- 1Department of Plant Biosecurity, College of Plant Protection, China Agricultural University, Beijing, China
- 2School of Biology and Environmental Sciences, Queensland University of Technology (QUT), Brisbane, QLD, Australia
Fruit flies are a well-known invasive species, and climate-based risk modeling is used to inform risk analysis of these pests. However, such research tends to focus on already well-known invasive species. This paper illustrates that appropriate risk modeling can also provide valuable insights for flies which are not yet “on the radar.” Carpomya pardalina is a locally important cucurbit-infesting fruit fly of western and central Asia, but it may present a risk to other temperate countries where melons are grown. MaxEnt models were used to map the risk area for this species under historical and future climate conditions averaged from three global climate models under two shared socio-economic pathways in 2030 and 2070 from higher climate sensitivity models based on the upcoming 2021 IPCC sixth assessment report. The results showed that a total of 47.64% of the world’s land mass is climatically suitable for the fly; it could establish widely around the globe both under current and future climates with host availability. Our MaxEnt modeling highlights particularly that Western China, Russia, and other European countries should pay attention to this currently lesser-known melon fly and the melons exported from the present countries. The current and expanding melon trade could offer direct invasion pathways to those regions. While this study offers specific risk information on C. pardalina, it also illustrates the value of applying climate-based distribution modeling to species with limited geographic distributions.
Introduction
Climate change and biological invasion are two interlinked global challenges. Invasive species can cause far-reaching ecological and economic impacts in invaded regions (Mack et al., 2000; Cook et al., 2007; Hulme, 2009), while climate change can assist invasive species by increasing their probability of establishing if areas which are currently environmentally unsuitable become more suitable (Early et al., 2016; Hulme, 2017). This is particularly true for insects, which depend on local environmental conditions for survival and development within their thermal limits (McGeoch et al., 2010; Cornelissen et al., 2019). Because of the negative impacts pest insects have on agriculture, and their well-documented invasiveness (Bradshaw et al., 2016), studies on the impacts of climate change on the distribution of agricultural insects is considered a fundamental aspect of assessing the future risk they pose (Biber-Freudenberger et al., 2016; Wang et al., 2017; Qin et al., 2019; Santana et al., 2019).
The tephritid fruit flies (Diptera: Tephritidae) include approximately 250 species of economic importance (White and Elson-Harris, 1992). Frugivorous tephritids lay their eggs into fruit, where the subsequent larvae develop, sometimes resulting in almost total crop failure (Liang, 2011). Because tephritid eggs and larvae can be moved within fruit via trade or personal carriage they are highly invasive and of quarantine concern to many countries around the globe (Papadopoulos, 2014; Clarke, 2019). Fruit flies attract a great deal of attention in the fields of plant quarantine and invasion biology in order to limit their further spread and the huge damage they can cause (Stanaway et al., 2001; Qin et al., 2015). Well-known fruit fly pests, such as the Oriental fruit fly (Bactrocera dorsalis), Mediterranean fruit fly (Ceratitis capitata), and melon fly (Zeugodacus cucurbitae), have had their current and potential future distributions modeled (Hill et al., 2016) as a direct aid to quarantine and risk assessment (Biosecurity Australia, 2009; Baker et al., 2019). However, the vast bulk of the “lesser” pest fruit flies tend to fall under quarantine raiders. In this study we model the potential distribution of one such fly to illustrate this point.
Carpomya pardalina (Bigot, 1891) (Diptera: Tephritidae) (a.k.a. the Baluchistan Melon fly or the Russian melon fly) is a cucurbit pest of far-western Europe and western and central Asia. Originally described from Baluchistan, an area extending from southeastern Iran to western Pakistan (EPPO, 2013), C. pardalina has spread to central and west Asia and the recent movement of this fly into southern Kazakhstan threatens economically important cucurbit crops which are grown for export to Europe and Russia (Toyzhigitova et al., 2019). The main host of C. pardalina is Cucumis melo (melon), and can also attack other cultivated Cucurbitaceae including Citrullus lanatus (watermelon), Cucumis melo var. flexuosus (snake melon), Cucumis sativus (cucumber), as well as weeds (Cucumis trigonus, Ecballium elaterium) (EPPO, 2013). Females lay viable eggs into melons, the larvae then feed on the seed cavity, with infested melons turning brown disrupting the taste and aroma of melons (Stonehouse et al., 2006; Baris and Cobanoglu, 2013; Baris et al., 2016). Generally, the pest causes crop losses of around 10–25%, but crop losses of up to 100% can occur (Toyzhigitova et al., 2019). During summer, there may be two to three overlapping generations (even four in southern and eastern Iran), the generation durations lasting approximately 30 days (Baris and Cobanoglu, 2013; EPPO, 2013; Toyzhigitova et al., 2017). During winter, C. pardalina survives snowy and sub-zero temperatures as an overwintering pupa at a depth of 5–15 cm, and prefers the first 6 cm of soil (Stonehouse et al., 2006; Baris and Cobanoglu, 2013). While currently with a restricted geographic distribution, the fly constitutes a potential but currently unknown risk to other regions where melons are grown. Carpomya pardalina was formerly on the EPPO Alert list (EPPO, 2013), but has since been removed. The fly is listed as a quarantine pest in China, but the extent of the risk the fly poses to that country is unknown.
Maximum entropy (MaxEnt) (Phillips et al., 2004) is one of the most popular tools for species distribution and environmental niche modeling, with thousands of applications published (Merow et al., 2013). MaxEnt requires only species occurrence and environmental data to predict the potential distribution of the species and allows for the exploration of the climate change effects on a species’ future distribution (Elith et al., 2011). Compared with other methods, MaxEnt is popular because it is considered to produce robust results with sparse, irregularly sampled data, and minor location errors (Hernandez et al., 2006; Elith et al., 2011). In this study, we applied MaxEnt to assess the habitat suitability of C. pardalina at a global scale and projected climate change impacts on the species’ risk area; host availability was also considered in order to provide the basis for future management of this pest.
Materials and Methods
Occurrence Data and Climate Data
The species occurrence data of C. pardalina were obtained from the EPPO Global Database (EPPO)1 and literature (Akkaya and Uygun, 1999; Stonehouse et al., 2006; Pavlov, 2012; Baris et al., 2016; Toyzhigitova et al., 2019). A total of 34 occurrence points of C. pardalina were identified across the following countries: Afghanistan, India, Iran, Iraq, Jordan, Kazakhstan, Kyrgyzstan, Lebanon, Pakistan, Syria, Tajikistan, Turkmenistan, Uzbekistan, Armenia, Azerbaijan, Cyprus, Georgia, central and southern Russia, Turkey, and Ukraine (Supplementary Table 1 and Figure 1A). The occurrence data were assigned to 9 km × 9 km climate data grids in ArcGIS 10.2 (ESRI Inc., Redlands, CA, United States) to reduce spatial autocorrelation and sample bias.
