test (Mann-Whitney for non-normally distributed data) to identify any interaction that needed to be controlled in multivariate analysis. Results from bivariate analysis were presented with frequency, percentage and mean difference with p-value and 95% confidence interval (for Independent T-test/Mann-Whitney test) and correlation coefficient with p-value and 95% confidence interval (for Pearson’s/Spearman correlation test). All variables with significant results in bivariate analysis were further analyzed in multivariate analyses. Multivariate analysis was done using Poisson regression under generalized linear models. Poisson regression was chosen since the outcomes variables were in the form of discrete data. Variables with significance value (p < 0.25) in the univariate regression analysis were included in multivariate analysis. Results for multivariate analysis were presented with p-value, 95% confidence interval and odds ratio. Variables with significant values (p < 0.05) were included in the final regression model.
Results
Healthcare Utilization of Haze-Related Respiratory Illnesses
Overall, 192 monthly readings were collected for all air pollutants and ecological factors from four CAQM stations (located in Petaling, Klang, Kuala Selangor, and Banting). These data were matched with monthly healthcare utilization data from public hospitals and health clinics located in the same district from 2012 to 2015 (12 readings for monthly data in a year × 4 districts × 4 years). PM10 level was used as a reference to define a haze episode based on the World Health Organization Air Quality Guidelines (WHO AQG) for PM10. PM10 levels of ≥ 51 μg/m3 were categorized as a haze episode and PM10 levels of ≤ 50 μg/m3 were categorized as a non-haze episode. Of the 192 readings, the highest daily PM10 level recorded was 595.1 in July 2013. 129 (67.19%) were classified as haze episodes and 63 (32.81%), as non-haze episodes. The total utilization of public healthcare facilities (for inpatient and outpatient cases of AEBA and AECOPD) in the studied districts was 62,051. Of these, 11,670 (18.81%) were inpatient cases and 50,381 (81.19%) were outpatient cases. The majority of inpatient cases were diagnosed with AEBA (n = 7,894, 12.72%), compared to 3,776 (6.09%) for AECOPD. The number of AEBA outpatient cases (n = 47,897, 77.19%) was also higher compared to AECOPD (n = 2,484, 4.00%).
Data from the MOH did not contain information on the age, gender and ethnicity of patients who sought treatment in its facilities. Therefore, a sub-analysis for socio-demographic characteristics was conducted using healthcare utilization data collected at UMMC. The total numbers of inpatient and outpatient cases that received treatment for AEBA and AECOPD in UMMC from 2012 to 2015 were 1,068 and 3,124, respectively. More than half of these patients were male and approximately two-thirds of them were Malay, followed by Indian and Chinese. Details of healthcare utilization rates and socio-demographic characteristics are summarized in Tables 1, 2.
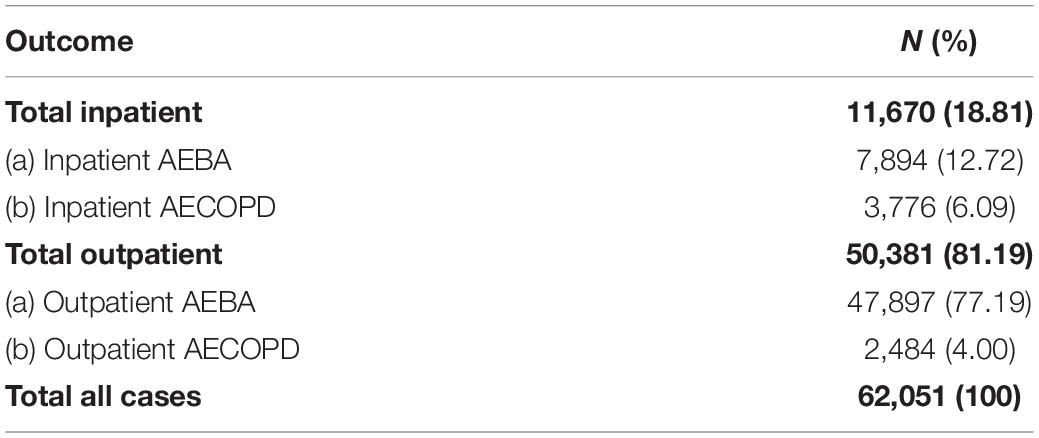
Table 1. Healthcare utilization for AEBA and AECOPD in public healthcare facilities for all studied districts 2012–2015.
