- 1Department of Biology, Missouri State University, Springfield, MO, United States
- 2Department of Ecology and Evolutionary Biology, University of Arizona, Tucson, AZ, United States
Mutualisms involve cooperation, but also frequently involve conflict. Plant-pollinator mutualisms are no exception. To facilitate animal pollination, flowering plants often offer pollen (their male gametes) as a food reward. Since plants benefit by maximizing pollen export to conspecific flowers, we might expect plants to cheat on pollen rewards. In intersexual floral mimicry, rewarding pollen-bearing male flowers (models) are mimicked by rewardless female flowers (mimics) on the same plant. Pollinators should therefore learn to avoid the unrewarding mimics. Plants might impede such learning by producing phenotypically variable flowers that cause bees to generalize among models and mimics during learning. In this laboratory study, we used partially artificial flowers (artificial petals, live reproductive parts) modeled after Begonia odorata to test whether variation in the size of rewarding male flowers (models) and unrewarding female flowers (mimics) affected how quickly bees learned both to recognize models and to reject mimics. Live unrewarding female flowers have 33% longer petals and have 31% greater surface area than live rewarding male flowers, which bees should easily discriminate. Yet while bees rapidly learned to reduce foraging effort on mimics, learning was not significantly affected by the degree to which flower size varied. Additionally, we found scant evidence that this was a result of bees altering response speed to maintain decision accuracy. Our study failed to provide evidence that flower size variation in intersexual floral mimicry systems exploits pollinator cognition, though we cannot rule out that other floral traits that are variable may be important. Furthermore, we propose that contrary to expectation, phenotypic variability in a Batesian mimicry system may not necessarily have significant effects on whether receivers effectively learn to discriminate models and mimics.
Introduction
Conflicts of interest are common in plant-pollinator mutualisms (Bronstein, 2001; Thompson et al., 2013; van der Kooi et al., 2021). To facilitate pollination, flowering plants typically offer pollinators resources, such as pollen and nectar (“floral rewards”; Simpson and Neff, 1981). However, when floral rewards are costly to the plant to produce, maintain, and/or give up, the plant may benefit by exploiting the pollinator. For instance, the plant may benefit by withholding rewards or falsely advertising rewards, if it is still pollinated (Schiestl, 2005; Essenberg, 2021). Likewise, because foraging can be costly, the pollinator may benefit by exploiting the plant. For example, the pollinator might reduce time spent foraging by bypassing the floral sex organs to extract floral rewards (“robbing”) (Maloof and Inouye, 2000; Barker et al., 2018). Such reciprocal exploitation between plant and pollinator is common and frequently involves plants deceiving pollinators into pollinating flowers that lack rewards. Batesian mimicry, in which pollinators are deceived into visiting rewardless flowers that mimic rewarding flowers (models) is particularly widespread and is found in more than 32 plant families (Schiestl and Johnson, 2013; Johnson and Schiestl, 2016; de AvilaJr., Oleques et al., 2017). Successful Batesian mimicry is thought to rely on exploiting constraints on pollinator cognition, because pollinators otherwise will learn to avoid less profitable flowers (Smithson and Macnair, 1997; Whitehead and Peakall, 2012; Russell et al., 2020). Yet how constraints on pollinator learning are exploited in Batesian floral mimicry systems is still poorly understood (Dukas, 1987; Gigord et al., 2001; Schiestl and Johnson, 2013; Johnson and Schiestl, 2016; Goodrich and Jurgens, 2017; but see Kunze and Gumbert, 2000; de Jager and Ellis, 2014; Russell et al., 2020).
Naïve pollinators are expected to adjust their behavior with experience to avoid visiting rewardless mimics, because visiting them is costly to the pollinator (e.g., Ayasse et al., 2000; Schiestl, 2005). Thus, Batesian floral mimics might maximize their benefits by reducing how quickly and how well pollinators learn to discriminate mimics from models (Dukas, 1987; Abbott and Sherratt, 2013; de Jager et al., 2016). One way in which Batesian floral mimics might impede learning is by closely matching the phenotype of models (Sherratt, 2002; Kikuchi and Pfennig, 2013; de Jager et al., 2016). Yet accurate (“perfect”) mimicry may not always be achievable, as when there are developmental constraints on the precision of the mimicry (Kikuchi and Pfennig, 2013), or may not even be adaptive, as when imperfect mimicry exploits pollinator sensory biases (Schaefer and Ruxton, 2010; Russell et al., 2020). Given constraints on pollinator cognition, perfect mimicry may also be unnecessary for successful Batesian mimicry. For example, even if floral models and mimics vary in phenotype, pollinators might generalize models and mimics (Wright and Smith, 2003; Lynn et al., 2005). In non-pollinator systems, variation is in fact thought to promote generalization (Amézquita et al., 2013; Gamberale-Stille et al., 2018; Arias et al., 2020). This is thought to be a result of variation increasing the width of the signal distribution, which enhances the perceived similarity of model and mimic (Figure 1; Lynn et al., 2005). While generalization is thought to be a fundamental property of learning in animals (Kalish, 1969; Mackintosh, 1974; Enquist and Johnstone, 1997; Cheng, 2002), its role in mediating the success of Batesian mimicry has seldom been examined (but see Ham et al., 2006; Gamberale-Stille et al., 2018).
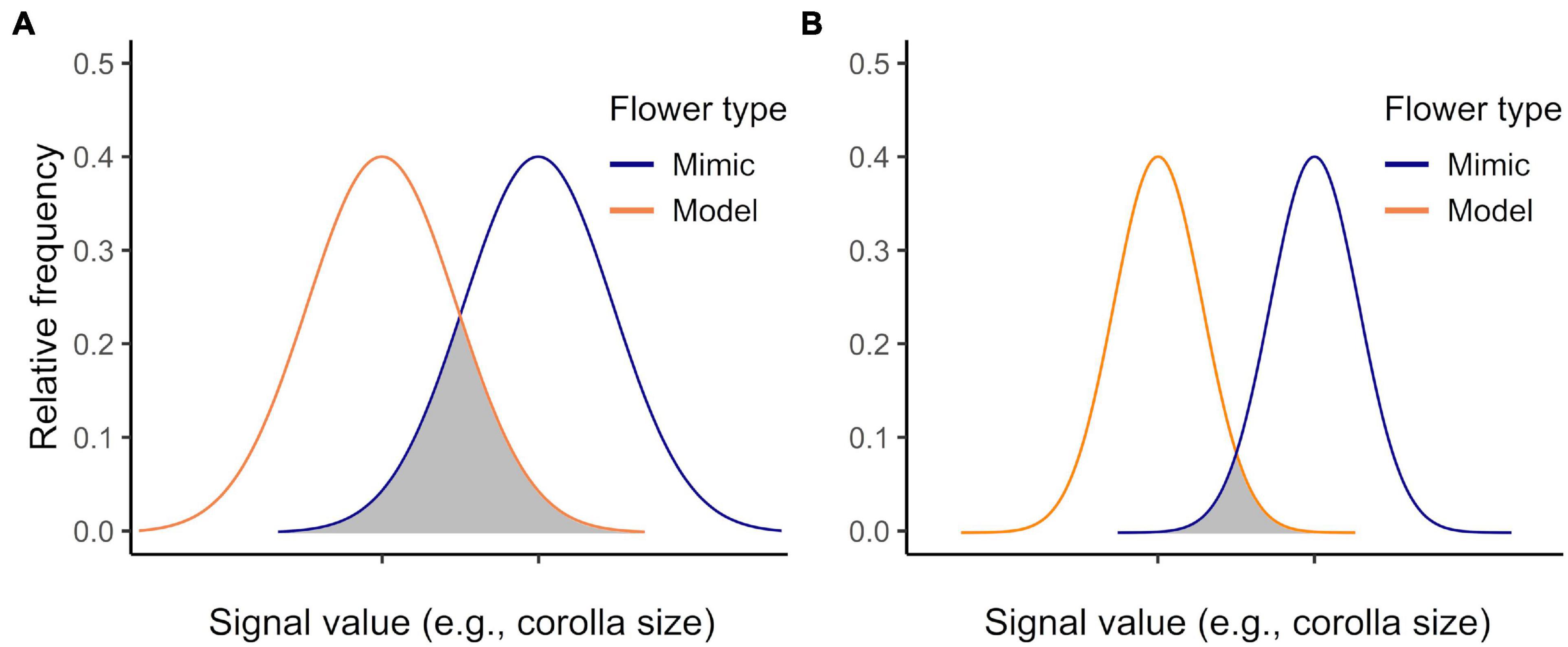
Figure 1. How signal parameters of models and mimics influence receiver behavioral responses. Signal parameters are modeled as Gaussian probability density functions. The more the signal distributions overlap, the greater the uncertainty of signal stimuli for the receiver (grey shading; compare greater overlap in “A” to “B”) (see also Lynn et al., 2005).
In flowering plant species that exhibit intersexual floral Batesian mimicry, a single plant species produces male flowers that typically offer a pollen reward to pollinators (primarily bees) and female flowers that are deceptive rewardless mimics (Johnson and Schiestl, 2016). Intraspecific phenotypic differences between male and female flowers are common, with differences in size being particularly obvious and well-documented (Ågren and Schemske, 1995; Schemske and Ågren, 1995; Castillo et al., 2012). Likewise, intrasexual flower size variation is also common (Ågren and Schemske, 1995; Schemske and Ågren, 1995; Galen, 1999; Castillo et al., 2012; Hattori et al., 2016). Given that pollinators, such as bees can learn flower size cues in other contexts and may generalize among different sized flowers of a given plant (Yoshioka et al., 2007; Essenberg et al., 2015; Dixit et al., 2020), intrasexual flower size variation in plant species with intersexual floral mimicry may function to promote pollinator generalization while learning.
