- 1Department of Ecology and Evolutionary Biology, University of California, Los Angeles, Los Angeles, CA, United States
- 2Department of Biology, Stanford University, Stanford, CA, United States
A small number of extraordinary “Major Evolutionary Transitions” (METs) have attracted attention among biologists. They comprise novel forms of individuality and information, and are defined in relation to organismal complexity, irrespective of broader ecosystem-level effects. This divorce between evolutionary and ecological consequences qualifies unicellular eukaryotes, for example, as a MET although they alone failed to significantly alter ecosystems. Additionally, this definition excludes revolutionary innovations not fitting into either MET type (e.g., photosynthesis). We recombine evolution with ecology to explore how and why entire ecosystems were newly created or radically altered – as Major System Transitions (MSTs). In doing so, we highlight important morphological adaptations that spread through populations because of their immediate, direct-fitness advantages for individuals. These are Major Competitive Transitions, or MCTs. We argue that often multiple METs and MCTs must be present to produce MSTs. For example, sexually-reproducing, multicellular eukaryotes (METs) with anisogamy and exoskeletons (MCTs) significantly altered ecosystems during the Cambrian. Therefore, we introduce the concepts of Facilitating Evolutionary Transitions (FETs) and Catalysts as key events or agents that are insufficient themselves to set a MST into motion, but are essential parts of synergies that do. We further elucidate the role of information in MSTs as transitions across five levels: (I) Encoded; (II) Epigenomic; (III) Learned; (IV) Inscribed; and (V) Dark Information. The latter is ‘authored’ by abiotic entities rather than biological organisms. Level IV has arguably allowed humans to produce a MST, and V perhaps makes us a FET for a future transition that melds biotic and abiotic life into one entity. Understanding the interactive processes involved in past major transitions will illuminate both current events and the surprising possibilities that abiotically-created information may produce.
Introduction
All adaptations, by definition, increase the fitness of organisms in their environments, but few merit special consideration as extraordinary (Stebbins, 1969; Bonner, 1974; Buss, 1987; Queller, 2000; Bourke, 2011a; Calcott and Sterelny, 2011; Szathmáry, 2015; West et al., 2015). Maynard Smith and Szathmáry in an influential book (1995) and paper (Szathmáry and Maynard Smith, 1995) listed eight as Major Evolutionary Transitions (METs) (Table 1). Bourke (2011a) and Szathmáry (2015) amended this list, with the latter explicitly stating that the MET concept is not in relation to “ecosystem complexity, but [to] the complexity of the players … acting in the ecological theater” (p. 10,104). Overall, across these contrasting lists (Table 1) there are two broad classes of adaptations that qualify as gains in “organismal complexity” and constitute METs. One is ‘Fusions,’ where independently reproducing entities combine into higher, integrated levels of obligate reproductive cooperation (Buss, 1987; Michod, 1996; Szathmáry, 2015; West et al., 2015). Factors that could both favor and stabilize formation of Fusions include selective advantages of division of labor and mutual dependence (West et al., 2015), the maximization of inclusive fitness (Bourke, 2011a; West et al., 2015), and the ability to punish cheaters (Ågren et al., 2019). The other class is ‘Information Leaps’: novel forms of information storage or transmittal across individuals, ranging from genes to symbolic writing (Jablonka and Lamb, 2006).
Limiting METs to Fusions or Information Leaps, regardless of ecosystem-level impacts (Szathmáry, 2015), excludes a number of other possibly “major” events. Although such designations can vary subjectively, considering a wider array of events can illuminate intriguing commonalities, dependencies, and unanswered questions that contribute to understanding the history of life on Earth. In this paper, we: (1) Introduce a more inclusive set of terminology to improve future discourse on major transitions (Figure 1), and (2) explore how major ecosystem transitions arise within broad frameworks that can include multiple Fusions and Information Leaps, morphological innovations, catalytic actors and events, and variation in the selective processes involved.
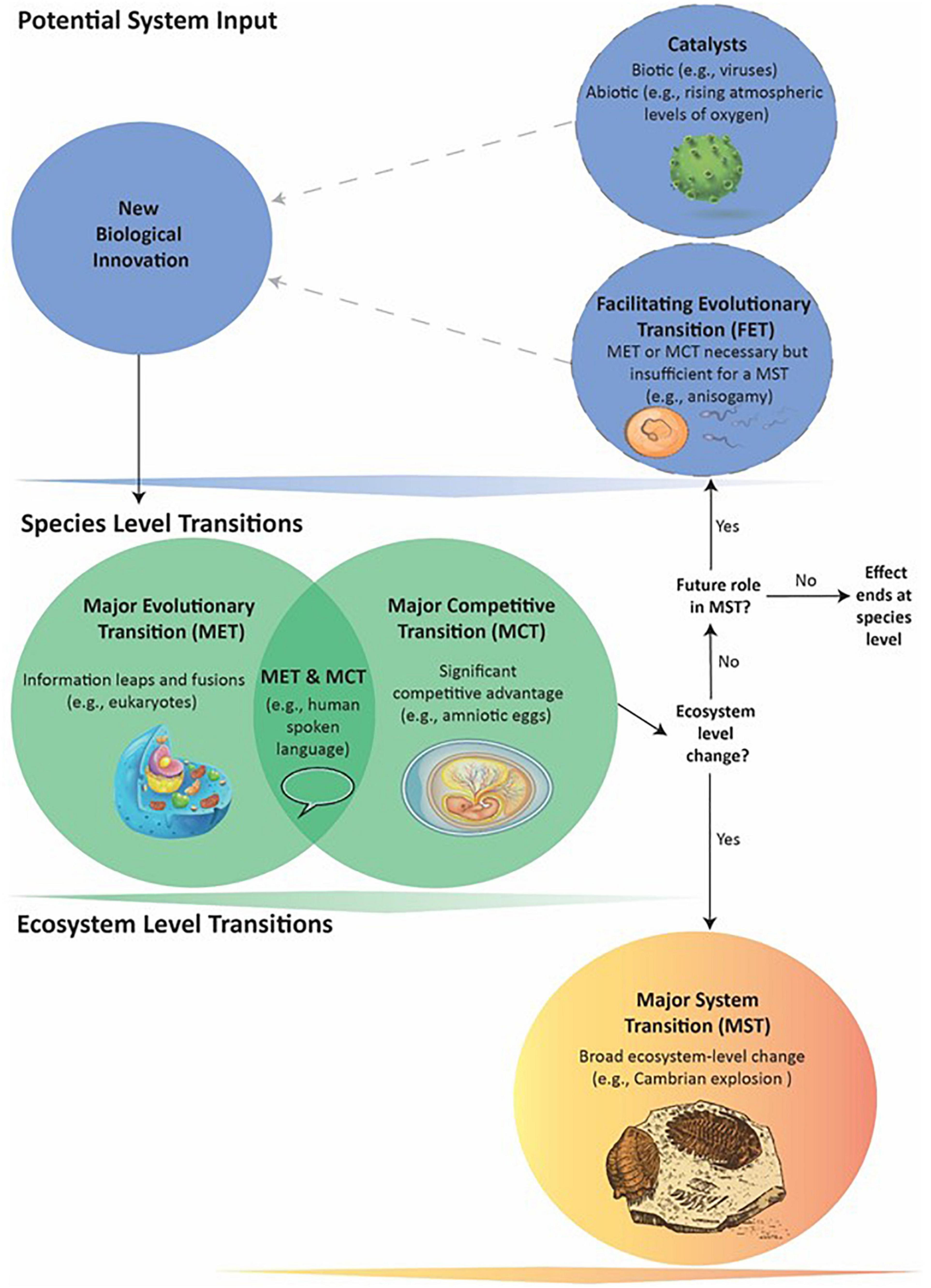
Figure 1. General schematic of how a series of events can lead to a Major System Transition (MST). Biological innovations are system inputs that may result in Major Evolutionary or Competitive Transitions (METs and MCTs – see text for definitions). An innovation can become a MET or MCT: (1) through its own direct effects; (2) with requiring a previous MET or MCT that acts as a Facilitating Evolutionary Transition (FET); or (3) as catalyzed by biotic agents or abiotic events. The dotted lines indicate FETs and catalysts may or may not be present. An innovation producing a MET, MCT, or both occurs at the Species level and its downstream effects can directly lead to MSTs: large-scale, ecosystem-level transformations affecting many species. Not all individual METs and MCTs, however, appear capable of causing MSTs. Nevertheless, they can still potentially act as FETs that in combination with later METs or MCTs do lead to a MST. In such cases, the evolutionary process cycles through more than one Species-level transition before the MST.
Major Events in the History of Life
We retain Szathmáry’s (2015) definition of Major Evolutionary Transitions (METs) as being Fusions and Information Leaps, and introduce the term Major System Transitions (MSTs) to describe large-scale ecosystem transformations that appear irreversible. We note that even in the most extreme mass extinctions, while many or most species went extinct, we know of no case in which an entire large-scale ecosystem disappeared or reverted to an earlier state (e.g., the Permian extinction did not reset the world back to the Ediacaran).
Additionally, we consider morphological adaptations that confer significant direct-fitness advantages. For instance, many regard the water-to-land (Knoll and Bambach, 2000; Qiu, 2008; Van Etten and Bhattacharya, 2020) and land-to-water transitions (Aubret et al., 2007; Schwab et al., 2020) as major evolutionary events, although these did not produce novel forms of individuality or information. We define such remarkable morphological adaptations as Major Competitive Transitions (MCTs), while acknowledging the definition’s subjective nature. Here, “competitive” implies more than a slight advantage in terms of survival and/or reproduction. It implies great fitness benefits due to, for example, creating a new niche (e.g., the evolution of flight), or dominating an existing niche in a novel way (e.g., vascular tissue in land plants). Clearly, there are many examples of innovative morphologies that could potentially qualify as MCTs and lead to MSTs.
Whereas MSTs happen to ecosystems, METs and MCTs happen to species. MET and MCT categories are not mutually exclusive. For example, the evolution of larger brains and greater cognitive abilities (a MCT) can simultaneously lead to an Information Leap of complex human spoken language (a MET). However, not every MET, MCT or combination of the two necessarily immediately leads to a MST. Consider the evolution of eukaryotes from prokaryotes.
Eukaryotic single-celled organisms appear in the fossil record perhaps by 1.6 BYA (Knoll et al., 2006). Yet for a “boring billion” years of evolutionary history, they remain minor components in bacterial-dominated ecosystems before explosively radiating as large, multicellular species in an Ediacaran and Cambrian MST. Eukaryotes are obviously essential for this MST, as all animals, plants and fungi are eukaryotes. However, the initial appearance of eukaryotic cells seems insufficient for a MST. Thus, “eukaryote” is both a MET (sensu Maynard Smith and Szathmáry) and a Facilitating Evolutionary Transition, or FET, for the later MST. We define a FET as either a MET or a MCT that is absolutely necessary, yet insufficient alone, to set into motion a cascade of events that result in a MST. In other words, species that have undergone a FET require additional events (e.g., other MCTs, METs, or external “catalysts”) before the combination of events alters environments enough to transform or create entire ecosystems (Figure 1). A catalyst can be an abiotic event such as rising levels of free oxygen in the atmosphere making possible the energetic lifestyles of multicellular organisms (Och and Shields-Zhou, 2012; Lyons et al., 2014). Catalysts can also be biotic actors such as viruses and bacteria that through coevolutionary arms races and horizontal gene transfers help drive certain METs, MCTs, and MSTs (Figure 1).
Levels of Information and Selective Processes
Information – defined here as data in a repository that organisms may use to respond to problems they face or to manipulate their environments – can play a fundamental role in METs that produce MSTs. Building on the framework of Jablonka and Lamb (2006), we categorize information into five distinct levels (Table 2). Level I information is encoded in genomes. The simplest currently existing units using only Level I information are viruses of less than 10 genes. Level II information is epigenetic modification of gene expression. As such, Level II information can alter how organisms use Level I information throughout their lifetimes due to interactions with environments. Beyond Level II, bounds on total information content are progressively lifted. At Level III, information is learned and stored in neural cell repositories, and potentially interchangeable across entire populations. Level IV information is stored outside of organisms in the physical environment, either as biological icons (e.g., scent marks or pheromone trails) or instructional (symbolically inscribed). Level IV information reaches its apogee with the advent of symbolic representation of human language into quantitatively unlimited written and electronic formats. Finally, we propose a new and nascent level of information. Level V is dark information created by complex computer algorithms rather than biological beings. It is ‘dark’ in the sense that informational outcomes cannot be replicated without the technology. For example, the only test of facial recognition by machine learning is the accuracy of output – the generating process may be impenetrable to human understanding.
We note that the ‘body of knowledge’ contained at any of the five levels across entire populations can rarely, if ever, be wholly available to or expressed in any single individual. Populations will be genomically more diverse, learn a larger variety of things, and write and read more books than any individual possibly can. Furthermore, although Information Leaps create potential for new and larger pools of information, the initial benefits of such information may be small. For example, the first organisms with any Level III learning ability undoubtedly had a meager capacity for ‘knowing’ more than their antecedents with only Levels I and II. It likely took millennia for the ability to acquire and use such information to confer significant selective advantages.
Finally, the important evolutionary processes may differ across major transitions. For instance, the evolution of some METs might require kin selection or multilevel selection (Bourke, 2011a; Szathmáry, 2015; West et al., 2015), whereas MCTs spread through populations because of their direct-fitness advantages. In addition, major transitions can themselves create novel selective processes. For example, sexual reproduction through meiosis (a MET) was likely initially isogamous (Hanschen et al., 2018). Not until anisogamy (a MCT) evolved could sexual selection become a major evolutionary force. In essence, some biological innovations create a morphology-to-process feedback loop. Thus, anisogamy creates different selective pressures for individuals that produce eggs or sperm. Once sexual selection exists, it is not limited to affecting gametes, but also affects other morphologies and behaviors. It may be this newly-altered fitness landscape that enables a future MST rather than any intrinsic ecological advantage of different sized gametes.
In this paper, we reconsider the major events in the history of life on Earth, from the first cells to the recent technological developments of human societies. We focus primarily on which METs identified by Maynard Smith and Szathmáry (1995) have produced MSTs, either directly or in combination with MCTs and catalysts. In reexamining these major transitions, we also highlight the importance of information for both the METs and the resulting MSTs, and speculate upon the role that Level V dark information may play in a future major transition.
Independent Replicators to Cells With Chromosomes
The first MET is the transition of self-replicating molecules into compartmentalized cells with chromosomes (Table 1). These higher-level units arguably constitute the first living organisms – entities capable of responding to stimuli, acquiring resources, metabolizing, maintaining homeostasis, and replicating. To the degree they gained a competitive advantage relative to simple replicators, this MET might also be a MCT. Because it produced Earth’s first ecosystem, it is a MST. Although many questions about the origin of cells remain unanswered, a variety of hypothesized scenarios may have led to this transition.
Consider a population of short, non-cooperative, independently replicating molecules. Some replicators drive others to extinction, reducing the overall amount of available information (Szathmáry and Maynard Smith, 1995). Linking these replicators into chromosomes reduces competition, but increasingly longer molecules become susceptible to major mutations that disrupt replication (Eigen, 1971). This creates a circularity – Eigen’s paradox – where larger genomes require enzymes that increase replication fidelity, but substantially larger genomes are needed to code for such enzymes. Two models are proposed to overcome Eigen’s paradox and thus explain how this MET arose: the hypercycle and stochastic corrector (SC).
In the hypercycle model, simple replicators facilitate their own as well as others’ replication in a catalytic loop (Eigen, 1971; Eigen and Schuster, 1979; Zintzaras et al., 2002; Kun et al., 2005; Szathmáry, 2006; Saakian et al., 2011; Boza et al., 2014). ‘Parasitic’ molecules that do not catalyze the replication of others can invade, however, if hypercycles catalyze the parasite’s replication (Bresch et al., 1980; Hogeweg and Takeuchi, 2003). Compartmentalization into vesicle-bound “chemotons” is proposed to limit such parasitism (Eigen et al., 1981; Michod, 1983; Szathmáry and Demeter, 1987; Zintzaras et al., 2002; Gánti, 2003). In the SC model, replicators are bound in protocells and their replication is catalyzed by non-specific replicase molecules (Szathmáry and Demeter, 1987; Szathmáry, 1989; Grey et al., 1995; Zintzaras et al., 2002). Certain compositions of replicators are optimal for overall cell growth and excessive competition between them leads to failure of the entire protocell (Grey et al., 1995).