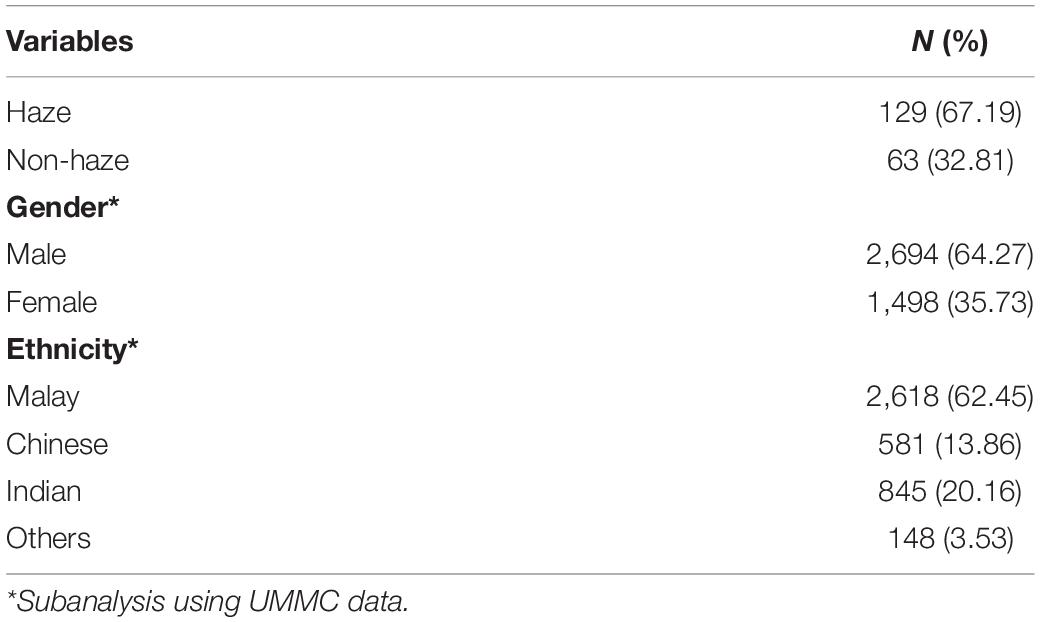
Table 2. Number of haze episodes and socio-demographic characteristics of AEBA and AECOPD cases 2012–2015 (from UMMC data).
Association Between Healthcare Utilization and Haze Episodes
For AEBA, healthcare utilization for both inpatient and outpatient cases was higher during haze episodes. The average monthly admission for AEBA was 50 cases during a haze episode compared to 23 cases during non-haze periods. Similarly, the average monthly numbers of AEBA outpatient cases were 307 and 132 during haze and non-haze episodes, respectively. AECOPD also recorded a higher average monthly admission of 24 cases during haze compared to 11 during non-haze episodes. A different pattern, however, was observed for AECOPD outpatient cases, which recorded a higher average monthly visit rate during non-haze periods (14 cases) compared to that during haze episodes (13 cases). For total inpatient cases (for both AEBA and AECOPD), the average number of monthly admissions was 74 compared to 34 during non-haze episodes. Average monthly outpatient cases were also higher during haze episodes (320 cases) compared to non-haze episodes (146 cases). The difference in healthcare utilization between haze and non-haze episodes was statistically significant (p-value < 0.05) for all types of healthcare utilization except AECOPD outpatient cases. Results for the association between healthcare utilization and haze episodes are summarized in Table 3.
Association Between Healthcare Utilization and Other Pollutants and Ecological Factors
To identify possible confounders among the covariates (other air pollutants and ecological factors), a correlation test was conducted to determine whether there was any significant association between the covariates and healthcare utilization. Inpatient AEBA cases were not significantly associated with any of the air pollutants or ecological factors. For the inpatient AECOPD cases, significant negative correlations were observed with CO (r = −0.24, p-value = 0.01) and temperature (r = −0.20, p-value = 0.02). Outpatient AEBA cases were significantly correlated with all parameters except for SO2 (r = 0.05, p-value = 0.65) and NO2 (r = 0.19, p-value = 0.06). For outpatient AECOPD cases, significant correlations were also observed with CO (r = 0.30, p-value < 0.001), NO2 (r = 0.26, p-value = 0.02), and humidity (r = −0.30, p-value < 0.001). For total inpatient cases, significant correlations were recorded with CO (r = −0.22, p-value = 0.02), NO2 (r = −0.25, p-value = 0.03), and temperature (r = −0.19, p-value = 0.03). All parameters were significantly correlated with total outpatient cases except for SO2 (r = 0.05, p-value = 0.66) and NO2 (r = 0.20, p-value = 0.06). Although some of the air pollutants and ecological parameters were significantly associated with healthcare utilization (p-value < 0.05), none of the correlation coefficient (r) values was more than 0.5, indicating that there was no strong correlation between these variables. Results for correlations between healthcare utilization and other pollutants and ecological factors are summarized in Table 4.