How then might a pollinator respond to exploitation by a plant that uses variation in models and/or mimics as a strategy? One possibility is that the pollinator may compensate for a more challenging learning task via a speed-accuracy tradeoff (Chittka et al., 2003; Ings and Chittka, 2008; Kulahci et al., 2008; Chittka et al., 2009). In other words, when uncertainty is high, such as when model and mimic flower phenotypes are highly variable and overlapping in phenotype, the pollinator may take more time to decide whether to reject or visit a given flower vs. when uncertainty is low, such as when model and mimic flower phenotypes are relatively invariant and have relatively low phenotypic overlap.
In this laboratory study, we tested whether intrasexual flower size variation in a simultaneously monoecious plant species (Begonia odorata) exhibiting intersexual Batesian mimicry caused generalization for a generalist bumble bee (Bombus impatiens). Here, intersexual mimicry is observed in terms of overall flower color pattern and divided styles resembling anthers in form and color, i.e., pseudanthery (Johnson and Schiestl, 2016; de Jager and Anderson, 2019). We hypothesized that when intrasexual flower size did not vary, bees would learn more quickly to avoid female flowers than when intrasexual flower size varied. We assessed differences in learning by examining how the rate of correct decisions (approaching and landing on models and approaching but not landing on mimics), incorrect decisions (approaching but not landing on models, approaching and landing on mimics), correct detections (approaching and landing on models), and correct rejections (approaching but not landing on mimics, i.e., false alarms) changed with experience (following Russell et al., 2020). We also predicted that bees might avoid exploitation by the plant to some extent via a speed-accuracy tradeoff and thus take more time to make decisions when intrasexual flower size varied vs. when it did not. To manipulate flower size precisely we used artificial corollas that closely resembled live corollas, to which we attached live reproductive parts (Figure 2).
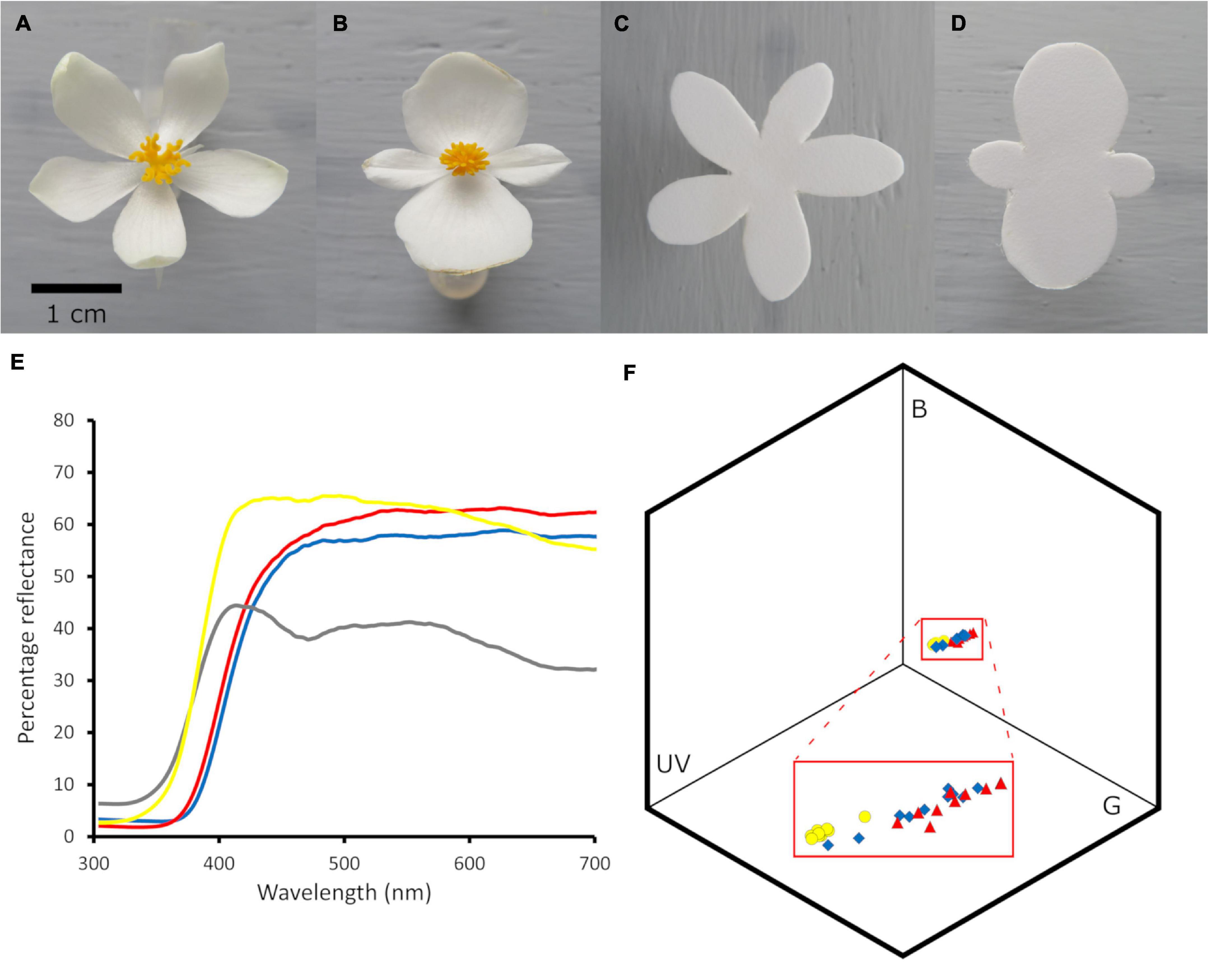
Figure 2. Imperfect mimicry among female (mimics) and male (models) in Begonia odorata flowers. (A) Female and (B) male flowers and artificial corollas of (C) female and (D) male medium-sized flowers. (E) The mean reflectance spectra of the female (red line) and male (blue line) flowers, the artificial corolla (yellow line), and the arena background (grey line) against which flowers were displayed; spectra smoothed using a 100 point moving average in Microsoft Excel. (F) The loci in Bombus impatiens color space of male petals (blue diamonds), female petals (red triangles), and artificial corollas (yellow circles) against the test arena background: artificial corollas resemble the color of live flower corollas (N = 10, 10, 10 male, female, and artificial flowers, respectively). On average, artificial petals and live petals differed from each other by 0.09 color units and from the background by 0.14 and 0.23 color units, respectively.
Materials and Methods
Test Subjects
We maintained three colonies (Koppert Biological Systems, Howell, MI, United States) of the common eastern bumble bee Bombus impatiens following Russell et al. (2020). In brief, we allowed colonies to forage freely on 2 M sucrose solution and pulverized honeybee-collected pollen (Koppert Biological Systems) from artificial feeders within enclosed foraging arenas (length, width, height: 82 × 60 × 60 cm) set to a 14 h: 10 h light: dark cycle.
We used fresh male and female flowers with mature anthers and styles, respectively, from 10 simultaneously monoecious Begonia odorata plants raised in a university greenhouse with supplemental halogen lights to extend day length to a 14: 10 h cycle and with fertilizer applications every other week (Plant Tone, NPK 5: 3: 3). While female B. odorata flowers are rewardless and produce neither pollen nor nectar, male B. odorata flowers offer pollen, their sole reward to their primary pollinators, bees; bumble bees are among the bee genera known to visit closely related Begonia species (Schemske et al., 1996; Pemberton and Wheeler, 2006; Wyatt and Sazima, 2011; de AvilaJr., Oleques et al., 2017).
Female B. odorata flowers closely resemble male flowers in bumble bee color vision (Figure 2); both flower sexes have creamy white dissected petals, and the female flower’s yellow and highly divided styles closely resemble the male flower’s numerous yellow stamens (see also Russell et al., 2020). Strikingly, the frontal surface area of female flowers is on average 30.7% greater than that of male flowers (a difference of 141.4 mm2) and female flowers have on average 33.3% longer petals than male flowers (a difference of 4.8 mm) (N = 84 and 86 female and male flowers measured, respectively; from 7 plants; ∼12 flowers/plant; Figure 3). In addition to significant intersexual differences in flower size, intrasexual flower size variation is substantial and flower sexes overlap in size (Figure 3). We used ImageJ (National Institutes of Health, Bethesda, MD)1 to measure flowers that had been photographed at a standard height with their petals gently flattened by glass slides.
