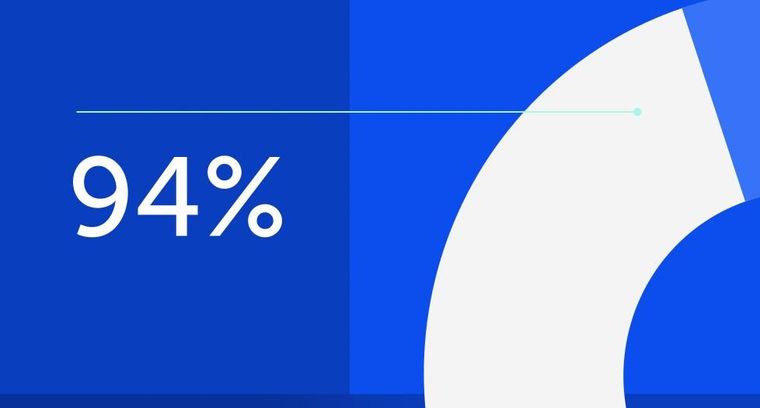
94% of researchers rate our articles as excellent or good
Learn more about the work of our research integrity team to safeguard the quality of each article we publish.
Find out more
REVIEW article
Front. Ecol. Evol., 21 July 2021
Sec. Conservation and Restoration Ecology
Volume 9 - 2021 | https://doi.org/10.3389/fevo.2021.698131
Many ecologists have lamented the demise of natural history and have attributed this decline to a misguided view that natural history is outdated and unscientific. Although there is a perception that the focus in ecology and conservation have shifted away from descriptive natural history research and training toward hypothetico-deductive research, we argue that natural history has entered a new phase that we call “next-generation natural history.” This renaissance of natural history is characterized by technological and statistical advances that aid in collecting detailed observations systematically over broad spatial and temporal extents. The technological advances that have increased exponentially in the last decade include electronic sensors such as camera-traps and acoustic recorders, aircraft- and satellite-based remote sensing, animal-borne biologgers, genetics and genomics methods, and community science programs. Advances in statistics and computation have aided in analyzing a growing quantity of observations to reveal patterns in nature. These robust next-generation natural history datasets have transformed the anecdotal perception of natural history observations into systematically collected observations that collectively constitute the foundation for hypothetico-deductive research and can be leveraged and applied to conservation and management. These advances are encouraging scientists to conduct and embrace detailed descriptions of nature that remain a critically important component of the scientific endeavor. Finally, these next-generation natural history observations are engaging scientists and non-scientists alike with new documentations of the wonders of nature. Thus, we celebrate next-generation natural history for encouraging people to experience nature directly.
Although the term natural history is familiar to all biologists, defining its boundaries is challenging. Tewksbury et al. (2014) suggested that natural history is “the observation and description of the natural world, with the study of organisms and their linkages to the environment being central.” Barrows et al. (2016) more concisely defined it as the “observational study of organisms in their environment.” Although definitions vary, all share the central theme of “direct observation and description of organisms, communities, and habitats, including attentiveness to associated geology, hydrology, and other physical factors” (Fleischner et al., 2017). Noticeably absent in this common understanding is an explicit mention of formal hypotheses or generalizable theory, which Wilson and Armesto (2006) note has led to the “prevailing view” that the natural history mode of biological sciences is “hopelessly old-fashioned, unscientific, sloppy, and useless.” Gould (2002) attributes this rift between natural history and biology to the misconception that rigorous science should not rely on anecdotal descriptive observations but should explain natural phenomena by general laws. These criticisms question the quality and objectivity of natural history and the very value of proper description in the scientific enterprise. The effects of these damning criticisms have reverberated throughout academic institutions (Disney, 1968), where taxon-specific biology and systematics courses such as mammalogy, ornithology, and ichthyology are increasingly no longer taught; natural history collections are being abandoned, fewer field courses are offered, peer-reviewed natural history publications are in steep decline, and research funding is being diverted away from natural history (Stokstad, 2003; Bury, 2006; McCallum and McCallum, 2006; Tewksbury et al., 2014). Yet, as others have argued (Tewksbury et al., 2014), we believe natural history maintains a valuable position in science and society and lament the decline of natural history in training, practice, and publication. In fact, we propose that natural history is far from doomed; it has entered a renaissance that we call “next-generation natural history.” This new phase has been revitalized by embracing advances in technology that transform the anecdotal character of few direct observations into scientific studies that rely on systematically collected observations using remotely sensed and non-invasive methods (Figure 1). Such technological advances propel natural history into a contemporary, meticulous, and systematic discipline with utility in both the hypothetico-deductive approach to science (Winker, 2004; Willson and Armesto, 2006; Tewksbury et al., 2014; Betts et al., 2021) and the conservation and management of species.