In both hypercycle and SC models, selection against cheating may occur through aggregation of the interacting elements into bounded and competing groups. This would be the first instance of group-level selection. Alternatively, catalyzation of others’ replication may be the first manifestation of kin selection if the interacting replicators are morphologically identical or similar (Levin and West, 2017). Kin and group selection frameworks, however, converge when compartmentalization in protocells enables assortment of these identical or similar individuals (Szathmáry and Demeter, 1987; Hogeweg and Takeuchi, 2003; Levin and West, 2017).
With a self-sustaining population of protocells, novel machinery can evolve. Simulations in an SC context find that division of labor arises where RNA strands diverge into biased function for either information storage or enzymatic activity (Boza et al., 2014). Similarly, it seems plausible that some replicators in a hypercycle evolve as the system’s metabolism, creating a division of labor that leaves only one type of replicator as the essential heritable repository for Level I information.
Chromosomes
The next question about the first living organisms pertains to information organization and amalgamation of separate, replicating genes into a single unit – a chromosome. The linking of genes into “proto-chromosomes” may have occurred in primordial RNAs before transitions to DNA and translation (Weiner and Maizels, 1987; Maynard Smith and Szathmáry, 1993). Advantages of simultaneously replicating chromosomes may include: (1) decreased reproductive competition among individual segments; (2) increased likelihood that cell division provides entire genomic complements to both daughter cells; and (3) increased capacity to reduce mutation loads (Maynard Smith and Szathmáry, 1993; Santos, 1998). Even if replication of two linked genes takes twice as long as for one gene, models find that linkages increase in frequency under some conditions (Maynard Smith and Szathmáry, 1993). However, the exact mechanism by which chromosomes initially formed and conditions that favored their evolution remains unknown.
Overall, the transition from simple replicators to cells with chromosomes is a Fusion MET and a MST that produced the first ecosystem of living organisms. Details of how this MST occurred are unclear, with possible facilitating adaptations and catalysts yet to be discovered.
Transition to DNA, Genome Expansion, and Epigenetic Modifications
The amount of information encoded in Levels I and II depends on which nucleic acid (RNA or DNA) constructs the genome, the genome’s size, and the degree to which genetic sequences produce different states of expression (e.g., epigenetic modification adds another layer of information ‘above’ the fixed nucleotide sequence).
RNA Versus DNA
Molecular RNA is thought to be closely associated with the origin of life because it has the potential to both store information and simultaneously retain enzymatic activity (Bernhardt, 2012). The information storage capability, but not the self-replicating ability, is observed in the current-day RNA viruses. However, such genomes are highly mutable and therefore RNA viruses succeed within hosts as evolving mutant swarms rather than stable clonal lineages (Ebrahimi and Nonacs, 2021). RNA genomes especially risk crossing into ‘error catastrophe’ where mutations accumulate at rates that make entire populations non-viable (Summers and Litwin, 2006; Manrubia et al., 2010). Thus, relying on RNA for information storage likely limits organisms to viral-size genomes and severely restricts the potential for future morphological and behavioral adaptations.
The transition to DNA may have been catalyzed by coevolutionary arms races between the ancestors of cells and viruses (Durzyńska and Goździcka-Józefiak, 2015). The first functional cells must have had two salient characteristics: (1) higher concentrations of needed building materials for life than found in the outside environment, making them rich targets, and (2) poor defenses, given that no cellular predators yet existed. Undefended rich targets would create selection for alternative life histories (Koonin, 2016). One life form – the protocells – hunts for resources to grow and divide. The second form – the first viruses – hunts protocells in order to invade and usurp their genomes. Future major transitions may have been impossible without viral catalysts (Figure 1) creating a predator-prey world.
With a transformed cellular world of parasite/predator and host/prey, host defenses would evolve by natural selection. One defense may be the inactivation or destruction of invading RNA genomes (Forterre, 2002). To evade such destruction, viruses may have evolved to retrotranscribe RNA into DNA. This would not only increase their chances of taking control of cells, but also confer a selective advantage because DNA is the more stable information repository (Forterre and Prangishvili, 2013). Alternatively, DNA could have been repurposed in hosts from a structural role in order to segregate information from viral invaders (Wolf and Koonin, 2007; Koonin, 2016). Once DNA began storing information, however, its spread throughout protocells may have occurred rapidly due to the superior molecular stability.
Therefore, the switch from RNA to DNA is an Information Leap MET that substantially increases the capacity for Levels I and II information storage (Maynard Smith and Szathmáry, 1995). Whether the initial advantages of DNA were great enough to constitute a MCT, and the extent to which this transition alone transformed ecosystems, may never be known. If not a MST, however, this transition is certainly a FET that enabled the entire subsequent history of life and future MSTs.
Genome Expansion
There are huge variations in genome size and cellular complexity across the three domains of life. Eubacterial and archaeal genomes are generally 0.5–7.6 megabases, while eukaryote genomes are at least 100 megabases (Lynch and Conery, 2003). To the degree that organismal complexity is limited by genome size (Bird, 1995), it is important to understand the processes by which eukaryotic genomes grew in size and information content. Initially, Level I information in eukaryotes was likely similar to that of prokaryotes, and the increase to present-day Level I information required various mechanisms that enlarge genomes. It seems likely that increasing Level I information contributed as a MCT that allowed eukaryotes to remain competitive in prokaryote dominated ecosystems.
Genome duplication immediately increases genome size. Duplications can be autopolyploid (within species, with multiple sets of the same chromosomes) or allopolyploid (e.g., the doubling of a hybrid genome to restore the homologous chromosome state). Over evolutionary time, initially redundant genes can diverge to produce novel functions. Several such genome expansion events appear to have occurred in eukaryotes (Van de Peer et al., 2017).
Horizontal transfer of DNA across species’ genomes can add one or more genes at a time. For example, lysogenic viruses enter nuclear genomes and may either never leave or leave behind DNA transported from other species (Hernández and Podbilewicz, 2017; Valansi et al., 2017). Over time imported elements can be co-opted within host genomes to produce novel functions (Werren, 2011), enhance gene regulation (Diehl et al., 2020), or modify horizontally imported genes for analogous functions. Indeed, key processes involved in sexual reproduction appear to be made possible only through imported viral genes (Hernández and Podbilewicz, 2017).
Additionally, endosymbionts (organisms that live within the cells of others), such as the bacterial ancestor to the mitochondria or the parasite Wolbachia, often exhibit a biased flow of genetic material into nuclear chromosomes. Even if initial DNA transfers are random in direction, the consequences of meiotic cell division result in nuclei becoming the absorbing collectors of genetic information (Nonacs and Tolley, 2014).
Epigenetics
Epigenetic modifications to DNA (Level II information) occur in all three domains of life (Casadesús and Low, 2006; Willbanks et al., 2016). Furthermore, mRNAs can also be epigenetically modified, suggesting this process could have been present in the RNA world (Garber, 2019). Eukaryotes, however, exhibit more sophisticated epigenetic regulation of gene expression than prokaryotes (Willbanks et al., 2016). This regulation likely preceded the evolution of multicellularity as single-celled eukaryotic species change their epigenetic states in fluctuating environments without altering genome sequences (Kundu et al., 2007; Payne et al., 2019; Bheda et al., 2020).
Level II epigenetic information creates alternative readings of Level I information, and empowers Lamarckian inheritance to transmit acquired characteristics across several generations of cell division (Jablonka et al., 1998; Jablonka and Lamb, 2006). In multicellular eukaryotes, such epigenetic modification can aid in differentiating dividing clonal cell lineages into specialized functions (Juliandi et al., 2010; Wang et al., 2021). Whether or not the appearance of epigenetic information significantly altered ecosystems (a MST) or provided a large fitness advantage (a MCT) is unknown. However, the evolution of epigenetics clearly qualifies as an Information Leap MET because it constitutes a novel form of information content and transmittal. It may also have served as a FET for the MST that occurred during the Cambrian explosion, due to its potential role in the transition to multicellularity (Wang et al., 2021).
Prokaryotes to Eukaryotes
The adaptive radiation and ecosystem transformation that occurred in the Cambrian (and likely also in the Ediacaran) is perhaps the single most physically apparent MST throughout history. The eukaryotes that transformed these ancient ecosystems differed in multiple major characteristics from the prokaryotes that dominated the previous two billion years. At the intracellular level their differences are profound. Unique to eukaryotes are: (1) multiple diploid, linear chromosomes (Goodenough and Heitman, 2014); (2) segregation of chromosomes into a nucleus; (3) mitochondria (Margulis and Fester, 1991); and (4) other structures, such as the endoplasmic reticulum (Butterfield, 2015). ‘Eukaryotic’ fossil cells are recognizable as larger than contemporary prokaryotes, with evidence of nuclei (Sun et al., 2020). Eukaryotes first appear about 1.6–1.8 BYA, but remain relatively minor components of ecosystems for another billion years, until about 635 MYA when the Cambrian MST occurred (Butterfield, 2000; Knoll et al., 2006; Knoll, 2011).
The Cambrian MST occurred only in conjunction with later biological innovations of complex multicellularity (i.e., plants, animals, and fungi) and meiotic sex (Goodenough and Heitman, 2014), which likely required significantly more Level I and II information than present in early eukaryotes. Hence, the previously described genome expansion in eukaryotes must have played a facilitating role in this MST. Moreover, at least three separate innovations produced mitochondria, nuclei, and diploidy, each of which also played a facilitating role in the Cambrian MST (Figure 2). Understanding the ecological impact of eukaryotes may therefore require considering synergies across multiple biological innovations, at least one MET and possibly multiple MCTs acting as FETs, along with biotic and abiotic catalysts.
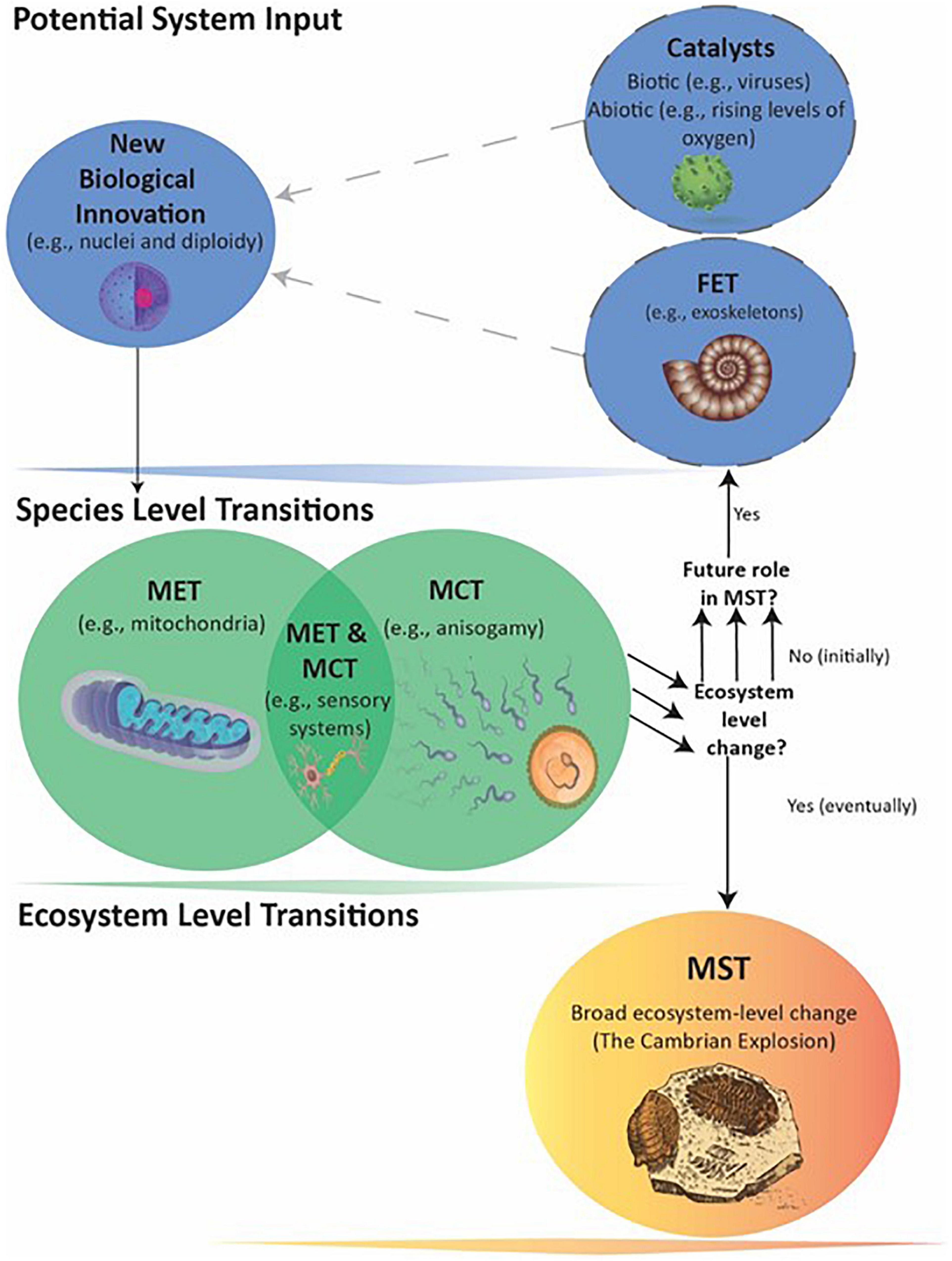
Figure 2. Events leading to the Cambrian MST. The transitions from prokaryote to large eukaryotes with tissues and organs likely involved multiple Fusions and Information Leaps, MCTs, and catalytic agents and events. The multiple arrows indicate that a number of separate Species-level transitions created multiple FETs that eventually made the Ecosystem-level MST possible. The exact order in which the FETs evolved is not conclusively known (see text).
Mitochondrial Symbiosis
There is strong evidence that mitochondria arose through endosymbiosis between a free-living relative of α-proteobacteria (the endosymbiont) and an early archaeon (Margulis and Fester, 1991), although how this endosymbiosis occurred and whether phagocytosis was involved continues to be hotly debated (Cavalier-Smith, 2009; Lane, 2011; Spang et al., 2015). Nevertheless, the mitochondrial symbiosis is clearly a Fusion MET (Szathmáry, 2015). Interestingly, all existing mitochondria and chloroplast symbioses may trace back to no more than three initiating events (Margulis and Fester, 1991): one for the former, and two for the latter. Lane (2011), a proponent of the “phagocytosis-second” hypothesis, suggests that the improbability of acquiring an endosymbiont without phagocytosis may help explain why eukaryotes arose only once. Another explanation is that the initial stages of such relationships are not particularly advantageous for either participant (i.e., not a MCT), and require additional adaptations to produce significant benefits and persist.
Evolution of the Nucleus
Three competing hypotheses for the origin of the nucleus are: endosymbiosis (Baluška and Lyons, 2018a, b), autokaryogenesis (Lisitsyna and Sheval, 2016), and the “inside out” hypothesis (Baum and Baum, 2014). Under the endosymbiosis hypothesis, possible host cell identities include an ameba (Mereschkowsky, 1905), bacterium (Forterre, 2011), proteo-bacterium (Lake and Rivera, 1994), or archaeon (Margulis et al., 2000), and candidates for the endosymbiont are an archaeon (Lake and Rivera, 1994; Forterre, 2011), spirochete (Margulis et al., 2000), or virus (Bell, 2001; Takemura, 2001). Any of these endosymbiosis scenarios would be a Fusion MET. The other two hypothesized scenarios, however, would not be METs. Under the autokaryogenesis hypothesis, nuclear membranes and closely connected endoplasmic reticulum formed from invaginations of inner membranes of prokaryotes (Lisitsyna and Sheval, 2016). Finally, the inside-out hypothesis posits that a free-living cell ancestral to the nucleus increased its surface area through developing extracellular protrusions (as are common in archaea), which ultimately gave rise to the endoplasmic reticulum and cytoplasm (Baum and Baum, 2014).
