- 1Environmental and Life Sciences Graduate Program, Trent University, Peterborough, ON, Canada
- 2Mammals Research Section, Colorado Parks and Wildlife, Fort Collins, CO, United States
- 3Department of Biology, York University, Toronto, ON, Canada
- 4Department of Fish, Wildlife and Conservation Biology, Colorado State University, Fort Collins, CO, United States
- 5Ontario Ministry of Natural Resources and Forestry, Peterborough, ON, Canada
Understanding how animals use information about their environment to make movement decisions underpins our ability to explain drivers of and predict animal movement. Memory is the cognitive process that allows species to store information about experienced landscapes, however, remains an understudied topic in movement ecology. By studying how species select for familiar locations, visited recently and in the past, we can gain insight to how they store and use local information in multiple memory types. In this study, we analyzed the movements of a migratory mule deer (Odocoileus hemionus) population in the Piceance Basin of Colorado, United States to investigate the influence of spatial experience over different time scales on seasonal range habitat selection. We inferred the influence of short and long-term memory from the contribution to habitat selection of previous space use within the same season and during the prior year, respectively. We fit step-selection functions to GPS collar data from 32 female deer and tested the predictive ability of covariates representing current environmental conditions and both metrics of previous space use on habitat selection, inferring the latter as the influence of memory within and between seasons (summer vs. winter). Across individuals, models incorporating covariates representing both recent and past experience and environmental covariates performed best. In the top model, locations that had been previously visited within the same season and locations from previous seasons were more strongly selected relative to environmental covariates, which we interpret as evidence for the strong influence of both short- and long-term memory in driving seasonal range habitat selection. Further, the influence of previous space uses was stronger in the summer relative to winter, which is when deer in this population demonstrated strongest philopatry to their range. Our results suggest that mule deer update their seasonal range cognitive map in real time and retain long-term information about seasonal ranges, which supports the existing theory that memory is a mechanism leading to emergent space-use patterns such as site fidelity. Lastly, these findings provide novel insight into how species store and use information over different time scales.
Introduction
Animal movement is a fundamental process that underpins the relationship between species and their environment (Nathan et al., 2008; Morales et al., 2010). For decades, the study of animal movement has informed our understanding of important ecological processes, including patterns of distribution and abundance (Turchin, 1989, 1991), optimal foraging (Owen-Smith et al., 2010; Middleton et al., 2018), species interactions (Schlaegel et al., 2019), and habitat selection (Byrne et al., 2014; Avgar et al., 2016). By quantifying the factors that drive animal movement, we can better understand these ecological processes and improve predictions of when and where we observe species, which has important applications for species management and conservation (Jeltsch et al., 2013; Berger-Tal and Saltz, 2014; Allen and Singh, 2016; Tucker et al., 2018). Thus, the study of animal movement is central to both basic and applied ecology.
Animal movement is the direct result of the complicated interplay between the physiological state of the animal, the influence of abiotic and biotic environmental factors, and the constraints of the cognitive and physical capacities of the individual (Nathan et al., 2008). Animals move to fulfill basic biological needs that promote fitness (e.g., finding a mate, locating forage, avoiding risk, etc.), and these movements are directly influenced by environmental conditions (e.g., the locations of conspecifics, distribution of resources, predator abundance etc.; Nathan et al., 2008; Avgar et al., 2013b). Although the influence of environmental factors has been a primary focus of movement ecology, in recent years, studies have increasingly recognized the importance of quantifying the influence of cognition on animal movement (Schmidt et al., 2010; Avgar et al., 2013a; Fagan et al., 2013; Bracis et al., 2015; Spiegel and Crofoot, 2016). Cognitive processes, such as learning and memory, are the mechanisms that allow species to store and use information about their environment (Shettleworth, 2001). By tracking experience with their environment, animals can adjust their movements to better exploit local environments to fulfill biological needs (Benhamou, 1994). Thus, accurate explanations and predictions of movement depend on appropriately quantifying the cognitive capabilities of animals and accounting for the influence of cognition on movement decisions.
Due to advancements in field technology and analytical techniques, our ability to infer memory and its effect on movement of free-ranging animals has improved in recent years (Fagan et al., 2013). Research integrating cognitive processes into studies of animal movement has yielded important discoveries about space-use behavior, habitat selection, and foraging theory (Van Moorter et al., 2009; Wolf et al., 2009; Avgar et al., 2015; Bracis et al., 2015). This work has demonstrated that species use memory to follow annual migration routes (Bracis and Mueller, 2017; Merkle et al., 2019), and generate and maintain home range boundaries (Boerger et al., 2008; Van Moorter et al., 2009; Spencer, 2012). Further, memory can reinforce territoriality through routine patrolling of territory boundaries (Schlaegel et al., 2017). Cognition allows species to remember locations of resources (i.e., spatial memory) and their relative quality (i.e., attribute memory; Fagan et al., 2013; Merkle et al., 2014). Species that use memory optimize resource gain by increasing access to high quality forage and reducing the energetic costs associated with movement (Mcnamara and Houston, 1985; Mitchell and Powell, 2012; Merkle et al., 2014; Bracis et al., 2015; Polansky et al., 2015).
Despite the documented benefits of using memory in making movement decisions, theory suggests that memory only provides an adaptive advantage under specific environmental contexts and the use of memory is limited by physiological constraints (Dukas, 1999; Riotte-Lambert and Matthiopoulos, 2020). Species are more likely to use memory to make movement decisions in semi- heterogeneous and semi-predictable environments (Barraquand and Benhamou, 2008; Boyer and Walsh, 2010; Esposito et al., 2010; Bracis et al., 2015). When environments are highly predictable or unpredictable, there is little benefit to memory, and other factors are more likely to guide movement decisions including reliance on ingrained behaviors and the transfer of socially acquired information (Mcnamara and Houston, 1987; Riotte-Lambert and Matthiopoulos, 2020). Furthermore, processing and storing information in memory induces an energetic cost, therefore, memory is limited by storage capacities, and the accuracy and availability of information decays over time (Dukas, 1999; Burns et al., 2011). Species are regularly exposed to an abundance of information; therefore, the information that gets stored in memory must be prioritized by its relevance and how long it is useful to the individual. Memory can be compartmentalized into different memory types: short-term (or working) memory and long-term (or reference) memory (Howery et al., 1999; Cowan, 2008). Information in short-term memory decays quickly, meaning new information can be processed without reaching storage capacity. In contrast, long-term memory has minimal decay, but exacts a greater physiological cost for retaining the accuracy of information (Cowan, 2008). Although uncommon in the animal ecology literature, a few empirical studies have demonstrated that species can rely on different memory types when making movement decisions (Mettke-Hofmann and Gwinner, 2003; Oliveira-Santos et al., 2016; Vergara et al., 2019).
The above suggests that the influence of memory on animal movement will depend on the environmental and physiological constraints on cognition, and therefore it is important that we account for how these constraints might limit or promote how species use memory to make movement decisions. Within and among species, individuals are likely to rely on memory differently depending on life stage, season, life history strategies, internal state (e.g., hunger, reproduction), other animals (e.g., conspecifics, predation risk), and current environmental conditions (Mettke-Hofmann and Gwinner, 2003; Sulikowski and Burke, 2011; Morand-Ferron et al., 2019; Snell-Rood and Steck, 2019). Furthermore, given these conditions, species are likely to use memory differently depending on their physiological capacity, for example relying on different memory types or experiencing accelerated or slowed decay of information (Mettke-Hofmann, 2014). Therefore, by studying the conditions and constraints that influence how and when species use memory when making movement decisions, we can deepen our understanding of how animal cognition has evolved and gain a more mechanistic understanding of how cognition drives habitat selection, optimal foraging theory, and gives rise to emergent space-use patterns.
Our objective was to assess the influence of short- and long-term memory on habitat selection of a migratory mule deer population (Odocoileus hemionus) when occupying seasonal ranges. Memory typically cannot be directly observed from animal movement data, but in several past studies, memory was inferred by measuring the influence of past experience on current movement decisions (e.g., return to previously visited locations; Fagan et al., 2013; Merkle et al., 2014; Oliveira-Santos et al., 2016; Jakopak et al., 2019). To address our objectives, we inferred short- and long-term memory effects on habitat selection from utilization distributions (UDs) measured from recent (e.g., short-term) and past (e.g., long-term) space use of deer. We incorporated the UDs, which represented landscape experience, and current environmental covariates into step-selection functions, which provided inference to habitat selection by comparing locations used by animals to those deemed immediately available to them in a spatially restricted area (SSF; Fortin et al., 2005; Thurfjell et al., 2014). First, we aimed to establish if experience with the landscape, relative to environmental covariates, was an important driver of mule deer seasonal range movement. Then we assessed the degree to which covariates representing recent and past experience (i.e., use) with the landscape influenced mule deer habitat selection within and between seasons. From these results, we can gain insight to the underlying cognitive processes driving seasonal range movement. Mule deer were ideally suited for this analysis because they display strong fidelity across multiple spatial scales (e.g., return to locations, repeat migration routes, and return to seasonal ranges), which supports the likely role of memory in driving seasonal habitat selection (Northrup et al., 2016; Jakopak et al., 2019; Merkle et al., 2019; Sawyer et al., 2019). Furthermore, mule deer in this system migrate annually between summer and winter ranges, which divides the experience an animal has with the landscape and allows for assessment of the degree to which deer rely on recent compared to past experience when selecting habitat.
If memory is an important driver of habitat selection, then covariates representing previous experience should show stronger effects on habitat selection compared to environmental covariates alone in both seasons. Further, we can infer the relative influence of short- and long-term memory on deer habitat selection based on how deer select for recent experience (e.g., short-term memory) and past experience (e.g., long-term memory) with a location. We also expected that deer would demonstrate stronger selection for familiar locations in the summer compared to winter because during the summer deer birth and raise fawns and must maximize forage intake to cope with a comparatively resource-poor environment on winter ranges. Thus, we expected that the value of selecting familiar locations would be greater when there is increased pressure to maximize access to forage and offspring are most vulnerable to predation. Further, we expected that past experience would be more important in the summer based on past work showing higher fidelity on summer ranges (Northrup et al., 2021; see Figure 1).
