- 1Department of Mathematics and Statistics, University of Ottawa, Ottawa, ON, Canada
- 2Department of Biology, McGill University, Montreal, QC, Canada
- 3Centre for Biodiversity Theory and Modelling, Theoretical and Experimental Ecology Station, French National Centre for Scientific Research (CNRS), Moulis, France
- 4French National Institute for Agriculture, Food, and Environment (INRAE), Aquatic Ecosystems and Global Change Research Unit, Cestas, France
First derivations of the functional response were mechanistic, but subsequent uses of these functions tended to be phenomenological. Further understanding of the mechanisms underpinning predator-prey relationships might lead to novel insights into functional response in natural systems. Because recent consideration of the physical properties of the environment has improved our understanding of predator-prey interactions, we advocate the use of physics-based approaches for the derivation of the functional response from first principles. These physical factors affect the functional response by constraining the ability of both predators and prey to move according to their size. A physics-based derivation of the functional response should thus consider the movement of organisms in relation to their physical environment. One recent article presents a model along these criteria. As an initial validation of our claim, we use a slightly modified version of this model to derive the classical parameters of the functional response (i.e., attack rate and handling time) of aquatic organisms, as affected by body size, buoyancy, water density and viscosity. We compared the predictions to relevant data. Our model provided good fit for most parameters, but failed to predict handling time. Remarkably, this is the only parameter whose derivation did not rely on physical principles. Parameters in the model were not estimated from observational data. Hence, systematic discrepancies between predictions and real data point immediately to errors in the model. An added benefit to functional response derivation from physical principles is thus to provide easy ways to validate or falsify hypotheses about predator-prey relationships.
Introduction
The study of prey consumption by a predator (i.e., the functional response) began several decades ago (Gause, 1934; Gause et al., 1936) and was accompanied by the development of a theoretical framework based on mechanistic principles (Lotka, 1923; Volterra, 1926; Beverton and Holt, 1957; Watt, 1959). The model proposed by Holling (1959, 1961, 1966) is one of the best known. This mechanistic model defined fundamental parameters such as attack rate (the rate at which a predator encounters and captures prey) and handling time (the time needed by the predator to subdue, ingest and digest the captured prey, and during which the predator cannot attack another prey). These parameters can be measured concomitantly, and they give information about factors that constrain predation on a given prey, which is a strength of this mechanistic approach.
Holling’s type-I, II and III models and subsequently derived models (e.g., Rogers, 1972) are still widely used as a framework to derive the values of attack rate and handling time from empirical data (e.g., Andresen and van der Meer, 2010; Farhadi et al., 2010; Papanikolaou et al., 2011). These approaches give valuable information on the studied systems, and they allow hypothesis testing, such as the effects of temperature (Archer et al., 2019) and predator satiation (Li et al., 2018) on the functional response. However, these studies have been mostly carried out in the laboratory, where many external factors do not play a role (Abrams, 1982). Hence, the results are hard to generalize and transpose to natural situations. Nonetheless, Holling’s model has been a very successful approach founded on mechanistic principles.
Several studies have investigated the role played by specific factors known to affect the functional response, such as feeding saturation (DeAngelis et al., 1975) and interactions between predators (Beddington, 1975; Sih, 1979). In particular, the body size of both predator and prey are known to strongly affect the functional response (Aljetlawi et al., 2004; Vucic-Pestic et al., 2010). Body size is a good predictor of trophic position (Miller et al., 1992; Williams et al., 2010) and affects the overall dynamics of the interaction (Yodzis and Innes, 1992). Strikingly, the surrounding physical medium remains absent or, at least, only implicit in most studies, despite the tight relationship between body size and the physical environment as experienced by the organism (Purcell, 1977; Bonner, 2006). Although, in his pioneer work, Tansley (1935) stated that organisms should not be separated from their “special environment, with which they form one physical system,” the role played by the physical medium in constraining the functional response remains largely unexplored. In the present paper, we argue that including physical features into predator-prey models is likely to lead to novel insights about species interactions.
Empirical Evidence of the Impact of the Physical Properties of the Medium on the Functional Response
Early developments of the functional response theory considered physical factors, notably spatial heterogeneity, only implicitly (Hardman and Turnbull, 1974), with the notable exception of temperature (Mack et al., 1981). However, experimental investigation of the effect of one or the other physical property of the environment can be found here and there in the literature.
Temperature, the most thoroughly investigated factor, has been found to affect both attack rate and handling time, although its effects may vary according to the taxonomic group of the consumer, and the dimensionality of the interaction (Uiterwaal and DeLong, 2020). Turbulence, another reasonably well-studied factor in aquatic habitats, was found to affect predator attack rate of small aquatic predators, especially when prey abundance is low (MacKenzie and Kiørboe, 1995), although this effect seems to vary with feeding modes (Saiz et al., 2003). Medium viscosity is another factor that is known to affect feeding efficiency of planktonic predators by modifying their mobility, which in turn affects predator-prey encounter rate (Luckinbill, 1973; Tyrell and Fisher, 2019). Last, turbidity is an important factor for predators relying on visual cues to detect their prey, as it is likely to affect predator-prey encounter rate (Turesson and Brönmark, 2007).
This short overview shows that experimental investigation of the physical dimension of functional responses is far from being exhaustive, or even well advanced, with the notable exceptions of temperature, and to a lesser extent, turbulence. Perhaps lacking is a comprehensive theoretical framework that would provide the impetus for empirical studies that would reach beyond the specific interest of the various investigators of the functional response.
Theoretical Approaches to the Role of Physical Features of the Environment in Predation
Previous studies that have considered the surrounding medium have usually focused on specific aspects of predation or on specific taxa (Domenici et al., 2011), or have investigated one specific aspect of the medium such as dimensionality (Pawar et al., 2012, 2015) or habitat complexity (Barrios-O’Neill et al., 2016), more rarely two factors simultaneously (Wasserman et al., 2016). But the overall role played by the surrounding medium acting on the predator-prey relationship, which drives the functional response, remains to be explored.
