- Department of Genetics, Wrocław University of Environmental and Life Sciences, Wrocław, Poland
Significant development of genetic tools during the last decades provided opportunities for more detailed analyses and deeper understanding of species hybridization. New genetic markers allowed for reliable identification of admixed individuals deriving from recent hybridization events (a few generations) and those originating from crossings up to 19 generations back. Implementation of microsatellites (STRs) together with Bayesian clustering provided abundant knowledge regarding presence of admixed individuals in numerous populations and helped understand the problematic nature of studying hybridization (i.a., defining a reliable thresholds for recognizing individuals as admixed or obtaining well-grounded results representing actual proportion of hybrids in a population). Nevertheless, their utilization is limited to recent crossbreeding events. Single Nucleotide Polymorphisms (SNPs) proved to be more sensible tools for admixture analyses furnishing more reliable knowledge, especially for older generation backcrosses. Small sets of Ancestry Informative Markers (AIMs) of both types of markers were effective enough to implement in monitoring programs, however, SNPs seem to be more appropriate because of their ability to identify admixed individuals up to 3rd generations. The main aim of this review is to summarize abundant knowledge regarding identification of wolf-dog hybrids in Europe and discuss the most relevant problems relating to the issue, together with advantages and disadvantages of implemented markers and approaches.
Introduction
Recent decades have witnessed rising awareness of the need to protect species in order to maintain or restore ecosystem balance. Extensive knowledge provided by ecologists has improved our understanding of the key significance of numerous species in preserving properly functioning ecosystems. With reintroductions, one can observe how particular species like top-order predators may have a fundamental impact, both on their prey and on plants and other species, aspects that at first sight may seem unrelated (e.g., Hayward and Somers, 2009).
The gray wolf (Canis lupus) is one such species. Its huge significance was discovered after its reintroduction to the Yellowstone National Park (YNP) in 1995 and 1996 (Ripple et al., 2001; Wilmers et al., 2003a), which emphasized the gray wolf’s importance as a keystone species, highlighting its universal role not only as a limiter of prey numbers but also as a species creating appropriate conditions for many others. Firstly, wolves provide carcasses for scavengers, significantly changing the latter’s behavior: for instance, some grizzly bears (Ursus arctos) in the Glacier National Park do not hibernate, because the amount of carrion left by wolves is enough for them to survive the winter (Wilmers et al., 2003a). Ravens (Corvus corax) associate with wolves and follow them to find sources of food (Stahler et al., 2002). Moreover, comparison of carcasses left by wolves and hunters in the YNP indicates differences in the consumption of leftovers: carrion left by hunters may be a food source for greater numbers of individuals, but carcasses left by wolves attract a much more diverse spectrum of species (Wilmers et al., 2003b). Wolves may be responsible for increased plant growth by limiting numbers of herbivores and impacting their behavior. In turn, such changes may result in the appearance of beavers and other species (e.g., Beyer et al., 2007; Smith and Tyers, 2012; Runyon et al., 2014). Furthermore, wolves limit the numbers of stray dogs (Canis lupus familiaris), a potential threat to livestock (Blanco et al., 1992). Wolves are also crucial in limiting numbers of prey species in that they select these in a very different way to hunters. For example, wolves prey mainly on red deer calves and old females, whose reproductive potential is low, whereas hunters seek bulls and young females, which have a much higher reproductive potential (Wright et al., 2006). In the light of all the above, the environmental significance of wolves is undeniable.
According to the IUCN Red List, the gray wolf’s current conservation status is LC (Least Concern). Despite this, the species is listed in Appendix II of the Bern Convention (1979), Appendix II in CITES (1973) and Annexes II and IV of the Habitat Directive (European Communities [EC], 1992). Consequently, the gray wolf is protected in many countries. There are several ways of protecting wolves and even of restoring them to their natural environments, with aforementioned reintroduction to the YNP being one of the most spectacular examples. Nevertheless, despite the many measures taken to protect gray wolves and guidance provided by international legislation regarding hybrids treatment, many European countries show insufficient compliance neglecting possible threats from the hybrids toward the gray wolf populations. Moreover, lack of cooperation in hybrid detection and treatment between countries inhabited by the same wolf populations decreases the reliability of results of such measures (Salvatori et al., 2020).
Hybridization has been defined as the intercross between two different taxa (species/subspecies) or genetically different populations or varieties (Gompert and Buerkle, 2016). But as the authors have suggested, this definition does not properly separate gene flow from hybridization. They therefore propose to treat hybridization as a continuous and quantitative process. A process where gene flow and outcrossing occur between populations which are distinct in numerous loci or possess heritable features that impact on an individual’s fitness. According to the authors, a clear, qualitative distinction between hybridization and gene flow is not possible (Gompert and Buerkle, 2016).
In recent decades, awareness of hybridization as an important factor in conservation has been increasing rapidly. More and more researchers from different disciplines are joining the discussion about the potential outcomes of the process in both plants and animals (e.g., Vilà et al., 2000; Allendorf et al., 2001; Gompert and Buerkle, 2016), being of the opinion that these outcomes can vary dramatically, depending on the situation. They might be neutral and result in: (i) the establishment of a natural hybrid zone that does not cause the disappearance of a parental species (Grudzien et al., 1987); (ii) natural introgression, which may lead to genetic similarity despite morphological and ecological distinctiveness (Clarke et al., 1996); (iii) the appearance of a new taxon, which does not cause the extinction of the parental taxa (Berger, 1973). Furthermore there are cases, in the context of climate change, when hybridization may have a positive influence on the survival of the parental species (Becker et al., 2013). On the other hand, hybrids may have a greater fitness as a result of heterosis, a process which in natural conditions is a part of speciation but which can also lead to the extinction of a parental species, especially when hybridization is anthropogenic (Dagilis et al., 2019).
It is worth stressing that extinction may be the upshot of many different processes, which become apparent when hybrids appear. Firstly, if they are fertile, they can breed with one of the parental species, which leads to introgression. By disrupting the genetic integrity of the wild population, this may end in the disappearance of alleles, decreasing fitness or even threatening the existence of the parental species (Rhymer and Simberloff, 1996). Consequently, this often becomes a conservation concern, when a parental species is considered to be endangered (Allendorf and Leary, 1988). In this case, identifying the individual as a first generation hybrid (F1), second generation (F2), later generation or a backcross is essential in determining potential management actions (Allendorf et al., 2001). Secondly, even if hybrids are unable to breed, they usually thrive in the same or similar conditions as their parental species, so they are a source of a competition for food and space (Rhymer and Simberloff, 1996; Simberloff, 1996); this has also been documented in wolf-dog hybridization studies (WDH) (e.g., Bassi et al., 2017). Moreover, such hybridization in an endangered species is a waste of reproductive effort. Lastly, if there is no selection against hybrids, these can dominate the parental species, leading to decrease in number of pure individuals or even extinction (Allendorf et al., 2001).
In the light of the above, it is clear that results of a hybridization might vary drastically. Therefore, the question is: when to manage a species, if there is a suspicion of hybridization? An answer for the question is not easy. Numerous factors should be taken under consideration before any decision is made. First of all, what level of admixture should be taken into account as decisive for defining an individual as a hybrid? Secondly, defining an origin of hybridization should be equally important. According to Allendorf et al. (2001) one can divide hybridization into six types: three types of natural origin and three types resulting from anthropogenic activity. Each case is unique and should be examined carefully and separately. Nevertheless, defining the origin of hybridization as anthropogenic is usually inducing for management actions, especially when hybrid swarms are common (populations with frequent introgression, and numerous multigenerational hybrids) (Allendorf et al., 2001).
What is worth mentioning, interspecific hybridization is widely occurring in Canids. Gene flow between species in this family resulted not only in appearances of new species [i.a., African golden wolf (Canis lupaster)], but also has an impact on population structures of the existing ones (African golden wolf have been hybridizing after emerging as a separate species with both parental species populations—gray wolves and Ethiopian wolves (Canis simensis) (Gopalakrishnan et al., 2018). Remarkable influence of hybridization and gene flow among Canids in shaping phylogenetic relationships and population structures highlights potential significance of impact of wolf-dog hybridization on existing gray wolf populations. From a management point of view, such changes resulting from hybridization of anthropogenic origin might be at least unwelcome.
Interest in wolf-dog hybridization specifically, arose long before it was considered a potential conservation problem (e.g., Przibram, 1910; Spillman et al., 1910; Iljin, 1941). However, back then it was investigated mainly in the context of dog breeding, anatomy and behavior. With the growth in genetic knowledge and the better understanding of the part genetics plays in evolution, this has become an important subdiscipline in biological conservation. Consequently, ever more scientists studying gray wolves have noticed that hybridization poses a potential threat to their conservation.
In the second half of the 20th century, wolf-dog hybrids were reported from many countries: Belarus, the United States, Latvia, Ukraine, Moldova, Portugal, Uzbekistan, Turkmenistan, the USSR, Spain, Kazakhstan, Israel, and Serbia (Bibikov, 1982; Blanco et al., 1992; Andersone et al., 2002; Milenković et al., 2006 and all the references in these four papers). However, they were identified mostly by their appearance (e.g., Blanco et al., 1992). Morphological identification is faster, cheaper and often simply convenient, but it does have certain disadvantages: firstly, it may overlook some individuals, as hybrids may not be morphologically differentiated from pure wolves, and secondly, such identification provides few details and is unreliable (Galaverni et al., 2017; Kusak et al., 2018).