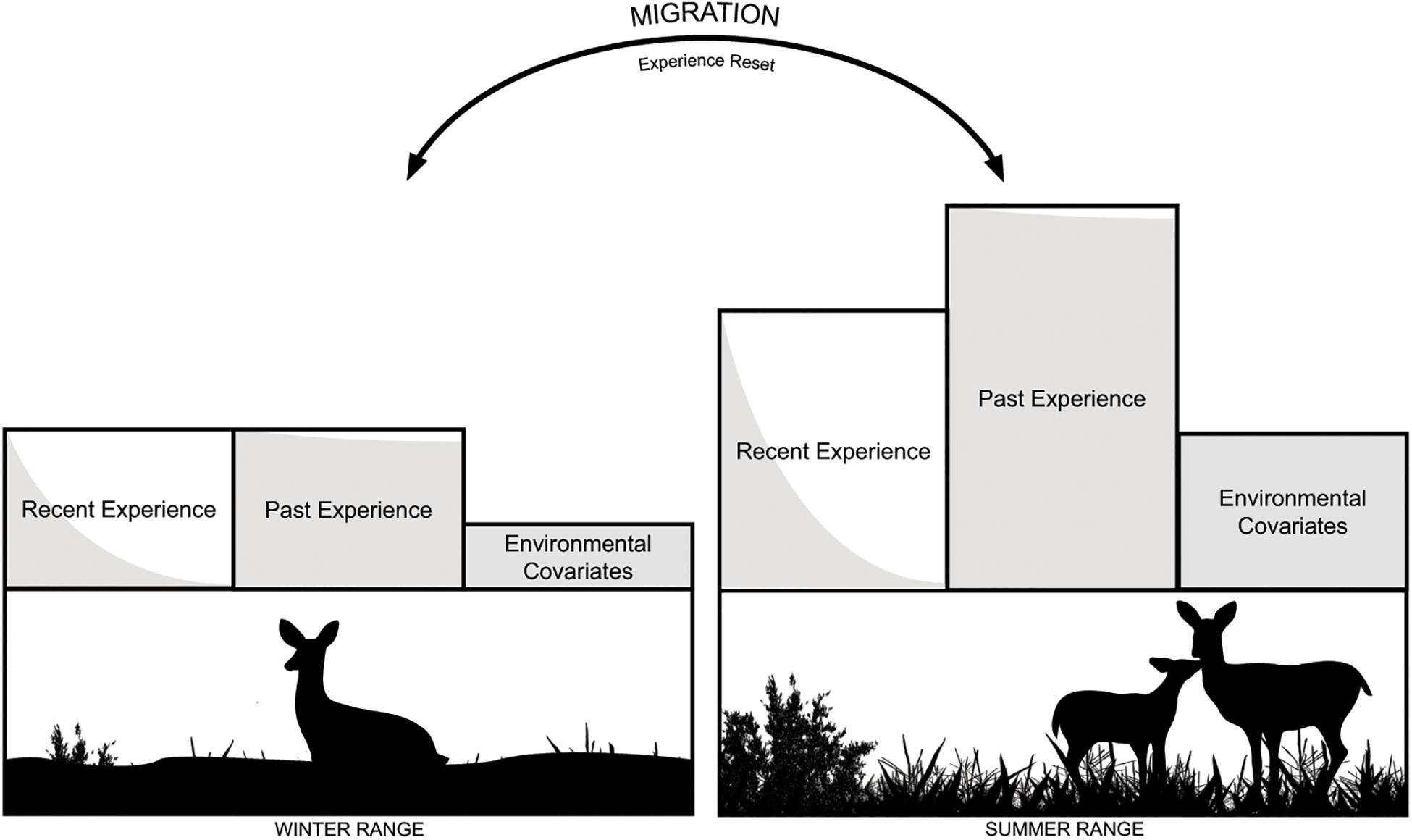
Figure 1. Annual migration between seasonal ranges effectively resets the experience an animal has with the landscape, allowing for an assessment of the relative effects of previous spatial experience across multiple scales (i.e., recent experience, measured from current season space use, and past experience, measured from previous season space use) on current season habitat selection. From the influence of covariates measuring spatial experience and current environmental conditions, we infer how mule deer store and use information in both their short-term (i.e., recent experience) and long-term (i.e., past experience) memory. We expected that if memory is an important driver of habitat selection, covariates measuring both types of experience would show stronger effects on habitat selection than environmental covariates. Summer rangers have greater resource availability than winter ranges, and deer birth and raise fawns during the summer months. Accordingly, we expected that deer would demonstrate stronger selection for covariates measuring experience in the summer compared to winter, and the influence of past experience would be greater in the summer.
Materials and Methods
Study Area
This study took place in the Piceance Basin of northwestern Colorado, United States, near the town of Meeker (Figure 2). This area is topographically diverse, and the dominant vegetation consists of big sagebrush (Artemisia tridentata), and a pinyon pine (Pinus edulis)-Utah juniper (Juniperus osteosperma) shrubland complex at lower elevations and a mix of mountain shrublands, quaking aspen (Populus tremuloides), big sagebrush and a variety of coniferous trees at higher elevations. The region experiences warm, dry summers and cold winters, with most of the moisture falling as snow during the winter. The area is popular for hunting and over the last 15 years has seen extensive exploration for and development of natural gas resources (Northrup et al., 2021). Mule deer in this area are migratory, moving between low elevation winter range and high elevation summer range. Deer typically occupy winter ranges between October and April and occupy summer ranges between May and September, though this time can vary substantially (Lendrum et al., 2014; Northrup et al., 2014b).
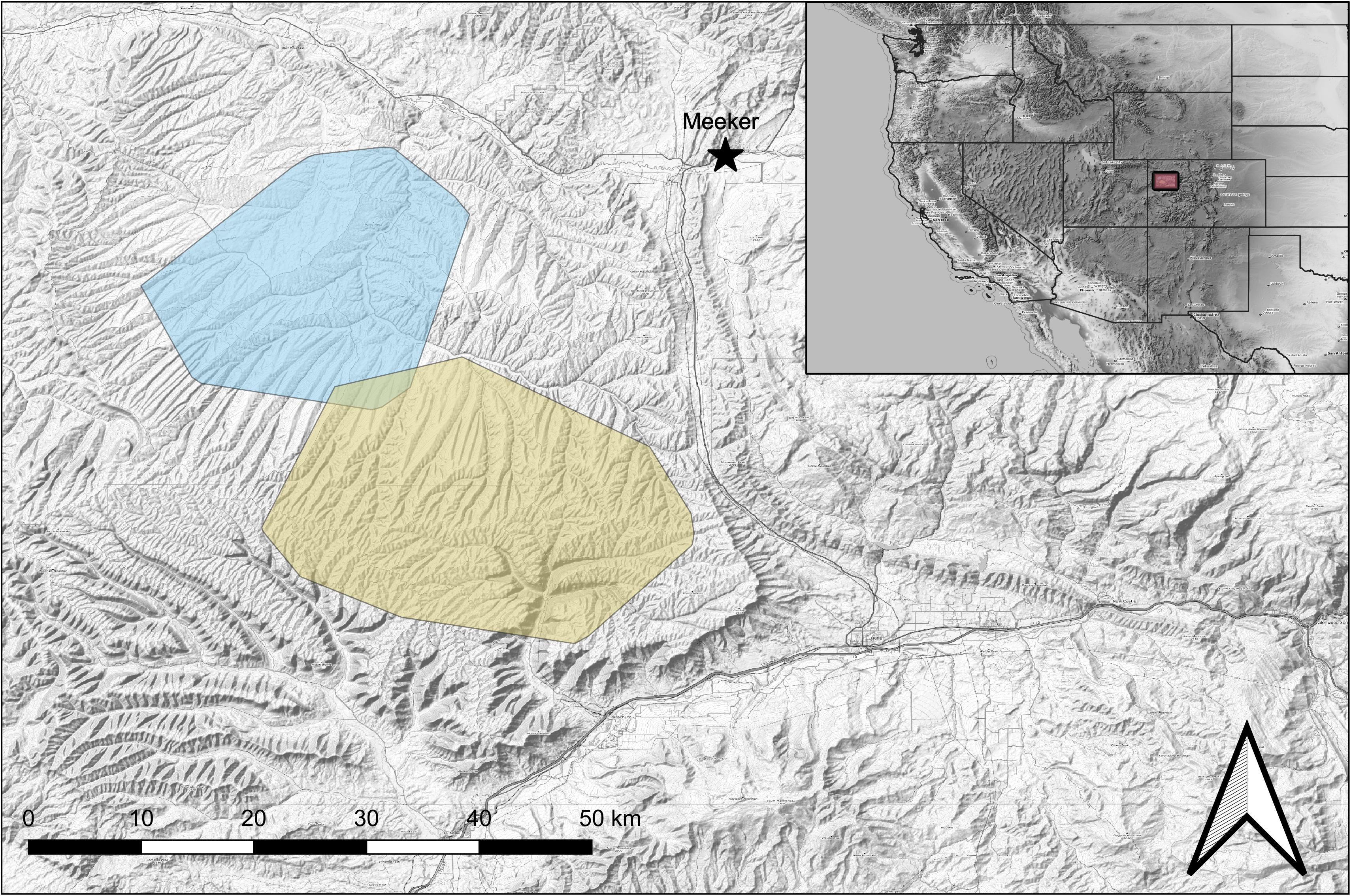
Figure 2. Winter (blue) and summer (yellow) ranges for mule deer in the Piceance Basin, Colorado, United States. Map was produced using QGIS 3.6.3 (QGIS Development Team, 2019. QGIS Geographic Information System. Open Source Geospatial Foundation Project. http://www.qgis.osgeo.org/). Base map by OpenTopoMap (Kartendarstellung: © OpenTopoMap; http://www.opentopomap.org) under creative common license CC BY-SA 3.0 (https://creativecommons.org/licenses/by-sa/3.0/).
Mule Deer Data
Adult female mule deer were captured as a part of a larger research program that took place between January 2008 and April 2018. Throughout this time, deer were captured during December and March for a variety of project objectives. For initial captures, winter range study area boundaries were flown in a helicopter and deer were captured opportunistically using net gunning (Krausman et al., 1985). Upon capture, deer were blindfolded, hobbled, and administered 0.5 mg/kg of midazolam and 0.25 mg/kg of Azaperone. Deer were then transferred to a central processing site, where a suite of standard measures and samples were taken, deer were fit with global positioning system radio collars (G2110D Advanced Telemetry Systems, Isanti, MN, United States) and released on site. Northrup et al. (2014a) provide more in-depth detail on the capture procedure and the suite of measures and samples taken. All procedures were approved by the Colorado Parks and Wildlife Institutional Animal Care and Use Committee (protocol numbers 17-2008 and 01-2012) and followed the guidelines of the American Society of Mammalogists (Sikes, 2016).