The hypothetico-deductive approach relies on a cycle in which patterns emerge from groups of observations. In the inductive reasoning part of the cycle, these patterns produce theories. In the deductive reasoning part of the cycle, these theories lead to hypotheses that generate predictions, which can then be tested with new observations (Figure 2). Natural history fits squarely in the section of observations and patterns within this cycle. Observations are a privileged part of this cycle as both the origin and validation of theory, and next-generation natural history provides substantial improvement to the quantity and quality of observations. We argue that these natural history observations are inherently important but also serve as fuel for the engine that is science.
Figure 2. Conceptual framework of the scientific method where observations both form the foundation of ecological theories through inductive reasoning and serve as the validation of ecological theories through deductive reasoning.
Next-generation natural history is once again encouraging students, scientists, and non-scientists to go into the field to non-invasively observe organisms using their newfound ability to generate many natural history observations at broad spatial and temporal scales. Because these types of biomonitoring and other next-generation natural history observations amplify and build upon natural history from field notebooks into graphical or auditory forms that are viewable by the world, we argue that next-generation natural history has the power to inspire enthusiasm and awe, and to motivate scientific and conservation efforts.
This transition to next-generation natural history is driven by technological advancements in both remote data collection and statistical advances to extract patterns in nature. These approaches have increased dramatically within the scientific literature in recent years (Figure 3). Here we review how electronic sensors such as camera traps and acoustic recorders, aircraft- and satellite-based remote sensing of nature, animal-borne biologgers, advances in genetics and genomics, and burgeoning community science programs have been used to generate robust next-generation natural history datasets (Figure 1). We also review how statistical advances allow us to analyze this growing quantity of data to make more reliable observational inferences about the natural world that are once again integrating natural history as a robust part of ecological science. Finally, we review how next-generation natural history is being applied to conservation and management and connecting more people with nature.
Figure 3. Temporal change in the use of next-generation natural history keywords and control keywords in a Web of Science literature search from 1965 to 2018. Keywords are grouped by technological category. Percent of total publications calculated by dividing the number of publications in any given year by the total number of publications that contain the keyword.
The advent of high-quality and affordable sensors deployed in natural environments to detect species now allows for the exploration of aquatic and terrestrial environments that were previously challenging to directly observe rigorously and systematically. These devices record what exists, where it exists, and when it exists within an ecosystem, facilitating descriptions of patterns and communities at unprecedented spatial and temporal scales. Camera traps are especially useful for capturing visual evidence of rare wildlife, as seen in studies focused on predators such as ocelots (Maffei et al., 2005). They also capture cryptic and elusive species, such as small burrowing rodents (Diete et al., 2014). Camera trapping is increasingly used to estimate wildlife population sizes (Maffei et al., 2005; Green et al., 2020) and life history traits (Burton et al., 2015; Norouzzadeh et al., 2018). Thermal imaging cameras have been used less frequently, but have found unique applications such as estimating bat populations non-invasively (Betke et al., 2008) or studying animal behavior by recording and categorizing emotional states of rhesus monkeys (Nakayama et al., 2005).
Similar to camera traps, passive acoustic monitoring is a fast-growing area of wildlife research (Laiolo, 2010; Shonfield and Bayne, 2017; Sugai et al., 2018). Autonomous recording units can be programmed for scheduled recording times over long survey durations of weeks or months without the need for direct observations, while providing permanent records of sound and increasing the quantity of survey data (Tegeler et al., 2012). Passive acoustic monitoring is a versatile approach that may be used with a variety of taxa that either vocalize or make audible movements, including birds, bats, terrestrial and marine mammals, fish, amphibians, and insects (Newson et al., 2017; Guazzo et al., 2019; Duchac et al., 2020). Recent examples of passive acoustic monitoring for next-generation natural history include tracking phenological shifts of frogs (Llusia et al., 2013) in relation to climate change, estimating population sizes of forest elephants (Wrege et al., 2017), and monitoring the impacts of anthropogenic noise on terrestrial and marine communities (Hatch et al., 2012).