In the late XX and early XXI century, studies of WDH yielded somewhat unexpected results. Despite earlier reports of the existence of wolf-dog hybrids aforementioned above, hardly any were encountered during a few different studies: (i) Vilà et al. (1997) was one of the earliest genetic studies including information regarding wolf-dog hybridization and wolf samples from Europe, however, the main focus of the study was the ancestry of dogs. Based on the small sequence (261 bp) of mtDNA control region the authors defined haplotypes for both dogs and wolves and based on high similarity of a few haplotypes they inferred about possible recent hybridization (ii) Randi (1993), using vertical and horizontal polyacrylamide gel electrophoresis, failed to find any hybrids among more than 30 wolves, (iii) Lorenzini and Fico (1995) studied the genetic structure of wolves based on enzyme polymorphism but found no signs of extensive hybridization in 46 animals, (iv) Blanco et al. (1992) examined 600 gray wolf carcasses from its populations in Spain but failed to find any hybrids based on morphological traits, (v) Randi et al. (2000) studied genetic variability based on mtDNA CR (control region) in Italian (n = 101) and eastern European (n > 29) wolves. They detected no hybrids in the Italian samples, but seven possible hybrids in the eastern European ones. In 1999, Vilà and Wayne questioned the commonly-held at that time view about the potential threat of WDH to gray wolf conservation.
What is worth mentioning, methodology of identification of admixed individuals and its reliability in the aforementioned studies varied significantly (more details in the subsequent subsections). In the following years interest in WHD studies rose rapidly, providing more sophisticated and reliable methodology for identification of admixed individuals and, at the same time, highlighting the flaws of some of the approaches presented above. Moreover, the development of genetic tools provided new opportunities for hybrid identification. Microsatellites, mtDNA/genome sequencing and SNPs (Single Nucleotide Polymorphisms) analyses all facilitated more detailed studies and the identification of hybrids lacking any outward signs suggesting admixtures of dog DNA. Moreover, such studies provide more information, e.g., which generation of hybrids we are dealing with, the degree and direction of hybridization. Therefore, numerous of the 21st century studies of WDH are genetically based. Although obtaining materials for genetic analysis may be easier than identifications based on morphological traits, the cost of genetically based research is correspondingly higher.
Taking into consideration the importance of defining a proportion of admixture and to identify type of hybrids (F1, F2, etc.), genetic tools are inevitable for appropriate analysis of hybridization and are essential for taking proper management actions. Therefore, numerous studies have been conducted in order to find the most reliable and convenient genetic tools for this purpose. As the amount of information is significant and still growing, the main aims of this article are to summarize and discuss the brief history and current knowledge regarding wolf-dog hybrid identification in Europe, with emphasis on molecular markers and their efficiency. Note that defining the extent of admixture in the individual’s genome, from which it is acknowledged as a hybrid is not a part of this article. Moreover, in numerous studies presented below, threshold values for identifying individuals as hybrids vary greatly, therefore using the word hybrid I usually refer to conclusions from this particular research instead of some general assumptions.
Methods
During 2019, 2020, and 2021 suitable literature have been searched using different combinations of the following keywords and phrases: “wolf,” “Canis lupus,” “dog,” “hybridization,” “WDH,” “hybrid,” “SNPs,” “conservation,” “genetics,” “microsatellites,” “identification,” “whole genome sequencing.” Throughout 2019 and 2020 searching has been conducted using Google Scholar and ResearchGate. In the year 2021 the list of articles was updated, researching through all databases in Web of Science and using search engines mentioned above. Moreover, Google Alerts have been set up for some of the phrases (“wolf,” “Canis lupus,” “WDH,” “wolf hybridization”). Despite focusing on articles in English, no limits or search filters were used for the searching. To supplement the papers that were collected this way, the references have been checked in them and the most relevant ones were added to the list. Thus, it was possible to cover in the article most of the studies relating to WDH in Europe, including those supplying the most important and most often cited results. Last search for every strategy was made: (i) Google scholar1 VIII.2021, (ii) Web of Science2 VIII.2021, (iii) ResearchGate3 VIII.2021, and (iv) Google Alerts XII.2020.
Microsatellites in Wolf-Dog Hybridization Studies
Microsatellites (known also as STRs—Short Tandem Repeats or SSRs—Simple Sequence Repeats) are defined as short sequences with tandem repeats of 1–6 bp [typically, however, sometimes repeats of 7–12 bp are also considered as STRs (Hindrikson et al., 2017)]. Their utility in genetic studies is owed to a few different factors, i.a., abundance and common presence in the genomes of higher organisms, high polymorphism and neutrality (their occurrence in the non-coding DNA prevents from favoring by natural selection). With an implementation of suitable statistics, based on variations in the number of sequence repeats one can infer about population structure or substructure (Ellegren, 2004; Selkoe and Toonen, 2006).
Randi and Lucchini (2002) were one of the first authors in Europe implementing microsatellites in WDH studies. Four of more than 100 wolves in the study were known to be hybrids and were living in captivity, and three other wolves were suspected of being hybrids based on their morphological traits. To identify the origins of the wolves and dogs, three different methods were implemented: PCA (Principal Component Analysis) using PCAGEN4, NJ (Neighbor-Joining) (Saitou and Nei, 1987) in Phylip 3.5C5 and Bayesian clustering in STRUCTURE (Pritchard et al., 2000). PCA placed nine animals between dog and wolf clusters, including all the hybrids. However, only one axis differentiated the wolves from the dogs; on the other axis they overlapped. The NJ clustering algorithm identified three of the four hybrids as wolf-dog intermediates, while the fourth was assigned to the wolf cluster. Three wolves with morphological traits suggesting hybridization were also assigned to the wolf cluster. Moreover, a few different wolves were identified as intermediate between wolves and dogs. Clustering in STRUCTURE without any prior phenotypic information identified five admixed individuals. The results included all the hybrids and one wolf with morphological traits suggesting hybridization. The authors assumed that Bayesian clustering was the most efficient of the implemented methods. On the other hand, PCA was unable to correctly differentiate the overlapping clusters, and NJ did not identify all the hybrids.
The methodology employed in subsequent studies largely utilized Bayesian clustering in STRUCTURE, as the most common tool for differentiating hybrids from pure individuals (Table 1). Its reliability and efficiency in hybridization studies were analyzed and compared with other methods and algorithms providing important knowledge regarding advantages and limitations of such methodology (e.g., Vähä and Primmer, 2006; Bohling et al., 2013; Neophytou, 2014). A few important aspects need to be noted with accordance to Bayesian clustering in STRUCTURE from hybridization point of view: (i) it is able to properly assign individuals to the population of their origin even in case of low differentiation between populations (for Fst = 0.05, 92% individuals were correctly assigned) (Latch et al., 2006) (ii) based on a simulated dataset identification of F1 hybrids was efficient with implementation of 12–24 loci, but for the reliable identification of less admixed individuals the number of STRs implemented in the study should be accordingly higher, unless there is high differentiation between populations (e.g., for backcrosses it was 48 microsatellites) (Vähä and Primmer, 2006). Taking into consideration that the number of STRs used in hybridization studies usually does not exceed 20 (Table 1), one could question what level of efficiency may be achieved taking into account these circumstances? Majority of admixed individuals are older generations and inability of the software to distinguish them from pure individuals may drastically underestimate the number of admixed wolves. In contrast, analyses conducted by Bohling et al. (2013) provided distinct results-based on empirical dataset with a detailed pedigree and using 17 microsatellites the authors noticed that decreasing the number of STRs did not result in significant increase in misclassification. As the authors suggested, such dissimilarities may arise from higher differentiation between populations of origin in their studies than in the research conducted by Vähä and Primmer (2006). In the following studies, differentiation between wolf and dog populations significantly exceeds the one presented by Vähä and Primmer (2006), therefore implementing smaller sets of microsatellites is completely reasonable, what is also confirmed in the study conducted by Godinho et al. (2015), (iii) including reference samples in the software did not significantly change the results, (iv) it has tendency to identify pure individuals as admixed, and (v) choice of threshold q-value should be made with accordance to the aim of the study, as it has crucial impact on efficiency and accuracy of hybrid identification (Vähä and Primmer, 2006; Bohling et al., 2013).
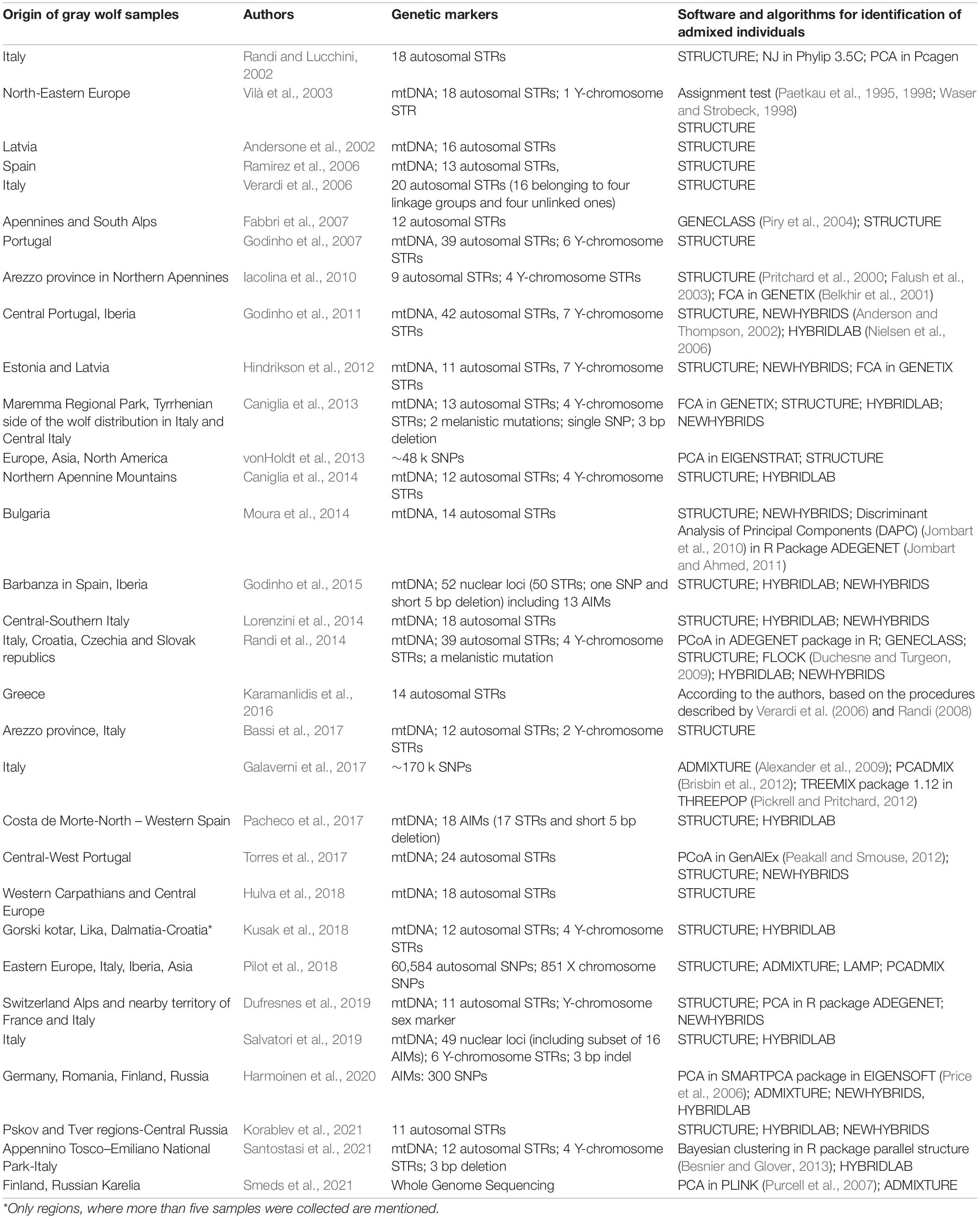
Table 1. Summary of implemented markers and software for identification of admixed individuals in studies mentioned in the review and Supplementary Information 1. For information regarding periods of sampling in particular regions and number of admixed individuals confirmed in particular populations see Table 1 in Dufresnes et al. (2019).