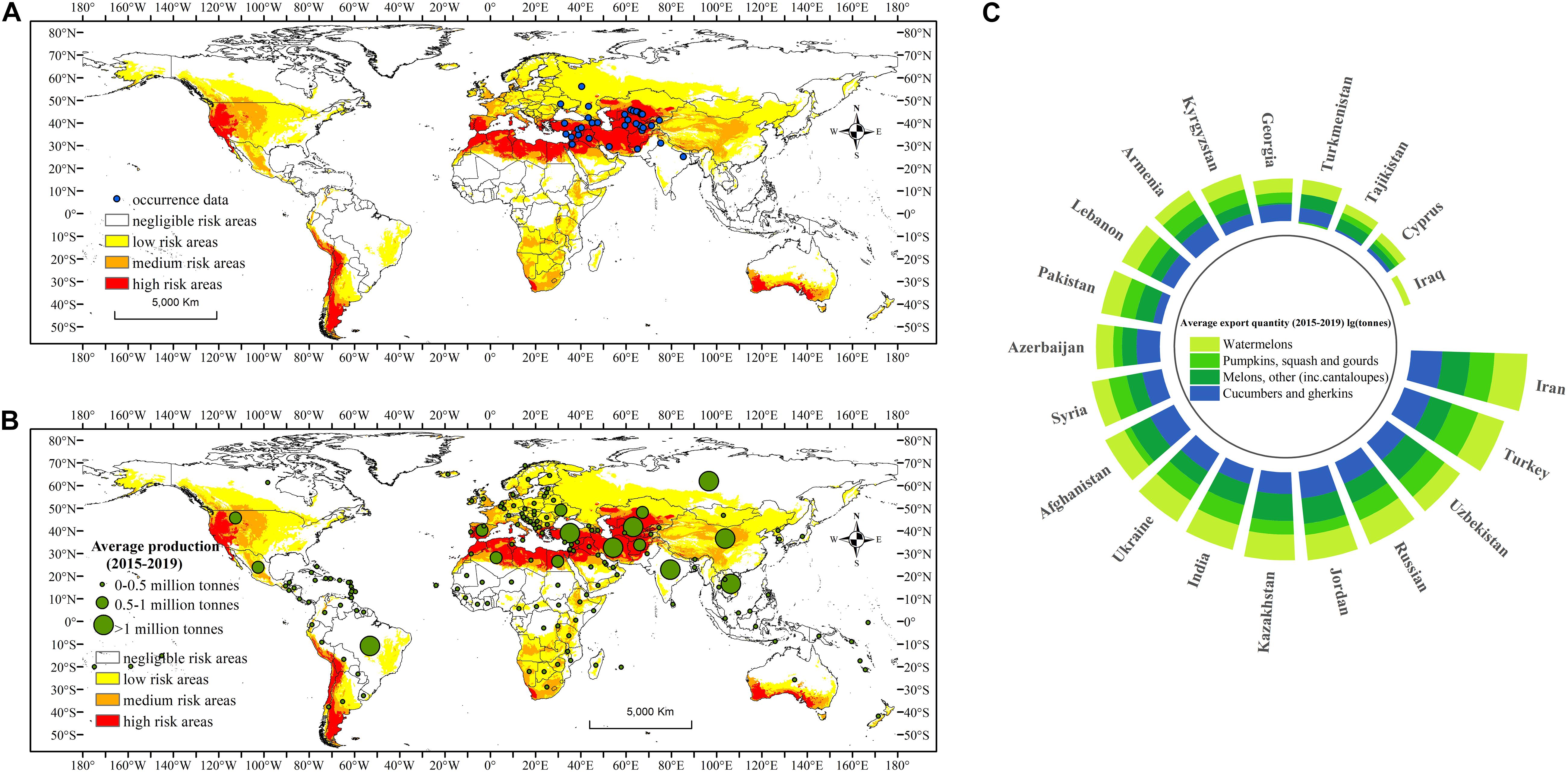
Figure 1. Potential geographical distribution of Carpomya pardalina based on MaxEnt (v3.4.4) predictions using near-current climate data (averaged from 1970 to 2000). White indicates negligible risk areas (0.00–0.08), yellow indicates low risk areas (0.08–0.23), orange indicates medium risk areas (0.23–0.62), and red indicates high risk areas (0.62–1.00). (A) Blue points are occurrence data used to build and evaluate the model. (B) Green points are host production data averaged from 2015 to 2019. (C) Host export quantity of present countries averaged from 2015 to 2019 after logarithm.
Climate data were accessed from the WorldClim website2 version 2.1. Historical (near current) climate data included 19 bioclimatic variables with a spatial resolution at 5 arc-min (9 km at the equator) which were the average monthly climate data for minimum, mean, and maximum temperature and precipitation for the period 1970–2000 (Fick and Hijmans, 2017). Multicollinearity among climate variables could hinder species-environment relationships analysis (Heikkinen et al., 2006). Principal component analysis (PCA) and correlation analysis were conducted in IBM SPSS Statistics version 223 to select a set of variables with Pearson correlation coefficients having absolute values < 0.8 that were uncorrected and eco-physiologically relevant for modeling (Qin et al., 2019).
Future climate conditions were assessed with global climate model (GCM) data downscaled from Coupled Model Intercomparison Projects (CMIP) 6 (World Climate Research Programme)4 with WorldClim v2.1 as the baseline climate. The 2013 IPCC fifth assessment report (AR5) featured climate models from CMIP5, while the upcoming 2021 IPCC sixth assessment report (AR6) will feature the new state-of-the-art CMIP6 models. The (CMIP) 6 models used in our study have notably higher climate sensitivity than models in CMIP5, and contribute to the projections of greater warming in this century (around 0.4°C warmer than similar scenarios run in CMIP5) (Eyring et al., 2016; Hausfather, 2019), which is why we chose them. To reduce the uncertainties arising from different global climate model (GCM) projections (Guisan et al., 2013), we selected three GCMs: BCC-CSM2-MR (BCC), IPSL-CM6A-LR (IP), and MIROC-ES2L (MI), estimated for 2030 (average for 2021--2040) and 2070 (average for 2061--2080) to offer a wide range of temperature and rainfall changes. Data editing and conversion were conducted in ArcGIS 10.2.5 The models were each run under two shared socio-economic pathways (SSPs) updated from the IPCC fifth assessment report (AR5): the first SSP was a scenario with very high greenhouse gas emissions (SSP5-8.5), and the second SSP was a stringent mitigation scenario (SSP1-2.6).
Host data were downloaded from FAOSTAT.6 Four items including watermelons; pumpkins, squash, and gourds; melons, other (including cantaloupes); and cucumbers and gherkins. The production for each country and export quantity for the present countries were averaged for the last 5 years (2015–2019).
MaxEnt Modeling
The potential geographical distribution of C. pardalina under historical and projected future climate scenarios was conducted in MaxEnt (v3.4.4)7 with presence-only data (Phillips et al., 2006). In this study, models were calibrated using 25% random test percentage, 5,000 maximum iterations, the 10 percentile training presence threshold rule, and 10 replicates under the subsample run type following Young et al. (2011) and Qin et al. (2019). Fifty-thousand randomly chosen background points in areas of C. pardalina current occurrence were selected, as recommended in MaxEnt studies that are carried out on a global scale (Rank et al., 2020). The MaxEnt “fade-by-clamping” option was used to eliminate extrapolations outside of the environmental range (Owens et al., 2013; Rank et al., 2020). ENMeval, an R package, was used to avoid overfitting and improve the performance of MaxEnt by tuning the regularization multiplier (RM) and feature types (Muscarella et al., 2014; Wei et al., 2020). The feature combinations (FC) included linear (L), quadratic (Q), product (P), threshold (T), and hinge (H). The RM values were set from 0.5 to 4 with increments of 0.5. “Checkerboard2” was used to calculate the Akaike information criterion (AICc) values. The lowest delta AICc values corresponding to RM = 0.5 and FC = LQ were applied to the final model (Supplementary Figure 1).