One major advantage of nuclei is physically separating the process of translation from the processes of transcription and splicing (Szathmáry, 2015; Lisitsyna and Sheval, 2016). Splicing removes introns from a precursor RNA, leaving only exons, and alternative splicing creates different isoforms from the same gene (Keren et al., 2010). Because splicing is slow compared to translation, the physical separation of these processes appears essential to the completion of splicing before translation (Martin and Koonin, 2006). Eukaryotic gene regulation with introns may be “impossible” without such physical separation (Szathmáry, 2015).
Diploidy
Eukaryotes differ from prokaryotes in being diploid with homologous, but non-identical, chromosomes for at least a portion of their life cycle. Diploidy can arise by endomitosis wherein cells duplicate their genetic material (Tüzel et al., 2001). Cells with non-haploid states quite possibly predate the appearance of eukaryotes, as many extant species of bacteria and archaea are polyploid. Indeed, some species routinely have thousands of copies of their chromosome per cell (Soppa, 2014, 2017). Even viruses have been found to package multiple genomes into virions (Chou et al., 2012). Interestingly, although the ploidy of the archaeon Lokiarchaeota, which may have been ancestral to eukaryotes, remains unknown, features such as the presence of histones strongly suggests polyploidy (Markov and Kaznacheev, 2016).
Genomes becoming diploid increases storage for Level I information, but doubling a prokaryotic genome alone does not come close to modern-day size differences between prokaryotic and eukaryotic genomes (Bird, 1995). The initial spread of diploidy likely required other competitive advantages beyond slightly more Level I information. Proposed advantages could include mechanisms to repair damaged DNA, and compensating or hiding deleterious mutations on one gene copy with redundant functional copies on the other (Bernstein et al., 1981). In haloarchaea, polyploidy provides increased resistance to desiccation, and is a storage vessel for phosphate in times of scarcity (Ludt and Soppa, 2019). In environments with limited resources and highly competitive haploid organisms, diploidy could be a “last chance” alternative life history strategy where two low-fitness haploids fuse into a competitively enhanced diploid (Jan et al., 2000). Combining diploidy with sexual reproduction can further create greater degrees of variation in offspring due to recombination (Bernstein et al., 1981). Non-haploid states, however, also create costs (Markov and Kaznacheev, 2016) as genome copy number can vary across cells due to random segregation at cell division (Ludt and Soppa, 2019). Accurate segregation, as occurs with mitosis, avoids this cost while retaining the advantages. Indeed, in simulations, mitotic diploidy can be favored over haploidy (Tüzel et al., 2001).
In conclusion, the evolution of eukaryotes with mitochondria, nuclei, and diploidy with multiple linear chromosomes must have required at least three distinct events. However, they all apparently evolved so closely together in time that we may never know which came first and the degree to which one event facilitated a second or third. There are no known intermediate eukaryotes with only one or two of these characters (although some species subsequently lost mitochondria over their evolutionary history; e.g., Karnkowska et al., 2016). One possible explanation for the lack of extant intermediates and the fact that eukaryotes arose only once, despite the continual existence of biodiverse and interactive prokaryotic communities, is that any one of these events alone was not particularly advantageous (i.e., not a MCT), and persisted only when followed by a fortuitous second or third event in quick succession. For instance, a symbiosis between mitochondrion-like α-proteobacteria and host could have required rapid suppression of selfish intragenomic selection in the former in order to persist (Bourke, 2011a). This may have only been possible by “genomically neutering” the mitochondrion through differential movement of essential genes from their genome into linear chromosomes segregated behind nuclear membranes (Nonacs and Tolley, 2014). Ultimately, regardless of whether any of the events that produced eukaryotes qualify as MCTs, the mitochondrial symbiosis nevertheless renders eukaryotes a Fusion MET. Moreover, this MET is a FET for the subsequent Cambrian MST (Figure 2).
The Evolution of Sex
Sexual reproduction is defined by meiosis followed by syngamy, the fusion of two cells and their nuclei (Santos et al., 2002). Sexual reproduction is found only among eukaryotes and apparently universally so, suggesting it appeared early in their evolution (Cavalier-Smith, 2002). In fact, critical features needed for sexual reproduction possibly preceded the mitochondrial symbiosis. For example, the fusion of gametes is mediated by cell surface glycoproteins, which appear to predate the last common ancestor of eukaryotes and archaebacteria (Cavalier-Smith, 1987). However, these may originate from ‘old’ viral genes that were horizontally acquired by a previously asexual eukaryote (Valansi et al., 2017). The difficulty in delineating the timelines of specific eukaryote-affiliated events led Szathmáry (2015) to subsume the evolution of sex into a single combined transition from prokaryotes to eukaryotes (Table 1). We differ from Szathmáry (2015) in that the origin of eukaryotes is considered not as a single event, but as a possible series of MET(s) and MCT(s) acting as facilitating evolutionary transitions [FET(s)]. Similarly, we consider the evolution of sex a MET and the evolution of anisogamous sexual reproduction a MCT (Figure 2), both of which are also FETs. Additionally, viruses appear to have played an important catalytic role through gene transfer.
Our classification of the evolution of sex as a MET follows from three consequences for which sexual reproduction is uniquely responsible: (1) changing the units of selection; (2) producing a novel form of Level I and II information transmission that increases the rates at which beneficial information spreads through populations and deleterious information is eliminated; and (3) altering natural selection by creating or revolutionizing the processes of sexual, kin and intragenomic selection.
Units of Selection
Sexual reproduction is, in a sense, a return to the earliest stages of life on Earth – the era of replicator molecules. Sex with recombination makes genes the units of selection instead of genomes (Jablonka and Lamb, 2006). On a second level of selection, reproduction requires the fusion of two distinct entities (e.g., egg and sperm). With the exception of species capable of self-fertilization, this requires coordination between two individuals and implies that mating pairs are a higher-level unit of selection (Michod, 2011). Clearly, such pairings experience unique selection pressures pertaining to partner choice (Andersson, 1994) and postcopulatory sexual selection (Birkhead and Pizzari, 2002), resulting in novel morphologies and behaviors. The mating pair, however, is not an indivisible individual, such as organelles in eukaryotes. Therefore, one could argue that sex is not a MET because mating pairs do not explicitly generate mating pairs as the propagating units (Calcott and Sterelny, 2011). Nevertheless, the offspring of a mating equally represent both parents. They are also indivisible individuals. Hence, this objection is a quantitative one – an offspring of a mating pair only partially, instead of entirely, replicates the pair.
Information Availability and Management
Sexual reproduction expands the dynamism of heredity. Recombination through sexual reproduction aggregates beneficial mutations and eliminates harmful ones more rapidly than asexual reproduction (i.e., escaping Müller’s Ratchet: Bernstein et al., 1981; Fagerström et al., 1998). Although individuals only directly access their own genotypes, their descendants potentially access all the Levels I and II information in populations. Therefore, sexual populations can rapidly create genetic combinations that are adapted to local environments in ways not previously observed in asexual organisms (Lively and Morran, 2014; Sharp and Otto, 2016). For example, recombination allows hosts to develop defenses against more rapidly reproducing asexual pathogens (Lively and Morran, 2014; Sharp and Otto, 2016). This benefit in the Red Queen arms race (Van Valen, 1973) can offset the disadvantage of longer generation times in sexually reproducing eukaryotes relative to asexual prokaryotes. Furthermore, the Level I information contained within an asexual pathogen must compete against the entire Level I information present in the sexual population of its host. Notably, no asexual variant has been observed to out-compete and displace their sexually reproducing conspecific, even in stable environments (Niklas et al., 2014).
Sexual, Kin, and Intragenomic Selection
Sexual reproduction changes evolutionary processes, perhaps more so than any previous innovation. The evolution of anisogamy first creates sexual selection. Fitness now depends on both survival and the ability to secure a mate. Only sexual selection provides a coherent explanation for the many costly and flashy traits that serve primarily to win intrasexual contests or increase attractiveness to the opposite sex, while simultaneously reducing the bearer’s survivability (Darwin, 1871; Andersson, 1994).
Sex also revolutionizes kin selection. In completely asexual organisms, individuals functionally become their own reproductively-isolated species. The Levels I and II information in two daughter cells from a fission event are as separated going forward in time as the information in two cells last sharing a common ancestor a billion generations ago. In asexual populations, kin selection may be invoked when genetically similar individuals assort non-randomly (Levin and West, 2017), or social heterosis invoked when heterogeneous groups are the more productive (Nonacs and Kapheim, 2007), although arguably individual-level selection across clonal lineages provides an equally adequate explanation (Nowak et al., 2010). Sex, however, creates more variable and quantifiable classes of kinship and many potential cooperative and competitive interactions predicated on the degree of shared genes, with examples such as nepotism, parent-offspring conflict, siblicide, adaptive suicide and senescence (Forbes, 2005; Bourke, 2011a, b). Interestingly, the evolution of highly eusocial species (i.e., those having morphologically distinct reproductive and worker castes) is attributed to kin selection and has occurred far more often in sexual than asexual species (Bourke, 2011a, b).
Finally, sexual reproduction creates a new level of selection through intragenomic conflict. For instance, a variety of sex-ratio distorting genetic elements exist by biasing the outcomes of meiosis in their favor (Queller, 1997; Burt and Trivers, 2006). When genomes come equally from mothers and fathers, the two halves may not necessarily maximize their fitness in the same way. Parentally imprinting genes (i.e., Level II epigenetics), therefore, can cause differential expression in offspring (Reik and Walter, 2001). In mammals, this results in paternal and maternal genomic conflict within individuals over the amount of resources extracted from mothers during gestation (Haig, 2015).
Major Competitive Transitions and Catalysts
The origin and elaboration of sexual reproduction required a number of critical morphological innovations, including those involved in the fusion of cells and the transition from isogamy to anisogamy. In cellular fusion, sperm and egg cell membranes merge into a single, unbroken one (Clark, 2018). Viral genomes enter host cells by a similar process, made possible by an essential class of proteins (fusexins). Genes coding for these proteins are very similar across viruses and eukaryotes (Valansi et al., 2017). Thus, sexual reproduction appears to be made possible because of horizontal transfer of viral fusogens (Hernández and Podbilewicz, 2017). Without a virus as a catalytic agent, the evolution of sexual reproduction may not have been mechanically possible.
Within multicellular eukaryotes, anisogamous gamete production predominates. It is unknown whether the evolution of complex multicellularity with differentiated tissues needed to precede anisogamy or vice versa (Hanschen et al., 2018). However, it is clear that the first sexually reproducing eukaryotes were likely isogamous (Parker et al., 1972; Yang, 2010; da Silva, 2018). Mathematical models predict that such a state is often evolutionarily unstable. Mutants that tradeoff motility for larger size increase relative fitness through higher offspring survival (Parker et al., 1972), and others with motile gametes can increase their numbers by decreasing individual cell size. The disruptive selection eventually results in large, immobile eggs and motile sperm of minimal size (da Silva, 2018). If there is a further significant transport cost in finding receptive gametes, then two alternative reproductive strategies result: either produce many searching sperm or few eggs waiting to be found (Yang, 2010). Because it is likely that the initial benefits of anisogamy relative to isogamy were great, the evolution of anisogamy is a MCT.
In conclusion, sexually-reproducing eukaryotic species are and have been significant parts of almost every known ecosystem since the Cambrian (Santos et al., 2002; Jablonka and Lamb, 2006; Szathmáry, 2015). It is also notable that among the many metazoan lineages, only bdelloid rotifers have maintained a non-sexual and non-meiotic lifecycle on a geological timescale, through employing alternative methods to generate genetic diversity (Flot et al., 2013). Given the magnitude of sexual species’ effects on ecosystems, the evolution of sex is a critical FET composed of a MET and at least one MCT – that together paved the way to a MST.
The Evolution of Multicellularity
Egalitarian and Fraternal Associations
Multicellularity has been defined in a variety of ways, ranging from functionally independent cells clumping as groups to indivisible multicellular entities (Hammerschmidt and Rose, 2021). Such associations can be “egalitarian” where cells do not derive from a recent common ancestor (Queller, 1997). Cheating, however, can be a serious problem. For example, cooperators could produce costly compounds to stick together as mats and increase reproduction for all cells (Velicer and Yu, 2003). Cheaters not producing the compounds gain these benefits without incurring the costs. They, therefore, increase in frequency until mats collapse to everyone’s detriment (Tarnita, 2017). Overall, egalitarian systems may lack stabilizing mechanisms needed to maintain equal intragroup reproduction; severely limiting evolutionary potential (Rainey and Kerr, 2011).
Alternatively, multicellular associations can be “fraternal” where all cells share a recent common ancestor (Queller, 1997). In clonal associations, direct and indirect fitness are indistinguishable by genetic relatedness (Bourke, 2011a; West et al., 2015). Therefore, the evolutionary process favoring cooperation in clones may be conceptualized as either kin, group or lineage selection. Consider a multicellular clone with reproductive division of labor across germline and somatic cells that is competing with another where all cells reproduce. If the former produces more total offspring, that clone should increase in frequency.
Interestingly, mutations that diversify growing fraternal clones can create a kin-selective scenario that evolutionarily stabilizes reproductive specialization. If cells divide at unequal rates, the most rapidly dividing lineages will accumulate more divergent mutations. At this point, associations are no longer completely identical clones and distinctions arise between direct and indirect fitness. Ideally, each genetically unique cell or lineage should strive to reproduce while suppressing all others. If none can dominate all competitors, then the stable solution favors a consensus ‘second best’ relative as the reproductive (Reeve and Jeanne, 2003). The competing lineages will likely all have the same closest relative – those cells that divided the least and accumulated the fewest mutations (Queller, 2000). This group of cells would, therefore, be acceded “virtual dominance” over reproduction without needing an intrinsic ability to suppress competitors (Reeve and Jeanne, 2003). Although looking like a ‘cheater,’ the virtual dominant would be tolerated and favored to become the germline in the maturing clone (Veit, 2019). Indeed, in many animals, some cells are segregated very early in development and these become the gamete producers (Kumano, 2015).
Simple Multicellularity
The transition from a population of cells into an integrated multicellular organism requires several developmental innovations, such as cells adhering to one another and exchanging signals to coordinate activities (Niklas, 2014). Mathematical models suggest that a wide variety of factors favors such transitions from a solitary cell to a grouped state (Staps et al., 2021). This would be Stage 1 in the evolution of multicellularity, where each cell directly benefits from being in a larger association (Hammerschmidt and Rose, 2021). The evolutionary transition to Stage 2 occurs when group-level reproductive success becomes dependent on cooperation between cells, and entire assemblages act as multicellular individuals (Hammerschmidt and Rose, 2021).
Nevertheless, fossil records provide little evidence that early multicellular organisms had significant competitive advantages (MCTs) over single-celled ones or greatly altered ecosystems (producing a MST). Indeed, many extant species with Stages 1 and 2 multicellularity still retain significant single-celled portions of their life history, or readily switch back and forth as environmental conditions change (Staps et al., 2021). Moreover, the simplest possible multicellular associations – rudimentary bacterial mats – changed little over their initial billions of years of life. Thus, the first appearance of multicellularity was neither a MCT nor a MST. Simple multicellularity is also not considered a MET (Table 1) because it is neither a fully formed, higher-level individual nor a novel form of information storage or transmission. Simple multicellularity would appear in Figure 1 only as a ‘new biological innovation’ that was needed for the eventual MET of complex multicellularity (Stage 3: with obligate ontogeny of differentiated tissues; Hammerschmidt and Rose, 2021).
Abiotic Catalyst
Complex multicellularity has arisen independently in multiple eukaryotic lineages, with a common feature: organisms’ overall energy demands are met by subsets of cells dedicated to meeting them. A critical abiotic catalyst for this transition (Figure 1) is a sufficient level of free oxygen to support high-energy metabolisms based on oxidative phosphorylation. Earth’s history reflects two periods of rapid oxygen increase: (1) the Great Oxygenation Event (∼2.5–∼2.0 Gya), when oxygen first became consistently available in low but biologically significant concentrations; and (2) the Neoproterozoic Oxygenation Event (∼0.8–∼0.54 Gya) when concentrations rose to near present levels (Och and Shields-Zhou, 2012; Lyons et al., 2014). Significantly, complex multicellular forms only appear in the late Neoproterozoic (Nursall, 1959; Budd and Jensen, 2000; Lyons et al., 2014). Thus, this second event was probably an important abiotic catalyst for the evolution of complex multicellularity (Figure 1).