A few studies implemented Bayesian clustering in STRUCTURE with no hybrids detected i.a.: (i) Ramirez et al. (2006), where the authors conducted genetic assessment of wild and captive Iberian wolves (Canis lupus signatus). In addition to Bayesian clustering, NJ algorithm in MEGA 2.0 (Kumar et al., 2001) was implemented, however, no hybrids were detected among 74 individuals (nevertheless, 54 of all wolves were included in the breeding program and not all of them can be defined as “purely wild” individuals) (ii) Fabbri et al. (2007) did not yield any hybrids among 435 distinct genotypes. All the samples were collected in the Apennines and South Alps after the 1980s (though the majority of them were collected between 2000 and 2004) providing abundant knowledge regarding those populations (Fabbri et al., 2007). The drawback of the study is lack of the dog samples in the analyses, which could change the results of hybrid identification based on clustering (iii) Godinho et al. (2007) conducted research in Portugal using mtDNA, six Y-chromosome microsatellites and 39 autosomal microsatellites. Despite using maternal, paternal and numerous biparental markers no hybrids were detected in 55 wolf samples.
An important aspect of microsatellites is the fact that they are biparental markers. Andersone et al. (2002) (Supplementary Information 1) confirmed that mtDNA of the admixed individual belonged to the wolf haplotype and hybridization was identified through microsatellites. In the further studies presented in the review all include biparental markers. Such methodology allows for avoiding the misclassification of individuals, which is highly probable based solely on mtDNA. The reason is that WDH is usually the result of a female wolf mating with a male dog (e.g., Andersone et al., 2002; Vilà et al., 2003; Godinho et al., 2011) and a few explanations for such asymmetry are given in the literature. Hindrikson et al. (2012) considered that male wolves are typically more aggressive than females and often prey on dogs, so female dogs are likely to avoid them. Furthermore, female wolves more often look for a male dog to mate with than male wolves for a female dog: they have even been observed to use vocalization for this purpose. Such females are often older/injured, which decreases their chances of finding a wolf partner. Moreover, wolf pups have greater chances of surviving in the wild than dog pups. If a male wolf mates with a female dog, the hybrids may be easily overlooked and treated as mongrels (Hindrikson et al., 2012 and references therein). Lastly, there are important differences in the physiology of these two species: whereas male and female wolves are capable of reproducing during only one part of the year (January–April), male dogs can breed all the year round, and females usually have two estrous cycles per year. Therefore, mating between a male wolf and a female dog is less likely, as their breeding periods would have to overlap, whereas in the case of a female wolf and a male dog there is no such necessity (Vilà and Wayne, 1999). A few studies have demonstrated deviation from the asymmetry, indicated by situations where dog mtDNA was found in wolves (e.g., Muñoz-Fuentes et al., 2010; Hindrikson et al., 2012; Randi et al., 2014).
In 2006, Verardi et al. conducted research using a slightly different approach (for more studies utilizing “classical approach” see Supplementary Information 1). The authors suggested that when unlinked biparental markers were used to estimate the degree of WDH, this might still be underestimated. Therefore, instead of using only unlinked microsatellites, they used 16 STRs belonging to four linkage groups and four unlinked microsatellites. According to the authors, this should be more sensitive for identifying hybrids after more than a few generations because of the slower decrease in the linkage disequilibrium in linked loci than in unlinked ones. Also, they examined a far higher number of wolves than in the majority of earlier studies (n = 220 Italian wolves)—tissue samples had been collected for a long period between 1987 and 2002. The control group in this research consisted of seven known hybrids. Assignment to genetic clusters was performed using STRUCTURE with a threshold value of q > 0.8. Based on these methods, 11 hybrids were detected, i.e., 5% of all the wolf samples. Compared with earlier studies from Italy (Randi and Lucchini, 2002), where only one hybrid living in the wild was detected, and emphasizing that both studies included samples collected across the entire range of the Italian wolves population, the results showed an increase in the sensitivity of hybrid identification. Nevertheless, to the best of my knowledge there are no other studies examining WDH using a similar approach; as it is mostly unlinked microsatellites that are used, no reliable conclusions can be drawn concerning this methodology.
Another research worth mentioning is the one conducted by Godinho et al. (2011) in the Iberian Peninsula. The authors used a higher number of genetic markers to identify wolf-dog hybrids and the methodology was expanded to 42 autosomal microsatellites, six Y-chromosome STRs and mtDNA. These authors examined samples from 208 putative Iberian wolves collected across most of the wolf distribution in Iberia, 196 dogs and four wolves with morphological traits suggesting potential hybridization. Bayesian clustering analysis was performed in STRUCTURE (Pritchard et al., 2000; Falush et al., 2003). NEWHYBRIDS (Anderson and Thompson, 2002) software was used to obtain more detailed information regarding the hybrids, i.e., whether they were F1, F2, backcrosses with dog/wolf, and HYBRIDLAB (Nielsen et al., 2006) was used to assess the power of the markers and the model (the genotypes for every group were simulated and subsequently analyzed with methods used in the research). Based on Bayesian clustering (threshold q < 0.85), eight hybrids were detected in the samples (including individuals with a morphology suggesting hybridization). Subsequently, the hybrids were assigned to the following types: 3 × F1 with a probability > 0.9, and 3 × backcross with a wolf with a probability > 0.98; the last two animals could not be assigned with sufficient certainty. Moreover, all the hybrids had wolf mtDNA haplotypes, and four out of five male hybrids had the typical dog Y-chromosome haplotype. The results were similar to those of Verardi et al. (2006) and Iacolina et al. (2010) (Supplementary Information 1), i.e., the estimated frequency of hybridization was ca 5%. Therefore, despite the much higher number of microsatellites, the results were comparable.
Interesting studies in regards to phenotypic hybrid identifications were conducted in Italy and Croatia. The first one, was conducted by Lorenzini et al. (2014). One of the author’s goals was to link morphological traits of hybridization with admixed genotypes in order to find some descriptive relationship. Their research was based on 18 autosomal microsatellites. Moreover, the individuals with admixed genotypes were subjected to mtDNA CR analysis. Tissue or blood samples were obtained from wolves (n = 107) and dogs (n = 90). Hybrid identification was based on Bayesian clustering in STRUCTURE. Individuals were acknowledged to be hybrids if their proportion of membership in a parental cluster was <0.9. Seven hybrids were identified (∼6.5%) and no clear connection between phenotypic traits and genetic admixtures was confirmed. While for some individuals with traits suggesting hybridization no admixture of dog DNA was identified, other wolves with no visible signs of potential admixtures were identified as hybrids [more information regarding coat color and hybrids may be found in Anderson et al. (2009)]. Furthermore, the authors indicated that hybrid identification in their research was closely dependent on the threshold level. For example, if q had been altered from 0.9 to 0.85, three hybrids instead of seven would have been identified. The importance of correct definition of threshold values for hybrid identification is discussed later.
Furthermore, in a study conducted by Kusak et al. (2018) 176 putative wolf samples and 49 dog samples were collected and genotyped using 12 autosomal microsatellites, four Y-chromosome microsatellites and mtDNA CR. Individual assignments were done in STRUCTURE. The animals with the highest membership (q > 0.98) were used to simulate parental and then hybrid genotypes in HYBRIDLAB. Based on phenotypic features, 157 out of 176 wild Canid samples were defined as wolves and 19 as suspected hybrids (10.8%). However, genetic analysis confirmed the presence of just five hybrids (2.8%). Although only a small number of hybrids were identified in this research, the results clearly indicated (similarly to Lorenzini et al., 2014) that morphological traits suggesting an admixture of dog DNA merely advocate further genetic analysis, and that they are not means of identifying hybrids (even two animals, a black one and an albino, were ultimately assigned to the wolf cluster). Therefore, using phenotypic features as the main methodology of hybrid identification may lead to incorrect results (see also Galaverni et al., 2017).