Model performance was evaluated by area under receiver operating characteristic (ROC) curves with (AUC) values averaged over the replicated runs. AUC values range from 0 to 1: models with an AUC value of 0.5 represent a model with discrimination ability no better than random, AUC values from 0.7 to 0.9 indicate satisfactory to moderate model performance, and values > 0.9 indicated high performance (Swets, 1988; Pearce and Ferrier, 2000; Peterson et al., 2008). It should be noted that the AUC calculated by MaxEnt can be overestimated, i.e., not present the “true” AUC, if background data used by the model are not an accurate reflection of true absences. Moreover, AUC weighs omission and commission errors equally, which should have been treated differently (Lobo et al., 2008; Yackulic et al., 2013; Zhu et al., 2017). In that case, we also used the partial ROC metric method (pROC) to evaluate model performance (Peterson et al., 2008). The pROC was calculated from NicheToolBox8, with 1,000 replicates and E = 0.05. The predictive contribution of environmental variables was estimated using Jackknife testing. Risk areas were depicted at four levels: negligible risk, low risk, medium risk, and high risk according to the MaxEnt plots and Jenks Natural Breaks Classification (Li et al., 2021). A “fixed cumulative value 5 Cloglog threshold,” robust to small samples and abnormal values (Kong et al., 2019), was also considered to divide the unsuitable and suitable areas.
Results were converted into raster files with a global map from Natural Earth9 and risk areas were calculated for Asia, Africa, North America, South America, Europe, and Oceania in ArcGIS 10.2. The host production for each country was classified and showed using ArcGIS 10.2. The host export quantity for the present countries was displayed in Origin Lab after logarithm.
Results
Bioclimatic Variables Selection
Principal component analysis and correlation analysis of 19 bioclimatic variables were conducted for variable selection. In PCA, the first four principal components explained 90.033% of the total variance with the first and fourth components mainly attributed to temperature (bio1, bio5, bio6, bio8, bio10, bio11) and the second and third attributed to precipitation (bio12, bio13, bio14, bio16, bio17). The Eigen vector with the highest explanatory value from each of the first four principal components (to avoid correlation between variables) were selected for MaxEnt modeling (Table 1). Finally, bio1 (annual mean temperature), bio8 (mean temperature of wettest quarter), and bio16 (precipitation of wettest quarter) positively related and bio14 (precipitation of driest month) negatively related were selected for modeling.
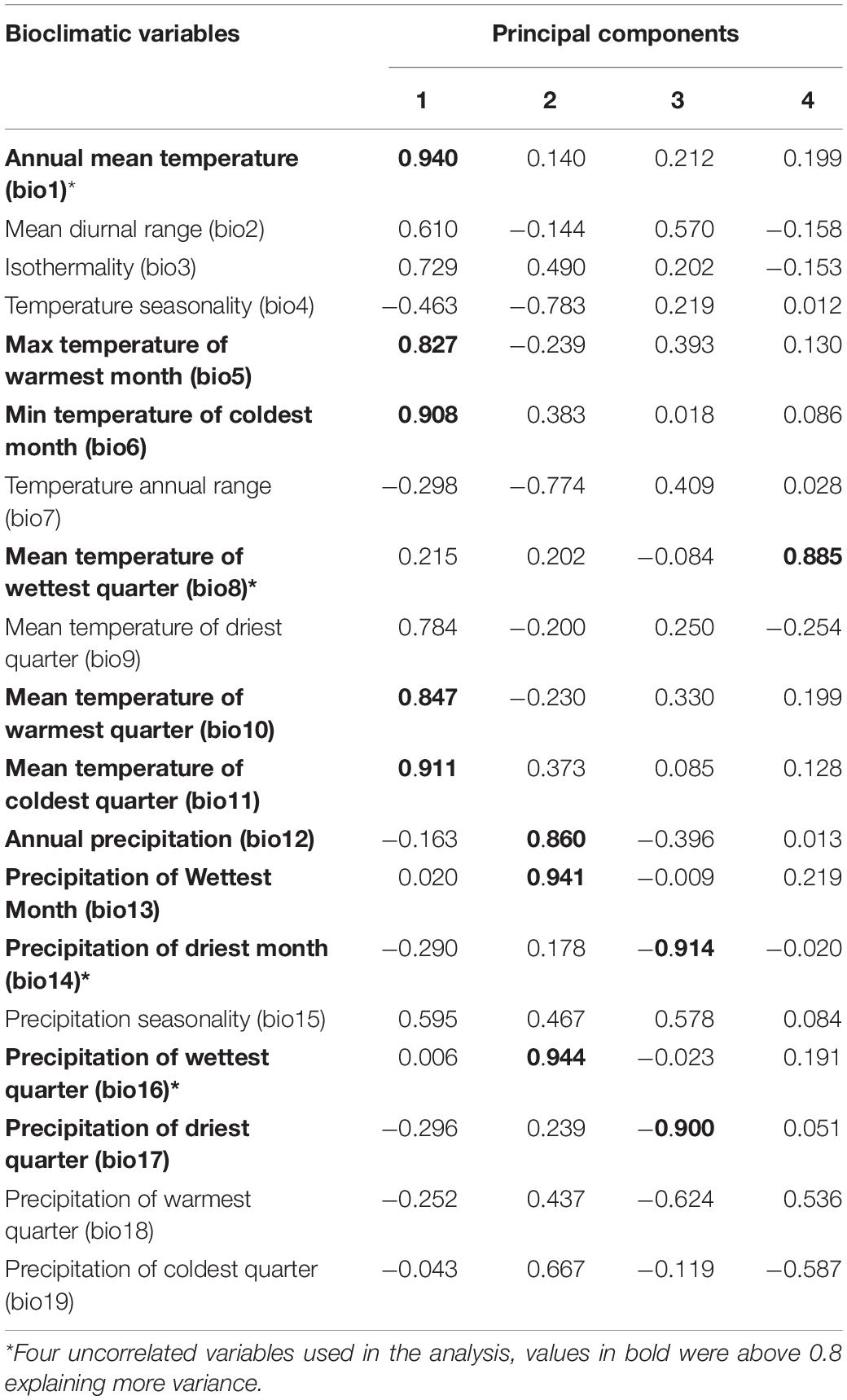
Table 1. Principal component analysis (PCA) performed on 19 bioclimatic variables to model distribution of Carpomya pardalina.
Model Performance and Variable Contributions
The averaged AUC value over 10 replicates was 0.930, and the mean value for partial AUC at 0.05 over 1000 replicates was 0.9257608 (p < 0.001), indicating a good performance of the MaxEnt models for predicting the risk area of C. pardalina (Figure 2A and Supplementary Figure 2). A “fixed cumulative value 5 Cloglog threshold” value of 0.08 was obtained. The Jackknife test indicated that the environmental variable with the highest gain when used in isolation was bio1 (annual mean temperature) which also decreased the gain the most when it was omitted (Figure 2B). Therefore, bio1 appeared to have the most useful information by itself and the most information that was not present in the other variables. The estimation of relative contributions of the selected bioclimatic variables to the MaxEnt model were 65.5% (bio1, annual mean temperature), 25.5% (bio8, mean temperature of wettest quarter), 6.5% (bio14, precipitation of driest month), and 2.5% (bio16, precipitation of wettest quarter).
