- 1Department for the Ecology of Animal Societies, Max Planck Institute of Animal Behavior, Baden-Württemberg, Germany
- 2Centre for the Advanced Study of Collective Behaviour, University of Konstanz, Konstanz, Germany
- 3Nicholas School of the Environment, Duke University, Durham, NC, United States
- 4University Program in Ecology, Duke University, Durham, NC, United States
- 5Agence Nationale des Parcs Nationaux, Libreville, Gabon
- 6Institut de Recherche en Ecologie Tropicale, Libreville, Gabon
- 7African Forest Ecology Group, School of Natural Sciences, University of Stirling, Stirling, United Kingdom
More refined knowledge of how tropical forests respond to changes in the abiotic environment is necessary to mitigate climate change, maintain biodiversity, and preserve ecosystem services. To evaluate the unique response of diverse Afrotropical forest communities to disturbances in the abiotic environment, we employ country-wide tree species inventories, remotely sensed climate data, and future climate predictions collected from 104 1-ha plots in the central African country of Gabon. We predict a 3–8% decrease in Afrotropical forest species richness by the end of the century, in contrast to the 30–50% loss of plant diversity predicted to occur with equivalent warming in the Neotropics. This work reveals that forecasts of community species composition are not generalizable across regions, and more representative studies are needed in understudied diverse biomes. This study serves as an important counterpoint to work done in the Neotropics by providing contrasting predictions for Afrotropical forests with substantially different ecological, evolutionary, and anthropogenic histories.
Introduction
The anticipated pace of global warming is predicted to result in large declines of tropical biodiversity (Aubry-Kientz et al., 2019), leading to biotic attrition of the lowland tropics (Sala et al., 2000; van Vuuren et al., 2006; Colwell et al., 2008; Feeley et al., 2011; Hooper et al., 2012; Dexter et al., 2018, but see Feeley and Silman, 2010). Effects of climate change may be indirect (Carley et al., 2021; Clark et al., 2021; Qiu et al., 2021), and most acutely felt by long-lived organisms like trees that endure several degrees of warming within a single lifetime, without the benefit of adaptive nimbleness available to shorter lived species (Malhi et al., 2014; Clark et al., 2021). Tropical forests contain over 40,000 tree species (Slik et al., 2015), shelter over half of all animal species (Pimm and Raven, 2000), and store much of the planet’s carbon (Sullivan et al., 2017; Beirne et al., 2019; Poulsen et al., 2020) while covering only 7% of the Earth’s surface (Corlett and Primack, 2011). Despite their outsize value, tropical forests are notably understudied, and most in situ species inventory data come from a few intensively studied sites in the Neotropics (Schimel et al., 2015; but see Blundo et al., 2021). Neotropical studies demonstrate that community composition and function are degrading in response to climate change (Engelbrecht et al., 2007; Bongers et al., 2009; Poorter et al., 2017; Whitworth et al., 2021), with early successional species thriving in warmer soil temperatures at the expense of late-successional species that require cooler microhabitats (Colwell et al., 2008). These processes have contributed to predictions of 30–50% loss of plant diversity with a 5°C temperature increase for most South American tropical forests (Colwell et al., 2008; Feeley and Silman, 2010).
It is unclear whether the world’s other tropical regions will respond similarly to climate change (Malhi and Wright, 2004; Maslin et al., 2005; Parmentier et al., 2007; Malhi et al., 2013; Mayaux et al., 2013; Enquist et al., 2017; Sullivan et al., 2017). Afrotropical forests are distinct in having comparatively few wet-affiliated species given their climate (Leal, 2009), and a high proportion of large trees that grow and recolonize rapidly (Fayolle et al., 2012; Gond et al., 2013). These differences in community-level traits are hypothesized to have arisen from Africa’s unique climatic past (Haffer, 1969; Maley, 1996; Maslin et al., 2005; Oslisly et al., 2013; Willis et al., 2013). In direct contrast to the Neotropics (Colinvaux et al., 1996, 2000, 2001), abnormally cool and dry conditions during the last glacial maximum reduced Afrotropical forests to small fragmented patches (Cohen et al., 2007). This may have selected for species able to survive extreme aberrations in temperature and precipitation and then quickly disperse from refugia to recolonize the landscape cleared by receding glaciers (Leal, 2009), potentially making them more resilient to climate change than their Amazonian or Asian counterparts (Hansen and DeFries, 2004; Gardner et al., 2007). Indeed, a pair of studies comparing changes in canopy structure found few lingering effects of drought on Afrotropical forest canopy (Asefi-Najafabady and Saatchi, 2013), but did find lingering canopy effects of drought on southwest Amazonia (Saatchi et al., 2013).
Neotropical species to climate change are unlikely to be an adequate proxy for Afrotropical forests, yet no landscape-scale predictions of Afrotropical community responses to climate have been made. This work is urgently needed—the current climate of Equatorial Africa is already near the lower temperature-precipitation threshold of rainforest viability (Malhi and Wright, 2004; Pan et al., 2011), after which a rapid shift in the ecosystem could occur (Willis et al., 2013). Accurate models of forest change in the Afrotropics are dependent on sampling both historical refugia and areas of expansion. Past reviews of paleoecological studies (Maley, 1996; Bonnefille, 2007) have interpreted the high degree of endemism in western Gabon relative to surrounding areas as an indicator that this area was an climatically stable refuge for tropical plants during the last glacial maxima. However, whereas recent genetic studies of some species support this claim (Dainou et al., 2010), still others indicate that these areas were not refuges for all species (Lowe et al., 2010, see also temperate examples: Comes and Kadereit, 1998; Petit et al., 2003), highlighting a need for systematically random census data in a topographically and abiotically diverse Afrotropical region.
To evaluate how Afrotropical tree species will respond to future climate change, we model tropical forest tree species distributions using a systematic country-wide array of randomly placed tree plots, remotely sensed historic climatic data, and future climate predictions for the densely forested central African country of Gabon. This first-of-its-kind study serves as an important counterpoint to work done in the Neotropics by providing contrasting novel predictions for Afrotropical forests with substantially different ecological, evolutionary, and anthropogenic histories. We hypothesize that the disturbance-rich past of Afrotropical communities will result in two divergent responses to forecasted climate change: (1) Afrotropical community forecasts will demonstrate lower levels of species loss than the 30–50% loss predicted for the Neotropics; and (2) early-successional species will increase in number at the expense of declining abundance of late-successional species.
Materials and Methods
Tree Inventory Data
In this study, we employ tree census data from Gabon’s National Resource Inventory—a national network of tree plots for estimating forest biomass and carbon stocks (Figure 1; Carlson et al., 2017; Poulsen et al., 2017a,2020; Beirne et al., 2019; Wade et al., 2019). Gabon is the second most forested country in the world, with a forest cover of 87%, a deforestation rate near zero (Sannier et al., 2014), and one of the highest densities of carbon in Central Africa (Saatchi et al., 2011). Between 2012 and 2013, trained technicians established 104 1-ha forest plots based on a stratified random sampling design that consisted of dividing the country into 100 50 × 50 km cells and randomly locating a sample site within each of the cells (Poulsen et al., 2020). This design ensured an unbiased sampling of Gabon’s forest (40.4% old-growth, 28.8% logged, 30.8% secondary) and edaphic types (69.2% terra firma, 22.1% seasonally flooded forest, 8.6% swamp). Every tree with a diameter-at-breast height (DBH) ≥ 10 cm was mapped, measured and identified to species by trained field teams following standard protocols for plot establishment and measurement (Phillips and Baker, 2002). A total of 621 species from 296 genera were cataloged. We analyzed data for all species that occurred on at least 30 of the 104 plots, resulting in 34,460 stems representing 76 of the most widely occurring species. The five most common taxa (31% of all stems) were Santiria trimera, Dichostemma glaucescens, Plagiostyles Africana, Aucoumea klaineana, and Diospyros spp. The five least common taxa included in the model (1% of all stems) were Duboscia macrocarpa, Ongokea gore, Zanthoxyllum heitzii, Klainedoxa sp., and Erythrophleum ivorense. Each species was assigned, if possible, into an “early-successional” or “late-successional” class based on growth form, available trait data, and habitat class (Whitmore, 1989; Raich and Khoon, 1990; Finegan, 1996; Davies and Semui, 2006; Chazdon et al., 2010; Chazdon, 2014).