The next article is highly valuable in terms of marker selection. The study conducted by Randi et al. (2014) was focused on evaluating the power of uniparental and biparental genetic markers used in WDH studies. To estimate the power of a different number of markers and to define the threshold values in STRUCTURE, genotypes were simulated using HYBRIDLAB. Assignment to specific hybrid types was conducted with NEWHYBRIDS. Samples were collected in different parts of Europe (Czechia, Slovakia, Croatia, Italy) from 99 wolves without any morphological traits suggesting hybridization, 69 dogs, 73 Czechoslovakian wolfdogs (as empirical hybrids) and 30 putative hybrids from Italy. The authors also attempted to connect the deletion of a specific allele Kb responsible for the black coat in German shepherd dogs with the black coat in Italian wolves (also Caniglia et al., 2013 in Supplementary Information 1) as a reason for inferring admixtures of dog DNA. However, five of the seven black-coated wolves had no admixtures; in addition, one wolf lacked the deletion, despite its black coat. Therefore, no unambiguous connection between the deletion and hybrid identification might be inferred based on the study. Nevertheless, the most important information from the study regards microsatellites—the set of 24 STRs was as efficient or even more so in individual assignment than the set of 39 microsatellites (Randi et al., 2014). In admixture analysis, therefore, the discriminant power of markers has a much higher impact on correct assignment than the number of markers. On the other hand, a small number of microsatellites might result in lower thresholds and wide credibility intervals, which are undesirable. In addition, the microsatellites should be chosen on the basis of the study area: in the study by Godinho et al. (2011), a few markers were very efficient, whereas in the research by Randi et al. (2014) their discriminatory power was low.
Microsatellites are the most common markers implemented in WDH studies so far (despite mtDNA, which is not reliable for hybrid identification itself because of its uniparental character). Abundance of known primers for Canines (e.g., Holmes et al., 1993; Ostrander et al., 1993; Francisco et al., 1996) provides a wide range of possibilities not only for WDH studies, but also for any genetic studies of Canines. As specific microsatellites may be monomorphic for one population, while being polymorphic in the others, such diversity is much desired, because it allows for a choice of the most informative markers for every population (e.g., Randi et al., 2014, where only part of the most discriminating markers overlapped with those from Godinho et al., 2011). Another important aspect of STRs is their number. While it is hard to define the minimum number of microsatellites, as it depends on their informativeness, it is already confirmed that insufficient quantity may result in false identifications (e.g., Randi et al., 2014). Therefore it is essential to define the power of microsatellites and construct sets based on their informativeness instead of the highest possible quantity. On the other hand, increasing the number of STRs allows for an increase in threshold values, on which defining the extent of hybridization is highly dependable (e.g., Lorenzini et al., 2014; Bassi et al., 2017). Therefore, determining the number of microsatellite markers is one of the most important aspects of every WDH study based on STRs. To accomplish this, comparisons between more or less random sets of STRs with Ancestry Informative Markers (AIMs) were conducted.
Ancestry Informative Markers
Usually AIMs are implemented in biogeographical studies to infer about individuals’ ancestry (Halder et al., 2008). Different variations of a specific marker might be connected with a specific geographic region and continent, and therefore allows for inferring about individuals’ origin. Similarly, in WDH studies particular marker variations will provide information regarding origin of the individuals—if they derive from wolves, dogs or hybridization between wolf and dog.
In 2015 Godinho et al. compared the results of an analysis based on 52 nuclear loci (50 microsatellites, one SNP and a short deletion) from which a subset of 13 AIMs was created. Samples from four putative hybrids and 38 scats, together with 242 reference wolves and 237 reference dogs were implemented in the study. HYBRIDLAB was used to simulate hybrid and parental genotypes, and the hybrids were assigned to their type using NEWHYBRIDS.
The set of 13 AIMs was much more successful in assigning individuals to their clusters than similar sets of nuclear loci (10 sets of 13 markers randomly chosen from the 52 used in the study and 18 microsatellites commercial set). Nevertheless, the use of 13 AIMs was no more effective than the analysis based on 52 nuclear loci, although the set of 13 AIMs was efficient enough to assign individuals to the correct cluster (based on simulated genotypes, the threshold values for wolves q = 0.9 and dogs q = 0.8). For F1, F2 and backcrossed hybrids, identification analysis using 13 AIMs conducted on simulated genotypes was 100% effective for F1 identification and 96.3% for first-generation backcrosses with wolves. Assignment to the second generation of backcrosses was not so efficient (66.4%). However, the results regarding the type of hybrids were not consistent between methods: whereas the whole set analyses based on tissue samples indicated the second generation of wolf backcrosses in four individuals, AIMs suggested that they were first-generation backcrosses. Nonetheless, identification of admixture resulting from recent hybridization was correct in both methods (Godinho et al., 2015).
Pacheco et al. (2017) implemented the same set of AIMs as Godinho et al. (2015). The authors attempted to estimate the degree of WDH in one generation, because many other studies were based on samples which had been collected over a few years. To acquire a better understanding of the process and its dynamic, the authors decided to analyze WDH in a 1-year breeding period. 332 samples were collected, 181 of which remained after quality control and were genotyped for 18 AIMs (which were chosen from 52 nuclear loci from the mentioned study). Ultimately, 167 samples comprising 130 distinct genotypes were analyzed. AIMs very efficiently differentiated wolves from dogs (Fst = 0.335). Bayesian clustering was performed in STRUCTURE. Reference samples from 250 Iberian wolves and more than 230 dogs were used. Based on these, the genotypes for every group were simulated in order to define the optimal thresholds for wolf and dog assignments, which were in results established at 0.91 and 0.85 respectively. 67 of the 130 genotypes were identified as wolves, 56 as dogs and seven were admixed. Three of them had dog mtDNA haplotypes, suggesting a rarer type of WDH. In addition, one of the hybrids was probably a first-generation backcross, the remainder multi-generational backcrosses. The introgression rate was established at ∼5% in the wolf population of Costa da Morte. The hybrids identified in this study were derived from different hybridization events—apart from two individuals, the other hybrids were unrelated. On the other hand, the low introgression rate indicated that the gray wolf population was resistant and that despite the occurrence of hybrids, introgression into wild populations was minimal (Pacheco et al., 2017). What is worth mentioning, the authors suggested that the width of Bayesian Credible Intervals (BCI) was useful for indicating potential hybridization (hybrids have a much wider BCI than pure individuals); this was also mentioned in other relevant research (e.g., Verardi et al., 2006; Godinho et al., 2011; Caniglia et al., 2013).
Salvatori et al. (2019) conducted another study implementing 49 nuclear loci (for tissue samples analyses) and a subset of 16 AIMs (for NIS analyses), which were chosen on the basis of their Fst differentiation and PID. The methodology also included six Y-chromosome microsatellites and indel, which often determines the appearance of a black coat. STRUCTURE software was applied to analyze the genotypes with a reference group of 52 putative pure wolves and 25 pure dogs. The threshold for the wolf reference samples was set at q > 0.99. Assessing for genotype identification was based on simulations conducted in HYBRIDLAB. Quality control yielded 72 individual genotypes of putative wolves. The minimum threshold for assignment to the wolf cluster was different for AIMs (q = 0.953) and the set of 49 nuclear loci (q = 0.982). The proportion of admixed individuals differed, depending on the set used for analysis: 22.9% for 16 nuclear loci, and 30.6% taking into consideration four more individuals identified using 49 microsatellites, which were considered wolves based on the subset. The authors stated that if the same proportion were maintained for NIS screened for 49 nuclear loci, the proportion of admixed individuals would reach ∼50%. In addition, 18 of the 72 genotypes showed signs of introgression, whereas only seven were identified as admixed. Phenotypic traits could not be linked with the results of genetic analysis (Salvatori et al., 2019). Nevertheless, the threshold values for assigning individuals to particular clusters were high (0.953 and 0.982). With such high q values the number of hybrids might be slightly overestimated. More conservative thresholds were implemented in previous studies (e.g., 0.91 and 0.86 for wolves and dogs respectively, which is 0.05 less than based on the simulated genotypes). Moreover, the samples were collected in a relatively small area (Grosseto province in Italy) and cannot be extrapolated for the whole Italian population.
Summarizing, whereas AIMs were more efficient than a similar number of nuclear loci markers, they were still less effective than a greater number of the markers (Godinho et al., 2015). Importantly, in Godinho et al. (2015) [and further in Pacheco et al. (2017)] nuclear loci were not chosen on the basis of their power to differentiate dogs from wolves. If the whole set of nuclear loci had been selected more carefully, the results might have been different. Nonetheless, with the cheaper and easier analyses based on AIMs one can identify hybrids with a similar certainty to the greater set of microsatellites. Hence, these approaches may be more desirable if the sole purpose is hybrid identification. On the other hand, if the focus is on obtaining more detailed information regarding hybrids (especially when older generation hybrids are to be identified), a larger set of nuclear loci is recommended.
Microsatellites and AIMs are very useful tools for identification of admixed individuals. The main drawback, however, is their lower effectiveness for identification of hybrids originating from older generations. While efficiency of correct identification of F1 and F2 hybrids is close to 100%, first generation backcrosses and admixed individuals bearing traces of older hybridization events are much harder to distinguish using these markers (Vähä and Primmer, 2006; Godinho et al., 2015; Korablev et al., 2021). For more detailed studies regarding hybridization events from past generations SNPs are more suitable markers.
Single Nucleotide Polymorphisms
In 2013, vonHoldt et al. used SNP array for genotyping ∼48 k SNPs in the sample including 85 dog breeds (912 individuals) and 155 non-admixed gray wolves. Main aim of the study was to provide a small panel of AIMs based on SNPs to facilitate identification of admixed individuals for i.a., monitoring programs. Based on PCA conducted in Eigenstrat (Price et al., 2006), the authors chose the most differentiating SNPs in order to construct sets comprising 100 and 24 SNPs. To test the ability of the subsets for correct assignments of admixed individuals, they compared results from identification of empirical samples and simulated genotypes. Using a model based approach, the subset of 100 SNPs was able to correctly assign 98.7% of F1 hybrids. Classification of backcrosses was less effective, with correct assignment of 91.1% of dog backcrosses and 71.9% of wolf backcrosses. The minor subset of 24 SNPs was effective only for identification of F1 hybrids (97.5%). Therefore, effectiveness of the subsets is similar to AIMs chosen by Godinho et al. (2015). On the other hand, the subsets were much more effective when implementing hybrid identification based on the plot of dosage and heterozygosity differences. Various hybrid classes were characterized by different proportions of heterozygous loci and such inferring allowed for identification to 3rd generation (F3) with no less than 87% correct assignments (vonHoldt et al., 2013). Such efficiency is much more reasonable and would be very efficient for cost—efficient identification, providing more reliable results than STR AIMs. However, European wolf representation in the sample is significantly lower than American wolves, and the subsets might be more efficient in North America than for European monitoring programs.