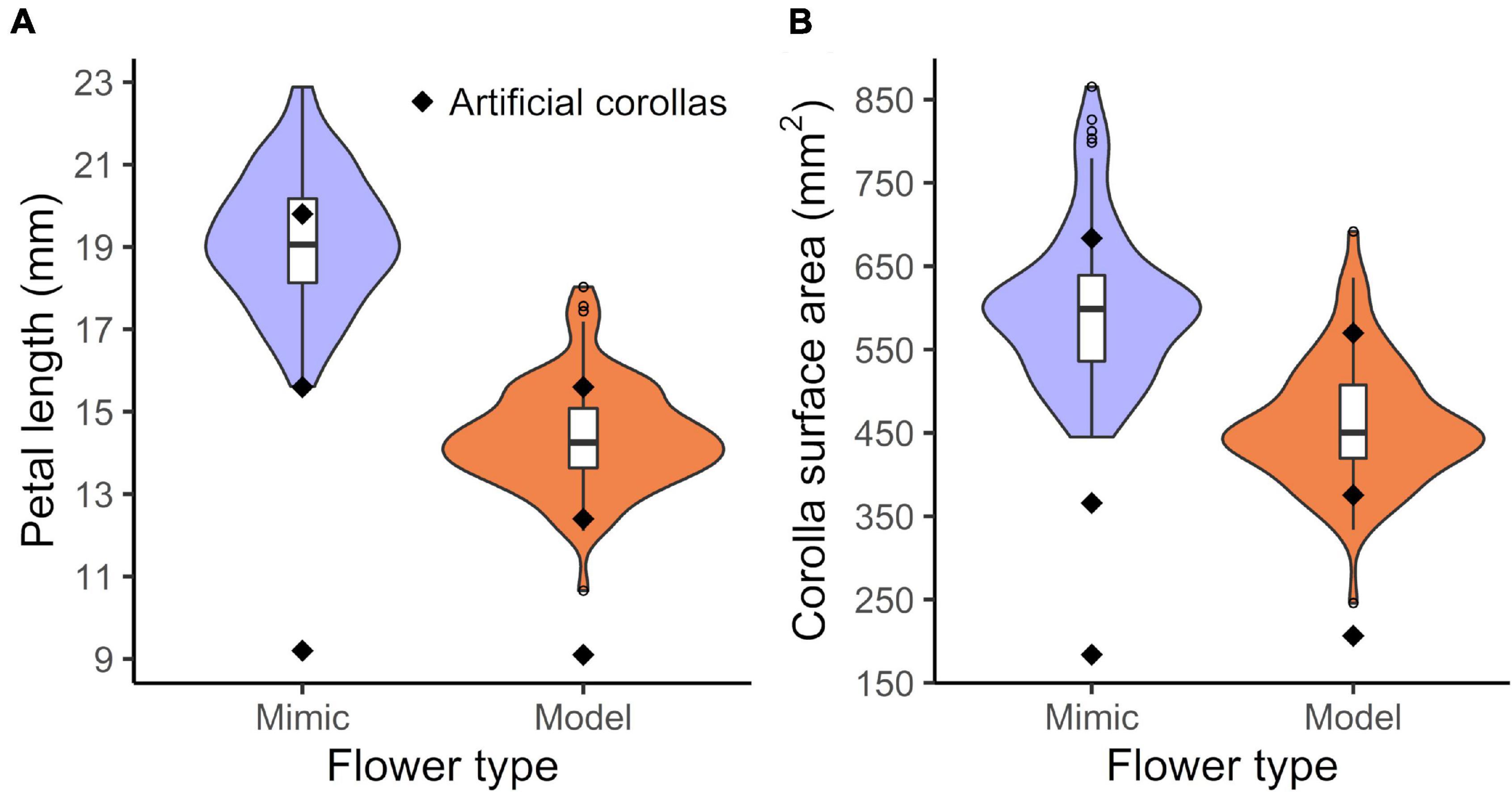
Figure 3. Variation in petal length and corolla surface area of Begonia odorata model (male) and mimic (female) flowers and corresponding measurements of artificial corollas. Boxplots of variation in mimic and model (A) mean petal length per flower and (B) corolla surface area. Artificial corollas (black diamonds) and distribution for the live flowers (violin plots) are also plotted. The artificial corollas were modeled on flowers growing prior to the COVID-19 lockdown—the lockdown delayed measuring a complete distribution of flowers and it appears the distribution of sizes subsequently shifted and narrowed. N = 84 and 86 mimic and model flowers, respectively.
Experiment
We tested whether the degree to which corolla size varied influenced how initially flower-naïve bees learned to sample among models (male flowers) and mimics (female flowers). We examined three primary components of sampling behavior (“visits”) made by bees visiting arrays of 18 flowers: approaches, landings without sonication (on male flowers such landings typically involved the bee collecting pollen via a behavior termed scrabbling; see Russell et al., 2017 for a description), and landings with sonication (“buzzes” or “buzzing”) (see flow diagram Supplementary Figure 1). An approach was defined as the bee in flight greatly reducing its velocity while facing the flower within 3 cm of the flower. All landings were preceded by an approach (i.e., “correct detections” for models; “false alarms” for mimics) and landings on male flowers (models) nearly exclusively involved collection of pollen. Not all approaches were followed by a landing (i.e., “missed detections” for models; “correct rejections” for mimics). Buzzes, which indicated an attempt at extracting pollen whether or not it was available, were identified by their distinctive sound and occurred only after a bee had landed (see Russell et al., 2016a). Buzzing a male flower constituted a correct behavioral response and buzzing a female flower constituted an incorrect behavioral response.
To precisely manipulate corolla size variation, we constructed artificial plastic corollas (from polypropylene Sterilite container lids) closely matched to the color, size, and shape of live B. odorata corollas (Figures 2, 4). The flowers used in behavioral trials were a combination of artificial plastic corollas and the live reproductive parts of freshly clipped male and female flowers, which were hot-glued into the center of the plastic corollas just prior to behavioral trials. Surrogate male flowers had artificial male corollas with male reproductive parts and surrogate female flowers had artificial female corollas with female reproductive parts (Figures 2, 4).
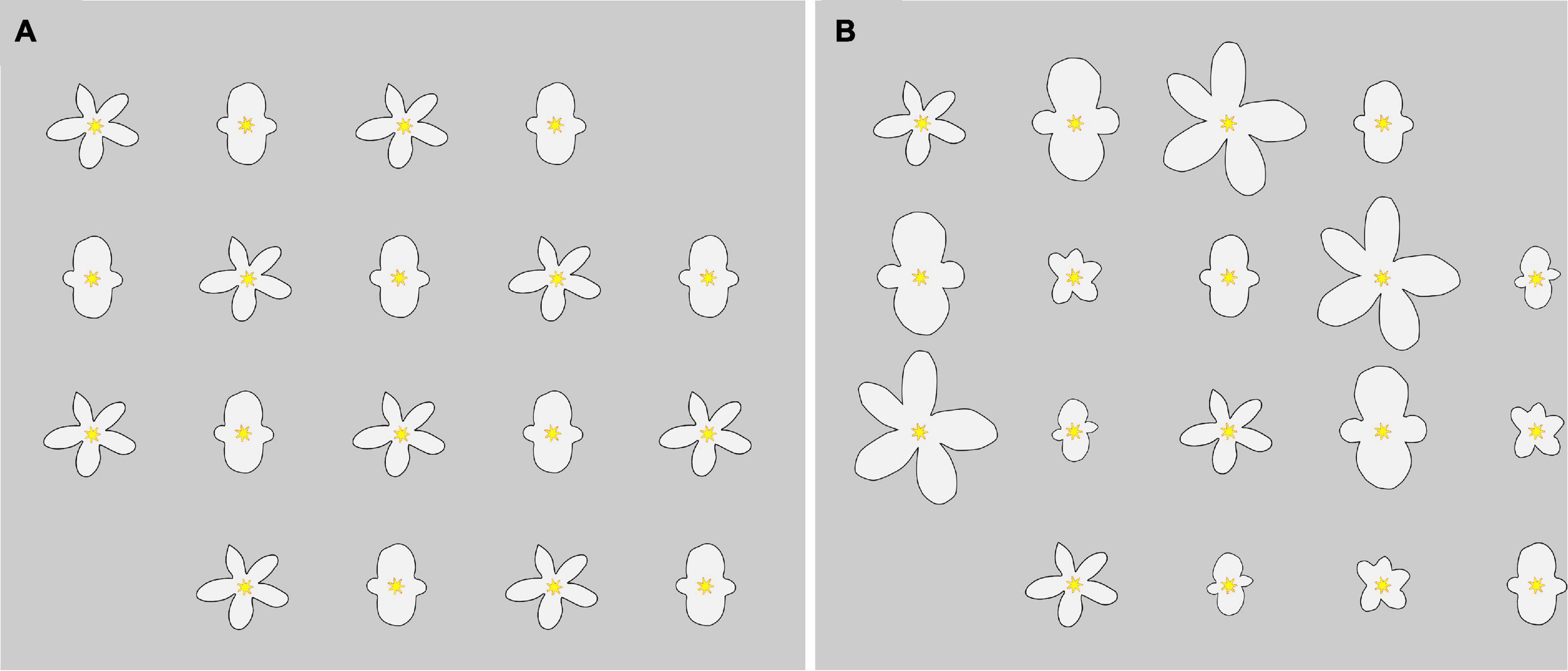
Figure 4. Two possible arrangements of flowers in a 5 × 4 Cartesian grid design for the two treatments. In the (A) no corolla size variation treatment (“control treatment”), all artificial corollas were modeled after medium-sized B. odorata male and female flowers, alternated by position. In the (B) corolla size variation treatment (“variation treatment”), artificial corollas were modeled after small, medium, and large-sized B. odorata male and female flowers, alternated by position in terms of reproductive organs and with size classes systematically distributed equivalently across the array.
We color matched artificial and live corollas using reflectance spectra of flowers and the arena wall background against which the flowers would be presented in behavioral trials. We measured a variety of plastics and papers and selected the material with qualitatively the least overall deviation from the reflectance spectra of the live flowers’ corollas. Each reflectance spectrum consisted of the mean of 10 measurements, taken from different flowers or parts of the arena wall background. All measurements were taken using an UV-VIS spectrometer (Ocean Optics USB2000) with a tungsten-deuterium light source (Ocean Optics DH2000-BAL) and a fluoropolymer white standard (WS-1-SL Spectralon; NH, United States). An RPH reflectance probe (Ocean Optics) was held at constant height and 45° angle above the samples using a holder that shielded the probe from extraneous light. All reflectance measurements were taken using a 5 ms integration time with 500 ms averaging in the same session. Irradiance within the flight arena was measured at the center of the flower array using a Q400-7-SR UV/VIS optical fiber (Ocean Optics), a CC-3-UV-S cosine-corrected (180 degrees) irradiance probe (Ocean Optics), and a tungsten-deuterium calibration light source (Ocean Optics DH-3P-CAL) and a 50 ms integration time and 50 ms averaging.
To characterize what bees perceived, we used our reflectance and irradiance measurements to plot corollas within a color space (e.g., the color hexagon) for B. impatiens following Russell et al. (2016b). In brief, the color space diagram was constructed following Chittka (1992), using receptor spectral sensitivities for B. impatiens from Skorupski and Chittka (2010) and transformed to spectral sensitivity curves following Stavenga et al. (1993). We used the arena wall on which the flowers were displayed as the background stimulus for the color hexagon and the irradiance of the overhead arena lights in calculations of receptor excitation values.