Complex Multicellularity
In complex multicellular species with differentiated tissues and organs, development follows the fraternal route. Life history proceeds through a single cell stage, most commonly the fusion of two gametes, with clonal growth into the mature state (Bourke, 2011a). That complex multicellularity is closely tied to sexual reproduction may be because such organisms necessarily reproduce much more slowly than unicellular organisms, and relying on purifying selection to remove deleterious mutants from asexual lineages is a huge disadvantage. Sexual reproduction, therefore, allows a more rapid purging of deleterious mutations through recombination and greater genetic variation in offspring for adapting to changing environments or co-evolutionary arms races with pathogens (Fagerström et al., 1998).
Waddington’s (1957) epigenetic landscape seemingly answers the question of how reproductive specialization is maintained across cell lineages. Somatic differentiation with Level II epigenetic modification limits lineages to terminal fates (Pacheco et al., 2014). Mechanistically, this prevents competition arising with virtual dominant germlines. Thus, non-germline lineages are freed to, for example, evolve as sensory and neural systems that receive and transmit information, or become specialized in provisioning and transporting of metabolites, nutrients and gasses to maintain overall homeostasis beyond what is possible by diffusion (Knoll and Lahr, 2016).
A strict reproductive segregation, however, is far from the case across all multicellular species. Many cnidarians, for instance, display remarkable abilities to transdifferentiate, seemingly including converting somatic cells into germ cells (Gold and Jacobs, 2013). Medusozoan cnidarians are even capable of “degrowing” gonadal structures or reverting to asexuality (Hamner and Jenssen, 1974; Piraino et al., 2004). Similarly, plants do not segregate germlines from somatic lineages, but rather derive germ cells from somatic lineages (Kawashima and Berger, 2014). Therefore, a Waddingtonian view of canalized germ cells cannot be absolutely necessary for the evolution of tissues and organs. Furthermore, cellular ‘cheaters’ (e.g., cancers) are much more common in bilaterian clades (Aktipis et al., 2015), correlating with evolutionary losses in cell totipotency as their tissues differentiate. It is paradoxical that cancer is rare or absent in cnidarians and ctenophores where somatic cells can quickly revert to being gametic and thus would seem to be especially vulnerable to mutations for cheating. Analogs to present-day animal oncogenes appear to have evolved well before the appearance of bilaterians (Trigos et al., 2019), but their causative propensities are most evident in taxonomic clades where cell sterility is normally obligate and not facultative.
Ultimately, although all stages of multicellularity create higher-order associations, only Stage 3, tissue-level cellular differentiation truly constitutes a MET (Table 1). It is when the component parts of an organism must developmentally specialize that there is a fully formed, higher-order “individual.”
An Evolutionary Scenario
Rising oxygen concentrations in the Neoproterozoic increased viability of aggregations of mitotically dividing eukaryotic cells, and a potential scenario emerges for evolution of complex multicellularity. Aggregations create opportunities for innovative cooperation and division of labor across cells (Wolpert and Szathmáry, 2002). Freeing cells from having to provide their own energetic and metabolic needs allowed diversification and specialization. The actual phenotypic innovations vary across groups. In plants, MCTs like vascular tissue enabled colonization of the terrestrial environment and the evolution of larger sizes (Stebbins, 1969) and flowers provided the opportunity to co-opt animals into being vectors for fertilization. In animals, the evolution of nervous systems repurposed cells for storage and transmission of information (Jablonka and Lamb, 2006). Level III Learning allowed the evolution of behaviors requiring predictive ability and flexibility such as parental care, territoriality, mate choice and sociality. New evolutionary rules for behavioral interactions such as direct and indirect reciprocity (Maynard Smith, 1982; Nowak, 2006) became important processes within natural selection. Given that multiple separate lineages evolved complex multicellularity almost simultaneously while using different genetic and developmental mechanisms, it seems these were METs merely waiting for the right set of opportunities to arrive.
By conservative estimate, complex multicellularity with tissues and organs emerged at least six times in sexually reproducing eukaryotes: animals, plants, red algae, brown algae, and at least twice among fungi (Bernstein et al., 1981). Observing the range of shared commonalities across lineages would lead to better understanding of how such a variety in the same category of MET occurred. Unfortunately, inferences are limited because the evolution of development is well studied only in lineages of bilaterian animals and higher plants.
The appearance of complex multicellularity fundamentally changed the planet; altering environments and creating entirely new ecological niches such as vertical communities of kelp forests (Teagle et al., 2017). Swimming animals in the oceans increase biomass export to the deep sea, sustaining the oxygenated state of the oceans (Butterfield, 2018). Land plants potentially stabilized climatic conditions throughout the Phanerozoic via their root systems increasing weathering of rock (Ibarra et al., 2019). Plant leaves alter the water cycle via transpiration and are responsible for the majority of global evaporation (Schlesinger and Jasechko, 2014; Wei et al., 2017). Where bacterial mat stromatolites once dominated, the evolution of animals restricted their range to stressful environments free from grazers (Walter and Heys, 1985; Sheehan and Harris, 2004). The many significant and continuing ecosystem-level impacts of complex multicellular species certainly qualifies as a MST.
Evolution of Eusociality
The term “eusociality” is applied to group-living species that divide reproductive labor, cooperatively care for offspring, and have overlapping generations of parents and adult offspring (Batra, 1966; Wilson, 1971). This broad definition covers a continuum of species, without a clear point of demarcation as to where any major transition happened (Bourke, 2019). Recently, the MET is proposed to be crossed when a ‘queen’ caste monopolizes reproduction, with an obligately sterile, morphologically-distinct worker caste (Boomsma and Gawne, 2018).
Contrary to current opinion (Maynard Smith and Szathmáry, 1995; Queller, 2000; Szathmáry, 2015; West et al., 2015; Boomsma and Gawne, 2018; Bourke, 2019), we propose that eusociality is not a MET (Figure 3). We argue that eusocial species neither fundamentally alter how information is stored and transmitted across individuals, produce a new level of individual, nor create new or enhance existing mechanisms within natural selection. However, many eusocial species are remarkably abundant and ecologically dominant, qualifying them as a MCT. In some cases, the evolution of more elaborate eusocial societies (e.g., ambrosia beetles and fungus-garden ants) may have required the catalyst of competition and mutualism with viruses, bacteria and fungi (Biedermann and Rohlfs, 2017) (Figure 3).
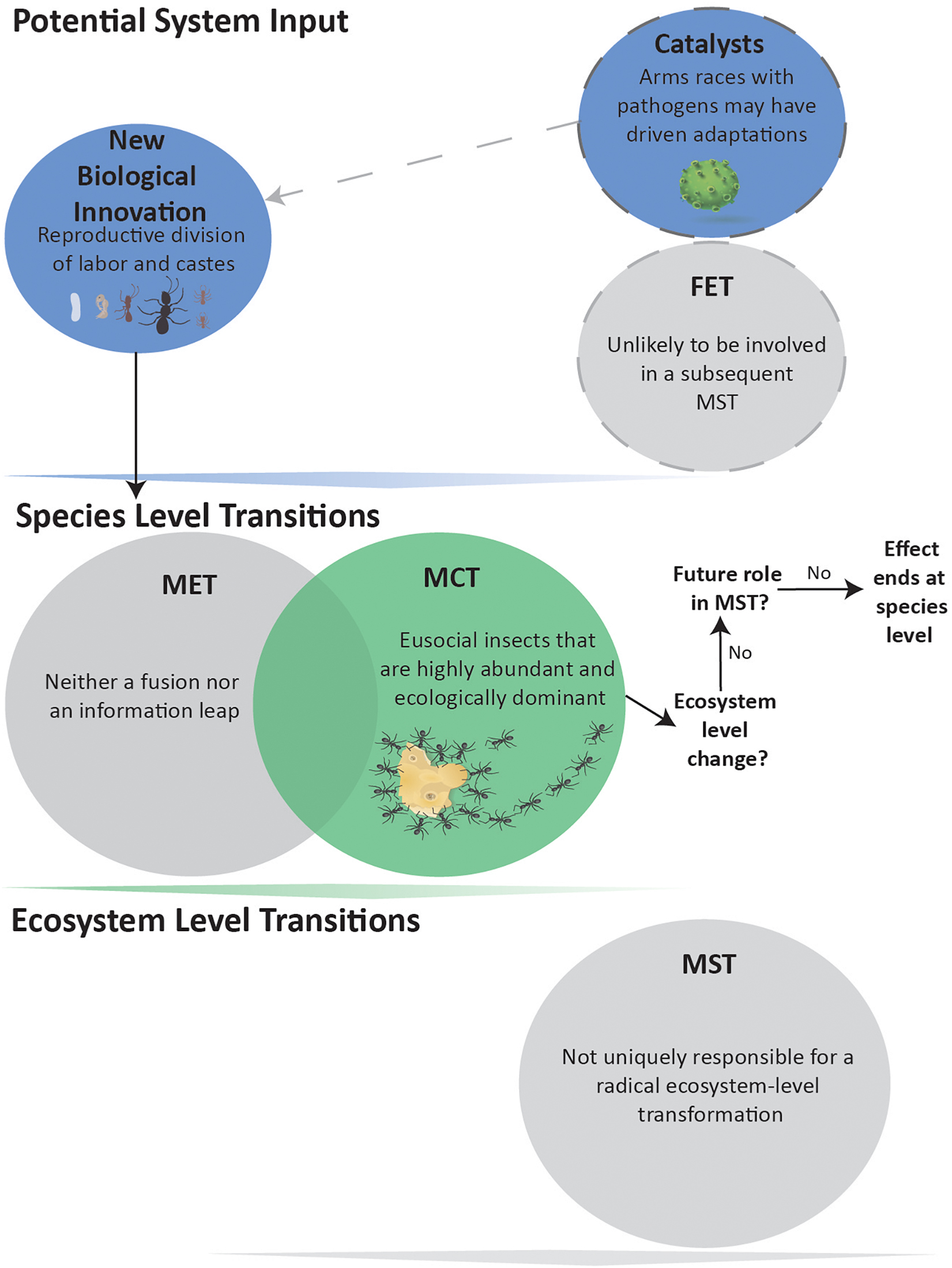
Figure 3. Eusocial evolution not leading to a MST. Evolution of morphologically distinct castes and reproductive division of labor is considered a MCT due to the abundance of social insects. These biological innovations are neither Fusions nor Information Leaps and, therefore, not a MET. The eusocial insect MCT has arguably not produced an Ecosystem-level MST, and shows no indication for being a FET in any such impending event.
Level I and II information in eusocial species is stored and transferred across generations no differently than in other sexual species. No matter how genetically diverse a group might be, any given offspring reflects a genetic bottleneck of no more than two parents. Eusocial groups make substantial use of Level III and IV (Iconic) information as multiple individuals rely upon chemical trails to track resources, activities of neighbors, or looming threats to a far greater degree than any single individual. However, chemical communication did not originate in eusocial species, and thus is not a qualitative Information Leap. Moreover, Level III and IV information is ephemeral across generations. The same structures and trails may be used across generations within a colony, but its dispersing offspring carry along none of this information. It is likely that parental birds pass more Level III information to their chicks than any social insect colony passes to a descendant colony. Overall, therefore, all known eusocial species fail the MET criterion of adding or expanding levels of information transmittal or storage.
Eusocial species in which individuals are so morphologically differentiated that group living is obligate would seem to strongly suggest a transition to a higher level of individuality (Boomsma and Gawne, 2018; Bourke, 2019). An alternative view, however, is that eusociality is a modification of complex multicellularity where non-reproductive worker castes are analogous to somatic tissue specializing in maintaining overall homeostasis. This diffuse ‘worker caste organ’ differs from others like hearts and kidneys in having regions of considerably weaker cell to cell adhesion and higher within-tissue genetic heterogeneity.
Evolving a genetically heterogeneous worker caste organ certainly qualifies as a biological innovation. It is a novel way to create an “extended genotype” that specializes in nurturing the gonadal tissue. Thus, rather than care for and raise their offspring directly, eusocial parents cooperate to create tissue (i.e., workers) that provides food, care and protection to the next generation of reproductive offspring. That worker castes evolved as purposeful constructions through parental manipulation is empirically supported across facultatively eusocial species (Kapheim et al., 2012, 2015; Rehan et al., 2014).
Some (e.g., Boomsma and Gawne, 2018) have included “irreversibility” as a criterion for METs; that is, higher levels of individuality cannot dissolve back into their antecedent parts (Bourke, 2011a, 2019). Although we do not invoke irreversibility in our MET definition, it is nevertheless worth briefly discussing whether eusociality meets this criterion. This is certainly not the case in eusocial species without morphologically distinct castes. Even in genera where group living is common, species can revert to solitary life histories as seen in primitively eusocial bees (Danforth et al., 2003; Cardinal and Danforth, 2011) and paper wasps (Liebert et al., 2005). In contrast, no reversals to solitary living are documented in species with obligate, morphologically differentiated castes (Boomsma and Gawne, 2018). However, species can evolutionarily lose entire morphological castes. Many inquiline parasites of other social species lose their worker castes (Sumner et al., 2003). Conversely, numerous species have lost the queen caste and workers have regained full reproductive capacities (Rabeling and Kronauer, 2013). Although such species continue to live in groups - as solitary living may simply be a poor strategy in a very competitive world - there seems no intrinsic reason why single individuals could not survive and reproduce on their own, under the right conditions. Eusocial species seem to fail, at least hypothetically, as a new level of irreversible individuality.
In terms of processes within natural selection, eusocial species can be affected by direct, kin and group selection (Trivers and Hare, 1976; Nonacs, 1986, 2017; Wilson and Hölldobler, 2005; Bourke, 2011a; West et al., 2015). However, the evolution of eusociality neither originated these processes nor qualitatively altered their operation relative to solitary species. Therefore, eusociality also fails this final possible MET criterion.
We therefore conclude that the evolution of eusociality better fits the category of MCT – a novel innovation that confers significant selective advantages. For example, eusocial species are dominant community members by biomass across a variety of non-arctic habitats (Wilson, 1990, 1992; King et al., 2013). Of the approximately 900,000 known insect species, ants and termites account for about 2%, yet constitute over 50% of insect biomass (Wilson and Hölldobler, 2005). This raises the question of whether species or clade-level abundance is enough to be considered a MST.
The evidence is not conclusive on the effects of eusocial species at ecosystem levels. The first appearance of eusocial termites, bees, wasps or ants has not obviously correlated with pronounced turnovers in fauna or flora. For example, ants first appear in the fossil record about 100 MYA (early Cretaceous), with molecular data suggesting a mid-Jurassic origin (140–168 MYA), but ants become ecologically significant only much later in the Eocene (Moreau et al., 2006). Furthermore, if eusocial species are not present, it seems that their niches within ecosystems are filled by other organisms. Pristine Hawaiian ecosystems, with no native eusocial species (Wilson and Holway, 2010), do not appear fundamentally different in their construction from mainland ones. In summary, eusocial species fall into a gray zone of whether their abundance and ecological persistence is enough to qualify them as a MST. From our perspective, it is not (Figure 3). Moreover, current eusocial societies do not appear to have any biological innovations whose downstream consequences are likely to act as a FET for a future MST.
Finally, regardless of whether eusociality is considered a new ‘individual’ (MET and MCT) or a new type of ‘organ’ (MCT only), the analogy of workers to somatic cells reveals an interesting parallel to cancers. Cancer or cancer-like cells are observed across many taxonomic groups, but far more commonly in sexual species that segregate gamete production into small populations of germline cells (Aktipis et al., 2015). Thus, cancer can be viewed as a within-individual cheater that rejects the imposition of lost cellular totipotency. In the eusocial hymenoptera, queens are the segregated germlines. Parasitic species reproducing at host expense have evolved in all the social hymenopteran groups (Wilson, 1971; Schmid-Hempel, 1998). Their evolution follows Emery’s rule, where social parasites likely originally evolve as a within-species alternative reproductive strategy that rejects imposed sterility (Wilson, 1971) – the equivalents of terminal “social cancers” (Oldroyd, 2002). In contrast, termites are far more indefinite in their developmental trajectories and can ‘dedifferentiate’ from becoming a worker and instead become a reproductive (Wilson, 1971). Unlike social hymenoptera, termites have no known cancer-like social parasites (Schmid-Hempel, 1998). Termites it seems, are like cancer-free cnidarians while hymenoptera are like cancer-prone vertebrates.