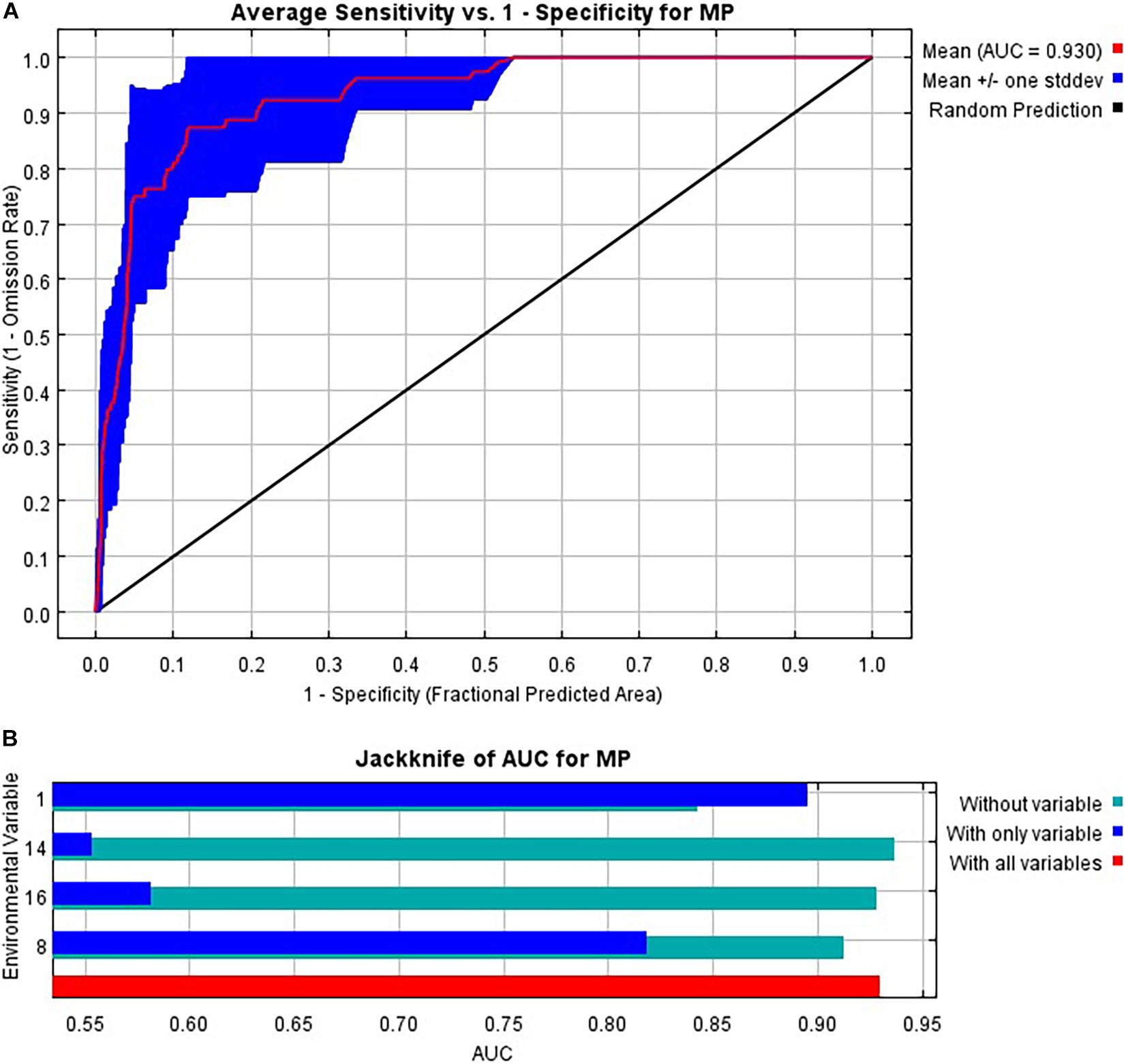
Figure 2. MaxEnt model performance and variable contributions for a predictive model of the global distribution of Carpomya pardalina. (A) Receiver operating characteristic (ROC) curve averaged over the replicate runs; (B) Jackknife test of variable importance averaged over the replicate runs.
Potential Geographical Distribution Under Historical Climate Including Host Availability
The MaxEnt-predicted potential geographical distribution of C. pardalina under near-current climate conditions (1970–2000) is shown in Figure 1A. We categorized risk areas into four levels: negligible risk (0.00–0.08), low risk (0.08–0.23), medium risk (0.23–0.62), and high risk (0.62–1.00) considering the MaxEnt plots of this species (Supplementary Figure 3), Jenks Natural Breaks Classification, and “Fixed cumulative value 5 Cloglog threshold.” Under near-current climate conditions, it was predicted that C. pardalina could potentially establish in west Asia, central Asia, and most parts of China and neighboring countries. European countries, most of Africa except the western part, Congo basin, and the Sahara, southern Australia and New Zealand, the United States, Mexico, and the southern part of South America were also suitable for the species (Figure 1A). Among these areas, central Asia, the coastal Mediterranean, western China, southern Australia, western United States, Chile, and southern Argentina exhibited a relatively high risk for C. pardalina establishment.
The extent of the land area that was climatically suitable for C. pardalina under near-current climate conditions was quantified for each continent (Table 2). A total of 47.64% of the world’s land mass (excluding Antarctica), or 6371.87 × 104 km2, was climatically suitable. Asia contributed most of the risk area, 31.36% of its total land area, followed by Africa (21.88%), Europe (17.35%), North America (16.40%), South America (8.55%), and Oceania (4.46%) (Table 2).
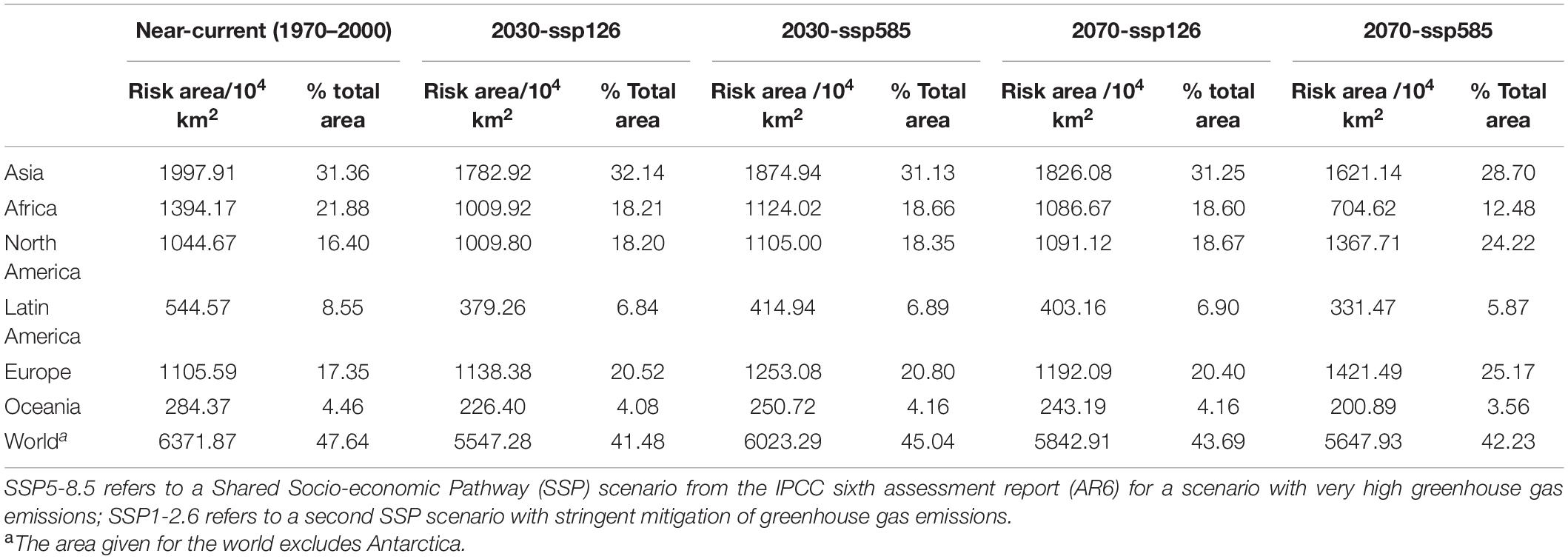
Table 2. Projected risk area globally for Carpomya pardalina under near-current and future (2030 and 2070) climate scenarios expressed as an area (104 km2) and as a percentage of the total area per continent, as predicted by MaxEnt modeling.