We split flower-naïve bees approximately equally among two treatments: a no corolla size variation treatment (“control treatment”) and a treatment in which corolla size varied (“variation treatment”) (Figure 4). In the control treatment, we constructed two types of artificial corollas, which were modeled after medium-sized B. odorata male and female flowers growing at the time, respectively (Figures 3, 4). In the variation treatment, we constructed six types of artificial corollas that were modeled after small, medium, and large B. odorata male and female flowers, respectively (Figures 3, 4). All artificial corollas were thus the size and shape of a sample of live flowers (petal length in mm, male: 9.1, 12.4, 15.6; female: 9.2, 15.6, 19.8; surface area in mm2, male: 183.5, 366.0, 683.6; female: 206.6, 375.5, 570.0; small, medium, and large artificial corollas, respectively). We visually inspected plants to find small and large flowers for modeling and we used their measurements to then find flowers of intermediate petal length. In both treatments, flowers were spaced 7 cm apart in a 5 × 4 Cartesian grid design on the arena wall and flower sexes (in terms of reproductive organs) were alternated by position and presented in equal frequency to bees (Figure 4). In the variation treatment, to avoid any possible position bias, we systematically distributed each flower size class equivalently across the array and changed the pattern of alternation each trial.
To initiate a behavioral trial, flowers were set up and a single flower-naïve worker bee was gently captured from the foraging arena using a 40 dram vial (Bioquip) and immediately released in the center of the test arena following Russell et al. (2017). We terminated the trial after 80 visits (or earlier if the bee stopped visiting flowers for 5 min) to avoid bees depleting models of pollen rewards. To terminate the trial we captured the bee in a 40 dram vial and euthanized it (mean 62 visits; range 7–80 visits; N = 37 bees). We tested bees individually and cleaned artificial corollas with 70% ethanol and glued on fresh reproductive parts of flowers for each trial. All trials were video recorded to permit analysis of response speed (see section “Data Analyses”).
Data Analyses
All data were analyzed using R v.4.1.0 (R Development Core Team, 2021).
To analyze flower-naïve bees’ naïve preference for models (male flowers) vs. mimics (female flowers) on their first flower landing (N = 37 bees), we used a G-test (DescTools package; Signorell et al., 2019). From all subsequent analyses we excluded four bees that did not pack pollen into their pollen baskets.
Using two different analyses, we examined how experience and corolla size variation affected the sampling behavior of initially naive bees. In the first analysis we restricted our analysis to bees that had reached a standard learning criterion of 8 of the last 10 visits made to the rewarding models, analyzing only visits up to this learning criterion (N = 26 bees). By excluding bees that failed to learn (N = 7), we reasoned we would be more likely to find evidence of how learned responses were affected by size variation. We fit a generalized linear mixed model with a binomial distribution (GLMM) using the glmmTMB() function (glmmTMB package; Magnusson et al., 2018), specifying type II Wald chi-square (χ2) tests via the Anova() function (car package; Fox, 2015). We checked model assumptions via the DHARMa package (Hartig, 2018). The response variable was sampling behavior (“correct decision,” combining correctly rejecting mimics and correctly detecting models) and the explanatory variables were “treatment” (control or variation) and “visit number” (experience). We included “bee” as a random factor, with “visit number” as repeated measures within bee (bee within colony would not converge). To examine whether corolla size variation affected the mean number of flower visits to reach the learning criterion, we fit a t-test via the t.test() function in R (assumptions of normality and equal variance were met via Shapiro-Wilk and F tests, respectively; mgcv package; Wood, 2021).
In the second analysis we fit and checked GLMMs as above to analyze how corolla size variation and experience affected the sampling behavior of all initially naïve bees (including all their visits; N = 33 bees). The response variable was sampling behavior (either “correct decision,” “correct detection,” “correct rejection,” “missed detection,” “false alarm,” “landing,” or “sonication given landing”) and the explanatory variables were “treatment” and “visit number.” We included “bee” and “colony” as random factors, with “visit number” as repeated measures within bee, within colony.
Because response time (flight time between sampling different flowers; i.e., the time between leaving a given flower and landing or rejecting the next flower, following Chittka et al., 2003) is known to trade off with discrimination accuracy in bumble bees (Chittka et al., 2003; Kulahci et al., 2008), we analyzed how corolla size variation, flower sex, discrimination accuracy, and experience affected response time by fitting a GLMM (N = 29 bees). The response variable was “response time” and the explanatory variables were “treatment,” “flower sex,” “accuracy” (whether the decision was correct or incorrect), and “visit number.” Random factors were specified as above. We added 0.1 to the response variable and log-transformed it and thereby normalized the residuals. To analyze how response time affected decision accuracy, we used a linear model (LM), with “mean correct decision” (the proportion of visits that involved a correct decision) as the response variable and “treatment” and “mean response time” as the explanatory variables. To characterize response time, we examined digital footage of behavioral trials frame by frame using Avidemux (version 2.7.6) and measured the time between the first 30 visits to the nearest 0.1 s for each behavioral trial. We timed a response starting with the bee ending its visit (i.e., having landed, the bee began beating its wings to leave, or, having approached a flower, the bee turned and accelerated away from the flower) and ending with the bee making its next visit. Of 813 measurements, we deleted 4 identified as outliers by the plot_model() function (sjPlot package; Lüdecke et al., 2021). From this analysis we also excluded 4 bees with corrupted video data or that had completed fewer than 10 approaches.
Results
Corolla Size Variation Had Little Effect on How Bees Learned to Sample
Initially flower-naïve bees strongly and significantly preferred mimics (rewardless female flowers) over models (rewarding male flowers), with 70.3% of first landings being on mimics (G test: G = 6.26, P < 0.013, N = 37 bees). Nonetheless, initially flower-naïve bees rapidly learned to discriminate between mimic and model flowers (Figure 5). Bees in both the control and corolla variation treatment that reached the learning criterion made proportionally more correct decisions (combining correctly rejecting mimics and correctly detecting models) across consecutive visits (Figure 5A; GLMM: χ21 = 12.71, P < 0.0004). However, these bees did not show any differences in learning between control and corolla variation treatments (Figure 5A; GLMM: treatment effect: χ21 = 1.47, P = 0.226; treatment × experience effect: χ21 = 0.43, P = 0.512) and both sets of bees required a similar number of flower visits to reach the learning criterion (t-test: t19.94 = 0.942, P = 0.357: mean no. visits ± SE: variation: 25 ± 3.4; control: 21 ± 2.3; N = 26 bees).
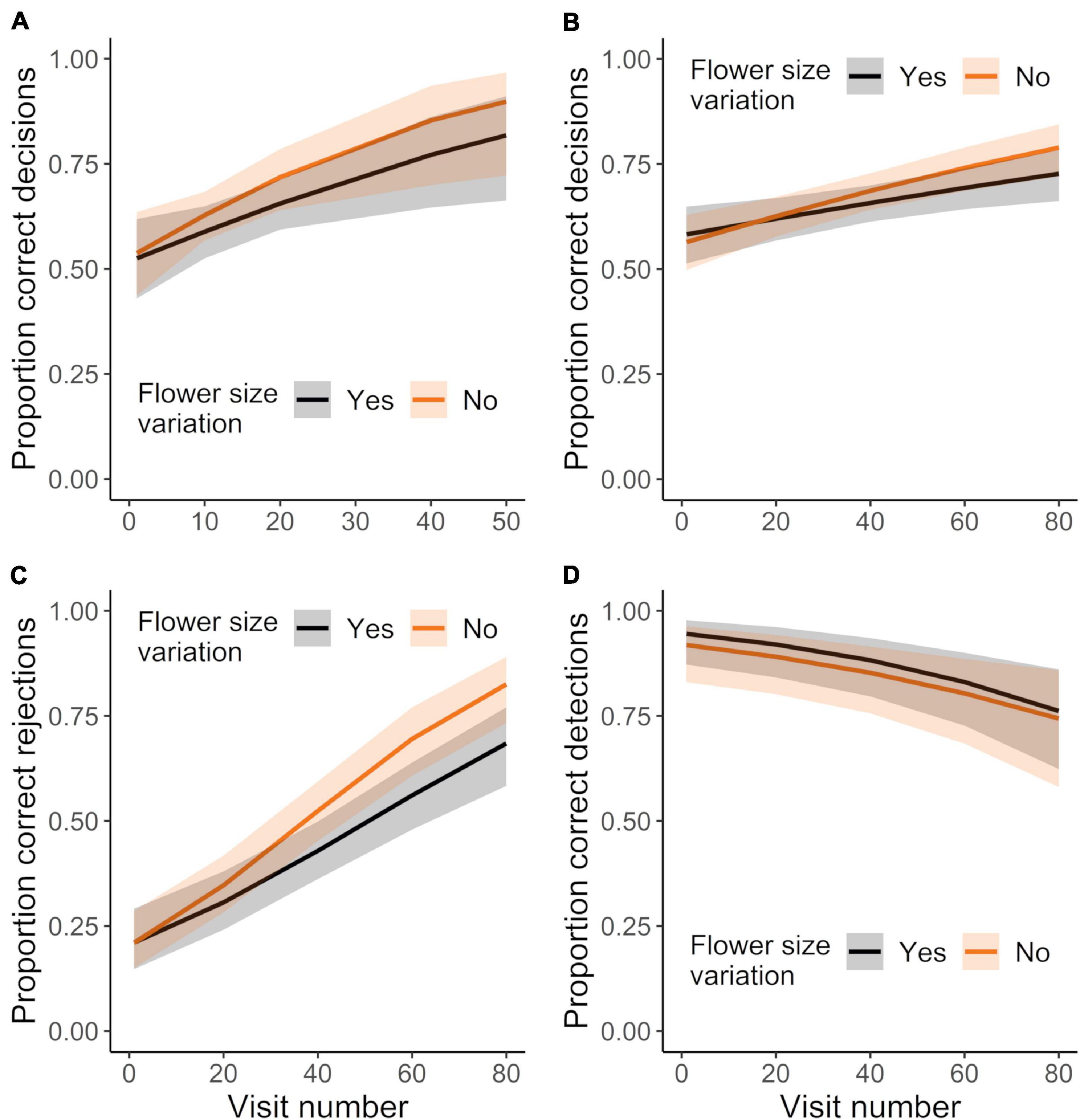
Figure 5. Sampling behavior of initially-naïve bees foraging in treatments that did or did not vary in corolla size. (A) Mean proportion of correct decisions for those bees that met the learning criterion, only considering visits up to the point of meeting the criterion. N = 14 and 12 bees in the control and corolla size variation treatment, respectively. Mean proportion of (B) correct decisions, (C) correct rejections, and (D) correct detections made by all bees making up to 80 visits. N = 15 and 18 bees in the control and variation treatment, respectively (includes those bees in panel “A”). Plotted lines indicate estimated means and shaded regions indicate 95% confidence intervals.