Clearly, living organisms are constrained by the physical properties of the surrounding medium (Denny, 1993, 2016; Vogel, 1996). These properties affect the way organisms move and/or interact with each other in different ways. For example, we already reviewed some of the evidence in aquatic systems showing that turbidity is an essential factor for predator or prey that rely on visual cues to detect each other (Martens et al., 2015). Another example was turbulence, which controls many planktonic organisms’ suspension within the water column (Rodríguez et al., 2001) and affects contact rate between predators and prey (Kiørboe and Saiz, 1995).
More fundamental are those factors that are typically mechanical (i.e., gravity, density and viscosity). These mechanical factors are ubiquitous, affect small (Kiørboe and Saiz, 1995) as well as large predators (Howland, 1974; Domenici et al., 2007) and are usually size-dependent. Since predation usually implies motion, these factors create mechanical constraints acting differently on predators in different physical environments (Cloyed et al., 2021). Clearly, aquatic organisms do not experience the effects of gravity as terrestrial organisms usually do because the medium density is much higher in water than in air, which creates higher buoyancy. Moreover, medium viscosity and density affect species’ motion according to body size through drag (Beveridge et al., 2010a,b), which is why the motion of planktonic organisms has very different features than that of larger organisms. Metrics such as the Reynolds number are commonly used to discriminate between organisms that experience viscous drag (low Reynolds number) and those that experience high inertia (high Reynolds number). These features affect species according to their size and shape (Koehl and Strickier, 1981; Koehl, 1996). Thus, incorporating mechanical constraints into models could lead to a better understanding of the size-based relationship between predators and prey, and hence of the size structure of food webs.
Due to this size dependence, models incorporating physical (including mechanical) factors into predation merge size-related biological and mechanical constraints in classical predator-prey systems. Several studies have begun to investigate this promising avenue. For example, the dimensionality of the physical medium was shown to constrain predator-prey interactions since predators are expected to capture pelagic and flying prey more efficiently than benthic and terrestrial prey (Pawar et al., 2012). Extending this framework to predict pairwise trophic interactions in natural situations, Pawar et al. (2019) fall short of deriving the parameters of their functional response model from physical factors other than dimensionality. Despite this narrow scope, their model successfully reproduces some important differences in the consumer-resource size structure of 2D versus 3D communities. However, dimensionality is only one feature of the physical medium. Some studies coupled several physical properties of the medium simultaneously in a plankton model (Baird and Emsley, 1999), including their effects on different resource-use strategies, such as photosynthesis, nutrient uptake and predation (Baird et al., 2006). Addition of these biomechanical mechanisms correctly predicted emergent ecosystem properties, such as deep chlorophyll maxima, where non-biomechanical models were unable to do so (Baird et al., 2004). This additional realism was due specifically to the inclusion of effects of hydromechanical processes such as advection and turbulent dissipation on planktonic organisms (Baird et al., 2004, 2006). This kind of approach was later extended to marine food webs using an oceanographic model, which proved interesting in its capacity to generate realistic food webs with relatively few generic rules (Baird and Suthers, 2007). But the validation of the model assumptions at a scale smaller than the ecosystem was less successful, due to the discrepancy between the small size of planktonic organisms, and the scale at which the model was applied (ocean basins and currents). Similarly, a framework for predicting the optimal motion of larger organisms as a function of size and internal and external factors is under development (Wilson et al., 2013, 2015). The importance of physical factors in determining motion has been acknowledged (Wilson et al., 2015), but their explicit and quantitative inclusion in this framework has started only very recently (Portalier et al., 2019). As successful as these milestone models have been, they did not provide for a mechanistically derived functional response, applicable over a wide range of different organisms and of well-defined physical conditions. However, we feel that their contributions bring the field to the brink of such a realization.
As an illustration to how the functional response can be derived from such models that consider physical factors explicitly, we present in the next section our own derivation of the functional response, that results from just a slight modification of Portalier et al.’s (2019) model. We see this derivation only as a first step, since only a handful of physical factors are considered (gravity, viscosity, and medium density). More work will be needed in order to integrate the other important factors, such as dimensionality and turbulence. Meanwhile, we conducted a comparison of the model predictions with actual data for aquatic organisms, with the hope that systematic deviations between observed and predicted data would reveal shortcomings of the model and thus point out to the next advances to pursue.
A First Case of an Inferring of the Functional Response From the Physical Properties of the Medium
In a recent study, Portalier et al. (2019) provided a biomechanical model that uses general laws of mechanics and well-known biological laws, all related to body size, to predict predator − prey interactions. This model predicts the occurrence of trophic links (e.g., the model predicts more than 80% of the predator-prey interactions in pelagic systems). It also provides a detailed mechanism for predation, where predators have to move around for searching, capturing and handling their prey. All these aspects depend on the body masses of both the predator and its prey. The model therefore provides values for encounter rate, capture time, and handling time, as well as energetic expenditure for the predator, but only at one nominal population density of the prey. In the present model, we apply the model to a range of prey abundance, and we focus on the time expenditure only (not energetic expenditure). The parameters of the functional response can be immediately computed from this biomechanical model. Hence, this model provides a novel method to parameterize a functional response based on individual traits, and on using mechanical laws. The biomechanical model assumes that both the predator and the prey can detect each other without any interference. This is why it is well suited for pelagic organisms. Benthic organisms living in two dimensions experience a more complex environment and would require additional features to be modeled.
The original model predicts the potential of predation to take place successfully. It does so by including the physical features of the medium: acceleration due to gravity, body density, medium density, and medium viscosity. Then, the model computes all the necessary information to predict feasible predator-prey interactions (i.e., encounter rate, capture probability, handling time and net energy gain for the predator).
Predation is broken down into three successive sequences: a predator needs to search, capture, and then handle its prey. Each predation sequence leads to a time expenditure and requires motion. Following the idea developed by Bejan and Marden (2006), motion is modeled as an oscillatory process that is decomposed into three sequences. First, an organismal stroke leads to a thrust that propels the body upwards (following Archimedes’ force, but facing gravity and drag (D) due to density and viscosity) and forwards (facing drag).