Between 2010 and 2013, we aimed to track individual deer over multiple years by recapturing them and replacing collars. In the years following the initial capture, 40 previously captured deer were located using very high frequency (VHF) radio telemetry and captured using net guns. All procedures described above were followed, but collars were replaced each December. For this current analysis, we focused on data collected between October 2011 and September 2013. During this time, collars were set to attempt a relocation every 30 min between October and April and every hour between May and September. We divided data into summer or winter range based on a visual assessment of the migrations of each individual deer because mule deer sometimes demonstrate irregular movements that other standard methods of defining migrations, such as net square displacement, would not accurately capture. We determined that a deer had left their summer or winter range when they made consistent movement away from their established range and did not return until the following season. We similarly determined that a deer had arrived on their winter or summer range when, after migration initiation, they showed localized movements in an area that eventually became part of their range. For the below analyses, we excluded all locations deemed to be during migration.
General Statistical Framework
We examined the habitat selection patterns of mule deer on their winter and summer ranges using step-selection functions. Following Avgar et al. (2016), we generated random movements by drawing step lengths (the distance between relocations) from a gamma distribution with mean and standard deviation for each individual equal to the empirical mean and standard deviation of step lengths. We drew turn angles (i.e., the difference in bearing between the previous and current movement) from a uniform circular distribution. For each used location, we drew 10 available locations and intersected them with the below environmental and memory covariates.
Environmental Covariates
Past research on mule deer in this area has found deer to respond to a number of natural and anthropogenic factors when selecting habitat (Northrup et al., 2015, 2021). Because our intent was to understand how previous experience, quantified through previous space use, influenced deer habitat selection while accounting for other environmental factors, we chose covariates based on these analyses. Specifically, for winter models, we assessed habitat selection relative to a terrain ruggedness index (TRI), elevation (elev), distance to treed edges (edge), snow depth (snow), and the land cover categories representing barren land (barren), shrublands (shrub), and grasslands (grass) with treed land cover as the reference category. For summer models, we examined habitat selection relative to TRI, elev, and edge as in the winter models, but also examined the normalized difference vegetation index (NDVI). Further, because there was little barren land cover, we combined barren and grass into a single category (open). In addition, because this area had ongoing active natural gas exploration and development, we also assessed the response of deer to the distance to well pads (dpads) and the distance to roads (drds) for models fit to both seasons.
Covariates Representing Recent and Past Spatial Experience
To meet our objectives, we aimed to quantify covariates representing recent and past experience with a location. Previous analysis of the deer movement data (Northrup et al., 2016) derived a UD for each individual in our sample by fitting continuous time correlated random walk models using the “crawl” package (Johnson et al., 2008; Johnson and London, 2018) in the R statistical software (R Core Team, 2020). These UDs were calculated at a 5 m × 5 m resolution and provide an estimate of the probability that an animal had been within a given 5 m × 5 m cell during the previous year, which we assumed equated to the relative amount of experience each deer had with that cell. Because deer migrate each year, the previous year’s UD should capture their past experience with each seasonal range. We used UDs fit to winter range data for winter 2011/2012 as a representation of past experience for habitat selection models (see below) fit to data from winter 2012/2013 and UDs fit to summer range data for summer 2012 for habitat selection models fit to data for summer 2013. We extracted these UD values for every used and available location and termed this covariate prev_ud.
To quantify recent experience, we undertook a procedure that calculates metrics equivalent to a daily UD. To do this, we estimated the probability that a deer had been at a given used or available location for every day during the current season prior to the day the focal fix was taken on. To do this, we followed the same general approach as Northrup et al. (2016). Using the “crawl” package (Johnson et al., 2008; Johnson and London, 2018), we fit continuous time correlated random walk models to data from each individual and season. Next, we used these models to predict the location of each animal for every minute they were on their seasonal range. Under this approach, for each minute, the model produces a bivariate normal distribution, which can be used to calculate the probability that the animal was at any location in the study area at that minute. By summing over every distribution for each minute prior to the focal location, we can obtain the probability that the animal had been at that location previously. We thus calculated this probability for each used and available location. To avoid overly weighting very recent experiences, we excluded distributions from the 24 h prior to the focal location from these calculations. Further, to ensure that our results were robust to our choice of the amount of time to exclude, we conducted a sensitivity analysis by also fitting our models excluding locations from the previous 7 days. We termed the covariate representing recent experience curr_ud to indicate that it represents the current year’s UD to that point in time.
Model Fitting
Previous research in this area has shown mule deer to have strong differences in habitat selection patterns by time of day (Northrup et al., 2015). Thus, we split data by summer and winter range and time of day, with nighttime determined to be the time between sunset and sunrise. Sunrise and sunset were calculated using geographical position and time of day. We then took a tiered approach to model fitting. We expected recent experience should decay over time because, presumably, recent experience should be stored in short-term memory, which has a limited capacity and the availability and accuracy of information decays quickly (Cowan, 2008; Merkle et al., 2014). To estimate decay of recent experience, we followed the general approach of Merkle et al. (2014), who used the following decay function for memory: where k is the decay coefficient, with larger values equating to faster decay of memory, and t representing the time since the animal was at a location. For each used and available location, we applied the decay function to discount the probability that the animal had been at a given location for each minute previous to that location. These probabilities were calculated as described above and after applying the decay function, we aggregated these discounted probabilities. For a given used or available location, this approach provides the probability that the animal had been there previously during that season, discounted according to the decay function such that more recent experience could be weighted more. To find the optimal k value for each individual, we fit individual models including all of the environmental and experience-based covariates outlined above, iterating through all possible values of k between 0 and 1 following the general approach of Merkle et al. (2014). We estimated k using maximum likelihood in a two-stage process with the Broyden-Fletcher-Goldfarb-Shanno (BFGS) optimizer in R. First, a k value was chosen, the probability values were discounted using the decay function above, and models were fit using maximum likelihood for the current value of k (see below for model specification). Then, “optim” was used to iterate through this process to estimate the value of k corresponding to the maximum likelihood. In this approach, k and the selection coefficients are estimated separately. We attempted to estimate these parameters simultaneously but were unable to achieve convergence. k parameters were estimated using this approach separately for each individual animal, with step-selection functions fit using conditional logistic regression in the “survival” package in R (Therneau and Grambsch, 2000; Therneau, 2015). In addition, we were interested in understanding how the importance of both recent and past experience changed throughout the season. Thus, we fit the models with an interaction between the landscape experience covariates and the time since the animal arrived on their summer or winter range, which provides inference to whether and how their selection for areas they were familiar with changed over the season.
After determining the optimal k value for each individual, we recalculated the recent experience covariate using this value, which should more appropriately represent a deer’s ability to remember recently visited locations, and we fit a hierarchical SSF where all coefficients (i.e., slopes) were allowed to vary by individual. Prior to model fitting, we calculated pairwise correlations among all covariates to ensure they were below 0.7 (see Supplementary Information). We fit models using integrated nested Laplace approximation (INLA) in R (Lindgren and Rue, 2015) with the addition of the PARDISO solver (Bollhöfer et al., 2019, 2020; Alappat et al., 2020) to reduce computation time and followed the guidance and coded examples in Muff et al. (2020). For comparison, we fit additional models: one excluding both recent and past experience, one excluding only recent experience, one excluding only past experience, and one excluding the environmental covariates. We compared models using Bayes Factor (Gelman et al., 2013), which we derived from the marginal likelihood by taking the difference between complex models and a reference model where no covariates were included (Gomez-Rubio, 2020). Bayes Factor represents the strength of evidence provided by the data in favor of one theory among two competing theories (Kass and Raftery, 1995). All continuous covariates except curr_ud were centered and scaled by subtracting the mean and dividing by the standard deviation. Covariates were scaled using means and standard deviations calculated over all used and available locations for all individuals. We refit the model with both experience types and all environmental covariates, but using the curr_ud covariate calculated excluding the most recent 7 days of experience to assess the sensitivity of our results to the most recently experienced locations (note that the entire model fitting process including the estimation of the decay parameters k was repeated for these models excluding the most recent 7 days). Due to the computational requirements, we fit all models using the Cedar cluster (computecanada.ca, RRG: hyf-453-ab).
Results
We fit models to 29 individual deer in summer and 31 individual deer in winter that had two complete winters and summers of data to fit our objectives. No pairwise correlations between covariates were >0.5 (see Supplementary Information). The results of our sensitivity analysis were nearly identical to when we dropped the most recent 24 h for three out of four models and fourth model didn’t converge. The coefficients all were in the same direction, with only minor changes to the magnitude, except in summer the magnitude of decay.currud increased considerably, and there were no changes to whether 95% credible intervals overlapped 0. For these reasons, we report our results from models excluding the previous 24 h from the covariate representing recent experience.
The influence on habitat selection from recent experience decayed for most individuals, with decay being substantially faster during the summer (Figure 3). For all combinations of season and time of day, models with both recent and past experience outperformed models with one type of experience or no experience at all (Table 1). Across both seasons and time periods, deer displayed strong selection for areas with which they had recent experience (locations visited within the same season) and past experience (locations visited last year), though their seasonal dynamics varied between winter and summer (Table 2). During the winter, selection for areas with which deer were familiar over the short- and long-term was generally constant across the season (Figure 4). However, during the summer, selection declined throughout the season (Figure 4). Both recent and past experience was substantially stronger drivers of selection during the summer, with effects nearly three times those of winter (Figure 4).