The average host production (2015–2019) including watermelons; pumpkins, squash, and gourds; melons, other (including cantaloupes); and cucumbers and gherkins for C. pardalina from 159 countries are shown on the map (Figure 1B). Almost all the areas predicted to be at risk were also able to offer hosts for C. pardalina. The host production was above one million tons in present countries Turkey, India, Russia, Uzbekistan, and Iran and absent countries China, Vietnam, and Brazil. The average host export quantity (2015–2019) from present countries after logarithm is displayed in Figure 1C. Among the 20 present countries of C. pardalina, the host export quantity from Iran was 0.78 million tons, followed by Turkey, Jordan, Kazakhstan, and Uzbekistan, where the export quantity was above 0.05 million tons. Among the four items, watermelon export quantity was the highest, up to 0.65 million tons from the 20 countries.
Climate Change Impact on the Potential Geographical Distribution
The potential geographical distribution maps of C. pardalina under a range of possible future climate scenarios for 2030 and 2070 are displayed in Figure 3. The potential range of C. pardalina was predicted to increase in America and Europe, and decrease in Asia, Africa, and Oceania (Figure 3). In Asia, the suitable area expanded in southern and central Russia, but reduced in south China, south Asia, and southeast Asia. In Africa, the risk area decreased notably in southern Africa and the risk severity also declined in northern Africa. In North America, the risk area increased in north-eastern Canada; while in South America at-risk areas decreased in Brazil and Argentina. In Europe, risk areas and threat changed minimally. In Oceania, the risk area retracted to southern temperate Australia (Figure 3 and Table 2).
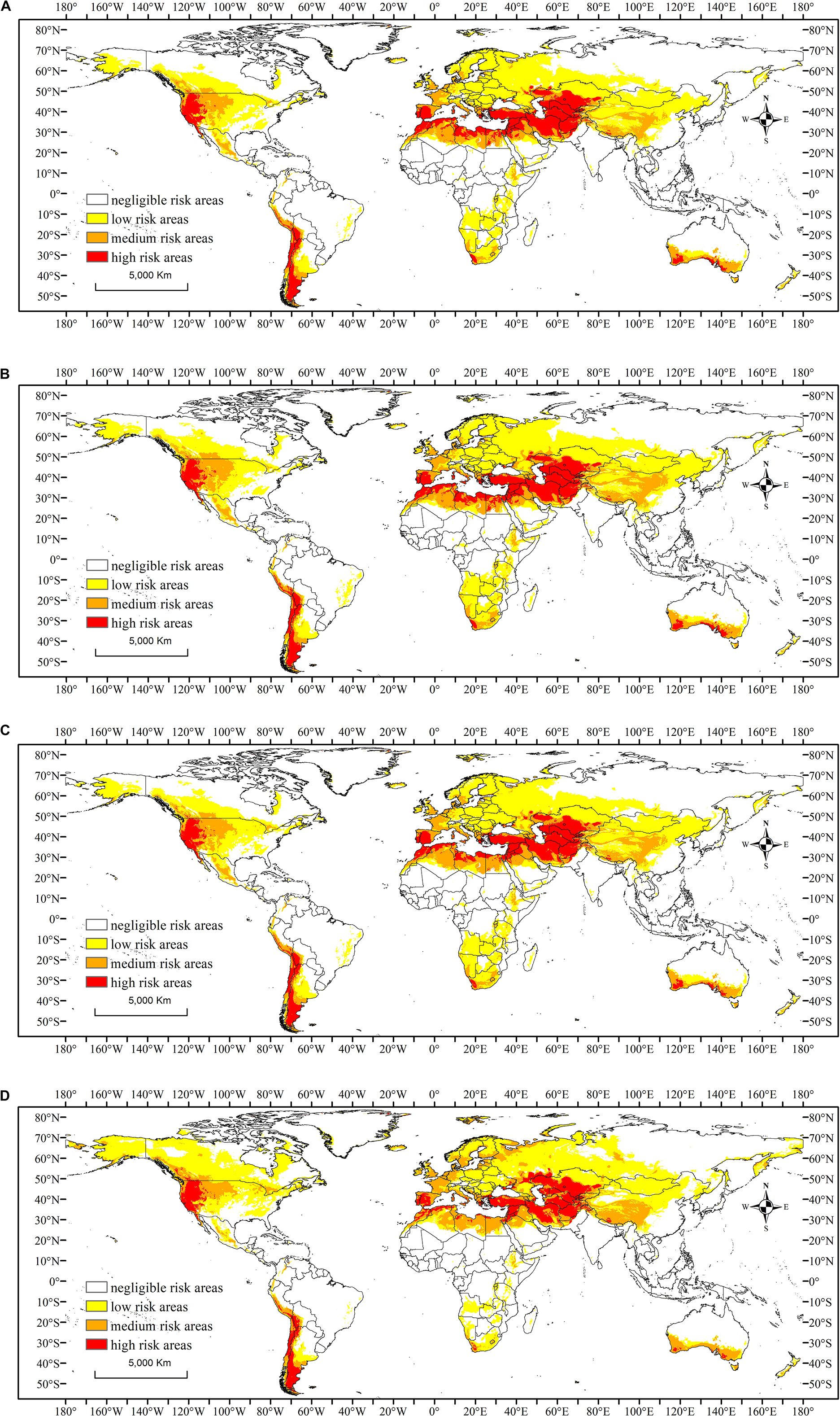
Figure 3. Potential geographical distribution of Carpomya pardalina in 2030 and 2070 under future climate conditions as predicted by MaxEnt modeling. Mean predicted results are from three global climate models [BCC-CSM2-MR (BCC), IPSL-CM6A-LR (IP), MIROC-ES2L (MI)] which were modeled under (A) 2030-SSP126; (B) 2030-SSP585; (C) 2070-SSP126; and (D) 2070-SSP585. White indicates negligible risk areas, yellow indicates low risk areas, orange indicates medium risk areas, and red indicates high risk areas. SSP5-8.5 refers to a Shared Socio-economic Pathway (SSP) scenario from the IPCC sixth assessment report (AR6) for a scenario with very high greenhouse gas emissions; SSP1-2.6 refers to a second SSP scenario with stringent mitigation of greenhouse gas emissions.
The total global suitable land mass decreased by 824.59 × 104 km2, or 0.06% under scenario SSP126 (stringent management of greenhouse gas emissions) and 348.58 × 104 km2, or 0.03% under scenario SSP585 (high greenhouse gas emissions) in 2030; and decreased by 528.96 × 104 km2, or 0.04% under scenario SSP126 and 723.94 × 104 km2, or 0.05% of land area under scenario SSP585 in 2070 (Table 2).
Discussion
While currently restricted to central Asia and far eastern Europe, MaxEnt modeling predicts that C. pardalina could establish widely around the globe, both in current and future climates. While invasion pathways for C. pardalina from central Asia to the Americas and Oceania are not obvious, there are very obvious and direct invasion pathways for the fly into Europe and China with host availability.
Within Europe, which imports melons from countries where the fly is already established (Toyzhigitova et al., 2019), most countries are at risk and Portugal, Spain, France, Italy, and England are at high or medium risk. Melons and other host plants of C. pardalina are widely grown in the EPPO region, in particular in southern Europe and around the Mediterranean Basin which were predicted to be risk areas (EPPO, 2013; Figure 1B). The risk area increased significantly in Russia under climate change which was also a big melon producer and exporter (Figures 1, 3). Carpomya pardalina was formerly, but is not currently, on the EPPO Alert list. Our climate modeling, and the possible transportation of infected melons through trade (Talhuk, 1969; Abdullah et al., 2007), suggests that this fly should be of much greater priority to Europe than is currently the case.