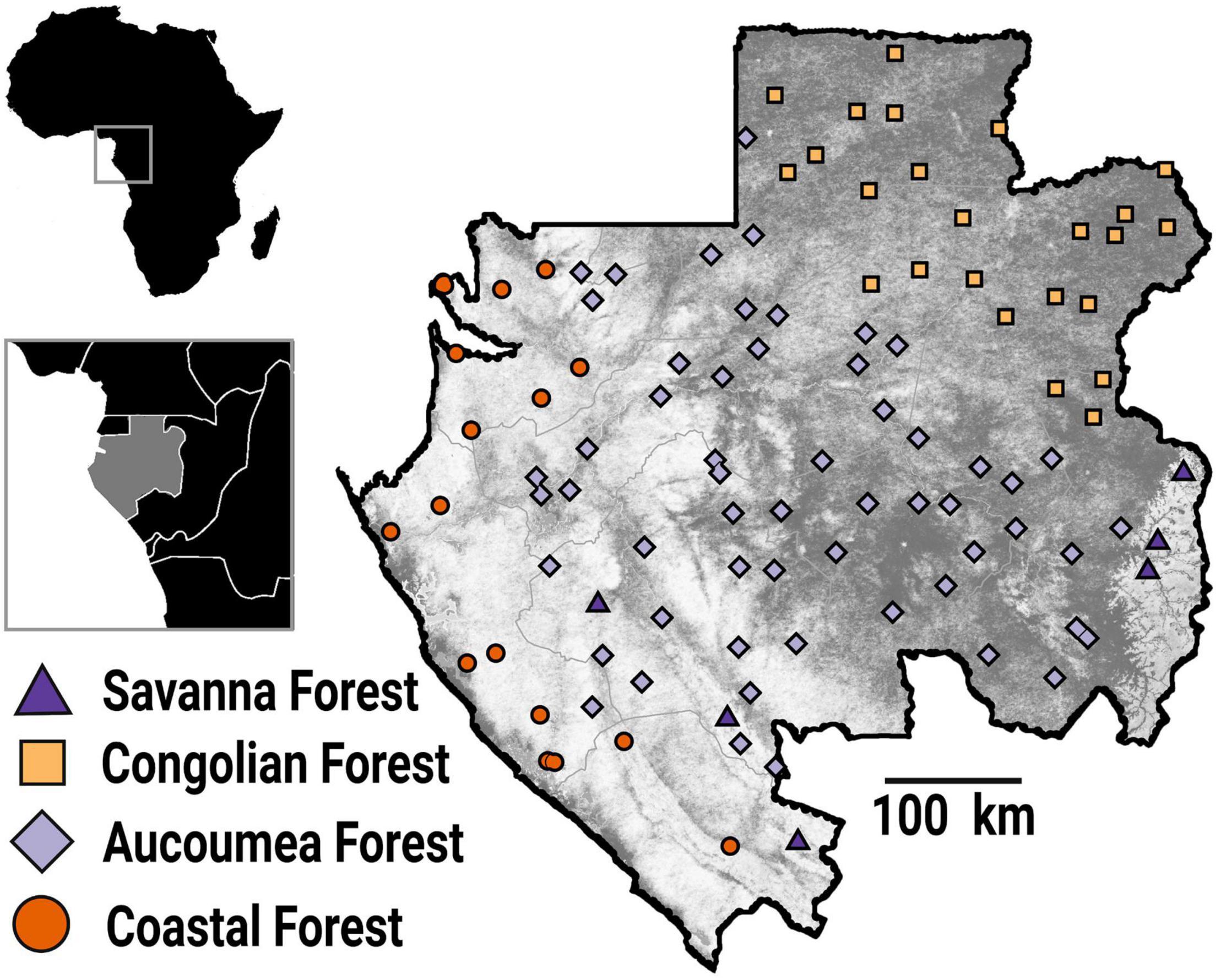
Figure 1. Map of all 104, 1-ha inventory plots located in Gabon. Plots were located by a systematic-random design to capture the full breadth of Gabon’s forest types and environmental conditions. Shapes indicate one of four possible forest-types of each plot: Savanna Forest (triangles), Congolian Forest (squares), Aucoumea Forest (diamonds), or Coastal Forest (circles).
Climate Data
Long term average historical precipitation and temperature data for each plot were derived from the NASA TerraClimate product (Abatzoglou et al., 2018) for 1985–2017, accessed using Google Earth Engine (Gorelick et al., 2017). We derived projected precipitation and temperature data for each plot using the NASA Earth Exchange Global Daily Downscaled Projections (NEX-GDDP) database at a resolution of 0.25 degrees (∼25 km × 25 km). This dataset provides downscaled projections for two of the most used Representative Concentration Pathways (RCP 4.5 and RCP 8.5) from the 21 General Circulation Models that were produced and distributed under the Coupled Model Intercomparison Project Phase 5 (CMIP5). All models of temperature agree that Gabon will continue to warm through the end of the century; thus, within each RCP scenario, we took the ensemble mean prediction of all 21 CMIP5 GCMs to forecast of forest response (Figures 2A,B). Models of precipitation disagree as to whether precipitation will increase or decrease, producing an ensemble mean that shows no substantive change by 2099 (Figure 2C). This is due in large part to a lack of rain gauges in Central Africa (Washington et al., 2013), and also from the northward shift of the inter-tropical convergence zone resulting from ocean-driven atmospheric circulation shifts (James et al., 2013). To acknowledge the uncertainty in whether the dry or wet models are more plausible, here we forecast community change given both a wet and a dry scenario. The wet scenario takes the mean prediction of the five models predicting the greatest increase in precipitation, while the dry scenario uses the mean prediction from the five models predicting the greatest decreases in precipitation (Supplementary Table 3). Although representing the extreme scenarios, this forecasted climate space is well represented by historical climate space (Supplementary Figure 1).
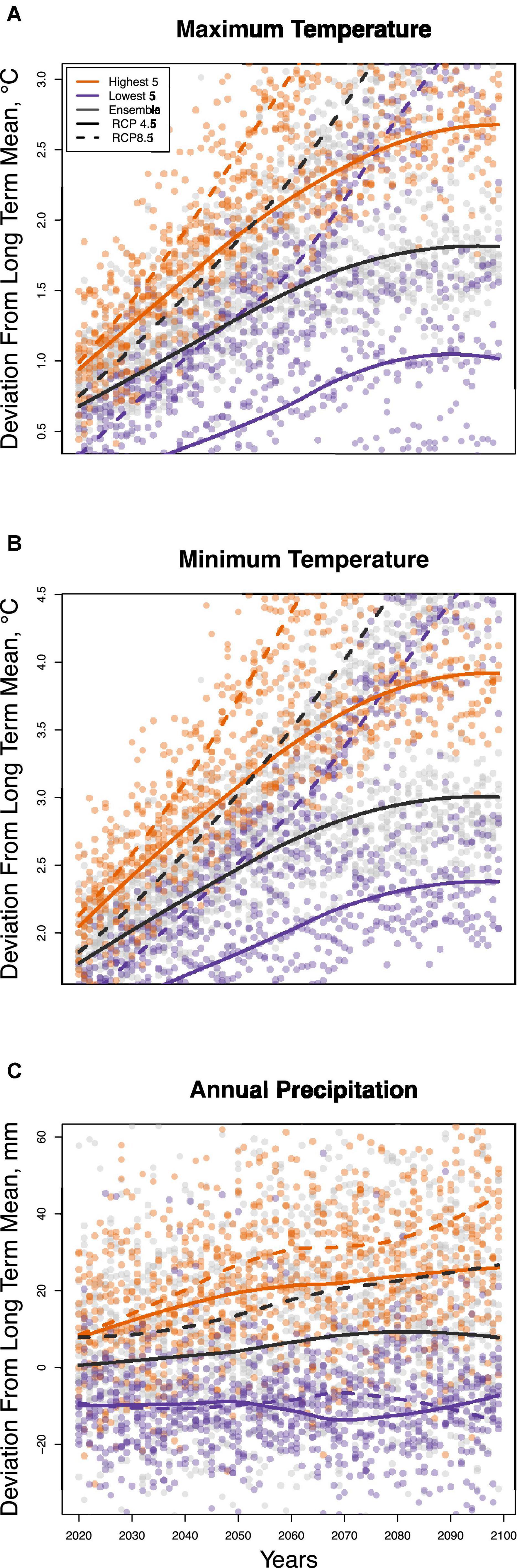
Figure 2. A comparison of average predicted maximum temperature (A), minimum temperature (B), and precipitation (C) in Gabon from the highest temperature increase models (orange), lowest five models (purple), and all model ensembles (gray) for RCP 4.5 (solid lines) and RCP 8.5 (dashed lines).