Humans
Human Spoken Language
Human language expands the communication of information and intentions between individuals, and is considered a MET (Table 1). Language, however, is found across many other species. Baboons and great apes can transmit and understand sophisticated and subtle concepts (Seyfarth et al., 2005). For instance, apes can communicate through gestures and vocalizations that functionally reference the environment, objects or behaviors (Byrne et al., 2017). Further, individuals actively alter gestures and vocalizations depending on social contexts and targeted audiences (Schel et al., 2013; Byrne et al., 2017). For example, chimpanzees make alarm calls consistent with criteria for intentional signaling when exposed to realistic model snakes, and they target warnings toward naïve individuals unaware of the threats (Schel et al., 2013). Hence, human spoken languages are increasingly viewed as quantitatively rather than qualitatively different from languages of other species. Circumstantial evidence suggests that archaic hominids exhibited communication that was conceptually very similar to that of modern humans (Harris and Bullock, 2002). Given that present-day humans are innately able to learn any language, fairly advanced communication probably predated the dispersion of modern Homo sapiens.
Human languages enable social groups to collate greater amounts of Level III information across generations than possible in any individual’s lifetime. Transmission of information occurs vertically (from parents to offspring), horizontally (peer to peer) and obliquely (non-parental exchanges across generations), and can occur between individuals that never met or even lived at the same time (Cavalli-Sforza and Feldman, 1981; Jablonka and Lamb, 2006; Danchin and Wagner, 2010; Navarro et al., 2018). This makes cultural evolution a powerful and swift process that heightens humans’ ability to adapt, modify and construct their environments relative to non-human species (Feldman and Laland, 1996; Jablonka et al., 1998; Mesoudi, 2017). Furthermore, adaptive cultural innovations are not dependent on occasional random beneficial mutations. Instead, innovations can intentionally arise through trial-and-error learning or as unique combinations of several existing traits (Creanza et al., 2017).
Specific regions in brains are associated with cognition and language ability (Kaup et al., 2011), and size positively correlates with functionality across species (Kotrschal et al., 2013). Large brains relative to body size impose massive metabolic costs at the expense of the body (Kuzawa et al., 2014). Offsetting such a cost in hominids are the benefits of information-rich language that larger brains enable, likely allowing social groups to solve a variety of ecological challenges (González-Forero and Gardner, 2018). Increased cognitive abilities also meant that groups could more effectively cooperate (Herrmann et al., 2007) and practice indirect reciprocity (Nowak, 2006). Indeed, there is no evidence for human language devolving in any ancestral populations, indicating strong positive selection for maintenance (Maynard Smith and Szathmáry, 1995; Szathmáry, 2010).
Therefore, humans’ large brains with enhanced language centers are a MCT, and enabled the MET of advanced languages that greatly expanded the amount and availability of Level III information. However, dramatic changes in ecosystems and large-scale environment modifications that qualify as a MST still awaited another innovation (Figure 4).
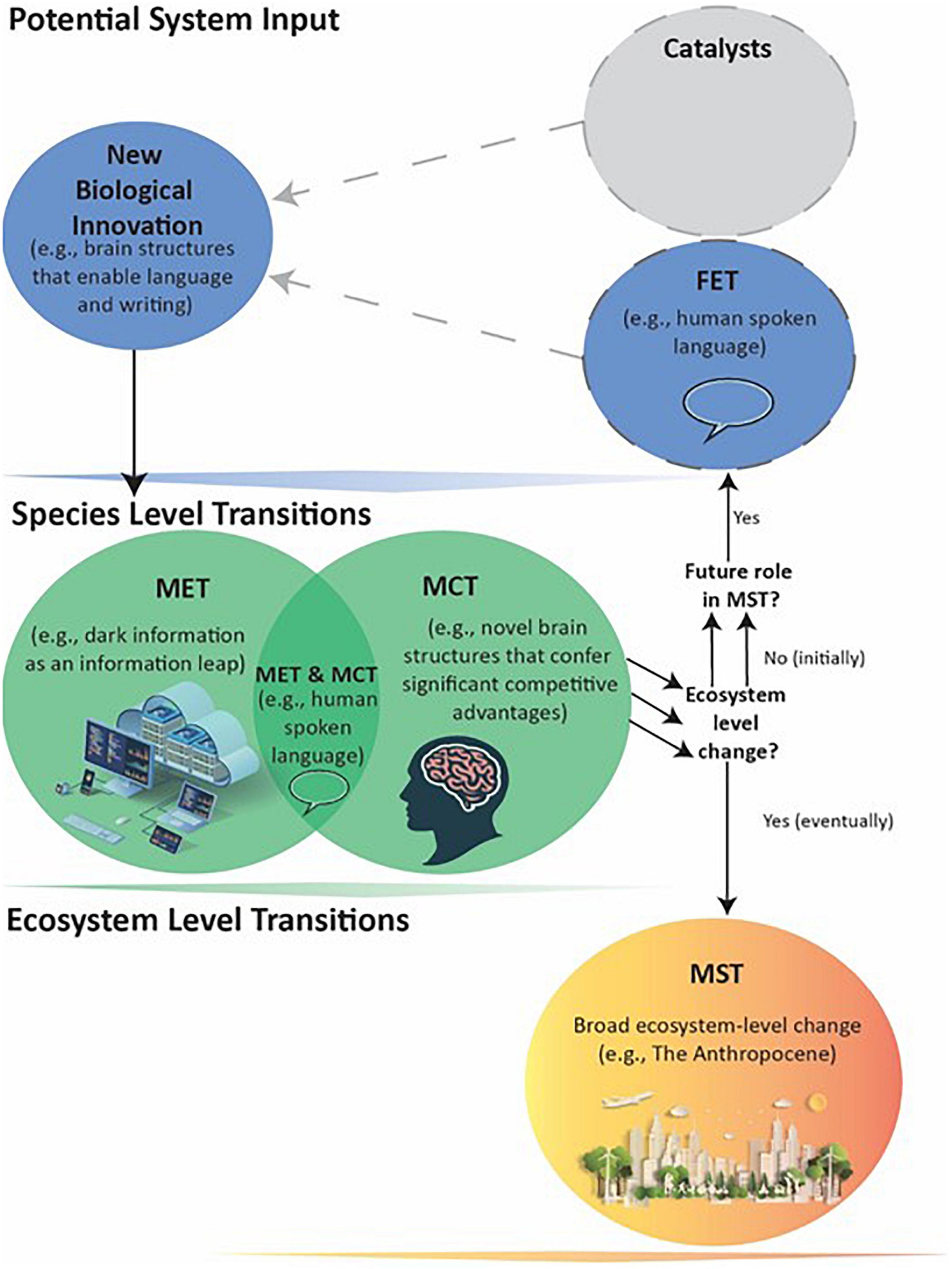
Figure 4. Human evolution leading to a MST. Humans produced broad Ecosystem-level effects through a combination of METs (e.g., spoken language in a Level III Information Leap) and MCTs (e.g., increased intelligence with larger brains). These became separate and essential FETs, as indicated by the multiple arrows, that eventually enabled the Level IV Information Leap MET of storable symbolic language and the MST. As MSTs can ‘set the stage’ for future METs, MCTs, and MSTs, it is thus possible that human-created technologies (e.g., robotics combined with Level V artificial intelligence) will lead to a future major transition that melds the biotic and abiotic into a new individual.
Symbolic Language
Both a MET and MCT, human language is also a critical FET enabling the emergence of Level IV (Instructional) information as inscribed symbolic representations (Table 2 and Figure 4). Information stored in abiotic formats adds another highly efficient method for passing non-genetically inherited cultural traits vertically, horizontally, and increasingly obliquely (e.g., book author – living or dead – to reader) (Feldman and Cavalli-Sforza, 1976; Jablonka and Lamb, 2006; Danchin and Wagner, 2010). This potentially immortalizes, in amount and accuracy, the information that can be transferred from past to current and future generations. Humans are the only known species to store and access extraordinary amounts of Level IV (Instructional) information.
The capacity for symbolic representation of language is critical for the emergence of technological innovations that expanded the realized niche for humans exponentially and paved the path to a global MST. We proliferated across every continent and environment on Earth while substantially impacting these ecosystems. One example of inscribed language producing global-altering information and technology is the very existence of the discipline of evolutionary science and the systematic study of life itself. Humans are uniquely able to understand how evolution works. This creates the novel opportunity to fundamentally alter or even void the processes of natural selection (Stock, 2008; Ghiorghita, 2020). Plants are intentionally manipulated to be more nourishing and harvestable, and animals domesticated for food and service. Through technological advances, humans alter or eliminate important natural selection factors that historically affected our species, such as predators and disease. Sexual and kin selection likewise may be diminishing in importance. For example, sperm donation and surrogacy allows individuals to pass on genes independent of having a mate or being able to become pregnant. Selectively editing human genomes is not inconceivable (Cai et al., 2016). Fetal genetic testing and potential genome editing may directly change population-level allele frequencies, with associated serious ethical concerns.
Human-produced technology creates a virtually limitless capacity for storage and retrieval of cultural information. Online cloud storage placing information in centralized and easily accessible repositories greatly reduces the need for physical storage space. It is estimated that the four major cloud-based storage systems: Google, Facebook, Amazon and Microsoft, have 1.2 million terabytes of information stored (Dastbaz, 2019). Cloud storage also drastically shifts information access, making a great multitude of data types simultaneously available from anywhere on the planet. This can increase the rate of cultural evolution and act as a homogenizing factor across cultures. Increasingly in the future, technologies, cultural institutions and biotic life will be locked into a continual process of co-evolution, where the selective processes involved will differ from those that heretofore affected the evolution of all life on earth. Overall, these changes will likely also influence biological evolution across the planet for millions of years (Gowdy and Krall, 2014). Therefore, humans’ explosive population proliferation and associated profound ecosystem effects bear the hallmarks of a MST.
Level V: Dark Information
Out of the technological advancements made possible through inscribed language a new level of information has emerged. Level V is information generated and transmitted by abiotic entities, such as computers. The process from input to generated output cannot be replicated except by computer, and therefore may remain inscrutably dark. Examples of such dark processes are increasing rapidly. Modern deep learning algorithms form representations of multiple layers of features of data without specific instructions regarding what to learn (LeCun et al., 2015). Human-directed computation and unsupervised machine learning explore difficult questions by searching the World Wide Web in medicine, mathematics, physics, economics, and engineering (Obermeyer and Emanuel, 2016; Sanchez-Lengeling and Aspuru-Guzik, 2018; Brummitt et al., 2020). Collection and analyses of internet searches and activity, phone, text and email communications, and GPS locations inform product marketing, terrorism prevention, and global markets (Greenwald and MacAskill, 2013; Einav and Levin, 2014; Xu et al., 2016). Artificial intelligence designs autonomous machine learning algorithms to diagnose cancer (Song et al., 2017) or control self-driving cars (Hecker et al., 2018). Further, algorithms are increasingly implemented to strategically decide and place user-specific advertisements, as well as generate novel content which can potentially perpetuate cultural features such as fashion trends (Lee and Cho, 2020). Finally, machine learning programs are expanding to the point of generating novel problem-solving algorithms without any added human input (Real et al., 2020). Thus, a key defining feature of Level V Dark information is the ability to create new information without any direct involvement of a biological entity.
In summary, human language and the brain that produces it qualify as a MET and MCT, respectively. The innovation of advanced forms of language increased and changed information content and transmittal, providing humans a critical competitive advantage (Jablonka and Szathmáry, 1995). Spoken language also served as a FET for the emergence of Level IV instructional information, the requisite component for our current ecological transformation and production of a MST (Figure 4). The ability to accumulate and immortalize Level IV information through stable storage systems and transfer it across generations enables development of increasingly advanced technologies and the advancement of knowledge, such as our fundamental understanding of evolutionary processes. The technological innovations made possible our manipulations of environments across every part of the globe, perhaps with irreversible ecosystem-level changes. Our understanding of natural selection presents the unique possibility of both exempting ourselves from its dictates and directing evolutionary changes in other species. Ongoing technological innovations also created for the first time: Level V Dark information, which in the span of a few decades is strongly affecting human interactions and societal institutions. It is too early to predict where the expanding Dark Information will lead, except that it will likely be quite impactful. One increasingly realistic possibility is that human-facilitated artificial intelligence, robotics, and Level V information will themselves be FETs for a future symbiotic MET that creates a new level of individual as a combination of biotic lifeforms with abiotic technologies.
Discussion
Today’s biotic world differs greatly from that of four billion years ago. In this timespan multiple Major System Transitions (MSTs) created or significantly altered ecosystems, ranging from the origins of life to humans’ large-scale modification of nearly every extant biome. Within this history, Maynard Smith and Szathmáry (1995) defined Major Evolutionary Transitions (METs) as leaps in organismal complexity forming higher-level ‘individuals’ or creating novel forms of information storage or transfer, regardless of any resulting ecosystem impacts (Szathmáry, 2015). Analogously, we classify as Major Competitive Transitions (MCTs) biological innovations that produced significant direct-fitness advantages within lineages, such as shelled eggs or endothermy (Huxley, 1942; McShea and Simpson, 2011), regardless of their broader ecosystem impacts.
Not all METs and MCTs result in a MST (Figure 1). We can distinguish those that result in or facilitate MSTs through thought experiments. Would today’s world look substantially different if a proposed MET or MCT failed to happen? For instance, the first two METs – culminating in a functioning prokaryotic cell with DNA (Szathmáry, 2015; Table 1) – are clearly essential precursors to every current ecosystem. If Level I information had remained RNA-based, genome size and total information content would be extremely limited. Without viable and replicating cells, no greater organismal complexity would be possible. Thus, the evolutionary events that led to cells with DNA genomes either produced MSTs or were critical for facilitating MSTs.
It is equally clear that if multicellular, sexually-reproducing eukaryotes with tissues and organs did not evolve or vanished, the remaining ecosystems would be transformatively different. History further suggests their current niches would not be easily refilled. For example, prokaryotes have not shown evidence of evolving multicellular complex beings. Similarly, early eukaryotes required perhaps a billion years, and multiple facilitating events, before the Cambrian MST occurred (Butterfield, 2000; Knoll et al., 2006; Knoll, 2011).
The long delay in transforming a world composed of algal mats to one full of plants and animals illustrates the conceptual importance of “facilitating evolutionary transitions” (FETs). These are innovations that are necessary, but insufficient alone, to initiate a cascade of events that culminates in a MST (Figure 1). For instance, the evolution of various sensory systems that conferred significant direct-fitness advantages (MCTs) may have facilitated a massive coevolutionary burst between predators and prey (McMenamin, 1988; Parker, 2011), which was a causal factor for the Cambrian MST. Another FET for the Cambrian MST was the evolution of sexual reproduction, which unleashed sexual selection and revolutionized kin selection. Finally, all these events happened only in eukaryotic lineages, suggesting this more complex cell type was critical for a MST (Figure 2). It is likely that without these FETs, there would not have been a MST.
Another proposed MET in the timeline of the history of life is the evolution of eusocial species (Table 1), but we argue that eusociality is neither a Fusion into a new level of individual nor an Information Leap. The spectacular abundance of eusocial species across ecosystems has the hallmarks of a MCT (Wilson, 1990), and it may or may not be a MST (Figure 3). Certainly, if all eusocial insects disappeared there would be immediate ecosystem-level effects. Indeed, some species inhabiting unique niches might never be replaced; such as leaf-cutting, fungus garden ants. On a longer time-scale, however, the effects may be transitory, which would suggest that eusocial species are not uniquely responsible for the state of the ecosystem. Consider that termites are extremely important members of decomposing communities. If termites had never evolved, however, it would seem extraordinarily likely that decomposing MCTs would have evolved in other non-eusocial lineages. The world would not today be covered 100 meters deep in dead wood. In an analogous real-world experiment, 67 million years ago all large terrestrial vertebrates were likely dinosaurs. From that ecosystem, one might conclude that dinosaurs were a MST. Yet the dinosaurs turned out to be ecologically replaceable by mammals, when they were given the chance. Thus, being abundant and ecologically dominant over a long time might qualify as a MCT (for dinosaurs, birds and mammals the MCT innovation could be endothermy), but would not necessarily imply that this group was responsible for an ecosystem transformation (a MST).