Relative speed of the predator and prey is a nexus in the model, because it determines whether the two organisms encounter and whether the one captures the other successfully. It is also the only calculated function that includes the effects of physical factors in the model because it is possible to numerically derive vertical speed from simple mechanical laws:
where v is instantaneous vertical speed, FMv is thrust vertical force, Mb is body mass, g is acceleration due to gravity, Vb is body volume, ρ is medium density, D is drag that varies with speed, body mass, density, and medium viscosity (μ). Second, when stroke ends, the body continues its ascending movement by inertia until it stops.
Third, the body returns by inertia to its original vertical position.
During this vertical oscillation, the body moves forward compared to its original horizontal position over a distance that depends on the forward component of thrust. The instantaneous horizontal speed can be derived using a method similar to vertical speed, but it considers only thrust and drag (see Supplementary Material for more details). Then, another sequence begins. The model computes the thrust force needed to propel the body (which is constrained by body size), the horizontal distance covered, the speed and the associated energetic cost that maximizes the probability to capture a prey, and the net energy gain from its consumption. We tested the model’s goodness of fit by computing the root mean squared deviation (RMSD) that represents the mean deviation of the predicted versus observed data. In addition, we checked for model bias by testing whether the slope and intercept of the regression of Observed versus Predicted data (OP) do not significantly differ from 1 and 0, respectively, and added body size as a cofactor. Predicted speeds fit data well (Figure 1, RMSD = 7.65). The model does not show any significant bias (i.e., OP slope and intercept do not significantly differ from 1 (p = 0.707) and 0 (p = 0.283), respectively, with no significant bias due to body size (p > 0.19, see Supplementary Material). Notice that the model did not include a constraint due to limitations of quickly available energy for the speed of large animals in our model (as Hirt et al., 2017 did). However, it will be an interesting aspect to consider in the future.
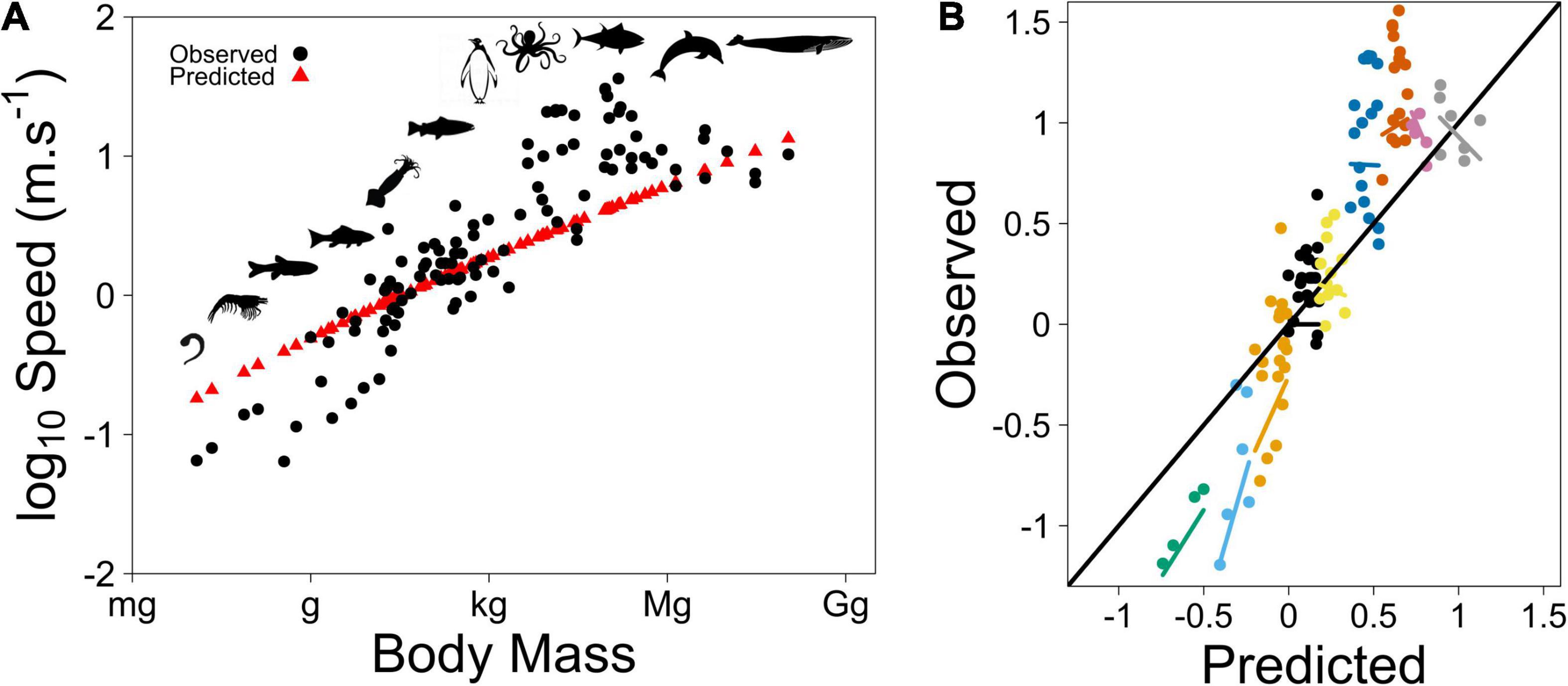
Figure 1. (A) Species-specific speed according to body size for organisms moving in aquatic systems. Speed increases with body size, since overall muscular power generating thrust increases with size. Despite variation among species, the predicted speed fits data well [data from Hirt et al. (2017)]. (B) Observed versus predicted data. Black line has a slope of 1 and intercept of 0. Color points represent the different size ranges. Colored lines are (non-significant) regression lines of the corresponding points.
Predation on a given prey requires first its encounter, followed by capture and finally handling. Encounter rate is determined by the speeds of the predator and prey calculated in the model (see above), and then used in a formula according to Rothschild and Osborn (1988). The relative speed between the predator and the prey calculated at the time of capture also determines the probability of capture (and therefore the total time for searching a prey that leads to a successful capture), and time for capture. Both predator and prey follow the same rules, with the difference that the prey only maximizes its probability to escape predation. Search time (ts) represents the time needed by a predator to contact a prey that leads to a successful capture (e.g., if the capture probability is 0.5, then the predator needs to contact a prey twice on average to successfully capture it). Capture time (tc) is the time needed to move toward a prey once detected and seize it. Last, handling time (th) is the time needed to consume and digest the prey. Handling time is the only component in the model of the functional response that is independent of speed and thus the mechanical factors mentioned above, although effects in reality cannot be totally brushed aside. It is also known to vary with other physical factors such as temperature (Rall et al., 2012). The functional response [f(N)] is defined as the inverse of the time needed for searching, capturing and handling one unit of prey of abundance N. The function may be written as follows (see Supplementary Material).