Community Composition Analysis
We use a generative Generalized Joint Attribute Model (GJAM) that predicts species abundance at the scale and context used to fit the model jointly, i.e., on the community scale (Clark et al., 2017). GJAM estimates can therefore be interpreted on the scale of the observations, accounting for sample effort. Full model specifications are available from Clark et al. (2017). Parameters in the model include matrices of coefficients B relating X to Y and the residual species covariance matrix Σ. In effect, Σ represents the covariance between species beyond what is explained by environmental covariates. This variation can come from interactions between species, unmeasured environmental variables, and other sources of error. The likelihood is: [Y1, …, YS|X, B, Σ], where subscripts refer to species 1 through S. Model fitting is done on the observation scale, and is based on the posterior distribution, [B, Σ|X, Y]∝[Y1, …, YS|B, Σ][B, Σ]. The right-hand side of the equation is the likelihood and the prior distribution, [B, Σ], which is non-informative. Traditional species distributions models cannot generate the covariance structure in Y or the proper uncertainty for B because they are not fitted jointly, i.e., because [Y1, …, YS|B, Σ, X] is not equal to likelihood of independent SDMs, [Y1|B, Σ, X] × … × [YS|B, Σ, X]. SDMs predict each species independently, which ignores their interactions. Joint modeling is frustrated by the different scales of measurement for many species and dominance of zeros in most data sets. GJAM allows for continuous and discrete data, including zeros, observed on different scales and with different levels of sample effort.
Forecasting Species Composition
The predictive distributions combine the posterior parameter estimates calibrated from long-term climate data with a prediction grid of forecasted covariate values (precipitation and temperature). X* is a vector of environmental covariates that generate a response vector of species Y*= Y1, …, YS, and (X*, Y*) is a pair of vector observations used to fit the model. The predictive distribution [Y*|X*] = ∫[Y*|B, Σ, X*][B, Σ|X, Y] d (B, Σ) is obtained by Monte Carlo integration. The two factors in the integrand are the likelihood and posterior distribution. The two factors in the integrand are the likelihood and posterior distribution. If the prediction is based on an uncertain scenario for climate X*, that uncertainty enters the prediction as [Y*] = ∫[Y*|X*][X*]dX*. In this analysis, [X*] is a prior distribution that is uniform over the full range of values that have been observed.
To quantify how changes in climate will affect total species counts regardless of forest type, we first run the model using forest type (Congolian, savanna, coastal, Acoumea) as a random effect. We then use forest type as a factor to make predictions about species counts within forest types. Covariates used to fit the model were limited to those for which there are predictions available from the NASA NEX GDDP GCM’s for years 2020–2099 and having variance inflation factors less than 3. Model estimates were taken from 100,000 iterations, discarding the first 1000 iteration as pre-convergence. We visually inspected trace plots to confirm convergence and adequate mixing (Supplementary Figure 3) and validated model fit by comparing predicted and observed discrete species abundances (Supplementary Figure 2).
Results
Of the 76 most abundant species analyzed, species richness in Gabon’s forests is projected to decrease by 3–8% by the end of the century, although there is variation among precipitation models and RCP scenarios (Figures 3, 4 and Supplementary Table 1). The dry model predicts a loss of 2.58 focal species per plot ± SE 5.36 in the low emissions scenario (RCP 4.5, Figure 4A), and −5.85 ± 5.12 species in the high emissions scenario (RCP 8.5, Figure 4C). The wet model predicts fewer species losses (RCP 4.5: −3.17 ± 5.37; RCP 8.5: −5.96 ± 5.11, Figures 3A,C). Species losses were consistent across forest types (Figures 3B,D, 4B,D and Supplementary Table 2) with greatest losses in the RCP 8.5 scenario (Figures 3D, 4D) compared to RCP 4.5 (Figures 3B, 4B). Of the 76 species we analyzed approximately one third are predicted to increase in abundance and two-thirds of species likely to decrease in abundance. The most likely species to increase in abundance include Diospyros spp., Aucoumea klaineana, and Staudia gabonensis. By contrast, Santira trimera, Plagiostyles africana, and Dichostemma glaucescens are predicted to decrease in abundance (Figure 5). Net change in predicted species abundance was similar between wet and dry models and among RCP scenarios (Figure 5 and Supplementary Table 4).
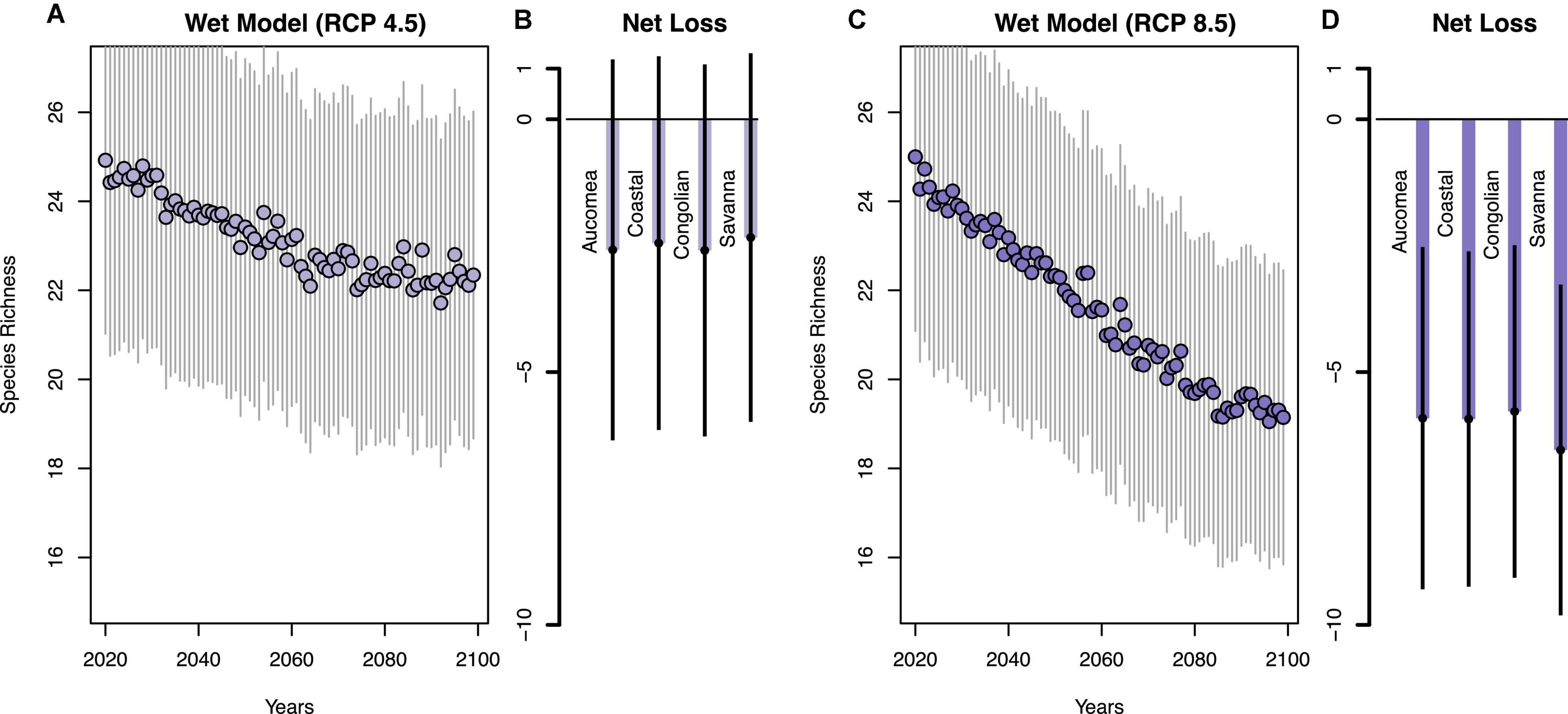
Figure 3. Declines in estimated plot-level species richness and the estimated net tree species loss for each forest type by the end of the century for RCP 4.5 (A,B) and RCP 8.5 (C,D) for the wet model.