Galaverni et al. (2017) implemented the Canine microarray for genotyping ∼170 k SNPs from 118 wolves, 31 dogs and 72 putative hybrids in order to provide information regarding hybridization events from older generations. Hybrids were identified in ADMIXTURE (Alexander et al., 2009) and THREEPOP (TREEMIX package 1.12; Pickrell and Pritchard, 2012), both yielding similar results – 92 possible hybrids for which the time of hybridization was estimated up to 19 generations before sampling (mainly three to four generations back). On the other hand, recent hybridization was found in only ∼8% of individuals, similarly to other studies from Italy (Verardi et al., 2006; Caniglia et al., 2014). The small panels of AIMs (48, 96, and 192) chosen from all implemented SNPs were more efficient in further hybrid identification than the 39 microsatellites used in other studies. While they were unable to clearly distinguish some of the admixed individuals from wolves, they did identify most of the hybrids correctly, providing a useful tool for hybrid identification in monitoring programs. Interestingly, the authors also managed to identify genomic regions which were connected to a few phenotypic traits (black coat, white claws and presence of spur on the hind leg).
Similarly, Harmoinen et al. (2020) conducted research in order to construct a small SNPs panel for identification of wolf-dog hybrids based on non-invasive samples. The authors analyzed 197 gray wolf samples from different countries (Table 1), 40 dogs, 12 known wolf-dog hybrids, three golden jackals and three foxes. Moreover, for statistical analyses, 95 more wolves were included from Italy (70) and Iberian Peninsula (25) and 274 dogs. Genotyping of all samples was characterized by a high successful rate (min. 0.93 for scats) and results for non-invasive and invasive samples from the same individuals were highly consistent. In the end, a panel consisted of 93 SNPs with the higher discriminating power (100% for F1 and F2 99% for backcrosses with wolf and 81–92% for second and third generation backcrosses with wolf) and high genotyping success. While the panel is a useful tool for monitoring programs and facilitates fast and cost-efficient hybrid identification, it is constructed for identification of hybrids living in the wild-distinguishing hybrids in dog populations is less efficient and should be avoided (Harmoinen et al., 2020).
Another study based on SNP was conducted by Pilot et al. (2018). The authors genotyped ∼ 61 k SNPs from 54 Eastern European wolves, 20 Italian wolves, six Iberian wolves, 17 putative wolf–dog hybrids, 28 Asian wolves and 127 dogs used in another study (vonHoldt et al., 2010). STRUCTURE and ADMIXTURE were used to find signs of dog ancestry. Ancestry block analysis was performed in LAMP (Sankararaman et al., 2008) in order to estimate the admixture status of putative hybrids and then replicated using PCAdmix (Brisbin et al., 2012) for accuracy assessment. The advantage of LAMP is that it does not require information about which individuals are not admixed. One of the European wolves was identified as an F1 hybrid, 11 as F2/F3 and eight as possible backcrosses. Importantly, the identification of hybrids from F1 to F3 was possible, but the subsequent identification of older generation admixtures was not. Based on ancestry block analyses, less than 5% of the SNPs found in the autosomes in the great majority of the wolves were derived from dogs. On the other hand, 62% of the genotyped wolves had small chromosomal blocks originating from dogs, which, however, could have resulted from older hybridization events. Therefore, there is no significant introgression of dogs genes into the wild wolf populations, but a small quantity of dogs genes might be found in the majority of cases. Results of the study suggest that WDH has been taking place for a long time and is not a recent sensation. What is also worth highlighting is confirmation for higher SNPs sensitivity in contrast to small microsatellites sets. While in previous studies 11 individuals were classified as admixed, in the research conducted by Pilot et al. (2018) based on SNPs widely distributed across genomes they were not significantly different than other wolves from the dataset.
Discussion
To summarize the most important conclusions drawn by the authors of the above studies: (i) hybrid identification based on phenotype is not reliable, and even individual wolves with morphological traits suggesting hybridization should be classified on the basis of genetic markers (Galaverni et al., 2017; Kusak et al., 2018) (ii) because of the asymmetrical character of WDH, the wolf dog hybrid identification should be based on biparental markers (e.g., Vilà et al., 2003; Hindrikson et al., 2012) (iii) the great majority of studies suggested a low proportion or complete lack of admixed individuals suggesting no threat to the gray wolf’s genetic integrity in both, small and widely distributed populations (i.a., Randi and Lucchini, 2002; Ramirez et al., 2006; Verardi et al., 2006; Godinho et al., 2007, 2011; Iacolina et al., 2010; Caniglia et al., 2014; Lorenzini et al., 2014; Dufresnes et al., 2019; Korablev et al., 2021; Smeds et al., 2021). However, there were a few exceptions in Europe-small, regional populations where the number of admixed individuals was significantly higher (Bassi et al., 2017; Salvatori et al., 2019; Santostasi et al., 2021). For these cases further studies and monitoring are recommended in order to provide more information and limit potential negative impact on neighboring wolf populations (iv) small sets of STR AIMs have proved to be a reliable tool for identification of hybrids derived from recent hybridization events, outperforming slightly higher numbers of nuclear loci. In the case of solely identification of recently admixed individuals, small STRs sets composed based on their power for distinguishing between wolves and dogs are enough. Nevertheless, for more detailed information regarding the hybrid types, a higher number of microsatellites is recommended (Godinho et al., 2015) or implementing SNPs as more sensitive markers v) SNPs provided more detailed information about WDH in the past owing to its greater sensitivity and ability to detect the incidence of hybridization many generations prior to the sampled individuals (Galaverni et al., 2017). Therefore, SNPs are more suitable markers for studying hybridization events from older generations. Furthermore, as they are more precise than analyses based on microsatellite loci (Pilot et al., 2018), it is recommended that they should be included in the methodology of future WDH studies—especially, taking into consideration their effectiveness even when the study is based on non-invasive samples (Harmoinen et al., 2020). This approach may yield results different from those obtained with nuclear loci and may alter the perception of WDH in gray wolf conservation. Moreover, it is much more practical for population monitoring and management.
Wolf-dog hybridization studies are characterized by a few problematic aspects regarding methodology and results interpretation. Firstly, some of the studies presented in this review were based on opportunistically or generally non-randomly collected samples (i.a., Andersone et al., 2002; Verardi et al., 2006; Hindrikson et al., 2012; Lorenzini et al., 2014). One can argue that inferring about the wolf populations based on such samples is unreliable, and to draw such conclusions analyses should be conducted on more statistically appropriately collected samples. While one would partially agree with such a statement, and results provided by such researches are extremely valuable, on the other hand the wolf biology does not cooperate very well with such methodology as was already pointed out by Lovari et al. (2007) or Lorenzini et al. (2014). As the wolf is a highly mobile species, which is under protection in numerous countries and thrives in large areas, conducting a research, where samples are collected representatively and completely randomly is at least troublesome. Moreover, every information that may be gathered might provide important knowledge and facilitate our progress on the way to a WDH understanding. Therefore, while it is important to provide the most well-grounded results, it is equally important to take into consideration information from studies based on less representatively collected samples. Nevertheless, there is an undeniable necessity of conducting more detailed monitoring in numerous European regions in order to provide more detailed knowledge regarding the actual state of WDH in the continent (see Caniglia et al., 2020; Salvatori et al., 2020).
The second problematic factor might be results interpretation in some specific situations. For instance, Andersone et al. (2002) and Hindrikson et al. (2012) studied Latvian and Estonian wolf populations and both indicated higher than usual number of admixed individuals. Nevertheless, in both studies the authors highlighted that some of them were closely related or even came from the same litter. Sometimes other authors reference those results as confirming wider occurrence of hybridization in those countries (e.g., Lorenzini et al., 2014; Torres et al., 2017). However, in Hindrikson et al. (2012) eight hybrids were detected, while at least half of them (if no more) are full siblings and could originate from a single hybridization event. Similarly, in Andersone et al. (2002) 12 hybrids were identified including a litter and its mother, which constituted seven of all the hybrids. While such numbers are significant taking into consideration the number of all samples, calculated proportions are not fully representative for the wolf populations in those countries. Therefore, it is crucial to include such information in speculations regarding the extent of WDH because such results are ambiguous and do not necessarily mean a high proportion of hybrids in studied populations.
Last but not least, defining reliable thresholds and suggesting the most efficient management actions. Thresholds for identifying individuals as admixed differ between studies (e.g., between Lorenzini et al., 2014; Godinho et al., 2015; Salvatori et al., 2019) but minor changes in their values may have significant impact on the final proportion of admixed individuals detected (Lorenzini et al., 2014). Caniglia et al. (2020) defined three categories for assigning individuals in hybridization studies and species monitoring—pure individuals, older admixed individuals and recent admixed individuals (membership proportion for the wolf cluster: qw > 0.995; 0.955 < qw < 0.995; qw < 0.955 respectively, based on 39 STRs). Based on particular categories, the authors defined management priority for them. Such approach surely facilitates not only decision making for conservation actions, but also provides a standardized approach for studies regarding WDH. Unified categorization of admixed individuals will allow for comparison of results from different countries and more reliable inferences regarding widely spread populations of the gray wolf in Europe. Another advantage of the approach suggested by the authors is establishing threshold values also for a smaller set of STRs (12), which are more likely to be used for monitoring programs (for more details regarding management suggestions based on the mentioned categorization see Caniglia et al., 2020).