We argue that such a thought experiment validates humans as a MST (Figure 4). Human behavior and activity over the last 10,000 years has substantially altered the world’s ecosystems and even changed the planet’s climate. Humans have also created a new ecosystem we can call the ‘technology biome.’ This novel ecosystem makes available to its most important inhabitant food, water and shelter, and buffers them from the majority of environmental extremes, predation and disease. It alters the selective processes that do or do not operate. Historically, there is no evidence of anything remotely similar. Therefore, should we disappear like the dinosaurs, it is unlikely that an extant species is currently waiting in the wings to replace us, and our self-constructed niche would soon permanently evaporate.
The key element in driving a human-centered MST is almost certainly the tremendous expansion of Level IV Inscribed instructional information. This continues a pattern where significant expansions in the amount of information available to organisms at any level may be critical drivers of MSTs.
One such driving event was switching Level I Encoded and Level II Epigenetic information from RNA to DNA. DNA can be ‘Functional,’ where its effects are under natural selection (Graur et al., 2015) or ‘Rubbish,’ where its effects are not under selection, but there can be individual-level costs of copying it or costs of meiotic driving elements (Burt and Trivers, 2006). The eukaryotic MET created, probably as a byproduct, genomes that appear particularly susceptible to collecting Rubbish (Graur et al., 2015). Diploidy and sexual reproduction create opportunities for intragenomic conflict that favors proliferation of transposable elements (Werren, 2011), and nuclei act as accumulators of horizontally transferred DNA (Nonacs and Tolley, 2014). Over the long-term, there can be evolutionary advantages to genomes enlarged by Rubbish, such as when mutations convert it into beneficial Functional DNA (Werren, 2011). Sexual reproduction in eukaryotes further increases the transmission of beneficial acquired genes throughout populations and more rapid elimination of harmful mutations (Lively and Morran, 2014; Sharp and Otto, 2016). Enlarged genomes can house additional Functional DNA brought in by transposable elements and viruses (Werren, 2011). It seems likely, therefore, that without all the processes that provided the raw material for creating novel Functional DNA, the evolution of higher plants, animals and fungi may have been impossible. Thus, growth in genomic information must have also facilitated the Cambrian MST.
Nevertheless, every new gene is a piece of information and theory predicts that more information is only valuable if its benefit exceeds the costs in gaining it (Stephens, 1989). This logic should also apply to gene number. Every additional functional gene adds costs for replication, making the product it codes for, and the possibility of harmful mutations. Diminishing returns with constant investment costs would set an upper bound on how much Level I information a genome could contain. Although this limits potential morphological complexity, increased levels of behavioral complexity can harness information not residing in the genome – i.e., Levels III–V. For example, humans are a MET, have undergone MCT(s), and have produced a MST – all achieved with fewer functional genes than a water flea has (Colbourne et al., 2011).
It is our extraordinarily large brain that is the MCT which gives humans access to enormous amounts of Level III and IV information and has manufactured the machines that make Level V information. A consequence of this information is that the majority of modern-day humans have exited natural ecosystems and live to varying degrees in our created technology biome. Is another MET possible in this new ecosystem? Although highly cooperative, humans are still far too individualistic to fuse into a higher level of individual, but our increasing reliance on technology could be early in the transition process for an obligate interspecific mutualism MET (Table 1). Currently, most humans rely on technology for survival and reproduction and likewise this technology would not exist or replicate without humans. In this case, the ‘mutualism’ is between a biological entity and an increasingly sophisticated abiotic entity. However, whether a machine can become part of an interspecific mutualism depends on the definition of ‘alive.’ It is conceivable that combining robotics with AI could produce machines capable of learning, gathering resources, and replication. Such entities would certainly be as sentient as bacteria and equally or more capable of evolving. The more salient question might be whether self-sustaining and self-replicating abiotic life forms would gain any benefit from mutualisms with biological ones.
By considering all the potentially interacting elements involved in producing a MST (Figure 1), it is clear that events can simultaneously fall into more than one category (MCT, MET, and FET). Similarly, categories can have variable numbers of events in them. Thus, the number of members in a category could imply something about the evolutionary likelihood of a given event. If obligate interspecific mutualisms are a type of MET (Table 1), then thousands of such METs have occurred throughout history. This strongly suggests that interspecific mutualism is not a particularly difficult problem to solve. Similarly, multicellularity and eusociality have both evolved independently multiple times, although quite a bit less often than interspecific mutualism, which seems at odds with a hypothesis that kin selection and high relatedness is a driving similarity across METs (Bourke, 2011a; West et al., 2015).
Of further interest is that not all the METs in the same category have had similar degrees of ecosystem-level impacts. For example, plants, animals and fungi are far more ecologically impactful than red algae although all are multicellular, sexual eukaryotes. Perhaps this difference resides in the particular MCTs that evolved in the former groups and not in red algae. One is left to wonder then: if red algae were the only existing complex multicellular clade, would any of their features be considered a MET, or just a curious anomaly?
On the other hand, a number of proposed METs appear to have happened only once. In the correlated triumvirate of eukaryote, sexual reproduction and multicellularity METs, only the last one appears to have evolved more than once. Similarly, nothing akin to humans appears previously in the historical record. This suggests that all of these were and are particularly unlikely events. As discussed earlier, the evolution of eukaryotes from prokaryotes might have required a particular series of fortuitous and concurrent biological innovations and catalysts, unlikely to ever be repeated. Finally, as regards the transition to humans – why has it happened only once, and taken four billion years to evolve a large-brained species capable of producing and using Level IV and V information? This remains a question to be answered.
In closing, major transitions are critical points of exploration to understand life’s history on Earth. We posit that two types of major transitions occur within species or clades: METs and MCTs. The former represents Fusions and/or Information Leaps, whereas the latter includes critical morphological innovations. Some METs and MCTs either by themselves or in concert with each other, have consequences that ripple across the globe as MSTs, altering ecosystems at large and possibly irreversible scales. The process of categorizing events into this new framework highlights the importance of facilitation and biotic and abiotic catalysts in driving ecosystem transformations. Furthermore, levels of information, from the origination of genomes to written language, and possibly machine-produced dark information, are intrinsic to facilitating major events. An interactive scheme encompassing evolutionary fusions, information leaps and morphological innovations with ecosystem changes, paints a broader and potentially more accurate picture of life’s past on Earth and provides glimpses into the future.
Data Availability Statement
The original contributions presented in the study are included in the article/supplementary material, further inquiries can be directed to the corresponding author.
Author Contributions
PN initially conceived and organized the project. ANR, KKD, ESHL, and PN led in editing and preparing the manuscript. All authors contributed to researching and writing on one or more sections.
Funding
ANR was supported by NSF-GRFP: 2017238448. ESHL was supported by NSF-GRFP: 2018262355. HPS was supported by NIH Training Grant in Genomic Analysis and Interpretation: T32HG002536.
Conflict of Interest
The authors declare that the research was conducted in the absence of any commercial or financial relationships that could be construed as a potential conflict of interest.
Publisher’s Note
All claims expressed in this article are solely those of the authors and do not necessarily represent those of their affiliated organizations, or those of the publisher, the editors and the reviewers. Any product that may be evaluated in this article, or claim that may be made by its manufacturer, is not guaranteed or endorsed by the publisher.
References
Ågren, J. A., Davies, N. G., and Foster, K. R. (2019). Enforcement is central to the evolution of cooperation. Nat. Ecol. Evol. 3, 1018–1029. doi: 10.1038/s41559-019-0907-1
Aktipis, C. A., Boddy, A. M., Jansen, G., Hibner, U., Hochberg, M. E., Maley, C. C., et al. (2015). Cancer across the tree of life: cooperation and cheating in multicellularity. Philos. Trans. R. Soc. B 370:20140219. doi: 10.1098/rstb.2014.0219
Aubret, F., Bonnet, X., and Shine, R. (2007). The role of adaptive plasticity in a major evolutionary transition: early aquatic experience affects locomotor performance of terrestrial snakes. Funct. Ecol. 21, 1154–1161. doi: 10.1111/j.1365-2435.2007.01310.x
Baluška, F., and Lyons, S. (2018a). Energide–cell body as smallest unit of eukaryotic life. Ann. Bot. 122, 741–745. doi: 10.1093/aob/mcy022
Baluška, F., and Lyons, S. (2018b). “Symbiotic origin of eukaryotic nucleus: from cell body to neo-energide,” in Concepts in Cell Biol. - History and Evolution, eds V. P. Sahi and F. Baluška ((Berlin: Springer), 39–66. doi: 10.1007/978-3-319-69944-8_3
Batra, S. W. T. (1966). Nests and social behavior of bees of India (Hymenoptera: Halictidae). Indian J. Entomol. 28, 375–393.
Baum, D. A., and Baum, B. (2014). An inside-out origin for the eukaryotic cell. BMC Biol. 12:76. doi: 10.1186/s12915-014-0076-2
Bell, P. J. L. (2001). Viral eukaryogenesis: Was the ancestor of the nucleus a complex DNA virus? J. Mol. Evol. 53, 251–256. doi: 10.1007/s002390010215
Bernhardt, H. S. (2012). The RNA world hypothesis: the worst theory of the early evolution of life (except for all the others). Biol. Direct 7:23. doi: 10.1186/1745-6150-7-23
Bernstein, H., Byers, G. S., and Michod, R. E. (1981). Evolution of sexual reproduction: importance of DNA repair, complementation, and variation. Am. Nat. 117, 537–549. doi: 10.1086/283734
Bheda, P., Kirmizis, A., and Schneider, R. (2020). The past determines the future: sugar source history and transcriptional memory. Curr. Genet. 66, 1029–1035. doi: 10.1007/s00294-020-01094-8
Biedermann, P. H. W., and Rohlfs, M. (2017). Evolutionary feedbacks between insect sociality and microbial management. Curr. Opin. Insect Sci. 22, 92–100. doi: 10.1016/j.cois.2017.06.003
Bird, A. P. (1995). Gene number, noise reduction and biological complexity. Trends Genet. 11, 94–100.
Birkhead, T. R., and Pizzari, T. (2002). Postcopulatory sexual selection. Nat. Rev. Genet. 3, 262–273.
Boomsma, J. J., and Gawne, R. (2018). Superorganismality and caste differentiation as points of no return: how the major evolutionary transitions were lost in translation: Superorganisms, eusociality and major transitions. Biol. Rev. 93, 28–54. doi: 10.1111/brv.12330
Bourke, A. F. G. (2011b). The validity and value of inclusive fitness theory. Proc. R. Soc. B 278, 3313–3320. doi: 10.1098/rspb.2011.1465
Bourke, A. F. G. (2019). Inclusive fitness and the major transitions in evolution. Curr. Opin. Insect Sci. 34, 61–67. doi: 10.1016/j.cois.2019.03.008
Boza, G., Szilágyi, A., Kun, Á., Santos, M., and Szathmáry, E. (2014). Evolution of the division of labor between genes and enzymes in the RNA world. PLoS Comp. Biol. 1:e1003936. doi: 10.1371/journal.pcbi.1003936
Bresch, C., Niesert, U., and Harnasch, D. (1980). Hypercycles, parasites and packages. J. Theor. Biol. 85, 399–405. doi: 10.1016/0022-5193(80)90314-8
Brummitt, C. D., Gomez-Lievano, A., Hausmann, R., and Bonds, M. H. (2020). Machine-learned patterns suggest that diversification drives economic development. J. R. Soc. Interface 17:20190283. doi: 10.1098/rsif.2019.0283
Budd, G. E., and Jensen, S. (2000). A critical reappraisal of the fossil record of the bilaterian phyla. Biol. Rev. Camb. Philos. Soc. 75, 253–295. doi: 10.1017/s000632310000548x
Burt, A., and Trivers, R. (2006). Genes in Conflict: The Biology of Selfish Genetic Elements. Cambridge, MA: Harvard University Press.
Butterfield, N. J. (2000). Bangiomorpha pubescens n. gen., n. sp.: implications for the evolution of sex, multicellularity, and the Mesoproterozoic/Neoproterozoic radiation of eukaryotes. Paleobiology 26, 386–404. doi: 10.1666/0094-8373(2000)026<0386:bpngns>2.0.co;2
Butterfield, N. J. (2015). Early evolution of the Eukaryota. Paleontology 58, 5–17. doi: 10.1111/pala.12139
Butterfield, N. J. (2018). Oxygen, animals and aquatic bioturbation: an updated account. Geobiology 16, 3–16. doi: 10.1111/gbi.12267
Byrne, R. W., Cartmill, E., Genty, E., Graham, K. E., Hobaiter, C., and Tanner, J. (2017). Great ape gestures: intentional communication with a rich set of innate signals. Anim. Cogn. 20, 755–769.
Cai, L., Fisher, A. L., Huang, H., and Xie, Z. (2016). CRISPR-mediated genome editing and human diseases. Genes Dis. 3, 244–251. doi: 10.1016/j.gendis.2016.07.003
Calcott, B., and Sterelny, K. (2011). The Major Transitions in Evolution Revisted. Cambridge, MA: MIT Press.
Cardinal, S., and Danforth, B. N. (2011). The antiquity and evolutionary history of social behavior in bees. PLoS One 6:e21086. doi: 10.1371/journal.pone.0021086
Casadesús, J., and Low, D. (2006). Epigenetic gene regulation in the bacterial world. Microbiol. Mol. Biol. Rev. 70, 830–856.
Cavalier-Smith, T. (1987). The origin of eukaryote and archaebacterial cells. Ann. N. Y. Acad. Sci. 503, 17–54. doi: 10.1111/j.1749-6632.1987.tb40596.x
Cavalier-Smith, T. (2002). Origins of the machinery of recombination and sex. Heredity 88, 125–141. doi: 10.1038/sj.hdy.6800034
Cavalier-Smith, T. (2009). Predation and eukaryote cell origins: a coevolutionary perspective. Int. J. Biochem. Cell Biol. 41, 307–322. doi: 10.1016/j.biocel.2008.10.002
Cavalli-Sforza, L. L., and Feldman, M. W. (1981). Cultural Transmission and Evolution. Princeton, NJ: Princeton University Press.
Chou, Y., Vafabakhsh, R., Doğanay, S., Gao, Q., Ha, T., and Palese, P. (2012). One influenza virus particle packages eight unique viral RNAs as shown by FISH analysis. Proc. Natl. Acad. Sci. U.S.A. 109, 9101–9106. doi: 10.1073/pnas.1206069109
Clark, T. (2018). HAP2/GCS1: mounting evidence of our true biological EVE? PLoS Biol. 16:e3000007. doi: 10.1371/journal.pbio.3000007
Colbourne, J. K., Pfrender, M. E., Gilbert, D., Thomas, W. K., Tucker, A., Oakley, T. H., et al. (2011). The ecoresponsive genome of Daphnia pulex. Science 331, 555–561.
Creanza, N., Kolodny, O., and Feldman, M. W. (2017). Cultural evolutionary theory: How culture evolves and why it matters. Proc. Natl. Acad. Sci. U.S.A. 114, 7782–7789. doi: 10.1073/pnas.1620732114
da Silva, J. (2018). The evolution of sexes: a specific test of the disruptive selection theory. Ecol. Evol. 8, 207–219. doi: 10.1002/ece3.3656
Danchin, É., and Wagner, R. H. (2010). Inclusive heritability: combining genetic and non-genetic information to study animal behavior and culture. Oikos 119, 210–218.