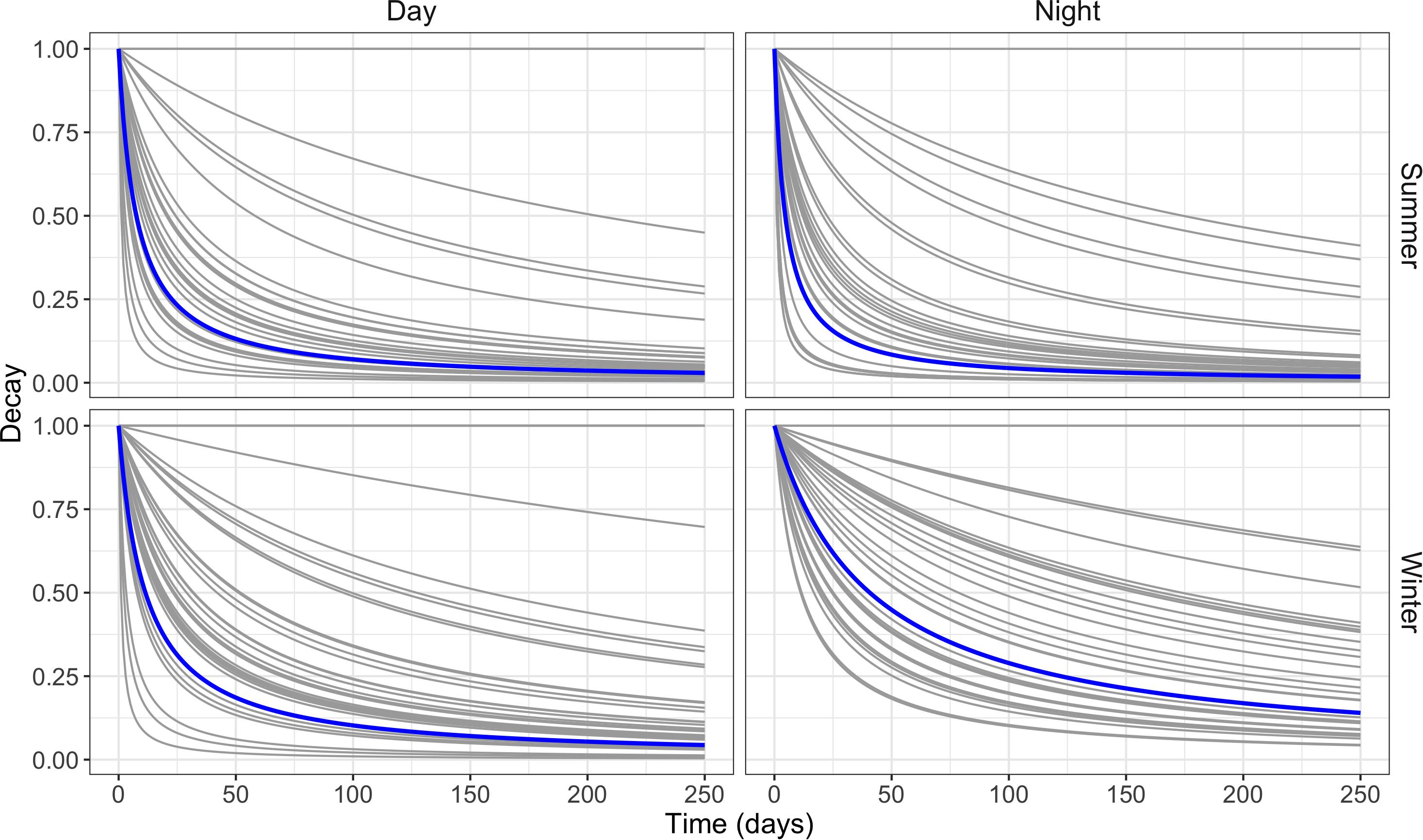
Figure 3. Estimates of short-term memory decay of recently visited locations (i.e., recent experience) for night and day models comparing summer and winter for individual mule deer in the Piceance Basin, Colorado, United States. The blue line indicates the population level mean.
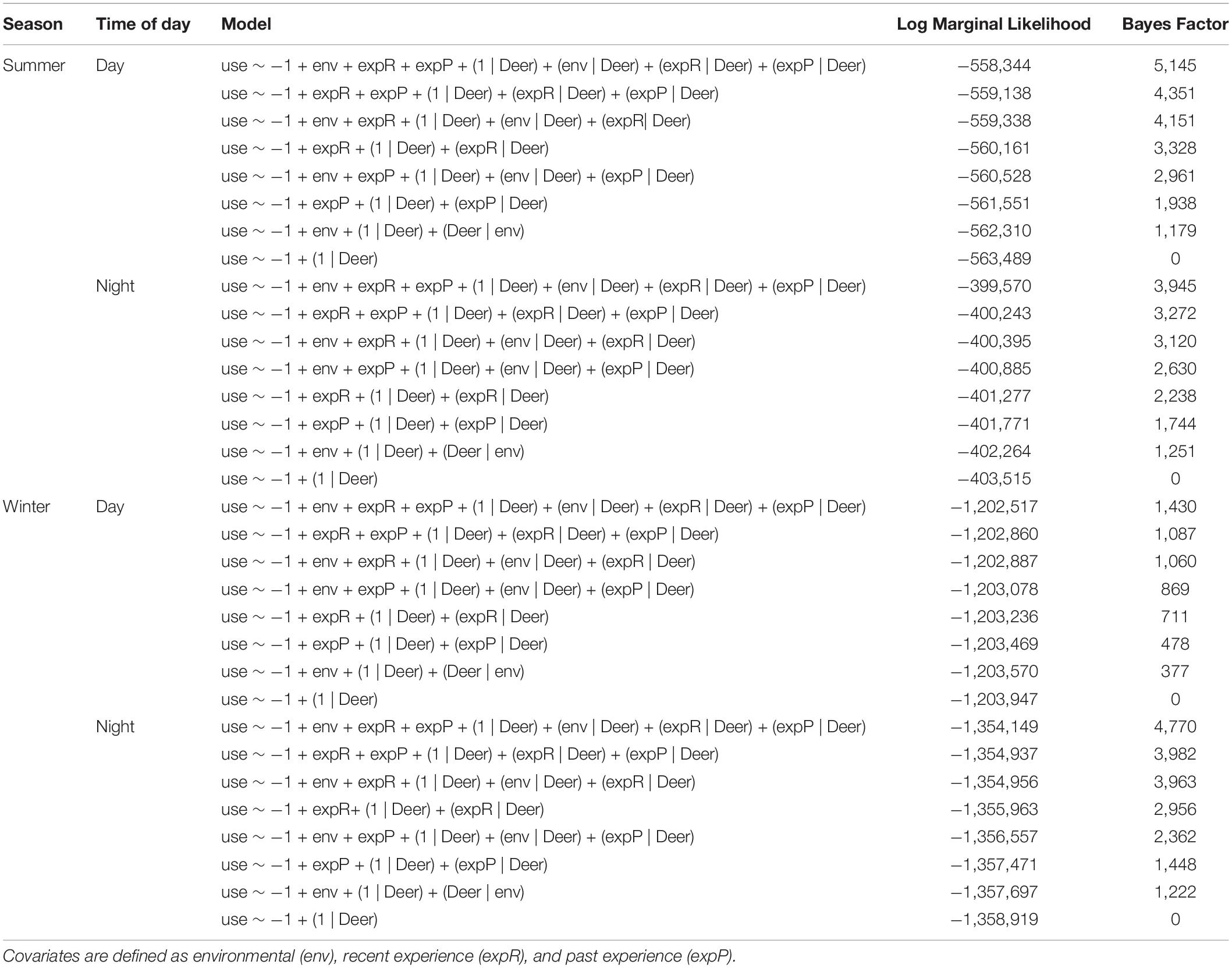
Table 1. Model comparison using Bayes Factors across season and time of day for hierarchical Step Selection Function models with coefficient estimates (i.e., slopes) varying by individual, fit to adult female mule deer GPS radio collar data from animals in the Piceance Basin of Northwestern Colorado, United States.
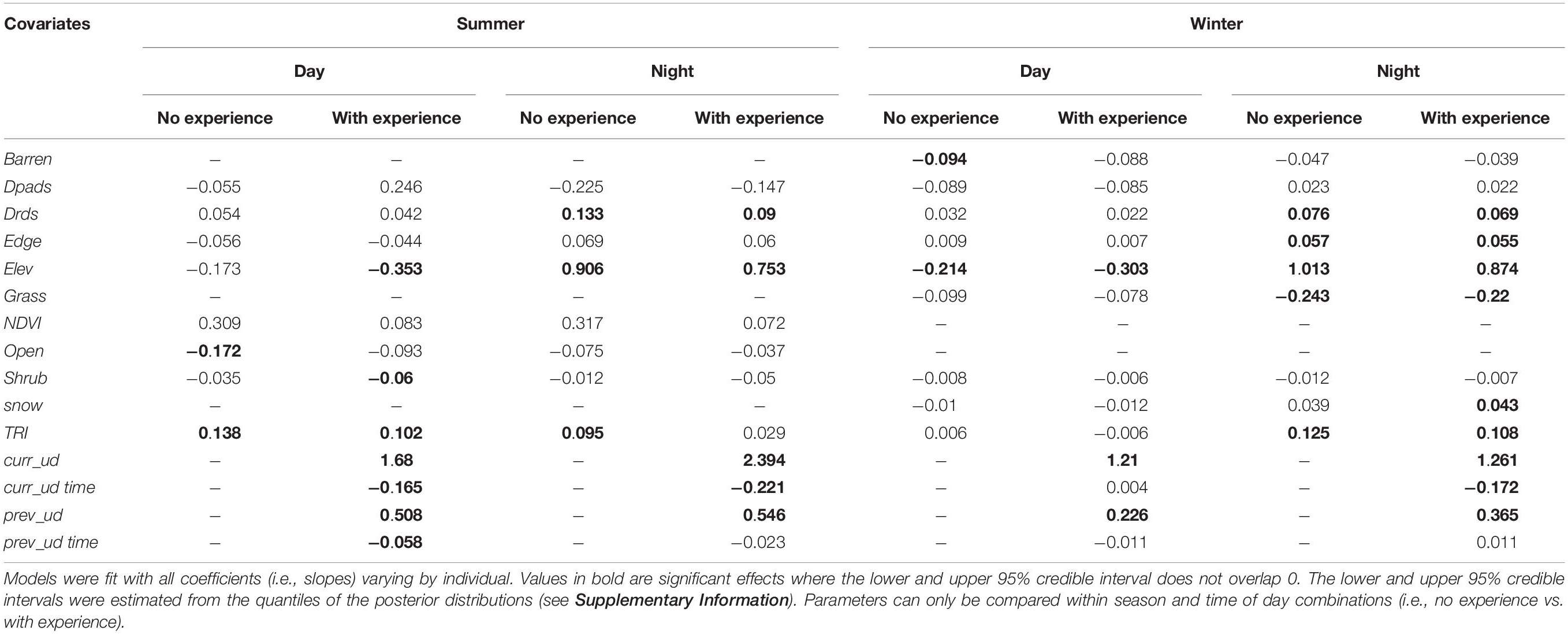
Table 2. Posterior means of population-level parameters for summer and winter night/day Step Selection Functions when experience coefficients are included or excluded for adult female mule deer in the Piceance Basin of Northwestern Colorado, United States.