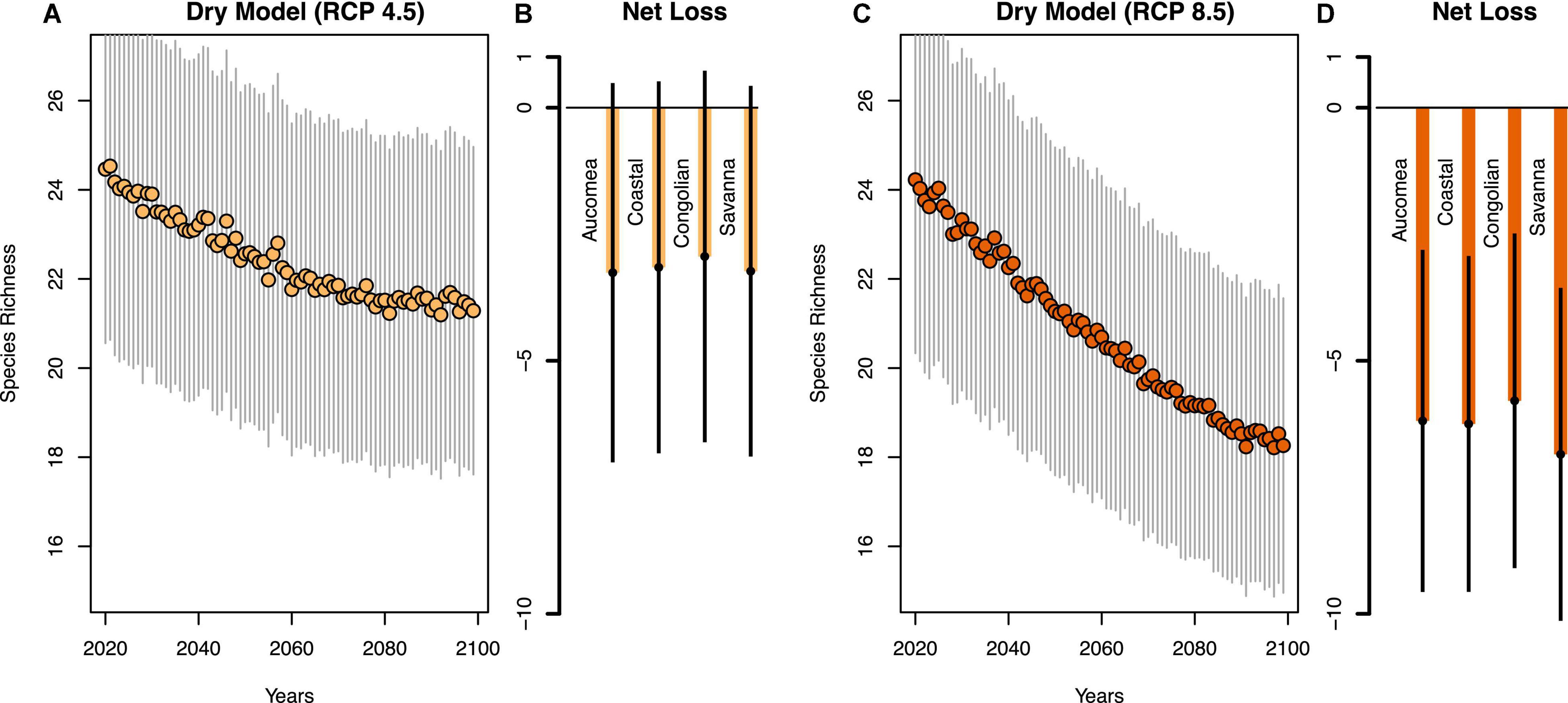
Figure 4. Declines in estimated plot-level species richness and the estimated net tree species loss for each for each forest type by the end of the century for RCP 4.5 (A,B) and RCP 8.5 (C,D) for the Dry model.
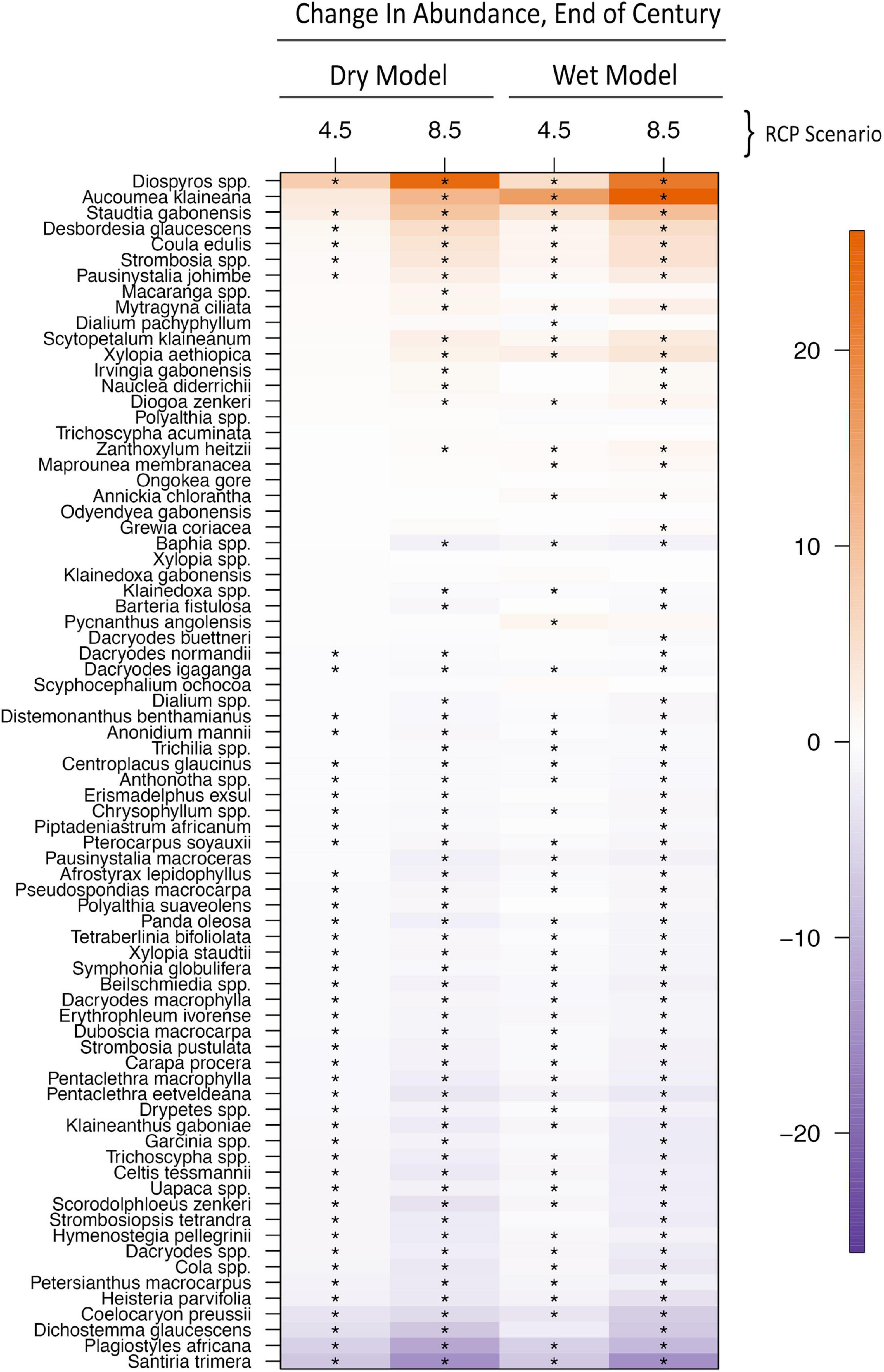
Figure 5. Predicted change in abundance for each tree species (y-axis) by the end of the century for RCP 4.5 and RCP 8.5 in both the “wet” and “dry” model ensembles (x-axis). Box colors correspond to estimated changes in species abundance. “*” symbol in box denotes species-model predictions that do not overlap with 0.
Discussion
We demonstrate a 3–8% decrease in Afrotropical forest species richness by the end of the century for the most abundant tree species. This prediction is substantially less severe than 30–50% predicted for the Neotropics (Colwell et al., 2008; Feeley and Silman, 2010), and lends support to the argument that the unique evolutionary past of Afrotropical forest communities (Malhi and Wright, 2004; Maslin et al., 2005; Parmentier et al., 2007; Malhi et al., 2013; Mayaux et al., 2013; Enquist et al., 2017; Sullivan et al., 2017) could have made them more resilient to climate change than their Amazonian or Asian counterparts (Hansen and DeFries, 2004; Gardner et al., 2007). It also indicates that predictions of tree species responses to climate change are not generalizable across continents. Surprisingly, we find that losses do not differ appreciably among forest types, indicating that no habitats will be immune from species changes. The equivalent responses may also be a result of the subjective distinction between our four forest types—communities are complex assemblages of species that may not always readily lend themselves to clear classification.