Within Asia, most of China is suitable for this species, while western China is predicted as a medium risk area. Carpomya pardalina is currently absent in China and is a listed quarantine pest. The General Administration of Customs in China has published requests to import melons from Kyrgyzstan in 2018 and Uzbekistan in 2019, for which C. pardalina was on the quarantine pest list of concern. Based on its modeled ability to establish in China and host availability (Figure 1), this quarantine concern is technically justified.
With respect to Chinese domestic quarantine, Xinjiang Province should be of particular concern. Located in western China, Xinjiang has borders with eight countries, including Kazakhstan, Kyrgyzstan, Tajikistan, Afghanistan, Pakistan, and India, all of which are entirely or partially within C. pardalina’s current distribution range. Xinjiang acts as a trade center between China and Central Asia, West Asia, and Europe, and due to its special geographical position suffers extensively from damage caused by invasive species. Ninety-five invasive species were reported from Xinjiang during the last 60 years, with a frequency of 2.88 new invasive species per year since 1990 (Guo et al., 2017). The “one belt, one road” development strategy offers more opportunity for invasive species as the number of China-Europe freight trains entering and exiting Khorgos Port, Xinjiang, already numbering > 4,500 in 2020, increases. With respect to the current study, Xinjiang province is famous for melons, which are the hosts of C. pardalina. Therefore, the surveillance and early warning of C. pardalina should be strengthened in Xinjiang Province to stop the likely entry and subsequent spread of the fly into China.
Fruit flies are highly invasive organisms and very significant effort goes into risk analysis, quarantine, and phytosanitary treatments in order to minimize their spread (Godefroid et al., 2015; Qin et al., 2015; Hill et al., 2016; Fang et al., 2019). Nevertheless, as demonstrated through the scientific literature and the priorities of national and regional plant protection organizations (as illustrated on their websites), nearly all attention is paid to just a handful of the 250 + tephritids which are known to have pest status. For just one of these lesser flies, C. pardalina, our study predicts that risk areas climatically suitable for the fly occur across the globe both currently and under future climate change scenarios with host availability.
Annual mean temperature (bio1) is the most important climatic variable contributing to the current global distribution of C. pardalina. Temperature variables contributed more than precipitation indicating temperature may be the driving force for this species. CMIP6 models used in this study project a “well-below 2°C” temperature change under the SSP1-2.6 scenario and a mean warming of 5.0°C this century (Eyring et al., 2016; Hausfather, 2019). Unlike tropical tephritid fruit flies, C. pardalina was predicted to not be suitable in southeast Asia and suitable in western Siberia under climate change, suggesting that this species may prefer cool conditions. Developmental temperature and survival threshold for the life cycle of C. pardalina needs to be carried out in order to better understand the distribution pattern of this species.
In addition, climatic factors and host availability were considered in the current study. Geographical factors, land use, human factors, and biotic factors will also have influences on the distribution of species (Chen et al., 2020; Liu et al., 2020). MaxEnt is popular among species distribution models (SDMs) to predict suitable habitats for species. There are also regression, machine learning, and classification methods used in other SDMs; model performance can vary significantly across different algorithms. Ensemble modeling will be an important direction for future research (Thuiller et al., 2009; Zhu et al., 2020).
Conclusion
In conclusion, our MaxEnt modeling highlights particularly that Western China, Russia, and other European countries should pay attention to this currently lesser-known melon fly and the melons exported from the present countries. While currently restricted in its geographic distribution, which likely explains its low international recognition, already existing and growing trade pathways could easily move this fly via melon exports east into China or west into Europe. Besides producing specific recommendations for C. pardalina, this study should also be used to alert quarantine agencies of the likely threats posed by other less-known fruit fly species.
Data Availability Statement
The raw data supporting the conclusions of this article will be made available by the authors, without undue reservation.
Author Contributions
YQ and ZL conceived and designed the research. YQ and YZ analyzed the data and wrote the first draft. YQ, YZ, AC, ZZ, and ZL discussed the idea and reviewed the draft. All authors revised the manuscript and approved the final version.
Funding
This work was supported by the International Collaboration Key Program of National Key Research and Development Project (No. 2018YFE0108700).
Conflict of Interest
The authors declare that the research was conducted in the absence of any commercial or financial relationships that could be construed as a potential conflict of interest.
Publisher’s Note
All claims expressed in this article are solely those of the authors and do not necessarily represent those of their affiliated organizations, or those of the publisher, the editors and the reviewers. Any product that may be evaluated in this article, or claim that may be made by its manufacturer, is not guaranteed or endorsed by the publisher.
Acknowledgments
We would like to thank all members of the Plant Quarantine and Invasion Biology Laboratory of China Agricultural University (CAUPQL). We acknowledge the World Climate Research Programme, which, through its Working Group on Coupled Modeling, coordinated and promoted CMIP6. We thank the climate modeling groups for producing and making available their model output, the Earth System Grid Federation (ESGF) for archiving the data and providing access, and the multiple funding agencies who support CMIP6 and ESGF.
Supplementary Material
The Supplementary Material for this article can be found online at: https://www.frontiersin.org/articles/10.3389/fevo.2021.724441/full#supplementary-material
Footnotes
- ^ https://gd.eppo.int/taxon/CARYPA/distribution
- ^ http://worldclim.org/
- ^ https://www.ibm.com/support/docview.wss?uid=swg21646821
- ^ https://www.wcrp-climate.org/wgcm-cmip/wgcm-cmip6
- ^ http://www.esri.com/sofware/arcgis/arcgis-for-desktop
- ^ http://www.fao.org/faostat/zh/#data/QC
- ^ https://biodiversityinformatics.amnh.org/open_source/maxent/
- ^ http://shiny.conabio.gob.mx:3838/nichetoolb2/
- ^ https://www.naturalearthdata.com/
References
Abdullah, K., Latif, A., Khan, S. M., and Khan, M. A. (2007). Field test of the bait spray on periphery of host plants for the control of the fruit fly, Myiopardalis Pardalina Bigot (Tephritidae: Diptera). Pak. Entomol. 29, 91–94.
Akkaya, A., and Uygun, N. (1999). Faunistic studies on harmful and beneficial insects on cucurbit vegetables in the southeastern Anatolian region of turkey. Acta Hortic. 67, 335–340. doi: 10.17660/ActaHortic.1999.492.46
Baker, R., Gilioli, G., Behring, C., Candiani, D., Gogin, A., Kaluski, T., et al. (2019). Bactrocera dorsalis Pest Report to Support Ranking of EU Candidate Priority Pests. Report No. EN-1641. Parma: European Food Safety Authority.
Baris, A., and Cobanoglu, S. (2013). Investigation on the biology of melon fly Myiopardalis pardalina (Bigot, 1891) (Diptera: Tephritidae) in Ankara province. Turk. Entomol. Derg. 37, 293–304.
Baris, A., Çobanoğlu, S., and Çavuşoğlu, Ş (2016). Determination of changes in tastes of İpsala and Kırkağaç melons against melon fly [Myiopardalis pardalina (Bigot, 1891) (Diptera: Tephritidae)]. Derim 33, 47–56. doi: 10.16882/derim.2016.04532
Biber-Freudenberger, L., Ziemacki, J., Tonnang, H. E. Z., and Borgemeister, C. (2016). Future risks of pest species under changing climatic conditions. PLoS One 11:e0153237. doi: 10.1371/journal.pone.0153237
Biosecurity Australia (2009). Report of the Assessment of Northern China’s Fruit Fly Pest Free Areas: Hebei, Shandong and Xinjiang. PFA Assessment Report 20090306. Canberra: Biosecurity Australia.