βPc represents the attack rate, where β is the encounter rate (constrained by predator and prey speeds), and Pc is the capture probability. Capture time and handling time are taken into account instead of handling time only. Under this form, one can recognize a modified version of Holling’s (1961) disk equation.
Given the assumptions made on the encounter rate (see Supplementary Material), the functional response behaves as a type-II response. However, Eq. 4 is flexible enough to allow for a type-III response, but it would require the addition of mechanisms to make the encounter rate dependent on the population density of the prey. All parameter values change according to both predator and prey sizes, while attack rate, capture probability and capture time also vary with the mechanical properties of the medium.
Case Study: Validation of the Model and Interpretation
Data were collected to test predictions from the model. Most data come from two meta-analyses (Hirt et al., 2017; Li et al., 2018), as well as our own literature search. To be pertinent, data have to mention predator and prey sizes explicitly. Most data are individual-based, which means that two individuals from the same species but with different sizes are treated separately. We computed the RMSD, and we tested whether the slope and intercept of the OP regression were significantly different from 1 and 0 respectively (see above). Body size was added as a cofactor (except for capture rate as the range of predator size in the dataset was not wide enough and was unbalanced), and the source of data (i.e., the original study where the data comes from) as a random factor.
Predicted attack rate, capture probability and handling time were compared to real data coming from aquatic systems (Figure 2). It appears that the model fits the data quite well for attack rate [RMSD = 1.2e-4, OP slope and intercept do not significantly differ from 1 (p = 0.19) and 0 (p = 0.16), respectively, and no significant bias in the model due to body size (p > 0.16), except for predators of size around 10 mg (p = 0.01), and no effect of the source of data, see Supplementary Material] and capture probability [RMSD = 0.23, OP slope and intercept do not significantly differ from 1 (p = 0.775) and 0 (p = 0.49), respectively]. Linking mechanical features from the medium and body size allows a good estimate of attack rate and capture probability for pelagic predators, without the need to extrapolate from data already collected. However, handling time is poorly estimated by the model, especially for small predators [RMSD = 559315.8, OP slope and intercept are significantly different from 1 (p < 2e-16) and 0 (p = 1.16e-7), respectively, body size has also a significant effect (p < 0.05), but not the source of data]. The discrepancies among predator sizes open the door to many hypotheses that remain to be tested. Note that handling time is not dependent on mechanical features of the medium in Portalier et al. (2019) but is determined only by physiological arguments and allometric laws. Thus, the results suggest that the relationship between predator size, prey size and handling time is driven by a more complex set of allometric laws that differ between small and large predators (Emerson et al., 1994), or that other factors affect handling time according to the size of the predators. Some studies also suggested that handling time may not be static for a given predator, but vary with prey abundance (Okuyama, 2010). These are examples of potential mechanisms that could be added to the model in the future.
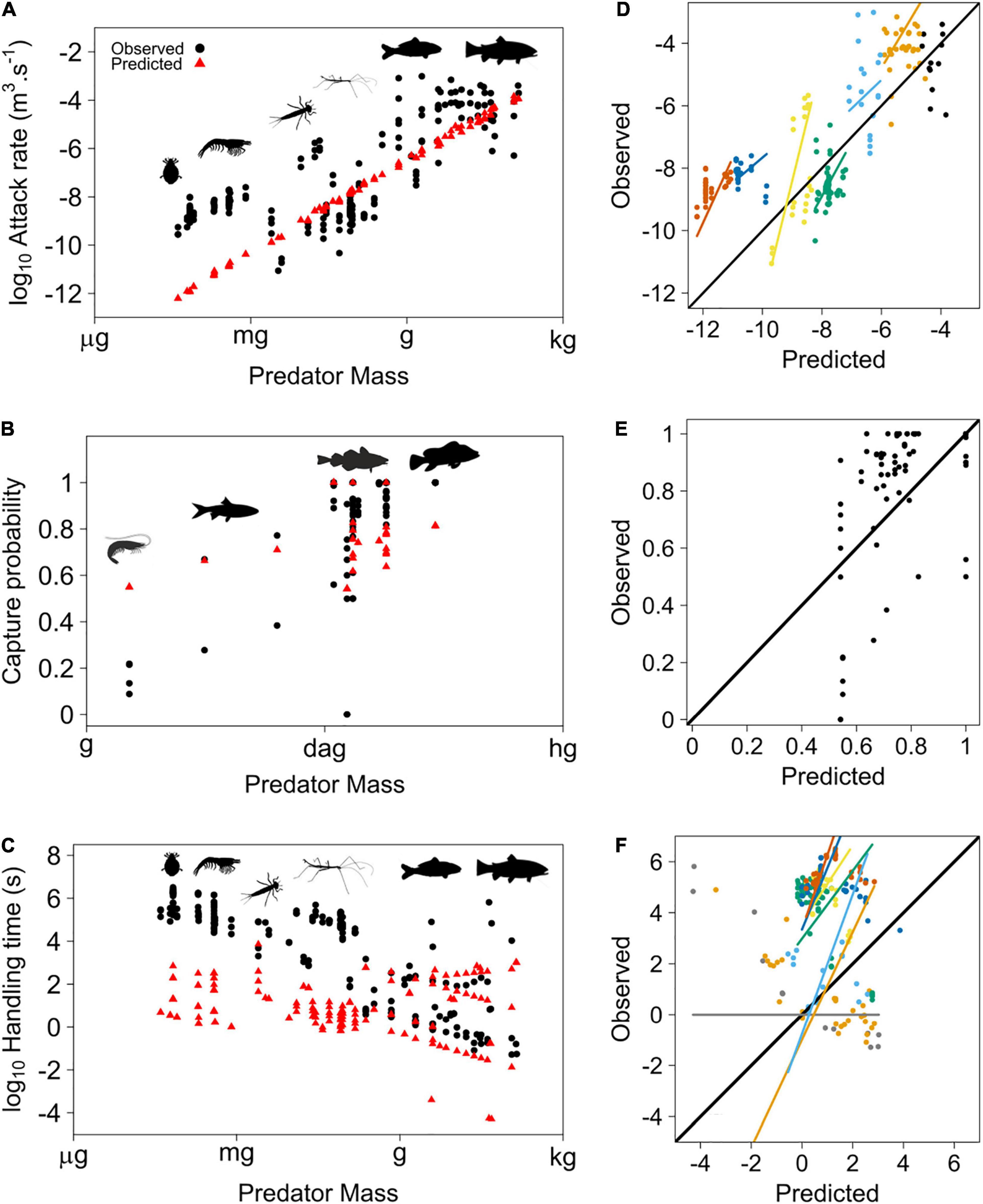
Figure 2. Predator attack rate (A), capture probability (B) and handling time (C) according to predator mass in aquatic systems. The model fits the data quite well for attack rate (except for very small organisms) and capture probability. However, data show some variability. Predictions for handling time are more accurate for relatively large predators than for smaller predators. This suggests that more investigations are needed in order to understand how mechanical factors constrain handling time for predators according to predator and prey sizes. (D–F) are the observed versus predicted data (same as Figure 1) for attack rate, capture probability and handling time, respectively. The colored regression lines are non-significant in (D), but significant in (F).