Association Between Healthcare Utilization and Sociodemographic Characteristics
A sub-analysis was conducted using UMMC data to determine the association between healthcare utilization and age, gender and ethnicity during haze and non-haze episodes. Overall, there were no significant gender differences in total inpatient [X2 (1, N = 1,068) = 0.335, p-value = 0.56] and total outpatient [X2 (1, N = 3,124) = 0.113, p-value = 0.74] cases during haze and non-haze episodes. Stratification based on ethnicity also revealed no significant difference in total inpatient [X2 (1, N = 1,068) = 6.078, p-value = 0.108] and total outpatient [X2 (1, N = 3,119) = 1.33, p-value = 0.72] cases during haze and non-haze episodes. There were also no significant age differences for both total inpatient [t (1,066) = 0.743, p-value = 0.17] and outpatient [t (3,122) = −0.489, p-value = 0.67] cases during haze and non-haze episodes.
Multivariate Analysis: Healthcare Utilization and Haze-Related Respiratory Illnesses
Univariate regression analyses were performed for each of the variables included to determine the crude odds ratio (OR). For inpatient cases, only haze episode (OR = 2.19, 95% CI: 1.611–2.964, p-value < 0.001) and NO2 (OR = 0.02, 95% CI: 0.992–0.999, p-value = 0.02) showed a significant association with the outcome. Both CO (OR = 0.96, 95% CI: 0.709–1.288, p-value = 0.79) and temperature (OR = 0.93, 95% CI: 0.817–1.057, p-value = 0.29) had a p-value of > 0.25 and thus were not considered in multivariate analysis. For outpatient cases, all variables had p-value of < 0.25. The highest crude OR was recorded by haze episode (OR = 2.20, 95% CI: 1.628–2.980, p-value < 0.001), followed by CO (OR = 1.97, 95% CI: 1.377–2.807, p-value < 0.001), wind speed (OR = 1.28, 95% CI: 1.184–1.386, p-value < 0.001), and temperature (OR = 1.14, 95% CI: 1.004–1.284, p-value = 0.05). The lowest OR was recorded by humidity (OR = 0.94, 95% CI: 0.924–0.960, p-value < 0.001), followed by O3 (OR = 0.96, 95% CI: 0.946–0.968, p-value < 0.001). All variables were then considered and included as in multivariate analysis.
Multivariate analyses were then conducted for both outcomes. For inpatient cases, both haze episode and NO2 recorded a significant p-value of < 0.01. The goodness of fit test showed a deviance/df ratio of 0.523 and Pearson chi-square/df ratio of 0.526. Since both tests showed a ratio of less than 2, the final regression model was considered to have a good model fit. For outpatient cases, initial multivariate analysis showed significant results (p-value < 0.05) for haze episodes and O3 only. The analysis was then rerun with the exclusion of non-significant variables. All variables included in the subsequent analysis were noted to have a p-value of < 0.001. Goodness of fit test showed a deviance/df ratio of 1.086 and Pearson chi-square/df ratio of 1.352. For inpatient cases, since both tests showed a ratio of less than 2, the final regression model was considered to have a good model fit. Based on the final regression model, the odds for both hospital admission and outpatient visit were 2.2 times higher during haze compared to non-haze episodes. Results of multivariate analysis are summarized in Tables 5, 6.
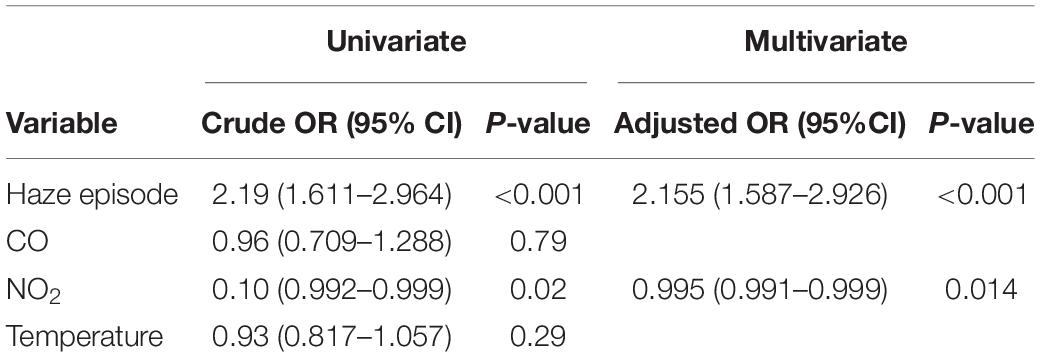
Table 5. Crude and adjusted odds ratios in univariate and multivariate regression analyses (inpatient).