A major advantage of next-generation natural history is that these sensors collect data on entire ecosystems including the biotic and abiotic components. Since these sensors create permanent records of images and sounds, data can be extracted later using continually improving methods, even if the study was only designed to monitor a single target species (Ruff et al., 2020). Due to the ability to detect multiple species, these sensors are increasingly utilized to monitor species interactions and communities. Remote cameras have been used to identify pollination services provided by bats (Frick, 2013), the relative seed dispersal services provided by bears and birds (Harrer and Levi, 2018; Figure 4F), small mammal foraging activity and behavior as secondary seed dispersers from bear scats (Shakeri et al., 2018), otherwise unobservable predator-prey interactions in the tree canopy (Linnell and Lesmeister, 2020), and patterns of species coexistence and partitioning (Lesmeister et al., 2015). Auxiliary data from camera trapping can provide information on the phenology of plants, daily temperature, and snowpack (Hofmeester et al., 2019), which can be used to study climate change. Passive acoustic monitoring is particularly well-suited for large-scale multi-species biodiversity monitoring (e.g., Furnas and Callas, 2015; Rich et al., 2019). These natural history datasets allow us to investigate landscape-scale questions such as the impacts of anthropogenic activities on the environment and wildlife. One example is soundscape ecology, which focuses on using acoustic indices to infer ecosystem status independent of species identification. Soundscape ecology has been applied to human well-being (Pijanowski et al., 2011), assessments of noise pollution on protected areas and endangered species (Buxton et al., 2017), and the creation of rapid and non-invasive biodiversity indices (Ross et al., 2018).
Figure 4. Examples of next-generation natural history research yielding unique insights about descriptions of how nature works. (A) New technologies can be combined. For example, Ruprecht et al. (2020) integrated information from GPS telemetry, camera traps to resight marked individuals, and genotypes from scats to estimate local population densities of four carnivores while testing a suite of methods for animal density estimation. (B) Levi et al. (2019) showed that flow-corrected eDNA rates (blue line) are predictive of actual salmon counts at a weir (black dots), suggesting the environmental DNA can be used to enumerate salmon and track their phenology on larger scales using water samples. (C) Animals can also now be counted from space, including animals as small as albatross, which Dolliver (2019) counted using imagery from WorldView-3. (D) Bioacoustics is now being used to detect Spotted Owls, Barred Owls, and myriad other species (Ruff et al., 2021) as part of Federal monitoring responsibilities under the Northwest Forest Plan. This will provide information about species occurrence, and migratory phenology of songbirds, from Central California to the Canadian border. (E) Metrics of species occurrence can now be linked to detailed measurements of habitat structure from remote sensing including lidar, which can provide a detailed look at tree size, density, composition, and structure. As such detailed characterizations become available on large spatial scales, a growing suite of species distribution models can help researchers predict where species are likely to occur and why they occur there. (F) Camera traps and eDNA have recently been used to describe species interactions such as the identity of seed dispersers for Devil’s club (Oplopanax horridus). By monitoring fruit clusters with cameras or swabbing consumed clusters to genetically identify bear species and sex, Harrer and Levi (2018) showed that brown bears were the dominant dispersers, but that black bears became important once salmon were available, that female bears dispersed more seeds than male bears, and that birds dispersed far fewer seeds than did bears. (G) Diet analysis was once the type of descriptive research that had become discouraged in the academy, but DNA metabarcoding has increased the throughput and taxonomy breadth such that Kartzinel et al. (2015) were able to publish diet analysis for seven African herbivores in Proceeding of the National Academy of Sciences. Their fundamental insight was that herbivores were partitioning the savanna vegetation structure, which facilitates coexistence. (H) Recent advances in biologging and analytical methods now allow researchers to determine not only where animals go, but what behaviors they are exhibiting, and how much energy they are expending by combining GPS with accelerometers. Here Wilmers et al. (2017) were able to characterize the locations of an adult male leopard that killed a domestic goat and then carried it across a river to feed. The leopard exhibited meandering behavior followed by a kill surge (10:00 on accelerometer trace III) followed by directed travel (IV), meandering across the river, followed by feeding.