Conclusion and Future Directions
Although theoretical considerations of the physical properties of the medium in the study of predator-prey interactions are still in their early stages of development, they provide novel understanding and good fit to various aspects of the interaction.
Here, we applied one of the models to generate quantitative predictions for the parameters of the functional response of aquatic metazoans. The model we chose used fundamental mechanical properties of the medium to develop a mechanistic approach to the functional response. However, it considers only a limited set of physical factors. The model could be improved in several ways. Future studies could include additional physical factors such as dimensionality, hydrodynamics and temperature, which affect the physical properties of the medium (MacKenzie and Kiørboe, 1995; Larsen and Riisgård, 2009; Uiterwaal and DeLong, 2020), and organisms’ metabolism (Brown et al., 2004). They could also consider factors that affect prey detection such as light and chemical cues. These factors diffuse differently in air and water, and the perception ability of predators seems to be related to size (Martens et al., 2015). This novel framework is promising because it provides easy ways to validate or falsify hypotheses. Hence, any discrepancy between predictions and real data points immediately toward an error in the model, or it means that important mechanisms are missing (as shown for handling time in our case study). It can also suggest novel hypotheses to be empirically or theoretically tested.
In our model, the processes based on mechanical factors (i.e., speed, attack rate, capture probability) fit data well, although discrepancies occur at low predator sizes, which suggests that further refinements are needed. Handling time shows the lowest goodness of fit, and it is the only one that does not include any mechanical factors. A better mechanism for handling is thus needed. Ingestion has received some attention in the existing literature, especially for aquatic organisms (Holzman et al., 2012). Mechanisms driving digestion have also received some attention. For example, there are models of gut motility according to prey size and gut volume (Salvanes et al., 1995), although they usually do not include physical factors from the medium that may affect the process (e.g., temperature, pressure). However, both ingestion and digestion models might be difficult to generalize to a large variety of species (and sizes). Moreover, other aspects of handling time are likely to play a role. For instance, prey subjugation before ingestion is an essential aspect. Unfortunately, studies on this topic seem to focus either on dangerous (e.g., poisonous) prey (Mukherjee and Heithaus, 2013), or on specific species (Schatz et al., 1997), which makes them difficult to generalize. Last, predator satiation or hunger remains a fundamental aspect of predator activity (Jeschke et al., 2002; Jeschke, 2007). While it has been included in several studies, its underpinning processes remain to be modeled. Therefore, a generic mechanical description of handling that would cover its different components and be valid across a wide range of sizes would represent a significant improvement.
Similarly, the foraging mode of predators is also an important topic. Portalier et al.’s (2019) model assumes that both the predator and the prey are active and can detect each other without any interference. However, these assumptions are not valid for sit-and-wait predators (Kiørboe, 2011; Twardochleb et al., 2020). Indeed, the model can compute encounter rate between a moving prey and a non-moving predator by setting the speed of the predator to zero. But additional behavioral aspects (such as camouflage) would require additional features to the model. While these behavioral aspects are not related to size, they point to ways the model could be improved.
More generally, the strength of this kind of approach is to derive patterns at the community level from rules acting at the individual level within physical context of their environment. Thus, the functional response predicted is an emerging property of the ecosystem. One could even go further by including other aspects associated to predation such as behavioral features (e.g., predator avoidance, interference between predators, social aspects) that were already considered by Holling (1966). This approach opens up a promising avenue for new studies that would merge the biological and the physical component of the ecosystem.
Data Availability Statement
The data presented in the study are deposited in the Zenodo Repository, accession number https://doi.org/10.5281/zenodo.5781790 (Portalier et al., 2021a). The Matlab code used to implement the model is deposited in the Zenodo Repository, accession number https://doi.org/10.5281/zenodo.5781805 (Portalier et al., 2021b).
Author Contributions
SP led the writing of the manuscript, implemented the model, gathered data, and performed simulations and analysis. SP and MC led the revision of the manuscript. MC, GF, and ML provided conceptual advices and guidance. All authors discussed the results and contributed equally to earlier drafts.
Funding
SP received funding from the Healthy Forest Partnership. ML was supported by the TULIP Laboratory of Excellence (ANR-10-LABX-41).
Conflict of Interest
The authors declare that the research was conducted in the absence of any commercial or financial relationships that could be construed as a potential conflict of interest.
Publisher’s Note
All claims expressed in this article are solely those of the authors and do not necessarily represent those of their affiliated organizations, or those of the publisher, the editors and the reviewers. Any product that may be evaluated in this article, or claim that may be made by its manufacturer, is not guaranteed or endorsed by the publisher.