Bradshaw, C. J. A., Leroy, B., Céline, B., Roiz, D., Céline, A., Fournier, A., et al. (2016). Massive yet grossly underestimated global costs of invasive insects. Nat. Commun. 7:12986. doi: 10.1038/ncomms12986
Chen, S., Ding, F. Y., Hao, M. M., and Jiang, D. (2020). Mapping the potential global distribution of red imported fire ant (solenopsis invicta buren) based on a machine learning method. Sustainability 12:10182. doi: 10.3390/su122310182
Clarke, A. R. (2019). Biology and Management of Bactrocera and Related Fruit Flies. Wallingford: CAB International.
Cook, D. C., Thomas, M. B., Cunningham, S. A., Anderson, D. L., and De Barro, P. J. (2007). Predicting the economic impact of an invasive species on an ecosystem service. Ecol. Appl. 17, 1832–1840. doi: 10.1890/06-1632.1
Cornelissen, B., Neumann, P., and Schweiger, O. (2019). Global warming promotes biological invasion of a honey bee pest. Glob. Chang. Biol. 25, 3642–3655. doi: 10.1111/gcb.14791
Early, R., Bradley, B. A., Dukes, J. S., Lawler, J. J., Olden, J. D., Blumenthal, D. M., et al. (2016). Global threats from invasive alien species in the twenty-first century and national response capacities. Nat. Commun. 7:12485. doi: 10.1038/ncomms12485
Elith, J., Phillips, S. J., Hastie, T., Dudík, M., Chee, Y. E., and Yates, C. J. (2011). A statistical explanation of Maxent for ecologists. Divers. Distrib. 17, 43–57. doi: 10.1111/j.1472-4642.2010.00725.x
EPPO (2013). Outbreaks of Myiopardalis pardalina (Baluchistan melon fly) in Central Asia: Addition to the EPPO Alert List. Available at: https://gd.eppo.int/reporting/article-2590
Eyring, V., Bony, S., Meehl, G. A., Senior, C. A., Stevens, B., Stouffer, R. J., et al. (2016). Overview of the Coupled Model Intercomparison Project Phase 6 (CMIP6) experimental design and organization. Geosci. Model. Dev. 9, 1937–1958. doi: 10.5194/gmd-9-1937-2016
Fang, Y., Kang, F., Zhan, G. P., Ma, C., Li, Y. G., Wang, L., et al. (2019). The effects of a cold disinfestation on Bactrocera dorsalis survival and navel orange quality. Insects 10:452. doi: 10.3390/insects10120452
Fick, S. E., and Hijmans, R. J. (2017). WorldClim 2: new 1km spatial resolution climate surfaces for global land areas. Int. J. Climatol. 37, 4302–4315. doi: 10.1002/joc.5086
Godefroid, M., Cruaud, A., Rossi, J. P., and Rasplus, J. Y. (2015). Assessing the risk of invasion by tephritid fruit flies: intraspecific divergence matters. PLoS One 10:e0135209. doi: 10.1371/journal.pone.0135209
Guisan, A., Tingley, R., Baumgartner, J. B., Naujokaitis-Lewis, I., Sutcliffe, P. R., Tulloch, A., et al. (2013). Predicting species distributions for conservation decisions. Ecol. Lett. 16, 1424–1435.
Guo, W. C., Zhang, X. L., Wu, W., Zhang, W., Fu, K. Y., Tuerxun, A. H. M. T., et al. (2017). Occurrence status, trend and research progress of alien invasive organisms in agriculture and forestry in Xinjiang. J. Biosaf. 26, 1–11.
Hausfather, Z. (2019). CMIP6: The Next Generation of Climate Models Explained. Available online at: https://www.carbonbrief.org/ (accessed December 2, 2019).
Heikkinen, R. K., Luoto, M., Araujo, M. B., Virkkala, R., Thuiller, W., and Sykes, M. T. (2006). Methods and uncertainties in bioclimatic envelope modelling under climate change. Prog. Phys. Geogr. 30, 1–27. doi: 10.1177/0309133306071957
Hernandez, P. A., Graham, C. H., Master, L. L., and Albert, D. L. (2006). The effect of sample size and species characteristics on performance of different species distribution modeling methods. Ecography 5, 773–785. doi: 10.1111/j.0906-7590.2006.04700.x
Hill, M. P., Bertelsmeier, C., Clusella-Trullas, S., Garnas, J., Robertson, M. P., and Terblanche, J. S. (2016). Predicted decrease in global climate suitability masks regional complexity of invasive fruit fly species response to climate change. Biol. Invasions 18, 1105–1119. doi: 10.1007/s10530-016-1078-5
Hulme, P. (2017). Climate change and biological invasions: evidence, expectations, and response options. Biol. Rev. 92, 1297–1313. doi: 10.1111/brv.12282
Hulme, P. E. (2009). Trade, transport and trouble: managing invasive species pathways in an era of globalization. J Appl. Ecol. 46, 10–18. doi: 10.1111/j.1365-2664.2008.01600.x
Kong, W. Y., Li, X. H., and Zou, H. M. (2019). Optimizing MaxEnt model in the prediction of species distribution. Chin. J. of Appl. Ecol. 30, 2116–2128. doi: 10.13287/j.1001-9332.201906.029
Li, Y., Wan, Y., Lin, W., Ernstsons, A. S., and Gao, L. (2021). Estimating potential distribution of sweetgum pest acanthotomicus suncei and potential economic losses in nursery stock and urban areas in China. Insects 12:155. doi: 10.3390/insects12020155
Liang, G. Q. (2011). Fruit flies (Diptera: Tephritidae: Dacinae: Trypetinae: Tephritinae). Beijing: China Agricultural Press.
Liu, T., Wang, J., Hu, X., and Feng, J. (2020). Land-use change drives present and future distributions of fall armyworm, Spodoptera frugiperda (JE Smith)(Lepidoptera: Noctuidae). Sci. Total Environ. 706:135872. doi: 10.1016/j.scitotenv.2019.135872
Lobo, J. M., Jiménez-Valverde, A., and Real, R. (2008). AUC: a misleading measure of the performance of predictive distribution models. Glob. Ecol. Biogeogr. 17, 145–151. doi: 10.1111/j.1466-8238.2007.00358.x
Mack, R. N., Simberloff, D., Lonsdale, W. M., Evans, H., Clout, M., and Bazzaz, F. A. (2000). Biotic invasions: causes, epidemiology, global consequences, and control. Ecol. Appl 10, 689–710.
McGeoch, M. A., Butchart, S. H., Spear, D., Marais, E., Kleynhans, E. J., Symes, A., et al. (2010). Global indicators of biological invasion: species numbers, biodiversity impact and policy responses. Divers. Distrib. 16, 95–108. doi: 10.1111/j.1472-4642.2009.00633.x
Merow, C., Smith, M. J., and Silander, J. A. (2013). A practical guide to MaxEnt for modeling species’ distributions: what it does, and why inputs and settings matter. Ecography 36, 1058–1069. doi: 10.1111/j.1600-0587.2013.07872.x
Muscarella, R., Galante, P. J., Soley-Guardia, M., Boria, R. A., Kass, J. M., Uriarte, M., et al. (2014). ENMeval: an R package for conducting spatially independent evaluations and estimating optimal model complexity forMaxentecological niche models. Methods Ecol. Evol. 5, 1198–1205. doi: 10.1111/2041-210X.12261
Owens, H. L., Campbell, L. P., Dornak, L. L., Saupe, E. E., Barve, N., Soberón, J., et al. (2013). Constraints on interpretation of ecological niche models by limited environmental ranges on calibration areas. Ecol. Modell. 263, 10–18. doi: 10.1016/j.ecolmodel.2013.04.011
Papadopoulos, N. (2014). “Fruit fly invasion: historical, biological, economic aspects and management,” in Trapping and the Detection, Control, and Regulation of Tephritid Fruit Flies, eds T. Shelly, N. Epsky, E. B. Jang, J. Reyes-Flores, and R. Vargas (Dordrecht: Springer), 219–252.