For subsequent analyses we assessed learning by all initially flower-naïve bees (including those that did not reach the learning criterion) across all their visits, including visits past the learning criterion. These bees also made proportionally more correct decisions with experience (Figure 5B; GLMM: χ21 = 23.53, P < 0.0001; N = 33 bees). This effect of experience was also unaffected by corolla size variation (Figure 5B; GLMM: treatment × experience effect: χ21 = 1.47, P = 0.226). Bees greatly improved their ability to correctly reject mimics with experience, but became somewhat worse at correctly detecting models (Figures 5C,D; correct rejections: χ21 = 88.96, P < 0.0001; correct detections: χ21 = 17.87, P < 0.0001). These patterns were not affected by corolla size variation (Figures 5C,D; GLMMs: correct rejections, treatment effect: χ21 = 2.78, P = 0.095; treatment × experience effect: χ21 = 2.26, P = 0.132; correct detections, treatment effect: χ21 = 0.78, P = 0.377; treatment × experience effect: χ21 = 0.24, P = 0.622).
Although bees missed more detections with experience, they nevertheless improved their proportion of landings on models relative to mimics with successive visits (Figure 6A; GLMM: χ21 = 19.51, P < 0.0001). The effect of experience did not depend on the degree of corolla variation (Figure 6A; GLMM: treatment effect: χ21 = 0.94, P = 0.331; treatment × experience effect: χ21 = 1.56, P = 0.212). Additionally, upon landing, bees sonicated mimics significantly less and models significantly more with experience (Figures 6B,C; GLMMs: sonicating mimics: χ21 = 13.56, P < 0.0003; sonicating models: χ21 = 45.15, P < 0.0001). Corolla size variation affected the pattern of bees sonicating models, but not mimics, such that bees in the variation treatment initially buzzed proportionally fewer flowers than bees in the control treatment (Figures 6B,C: GLMMs: sonicating mimics, treatment effect: χ21 = 0.82, P = 0.364; treatment × experience effect: χ21 = 0.64, P = 0.426; sonicating models, treatment effect: χ21 = 0.12, P = 0.728; treatment × experience effect: χ21 = 3.95, P < 0.047).
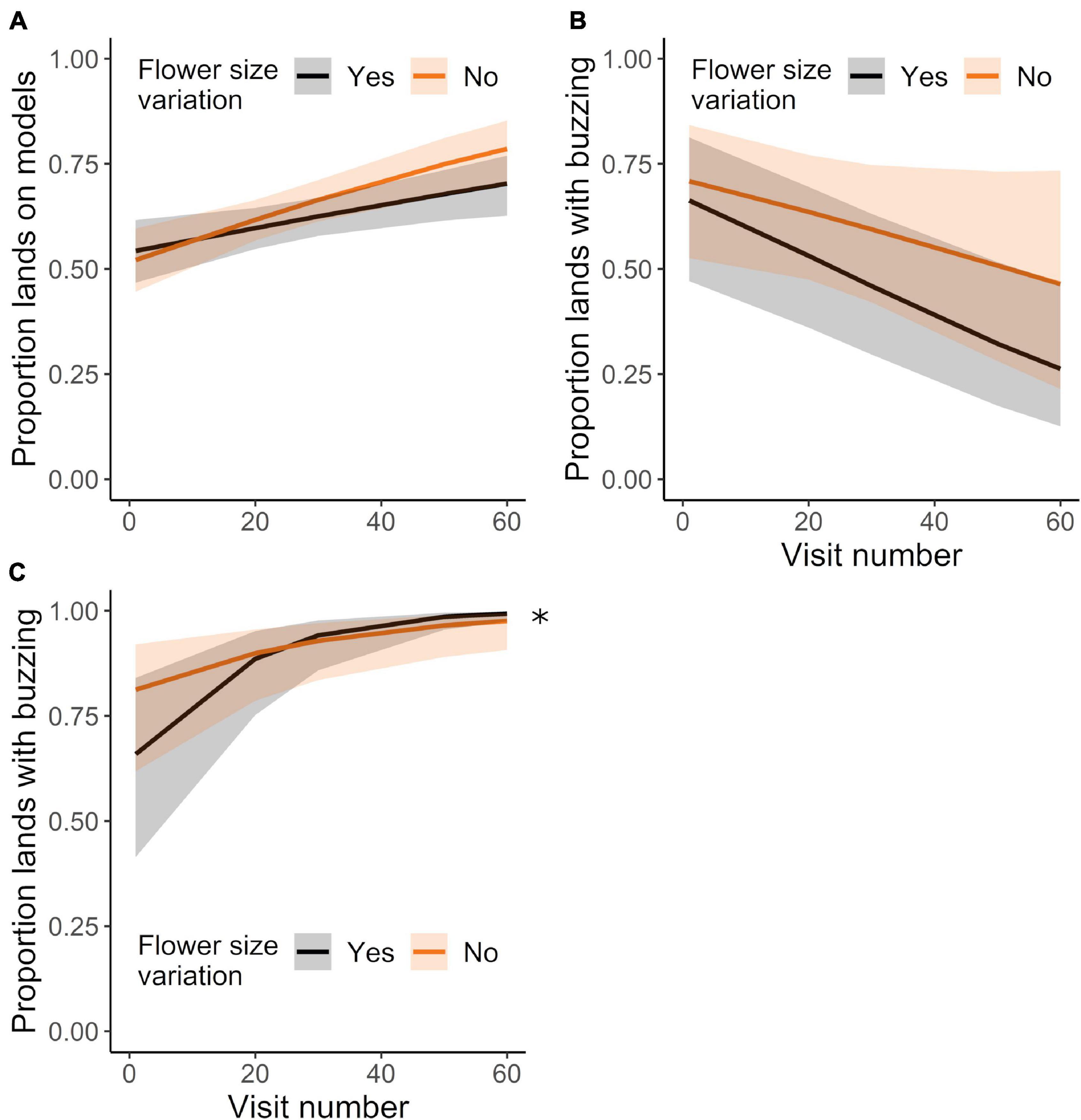
Figure 6. Landing behavior of initially-naïve bees (same dataset as in Figures 5B–D, analyzed for different sampling behavior) foraging in the control or corolla size variation treatments. Mean proportion of lands made on (A) models (vs. mimics), (B) mimics during which the bee buzzed, and on (C) models during which the bee buzzed, over the course of up to 60 landings. N = 15 and 18 bees in the control and variation treatment, respectively. Plotted lines indicate estimated means and shaded regions indicate 95% confidence intervals. Asterisk indicates a significant difference among treatments at P < 0.05.
Corolla Size Variation Had Little Effect on the Speed of Bee Responses
The general absence of differences between control and variation treatments could have been a result of bees altering their response time to maintain the accuracy of their decisions. While we found that bees responded faster with experience, response time did not significantly differ between control and variation treatments (Figure 7A; GLMM: experience effect: χ21 = 9.22, P < 0.003; treatment effect: χ21 = 0.11, P = 0.746; Table 1). However, response time decreased more quickly with experience in the control treatment vs. the variation treatment, with this effect of experience being stronger when visiting mimics (vs. models) or when the decision was incorrect vs. correct (GLMM: treatment × experience × flower sex effect: χ21 = 4.04, P < 0.045; treatment × experience × decision type effect: χ21 = 6.42, P < 0.012; Table 1). We also found that differences in response time on model and mimic flowers depended on whether the decision was correct or incorrect, such that response times to model and mimic were more different when the decision was incorrect vs. when it was correct (Figure 7B; GLMM: flower sex × decision type effect: χ21 = 27.95, P < 0.0001). Finally we did not find evidence for a speed-accuracy tradeoff: accuracy and response time were in fact negatively correlated across treatments (Figure 7C; LM: response time effect: χ21 = 7.51, P < 0.007; treatment effect: χ21 = 0.04, P = 0.839; treatment × response time effect: χ21 = 0.42, P = 0.518; R2 = 0.22).