Supplementary Material
The Supplementary Material for this article can be found online at: https://www.frontiersin.org/articles/10.3389/fevo.2021.761984/full#supplementary-material
References
Abrams, P. A. (1982). Functional responses of optimal foragers. Am. Nat. 120, 382–390. doi: 10.1086/283996
Aljetlawi, A. A., Sparrevik, E., and Leonardsson, K. (2004). Prey-predator size-dependent functional response: derivation and rescaling to the real world. J. Anim. Ecol. 73, 239–252. doi: 10.1111/j.0021-8790.2004.00800.x
Andresen, H., and van der Meer, J. (2010). Brown shrimp (Crangon crangon, L.) functional response to density of different sized juvenile bivalves Macoma balthica (L.). J. Exp. Mar. Biol. Ecol. 390, 31–38. doi: 10.1016/j.jembe.2010.04.027
Archer, L. C., Sohlström, E. H., Gallo, B., Jochum, M., Woodward, G., Kordas, R. L., et al. (2019). Consistent temperature dependence of functional response parameters and their use in predicting population abundance. J. Anim. Ecol. 88, 1670–1683. doi: 10.1111/1365-2656.13060
Baird, M., and Emsley, S. M. (1999). Towards a mechanistic model of plankton population dynamics. J. Plankton Res. 21, 85–126. doi: 10.1093/plankt/21.1.85
Baird, M. E., and Suthers, I. M. (2007). A size-resolved pelagic ecosystem model. Ecol. Model. 203, 185–203. doi: 10.1016/j.ecolmodel.2006.11.025
Baird, M. E., Oke, P. R., Suthers, I. M., and Middleton, J. H. (2004). A plankton population model with biomechanical descriptions of biological processes in an idealised 2D ocean basin. J. Mar. Syst. 50, 199–222. doi: 10.1016/j.jmarsys.2004.02.002
Baird, M. E., Timko, P. G., Suthers, I. M., and Middleton, J. H. (2006). Coupled physical-biological modelling study of the East Australian current with idealised wind forcing. Part I: biological model intercomparison. J. Mar. Syst. 59, 249–270. doi: 10.1016/j.jmarsys.2005.09.005
Barrios-O’Neill, D., Kelly, R., Dick, J. T. A., Ricciardi, A., MacIsaac, H. J., and Emmerson, M. C. (2016). On the context-dependent scaling of consumer feeding rates. Ecol. Lett. 19, 668–678. doi: 10.1111/ele.12605
Beddington, J. R. (1975). Mutual interference between parasites or predators and its effect on searching efficiency. J. Anim. Ecol. 44, 331–340. doi: 10.2307/3866
Bejan, A., and Marden, J. H. (2006). Unifying constructal theory for scale effects in running, swimming and flying. J. Exp. Biol. 209, 238–248. doi: 10.1242/jeb.01974
Beveridge, O. S., Petchey, O. L., and Humphries, S. (2010a). Direct and indirect effects of temperature on the population dynamics and ecosystem functioning of aquatic microbial ecosystems. J. Anim. Ecol. 79, 1324–1331. doi: 10.1111/J.1365-2656.2010.01741.X
Beveridge, O. S., Petchey, O. L., and Humphries, S. (2010b). Mechanisms of temperature-dependent swimming: the importance of physics, physiology and body size in determining protist swimming speed. J. Exp. Biol. 213, 4223–4231. doi: 10.1242/JEB.045435
Beverton, R. J. H., and Holt, S. J. (1957). On the dynamics of exploited fish population. Fish. Investig. 19, 1–533.
Bonner, J. T. (2006). Why Size Matters. Princeton: Princeton University Press, doi: 10.1515/9781400837557
Brown, J. H., Gillooly, J. F., Allen, A. P., Savage, V. M., and West, G. B. (2004). Toward a metabolic theory of ecology. Ecology 85, 1771–1789. doi: 10.1890/03-9000
Cloyed, C. S., Grady, J. M., Savage, V. M., Uyeda, J. C., and Dell, A. I. (2021). The allometry of locomotion. Ecology 102:e03369. doi: 10.1002/ECY.3369
DeAngelis, D. L., Goldstein, R. A., and O’Neill, R. V. (1975). A model for tropic interaction. Ecology 56, 881–892. doi: 10.2307/1936298
Denny, M. W. (1993). Air and Water: The Biology and Physics of Life’s Media. Princeton, NJ: Princeton University Press.
Denny, M. W. (2016). Ecological Mechanics: Principles of Life’s Physical Interactions. Princeton, NJ: Princeton University Press.
Domenici, P., Blagburn, J. M., and Bacon, J. P. (2011). Animal escapology I: theoretical issues and emerging trends in escape trajectories. J. Exp. Biol. 214, 2463–2473. doi: 10.1242/jeb.029652
Domenici, P., Claireaux, G., and McKenzie, D. J. (2007). Environmental constraints upon locomotion and predator-prey interactions in aquatic organisms: an introduction. Philos. Trans. R. Soc. Lond. B Biol. Sci. 362, 1929–1936. doi: 10.1098/rstb.2007.2078
Emerson, S. B., Greene, H. W., and Charnov, E. L. (1994). “Allometric aspects of predator-prey interactions,” in Ecological Morphology: Integrative Organismal Biology, eds P. C. Wainwright and S. M. Reilly (Chicago, IL: University of Chicago Press), 123–139. doi: 10.1111/ele.12147
Farhadi, R., Allahyari, H., and Juliano, S. A. (2010). Functional response of larval and adult stages of Hippodamia variegata (Coleoptera: Coccinellidae) to different densities of Aphis fabae (Hemiptera: Aphididae). Environ. Entomol. 39, 1586–1592. doi: 10.1603/EN09285
Gause, G. F., Smaragdova, N. P., and Witt, A. A. (1936). Further studies of interaction between predators and prey. J. Anim. Ecol. 5, 1–18. doi: 10.2307/1087
Hardman, J. M., and Turnbull, A. L. (1974). The interaction of spatial heterogeneity, predator competition and the functional response to prey density in a laboratory system of wolf spiders (Araneae: Lycosidae) and fruit flies (Diptera: Drosophilidae). J. Anim. Ecol. 43, 155–171. doi: 10.2307/3164
Hirt, M. R., Jetz, W., Rall, B. C., and Brose, U. (2017). A general scaling law reveals why the largest animals are not the fastest. Nat. Ecol. Evol. 1, 1116–1122. doi: 10.1038/s41559-017-0241-4
Holling, C. S. (1959). The components of predation as revealed by a study of small-mammal predation of the european pine Sawfly1. Can. Entomol. 91, 293–320. doi: 10.4039/ENT91293-5
Holling, C. S. (1961). Principles of insect predation. Annu. Rev. Entomol. 6, 163–182. doi: 10.1146/annurev.en.06.010161.001115