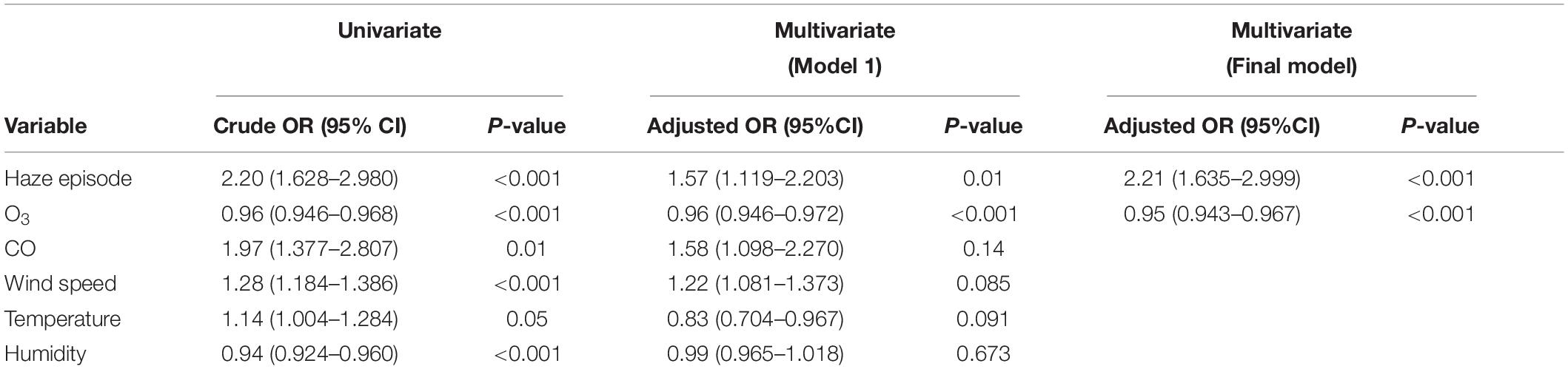
Table 6. Crude and adjusted odds ratios in univariate and multivariate regression analyses (outpatient).
Discussion
Except in 2014, PM10 concentration reached its maximum level around May to September each year. This pattern was observed in all CAQM stations (Figure 2). The maximum level recorded during these periods coincided with biomass burning from forest and peat fires (as a result of agricultural land clearing in Indonesia) (Azmi et al., 2010; Abdullah et al., 2012; Norela et al., 2013) It was aggravated by the south-west monsoon season (June–September) (Azmi et al., 2010; Rahman et al., 2015). The southwest monsoon wind from Sumatra took only 48 h to reach all CAQM stations in Selangor (Azmi et al., 2010). The wind carried the particles and gaseous pollutants from the affected area to the western part of the Malaysian Peninsular, particularly Selangor. In addition, unlike the northeast monsoon, the southwest monsoon is commonly associated with the “dry season” (Azmi et al., 2010). PM10 is usually “washed out” of the atmosphere through a chemical reaction with water vapor (Norela et al., 2013). Low humidity levels together with less rainfall during this period resulted in an accumulation of PM10 in the atmosphere for a longer duration and caused deterioration in air quality (Azmi et al., 2010; Juneng et al., 2011; Norela et al., 2013; Rahman et al., 2015).
Overall results from this study showed that healthcare utilization for haze-related respiratory illnesses was 4.5 times higher during haze compared to non-haze episodes. These results were based on the total and the average number of hospital admissions and outpatient visits for both AEBA and AECOPD. The peak utilization of healthcare services was observed during the haze episode especially during the “dry season” where PM10 concentrations were consistently high almost every day and reached their maximum level. For total inpatient cases, the number of hospital admissions recorded was 4.5 times higher during haze episodes. Similarly, the number of outpatient visits for AEBA and AECOPD was also 4.5 times higher during haze episodes. Results from the multivariate analysis showed that the odds for both hospital admissions and outpatient visits were 2.2 times higher during haze episodes.
Although the effect sizes varied, the results from this study were consistent with findings from other literature and within the expected outcome. A local study showed significantly higher weekly mean (SD) hospital admissions during haze, with 27 (9.2) cases, compared to non-haze episodes with 15.7 (6.7) cases (Ming et al., 2018). Similar to our findings, the odds for hospital admission were 1.95 and 2.13 times higher during haze episodes for AEBA and AECOPD, respectively. A higher number of intensive care admissions and longer hospital stays were also recorded during haze episodes (Ming et al., 2018). The results from this study were also supported by another local study which showed a 31% increase in hospital admissions during haze episodes (Othman et al., 2014). Similarly, a systematic review of the health impacts of haze in Southeast Asian countries showed an increase in respiratory morbidity, hospital admissions, and clinic visits during haze episodes (Ramakreshnan et al., 2017).