Conclusion
Beginning of the 2000s and quick development of genetics provided a well-grounded methodology for studies regarding WDH. Initially, scientists were focused mainly on implementation of different methodology and its comparison and defining the proportion of admixed individuals in particular populations. Numerous problems were encountered during the last two decades [marker selection, implementation of suitable and reliable software, defining thresholds for efficient hybrid identification in order to avoid Type I and Type II errors (i.e., classification of pure individuals as hybrids and hybrids as pure individuals respectively)]. Having all the knowledge obtained through the last 20 years, now is the time to unify methodology of hybrid detection and lean toward its wide application in conservation and population management (Caniglia et al., 2020; Salvatori et al., 2020). Numerous wolf populations are still in a need for defining the proportion of admixed individuals and that can be reliably done only using unified, reliable methodology implemented widely by European countries in statistically appropriate monitoring programs. Only knowledge obtained through this well-grounded methodology will be an appropriate base for management decisions.
Author Contributions
The author confirms sole responsibility for study conception and design, data collection, analysis and interpretation of results, and manuscript preparation.
Conflict of Interest
The author declares that the research was conducted in the absence of any commercial or financial relationships that could be construed as a potential conflict of interest.
Publisher’s Note
All claims expressed in this article are solely those of the authors and do not necessarily represent those of their affiliated organizations, or those of the publisher, the editors and the reviewers. Any product that may be evaluated in this article, or claim that may be made by its manufacturer, is not guaranteed or endorsed by the publisher.
Acknowledgments
The author would like to thank dr hab. H. Wierzbicki (Wrocław University of Environmental and Life Sciences) for all suggestions and supervision over the article.
Supplementary Material
The Supplementary Material for this article can be found online at: https://www.frontiersin.org/articles/10.3389/fevo.2021.760160/full#supplementary-material
Footnotes
- ^ https://scholar.google.com/
- ^ https://www.researchgate.net/
- ^ https://www.webofscience.com/wos/woscc/basic-search
- ^ https://www2.unil.ch/popgen/softwares/pcagen.htm
- ^ http://evolution.genetics.washington.edu/phylip.html
References
Alexander, D. H., Novembre, J., and Lange, K. (2009). Fast model-based estimation of ancestry in unrelated individuals. Genome Res. 19, 1655–1664. doi: 10.1101/gr.094052.109
Allendorf, F. W., and Leary, R. F. (1988). Conservation and distribution of genetic variation in a polytypic species, the cutthroat trout. Conserv. Biol. 2, 170–184.
Allendorf, F. W., Leary, R. F., Spruell, P., and Wenburg, J. K. (2001). The problems with hybrids: setting conservation guidelines. Trends Ecol. Evol. 16, 613–622. doi: 10.1016/S0169-5347(01)02290-X
Anderson, E., and Thompson, E. (2002). A model-based method for identifying species hybrids using multilocus genetic data. Genetics 160, 1217–1229.
Anderson, T., von Holdt, B., Candille, S., Musiani, M., Greco, C., Stahler, D., et al. (2009). Molecular and Evolutionary History of Melanism in North American Gray Wolves. Science 323, 1339–1343. doi: 10.1126/science.1165448
Andersone, Z., Lucchini, V., Randi, E., and Ozolins, J. (2002). Hybridisation between wolves and dogs in Latvia as documented using mitochondrial and microsatellite DNA markers. Mamm. Biol. 67, 79–90. doi: 10.1078/1616-5047-00012
Bassi, E., Canu, A., Firmo, I., Mattioli, L., Scandura, M., and Apollonio, M. (2017). Trophic overlap between wolves and free-ranging wolf×dog hybrids in the Apennine Mountains. Italy. Global Ecol. Conserv. 9, 39–49. doi: 10.1016/j.gecco.2016.11.002
Becker, M., Gruenheit, N., Steel, M., Voelckel, C., Deusch, O., Heenan, P. B., et al. (2013). Hybridization may facilitate in situ survival of endemic species through periods of climate change. Nat. Clim. Change 3, 1039–1043. doi: 10.1038/nclimate2027
Belkhir, K., Borsa, P., Chikhi, L., Raufauste, N., and Bonhomme, F. (2001). GENETIX, Logiciel Sous Windows TM Pour la Génétique des Populations. Laboratoire Génome, Populations, Interactions CNRS UMR 5000. Montpellier, France: Université de Montpellier II.
Berger, L. (1973). Systematics and Hybridization in European Green Frogs of Rana esculenta Complex. J. Herpetol. 7, 1–10. doi: 10.2307/1562822
Bern Convention (1979). Convention on the Conservation of European Wildlife and Natural Heritage. Strasbourg: Council of Europe.
Besnier, F., and Glover, K. A. (2013). ParallelStructure: a R package to distribute parallel runs of the population genetics program STRUCTURE on multi-core computers. PLoS One 8:e70651. doi: 10.1371/journal.pone.0070651
Beyer, H. L., Merril, E. H., Varley, N., and Boyce, M. S. (2007). Willow on Yellowstone’s northern range: evidence for a trophic cascade? Ecol. Appl. 17, 6, 1563–1571. doi: 10.1890/06-1254.1
Bibikov, D. (1982). “Wolf ecology and management in the USSR,” in Wolves of the World. Perspectives of Behavior, Ecology and Conservation, eds F. H. Harrington and P. C. Paquet (New Jersey: Noyes Publications), 120–133.
Blanco, J. C., Reig, S., and Cuesta, L. (1992). Distribution, status and conservation problems of the wolf Canis lupus in Spain. Biol. Conserv. 60, 73–80. doi: 10.1016/0006-3207(92)91157-N
Bohling, J., Adams, J., and Waits, L. (2013). Evaluating the ability of Bayesian clustering methods to detect hybridization and introgression using an empirical red wolf data set. Mol. Ecol. 22, 74–86. doi: 10.1111/mec.12109
Brisbin, A., Bryc, K., Byrnes, J., Zakharia, F., Omberg, L., Degenhardt, J., et al. (2012). PCAdmix: principal components-based assignment of ancestry along each chromosome in individuals with admixed ancestry from two or more populations. Hum. Biol. 84, 343–364. doi: 10.3378/027.084.0401
Caniglia, R., Fabbri, E., Galaverni, M., Milanesi, P., and Randi, E. (2014). Noninvasive sampling and genetic variability, pack structure, and dynamics in an expanding wolf population. J. Mamm. 95, 41–59. doi: 10.1644/13-mamm-a-039
Caniglia, R., Fabbri, E., Greco, C., Galaverni, M., Manghi, L., Boitani, L., et al. (2013). Black coats in an admixed wolf × dog pack is melanism an indicator of hybridization in wolves? Eur. J. Wildlife Res. 59, 543–555. doi: 10.1007/s10344-013-0703-1
Caniglia, R., Galaverni, M., Velli, E., Mattucci, F., Canu, A., Apollonio, M., et al. (2020). A standardized approach to empirically define reliable assignment thresholds and appropriate management categories in deeply introgressed populations. Sci. Rep. 10:2862. doi: 10.1038/s41598-020-59521-2
CITES (1973). Convention on International Trade in Endangered Species of Wild Fauna and Flora. Geneva: UNEP.
Clarke, B., Johnson, M. S., Murray, J., Hewitt, G., and Wragg, G. M. (1996). Clines in the genetic distance between two species of island land snails: how ‘Molecular Leakage’ can mislead us about speciation. Philos. Trans. R. Soc. B Biol. Sci. 351, 773–780. doi: 10.1098/rstb.1996.0072
Dagilis, A. J., Kirkpatrick, M., and Bolnick, D. I. (2019). The evolution of hybrid fitness during speciation. PLoS Genet. 15:e1008125. doi: 10.1371/journal.pgen.1008125
Duchesne, P., and Turgeon, J. (2009). FLOCK: a method for quick mapping of admixture without source samples. Mol. Ecol. Res. 9, 1333–1344. doi: 10.1111/j.1755-0998.2009.02571.x
Dufresnes, C., Remollino, N., Stoffel, C., Manz, R., Weber, J.-M., and Fumagalli, L. (2019). Two decades of non-invasive genetic monitoring of the grey wolves recolonizing the Alps support very limited dog introgression. Sci. Rep. 9:148. doi: 10.1038/s41598-018-37331-x
Ellegren, H. (2004). Microsatellites: simple sequences with complex evolution. Nat. Rev. Genet. 5, 435–445. doi: 10.1038/nrg1348
European Communities [EC] (1992). Council Directive 92/43/EEC of 21 May 1992 on the Conservation of Natural Habitats and of Wild Fauna and Flora. Brussels: European Communities.
Fabbri, E., Miquel, C., Lucchini, V., Santini, A., Caniglia, R., Duchamp, C., et al. (2007). From the Apennines to the Alps: colonization genetics of the naturally expanding Italian wolf (Canis lupus) population. Mol. Ecol. 16, 1661–1671. doi: 10.1111/j.1365-294x.2007.03262.x
Falush, D., Stephens, M., and Pritchard, J. K. (2003). Inference of population structure using multilocus genotype data: linked loci and correlated allele frequencies. Genetics 164, 1567–1587.
Francisco, L. V., Langsten, A. A., Mellersh, C. S., Neal, C. L., and Ostrander, E. A. (1996). A class of highly polymorphic tetranucleotide repeats for canine genetic mapping. Mamm. Genome 7, 359–362. doi: 10.1007/s003359900104
Galaverni, M., Caniglia, R., Pagani, L., Fabbri, E., Boattini, A., and Randi, E. (2017). Disentangling Timing of Admixture, Patterns of Introgression, and Phenotypic Indicators in a Hybridizing Wolf Population. Mol. Biol. Evol. 34, 2324–2339. doi: 10.1093/molbev/msx169
Godinho, R., Llaneza, L., Blanco, J. C., Lopes, S., Álvares, F., García, E. J., et al. (2011). Genetic evidence for multiple events of hybridization between wolves and domestic dogs in the Iberian Peninsula. Mol. Ecol. 20, 5154–5166. doi: 10.1111/j.1365-294x.2011.05345.x
Godinho, R., Lopes, S., and Ferrand, N. (2007). Estudo da Diversidade e Estruturação Genética das Populações de Lobo (Canis lupus) em Portugal. Relatório Final. CIBIO/UP. 56.