We find that species-specific changes in abundance varied considerably, with approximately a third of all species likely increasing in abundance and two-thirds of species likely decreasing in abundance. Our hypothesis that disturbance-related species would increase at the expense of climax species was not well supported. Of the ten species most likely to increase in abundance, only two are considered early successional. Of the ten species most likely to decrease in abundance, five were early successional. It is interesting that the species increasing are not just those that are fast growing, low wood density species associated with disturbance. For example, two of the species most likely to increase are Acoumea klaineana (a light-loving, low wood density species), and Diospyros spp., the genus that incudes ebony (high wood density, slow growing). Among the species expected to decrease in abundance is Santira trimera, one of the most widespread species throughout West and Central African rainforests, often in moist secondary forests or along rivers. Also predicted to decrease is Dichostemma glaucescens, a small slender tree prone to climbing other trees. These species-specific responses suggest that rather than functional groups responding similarly, tropical species respond individualistically to tropical climate change. This pattern agrees with past research (Bush, 2002; Bush et al., 2004) and may arise from unique relationships to unmeasured abiotic variables that contribute to its response to disturbance (Nuñez et al., 2019a). A comparison of the forecasts produced by both dry and wet models yields surprising little difference in predicted species richness. Although the total water available to trees are the product of both precipitation and temperature, these results suggest that species will respond more strongly to increases in temperature, not precipitation as it is in the Neotropics. For this reason, the increasing radiative forcing associated with RCP 8.5 resulted in greater species loss than the more conservative RCP 4.5 scenario. Temperature specific responses are consistent with the theory that a unique ecological history in the Afrotropics (Malhi and Wright, 2004; Maslin et al., 2005; Parmentier et al., 2007; Malhi et al., 2013; Mayaux et al., 2013; Enquist et al., 2017; Sullivan et al., 2017) cultivated tree communities with few wet-affiliated species (Leal, 2009).
Several considerations are necessary to situate this study in the literature. First and foremost, this analysis considered only the 76 most common species across plots. Rare species (occurring on fewer than 30/104 plots) have few observations and provide insufficient information on their relationship with environmental predictors to make accurate predictions, perpetuating the “rare species modeling paradox” (Lomba et al., 2010). This could mean that we are ultimately underestimating losses of total species richness because it is precisely these rare species that are most vulnerable to climate change (Ohlemüller et al., 2008; Pacifici et al., 2015). However, limiting analyses to species with adequate data is a common component of many analyses, including those predicting 30–50% loss in the Neotropics (Wisz et al., 2008; Feeley and Silman, 2010).
The model predicts at the scale of the data, i.e., the community level, allowing a comparison of a species likelihood of presence in a plot with full uncertainty. However, the model does not explicitly consider mechanistic changes in recruitment, carbon enrichment, seedling survival, or changes in dispersal. As such, the model does not account for plasticity that may allow for species to occur in plots outside their current climate space. Although such mechanistic understanding is needed, the type and resolution of data currently available make this impossible. These results do not consider other types of disturbance that are likely to affect biodiversity, but are less clearly measurable, like the loss of medium and large animals from hunting (Koerner et al., 2017; Poulsen et al., 2017b,2018, 2021; Beirne et al., 2019; Nuñez et al., 2019c). Finally, although the geographic scope of this analysis is uncommonly expansive, covering the entire country of Gabon (267,667 km2), it may not be directly comparable to the diverse terrain contained in the 2,250 km2 assessed in the Neotropics (Feeley and Silman, 2010; Maicher et al., 2021). Care must also be taken when generalizing our results—although the impacts predicted here appear to be modest relative to the new world Tropics, elsewhere in sub-Saharan Africa has already seen devastating effects of climate change (Willis and Bhagwat, 2009; Willis et al., 2013; Serdeczny et al., 2017).
This study demonstrates that community forecasts are not generalizable across regions, and more studies are needed in understudied biomes like the Afrotropics. Nascent data sets (Enquist et al., 2017; Fyllas et al., 2017), increased availability of high quality remote sensing (Patterson and Healey, 2015; Stavros et al., 2017; Silva et al., 2018), and new statistical techniques capable of synthesizing multiple types of data (Clark et al., 2017) will help in further resolving the responses of the world’s ecosystems. This study serves as an important counterpoint to work done in the Neotropics by providing contrasting predictions for Afrotropical forests with substantially different ecological, evolutionary, and anthropogenic histories. Even though we are predicting a comparatively small reduction in species richness, the effects reported here will have ramifications for whole food webs (Dirzo et al., 2014; Clark et al., 2019), and potentially threaten the ecosystem services on which humans depend (McCann, 2000; Hooper et al., 2005; Balvanera et al., 2006; Cardinale et al., 2012; Schweiger et al., 2018). The differences exposed by this work should serve as motivation for future research using fine scale data to compare the differing responses of tropical biomes to global change.
Data Availability Statement
The data analyzed in this study is subject to the following licenses/restrictions: The data are subject to third party restrictions. The data that support the findings of this study are available from Le Ministère des Eaux, de la Forest, de la Mer, de l’Environnement. Restrictions apply to the availability of these data, which were used under license for this study. Data are available from the corresponding author with the permission of Le Ministère des Eaux, de la Forest, de la Mer, de l’Environnement. Requests to access these datasets should be directed to JP, am9obi5wb3Vsc2VuQGR1a2UuZWR1.
Author Contributions
CN conducted the analysis and wrote the manuscript. CN, JP, VM, and LW designed the experiment and edited the manuscript. All authors contributed to the article and approved the submitted version.
Funding
CN was supported by National Science Foundation Fellowship (GRF-1106401) and a Neil Williams Presidential Fellowship.
Conflict of Interest
The authors declare that the research was conducted in the absence of any commercial or financial relationships that could be construed as a potential conflict of interest.
Publisher’s Note
All claims expressed in this article are solely those of the authors and do not necessarily represent those of their affiliated organizations, or those of the publisher, the editors and the reviewers. Any product that may be evaluated in this article, or claim that may be made by its manufacturer, is not guaranteed or endorsed by the publisher.
Acknowledgments
We thank the Gabon National Climate Change Council who authorized the establishment of the National Resource Inventory, as well as the Ministry of Forests for their support in the training of project technicians. We also thank the Gabon Parks Agency (ANPN) for implementing the NRI, and OLAM-Gabon, US Government SilvaCarbon Program, FAO and the Gabonese Government for financially supporting this work. We thank Dr. Alie Ashbury, Kim and Dave Littman, and referees for their constructive comments that improved the manuscript. An earlier version of this work was made available as pre-print (Nuñez et al., 2019b).