Holling, C. S. (1966). The functional response of invertebrate predators to prey density. Mem. Entomol. Soc. Canada 98, 5–86. doi: 10.4039/entm9848fv
Holzman, R., Collar, D. C., Mehta, R. S., and Wainwright, P. C. (2012). An integrative modeling approach to elucidate suction-feeding performance. J. Exp. Biol. 215, 1–13. doi: 10.1242/jeb.057851
Howland, H. C. (1974). Optimal strategies for predator avoidance: the relative importance of speed and manoeuvrability. J. Theor. Biol. 47, 333–350. doi: 10.1016/0022-5193(74)90202-1
Jeschke, J. M. (2007). When carnivores are “full and lazy”. Oecologia 152, 357–364. doi: 10.1007/S00442-006-0654-2
Jeschke, J. M., Kopp, M., and Tollrian, R. (2002). Predator functional responses: discriminating between handling and digesting prey. Ecol. Monogr. 72, 95–112. doi: 10.1890/0012-9615(2002)072[0095:pfrdbh]2.0.co;2
Kiørboe, T. (2011). How zooplankton feed: mechanisms, traits and trade-offs. Biol. Rev. 86, 311–339. doi: 10.1111/j.1469-185X.2010.00148.x
Kiørboe, T., and Saiz, E. (1995). Planktivorous feeding in calm and turbulent environments, with emphasis on copepods. Mar. Ecol. Prog. Ser. 122, 135–145. doi: 10.3354/meps122135
Koehl, M. A. R. (1996). When does morphology matter? Annu. Rev. Ecol. Syst. 27, 501–542. doi: 10.1146/annurev.ecolsys.27.1.501
Koehl, M. A. R., and Strickier, J. R. (1981). Copepod feeding currents: food capture at low Reynolds number. Limnol. Oceanogr. 26, 1062–1073. doi: 10.4319/lo.1981.26.6.1062
Larsen, P. S., and Riisgård, H. U. (2009). Viscosity and not biological mechanisms often controls the effects of temperature on ciliary activity and swimming velocity of small aquatic organisms. J. Exp. Mar. Biol. Ecol. 381, 67–73.
Li, Y., Rall, B. C., and Kalinkat, G. (2018). Experimental duration and predator satiation levels systematically affect functional response parameters. Oikos 127, 590–598. doi: 10.1111/oik.04479
Lotka, A. J. (1923). Contribution to quantitative parasitology. J. Washingt. Acad. Sci. 13, 152–158.
Luckinbill, L. S. (1973). Coexistence in laboratory populations of paramecium aurelia and its predator Didinium nasutum. Ecology 54, 1320–1327. doi: 10.2307/1934194
Mack, T. P., Bajusz, B. A., Nolan, E. S., and Smilowitz, Z. (1981). Development of a temperature-mediated functional response equation. Environ. Entomol. 10, 573–579. doi: 10.1093/EE/10.5.573
MacKenzie, B. R., and Kiørboe, T. (1995). Encounter rates and swimming behavior of pause-travel and cruise larval fish predators in calm and turbulent laboratory environments. Limnol. Oceanogr. 40, 1278–1289. doi: 10.4319/LO.1995.40.7.1278
Martens, E. A., Wadhwa, N., Jacobsen, N. S., Lindemann, C., Andersen, K. H., and Visser, A. (2015). Size structures sensory hierarchy in ocean life. Proc. R. Soc. B 282:20151346. doi: 10.1098/rspb.2015.1346
Miller, T. J., Crowder, L. B., Rice, J. A., and Binkowski, F. P. (1992). Body size and the ontogeny of the functional response in fishes. Can. J. Fish. Aquat. Sci. 49, 805–812. doi: 10.1139/F92-091
Mukherjee, S., and Heithaus, M. R. (2013). Dangerous prey and daring predators: a review. Biol. Rev. 88, 550–563. doi: 10.1111/brv.12014
Okuyama, T. (2010). Prey density-dependent handling time in a predator-prey model. Commun. Ecol. 11, 91–96. doi: 10.1556/ComEc.11.2010.1.13
Papanikolaou, N. E., Martinou, A. F., Kontodimas, D. C., Matsinos, Y. G., and Milonas, P. G. (2011). Functional responses of immature stages of Propylea quatuordecimpunctata (Coleoptera: Coccinellidae) to Aphis fabae (Hemiptera: Aphididae). Eur. J. Entomol. 108:391. doi: 10.14411/eje.2011.049
Pawar, S., Dell, A. I., Lin, T., Wieczynski, D. J., and Savage, V. M. (2019). Interaction dimensionality scales up to generate bimodal consumer-resource size-ratio distributions in ecological communities. Front. Ecol. Evol. 7:202. doi: 10.3389/FEVO.2019.00202
Pawar, S., Dell, A. I., and Savage, V. M. (2012). Dimensionality of consumer search space drives trophic interaction strengths. Nature 486, 485–489. doi: 10.1038/nature11131
Pawar, S., Dell, A. I., and Savage, V. M. (2015). “From metabolic constraints on individuals to the dynamics of ecosystems,” in Aquatic Functional Biodiversity: An Ecological and Evolutionary Perspective, eds A. Belgrano, G. Woodward, and U. Jacob (Amsterdam: Elsevier Inc), 3–36. doi: 10.1016/b978-0-12-417015-5.00001-3
Portalier, S. M. J., Fussmann, G. F., Loreau, M., and Cherif, M. (2019). The mechanics of predator-prey interactions: first principles of physics predict predator-prey size ratios. Funct. Ecol. 33, 323–334. doi: 10.1111/1365-2435.13254
Portalier, S. M. J., Fussmann, G. F., Loreau, M., and Cherif, M. (2021a). Inferring size-based functional responses from the physical properties of the medium. Front. Ecol. Evol. doi: 10.5281/zenodo.5781790
Portalier, S. M. J., Fussmann, G. F., Loreau, M., and Cherif, M. (2021b). Inferring size-based functional responses from the physical properties of the medium. Front. Ecol. Evol. doi: 10.5281/zenodo.5781805
Rall, B. C., Brose, U., Hartvig, M., Kalinkat, G., Schwarzmuller, F., Vucic-Pestic, O., et al. (2012). Universal temperature and body-mass scaling of feeding rates. Philos. Trans. R. Soc. B Biol. Sci. 367, 2923–2934. doi: 10.1098/rstb.2012.0242
Rodríguez, J., Tintoré, J., Allen, J. T., Blanco, J. M., Gomis, D., Reul, A., et al. (2001). Mesoscale vertical motion and the size structure of phytoplankton in the ocean. Nature 410, 360–363. doi: 10.1038/35066560
Rogers, D. (1972). Random search and insect population models. J. Anim. Ecol. 41, 369–383. doi: 10.2307/3474
Rothschild, B. J., and Osborn, T. R. (1988). Small-scale turbulence and plankton contact rates. J. Plankton Res. 10, 465–474. doi: 10.1093/plankt/10.3.465
Saiz, E., Calbet, A., and Broglio, E. (2003). Effects of small-scale turbulence on copepods: the case of Oithona davisae. Limnol. Oceanogr. 48, 1304–1311.