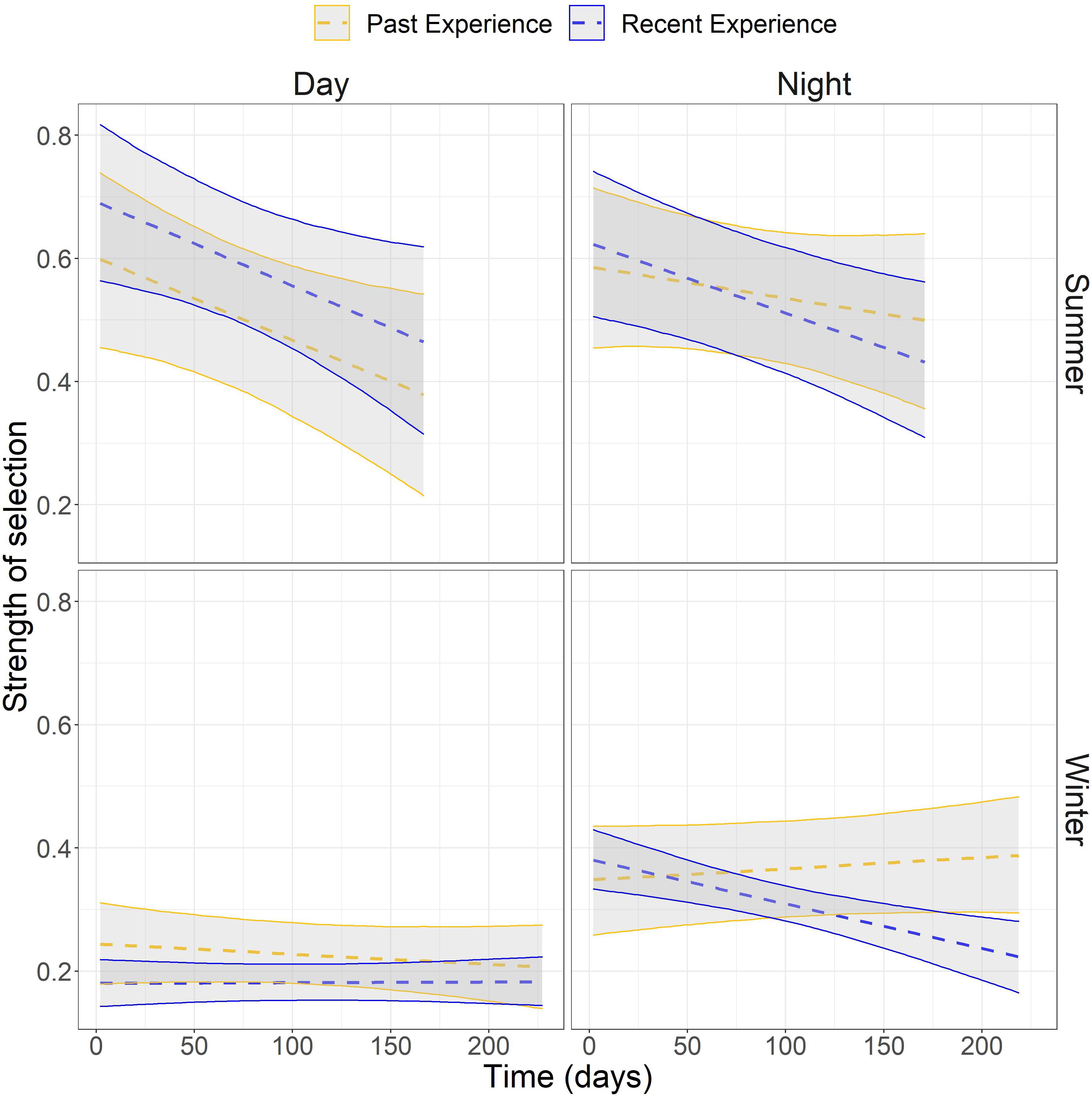
Figure 4. The strength of selection for covariates representing recent- and past experience estimated from population-level parameters from step-selection functions for mule deer within their winter and summer range across time of day in the Piceance Basin, Colorado, United States.
In both seasons, experience with the landscape appeared to be stronger drivers of selection behavior than most of the environmental covariates, having larger magnitude and less uncertainty than other covariates (Table 2). Deer also showed strong selection relative to elevation, but the direction of this effect varied by time of day, with deer selecting for lower elevations during the day and higher elevations at night in both seasons. During the summer, few other covariates had strong influence on selection, but during the winter, at nighttime, deer showed strong selection for numerous anthropogenic and landscape features (Table 2). In addition to these selection patterns, there were some major shifts in coefficient magnitudes between models fit with and without experience covariates to the same data. Specifically, in the summer, models with no experience had coefficient estimates for NDVI that were more than 3x larger than models with experience, though 95% credible intervals overlapped in all cases (Table 2; see Supplementary Information for a table version with credible intervals). There were similar, though weaker, shifts in land cover covariates for summer models, but less evidence of such shifts in winter models.
Discussion
Memory is a fundamental component of animal movement decision-making, and our findings build on the cognitive movement ecology literature by providing evidence that memory is an important cognitive process driving mule deer habitat selection (Merkle et al., 2014; Avgar et al., 2015; Oliveira-Santos et al., 2016; Bracis and Mueller, 2017; Marchand et al., 2017). Our results demonstrate that mule deer select for familiar locations, both from recent and past experience, to a greater extent than most measured environmental factors. Although measuring memory directly in observational studies is difficult, we suggest that these covariates are at least partially representative of memory. Thus, these findings indicate that mule deer store information using short- (defined here as information accrued during the season of analysis) and long-term (defined as information accrued the previous year) memory. If deer had no capacity for memory, we would expect that past experience would only influence habitat selection to the degree to which it was correlated with current environmental conditions. While it is possible that experience could represent temporally static environmental factors not captured in our array of landscape covariates, past research on this species has documented the likely use of memory (Jakopak et al., 2019; Merkle et al., 2019), and thus the re-selection of areas that were previously used is more simply and logically explained by memory than by animals repeatedly randomly encountering the same locations. Further, if deer had a more limited capacity for memory, we expect they would have selected primarily for recently visited locations, which would indicate memory is reset when deer leave seasonal ranges. However, the strong influence of past experience (e.g., last season’s UD) and the consistent finding of the importance of memory when excluding the previous week of data suggests that mule deer have a strong capacity for long- and short-term memory.
The strong influence of long-term experience has important implications for our understanding of the evolution of long-term memory and migratory behavior (Mettke-Hofmann, 2014). Species migrate to access seasonally variable resources and being able to remember information about the location of resources on seasonal ranges, despite periodic absence, would be critical for exploiting them efficiently (Aikens et al., 2020). Migratory species have been shown to have greater capacity to store and utilize long-term memory, underscoring its importance in informing long distance movements (Mettke-Hofmann and Gwinner, 2003; Pravosudov et al., 2006; Mettke-Hofmann, 2014). Conversely, other research comparing short- and long-term memory found evidence that resident species (e.g., non-migratory) rely more on short-term memory (Oliveira-Santos et al., 2016; Vergara et al., 2019). Our results support these past findings and suggest that both short and long term memory are critical for allowing migratory species to exploit local resources. Further assessments of the relationship between memory types and movement strategies, such as long-term memory and migration, will provide insight to how cognition and movement phenomena have evolved.
The roughly equal influence of both recent and past experience indicates that when mule deer leave seasonal ranges, they retain a certain level of familiarity that influences their detailed movement decisions and thus patterns of space use in the following year. Our findings support several recent papers, which suggest that memory is an important mechanism generating emergent space-use patterns of animals (Van Moorter et al., 2009; Piper, 2011; Spencer, 2012; Avgar et al., 2015). Animals constrain their space use, which results in home range behavior, site fidelity, and recursion (Brown and Orians, 1970; Boerger et al., 2008). Environmental conditions partially explain why these patterns exist, because species are likely to constrain their space use depending on the availability and quality of habitat and forage (Mitchell and Powell, 2012), population density (Trewhella et al., 1988), breeding status (Gaulin and FitzGerald, 1988), and anthropogenic influence (Martin et al., 2010). However, tracking experience with a landscape allows species to better exploit environmental factors (Wolf et al., 2009; Schmidt et al., 2010; Merkle et al., 2014; Bracis et al., 2015; Forrester et al., 2015). Mule deer in this system exhibit strong fine-scale fidelity to seasonal ranges between successive years, especially in the summer (Northrup et al., 2021). Our results suggest that memory is the mechanistic driver of philopatric behavior in this population. These findings further suggest that general movement paths have been reinforced over successive years and are likely consolidated into long-term memory, which could explain how philopatric patterns emerge at broader scales (Owen-Smith et al., 2010; Merkle et al., 2019). Thus, we propose that long-term memory is likely the mechanism promoting return to seasonal ranges and short-term memory allows for deer to alter their habitat selection based on updated information about dynamic variables in the landscape (Spencer, 2012).