Danforth, B. N., Conway, L., and Ji, S. (2003). Phylogeny of eusocial Lasioglossum reveals multiple losses of eusociality within a primitively eusocial clade of bees (Hymenoptera: Halictidae). Syst. Biol. 52, 23–36. doi: 10.1080/10635150390132687
Dastbaz, M. (2019). “Industry 4.0 (i4.0): the hype, the reality, and the challenges ahead,” in Industry 4.0 and Engineering for a Sustainable Future, eds M. Dastbaz and P. Cochrane (Berlin: Springer), 1–11. doi: 10.1007/978-3-030-12953-8_1
Diehl, A. G., Ouyang, N., and Boyle, A. P. (2020). Transposable elements contribute to cell and species-specific chromatin looping and gene regulation in mammalian genomes. Nat. Commun. 11:1796. doi: 10.1038/s41467-020-15520-5
Durzyńska, J., and Goździcka-Józefiak, A. (2015). Viruses and cells intertwined since the dawn of evolution. Virol. J. 12:169. doi: 10.1186/s12985-015-0400-7
Ebrahimi, S., and Nonacs, P. (2021). Genetic diversity through social heterosis can increase virulence in RNA viral infections and cancer progression. R. Soc. Open Sci. 8:202219. doi: 10.1098/rsos.202219
Eigen, M. (1971). Selforganization of matter and the evolution of biological macromolecules. Naturwissenschaften 58, 465–523. doi: 10.1007/bf00623322
Eigen, M., Gardiner, W., Schuster, P., and Winkler-Oswatitsch, R. (1981). The origin of genetic information. Sci. Am. 244, 88–119. doi: 10.1038/scientificamerican0481-88
Eigen, M., and Schuster, P. (1979). The Hypercycle: A Principle of Natural Self-Organization. Berlin: Springer-Verlag.
Fagerström, T., Briscoe, D. A., and Sunnucks, P. (1998). Evolution of mitotic cell-lineages in multicellular organisms. Trends Ecol. Evol. 13, 117–120. doi: 10.1016/s0169-5347(97)01314-1
Feldman, M. W., and Cavalli-Sforza, L. L. (1976). Cultural and biological evolutionary processes, selection for a trait under complex transmission. Theor. Pop. Biol. 9, 238–259. doi: 10.1016/0040-5809(76)90047-2
Feldman, M. W., and Laland, K. N. (1996). Gene-culture coevolutionary theory. Trends Ecol. Evol. 11, 453–457.
Flot, J.-F., Hespeels, B., Li, X., Noel, B., Arkhipova, I., Danchin, E. G. J., et al. (2013). Genomic evidence for ameiotic evolution in the bdelloid rotifer Adineta vaga. Nature 500, 453–457.
Forterre, P. (2002). The origin of DNA genomes and DNA replication proteins. Curr. Opin. Microbiol. 5, 525–532. doi: 10.1016/s1369-5274(02)00360-0
Forterre, P. (2011). A new fusion hypothesis for the origin of Eukarya: better than previous ones, but probably also wrong. Res. Microbiol. 162, 77–91. doi: 10.1016/j.resmic.2010.10.005
Forterre, P., and Prangishvili, D. (2013). The major role of viruses in cellular evolution: facts and hypotheses. Curr. Opin. Virol. 3, 558–565. doi: 10.1016/j.coviro.2013.06.013
Gold, D. A., and Jacobs, D. K. (2013). Stem cell dynamics in Cnidaria: are there unifying principles? Dev. Genes Evol. 223, 53–66. doi: 10.1007/s00427-012-0429-1
González-Forero, M., and Gardner, A. (2018). Inference of ecological and social drivers of human brain-size evolution. Nature 557, 554–557. doi: 10.1038/s41586-018-0127-x
Goodenough, U., and Heitman, J. (2014). Origins of eukaryotic sexual reproduction. Cold Spring Harb. Perspect. Biol. 6:a016154. doi: 10.1101/cshperspect.a016154
Gowdy, J., and Krall, L. (2014). Agriculture as a major evolutionary transition to human ultrasociality. J. Bioecon. 16, 179–202. doi: 10.1007/s10818-013-9156-6
Graur, D., Zheng, Y., and Azevedo, R. B. (2015). An evolutionary classification of genomic function. Genome Biol. Evol. 8, 642–645.
Greenwald, G., and MacAskill, E. (2013). NSA Prism program taps into user data of Apple, Google and others. Guardian 6, 1–43.
Grey, D., Hutson, V., and Szathmáry, E. (1995). A re-examination of the stochastic corrector model. Proc. R. Soc. Lond. B 262, 29–35. doi: 10.1098/rspb.1995.0172
Haig, D. (2015). Maternal–fetal conflict, genomic imprinting and mammalian vulnerabilities to cancer. Philos. Trans. R. Soc. B 370:20140178. doi: 10.1098/rstb.2014.0178
Hammerschmidt, K., and Rose, C. J. (2021). What do we mean by multicellularity? The Evolutionary Transitions Framework provides answers. Front. Ecol. Evol. 9:730714. doi: 10.3389/fevo.2021.730714
Hamner, W. M., and Jenssen, R. M. (1974). Growth, degrowth, and irreversible cell differentiation in Aurelia aurita. Am. Zool. 14, 833–849. doi: 10.1093/icb/14.2.833
Hanschen, E. R., Herron, M. D., Wiens, J. J., Nozaki, H., and Michod, R. E. (2018). Multicellularity drives the evolution of sexual traits. Am. Nat. 192, E93–E105.
Harris, D., and Bullock, S. (2002). “Enhancing game theory with coevolutionary simulation models of honest signalling,” in Proceedings of the 2002 Congress on Evolutionary Computation, Washington, DC, 1594–1599.
Hecker, S., Dai, D., and Van Gool, L. (2018). “End-to-end learning of driving models with surround-view cameras and route planners,” in Proceedings of the 2018 European Conference on Computer Vision, Munich, 435–453.
Hernández, J. M., and Podbilewicz, B. (2017). The hallmarks of cell-cell fusion. Development 144, 4481–4495. doi: 10.1242/dev.155523
Herrmann, E., Call, J., Hernandez-Lloreda, M. V., Hare, B., and Tomasello, M. (2007). Humans have evolved specialized skills of social cognition: the cultural intelligence hypothesis. Science 317, 1360–1366. doi: 10.1126/science.1146282
Hogeweg, P., and Takeuchi, N. (2003). Multilevel selection in models of prebiotic evolution: compartments and spatial self-organization. Origins Life Evol. Biosphere 33, 375–403. doi: 10.1023/a:1025754907141
Ibarra, D. E., Rugenstein, J. K. C., Bachan, A., Baresch, A., Lau, K. V., Thomas, D. L., et al. (2019). Modeling the consequences of land plant evolution on silicate weathering. Am. J. Sci. 319, 1–43. doi: 10.2475/01.2019.01
Jablonka, E., and Lamb, M. J. (2006). The evolution of information in the major transitions. J. Theor. Biol. 239, 236–246.
Jablonka, E., Lamb, M. J., and Avital, E. (1998). ‘Lamarckian’ mechanisms in darwinian evolution. Trends Ecol. Evol. 13, 206–210. doi: 10.1016/s0169-5347(98)01344-5
Jablonka, E., and Szathmáry, E. (1995). The evolution of information storage and heredity. Trends Ecol. Evol. 10, 206–211.
Jan, N., Stauffer, D., and Moseley, L. (2000). A hypothesis for the evolution of sex. Theory Biosci. 119, 166–168. doi: 10.1007/s12064-000-0013-5
Juliandi, B., Abematsu, M., and Nakashima, K. (2010). Epigenetic regulation in neural stem cell differentiation. Dev. Growth Differ. 52, 493–504. doi: 10.1111/j.1440-169x.2010.01175.x
Kapheim, K. M., Nonacs, P., Smith, A. R., Wayne, R. K., and Wcislo, W. T. (2015). Kinship, parental manipulation and evolutionary origins of eusociality. Proc. R. Soc. Lond. B 282:20142886. doi: 10.1098/rspb.2014.2886
Kapheim, K. M., Smith, A. R., Ihle, K. E., Amdam, G. V., Nonacs, P., and Wcislo, W. T. (2012). Physiological variation as a mechanism for developmental caste-biasing in a facultatively eusocial sweat bee. Proc. R. Soc. Lond. B 279, 1437–1446. doi: 10.1098/rspb.2011.1652
Karnkowska, A., Vacek, V., Zubáèová, Z., Treitli, S. C., Petrželková, R., Lukáš, L. E., et al. (2016). A eukaryote without a mitochondrial organelle. Curr. Biol. 26, 1274–1284. doi: 10.1016/j.cub.2016.03.053
Kaup, A. R., Mirzakhanian, H., Jeste, D. V., and Eyler, L. T. (2011). A review of the brain structure correlates of successful cognitive aging. J. Neuropsychiatry Clin. Neurosci. 23, 6–15. doi: 10.1176/appi.neuropsych.23.1.6
Kawashima, T., and Berger, F. (2014). Epigenetic reprogramming in plant sexual reproduction. Nat. Rev. Genet. 15, 613–624. doi: 10.1038/nrg3685
Keren, H., Lev-Maor, G., and Ast, G. (2010). Alternative splicing and evolution: diversification, exon definition and function. Nat. Rev. Genet. 11, 345–355. doi: 10.1038/nrg2776
King, J. R., Warren, R. J., and Bradford, M. A. (2013). Social insects dominate Eastern US temperate hardwood forest macroinvertebrate communities in warmer regions. PLoS One 10:e75843. doi: 10.1371/journal.pone.0075843
Knoll, A. H. (2011). The multiple origins of complex multicellularity. Annu. Rev. Earth Planet. Sci. 39, 217–239.
Knoll, A. H., and Bambach, R. K. (2000). Directionality in the history of life: diffusion from the left wall or repeated scaling of the right? Paleobiology 26, 1–14.
Knoll, A. H., Javaux, E. J., Hewitt, D., and Cohen, P. (2006). Eukaryotic organisms in Proterozoic oceans. Philos. Trans. R. Soc. B 361, 1023–1038.
Knoll, A. H., and Lahr, D. J. G. (2016). Fossils, Feeding and the Evolution of Complex Multicellularity. Cambridge, MA: MIT Press.
Koonin, E. V. (2016). Viruses and mobile elements as drivers of evolutionary transitions. Philos. Trans. R. Soc. B 371:20150442. doi: 10.1098/rstb.2015.0442
Kotrschal, A., Rogell, B., Bundsen, A., Svensson, B., Zajitschek, S., Brännström, I., et al. (2013). Artificial selection on relative brain size in the guppy reveals costs and benefits of evolving a larger brain. Curr. Biol. 23, 168–171. doi: 10.1016/j.cub.2012.11.058
Kumano, G. (2015). Evolution of germline segregation processes in animal development. Dev. Growth Differ. 57, 324–332. doi: 10.1111/dgd.12211
Kun, Á., Santos, M., and Szathmáry, E. (2005). Real ribozymes suggest a relaxed error threshold. Nat. Genet. 37, 1008–1011. doi: 10.1038/ng1621
Kundu, S., Horn, P. J., and Peterson, C. L. (2007). SWI/SNF is required for transcriptional memory at the yeast GAL gene cluster. Genes Dev. 21, 997–1004. doi: 10.1101/gad.1506607
Kuzawa, C. W., Chugani, H. T., Grossman, L. I., Lipovich, L., Muzik, O., Hof, P. R., et al. (2014). Metabolic costs and evolutionary implications of human brain development. Proc. Natl. Acad. Sci. U.S.A. 111, 13010–13015. doi: 10.1073/pnas.1323099111
Lake, J. A., and Rivera, M. C. (1994). Was the nucleus the first endosymbiont? Proc. Natl. Acad. Sci. U.S.A. 91, 2880–2881.
Lane, N. (2011). Energetics and genetics across the prokaryote-eukaryote divide. Biol. Direct 6:35. doi: 10.1186/1745-6150-6-35
Lee, H., and Cho, C. H. (2020). Digital advertising: present and future prospects. Int. J. Advert. 39, 332–341.
Levin, S. R., and West, S. A. (2017). Kin selection in the RNA world. Life 7:53. doi: 10.3390/life7040053
Liebert, A. E., Nonacs, P., and Wayne, R. K. (2005). Solitary nesting and reproductive success in the paper wasp Polistes aurifer. Behav. Ecol. Sociobiol. 57, 445–456. doi: 10.1007/s00265-004-0875-5
Lisitsyna, O. M., and Sheval, E. V. (2016). Origin and early evolution of the nuclear envelope. Biochem. Supp. Ser. A 10, 251–258. doi: 10.1134/s1990747816030156
Lively, C. M., and Morran, L. T. (2014). The ecology of sexual reproduction. J. Evol. Biol. 27, 1292–1303.
Ludt, K., and Soppa, J. (2019). Polyploidy in halophilic archaea: regulation, evolutionary advantages, and gene conversion. Biochem. Soc. Trans. 47, 933–944.
Lynch, M., and Conery, J. S. (2003). The origins of genome complexity. Science 302, 1401–1404. doi: 10.1126/science.1089370
Lyons, T. W., Reinhard, C. T., and Planavsky, N. J. (2014). The rise of oxygen in Earth’s early ocean and atmosphere. Nature 506, 307–315. doi: 10.1038/nature13068
Manrubia, S. C., Domingo, E., and Lázaro, E. (2010). Pathways to extinction: Beyond the error threshold. Philos. Trans. R. Soc. B. 365, 1943–1952. doi: 10.1098/rstb.2010.0076
Margulis, L., Dolan, M. F., and Guerrero, R. (2000). The chimeric eukaryote: Origin of the nucleus from the karyomastigont in amitochondriate protists. Proc. Natl. Acad. Sci. U.S.A. 97, 6954–6959. doi: 10.1073/pnas.97.13.6954
Margulis, L., and Fester, R. (1991). Symbiosis as a Source of Evolutionary Innovation. Cambridge, MA: MIT Press.
Markov, A. V., and Kaznacheev, I. S. (2016). Evolutionary consequences of polyploidy in prokaryotes and the origin of mitosis and meiosis. Biol. Direct 11:28. doi: 10.1186/s13062-016-0131-8
Martin, W., and Koonin, E. V. (2006). Introns and the origin of nucleus–cytosol compartmentalization. Nature 440, 41–45. doi: 10.1038/nature04531
Maynard Smith, J., and Szathmáry, E. (1993). The origin of chromosomes I. Selection for linkage. J. Theor. Biol. 164, 437–446.
Maynard Smith, J., and Szathmáry, E. (1995). The Major Transitions in Evolution. Oxford: Oxford University Press.
McMenamin, M. A. S. (1988). Paleoecological feedback and the Vendian-Cambrian transition. Trends Ecol. Evol. 3, 205–208. doi: 10.1016/0169-5347(88)90008-0
McShea, D. W., and Simpson, C. (2011). “The miscellaneous transitions in evolution,” in The Major Transitions in Evolution Revisted, eds B. Calcott and K. Sterelny (Cambridge, MA: MIT Press), 19–34. doi: 10.7551/mitpress/9780262015240.003.0002
Mereschkowsky, C. (1905). Uber Natur und Ursprung der Chromatophoren im Pflanzenreiche. Biologisch. Centralblatt 25, 293–604.
Mesoudi, A. (2017). Pursuing Darwin’s curious parallel: prospects for a science of cultural evolution. Proc. Natl. Acad. Sci. U.S.A. 114, 7853–7860. doi: 10.1073/pnas.1620741114
Michod, R. E. (1983). Population biology of the first replicators: on the origin of the genotype, phenotype and organism. Am. Zool. 23, 5–14. doi: 10.1093/icb/23.1.5
Michod, R. E. (1996). Cooperation and conflict in the evolution of individuality. II. Conflict mediation. Proc. R. Soc. Lond. B. 263, 813–822. doi: 10.1098/rspb.1996.0121
Michod, R. E. (2011). “Evolutionary transitions in individuality, multicellularity, and sex,” in The Major Transitions in Evolution Revisted, eds B. Calcott and K. Sterelny (Cambridge, MA: MIT Press), 169–198. doi: 10.7551/mitpress/9780262015240.003.0010
Moreau, C. S., Bell, C. D., Vila, R., Archibald, S. B., and Pierce, N. E. (2006). Phylogeny of the ants: diversification in the age of angiosperms. Science 312, 101–104. doi: 10.1126/science.1124891
Navarro, D. J., Perfors, A., Kary, A., Brown, S. D., and Donkin, C. (2018). When extremists win: cultural transmission via iterated learning when populations are heterogeneous. Cogn. Sci. 42, 2108–2149. doi: 10.1111/cogs.12667
Niklas, K. J. (2014). The evolutionary-developmental origins of multicellularity. Am. J. Bot. 101, 6–25.