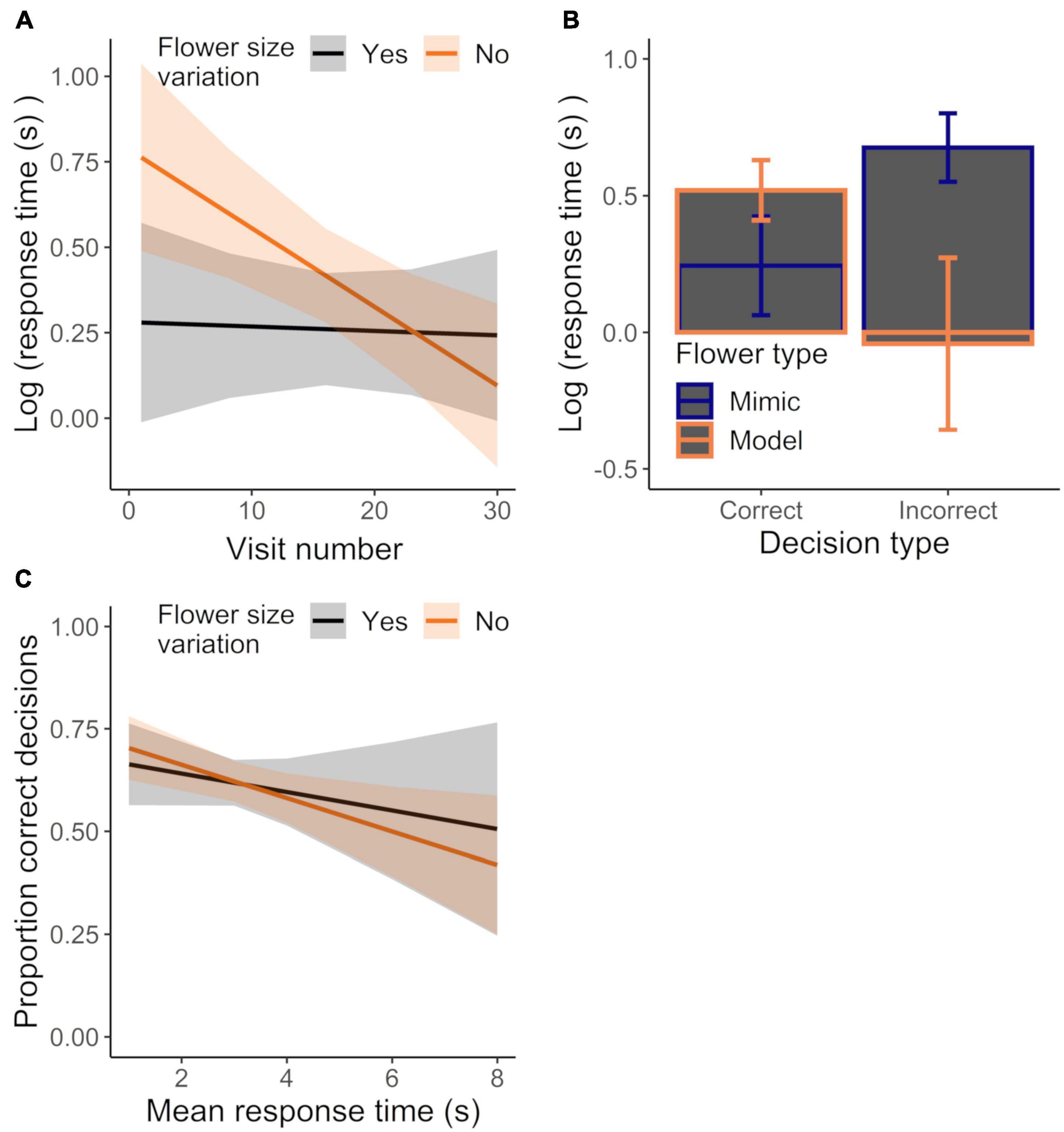
Figure 7. Effect of (A) experience on mean response time for initially-naive bees (same dataset as in Figures 5B–D) foraging in the control or corolla size variation treatments or (B) sampling behavior (incorrect vs. correct decision) and flower sex on response time, regardless of treatment. Data were analyzed in a single model, but effects of different explanatory variables are shown in separate panels for ease of presentation. (C) Mean response time plotted against the mean proportion of correct decisions for bees foraging in the control or variation treatment. N = 16 and 13 bees in the control and variation treatment, respectively. Plotted lines indicate estimated means and shaded regions indicate 95% confidence intervals.
Discussion
Generalization is thought to be a key mechanism sustaining Batesian mimicry, because when mimics resemble models well enough, receivers are expected to generalize their learned responses to models, to mimics (Ham et al., 2006; Ruxton et al., 2008; Speed and Ruxton, 2010; Rönkä et al., 2018). Signal detection theory predicts that receivers perceive signals as more similar when the signal distribution is broader, which can be a result of phenotypic variation among models and mimics (Figure 1; Lynn et al., 2005). Thus variation in model and mimic appearance should promote generalization (Amézquita et al., 2013; Gamberale-Stille et al., 2018; Arias et al., 2020), making it more difficult for bees to learn to avoid mimics (e.g., Gaskett, 2012; Paulus, 2019). We were therefore surprised that when we manipulated variation in model and mimic phenotype in an intersexual floral mimicry system, we found only modest evidence that bumble bees generalized learned responses among model and mimic flowers. Bees tended to reject mimics less when corolla size varied, consistent with variation promoting generalization. However, phenotypic variation in the mimicry did not affect learning to make more correct decisions overall or learning to make more landings on models. Variation also did not affect bees becoming worse at detecting models with experience (potentially a tradeoff with increasing avoidance of mimics, consistent with signal detection theory; Lynn et al., 2005, see also Russell et al., 2020). We also did not find much evidence that bees took longer to learn responses when corolla size varied. Only in terms of the pollen collection motor routine did bees take longer to respond appropriately. Specifically, when corolla size varied, bees buzzed models significantly less frequently, relying more on scrabbling, a less effective pollen extraction behavior (Russell et al., 2017). Because scrabbling is presumed to be less energetically expensive than buzzing (Russell et al., 2017), this response is also potentially consistent with bees “playing it safe” in response to increased uncertainty of model/mimic identity. Assuming our results are broadly representative and generalization is typically weak, intersexual floral mimicry can still be maintained when pollinators do not perfectly discriminate models and mimics and when there is a tradeoff between learning to avoid mimics and missing models, as we find here (see Russell et al., 2020 for an in-depth discussion).
Flower size in general is thought to be a salient signal for bees (Blarer et al., 2002; Armbruster et al., 2005; Gómez et al., 2008; Essenberg et al., 2015), is used by bees to discriminate among male or female flowers of other Begonia species (Schemske and Ågren, 1995; Schemske et al., 1996; Castillo et al., 2012), and differs significantly between model and mimic flowers of our study species, at least in the greenhouse (Figure 3). Why then were bee cognitive constraints only modestly affected by variation in flower size? One possibility is that experimental conditions insufficiently replicated conditions under which bees generalize. At least in terms of intrasexual difference in corolla size, surrogate models resembled live flowers. However, we manipulated corolla size using just three discrete size classes in experiments. Assuming greenhouse conditions approximated natural variation, model and mimic corolla size variation is continuous and approximately normally distributed (Figure 3). Evidence from other systems suggests that the greater the variation in the signal, the greater the generalization (e.g., Finch et al., 2016; Arias et al., 2020; Miller et al., 2020). Perhaps corolla size variation in our behavioral experiment then was too low, or too categorical, to observe generalization. Additionally, the ratio of models and mimics is thought to influence successful mimicry and could affect generalization if encounter rate influenced learning (Abbott and Sherratt, 2013; de Jager et al., 2016). However, recent work has demonstrated that Begonia sex ratio has only a marginal influence on learning (see Russell et al., 2020).
Another possibility is that intrasexual differences other than flower size might have been more salient, thus precluding generalization on the basis of corolla size. For example, corolla dissectedness (number of petals and degree of petal overlap) differs between B. odorata model and mimic, and while color (Figure 1) and scent (unpublished data; A. Mosher, T. Eltz, and A. Russell) of model and mimic reproductive parts are likely not discriminable by bees, shape may be. For instance, bees readily discriminate flowers with wide vs. narrow petals and prefer more dissected flowers (e.g., Yoshioka et al., 2007; Gómez et al., 2008). Indeed, preference for more dissected flowers might at least partly explain why bees in our study made more than 70% of their first landings on the mimics, an example of exploitation of pollinator sensory bias (consistent with Russell et al., 2020). Similarly, when flower size is a less reliable cue of flower sex (as it might be for the variation treatment), bees might have relied on other more reliable cues. Yet then bees in different treatments should probably have shown differences in their learning rate and learned responses, which they largely did not. Future work will be required to determine whether variation in traits potentially more salient than corolla size (see Essenberg et al., 2015) functions to promote generalization of model and mimic.
Alternatively, variation in flower size may in fact exploit constraints on learning, but bees in our study might have compensated by altering how they made decisions. For instance, by taking more time to respond when the learning task is more difficult, bees might have gathered enough information to make a more accurate response (i.e., a speed-accuracy tradeoff; see Abbott and Sherratt, 2013). We found that accuracy and response time were negatively correlated while bees were learning, not positively related as expected. However, this does not rule out the occurrence of a speed-accuracy tradeoff. Rather, learning may be both improving accuracy and reducing response time, and this dual effect of experience may have more than offset the expected accuracy-response time tradeoff. Accordingly, by assessing speed-accuracy tradeoffs after much of the learning has occurred (see Chittka et al., 2003; Ings and Chittka, 2008; Kulahci et al., 2008; Chittka et al., 2009), future work may reveal whether bees alter response speed to compensate for exploitation by intersexual mimicry. Of note, the change in response speed with experience did differ between corolla variation treatments, such that response time decreased more quickly in the control treatment. This result suggests learning was more difficult when corollas varied in size. Consistent with negative reinforcement driving learning, the decrease in response time was also affected more when visiting mimics and by making incorrect decisions.
In summary, we found scant evidence that flower size variation among models and mimics influenced the effectiveness of an intersexual floral mimicry. We corroborate previous work demonstrating that mimicry need not be perfect to be effective and that learning is a key mechanism by which pollinators can reduce exploitation by mimics (see Russell et al., 2020). While it appears unlikely that flower size variation is an evolved strategy on the part of the plant to exploit pollinator cognition, a fuller understanding will require disentangling effects of variation on cognition from speed-accuracy tradeoffs. Batesian models and mimics, including, but not limited to intersexual mimicry, often exhibit striking phenotypic variation, including in color, pattern, and size, and this variation is thought to be important in driving the evolution of mimicry (e.g., Heal, 1982; Joron and Mallet, 1998; Lynn et al., 2005; Penney et al., 2012; Kikuchi and Pfennig, 2013). Yet how phenotypic variation interacts with receiver cognition has only rarely been considered (but see Lynn et al., 2005; Abbott and Sherratt, 2013). Thus, while our study provides rare experimental evidence that phenotypic variation may not necessarily affect receiver cognition, it also indicates that additional investigation of this potential interaction is warranted.
Data Availability Statement
The original contributions presented in the study are included in the article/Supplementary Material, further inquiries can be directed to the corresponding author/s.