Theory suggests that memory only provides an adaptive advantage when the benefit of reusing information outweighs the cost of retaining it, which occurs most often in environments that have medium levels of heterogeneity and predictability (Mcnamara and Houston, 1987; Barraquand and Benhamou, 2008; Fagan et al., 2013; Bracis et al., 2015; Riotte-Lambert and Matthiopoulos, 2020). As such, we speculate that the stronger influence of previous experience in the summer, both recent and in the past, suggests that memory is more valuable when navigating the summer landscape. This could be a result of a highly predictable landscape related to resource quality and predation risk, in combination with increased pressure to maximize energy stores in the summer. Mule deer birth and raise fawns at this time, and summer ranges have ample forage available (Péron et al., 2018). Contrastingly, deer lose substantial energy stores during the winter due to poor resource availability (Northrup et al., 2021) and likely prioritize energy conservation. Thus, we suspect that good habitat during parturition and areas of high-quality forage are more valuable to remember from year to year on summer ranges, which would support a strong reliance on memory as a strategy for selecting habitat (Péron et al., 2018; Cameron et al., 2020). The lesser influence of experience in the winter suggests it is physiologically more efficient for deer to rely on other informational sources, such as conspecifics or engrained behavior, when moving through a more resource-poor landscape (Riotte-Lambert and Matthiopoulos, 2020). The strong influence of experience in the summer can explain previous observations that deer in this system maintain smaller range sizes in the summer (Northrup et al., 2016), which further supports our interpretation of the strong influence of memory in generating space use patterns. Furthermore, we estimated individuals experience a faster rate of decay of their short-term memory of recently visited locations in the summer compared to winter, which we speculate further supports the stronger reliance on memory in the summer, because when information is valuable, capacity limits of short-term memory may be reached at a faster rate (Spencer, 1992, 2012).
In addition to the above-noted differences between summer and winter, deer selected less often for recently visited locations as the summer season progressed but showed relatively consistent selection for these locations during winter. We believe this pattern could best be explained by within season variation in predation risk (Bracis et al., 2018). We believe at the beginning of the summer, when fawns are most vulnerable to predation, deer frequently revisit locations with ample cover to hide their fawns, which likely stays consistent from year to year. As fawns become more mobile as the summer progresses, by rule, deer return to the same location less often (Monteith et al., 2014; Cameron et al., 2020). Additionally, the value of forage declines as the summer progresses, potentially causing the selection of previously visited locations to become less favorable. We did not have sufficient data to test the relationship between environmental predictability, resource depletion and predation risk on selection of familiar locations within and between seasons (Lendrum et al., 2018), but we suspect these are the variables driving observed patterns in memory effects. Furthermore, we acknowledge that because we were unable to incorporate these variables, selection for habitat related to environmental predictability, resource quality, and predation risk could potentially occur absent memory effects. Thus, we encourage future assessments evaluating the role of environmental predictability and understanding how changes in environmental conditions influence when and how species use memory when selecting for habitat.
The strong influence of landscape experience could have negative implications for the ability of deer to respond to landscape change. Previous evaluations of memory suggest that a strong reliance on this process promotes rigid movement behavior that doesn’t allow species to respond to change (Merkle et al., 2015; Sawyer et al., 2019). These findings have raised concerns that memory could have maladaptive effects for populations experiencing environmental change related to anthropogenic disturbance (Andersen, 1991; Morrison et al., 2021). Thus, the strong reliance of mule deer on familiarity when selecting habitat suggests that they may not be able to cope well with the accelerated rates of change being documented in natural systems (Sih et al., 2011; Beever et al., 2017; Wyckoff et al., 2018). Alternatively, past work in this system by Northrup et al. (2021) suggests deer potentially can adapt behaviorally to some forms of landscape change with minimal demographic effect, but clearly more research is needed to understand the role of memory in promoting or restricting adaptability to landscape change.
Memory is complex, and thus our methods have limitations in providing inference to this process. First, memory is fundamentally a latent characteristic, and the degree to which it influences animal behavior can only be inferred (Fagan et al., 2013). We inferred memory from selection of areas that had been used in the past, but there are other possible interpretations. As discussed above, the role of memory in informing movement decisions may be confounded by selection of resources absent any significant influence of memory (Oliveira-Santos et al., 2016). If mule deer revisited specific locations based on their moment-to-moment perception of resource quality, we might find similar results, particularly if we were unable to quantify an important environmental resource in our models, such as predation risk. Further, mule deer in our system may also draw on conspecifics (Codling et al., 2007; Jesmer et al., 2018) and ingrained behavior (Riotte-Lambert and Matthiopoulos, 2020) to guide movement decisions. However, we did not sample a sufficiently large proportion of the population to adequately include variables representing conspecific influence, as well as the generally low sample size could have influenced our results. Further, inclusion of experience tended to reduce the magnitude of environmental covariate coefficients, which could impact interpretation of environmental drivers of space use.
However, we believe our results provide reasonably strong evidence that memory is an important driver of mule deer habitat selection, and mule deer store and use information about their environment in short- and long-term memory. There was little correlation between environmental and landscape experience covariates (see Supplementary Information) and models incorporating experience outperformed models with environmental covariates alone, lending additional support to previous research, which showed the inclusion of memory in simulation models was integral for reproducing empirical movement paths (Bracis et al., 2015; Merkle et al., 2019). Further, the annual absence of mule deer from seasonal ranges allowed for more robust inference to the influence of memory type effects based on how deer select for locations experienced recently (e.g., within the same season with decay) or in the past (e.g., the previous season). Lastly, our estimates of the effects of memory should be realistic because they are consistent with previous findings reported for mule deer and other ungulate species, including the capability for long term retention of information (Avgar et al., 2015; Jakopak et al., 2019; Merkle et al., 2019; Cameron et al., 2020) and similar decay rates for short-term memory (Bailey et al., 1989; Laca, 1998).
Our results further demonstrate the utility of including experience in the formulation of step selection functions to infer cognitive drivers of movement (Merkle et al., 2014; Oliveira-Santos et al., 2016). Our findings demonstrate mule deer reliance on recent and past experience varied in accordance with both regional (summer vs winter range) and local (within-range) conditions, lending insight to when memory is advantageous and how the influence of memory can lead to the emergence of space use patterns such as site fidelity. The specific processes driving this temporal and spatial variation in the use of memory, however, warrant further investigation. Therefore, where possible, we encourage future studies to include internal state variables (e.g., reproductive status) and additional external factors, such as resource predictability and predation risk in model formulations to further elucidate processes influencing when and how species use memory to inform movement decisions. Examining how and when species use different types of memory can provide insights to the adaptive advantages of memory-driven movement and the development of emergent space use patterns.
Data Availability Statement
The raw data supporting the conclusions of this article will be made available by the authors, without undue reservation.
Ethics Statement
The animal study was reviewed and approved by Colorado Parks and Wildlife Institutional Animal Care and Use Committee.
Author Contributions
CA coordinated data collection. GW and CA contributed funding for data collection. JN and RM performed data analyses. RM, TR, and MB developed tables and figures. HR led the drafting of the manuscript with contributions from JN and TR. All authors contributed to the conception and design of the study, manuscript revision, and have read and approved the submitted version.
Funding
Mule deer capture and monitoring was funded and/or supported by Colorado Parks and Wildlife (CPW), White River Field Office of Bureau of Land Management, ExxonMobil Production/XTO Energy, Federal Aid in Wildlife Restoration (W-185-R), Safari Club International, the Colorado State Severance Tax, EnCana Corp., Williams/WPX Energy, Shell Exploration and Production, Marathon Oil Corp., The Mule Deer Foundation and Colorado Mule Deer Assn. This work was supported by the Natural Sciences and Engineering Research Council of Canada Discovery Grant to JN. The funders were not involved in the study design, collection, analysis, interpretation of data, the writing of this article, or the decision to submit it for publication.
Conflict of Interest
The authors declare that the research was conducted in the absence of any commercial or financial relationships that could be construed as a potential conflict of interest.
Publisher’s Note
All claims expressed in this article are solely those of the authors and do not necessarily represent those of their affiliated organizations, or those of the publisher, the editors and the reviewers. Any product that may be evaluated in this article, or claim that may be made by its manufacturer, is not guaranteed or endorsed by the publisher.
Acknowledgments
We would like to thank L. Wolfe, M. Fisher, C. Bishop, D. Finley, and D. Freddy (CPW) and numerous field technicians for project assistance, L. Gepfert (CPW) and Coulter Aviation, Inc., for aircraft support, and Quicksilver Air, Inc. for helicopter support for mule deer captures. We would also like to thank D. B. Johnston and B. Walker for valuable feedback. We thank the reviewers, J. Becker and K. L. Monteith, and the handling editor, T. Avgar, for reviewing and providing feedback that improved the quality of this manuscript. This research was enabled in part by support provided by Compute Canada (www.computecanada.ca).
Supplementary Material
The Supplementary Material for this article can be found online at: https://www.frontiersin.org/articles/10.3389/fevo.2021.702818/full#supplementary-material
References
Aikens, E. O., Mysterud, A., Merkle, J. A., Cagnacci, F., Rivrud, I. M., Hebblewhite, M., et al. (2020). Wave-like patterns of plant phenology determine ungulate movement tactics. Curr. Biol. 30, 3444–3449. doi: 10.1016/j.cub.2020.06.032
Alappat, C., Basermann, A., Bishop, A. R., Fehske, H., Hager, G., Schenk, O., et al. (2020). A recursive algebraic coloring technique for hardware-efficient symmetric sparse matrix-vector multiplication. ACM Trans. Parallel Comput. 7:37. doi: 10.1145/3399732
Allen, A. M., and Singh, N. J. (2016). Linking movement ecology with wildlife management and conservation. Front. Ecol. Evol. 3:155. doi: 10.3389/fevo.2015.00155
Andersen, R. (1991). Habitat deterioration and the migratory behaviour of moose (Alces alces L.) in Norway. J. Appl. Ecol. 28, 102–108.
Avgar, T., Baker, J. A., Brown, G. S., Hagens, J. S., Kittle, A. M., Mallon, E. E., et al. (2015). Space-use behaviour of woodland caribou based on a cognitive movement model. J. Anim. Ecol. 84, 1059–1070. doi: 10.1111/1365-2656.12357
Avgar, T., Deardon, R., and Fryxell, J. M. (2013a). An empirically parameterized individual based model of animal movement, perception, and memory. Ecol. Modell. 251, 158–172. doi: 10.1016/j.ecolmodel.2012.12.002
Avgar, T., Mosser, A., Brown, G. S., and Fryxell, J. M. (2013b). Environmental and individual drivers of animal movement patterns across a wide geographical gradient. J. Anim. Ecol. 82, 96–106. doi: 10.1111/j.1365-2656.2012.02035.x
Avgar, T., Potts, J. R., Lewis, M. A., and Boyce, M. S. (2016). Integrated step selection analysis: bridging the gap between resource selection and animal movement. Methods Ecol. Evol. 7, 619–630. doi: 10.1111/2041-210X.12528
Bailey, D. W., Rittenhouse, L. R., Hart, R. H., and Richards, R. W. (1989). Characteristics of spatial memory in cattle. Appl. Anim. Behav. Sci. 23, 331–340.