Godinho, R., López-Bao, J. V., Castro, D., Llaneza, L., Lopes, S., Silva, P., et al. (2015). Real-time assessment of hybridization between wolves and dogs: combining noninvasive samples with ancestry informative markers. Mol. Ecol. Res. 15, 317–328. doi: 10.1111/1755-0998.12313
Gompert, Z., and Buerkle, C. A. (2016). What, if anything, are hybrids: enduring truths and challenges associated with population structure and gene flow. Evol. Appl. 9, 909–923. doi: 10.1111/eva.12380
Gopalakrishnan, S., Sinding, M.-H. S., Ramos-Madrigal, J., Niemann, J., Samaniego Castruita, J. A., Vieira, F. G., et al. (2018). Interspecific gene flow shaped the evolution of the genus Canis. Curr. Biol. 28, 3441–3449.e5. doi: 10.1016/j.cub.2018.08.041
Grudzien, T. A., Moore, W. S., Cook, J. R., and Tagle, D. (1987). Genic population structure and gene flow in the Northern Flicker (Colaptes auratus) hybrid zone. Auk 104, 654–664.
Halder, I., Shriver, M., Thomas, M., Fernandez, J. R., and Frudakis, T. (2008). A panel of ancestry informative markers for estimating individual biogeographical ancestry and admixture from four continents: utility and applications. Hum. Mutat. 29, 648–658. doi: 10.1002/humu.20695
Harmoinen, J., Von Thaden, A., Aspi, J., Kvist, L., Cocchiararo, B., Jarausch, A., et al. (2020). Reliable Wolf-Dog hybrid detection in Europe using a reduced SNP panel developed for non-invasively collected samples. Res. Square [Preprint]. doi: 10.21203/rs.3.rs-113866/v1
Hayward, M., and Somers, M. (2009). Reintroduction of Top-Order Predators. Hoboken: Blackwell Publishing Ltd.
Hindrikson, M., Männil, P., Ozolins, J., Krzywinski, A., and Saarma, U. (2012). Bucking the trend in Wolf-Dog hybridization: first evidence from Europe of hybridization between female dogs and male wolves. PLoS One 7:e46465. doi: 10.1371/journal.pone.0046465
Hindrikson, M., Remm, J., Pilot, M., Godinho, R., Stronen, A. V., Baltrūnaité, L., et al. (2017). Wolf population genetics in Europe: a systematic review, meta-analysis and suggestions for conservation and management. Biol. Rev. 92, 1601–1629. doi: 10.1111/brv.12298
Holmes, N. G., Humphreys, S. J., Binns, M. M., Holliman, A., Curtis, R., Mellersh, C. S., et al. (1993). Isolation and characterization of microsatellites from the canine genome. Anim. Genet. 24, 289–292. doi: 10.1111/j.1365-2052.1993.tb00313.x
Hulva, P., Černá Bolfíková, B., Woznicová, V., Jindřichová, M., Benešová, M., Mysłajek, R. W., et al. (2018). Wolves at the crossroad: fission-fusion range biogeography in the Western Carpathians and Central Europe. Divers. Distrib. 24, 179–192. doi: 10.1111/ddi.12676
Iacolina, L., Scandura, M., Gazzola, A., Cappai, N., Capitani, C., Mattioli, L., et al. (2010). Y-chromosome microsatellite variation in Italian wolves: a contribution to the study of wolf-dog hybridization patterns. Mamm. Biol. 75, 341–347. doi: 10.1016/j.mambio.2010.02.004
Jombart, T., and Ahmed, I. (2011). adegenet 1.3-1: new tools for the analysis of genome-wide SNP data. Bioinformatics 27, 3070–3071. doi: 10.1093/bioinformatics/btr521
Jombart, T., Devillard, S., and Balloux, F. (2010). Discriminant analysis of principal components: a new method for the analysis of genetically structured populations. BMC Genet. 11:94. doi: 10.1186/1471-2156-11-94
Karamanlidis, A., Czarnomska, S., Kopatz, A., Georgiadis, L., and Jędrzejewska, B. (2016). Wolf population genetics at the south-eastern edge of their European range. Mamm. Biol. 81, 506–510. doi: 10.1016/j.mambio.2016.06.007
Korablev, M. P., Korablev, N. P., and Korablev, P. N. (2021). Genetic diversity and population structure of the grey wolf (Canis lupus Linnaeus, 1758) and evidence of wolf × dog hybridisation in the centre of European Russia. Mamm. Biol. 101, 91–104. doi: 10.1007/s42991-020-00074-2
Kumar, S., Tamura, K., Jakobsen, I. B., and Nei, M. (2001). MEGA2: molecular evolutionary genetics analysis software. Bioinformatics 17, 1244–1245. doi: 10.1093/bioinformatics/17.12.1244
Kusak, J., Fabbri, E., Galov, A., Gomerčič, T., Arbanasič, H., Caniglia, R., et al. (2018). Wolf-dog hybridization in Croatia. Vet. Arh. 88, 375–395. doi: 10.24099/vet.arhiv.170314
Latch, E. K., Dharmarajan, G., Glaubitz, J. C., and Rhodes, O. E. (2006). Relative performance of Bayesian clustering software for inferring population substructure and individual assignment at low levels of population differentiation. Conserv. Genet. 7, 295–302. doi: 10.1007/s10592-005-9098-1
Lorenzini, R., Fanelli, R., Grifoni, G., Scholl, F., and Fico, R. (2014). Wolf–dog crossbreeding: “Smelling” a hybrid may not be easy. Mamm. Biol. 79, 149–156. doi: 10.1016/j.mambio.2013.07.080
Lorenzini, R., and Fico, R. (1995). A genetic investigation of enzyme polymorphisms shared by wolf and dog: suggestions for conservation of the wolf in Italy. Acta Theriol. 3, 101–110.
Lovari, S., Sforzi, A., Scala, C., and Fico, R. (2007). A wolf in the hand is worth two in the bush: a response to Ciucci et al. (2007). J. Zool. 273, 128–130. doi: 10.1111/j.1469-7998.2007.00380.x
Milenković, M., Habijan-Mikeš, V., and Matić, R. (2006). Cases of spontaneous interbreeding of wolf and domestic dog in the region of southeast Banat (Serbia). Arch. Biol. Sci. 58, 225–231. doi: 10.2298/ABS0604225M
Moura, A. E., Tsingarska, E., Dąbrowski, M. J., Czarnomska, S. D., Jędrzejewska, B., and Pilot, M. (2014). Unregulated hunting and genetic recovery from a severe population decline: the cautionary case of Bulgarian wolves. Conserv. Genet. 15, 405–417. doi: 10.1007/s10592-013-0547-y
Muñoz-Fuentes, V., Darimont, C. T., Paquet, P. C., and Leonard, J. A. (2010). The genetic legacy of extirpation and re-colonization in Vancouver Island wolves. Conserv. Genet. 11, 547–556. doi: 10.1007/s10592-009-9974-1
Neophytou, C. (2014). Bayesian clustering analyses for genetic assignment and study of hybridization in oaks: effects of asymmetric phylogenies and asymmetric sampling schemes. Tree Genet. Genomes 10, 273–285. doi: 10.1007/s11295-013-0680-2
Nielsen, E. E., Bach, L. A., and Kotlicki, P. (2006). hybridlab (version 1.0): a program for generating simulated hybrids from population samples. Mol. Ecol. Notes 6, 971–973. doi: 10.1111/j.1471-8286.2006.01433.x
Ostrander, E. A., Sprague, G. F. Jr., and Rine, J. (1993). Identification and characterization of dinucleotide repeat (CA)n markers for genetic mapping in dog. Genomics 16, 207–213. doi: 10.1006/geno.1993.1160
Pacheco, C., López-Bao, J. V., García, E. J., Lema, F. J., Llaneza, L., Palacios, V., et al. (2017). Spatial assessment of wolf-dog hybridization in a single breeding period. Sci. Rep. 7:42475. doi: 10.1038/srep42475
Paetkau, D., Calbert, W., Stirling, I., and Strobeck, C. (1995). Microsatellite analysis of population structure in Canadian polar bears. Mol. Ecol. 4, 347–354. doi: 10.1111/j.1365-294x.1995.tb00227.x
Paetkau, D., Shields, G. F., and Strobeck, C. (1998). Gene flow between insular, coastal and interior populations of brown bears in Alaska. Mol. Ecol. 7, 1283–1292.