Author Contributions
SS, LW, AR, and DP designed the study. SS, LW, and AR performed the research. AR performed the analyses and wrote the first draft of the manuscript. All authors revised the manuscript.
Funding
This work was supported by the Missouri State University and a Sigma Xi Grant in Aid of Research, awarded to SS.
Conflict of Interest
The authors declare that the research was conducted in the absence of any commercial or financial relationships that could be construed as a potential conflict of interest.
Publisher’s Note
All claims expressed in this article are solely those of the authors and do not necessarily represent those of their affiliated organizations, or those of the publisher, the editors and the reviewers. Any product that may be evaluated in this article, or claim that may be made by its manufacturer, is not guaranteed or endorsed by the publisher.
Acknowledgments
We are grateful to the Koppert Biologicals for the bee colonies, the Chicago Botanic Garden for plants, and Rachel Wilkins for assistance with flower measurements. We acknowledge this work was performed on unceded traditional territory of the Osage.
Supplementary Material
The Supplementary Material for this article can be found online at: https://www.frontiersin.org/articles/10.3389/fevo.2021.724712/full#supplementary-material
Footnotes
References
Abbott, K. R., and Sherratt, T. N. (2013). Optimal sampling and signal detection: unifying models of attention and speed– accuracy trade-offs. Behav. Ecol. 24, 605–616. doi: 10.1093/beheco/art001
Ågren, J., and Schemske, D. W. (1995). Sex allocation in the monoecious herb Begonia semiovata. Evolution 49, 121–130. doi: 10.1111/j.1558-5646.1995.tb05964.x
Amézquita, A., Castro, L., Arias, M., González, M., and Esquivel, C. (2013). Field but not lab paradigms support generalisation by predators of aposematic polymorphic prey: the Oophaga histrionica complex. Evol. Ecol. 27, 769–782. doi: 10.1007/s10682-013-9635-1
Arias, M., Davey, J. W., Martin, S., Jiggins, C., Nadeau, N., Joron, M., et al. (2020). How do predators generalize warning signals in simple and complex prey communities? Insights from a videogame. Proc. Roy. Soc. B Biol. Sci. 287:20200014. doi: 10.1098/rspb.2020.0014
Armbruster, W. S., Antonsen, L., and Pélabon, C. (2005). Phenotypic selection on Dalechampia blossoms: honest signaling affects pollination success. Ecology 86, 3323–3333. doi: 10.1890/04-1873
Ayasse, M., Schiestl, F. P., Paulus, H. F., Löfstedt, C., Hansson, B., Ibarra, F., et al. (2000). Evolution of reproductive strategies in the sexually deceptive orchid Ophrys sphegodes: how does flower-specific variation of odor signals influence reproductive success? Evolution 54, 1995–2006. doi: 10.1111/j.0014-3820.2000.tb01243.x
Barker, J. L., Dornhaus, A., Bronstein, J. L., and Muth, F. (2018). Learning about larceny: experience can bias bumble bees to rob nectar. Behav. Ecol. Sociobiol. 72:68.
Blarer, A., Keasar, T., and Shmida, A. (2002). Possible mechanisms for the formation of flower size preferences by foraging bumblebees. Ethology 108, 341–351. doi: 10.1046/j.1439-0310.2002.00778.x
Bronstein, J. L. (2001). The exploitation of mutualisms. Ecol. Lett. 4, 277–287. doi: 10.1046/j.1461-0248.2001.00218.x
Castillo, R. A., Caballero, H., Karina, B., Fornoni, J., and Domínguez, C. A. (2012). How to cheat when you cannot lie? Deceit pollination in Begonia gracilis. Oecologia 169, 773–782. doi: 10.1007/s00442-012-2250-y
Cheng, K. (2002). Generalization: mechanistic and functional explanations. Anim. Cogn. 5, 33–40. doi: 10.1007/s10071-001-0122-7
Chittka, L. (1992). The color hexagon: a chromaticity diagram based on photoreceptor excitations as a generalized representation of color opponency. J. Comp. Physiol. A 170, 533–543.
Chittka, L., Dyer, A. G., Bock, F., and Dornhaus, A. (2003). Bees trade off foraging speed for accuracy. Nature 424:388. doi: 10.1038/424388a
Chittka, L., Skorupski, P., and Raine, N. E. (2009). Speed–accuracy tradeoffs in animal decision making. Trends Ecol. Evol. 24, 400–407. doi: 10.1016/j.tree.2009.02.010
de Avila, R. Jr., Oleques, S. S., Marciniak, B., and Ribeiro, J. R. I. (2017). Effects of model-mimic frequency on insect visitation and plant reproduction in a self-mimicry pollination system. Ann. Bot. 9:lx044. doi: 10.1093/aobpla/plx044
de Jager, M., Newman, E., Theron, G., Botha, P., Barton, M., and Anderson, B. (2016). Pollinators can prefer rewarding models to mimics: consequences for the assumptions of Batesian floral mimicry. Plant Syst. Evol. 302, 409–418. doi: 10.1007/s00606-015-1276-0
de Jager, M. L., and Anderson, B. (2019). When is resemblance mimicry? Funct. Ecol. 39, 1586–1596. doi: 10.1111/1365-2435.13346
de Jager, M. L., and Ellis, A. G. (2014). Costs of deception and learned resistance in deceptive interactions. Proc. R. Soc. B 281:20132861. doi: 10.1098/rspb.2013.2861
Dixit, T., Riederer, J. M., Quek, Q., Belford, K., de Wand, T. T., Sicat, R., et al. (2020). Plasticity in flower size as an adaptation to variation in pollinator specificity. Ecol. Entom. 45, 1367–1372. doi: 10.1111/een.12921
Dukas, R. (1987). Foraging behavior of three bee species in a natural mimicry system: female flowers which mimic male flowers in Ecballium elaterium (Cucurbitaceae). Oecologia 74, 256–263. doi: 10.1007/bf00379368
Enquist, M., and Johnstone, R. A. (1997). Generalization and the evolution of symmetry preferences. Proc. Roy. Soc. B 264, 1345–1348. doi: 10.1098/rspb.1997.0186
Essenberg, C. J. (2021). Intraspecific relationships between floral signals and rewards with implications for plant fitness. Ann. Bot. 13:lab006. doi: 10.1093/aobpla/plab006
Essenberg, C. J., Easter, R. A., Simmons, R. A., and Papaj, D. R. (2015). The value of information in floral cues: bumblebee learning of floral size cues. Behav. Ecol. 26, 1335–1344. doi: 10.1093/beheco/arv061
Finch, D. D., Carvalho, P. F., and Goldstone, R. L. (2016). “Variability in category learning: the effect of context change and item variation on knowledge generalization,” in Paper Presented at the Cognitive Science 2016 Database, (Bloomington, IN).
Fox, J. (2015). Applied Regression Analysis and Generalized Linear Models, 3rd Edn. London: Sage Publications, Inc.
Galen, C. (1999). The functional ecology of variation in flower size and form within natural plant populations. BioScience 49, 631–640.
Gamberale-Stille, G., Kazemi, B., Balogh, A., and Leimar, O. (2018). Biased generalization of salient traits drives the evolution of warning signals. Proc. R. Soc B. Biol. Sci. 285:20180283. doi: 10.1098/rspb.2018.0283
Gaskett, A. C. (2012). Floral shape mimicry and variation in sexually deceptive orchids with a shared pollinator. Biol. J. Linn. Soc. 106, 469–481. doi: 10.1111/j.1095-8312.2012.01902.x
Gigord, L. D. B., Macnair, M. R., and Smithson, A. (2001). Negative frequency-dependent selection maintains a dramatic flower color polymorphism in the rewardless orchid Dactylorhiza sambucina (L.) Soó. Proc. Nat. Acad. Sci. U.S.A. 98, 6253–6255. doi: 10.1073/pnas.111162598
Gómez, J. M., Bosch, J., Perfectti, F., Fernandez, J. D., Abdelaziz, M., and Camacho, J. P. M. (2008). Association between floral traits and rewards in Erysimum mediohispanicum (Brassicaceae). Ann. Bot. 101, 1413–1420. doi: 10.1093/aob/mcn053
Goodrich, K. R., and Jurgens, A. (2017). Pollination systems involving floral mimicry of fruit: aspects of their ecology and evolution. New Phytologist 217, 74–81. doi: 10.1111/nph.14821
Ham, A. D., Ihalainen, E., Lindstrom, L., and Mappes, J. (2006). Does colour matter? The importance of colour in avoidance learning, memorability and generalisation. Behav. Ecol. Sociobiol. 60, 482–491. doi: 10.1007/s00265-006-0190-4
Hartig, F. (2018). DHARMa: Residual Diagnostics for Hierarchical (Multi-Level / Mixed) Regression Models. R package version 0.1.6. Available online at: https://cran.r-project.org/web/packages/DHARMa/index.html (accessed May, 2021).
Hattori, M., Nagano, Y., Shinohara, Y., and Itino, T. (2016). Pattern of flower size variation along an altitudinal gradient differs between Impatiens textori and Impatiensnoli-tangere. J. Plant Interact. 11, 152–157. doi: 10.1080/17429145.2016.1226437
Heal, J. R. (1982). Colour patterns of Syprhidae: IV. mimicry and variation in natural populations of Eristalis tenax. Heredity 49, 95–109. doi: 10.1038/hdy.1982.68
Ings, T. C., and Chittka, L. (2008). Speed-accuracy tradeoffs and false alarms in bee responses to cryptic predators. Curr. Biol. 18, 1520–1524. doi: 10.1016/j.cub.2008.07.074
Joron, M., and Mallet, J. L. B. (1998). Diversity in mimicry: paradox or paradigm? Tree 13, 461–467. doi: 10.1016/s0169-5347(98)01483-9
Kalish, H. I. (1969). “Generalization as an epiphenomenon,” in Learning Processes, ed. M. H. Marx (New York, NY: Macmillan), 207–297.