Supplementary Material
The Supplementary Material for this article can be found online at: https://www.frontiersin.org/articles/10.3389/fevo.2021.742626/full#supplementary-material
References
Abatzoglou, J. T., Dobrowski, S. Z., Parks, S. A., and Hegewisch, K. C. (2018). TerraClimate, a high-resolution global dataset of monthly climate and climatic water balance from 1958-2015. Sci. Data 5:170191. doi: 10.1038/sdata.2017.191
Asefi-Najafabady, S., and Saatchi, S. (2013). Response of African humid tropical forests to recent rainfall anomalies. Philos. Trans. R. Soc. B Biol. Sci. 368:20120306. doi: 10.1098/rstb.2012.0306
Aubry-Kientz, M., Rossi, V., Cornu, G., Wagner, F., and Hérault, B. (2019). Temperature rising would slow down tropical forest dynamic in the Guiana Shield. Sci. Rep. 9:10235. doi: 10.1038/s41598-019-46597-8
Balvanera, P., Pfisterer, A. B., Buchmann, N., He, J. S., Nakashizuka, T., Raffaelli, D., et al. (2006). Quantifying the evidence for biodiversity effects on ecosystem functioning and services. Ecol. Lett. 9, 1146–1156. doi: 10.1111/j.1461-0248.2006.00963.x
Beirne, C., Miao, Z., Nuñez, C. L., Medjibe, V. P., Saatchi, S., White, L. J. T., et al. (2019). Landscape-level validation of allometric relationships for carbon stock estimation reveals bias driven by soil type. Ecol. Appl. 29:e01987. doi: 10.1002/eap.1987
Blundo, C., Carilla, J., Grau, R., Malizia, A., Malizia, L., Osinaga-Acosta, O., et al. (2021). Taking the pulse of Earth’s tropical forests using networks of highly distributed plots. Biol. Conserv. 260:108849. doi: 10.1016/j.biocon.2020.108849
Bongers, F., Poorter, L., Hawthorne, W. D., and Sheil, D. (2009). The intermediate disturbance hypothesis applies to tropical forests, but disturbance contributes little to tree diversity. Ecol. Lett. 12, 798–805. doi: 10.1111/j.1461-0248.2009.01329.x
Bonnefille, R. (2011). “Rainforest responses to past climatic changes in tropical Africa,” in Tropical Rainforest Responses to Climatic Change, eds M. B. Bush, J. R. Flenley, and W. Gosling (Berlin: Springer), 125–184. doi: 10.1007/978-3-642-05383-2_5
Bush, M. B. (2002). Distributional change and conservation on the Andean flank: a palaeoecological perspective. Glob. Ecol. Biogeogr. 11, 463–473. doi: 10.1046/j.1466-822X.2002.00305.x
Bush, M. B., Silman, M. R., and Urrego, D. H. (2004). 48,000 years of climate and forest change in a biodiversity hot spot. Science 303, 827–829. doi: 10.1126/science.1090795
Cardinale, B. J., Duffy, J. E., Gonzalez, A., Hooper, D. U., Perrings, C., Venail, P., et al. (2012). Biodiversity loss and its impact on humanity. Nature 486, 59–67. doi: 10.1038/nature11148
Carley, L. N., Mojica, J. P., Wang, B., Chen, C. Y., Lin, Y. P., Prasad, K. V. S. K., et al. (2021). Ecological factors influence balancing selection on leaf chemical profiles of a wildflower. Nat. Ecol. Evol. 5, 1135–1144. doi: 10.1038/s41559-021-01486-0
Carlson, B. S., Koerner, S. E., Medjibe, V. P., White, L. J. T., and Poulsen, J. R. (2017). Deadwood stocks increase with selective logging and large tree frequency in Gabon. Glob. Chang. Biol. 23, 1648–1660. doi: 10.1111/gcb.13453
Chazdon, R. L. (2014). Second Growth: The Promise of Tropical Forest Regeneration in an Age of Deforestation. Chicago, IL: University of Chicago Press. doi: 10.7208/chicago/9780226118109.001.0001
Chazdon, R. L., Finegan, B., Capers, R. S., Salgado-Negret, B., Casanoves, F., Boukili, V., et al. (2010). Composition and dynamics of functional groups of trees during tropical forest succession in northeastern Costa Rica. Biotropica 42, 31–40. doi: 10.1111/j.1744-7429.2009.00566.x
Clark, J. S., Andrus, R., Aubry-Kientz, M., Bergeron, Y., Bogdziewicz, M., Bragg, D. C., et al. (2021). Continent-wide tree fecundity driven by indirect climate effects. Nat. Commun. 12:1242. doi: 10.1038/s41467-020-20836-3
Clark, J. S., Nemergut, D., Seyednasrollah, B., Turner, P. J., and Zhang, S. (2017). Generalized joint attribute modeling for biodiversity analysis: median-zero, multivariate, multifarious data. Ecol. Monogr. 87, 34–56. doi: 10.1002/ecm.1241
Clark, J. S., Nuñez, C. L., and Tomasek, B. (2019). Foodwebs based on unreliable foundations: spatiotemporal masting merged with consumer movement, storage, and diet. Ecol. Monogr. 89:e01381. doi: 10.1002/ecm.1381
Cohen, A. S., Stone, J. R., Beuning, K. R. M., Park, L. E., Reinthal, P. N., Dettman, D., et al. (2007). Ecological consequences of early Late Pleistocene megadroughts in tropical Africa. Proc. Natl. Acad. Sci. U.S.A. 104, 16422–16427. doi: 10.1073/pnas.0703873104
Colinvaux, P. A., De Oliveira, P. E., and Bush, M. B. (2000). Amazonian and neotropical plant communities on glacial time-scales: the failure of the aridity and refuge hypotheses. Quat. Sci. Rev. 19, 141–169.
Colinvaux, P. A., De Oliveira, P. E., Moreno, J. E., Miller, M. C., and Bush, M. B. (1996). A long pollen record from lowland Amazonia: forest and cooling in glacial times. Science 274, 85–88.
Colinvaux, P. A., Irion, G., Räsänen, M. E., Bush, M. B., and Nunes de Mello, J. A. S. (2001). A paradigm to be discarded: geological and paleoecological data falsify the Haffer & Prance refuge hypothesis of Amazonian speciation. Amaz. Limnol. Oecologia Reg. Syst. Fluminis Amaz. 16, 609–646.
Colwell, R. K., Brehm, G., Cardelús, C. L., Gilman, A. C., and Longino, J. T. (2008). Global warming, elevational range shifts, and lowland biotic attrition in the wet tropics. Science 322, 258–261. doi: 10.1126/science.1162547
Comes, H. P., and Kadereit, J. W. (1998). The effect of Quaternary climatic changes on plant distribution and evolution. Trends Plant Sci. 3, 432–438. doi: 10.1016/S1360-1385(98)01327-2
Corlett, R. T., and Primack, R. B. (2011). “Many tropical rain forests,” in Tropical Rain Forests (Chichester: John Wiley & Sons), 1–31. doi: 10.1002/9781444392296.ch1
Dainou, K., Bizoux, J., Doucet, J., Mahy, G., Hardy, O. J., and Heuertz, M. (2010). Forest refugia revisited: nSSRs and cpDNA sequences support historical isolation in a wide-spread African tree with high colonization capacity, Milicia excelsa (Moraceae). Mol. Ecol. 19, 4462–4477.
Davies, S. J., and Semui, H. (2006). Competitive dominance in a secondary successional rain-forest community in Borneo. J. Trop. Ecol. 22, 53–64. doi: 10.1017/S0266467405002944
Dexter, K. G., Pennington, R. T., Oliveira-Filho, A. T., Bueno, M. L., Silva de Miranda, P. L., and Neves, D. M. (2018). Inserting tropical dry forests into the discussion on biome transitions in the tropics. Front. Ecol. Evol. 6:104. doi: 10.3389/fevo.2018.00104
Dirzo, R., Young, H. S., Galetti, M., Ceballos, G., Isaac, N. J. B., and Collen, B. (2014). Defaunation in the anthropocene. Science 345, 401–406. doi: 10.1126/science.1251817
Engelbrecht, B. M. J., Comita, L. S., Condit, R., Kursar, T. A., Tyree, M. T., Turner, B. L., et al. (2007). Drought sensitivity shapes species distribution patterns in tropical forests. Nature 447, 80–82. doi: 10.1038/nature05747
Enquist, B. J., Bentley, L. P., Shenkin, A., Maitner, B., Savage, V., Michaletz, S., et al. (2017). Assessing trait-based scaling theory in tropical forests spanning a broad temperature gradient. Glob. Ecol. Biogeogr. 26, 1357–1373. doi: 10.1111/geb.12645
Fayolle, A., Engelbrecht, B., Freycon, V., Mortier, F., Swaine, M., Réjou-Méchain, M., et al. (2012). Geological substrates shape tree species and trait distributions in African moist forests. PLoS One 7:e0042381. doi: 10.1371/journal.pone.0042381
Feeley, K. J., and Silman, M. R. (2010). Biotic attrition from tropical forests correcting for truncated temperature niches. Glob. Chang. Biol. 16, 1830–1836. doi: 10.1111/j.1365-2486.2009.02085.x
Feeley, K. J., Silman, M. R., Bush, M. B., Farfan, W., Cabrera, K. G., Malhi, Y., et al. (2011). Upslope migration of Andean trees. J. Biogeogr. 38, 783–791. doi: 10.1111/j.1365-2699.2010.02444.x