Previous systematic reviews (SR) and meta-analyses (MA) reported similar findings for healthcare utilization during haze episodes (Cheong et al., 2019). Nevertheless, direct comparison of the effect size and associated risk were difficult as the methodologies and parameters used in those reviews differed from those used in this study. Instead of categorizing exposure into the haze and non-haze episodes, most of the SRs and MAs used particulate matter (PM2.5 and/or PM10) as a continuous variable to determine the linear relationship with healthcare utilization. In addition, the outcome parameters measured were in the form of daily data compared to monthly healthcare utilization data used in this study. The summary conclusion from the reviews was that for every 10 μg/m3 increase in PM10, there was a 0.51–3.7% increase in the hospital admission rate and a 0.3–3.7% increase in outpatient visits (Kochi et al., 2009; Adar et al., 2014; Atkinson et al., 2014; Lu et al., 2015). Recent research on the health impact of both PM10 and PM2.5 also showed an increase of 0.43% in respiratory hospital admission cases with every 10 μg/m3 increase in PM10 (Qiu et al., 2018) and 0.67–1.78% increase in emergency room visits with 10 μg/m3 increase in PM2.5 (Mo et al., 2018). Although the studies differed in the definition of haze episode, type of illnesses and parameters used, methodological approaches and effect size measurement, the outcomes were still consistent, supportive and comparable with the findings from this study.
During haze episodes, particulate matter was the most dominant air pollutant. Previous studies showed that particulate matter triggered inflammatory reactions, mainly affecting the respiratory system, and resulted in AEBA and AECOPD (Anderson et al., 2012; Athanazio, 2012). As the result of exposure to higher concentrations of particulate matter during haze episodes, the number of AEBA and AECOPD cases was also expected to rise and subsequently lead to an increase in healthcare utilization during haze episodes, as seen in this study.
Accordingly, two action plans—the National Haze Action Plan and the Haze Management Action Plan—were developed by DOE and MOH, respectively, to reduce the haze-related clinical burden in Malaysia and identify actions that need to be taken during haze episodes (Sulaiman, 2017). From a health perspective, the main focus was on increased awareness of and reduced exposure to haze among the public, to minimize the health impact of haze. The MOH played several roles, including public advisory, health education and promotion, disease surveillance, and disease management. Advice to reduce outdoor activities and wear protection (such as a face mask) was disseminated through social and electronic media, press releases and pamphlets at strategic locations to reach the target population. In addition, decisions to close schools and to cancel public events were also taken based on recommendations from the MOH (Sulaiman, 2017).
However, the strategies used to minimize the impact of haze on health were problematic because of the definition of haze episode used by the DOE. Interventions and advice were only deployed when the PM10 concentration level was more than 150 μg/m3 (API > 100). In fact, during the 2015 haze episode, the decision to close schools was only made when the PM10 concentration was more than 250 μg/m3 (API > 150) (Sulaiman, 2017). The daily PM10 concentration levels at that time were persistently high (250–350 μg/m3) for 2 months (September to November 2015) and recorded the longest streak of haze episodes compared to the year 2012, 2013, and 2014 (Jaafar, 2019). It is preferable to use a lower threshold for the definition of haze based on the World Health Organization Air Quality Guidelines (WHO AQG) as the main reference. All interventions and advice to minimize the health impact of haze should be initiated once the PM10 concentration exceeds the target level set by WHO AQG. If the action plan were based on the WHO AQG target level, there is a higher probability that the healthcare utilization rate during haze episodes could be reduced.
Apart from the target level used to define a haze episode, the effectiveness of the interventions is also open to question. For example, one recommended strategy is to use surgical masks and N95 respirators. While the N95 respirator offers better protection than a mask, its cost and the breathing discomfort associated with wearing it reduce compliance. Although the effectiveness of the surgical mask is still debated, it is more convenient, readily available and cheap, and is more widely used as personal protective equipment (PPE) from haze compared to the N95 respirator (Sulaiman, 2017). In addition, advice to reduce outdoor activities and stay indoors during haze fails to consider the indoor air quality and infiltration rate (indoor-outdoor ratio) of pollutants for different types of buildings (naturally ventilated or air-conditioned). The use of air filters should be considered as an intervention that can improve indoor air quality, especially during haze episodes (Sulaiman, 2017).
Apart from haze episodes, other factors that could contribute to the increase in healthcare utilization due to AEBA and AECOPD in this study were assumed to have comparable triggering capacity during haze and non-haze episodes. For instance, work-related exposure and indoor air pollution (apart from pollutants that infiltrate from outdoor sources) that can trigger the exacerbation were anticipated to be similar and dispersed among the population throughout the study period. In addition, 4 years of cumulative data were considered to account for seasonal variability that might be associated with the healthcare utilization rate for AEBA and AECOPD. In this study, all ecological factors were included and controlled in the multivariate analysis.