Salvanes, A. G. V., Aksnes, D. L., and Giske, J. (1995). A surface-dependent gastric evacuation model for fish. J. Fish Biol. 47, 679–695. doi: 10.1111/j.1095-8649.1995.tb01934.x
Schatz, B., Lachaud, J. P., and Beugnon, G. (1997). Graded recruitment and hunting strategies linked to prey weight and size in the ponerine ant Ectatomma ruidum. Behav. Ecol. Sociobiol. 40, 337–349. doi: 10.1007/s002650050350
Sih, A. (1979). Stability and prey behavioural responses to predator density. J. Anim. Ecol. 48, 79–89. doi: 10.2307/4101
Tansley, A. G. (1935). The use and abuse of vegetational concepts and terms. Ecology 16, 284–307. doi: 10.2307/1930070
Turesson, H., and Brönmark, C. (2007). Predator-prey encounter rates in freshwater piscivores: effects of prey density and water transparency. Oecologia 153, 281–290. doi: 10.1007/s00442-007-0728-9
Twardochleb, L. A., Treakle, T. C., and Zarnetske, P. L. (2020). Foraging strategy mediates ectotherm predator-prey responses to climate warming. Ecology 101:e03146. doi: 10.1002/ECY.3146
Tyrell, A. S., and Fisher, N. S. (2019). Separating viscous and thermal effects of temperature on copepod feeding. J. Plankton Res. 41, 865–878. doi: 10.1093/PLANKT/FBZ055
Uiterwaal, S. F., and DeLong, J. P. (2020). Functional responses are maximized at intermediate temperatures. Ecology 101:e02975. doi: 10.1002/ecy.2975
Vogel, S. (1996). Life in Moving Fluids: the Physical Biology of Flow. Princeton, NJ: Princeton University Press.
Volterra, V. (1926). Variazioni e fluttuazioni del numero d’individui in specie animali conviventi. Mem. Acad. Lincei 6, 31–113.
Vucic-Pestic, O., Rall, B. C., Kalinkat, G., and Brose, U. (2010). Allometric functional response model: body masses constrain interaction strengths. J. Anim. Ecol. 79, 249–256. doi: 10.1111/j.1365-2656.2009.01622.x
Wasserman, R. J., Alexander, M. E., Weyl, O. L. F., Barrios-O’neill, D., William Froneman, P., Dalu, T., et al. (2016). Emergent effects of structural complexity and temperature on predator-prey interactions. Ecosphere 7:e01239. doi: 10.1002/ECS2.1239
Watt, K. E. F. (1959). A mathematical model for the effect of densities of attacked and attacking species on the number attacked. Can. Entomol. 91, 129–144. doi: 10.4039/Ent91129-3
Williams, R. J., Anandanadesan, A., and Purves, D. (2010). The probabilistic niche model reveals the niche structure and role of body size in a complex food web. PLoS One 5:e12092. doi: 10.1371/journal.pone.0012092
Wilson, R. P., Griffiths, I. W., Legg, P. A., Friswell, M. I., Bidder, O. R., Halsey, L. G., et al. (2013). Turn costs change the value of animal search paths. Ecol. Lett. 16, 1145–1150. doi: 10.1111/ELE.12149
Wilson, R. P., Griffiths, I. W., Mills, M. G. L., Carbone, C., Wilson, J. W., and Scantlebury, D. M. (2015). Mass enhances speed but diminishes turn capacity in terrestrial pursuit predators. eLife 4, 1–18. doi: 10.7554/eLife.06487.001
Keywords: functional response, predator, prey, medium, body size, mechanics
Citation: Portalier SMJ, Fussmann GF, Loreau M and Cherif M (2022) Inferring Size-Based Functional Responses From the Physical Properties of the Medium. Front. Ecol. Evol. 9:761984. doi: 10.3389/fevo.2021.761984
Received: 20 August 2021; Accepted: 07 December 2021;
Published: 11 January 2022.
Edited by:
Thomas John Hossie, Trent University, CanadaReviewed by:
Jiqiu Li, Xiamen University, ChinaRochelle Diane Seitz, College of William & Mary, United States
Gregor Kalinkat, Leibniz-Institute of Freshwater Ecology and Inland Fisheries (IGB), Germany
Copyright © 2022 Portalier, Fussmann, Loreau and Cherif. This is an open-access article distributed under the terms of the Creative Commons Attribution License (CC BY). The use, distribution or reproduction in other forums is permitted, provided the original author(s) and the copyright owner(s) are credited and that the original publication in this journal is cited, in accordance with accepted academic practice. No use, distribution or reproduction is permitted which does not comply with these terms.
*Correspondence: Sébastien M. J. Portalier, c2ViYXN0aWVuLnBvcnRhbGllckBtYWlsLm1jZ2lsbC5jYQ==