Finegan, B. (1996). Pattern and process in neotropical secondary rain forests: the first 100 years of succession. Trends Ecol. Evol. 11, 119–124. doi: 10.1016/0169-5347(96)81090-1
Fyllas, N. M., Bentley, L. P., Shenkin, A., Asner, G. P., Atkin, O. K., Díaz, S., et al. (2017). Solar radiation and functional traits explain the decline of forest primary productivity along a tropical elevation gradient. Ecol. Lett. 20, 730–740. doi: 10.1111/ele.12771
Gardner, T. A., Barlow, J., Parry, L. W., and Peres, C. A. (2007). Predicting the uncertain future of tropical forest species in a data vacuum. Biotropica 39, 25–30. doi: 10.1111/j.1744-7429.2006.00228.x
Gond, V., Fayolle, A., Pennec, A., Cornu, G., Mayaux, P., Camberlin, P., et al. (2013). Vegetation structure and greenness in Central Africa from Modis multi-temporal data. Philos. Trans. R. Soc. B Biol. Sci. 368:20120309. doi: 10.1098/rstb.2012.0309
Gorelick, N., Hancher, M., Dixon, M., Ilyushchenko, S., Thau, D., and Moore, R. (2017). Google earth engine: planetary-scale geospatial analysis for everyone. Remote Sens. Environ. 202, 18–27. doi: 10.1016/j.rse.2017.06.031
Haffer, J. (1969). Speciation in amazonian forest birds. Science 165, 131–137. doi: 10.1126/science.165.3889.131
Hansen, M. C., and DeFries, R. S. (2004). Detecting long-term global forest change using continuous fields of tree-cover maps from 8-km Advanced Very High Resolution Radiometer (AVHRR) data for the years 1982-99. Ecosystems 7, 695–716. doi: 10.1007/s10021-004-0243-3
Hooper, D. U., Adair, E. C., Cardinale, B. J., Byrnes, J. E. K., Hungate, B. A., Matulich, K. L., et al. (2012). A global synthesis reveals biodiversity loss as a major driver of ecosystem change. Nature 486, 105–108. doi: 10.1038/nature11118
Hooper, D. U., Chapin, F. S., Ewel, J. J., Hector, A., Inchausti, P., Lavorel, S., et al. (2005). Effects of biodiversity on ecosystem functioning: a consensus of current knowledge. Ecol. Monogr. 75, 3–35. doi: 10.1890/04-0922
James, R., Washington, R., and Rowell, D. P. (2013). Implications of global warming for the climate of African rainforests. Philos. Trans. R. Soc. B Biol. Sci. 368:20120298. doi: 10.1098/rstb.2012.0298
Koerner, S. E., Poulsen, J. R., Blanchard, E. J., Okouyi, J., and Clark, C. J. (2017). Vertebrate community composition and diversity declines along a defaunation gradient radiating from rural villages in Gabon. J. Appl. Ecol. 54, 805–814. doi: 10.1111/1365-2664.12798
Leal, M. E. (2009). The past protecting the future: locating climatically stable forests in West and Central Africa. Int. J. Clim. Chang. Strateg. Manag. 1, 92–99. doi: 10.1108/17568690910934426
Lomba, A., Pellissier, L., Randin, C., Vicente, J., Moreira, F., Honrado, J., et al. (2010). Overcoming the rare species modelling paradox: a novel hierarchical framework applied to an Iberian endemic plant. Biol. Conserv. 143, 2647–2657. doi: 10.1016/j.biocon.2010.07.007
Lowe, A. J., Harris, D., Dormontt, E., and Dawson, I. K. (2010). Testing putative African tropical forest refugia using chloroplast and nuclear DNA phylogeography. Trop. Plant Biol. 3, 50–58.
Maicher, V., Clark, C. J., Harris, D. J., Medjibe, V. P., and Poulsen, J. R. (2021). From town to national park: understanding the long-term effects of hunting and logging on tree communities in Central Africa. For. Ecol. Manage. 499:119571. doi: 10.1016/j.foreco.2021.119571
Maley, J. (1996). The African rain forest - Main characteristics of changes in vegetation and climate from the Upper Cretaceous to the Quaternary. Proc. R. Soc. Edinburgh Sect. B Biol. Sci. 104, 31–73. doi: 10.1017/S0269727000006114
Malhi, Y., Adu-Bredu, S., Asare, R. A., Lewis, S. L., and Mayaux, P. (2013). African rainforests: past, present and future. Philos. Trans. R. Soc. B Biol. Sci. 368:20120312. doi: 10.1098/rstb.2012.0312
Malhi, Y., Gardner, T. A., Goldsmith, G. R., Silman, M. R., and Zelazowski, P. (2014). Tropical forests in the anthropocene. Annu. Rev. Environ. Resour. 39, 125–159. doi: 10.1146/annurev-environ-030713-155141
Malhi, Y., and Wright, J. (2004). Spatial patterns and recent trends in the climate of tropical rainforest regions. Philos. Trans. R. Soc. B Biol. Sci. 359, 311–329. doi: 10.1098/rstb.2003.1433
Maslin, M., Malhi, Y., Phillips, O., and Cowling, S. (2005). New views on an old forest: assessing the longevity, resilience and future of the Amazon rainforest. Trans. Inst. Br. Geogr. 30, 477–499. doi: 10.1111/j.1475-5661.2005.00181.x
Mayaux, P., Pekel, J. F., Desclée, B., Donnay, F., Lupi, A., Achard, F., et al. (2013). State and evolution of the African rainforests between 1990 and 2010. Philos. Trans. R. Soc. B Biol. Sci. 368:20120300. doi: 10.1098/rstb.2012.0300
Nuñez, C. L., Clark, J. S., Clark, C. J., and Poulsen, J. R. (2019a). Low-intensity logging and hunting have long-term effects on seed dispersal but not fecundity in Afrotropical forests. AoB Plants 11:ply074. doi: 10.1093/aobpla/ply074
Nuñez, C. L., Clark, J. S., and Poulsen, J. R. (2019b). Afrotropical tree communities may have distinct responses to forecasted climate change. bioRxiv [Preprint] doi: 10.1101/823724
Nuñez, C. L., Froese, G., Meier, A. C., Beirne, C., Depenthal, J., Kim, S., et al. (2019c). Stronger together: comparing and integrating camera trap, visual, and dung survey data in tropical forest communities. Ecosphere 10:e02965. doi: 10.1002/ecs2.2965
Ohlemüller, R., Anderson, B. J., Araújo, M. B., Butchart, S. H. M., Kudrna, O., Ridgely, R. S., et al. (2008). The coincidence of climatic and species rarity: high risk to small-range species from climate change. Biol. Lett. 4, 568–572. doi: 10.1098/rsbl.2008.0097
Oslisly, R., White, L., Bentaleb, I., Favier, C., Fontugne, M., Gillet, J. F., et al. (2013). Climatic and cultural changes in the west Congo Basin forests over the past 5000 years. Philos. Trans. R. Soc. B Biol. Sci. 368:20120304. doi: 10.1098/rstb.2012.0304
Pacifici, M., Foden, W. B., Visconti, P., Watson, J. E. M., Butchart, S. H. M., Kovacs, K. M., et al. (2015). Assessing species vulnerability to climate change. Nat. Clim. Chang. 5, 215–225. doi: 10.1038/nclimate2448
Pan, Y., Birdsey, R. A., Fang, J., Houghton, R., Kauppi, P. E., Kurz, W. A., et al. (2011). A large and persistent carbon sink in the world’s forests. Science 333, 988–993. doi: 10.1126/science.1201609
Parmentier, I., Malhi, Y., Senterre, B., Whittaker, R. J., Balinga, M. P. B., Alonso, A., et al. (2007). The odd man out? Might climate explain the lower tree α-diversity of African rain forests relative to Amazonian rain forests? J. Ecol. 95, 1058–1071. doi: 10.1111/j.1365-2745.2007.01273.x
Patterson, P., and Healey, S. (2015). “Global ecosystem dynamics investigation (GEDI) LiDAR sampling strategy,” in Proceedings of the Pushing Boundaries: New Directions in Inventory Techniques and Applications: Forest Inventory and Analysis (FIA) Symposium 2015 (Fort Collins, CO), 245.
Petit, R. J., Aguinagalde, I., de Beaulieu, J.-L., Bittkau, C., Brewer, S., Cheddadi, R., et al. (2003). Glacial refugia: hotspots but not melting pots of genetic diversity. Science 300, 1563–1565.
Phillips, O. L., and Baker, T. R. (2002). Field Manual for Plot Establishment and Remeasurement (RAINFOR), 1st Edn. Swindon: Natural Environment Research Council.
Pimm, S. L., and Raven, P. (2000). Extinction by numbers. Nature 403, 843–845. doi: 10.1038/35002708
Poorter, L., van der Sande, M. T., Arets, E. J. M. M., Ascarrunz, N., Enquist, B., Finegan, B., et al. (2017). Biodiversity and climate determine the functioning of Neotropical forests. Glob. Ecol. Biogeogr. 26, 1423–1434. doi: 10.1111/geb.12668
Poulsen, J. R., Beirne, C., Rundel, C., Baldino, M., Kim, S., Knorr, J., et al. (2021). Long distance seed dispersal by forest elephants. Front. Ecol. Evol. 9:962.