Kikuchi, D. W., and Pfennig, D. W. (2013). Imperfect mimicry and the limits of natural selection. Q. Rev. Biol. 88, 297–315. doi: 10.1086/673758
Kulahci, I. G., Dornhaus, A., and Papaj, D. R. (2008). Multimodal signals enhance decision making in foraging bumble-bees. Proc. Biol. Sci. 275, 797–802. doi: 10.1098/rspb.2007.1176
Kunze, J., and Gumbert, A. (2000). The combined effect of color and odor on flower choice behavior of bumble bees in flower mimicry systems. Behav. Ecol. 12, 447–456. doi: 10.1093/beheco/12.4.447
Lüdecke, D., Bartel, A., Schwemmer, C., Powell, C., Kjalovski, A., and Titz, J. (2021). sjPlot: Data Visualization for Statistics in Social Science. R Package Version 2.8.8. Available online at: https://cran.r-project.org/web/packages/sjPlot/index.html (accessed May, 2021).
Lynn, S. K., Cnaani, J., and Papaj, D. R. (2005). Peak shift discrimination learning as a mechanism of signal evolution. Evolution. 1300–1305.
Magnusson, A., Skaug, H., Nielsen, A., Berg, C., Kristensen, K., Maechler, M., et al. (2018). glmmTMB: Generalized Linear Mixed Models using Template Model Builder. R Package Version 1.0.2.1. Available online at: https://cran.r-project.org/web/packages/glmmTMB/index.html (accessed May, 2021).
Maloof, J. E., and Inouye, D. W. (2000). Are nectar robbers cheaters or mutualists? Ecology 81, 2651–2661. doi: 10.1890/0012-9658(2000)081[2651:anrcom]2.0.co;2
Miller, I. D., Dumay, N., Pitt, M., Lam, B., and Armstrong, B. C. (2020). “Context variability promotes generalization in reading aloud: insight from a neural network simulation,” in Proceedings of the 42nd Annual Meeting of the Cognitive Science 2020 database, (Armstrong, BC: Cognitive Science Society).
Paulus, H. F. (2019). Speciation, pattern recognition and the maximization of pollination: general questions and answers given by the reproductive biology of the orchid genus Ophrys. J. Comp. Physiol. A Neuroethol. Sens. Neural Behav Physiol. 205, 285–300. doi: 10.1007/s00359-019-01350-4
Pemberton, R. W., and Wheeler, G. S. (2006). Orchid bees don’t need orchids: evidence from the naturalization of an orchid bee in Florida. Ecology 87, 1995–2001. doi: 10.1890/0012-9658(2006)87[1995:obdnoe]2.0.co;2
Penney, H. D., Hassall, C., Skevington, J. H., Abbott, K. R., and Sherratt, T. N. (2012). A comparative analysis of the evolution of imperfect mimicry. Nature 483, 461–464. doi: 10.1038/nature10961
R Development Core Team. (2021). R: A Language and Environment for Statistical Computing.: R Foundation for Statistical Computing. Vienna: R Development Core Team.
Rönkä, K., De Pasqual, C., Mappes, J., Gordon, S., and Rojas, B. (2018). Colour alone matters: no predator generalization among morphs of an aposematic moth. Anim. Behav. 135, 153–163. doi: 10.1016/j.anbehav.2017.11.015
Russell, A. L., Buchmann, S. L., and Papaj, D. R. (2017). How a generalist bee achieves high efficiency of pollen collection on diverse floral resources. Behav. Ecol. 28, 991–1003. doi: 10.1093/beheco/arx058
Russell, A. L., Kikuchi, D. W., Giebink, N. W., and Papaj, D. R. (2020). Sensory bias and signal detection tradeoffs maintain intersexual floral mimicry. Philos. Trans. R. Soc. Lond. B Biol. Sci. 375:20190469. doi: 10.1098/rstb.2019.0469
Russell, A. L., Leonard, A. S., Gillette, H. D., and Papaj, D. R. (2016a). Concealed floral rewards and the role of experience in floral sonication by bees. Anim. Behav. 120, 83–91. doi: 10.1016/j.anbehav.2016.07.024
Russell, A. L., Newman, C. R., and Papaj, D. R. (2016b). White flowers finish last: pollen-foraging bumble bees show biased learning in a floral color polymorphism. Evol. Ecol. 31, 173–191. doi: 10.1007/s10682-016-9848-1
Ruxton, G. D., Franks, D. W., Balogh, A. C., and Leimar, O. (2008). Evolutionary implications of the form of predator generalization for aposematic signals and mimicry in prey. Evolution 62, 2913–2921. doi: 10.1111/j.1558-5646.2008.00485.x
Schaefer, H. M., and Ruxton, G. D. (2010). Deception in plants: mimicry or perceptual exploitation? Trends Ecol. Evol. 24, 676–685. doi: 10.1016/j.tree.2009.06.006
Schemske, D. W., and Ågren, J. (1995). Deceit pollination and selection on female flower size in Begonia involucrata: an experimental approach. Evolution 49, 207–214. doi: 10.2307/2410306
Schemske, D. W., Ågren, J., and Le Corff, J. (1996). “Deceit Pollination in the monoecious, neotropical herb Begonia oaxacana (Begoniaceae),” in Floral Biology: Studies on Floral Evolution in Animal-Pollinated Plants, eds D. G. Lloyd and S. C. H. Barrett (Boston, MA: Chapman and Hall), 292–318. doi: 10.1007/978-1-4613-1165-2_11
Schiestl, F. P. (2005). On the success of a swindle: pollination by deception in orchids. Naturwissenschaften 92, 255–264. doi: 10.1007/s00114-005-0636-y
Schiestl, F. P., and Johnson, S. D. (2013). Pollinator-mediated evolution of floral signals. Trends. Ecol. Evol. 28, 307–315. doi: 10.1016/j.tree.2013.01.019
Sherratt, T. N. (2002). The evolution of imperfect mimicry. Behav. Ecol. 6, 821–826. doi: 10.1093/beheco/13.6.821
Signorell, A., Aho, K., Alfons, A., Anderegg, N., Aragon, T., Arachchige, C., et al. (2019). DescTools: Tools for Descriptive Statistics. R Package Version 0.99.30. Available online at: https://cran.r-project.org/web/packages/DescTools/index.html (accessed May, 2021).
Simpson, B. B., and Neff, J. L. (1981). Floral rewards: alternatives to pollen and nectar. Ann. Miss. Bot. Garden 68, 301–322. doi: 10.2307/2398800
Skorupski, P., and Chittka, L. (2010). Photoreceptor spectral sensitivity in the bumblebee, Bombus impatiens (Hymenoptera: Apidae). PLoS One 5:e12049. doi: 10.1371/journal.pone.0012049
Smithson, A., and Macnair, M. R. (1997). Negative frequency-dependent selection by pollinators on artificial flowers without rewards. Evolution 51, 715–723. doi: 10.2307/2411148
Speed, M. P., and Ruxton, G. D. (2010). Imperfect Batesian mimicry and the conspicuousness costs of mimetic resemblance. Am. Nat. 176, E1–E14.
Stavenga, D. G., Smiths, R. P., and Hoenders, B. J. (1993). Simple exponential functions describing the absorbance bands of visual pigment spectra. Vis. Res. 33, 1011–1017. doi: 10.1016/0042-6989(93)90237-q
Thompson, J. N., Schwind, C., Guimaraes, P. R. Jr., and Friberg, M. (2013). Diversification through multitrait evolution in a coevolving interaction. Proc. Natl. Acad. Sci. U.S.A. 110, 11487–11492. doi: 10.1073/pnas.1307451110
van der Kooi, C. J., Vallejo-Marin, M., and Leonhardt, S. D. (2021). Mutualisms and (a)symmetry in plant–pollinator interactions. Curr. Biol. 31, R91–R99. doi: 10.1016/j.cub.2020.11.020
Whitehead, M. R., and Peakall, R. (2012). Short-term but not long-term patch avoidance in an orchid-pollinating solitary wasp. Behav. Ecol. 24, 162–168. doi: 10.1093/beheco/ars149
Wood, S. (2021). mgcv: Mixed GAM Computation Vehicle with Automatic Smoothness Estimation. R Package Version 1.8-36. Available online at: https://cran.r-project.org/web/packages/mgcv/index.html (accessed May, 2021).
Wright, G. A., and Smith, B. H. (2003). Variation in complex olfactory stimuli and its influence on odour recognition. Proc. R. Soc. Lond. B Biol. Sci. 271, 147–152. doi: 10.1098/rspb.2003.2590
Wyatt, G. E., and Sazima, M. (2011). Pollination and reproductive biology of thirteen species of Begonia in the Serra do Mar state park. São Paulo, Brazil. J. Pollinat. Ecol. 6, 95–107.
Keywords: signal detection, Batesian mimicry, cognition, learning, intersexual mimicry, imperfect mimicry, flower size, generalization
Citation: Russell AL, Sanders SR, Wilson LA and Papaj DR (2021) The Size of it: Scant Evidence That Flower Size Variation Affects Deception in Intersexual Floral Mimicry. Front. Ecol. Evol. 9:724712. doi: 10.3389/fevo.2021.724712
Received: 14 June 2021; Accepted: 05 August 2021;
Published: 25 August 2021.
Edited by:
Casper J. Van Der Kooi, University of Groningen, NetherlandsReviewed by:
Scarlett Howard, Deakin University, AustraliaJurene Ellen Kemp, University of Stirling, United Kingdom
Copyright © 2021 Russell, Sanders, Wilson and Papaj. This is an open-access article distributed under the terms of the Creative Commons Attribution License (CC BY). The use, distribution or reproduction in other forums is permitted, provided the original author(s) and the copyright owner(s) are credited and that the original publication in this journal is cited, in accordance with accepted academic practice. No use, distribution or reproduction is permitted which does not comply with these terms.
*Correspondence: Avery L. Russell, QXZlcnlSdXNzZWxsQG1pc3NvdXJpc3RhdGUuZWR1
†These authors share first authorship