Niklas, K. J., Cobb, E. D., and Kutschera, U. (2014). Did meiosis evolve before sex and the evolution of eukaryotic life cycles? Bioessays 36, 1091–1101.
Nonacs, P. (2017). Go high or go low? Adaptive evolution of high and low relatedness societies in social hymenoptera. Front. Ecol. Evol. 5:87. doi: 10.3389/fevo.2017.00087
Nonacs, P., and Kapheim, K. M. (2007). Social heterosis and the maintenance of genetic diversity. J. Evol. Biol. 20, 2253–2265.
Nonacs, P., and Tolley, S. J. (2014). Certainty versus stochasticity: cell replication biases DNA movement from endosymbionts and organelles into nuclei. Evol. Ecol. Res. 16, 195–202.
Nowak, M. A. (2006). Five rules for the evolution of cooperation. Science 314, 1560–1563. doi: 10.1126/science.1133755
Nowak, M. A., Tarnita, C. E., and Wilson, E. O. (2010). The evolution of eusociality. Nature 466, 1057–1062.
Nursall, J. (1959). Oxygen as a prerequisite to the origin of the Metazoa. Nature 183, 1170–1172. doi: 10.1038/1831170b0
Obermeyer, Z., and Emanuel, E. J. (2016). Predicting the future — big data, machine learning, and clinical medicine. N. Engl. J. Med. 375, 1216–1219.
Och, L. M., and Shields-Zhou, G. A. (2012). The Neoproterozoic oxygenation event: environmental perturbations and biogeochemical cycling. Earth Sci. Rev. 110, 26–57. doi: 10.1111/gbi.12230
Oldroyd, B. P. (2002). The Cape honeybee: an example of a social cancer. Trends Ecol. Evol. 17, 249–251. doi: 10.1016/s0169-5347(02)02479-5
Pacheco, J. M., Santos, F. C., and Dingli, D. (2014). The ecology of cancer from an evolutionary game theory perspective. Interface Focus 4:20140019.
Parker, G. A., Baker, R. R., and Smith, V. G. F. (1972). The origin and evolution of gamete dimorphism and the male-female phenomenon. J. Theor. Biol. 36, 529–553. doi: 10.1016/0022-5193(72)90007-0
Payne, S., Facciotti, M., Cott, K. V., Yao, A., Wilson, M., Sutter, S., et al. (2019). Conservation of an ancient oxidation response that controls archaeal epigenetic traits through chromatin protein networks. bioRχiv [Preprint]. doi: 10.1101/801316
Piraino, S., De Vito, D., Schmich, J., Bouillon, J., and Boero, F. (2004). Reverse development in Cnidaria. Can. J. Zool. 82, 1748–1754. doi: 10.1139/z04-174
Qiu, Y. (2008). Phylogeny and evolution of charophytic algae and land plants. J. Syst. Evol. 46, 287–306.
Queller, D. C. (2000). Relatedness and the fraternal major transitions. Phil. Trans. R. Soc. Lond. B 355, 1647–1655. doi: 10.1098/rstb.2000.0727
Rabeling, C., and Kronauer, D. J. C. (2013). Thelytokous parthenogenesis in eusocial hymenoptera. Annu. Rev. Entomol. 58, 273–292. doi: 10.1146/annurev-ento-120811-153710
Rainey, P. B., and Kerr, B. (2011). “Conflicts among levels of selection as fuel for the evolution of individuality,” in The Major Transitions in Evolution Revisted, eds B. Calcott and K. Sterelny (Cambridge, MA: MIT Press), 141–162. doi: 10.1186/s12916-017-0900-y
Real, E., Liang, C., So, D. R., and Le, Q. V. (2020). AutoML-Zero: evolving machine learning algorithms from scratch. arXiv [Preprint]. Available online at: https://arxiv.org/abs/2003.03384
Reeve, H. K., and Jeanne, R. L. (2003). From individual control to majority rule: extending transactional models of reproductive skew in animal societies. Proc. R. Soc. Lond. B 270, 1041–1045. doi: 10.1098/rspb.2002.2253
Rehan, S. M., Richards, M. H., Adams, M., and Schwarz, M. P. (2014). The costs and benefits of sociality in a facultatively social bee. Anim. Behav. 97, 77–85. doi: 10.1098/rspb.2021.0033
Reik, W., and Walter, J. (2001). Genomic imprinting: parental influence on the genome. Nat. Rev. Genet. 2, 21–32.
Saakian, D. B., Biebricher, C. K., and Hu, C.-K. (2011). Lethal mutants and truncated selection together solve a paradox of the origin of life. PLoS One 6:e21904. doi: 10.1371/journal.pone.0021904
Sanchez-Lengeling, B., and Aspuru-Guzik, A. (2018). Inverse molecular design using machine learning: generative models for matter engineering. Science 361, 360–365. doi: 10.1126/science.aat2663
Santos, M., Zintzaras, E., and Szathmáry, E. (2002). Origin of sex revisited. Orig. Life Evol. Biosph. 33, 305–432.
Schel, A. M., Townsend, S. W., Machanda, Z., Zuberbühler, K., and Slocombe, K. E. (2013). Chimpanzee alarm call production meets key criteria for intentionality. PLoS One 8:e76674. doi: 10.1371/journal.pone.0076674
Schlesinger, W. H., and Jasechko, S. (2014). Transpiration in the global water cycle. Agric. For. Meteorol. 189, 115–117. doi: 10.1016/j.agrformet.2014.01.011
Schwab, J. A., Young, M. T., Neenan, J. M., Walsh, S. A., Witmer, L. M., Herrera, Y., et al. (2020). Inner ear sensory system changes as extinct crocodylomorphs transitioned from land to water. Proc. Natl. Acad. Sci. U.S.A. 117, 10422–10428. doi: 10.1073/pnas.2002146117
Seyfarth, R. M., Cheney, D. L., and Bergman, T. J. (2005). Primate social cognition and the origins of language. Trends Cogn. Sci. 9, 264–266. doi: 10.1016/j.tics.2005.04.001
Sharp, N. P., and Otto, S. P. (2016). Evolution of sex: using experimental genomics to select among competing theories. Bioessays 38, 751–757. doi: 10.1002/bies.201600074
Sheehan, P. M., and Harris, M. T. (2004). Microbialite resurgence after the Late Ordovician extinction. Nature 430, 75–78. doi: 10.1038/nature02654
Song, T., Cao, S., Tao, S., Liang, S., Du, W., and Liang, Y. (2017). A novel unsupervised algorithm for biological process-based analysis on cancer. Sci. Rep. 7:4671. doi: 10.1038/s41598-017-04961-6
Soppa, J. (2014). Polyploidy in Archaea and Bacteria: about desiccation resistance, giant cell size, long-term survival, enforcement by a eukaryotic host and additional aspects. J. Mol. Microbiol. Biotechnol. 24, 409–419. doi: 10.1159/000368855
Spang, A., Saw, J. H., Jørgensen, S. L., Zaremba-Niedzwiedzka, K., Martijn, J., Lind, A. E., et al. (2015). Complex archaea that bridge the gap between prokaryotes and eukaryotes. Nature 521, 173–179.
Staps, M., van Gestel, J., and Tarnita, C. E. (2021). “Life cycles as a central organizing theme for studying multicellularity,” in The Evolution of Multicellularity, eds M. D. Herron, P. L. Conlin, and W. C. Ratcliff (Boca Raton, FL: CRC Press).
Stebbins, G. L. (1969). The Basis of Progressive Evolution. Chapel Hill, NC: University North Carolina Press.
Stephens, D. W. (1989). Variance and the value of information. Am. Nat. 134, 128–140. doi: 10.1086/284969
Stock, J. T. (2008). Are humans still evolving? Technological advances and unique biological characteristics allow us to adapt to environmental stress. Has this stopped genetic evolution? EMBO Rep. 9, S51–S54. doi: 10.1038/embor.2008.63
Summers, J., and Litwin, S. (2006). Examining the theory of error catastrophe. J. Virol. 80, 20–26. doi: 10.1128/jvi.80.1.20-26.2006
Sumner, S., Nash, D. R., and Boomsma, J. J. (2003). The adaptive significance of inquiline parasite workers. Proc. R. Soc. Lond. B 270, 1315–1322. doi: 10.1098/rspb.2003.2362
Sun, W., Yin, Z., Cunningham, J. A., Liu, P., Zhu, M., and Donoghue, P. C. J. (2020). Nucleus preservation in early Ediacaran Weng’an embryo-like fossils, experimental taphonomy of nuclei and implications for reading the eukaryote fossil record. Interface Focus 10:20200015. doi: 10.1098/rsfs.2020.0015
Szathmáry, E. (2006). The origin of replicators and reproducers. Philos. Trans. R. Soc. B 361, 1761–1776. doi: 10.1098/rstb.2006.1912
Szathmáry, E. (2010). “Evolution of language as one of the major evolutionary transitions,” in Evolution of Communication and Language in Embodied Agents, eds S. Nolfi and M. Mirolli (Berlin: Springer), 37–53. doi: 10.1007/978-3-642-01250-1_3
Szathmáry, E. (2015). Toward major evolutionary transitions theory 2.0. Proc. Natl. Acad. Sci. U.S.A. 112, 10104–10111. doi: 10.1073/pnas.1421398112
Szathmáry, E., and Demeter, L. (1987). Group selection of early replicators and the origin of life. J. Theor. Biol. 128, 463–486.
Szathmáry, E., and Maynard Smith, J. (1995). The major evolutionary transitions. Nature 374, 227–232.
Takemura, M. (2001). Poxviruses and the origin of the eukaryotic nucleus. J. Mol. Evol. 52, 419–425. doi: 10.1007/s002390010171
Tarnita, C. E. (2017). The ecology and evolution of social behavior in microbes. J. Exp. Biol. 220, 18–24. doi: 10.1242/jeb.145631
Teagle, H., Hawkins, S. J., Moore, P. J., and Smale, D. A. (2017). The role of kelp species as biogenic habitat formers in coastal marine ecosystems. J. Exp. Mar. Biol. Ecol. 492, 81–98. doi: 10.1016/j.jembe.2017.01.017
Trigos, A., Pearson, R., Papenfuss, A., and Goode, D. (2019). Somatic mutations in early metazoan genes disrupt regulatory links between unicellular and multicellular genes in cancer. eLife 8:e40947. doi: 10.7554/eLife.40947
Trivers, R., and Hare, H. (1976). Haploidploidy and the evolution of the social insect. Science 191, 249–263. doi: 10.1126/science.1108197
Tüzel, E., Sevim, V., and Erzan, A. (2001). Evolutionary route to diploidy and sex. Proc. Natl. Acad. Sci. U.S.A. 98, 13774–13777. doi: 10.1073/pnas.241105498
Valansi, C., Moi, D., Leikina, E., Matveev, E., Graña, M., Chernomordik, L. V., et al. (2017). Arabidopsis HAP2/GCS1 is a gamete fusion protein homologous to somatic and viral fusogens. J. Cell Biol. 216, 571–581. doi: 10.1083/jcb.201610093
Van de Peer, Y., Mizrachi, E., and Marchal, K. (2017). The evolutionary significance of polyploidy. Nat. Rev. Genet. 18, 411–424. doi: 10.1038/nrg.2017.26
Van Etten, J., and Bhattacharya, D. (2020). Horizontal gene transfer in eukaryotes: Not if, but how much? Trends Genet. 36, 915–925. doi: 10.1016/j.tig.2020.08.006
Van Valen, L. (1973). A new evolutionary law. Evol. Theory 1, 1–30. doi: 10.4337/9781785361302.00005
Velicer, G. J., and Yu, Y. N. (2003). Evolution of novel cooperative swarming in the bacterium Myxococcus xanthus. Nature 425, 75–78. doi: 10.1038/nature01908
Walter, M. R., and Heys, G. R. (1985). Links between the rise of the metazoa and the decline of stromatolites. Precambrian Res. 29, 149–174. doi: 10.1016/0301-9268(85)90066-x
Wang, S. Y., Pollina, E. A., Wang, I. H., Pino, L. K., Bushnell, H. L., Takashima, K., et al. (2021). Role of epigenetics in unicellular to multicellular transition in Dictyostelium. Genome Biol. 22, 1–30. doi: 10.1186/s13059-021-02360-9
Wei, Z., Yoshimura, K., Wang, L., Miralles, D. G., Jasechko, S., and Lee, X. (2017). Revisiting the contribution of transpiration to global terrestrial evapotranspiration. Geophys. Res. Lett. 44, 2792–2801. doi: 10.1002/2016gl072235
Weiner, A. M., and Maizels, N. (1987). tRNA-like structures tag the 3’ ends of genomic RNA molecules for replication: implications for the origin of protein synthesis. Proc. Natl. Acad. Sci. U.S.A. 84, 7383–7387. doi: 10.1073/pnas.84.21.7383
Werren, J. H. (2011). Selfish genetic elements, genetic conflict, and evolutionary innovation. Proc. Natl. Acad. Sci. U.S.A. 108, 10863–10870. doi: 10.1073/pnas.1102343108
West, S. A., Fisher, R. M., Gardner, A., and Kiers, E. T. (2015). Major evolutionary transitions in individuality. Proc. Natl. Acad. Sci. U.S.A. 112, 10112– 10119.
Willbanks, A., Leary, M., Greenshields, M., Tyminski, C., Heerboth, S., Lapinska, K., et al. (2016). The evolution of epigenetics: from prokaryotes to humans and its biological consequences. Genet. Epigenet. 8, 25–36.
Wilson, E. E., and Holway, D. A. (2010). Multiple mechanisms underlie displacement of solitary Hawaiian Hymenoptera by an invasive social wasp. Ecology 91, 3294–3302. doi: 10.1890/09-1187.1
Wilson, E. O. (1990). Success and Dominance in Ecosystems: The Case of Social Insects. Berlin: Ecology Institute.
Wilson, E. O. (1992). The effects of complex social-life on evolution and biodiversity. Oikos 63, 13–18. doi: 10.2307/3545511
Wilson, E. O., and Hölldobler, B. (2005). Eusociality: origin and consequences. Proc. Natl. Acad. Sci. U.S.A. 102, 13367–13371.
Wolf, Y. I., and Koonin, E. V. (2007). On the origin of the translation system and the genetic code in the RNA world by means of natural selection, exaptation, and subfunctionalization. Biol. Direct 2:14. doi: 10.1186/1745-6150-2-14
Wolpert, L., and Szathmáry, E. (2002). Evolution and the egg multicellularity. Nature 420, 745–745. doi: 10.1038/420745a
Xu, Z., Frankwick, G. L., and Ramirez, E. (2016). Effects of big data analytics and traditional marketing analytics on new product success: a knowledge fusion perspective. J. Bus. Res. 69, 1562–1566.
Keywords: adaptation, innovation, facilitation, information, ecosystem
Citation: Robin AN, Denton KK, Horna Lowell ES, Dulay T, Ebrahimi S, Johnson GC, Mai D, O’Fallon S, Philson CS, Speck HP, Zhang XP and Nonacs P (2021) Major Evolutionary Transitions and the Roles of Facilitation and Information in Ecosystem Transformations. Front. Ecol. Evol. 9:711556. doi: 10.3389/fevo.2021.711556
Received: 31 May 2021; Accepted: 09 November 2021;
Published: 09 December 2021.
Edited by:
Alessandro Minelli, University of Padua, ItalyReviewed by:
Sebastian Leuzinger, Auckland University of Technology, New ZealandGwendolyn Peyre, University of Los Andes, Colombia
Copyright © 2021 Robin, Denton, Horna Lowell, Dulay, Ebrahimi, Johnson, Mai, O’Fallon, Philson, Speck, Zhang and Nonacs. This is an open-access article distributed under the terms of the Creative Commons Attribution License (CC BY). The use, distribution or reproduction in other forums is permitted, provided the original author(s) and the copyright owner(s) are credited and that the original publication in this journal is cited, in accordance with accepted academic practice. No use, distribution or reproduction is permitted which does not comply with these terms.
*Correspondence: Peter Nonacs, pnonacs@biology.ucla.edu