Poulsen, J. R., Koerner, S. E., Miao, Z., Medjibe, V. P., Banak, L. N., and White, L. J. T. (2017a). Forest structure determines the abundance and distribution of large lianas in Gabon. Glob. Ecol. Biogeogr. 26, 472–485. doi: 10.1111/geb.12554
Poulsen, J. R., Koerner, S. E., Moore, S., Medjibe, V. P., Blake, S., Clark, C. J., et al. (2017b). Poaching empties critical Central African wilderness of forest elephants. Curr. Biol. 27, R134–R135. doi: 10.1016/j.cub.2017.01.023
Poulsen, J. R., Medjibe, V. P., White, L. J. T., Miao, Z., Banak-Ngok, L., Beirne, C., et al. (2020). Old growth Afrotropical forests critical for maintaining forest carbon. Glob. Ecol. Biogeogr. 29, 1785–1798. doi: 10.1111/geb.13150
Poulsen, J. R., Rosin, C., Meier, A., Mills, E., Nuñez, C. L., Koerner, S. E., et al. (2018). Ecological consequences of forest elephant declines for Afrotropical forests. Conserv. Biol. 32, 559–567. doi: 10.1111/cobi.13035
Qiu, T., Aravena, M.-C., Andrus, R., Ascoli, D., Bergeron, Y., Berretti, R., et al. (2021). Is there tree senescence? The fecundity evidence. Proc. Natl. Acad. Sci.U.S.A. 118:e2106130118. doi: 10.1073/pnas.2106130118
Raich, J. W., and Khoon, G. W. (1990). Effects of canopy openings on tree seed germination in a malaysian dipterocarp forest. J. Trop. Ecol. 6, 203–217. doi: 10.1017/S0266467400004326
Saatchi, S., Asefi-Najafabady, S., Malhi, Y., Aragão, L. E. O. C., Anderson, L. O., Myneni, R. B., et al. (2013). Persistent effects of a severe drought on Amazonian forest canopy. Proc. Natl. Acad. Sci. U.S.A. 110, 565–570. doi: 10.1073/pnas.1204651110
Saatchi, S. S., Harris, N. L., Brown, S., Lefsky, M., Mitchard, E. T. A., Salas, W., et al. (2011). Benchmark map of forest carbon stocks in tropical regions across three continents. Proc. Natl. Acad. Sci. U.S.A. 108, 9899–9904. doi: 10.1073/pnas.1019576108
Sala, O. E., Chapin, F. S., Armesto, J. J., Berlow, E., Bloomfield, J., Dirzo, R., et al. (2000). Global biodiversity scenarios for the year 2100. Science 287, 1770–1774. doi: 10.1126/science.287.5459.1770
Sannier, C., McRoberts, R. E., Fichet, L. V., and Makaga, E. M. K. (2014). Using the regression estimator with landsat data to estimate proportion forest cover and net proportion deforestation in gabon. Remote Sens. Environ. 151, 138–148. doi: 10.1016/j.rse.2013.09.015
Schimel, D., Pavlick, R., Fisher, J. B., Asner, G. P., Saatchi, S., Townsend, P., et al. (2015). Observing terrestrial ecosystems and the carbon cycle from space. Glob. Chang. Biol. 21, 1762–1776. doi: 10.1111/gcb.12822
Schweiger, A. K., Cavender-Bares, J., Townsend, P. A., Hobbie, S. E., Madritch, M. D., Wang, R., et al. (2018). Plant spectral diversity integrates functional and phylogenetic components of biodiversity and predicts ecosystem function. Nat. Ecol. Evol. 2, 976–982. doi: 10.1038/s41559-018-0551-1
Serdeczny, O., Adams, S., Baarsch, F., Coumou, D., Robinson, A., Hare, W., et al. (2017). Climate change impacts in Sub-Saharan Africa: from physical changes to their social repercussions. Reg. Environ. Change 17, 1585–1600. doi: 10.1007/s10113-015-0910-2
Silva, C. A., Saatchi, S., Garcia, M., Labriere, N., Klauberg, C., Ferraz, A., et al. (2018). Comparison of small-and large-footprint lidar characterization of tropical forest aboveground structure and biomass: a case study from central gabon. IEEE J. Sel. Top. Appl. Earth Obs. Remote Sens. 11, 3512–3526. doi: 10.1109/JSTARS.2018.2816962
Slik, J. W. F., Arroyo-Rodríguez, V., Aiba, S. I., Alvarez-Loayza, P., Alves, L. F., Ashton, P., et al. (2015). An estimate of the number of tropical tree species. Proc. Natl. Acad. Sci. U.S.A. 112, 7472–7477. doi: 10.1073/pnas.1423147112
Stavros, E. N., Schimel, D., Pavlick, R., Serbin, S., Swann, A., Duncanson, L., et al. (2017). ISS observations offer insights into plant function. Nat. Ecol. Evol. 1:194. doi: 10.1038/s41559-017-0194
Sullivan, M. J. P., Talbot, J., Lewis, S. L., Phillips, O. L., Qie, L., Begne, S. K., et al. (2017). Diversity and carbon storage across the tropical forest biome. Sci. Rep. 7:39102. doi: 10.1038/srep39102
van Vuuren, D. P., Sala, O. E., and Pereira, H. M. (2006). The future of vascular plant diversity under four global scenarios. Ecol. Soc. 11:25. doi: 10.5751/ES-01818-110225
Wade, A. M., Richter, D. D., Medjibe, V. P., Bacon, A. R., Heine, P. R., White, L. J. T., et al. (2019). Estimates and determinants of stocks of deep soil carbon in Gabon, Central Africa. Geoderma 341, 236–248. doi: 10.1016/j.geoderma.2019.01.004
Washington, R., James, R., Pearce, H., Pokam, W. M., and Moufouma-Okia, W. (2013). Congo basin rainfall climatology: can we believe the climate models? Philos. Trans. R. Soc. B Biol. Sci. 368:20120296. doi: 10.1098/rstb.2012.0296
Whitmore, T. C. (1989). Canopy gaps and the two major groups of forest trees. Ecology 70, 536–538. doi: 10.2307/1940195
Whitworth, A., Beirne, C., Flatt, E., Froese, G., Nuñez, C., and Forsyth, A. (2021). Recovery of dung beetle biodiversity and traits in a regenerating rainforest: a case study from Costa Rica’s Osa Peninsula. Insect Conserv. Divers. 14, 439–454. doi: 10.1111/icad.12470
Willis, K. J., and Bhagwat, S. A. (2009). Biodiversity and climate change. Science 326, 806–807. doi: 10.1126/science.1178838
Willis, K. J., Bennett, K. D., Burrough, S. L., Macias-Fauria, M., and Tovar, C. (2013). Determining the response of African biota to climate change: using the past to model the future. Philos. Trans. R. Soc. B Biol. Sci. 368:20120491. doi: 10.1098/rstb.2012.0491
Keywords: afrotropics, Gabon, joint attribute modeling, biodiversity, community
Citation: Núñez CL, Poulsen JR, White LJT, Medjibe V and Clark JS (2022) Distinct Community-Wide Responses to Forecasted Climate Change in Afrotropical Forests. Front. Ecol. Evol. 9:742626. doi: 10.3389/fevo.2021.742626
Received: 16 July 2021; Accepted: 29 December 2021;
Published: 18 January 2022.
Edited by:
Jon Fjeldså, University of Copenhagen, DenmarkReviewed by:
Mário Gabriel Santiago Santos, University of Trás-os-Montes and Alto Douro, PortugalLuca Vitale, Institute for Agricultural and Forestry Systems in the Mediterranean, National Research Council of Italy (CNR), Italy
Copyright © 2022 Núñez, Poulsen, White, Medjibe and Clark. This is an open-access article distributed under the terms of the Creative Commons Attribution License (CC BY). The use, distribution or reproduction in other forums is permitted, provided the original author(s) and the copyright owner(s) are credited and that the original publication in this journal is cited, in accordance with accepted academic practice. No use, distribution or reproduction is permitted which does not comply with these terms.
*Correspondence: Chase L. Núñez, Y251bmV6QGFiLm1wZy5kZQ==