Results in this study showed that seasonal variability and ecological factors such as wind speed, temperature and humidity were not significantly associated with the healthcare utilization rate. Similar findings were reported from a local study, which concluded that the exacerbation episodes and healthcare utilization associated with asthma and COPD during haze episodes in Malaysia were not directly influenced by any seasonal factors (Othman et al., 2014). In addition, sub-analysis in the present study of the socio-demographic factors that can contribute to exacerbation episodes revealed no significant differences in the healthcare utilization rate according to gender, ethnicity, and age between haze and non-haze episodes. These findings were supported by a local study that showed no significant differences for respiratory admissions between haze and non-haze episodes by age and gender (Ming et al., 2018). Another local study showed that the total inpatient cases were higher among children, followed by young adults, adults and infants, during haze episodes (Othman et al., 2014). However, the study did not compare total inpatient cases during non-haze episodes to identify differences in the age of patients admitted for respiratory illnesses during haze and non-haze episodes. In another study conducted in Taiwan, a comparison was made on the contribution of indoor and outdoor air pollution to the 1-year prevalence of asthma. The study found that both exposures to smoking/indoor air pollution and exposure to total suspended particles (outdoor air pollution) recorded similar OR (1.29) of developing asthma (Wang et al., 1999).
The present study identified several limitations in the healthcare utilization data. First, the rate of utilization of healthcare services, especially for AECOPD outpatient cases, might be underestimated. There were missing data due to the failure of several health clinics to document the specific diagnosis. Hence the recorded incidence of AECOPD outpatient cases might be lower than the number of actual cases.
Second, for inpatient cases, the selection of healthcare facilities was based on the nearest hospital to the location of CAQM stations in that particular district. However, there is a possibility that patients might obtain treatment from a hospital that was not included in the study area, especially when other public or private hospitals were located quite close to the selected hospital, such as in Petaling district.
For outpatient cases, the healthcare utilization data collected were more complete with less risk of sample contamination. The main issue here was the determination of exposure level. If the haze episode was from a transboundary source, exposure to air pollutants could be assumed to be similar in all health clinics. If it was due to local sources, however, the level of air pollutants measured at the CAQM station might not reflect the actual exposure level at selected health clinics that were far from the stations, even though they were in the same district. For example, if domestic waste burning occurs nearer to a health clinic than to the CAQM station, the exposure levels experienced by people living near the burning site might be higher than the level recorded by the CAQM station.
In addition, no patient-based data were available in this study. The influence of age, socio-demographic background, comorbidity, and other contributing factors for AEBA and AECOPD were assumed to be similar during haze and non-haze episodes. Assumptions were also made that the exposure levels among the population were similar to the readings recorded at the CAQM stations located in the same selected district. The incidence rate might also be higher if self-treating and private patients were included. However, since this study focused on the impact of haze from the healthcare provider’s perspective, the exclusion of this information was justifiable.
Data Availability Statement
The data analyzed in this study is subject to the following licenses/restrictions: confidentiality of data involving healthcare utilization information. Requests to access these datasets should be directed to ZWhhQGRvZS5nb3YubXk=, bm9yYXplYW5AdW1tYy5lZHUubXk=, and bXV6YWxhd2F0aUBtb2guZ292Lm15.
Author Contributions
HJ, AA, MI, and MD developed concepts, researched, and wrote the manuscript. HJ and AA performed analyses. All authors had approved the final manuscript for submission.
Conflict of Interest
The authors declare that the research was conducted in the absence of any commercial or financial relationships that could be construed as a potential conflict of interest.
Publisher’s Note
All claims expressed in this article are solely those of the authors and do not necessarily represent those of their affiliated organizations, or those of the publisher, the editors and the reviewers. Any product that may be evaluated in this article, or claim that may be made by its manufacturer, is not guaranteed or endorsed by the publisher.
Acknowledgments
We would like to thank the UMMC, DOE, and MOH Malaysia for all the data shared for this study.