Barraquand, F., and Benhamou, S. (2008). Animal movements in heterogeneous landscapes: identifying profitable places and homogeneous movement bouts. Ecology 89, 3336–3348.
Beever, E. A., Hall, L. E., Varner, J., Loosen, A. E., Dunham, J. B., Gahl, M. K., et al. (2017). Behavioral flexibility as a mechanism for coping with climate change. Front. Ecol. Environ. 15:299–308. doi: 10.1002/fee.1502
Berger-Tal, O., and Saltz, D. (2014). Using the movement patterns of reintroduced animals to improve reintroduction success. Curr. Zool. 60, 515–526. doi: 10.1093/czoolo/60.4.515
Boerger, L., Dalziel, B. D., and Fryxell, J. M. (2008). Are there general mechanisms of animal home range behaviour? A review and prospects for future research. Ecol. Lett. 11, 637–650.
Bollhöfer, M., Eftekhari, A., Scheidegger, S., and Schenk, O. (2019). Large-scale sparse inverse covariance matrix estimation. SIAM J. Sci. Comput. 41, A380–A401. doi: 10.1137/17M1147615
Bollhöfer, M., Schenk, O., Janalik, R., Hamm, S., and Gullapalli, K. (2020). “State-of-the-art sparse direct solvers,” in Parallel Algorithms in Computational Science and Engineering, eds A. Grama and A. H. Sameh (Cham: Birkhäuser Springer International Publishing), 3–33.
Boyer, D., and Walsh, P. D. (2010). Modelling the mobility of living organisms in heterogeneous landscapes: does memory improve foraging success? Philos. Trans. R. Soc. A Math. Phys. Eng. Sci. 368, 5645–5659.
Bracis, C., Gurarie, E., Rutter, J. D., and Goodwin, R. A. (2018). Remembering the good and the bad: memory-based mediation of the food-safety trade-off in dynamic landscapes. Theor. Ecol. 11, 305–319. doi: 10.1007/s12080-018-0367-2
Bracis, C., Gurarie, E., Van Moorter, B., and Goodwin, R. A. (2015). Memory effects on movement behavior in animal foraging. PLoS One 10:e0136057. doi: 10.1371/journal.pone.0136057
Bracis, C., and Mueller, T. (2017). Memory, not just perception, plays an important role in terrestrial mammalian migration. Proc. R. Soc. B Biol. Sci. 284:20170449. doi: 10.1098/rspb.2017.0449
Brown, J. L., and Orians, G. H. (1970). Spacing patterns in mobile animals. Annu. Rev. Ecol. Syst. 1, 239–262.
Burns, J. G., Foucaud, J., and Mery, F. (2011). Costs of memory: lessons from ‘mini’ brains. Proc. R. Soc. B Biol. Sci. 278, 923–929.
Byrne, M. E., McCoy, J. C., Hinton, J. W., Chamberlain, M. J., and Collier, B. A. (2014). Using dynamic Brownian bridge movement modelling to measure temporal patterns of habitat selection. J. Anim. Ecol. 83, 1234–1243. doi: 10.1111/1365-2656.12205
Cameron, M. D., Joly, K., Breed, G. A., Mulder, C. P. H., and Kielland, K. (2020). Pronounced fidelity and selection for average conditions of calving area suggestive of spatial memory in a highly migratory ungulate. Front. Ecol. Evol. 8:564567. doi: 10.3389/fevo.2020.564567
Codling, E. A., Pitchford, J. W., and Simpson, S. D. (2007). Group navigation and the “many-wrongs principle” in models of animal movement. Ecology 88, 1864–1870.
Cowan, N. (2008). “Chapter 20 what are the differences between long-term, short-term, and working memory?,” in Essence of Memory, eds W. S. Sossin, J. C. Lacaille, V. F. Castellucci, and S. Belleville (Jordan Hill: Elsevier), 323–338.
Esposito, S., Incerti, G., Giannino, F., Russo, D., and Mazzoleni, S. (2010). Integrated modelling of foraging behaviour, energy budget and memory properties. Ecol. Modell. 221, 1283–1291.
Fagan, W. F., Lewis, M. A., Auger-Methe, M., Avgar, T., Benhamou, S., Breed, G., et al. (2013). Spatial memory and animal movement. Ecol. Lett. 16, 1316–1329. doi: 10.1111/ele.12165
Forrester, T. D., Casady, D. S., and Wittmer, H. U. (2015). Home sweet home: fitness consequences of site familiarity in female black-tailed deer. Behav. Ecol. Sociobiol. 69, 603–612. doi: 10.1007/s00265-014-1871-z
Fortin, D., Beyer, H. L., Boyce, M. S., Smith, D. W., Duchesne, T., and Mao, J. S. (2005). Wolves influence elk movements: behavior shapes a trophic cascade in Yellowstone National Park. Ecology. 86, 1320–1330.
Gaulin, S. J. C., and FitzGerald, R. W. (1988). Home-range size as a predictor of mating systems in microtus. J. Mammal. 69, 311–319.
Gelman, A., Carlin, J. B., Stern, H. S., Dunson, D. B., Vehtari, A., and Rubin, D. B. (2013). Bayesian Data Analysis, 3rd Edn. New York, NY: CRC Press.
Howery, L., Bailey, D., and Laca, E. (1999). Impact of spatial memory on habitat use. Grazing Behav. Livest. Wildl. 70, 91–100.
Jakopak, R. P., LaSharr, T. N., Dwinnell, S. P. H., Fralick, G. L., and Monteith, K. L. (2019). Rapid acquisition of memory in a complex landscape by a mule deer. Sci. Nat. 100:e02854.1.
Jeltsch, F., Bonte, D., Pe’er, G., Reineking, B., Leimgruber, P., Balkenhol, N., et al. (2013). Integrating movement ecology with biodiversity research-exploring new avenues to address spatiotemporal biodiversity dynamics. Mov. Ecol. 1:6. doi: 10.1186/2051-3933-1-6
Jesmer, B. R., Merkle, J. A., Goheen, J. R., Aikens, E. O., Beck, J. L., Courtemanch, A. B., et al. (2018). Is ungulate migration culturally transmitted? Evidence of social learning from translocated animals. Science 361, 1023–1025. doi: 10.1126/science.aat0985
Johnson, D. S., and London, J. M. (2018). Crawl: an R package for fighting continuous-time correlated random walk models to animal movement data. Zenodo doi: 10.5281/zenodo.596464
Johnson, D. S., London, J. M., Lea, M.-A., and Durban, J. W. (2008). Continuous-time correlated random walk model for animal telemetry data. Ecology 89, 1208–1215.
Krausman, P., Hervert, J., and Ordway, L. (1985). Capturing deer and mountain sheep with a net-gun. Wildl. Soc. Bull. 13, 71–73.
Laca, E. A. (1998). Spatial memory and food searching mechanisms of cattle. J. Range Manag. 51, 370–378.
Lendrum, P. E., Anderson, C. R. Jr., Monteith, K. L., Jenks, J. A., and Bowyer, R. T. (2014). Relating the movement of a rapidly migrating ungulate to spatiotemporal patterns of forage quality. Mamm. Biol. 79, 369–375. doi: 10.1016/j.mambio.2014.05.005
Lendrum, P. E., Northrup, J. M., Anderson, C. R., Liston, G. E., Aldridge, C. L., Crooks, K. R., et al. (2018). Predation risk across a dynamic landscape: effects of anthropogenic land use, natural landscape features, and prey distribution. Landsc. Ecol. 33, 157–170. doi: 10.1007/s10980-017-0590-z
Marchand, P., Garel, M., Bourgoin, G., Duparc, A., Dubray, D., Maillard, D., et al. (2017). Combining familiarity and landscape features helps break down the barriers between movements and home ranges in a non-territorial large herbivore. J. Anim. Ecol. 86, 371–383. doi: 10.1111/1365-2656.12616
Martin, J. M., Basille, M. B., Moorter, B. V., Kindberg, J., Allaine, D., and Swenson, J. E. (2010). Coping with human disturbance: spatial and temporal tactics of the brown bear (Ursus arctos). Can. J. Zool. 88, 875–883. doi: 10.1139/Z10-053
Mcnamara, J., and Houston, A. (1987). Memory and the efficient use of information. J. Theor. Biol. 125, 385–395.
Merkle, J. A., Cherry, S. G., and Fortin, D. (2015). Bison distribution under conflicting foraging strategies: site fidelity vs. energy maximization. Ecology 96, 1793–1801. doi: 10.1980/14-0805.1
Merkle, J. A., Fortin, D., and Morales, J. M. (2014). A memory-based foraging tactic reveals an adaptive mechanism for restricted space use. Ecol. Lett. 17, 924–931. doi: 10.1111/ele.12294
Merkle, J. A., Sawyer, H., Monteith, K. L., Dwinnell, S. P. H., Fralick, G. L., and Kauffman, M. J. (2019). Spatial memory shapes migration and its benefits: evidence from a large herbivore. Ecol. Lett. 22, 1797–1805. doi: 10.1111/ele.13362
Mettke-Hofmann, C. (2014). Cognitive ecology: ecological factors, life-styles, and cognition. Wiley Interdiscip. Rev. Cogn. Sci. 5, 345–360. doi: 10.1002/wcs.1289
Mettke-Hofmann, C., and Gwinner, E. (2003). Long-term memory for a life on the move. Proc. Natl. Acad. Sci. U.S.A. 100, 5863–5866.