Pavlov, A. V. (2012). On an incident of importation of the fly Carpomyia (Myiopardalis) pardalina Bigot, 1891 (Diptera: Tephritidae) into Vladimir Province (Centre of European Russia). Eversmannia 92, 29–30.
Pearce, J., and Ferrier, S. (2000). An evaluation of alternative algorithms for fitting species distribution models using logistic regression. Ecol. Modell. 128, 127–147. doi: 10.1016/S0304-3800(99)00227-6
Peterson, A. T., Papeş, M., and Soberón, J. (2008). Rethinking receiver operating characteristic analysis applications in ecological niche modeling. Ecol. Modell. 213, 63–72. doi: 10.1016/j.ecolmodel.2007.11.008
Phillips, S. J., Anderson, R. P., and Schapire, R. E. (2006). Maximum entropy modelling of species geographic distributions. Ecol. Modell. 190, 231–259. doi: 10.1016/j.ecolmodel.2005.03.026
Phillips, S. J., Dudik, M., and Schapire, R. E. (2004). “A maximum entropy approach to species distribution modelling,” in Proceedings of the 21st International Conference on Machine Learning (Banff: Association for Computing Machinery).
Qin, Y. J., Paini, D. R., Wang, C., Fang, Y., and Li, Z. H. (2015). Global establishment risk of economically important fruit fly species (Tephritidae). PLoS One 10:e0116424. doi: 10.1371/journal.pone.0116424
Qin, Y. J., Wang, C., Zhao, Z. H., Pan, X. B., and Li, Z. H. (2019). Climate change impacts on the global potential geographical distribution of the agricultural invasive pest, Bactrocera dorsalis (Hendel) (Diptera: Tephritidae). Clim. Chang. 155, 145–156. doi: 10.1007/s10584-019-02460-3
Rank, A., Ramos, R. S., Silva, R. S. D., Soares, J. R. S., Picanço, M. C., and Fidelis, E. G. (2020). Risk of the introduction of lobesia botrana in suitable areas for vitis vinifera. J. Pest. Sci. 93, 1167–1179. doi: 10.1007/s10340-020-01246-2
Santana, J. P. A., Kumar, L., Da Silva, R. S., Pereira, J. L., and Picanço, M. C. (2019). Assessing the impact of climate change on the worldwide distribution of Dalbulus maidis (DeLong) using MaxEnt. Pest. Manag. Sci. 75, 2706–2715. doi: 10.1002/ps.5379
Stanaway, M. A., Zalucki, M., Gillespie, P. S., Rodriguez, C. M., and Maynard, G. V. (2001). Pest risk assessment of insects in sea cargo containers. Aust. J. Entomol. 40, 180–192. doi: 10.1046/j.1440-6055.2001.00215.x
Stonehouse, J., Sadeed, S. M., Harvey, A., and Haiderzada, G. S. (2006). “Myiopardalis pardalina in Afghanistan,” in Proceedings of the 7th International Symposium on Fruit Flies of Economic Importance, Salvador.
Swets, J. A. (1988). Measuring the accuracy of diagnostic systems. Science 240:1285. doi: 10.1126/science.3287615
Talhuk, A. M. S. (1969). Myiopardalis pardalina Bigot: Insect and Mites Injurious to Crops om Middle Eastern Countries. Hamburg: Verlag Paul Paregy, 217–218.
Thuiller, W., Lafourcade, B., Engler, R., and Araújo, M. B. (2009). BIOMOD—A platform for ensemble forecasting of species distributions. Ecography 32, 369–373. doi: 10.1111/j.1600-0587.2008.05742.x
Toyzhigitova, B., Yskak, S., Dinasilov, A., Kochinski, P., Ershin, Z. R., Raimbekova, B., et al. (2017). Quarantine protective measures against the melon flies (Myiopardalis Pardalina Big.) in Kazakhstan. J. Biol. Sci. 17, 278–284. doi: 10.3844/ojbsci.2017.278.284
Toyzhigitova, B., Yskak, S., Łozowicka, B., Kaczyński, P., Dinasilov, A., Zhunisbay, R., et al. (2019). Biological and chemical protection of melon crops against Myiopardalis pardalina Bigot. J. Plant Dis. Protect 126, 359–366. doi: 10.1007/s41348-019-00231-x
Wang, C., Hawthorne, D., Qin, Y. J., Pan, X. B., Li, Z. H., and Zhu, S. F. (2017). Impact of climate and host availability on future distribution of Colorado potato beetle. Sci. Rep. 7:4489. doi: 10.1038/s41598-017-04607-7
Wei, J., Peng, L., He, Z., Lu, Y., and Wang, F. (2020). Potential distribution of two invasive pineapple pests under climate change. Pest. Manag. Sci. 76, 1652–1663. doi: 10.1002/ps.5684
White, I. M., and Elson-Harris, M. M. (1992). Fruit Flies of Economic Significance: Their Identification and Bionomics. Wallingford: CABI Publishing CAB Interregional.
Yackulic, C. B., Chandler, R., Zipkin, E. F., Royle, J. A., Nichols, J. D., Grant, E. H. C., et al. (2013). Presence-only modelling using MAXENT: when can we trust the inferences? Meth. Ecol. Evol. 4, 236–243. doi: 10.1111/2041-210x.12004
Young, N., Carter, L., and Evangelista, P. A. (2011). MaxEnt Model v3.3.3e Tutorial (ArcGIS v10). Fort Collins, CO: Natural Resource Ecology Laboratory at Colorado State University.
Zhu, G., Illan, J. G., Looney, C., and Crowder, D. W. (2020). Assessing the ecological niche and invasion potential of the Asian giant hornet. Proc. Natl. Acad. Sci. U.S.A. 117, 24646–24648. doi: 10.1073/pnas.2011441117
Keywords: Carpomya pardalina, MaxEnt, potential geographical distribution, climate change, host availability management
Citation: Qin Y, Zhang Y, Clarke AR, Zhao Z and Li Z (2021) Including Host Availability and Climate Change Impacts on the Global Risk Area of Carpomya pardalina (Diptera: Tephritidae). Front. Ecol. Evol. 9:724441. doi: 10.3389/fevo.2021.724441
Received: 13 June 2021; Accepted: 21 September 2021;
Published: 08 October 2021.
Edited by:
Bradley S. Case, Auckland University of Technology, New ZealandReviewed by:
Pairot Pramual, Mahasarakham University, ThailandJiufeng Wei, Shanxi Agricultural University, China
Copyright © 2021 Qin, Zhang, Clarke, Zhao and Li. This is an open-access article distributed under the terms of the Creative Commons Attribution License (CC BY). The use, distribution or reproduction in other forums is permitted, provided the original author(s) and the copyright owner(s) are credited and that the original publication in this journal is cited, in accordance with accepted academic practice. No use, distribution or reproduction is permitted which does not comply with these terms.
*Correspondence: Zhihong Li, lizh@cau.edu.cn
†These authors have contributed equally to this work