References
Abdullah, A. M., Abu Samah, M. A., and Jun, T. Y. (2012). An overview of the air pollution trend in Klang Valley, Malaysia. Open Environ. Sci. 6, 13–19. doi: 10.2174/1876325101206010013
Adar, S. D., Filigrana, P., Clements, N., and Peel, J. L. (2014). Ambient coarse particulate matter and human health: a systematic review and meta-analysis. Curr. Environ. Health Rep. 1, 258–274. doi: 10.1007/s40572-014-0022-z
Afroz, R., Hassan, M. N., and Ibrahim, N. A. (2003). Review of air pollution and health impacts in Malaysia. Environ. Res. 92, 71–77. doi: 10.1016/S0013-9351(02)00059-2
Anderson, J. O., Thundiyil, J. G., and Stolbach, A. (2012). Clearing the air: a review of the effects of particulate matter air pollution on human health. J. Med. Toxicol. 8, 166–175. doi: 10.1007/s13181-011-0203-1
Athanazio, R. (2012). Airway disease : similarities and differences between Asthma, COPD and bronchiectasis. Clinics 67, 1335–1343. doi: 10.6061/clinics/2012(11)19
Atkinson, R. W., Kang, S., Anderson, H. R., Mills, I. C., and Walton, H. A. (2014). Epidemiological time series studies of PM2.5 and daily mortality and hospital admissions: a systematic review and meta-analysis. Thorax 69, 660–665. doi: 10.1136/thoraxjnl-2013-204492
Awang, M. B., Jaafar, A. B., Abdullah, A. M., Ismail, M. B., Hassan, M. N., Abdullah, R., et al. (2000). Air quality in Malaysia: impacts, management issues and future challenges. Respirology (Carlton, Vic.) 5, 183–196. doi: 10.1046/j.1440-1843.2000.00248.x
Azmi, S. Z., Latif, M. T., Ismail, A. S., Juneng, L., and Jemain, A. A. (2010). Trend and status of air quality at three different monitoring stations in the Klang Valley, Malaysia. Air Qual. Atmos. Health 3, 53–64. doi: 10.1007/s11869-009-0051-1
Brauer, M., and Jamal, H. H. (1998). Fires in Indonesia: crisis and reaction. Environ. Sci. Technol. 32, 404–407. doi: 10.1021/es983677j
Brook, R. D., Franklin, B., Cascio, W., Hong, Y., Howard, G., Lipsett, M., et al. (2004). Air pollution and cardiovascular disease. Circulation 6083, 2655–2688. doi: 10.1161/01.CIR.0000128587.30041.C8
Cheng, Z., Wang, S., Jiang, J., Fu, Q., Chen, C., Xu, B., et al. (2013). Long-Term trend of haze pollution and impact of particulate matter in the Yangtze River Delta, China. Environ. Pollut. 182, 101–110. doi: 10.1016/j.envpol.2013.06.043
Cheong, K. H., Ngiam, N. J., Morgan, G. G., Pek, P. P., Tan, B. Y. Q., Lai, J. W., et al. (2019). Acute health impacts of the Southeast Asian Transboundary haze problem – a review. Int. J. Environ. Res. Public Health 16:3286. doi: 10.3390/ijerph16183286
Dominick, D., Talib, M., Zain, S. M., and Zaharin, A. (2012). Spatial assessment of air quality patterns in Malaysia using multivariate analysis. Atmos. Environ. 60, 172–181. doi: 10.1016/j.atmosenv.2012.06.021
Hyslop, N. P. (2009). Impaired visibility : the air pollution people see. Atmos. Environ. 43, 182–195. doi: 10.1016/j.atmosenv.2008.09.067
Jaafar, H. (2019). Respiratory Health Impacts of Haze Exposure and Its Financial Implications. Ph.D. thesis. Kuala Lumpur: University of Malaya.
Juneng, L., Latif, M. T., and Tangang, F. (2011). Factors in fluencing the variations of PM10 aerosol dust in Klang Valley, Malaysia during the summer. Atmos. Environ. 45, 4370–4378. doi: 10.1016/j.atmosenv.2011.05.045
Kochi, I., Donovan, G. H., Champ, P. A., and Loomis, J. B. (2010). The economic cost of adverse health effects from wildfire-smoke exposure: a review. Int. J. Wildland Fire 19, 803–817. doi: 10.1071/WF09077
Kochi, I., Loomis, J., Champ, P., and Donovan, G. (2009). “Health and economic impact of wildfires : literature review and impact assessment,” in Proceedings of the 3rd International Symposium on Fire Economics, Planning, and Policy: Common Problems and Approaches, ed. A. González-Cabán (Colarado), 1–14. Available online at: https://www.cabdirect.org/cabdirect/abstract/20113108269 (accessed February 4, 2021).
Krzyzanowski, M., and Cohen, A. (2008). Update of WHO air quality guidelines. Air Qual. Atmos. Health 1, 7–13. doi: 10.1007/s11869-008-0008-9
Laumbach, R. J., and Kipen, H. M. (2014). Respiratory health effects of air pollution : update on biomass smoke and traffic pollution. J. Allergy Clin. Immunol. 129, 3–11. doi: 10.1016/j.jaci.2011.11.021
Lu, F., Xu, D., Cheng, Y., Dong, S., Guo, C., Jiang, X., et al. (2015). Systematic review and meta-analysis of the adverse health effects of ambient PM2.5 and PM10 pollution in the Chinese population. Environ. Res. 136, 196–204. doi: 10.1016/j.envres.2014.06.029
Mehta, S., Shin, H., Burnett, R., North, T., and Cohen, A. J. (2013). Ambient particulate air pollution and acute lower respiratory infections : a systematic review and implications for estimating the global burden of disease. Air Qual. Atmos. Health 6, 69–83. doi: 10.1007/s11869-011-0146-3
Ming, C. R., Yu-Lin, A. B., Hamid, M. F. A., Latif, M. T., Mohammad, N., and Hassan, T. (2018). Annual Southeast Asia Haze increases respiratory admissions: a 2-year large single institution experience. Respirology 23, 914–920. doi: 10.1111/resp.13325
Mo, Z., Fu, Q., Zhang, L., Lyu, D., Mao, G., Wu, L., et al. (2018). Acute effects of air pollution on respiratory disease mortalities and outpatients in Southeastern China. Sci. Rep. 8:3461. doi: 10.1038/s41598-018-19939-1
Mohd Shahwahid, H. O. (2016). The Economic Value of the June 2013 Haze Impacts on Peninsular Malaysia (No. rr2016013). Laguna: Economy and Environment Program for Southeast Asia (EEPSEA).