Middleton, A. D., Merkle, J. A., McWhirter, D. E., Cook, J. G., Cook, R. C., White, P. J., et al. (2018). Green-wave surfing increases fat gain in a migratory ungulate. Oikos 127, 1060–1068. doi: 10.1111/oik.05227
Mitchell, M. S., and Powell, R. A. (2012). Foraging optimally for home ranges. J. Mammal. 93, 917–928. doi: 10.1644/11-MAMM-S-157.1
Monteith, K. L., Bleich, V. C., Stephenson, T. R., Pierce, B. M., Conner, M. M., Kie, J. G., et al. (2014). Life-history characteristics of mule deer: effects of nutrition in a variable environment. Wildl. Monogr. 186, 1–62. doi: 10.1002/wmon.1011
Morales, J. M., Moorcroft, P. R., Matthiopoulos, J., Frair, J. L., Kie, J. G., Powell, R. A., et al. (2010). Building the bridge between animal movement and population dynamics. Philos. Trans. R. Soc. B Biol. Sci. 365, 2289–2301. doi: 10.1098/rstb.2010.0082
Morand-Ferron, J., Hermer, E., Jones, T. B., and Thompson, M. J. (2019). Environmental variability, the value of information, and learning in winter residents. Anim. Behav. 147, 137–145. doi: 10.1016/j.anbehav.2018.09.008
Morrison, T. A., Merkle, J. A., Hopcraft, J. G. C., Aikens, E. O., Beck, J. L., Boone, R. B., et al. (2021). Drivers of site fidelity in ungulates. J. Anim. Ecol. 90, 955–966. doi: 10.1111/1365-2656.13425
Muff, S., Signer, J., and Fieberg, J. (2020). Accounting for individual-specific variation in habitat-selection studies: efficient estimation of mixed-effects models using Bayesian or frequentist computation. J. Anim. Ecol. 89, 80–92. doi: 10.1111/1365-2656.13087
Nathan, R., Getz, W. M., Revilla, E., Holyoak, M., Kadmon, R., Saltz, D., et al. (2008). A movement ecology paradigm for unifying organismal movement research. Proc. Natl. Acad. Sci. U.S.A. 105, 19052–19059.
Northrup, J. M., Anderson, C. R. Jr., Gerber, B. D., and Wittemyer, G. (2021). Behavioral and demographic responses of mule deer to energy development on winter range. Wildl. Monogr. 208, 1–37. doi: 10.1002/wmon.1060
Northrup, J. M., Anderson, C. R. Jr., and Wittemyer, G. (2015). Quantifying spatial habitat loss from hydrocarbon development through assessing habitat selection patterns of mule deer. Glob. Chang. Biol. 21, 3961–3970. doi: 10.1111/gcb.13037
Northrup, J. M., Anderson, C. R. Jr., and Wittemyer, G. (2016). Environmental dynamics and anthropogenic development alter philopatry and space-use in a North American cervid. Divers. Distrib. 22, 547–557. doi: 10.1111/ddi.12417
Northrup, J. M., Anderson, C. R. Jr., and Wittemyer, G. (2014a). Effects of helicopter capture and handling on movement behavior of mule deer. J. Wildl. Manage. 78, 731–738. doi: 10.002/jwmg.705
Northrup, J. M., Shafer, A. B. A., Anderson, C. R. Jr., Coltman, D. W., and Wittemyer, G. (2014b). Fine-scale genetic correlates to condition and migration in a wild cervid. Evol. Appl. 7, 937–948. doi: 10.1111/eva.12189
Oliveira-Santos, L. G. R., Forester, J. D., Piovezan, U., Tomas, W. M., and Fernandez, F. A. S. (2016). Incorporating animal spatial memory in step selection functions. J. Anim. Ecol. 85, 516–524. doi: 10.1111/1365-2656.12485
Owen-Smith, N., Fryxell, J. M., and Merrill, E. H. (2010). Foraging theory upscaled: the behavioural ecology of herbivore movement. Philos. Trans. R. Soc. B Biol. Sci. 365, 2267–2278.
Péron, G., Duparc, A., Garel, M., Marchand, P., Morellet, N., Saïd, S., et al. (2018). Circadian periodicity in space use by ungulates of temperate regions: how much, when and why? J. Anim. Ecol. 87, 1299–1308. doi: 10.1111/1365-2656.12857
Piper, W. H. (2011). Making habitat selection more “familiar”: a review. Behav. Ecol. Sociobiol. 65, 1329–1351. doi: 10.1007/s00265-011-1195-1
Polansky, L., Kilian, W., and Wittemyer, G. (2015). Elucidating the significance of spatial memory on movement decisions by African savannah elephants using state-space models. Proc. R. Soc. B Biol. Sci. 282:20143042. doi: 10.1098/rspb.2014.3042
Pravosudov, V. V., Kitaysky, A. S., and Omanska, A. (2006). The relationship between migratory behaviour, memory and the hippocampus: an intraspecific comparison. Proc. R. Soc. B Biol. Sci. 273, 2641–2649. doi: 10.1098/rspb.2006.3624
R Core Team (2020). R: A Language and Environment for Statistical Computing. Vienna: R Foundation for Statistical Computing.
Riotte-Lambert, L., and Matthiopoulos, J. (2020). Environmental predictability as a cause and consequence of animal movement. Trends Ecol. Evol. 35, 163–174. doi: 10.1016/j.tree.2019.09.009
Sawyer, H., Merkle, J. A., Middleton, A. D., Dwinnell, S. P. H., and Monteith, K. L. (2019). Migratory plasticity is not ubiquitous among large herbivores. J. Anim. Ecol. 88, 450–460. doi: 10.1111/1365-2656.12926
Schlaegel, U. E., Merrill, E. H., and Lewis, M. A. (2017). Territory surveillance and prey management: wolves keep track of space and time. Ecol. Evol. 7, 8388–8405. doi: 10.1002/ece3.3176
Schlaegel, U. E., Signer, J., Herde, A., Eden, S., Jeltsch, F., Eccard, J. A., et al. (2019). Estimating interactions between individuals from concurrent animal movements. Methods Ecol. Evol. 10, 1234–1245. doi: 10.1111/2041-210X.13235
Schmidt, K. A., Dall, S. R. X., and Gils, J. A. V. (2010). The ecology of information: an overview on the ecological significance of making informed decisions. Oikos 119, 304–316.
Sih, A., Ferrari, M. C. O., and Harris, D. J. (2011). Evolution and behavioural responses to human-induced rapid environmental change. Evol. Appl. 4, 367–387. doi: 10.1111/j.1752-4571.2010.00166.x
Sikes, R. S. (2016). 2016 guidelines of the american society of mammalogists for the use of wild mammals in research and education. J. Mammal. 97, 663–688.
Snell-Rood, E. C., and Steck, M. K. (2019). Behaviour shapes environmental variation and selection on learning and plasticity: review of mechanisms and implications. Anim. Behav. 147, 147–156. doi: 10.1016/j.anbehav.2018.08.007
Spencer, W. D. (1992). Space in the Lives of Vertebrates: On the Ecology and Psychology of Space Use. Ph. D. dissertation. Tucson, AZ: University of Arizona.
Spencer, W. D. (2012). Home ranges and the value of spatial information. J. Mammal. 93, 929–947. doi: 10.1644/12-MAMM-S-061.1
Spiegel, O., and Crofoot, M. C. (2016). The feedback between where we go and what we know - information shapes movement, but movement also impacts information acquisition. Curr. Opin. Behav. Sci. 12, 90–96. doi: 10.1016/j.cobeha.2016.09.009
Sulikowski, D., and Burke, D. (2011). Movement and memory: different cognitive strategies are used to search for resources with different natural distributions. Behav. Ecol. Sociobiol. 65, 621–631.
Therneau, T. (2015). A Package for Survival Analysis in S. Version 2.38. Available online at: https://CRAN.R-project.org/package=survival (accessed January 18, 2021).
Therneau, T. M., and Grambsch, P. M. (2000). Modeling Survival Data: Extending the Cox Model. New York, NY: Springer.
Thurfjell, H., Ciuti, S., and Boyce, M. S. (2014). Applications of step-selection functions in ecology and conservation. Mov. Ecol. 2:4. doi: 10.1186/2051-3933-2-4
Trewhella, W. J., Harris, S., and McAllister, F. E. (1988). Dispersal distance, home-range size and population density in the red fox (Vulpes vulpes): a quantitative analysis. J. Appl. Ecol. 25, 423–434.
Tucker, M. A., Boehning-Gaese, K., Fagan, W. F., Fryxell, J. M., Van Moorter, B., Alberts, S. C., et al. (2018). Moving in the anthropocene: global reductions in terrestrial mammalian movements. Science 359, 466–469. doi: 10.1126/science.aam9712
Turchin, P. (1991). Translating foraging movements in heterogeneous environments into the spatial distribution of foragers. Ecology 72, 1253–1266.
Van Moorter, B., Visscher, D., Benhamou, S., Borger, L., Boyce, M. S., and Gaillard, J. M. (2009). Memory keeps you at home: a mechanistic model for home range emergence. Oikos 118, 641–652.
Vergara, P. M., Soto, G. E., Rodewald, A. D., and Quiroz, M. (2019). Behavioral switching in Magellanic woodpeckers reveals perception of habitat quality at different spatial scales. Landsc. Ecol. 34, 79–92. doi: 10.1007/s10980-018-0746-5
Wolf, M., Frair, J., Merrill, E., and Turchin, P. (2009). The attraction of the known: the importance of spatial familiarity in habitat selection in wapiti Cervus elaphus. Ecography 32, 401–410.
Keywords: short-term memory, movement ecology, mule deer, step-selection functions, space use, Odocoileus hemionus, cognition, long-term memory
Citation: Rheault H, Anderson CR Jr, Bonar M, Marrotte RR, Ross TR, Wittemyer G and Northrup JM (2021) Some Memories Never Fade: Inferring Multi-Scale Memory Effects on Habitat Selection of a Migratory Ungulate Using Step-Selection Functions. Front. Ecol. Evol. 9:702818. doi: 10.3389/fevo.2021.702818
Received: 29 April 2021; Accepted: 08 July 2021;
Published: 27 July 2021.
Edited by:
Tal Avgar, Utah State University, United StatesReviewed by:
Justine Becker, University of Wyoming, United StatesKevin Lee Monteith, University of Wyoming, United States
Copyright © 2021 Rheault, Anderson, Bonar, Marrotte, Ross, Wittemyer and Northrup. This is an open-access article distributed under the terms of the Creative Commons Attribution License (CC BY). The use, distribution or reproduction in other forums is permitted, provided the original author(s) and the copyright owner(s) are credited and that the original publication in this journal is cited, in accordance with accepted academic practice. No use, distribution or reproduction is permitted which does not comply with these terms.
*Correspondence: Helena Rheault, aGVsZW5hcmhlYXVsdEB0cmVudHUuY2E=