Peakall, R., and Smouse, P. E. (2012). GenAlEx 6.5: genetic analysis in Excel. Population genetic software for teaching and research -an update. Bioinf. Appl. Note 28, 2537–2539. doi: 10.1093/bioinformatics/bts460
Pickrell, J. K., and Pritchard, J. K. (2012). Inference of Population Splits and Mixtures from Genome-Wide Allele Frequency Data. PLoS Genet. 8:e1002967. doi: 10.1371/journal.pgen.1002967
Pilot, M., Greco, C., Vonholdt, B. M., Randi, E., Jędrzejewski, W., Sidorovich, V. E., et al. (2018). Widespread, long-term admixture between grey wolves and domestic dogs across Eurasia and its implications for the conservation status of hybrids. Evol. Appl. 11, 662–680. doi: 10.1111/eva.12595
Piry, S., Alapetite, A., Cornuet, J.-M., Paetkau, D., Baudouin, L., and Estoup, A. (2004). GENECLASS2: a software for genetic assignment and first-generation migrant detection. J. Heredity 95, 536–539. doi: 10.1093/jhered/esh074
Price, A., Patterson, N., Plenge, R., Weinblatt, M., Shadick, N., and Reich, D. (2006). Principal components analysis corrects for stratification in genome-wide association studies. Nat. Genet. 38, 904–909. doi: 10.1038/ng1847
Pritchard, J. K., Stephens, M., and Donnelly, P. (2000). Inference of Population Structure Using Multilocus Genotype Data. Genetics 155, 945–959. doi: 10.1093/genetics/155.2.945
Purcell, S., Neale, B., Todd-Brown, K., Thomas, L., Ferreira, M. A. R., Bender, D., et al. (2007). PLINK: a tool set for whole-genome association and population-based linkage analyses. Am. J. Hum. Genet. 81, 559–575. doi: 10.1086/519795
Ramirez, O., Altet, L., Enseñat, C., Vilà, C., Sanchez, A., and Ruiz, A. (2006). Genetic assessment of the Iberian wolf Canis lupus signatus captive breeding program. Conserv. Genet. 7, 861–878. doi: 10.1007/s10592-006-9123-z
Randi, E. (1993). Effects of fragmentation and isolation on genetic variability of the Italian populations of wolf Canis lupus and brown bear Ursus arctos. Acta Theriol. 38, 113–120. doi: 10.4098/at.arch.93-46
Randi, E. (2008). Detecting hybridization between wild species and their domesticated relatives. Mol. Ecol. 17, 285–293. doi: 10.1111/j.1365-294X.2007.03417.x
Randi, E., Hulva, P., Fabbri, E., Galaverni, M., Galov, A., Kusak, J., et al. (2014). Multilocus Detection of Wolf x Dog hybridization in Italy, and guidelines for marker selection. PLoS One 9:e86409. doi: 10.1371/journal.pone.0086409
Randi, E., and Lucchini, V. (2002). Detecting rare introgression of domestic dog genes into wild wolf (Canis lupus) populations by Bayesian admixture analyses of microsatellite variation. Conserv. Genet. 3, 29–43. doi: 10.1023/a:1014229610646
Randi, E., Lucchini, V., Christensen, M. F., Mucci, N., Funk, S. M., Dolf, G., et al. (2000). Mitochondrial DNA variability in Italian and east European wolves: detecting the consequences of small population size and hybridization. Conserv. Biol. 14, 464–473. doi: 10.1046/j.1523-1739.2000.98280.x
Rhymer, J. M., and Simberloff, D. (1996). Extinction by hybridization and introgression. Ann. Rev. Ecol. Syst. 27, 83–109. doi: 10.1146/annurev.ecolsys.27.1.83
Ripple, W. J., Larsen, E. J., Renkin, R. A., and Smith, D. W. (2001). Trophic cascades among wolves, elk and aspen on Yellowstone National Park’s northern range. Biol. Conserv. 102, 227–234. doi: 10.1016/s0006-3207(01)00107-0
Runyon, M. J., Tyers, D. B., Sowell, B. F., and Gower, C. N. (2014). Aspen restoration using beaver on the Northern Yellowstone Winter Range under reduced ungulate Herbivory. Restorat. Ecol. 22, 555–561. doi: 10.1111/rec.12105
Saitou, N., and Nei, M. (1987). The neighbor-joining method: a new method for reconstructing phylogenetic trees. Mol. Biol. Evol. 4, 406–425. doi: 10.1093/oxfordjournals.molbev.a040454
Salvatori, V., Donfrancesco, V., Trouwborst, A., Boitani, L., Linnell, J. D. C., Alvares, F., et al. (2020). European agreements for nature conservation need to explicitly address wolf-dog hybridisation. Biol. Conserv. 248:108525. doi: 10.1016/j.biocon.2020.108525
Salvatori, V., Godinho, R., Braschi, C., Boitani, L., and Ciucci, P. (2019). High levels of recent wolf × dog introgressive hybridization in agricultural landscapes of central Italy. Eur. J. Wildlife Res. 65:73. doi: 10.1007/s10344-019-1313-3
Sankararaman, S., Sridhar, S., Kimmel, G., and Halperin, E. (2008). Estimating local ancestry in admixed populations. Am. J. Hum. Genet. 82, 290–303. doi: 10.1016/j.ajhg.2007.09.022
Santostasi, N. L., Gimenez, O., Caniglia, R., Fabbri, E., Molinari, L., Reggioni, W., et al. (2021). Estimating admixture at the population scale: taking imperfect detectability and uncertainty in hybrid classification seriously. J. Wildlife Manag. 85, 1031–1046. doi: 10.1002/jwmg.22038
Selkoe, K. A., and Toonen, R. J. (2006). Microsatellites for ecologists: a practical guide to using and evaluating microsatellite markers. Ecol. Lett. 9, 615–629. doi: 10.1111/j.1461-0248.2006.00889.x
Simberloff, D. (1996). Hybridization between native and introduced wildlife species: importance for conservation. Wildlife Biol. 2, 143–150. doi: 10.2981/wlb.1996.012
Smeds, L., Aspi, J., Berglund, J., Kojola, I., Tirronen, K., and Ellegren, H. (2021). Whole-genome analyses provide no evidence for dog introgression in Fennoscandian wolf populations. Evol. Appl. 14, 721–734. doi: 10.1111/eva.13151
Smith, D. W., and Tyers, D. B. (2012). The History and Current Status and Distribution of Beavers in Yellowstone National Park. Northw. Sci. 86, 276–88. doi: 10.3955/046.086.0404
Spillman, W. J., Simpson, Q. I., Kennedy, W. J., Castle, W. E., Mumford, F. B., Fogle, P. E., et al. (1910). Report of committee on animal hybrids. J. Heredity 1, 193–196.
Stahler, D., Heinrich, B., and Smith, D. (2002). Common ravens, Corvus corax, preferentially associate with grey wolves, Canis lupus, as a foraging strategy in winter. Anim. Behav. 64, 283–290. doi: 10.1006/anbe.2002.3047
Torres, R. T., Ferreira, E., Rocha, R. G., and Fonseca, C. (2017). Hybridization between wolf and domestic dog: first evidence from an endangered population in central Portugal. Mamm. Biol. 86, 70–74. doi: 10.1016/j.mambio.2017.05.001
Vähä, J.-P., and Primmer, C. R. (2006). Efficiency of model-based Bayesian methods for detecting hybrid individuals under different hybridization scenarios and with different numbers of loci. Mol. Ecol. 15, 63–72. doi: 10.1111/j.1365-294x.2005.02773.x
Verardi, A., Lucchini, V., and Randi, E. (2006). Detecting introgressive hybridization between free-ranging domestic dogs and wild wolves (Canis lupus) by admixture linkage disequilibrium analysis. Mol. Ecol. 15, 2845–2855. doi: 10.1111/j.1365-294x.2006.02995.x
Vilà, C., Savolainen, P., Maldonado, J., Amorim, I., Rice, J., Honeycutt, R., et al. (1997). Multiple and Ancient Origins of the Domestic Dog. Science 276, 1687–1689. doi: 10.1126/science.276.5319.1687
Vilà, C., Walker, C., Sundqvist, A.-K., Flagstad, Ø, Andersone, Z., Casulli, A., et al. (2003). Combined use of maternal, paternal and bi-parental genetic markers for the identification of wolf–dog hybrids. Heredity 90, 17–24. doi: 10.1038/sj.hdy.6800175
Vilà, M., Weber, E., and Antonio, C. M. (2000). Conservation implications of invasion by plant hybridization. Biol. Invasions 2, 207–217. doi: 10.1023/a:1010003603310
vonHoldt, B. M., Pollinger, J. P., Earl, D. A., Parker, H. G., Ostrander, E. A., and Wayne, R. K. (2013). Identification of recent hybridization between gray wolves and domesticated dogs by SNP genotyping. Mamm. Genome 24, 80–88. doi: 10.1007/s00335-012-9432-0
vonHoldt, B. M., Pollinger, J. P., Lohmueller, K. E., Han, E., Parker, H. G., Quignon, P., et al. (2010). Genome-wide SNP and haplotype analyses reveal a rich history underlying dog domestication. Nature 464, 898–902. doi: 10.1038/nature08837
Waser, P. M., and Strobeck, C. (1998). Genetic signatures of interpopulation dispersal. Trends Ecol. Evol. 13, 43–44. doi: 10.1016/s0169-5347(97)01255-x
Wilmers, C. C., Crabtree, R. L., Smith, D. W., Murphy, K. M., and Getz, W. M. (2003a). Trophic facilitation by introduced top predators: grey wolf subsidies to scavengers in Yellowstone National Park. J. Anim. Ecol. 72, 909–916. doi: 10.1046/j.1365-2656.2003.00766.x
Wilmers, C. C., Stahler, D. R., Crabtree, R. L., Smith, D. W., and Getz, W. M. (2003b). Resource dispersion and consumer dominance: scavenging at wolf- and hunter-killed carcasses in Greater Yellowstone, USA. Ecol. Lett. 6, 996–1003. doi: 10.1046/j.1461-0248.2003.00522.x
Keywords: Bayesian clustering, Canis lupus, domestic dog, gray wolf, methodology, microsatellites, SNPs, WDH
Citation: Dziech A (2021) Identification of Wolf-Dog Hybrids in Europe – An Overview of Genetic Studies. Front. Ecol. Evol. 9:760160. doi: 10.3389/fevo.2021.760160
Received: 17 August 2021; Accepted: 07 October 2021;
Published: 02 November 2021.
Edited by:
Carla Martins Lopes, São Paulo State University, Campus Rio Claro, BrazilReviewed by:
Pablo Martinez, Federal University of Sergipe, BrazilSaverio Bartolini Lucenti, University of Florence, Italy
Copyright © 2021 Dziech. This is an open-access article distributed under the terms of the Creative Commons Attribution License (CC BY). The use, distribution or reproduction in other forums is permitted, provided the original author(s) and the copyright owner(s) are credited and that the original publication in this journal is cited, in accordance with accepted academic practice. No use, distribution or reproduction is permitted which does not comply with these terms.
*Correspondence: Arkadiusz Dziech, YXJrYWRpdXN6LmR6aWVjaEB1cHdyLmVkdS5wbA==