Norela, S., Saidah, M. S., and Mahmud, M. (2013). Chemical composition of the Haze in Malaysia 2005. Atmos. Environ. 77, 1005–1010. doi: 10.1016/j.atmosenv.2013.05.024
Othman, J., Sahani, M., Mahmud, M., and Ahmad, M. K. (2014). Transboundary smoke haze pollution in Malaysia: inpatient health impacts and economic valuation. Environ. Pollut. 189, 194–201. doi: 10.1016/j.envpol.2014.03.010
Peacock, J. L., Anderson, H. R., Bremner, S. A., Marston, L., Seemungal, T. A., Strachan, D. P., et al. (2011). Outdoor air pollution and respiratory health in patients with COPD. Thorax 66, 591–596. doi: 10.1136/thx.2010.155358
Qiu, H., Yu, H., Wang, L., Zhu, X., Chen, M., Zhou, L., et al. (2018). The burden of overall and cause-specific respiratory morbidity due to ambient air pollution in Sichuan Basin, China: a multi-city time-series analysis. Environ. Res. 167, 428–436. doi: 10.1016/j.envres.2018.08.011
Rahman, S. R. A., Ismail, S. N. S., Ramli, M. F., Latif, M. T., Abidin, E. Z., and Praveena, S. M. (2015). The assessment of ambient air pollution trend in Klang Valley, Malaysia. World Environ. 5, 1–11. doi: 10.5923/j.env.20150501.01
Ramakreshnan, L., Aghamohammadi, N., Fong, C. S., Bulgiba, A., Zaki, R. A., Wong, L. P., et al. (2017). Haze and health impacts in ASEAN countries: a systematic review. Environ. Sci. Pollut. Res. 25, 2096–2111. doi: 10.1007/s11356-017-0860-y
Sahani, M., Zainon, N. A., Mahiyuddin, W. R. W., Latif, M. T., Hod, R., Khan, M. F., et al. (2014). A case-crossover analysis of forest fire haze events and mortality in Malaysia. Atmos. Environ. 96, 257–265. doi: 10.1016/j.atmosenv.2014.07.043
Sulaiman, L. H. (2017). Haze: An Overview of Public Health Action, Gaps and Challenges to Protect Public. Kuala Lumpur. Available online at: https://www.akademisains.gov.my/asm-publication/report-of-the-forum-on-the-impact-of-haze-on-human-health-in-malaysia/ (accessed June 30, 2021).
Wang, T. N., Ko, Y. C., Chao, Y. Y., Huang, C. C., and Lin, R. S. (1999). Association between indoor and outdoor air pollution and adolescent Asthma from 1995 to 1996 in Taiwan. Environ. Res. 81, 239–247. doi: 10.1006/enrs.1999.3985
WHO (2016). ICD-10. WHO. Available online at: http://apps.who.int/classifications/icd10/browse/2016/en#/J40-J47 (accessed April 8, 2021).
Keywords: haze, air pollution, healthcare, utilization, respiratory
Citation: Jaafar H, Azzeri A, Isahak M and Dahlui M (2021) The Impact of Haze on Healthcare Utilizations for Acute Respiratory Diseases: Evidence From Malaysia. Front. Ecol. Evol. 9:764300. doi: 10.3389/fevo.2021.764300
Received: 25 August 2021; Accepted: 08 November 2021;
Published: 30 November 2021.
Edited by:
Emily J. Flies, University of Tasmania, AustraliaReviewed by:
Ana Monteiro, University of Porto, PortugalIan Douglas, The University of Manchester, United Kingdom
Copyright © 2021 Jaafar, Azzeri, Isahak and Dahlui. This is an open-access article distributed under the terms of the Creative Commons Attribution License (CC BY). The use, distribution or reproduction in other forums is permitted, provided the original author(s) and the copyright owner(s) are credited and that the original publication in this journal is cited, in accordance with accepted academic practice. No use, distribution or reproduction is permitted which does not comply with these terms.
*Correspondence: Hafiz Jaafar, ZHIuaGFmaXpqYWFmYXJAdXNpbS5lZHUubXk=