A key challenge associated with surveys using non-invasive sensors is that they generate a massive amount of data, which has necessitated parallel advances in computer processing (Teixeira et al., 2019). Whereas an increased efficiency of data collection would generally constrain the storage, processing, analysis, and sharing of large datasets, advances in artificial intelligence (e.g., computer vision and deep learning), and high-performance computers have demonstrated the capability to relieve some of the bottlenecks in data management and processing. In addition, these advances make these technologies more accessible to ecologists because they are no longer cost prohibitive, limited to hardware that is physically accessible (e.g., cloud computing, remote computing, and virtual networks), or reliant on proprietary software for analyses (i.e., open-source software such as Program R) (Ruff et al., 2021).
Advances in computer processing provide a method to obtain data on the identity, demographic status, distribution, and even behavior of a wide variety of species. Computer algorithms can aid in identifying photographs and audio clips of species of interest (Norouzzadeh et al., 2018; Ruff et al., 2020) and even individuals (Schneider et al., 2019; Wood et al., 2021). In particular, deep learning methods such as convolutional neural networks have been successfully used to detect and identify animals from camera trap images (Norouzzadeh et al., 2018; Tabak et al., 2018) and acoustic recordings (Salamon and Bello, 2017; Ruff et al., 2020). Other applications of computer vision technologies to natural history include identifying plants from photographs (Wäldchen and Mäder, 2018), mapping vegetation structure (Dandois and Ellis, 2013), reconstructing colonies of Gentoo penguins (Pygoscelis papua; McDowall and Lynch, 2017), and re-identifying individual animals based on unique markings (Schneider et al., 2019).
The combination of electronic sensors, computational power, and advances in artificial intelligence are transforming how we catalog nature, describe species interactions, and study organism-environment interactions. While these technologies are being used for a variety of purposes, including hypothesis-driven research, we have reviewed their use in descriptive research of what is happening in nature, which we consider natural history in its truest sense. These observations are next-generation natural history because they are systematically collected with remote devices, but the resulting descriptions do not have the anecdotal feel of field observations by individual naturalists in nature. These works have found outlets in prestigious academic journals, which we argue demonstrates and inspires the renaissance of natural history research.
Perhaps no technological advancement applied to next-generation natural history research has advanced as quickly as molecular methods in genetics and genomics. In particular, the advent of environmental DNA (eDNA) now allows us to detect species and individuals using DNA shed into the environment rather than handling organisms themselves in much the same way that camera traps or passive acoustic monitoring allow us to detect organisms that we do not see or hear directly. The first use of contemporary eDNA to detect extant vertebrates was by Ficetola et al. (2008) to detect invasive bullfrogs. Such attempts to detect single species use real-time quantitative polymerase chain reaction (qPCR) or digital PCR to selectively amplify and quantify DNA of target organisms. In much the same way that natural historians catalog species at field sites from direct observations, eDNA now allows researchers to catalog species in aquatic and terrestrial systems without directly observing them (Figure 1). For aquatic systems, species, even those that are difficult to identify using morphological characteristics, can be identified through water samples. Single-species detection has proven to be particularly useful for monitoring the frontier of invasions in aquatic systems by vertebrates and invertebrates such as Asian carp (Hypophthalmichthys spp.) (Jerde et al., 2013), American bullfrog (Lithobates catesbeianus) (Dejean et al., 2012), and rusty (Orconectes rusticus) and signal crayfish (Pacifastacus leniusculus) (Larson et al., 2017). Recent evidence suggests that the quantity of DNA detected by real-time qPCR or digital PCR can provide reliable information about the abundance or biomass of organisms in addition to an occupancy record (Levi et al., 2019; Pochardt et al., 2020; Figure 4B).
In addition to single-species detection, high-throughput sequencing now allows for the amplification and sequencing of short taxonomically informative fragments of DNA called “barcodes” from environmental samples in order to profile whole communities. This technique, called DNA metabarcoding, has been used to reconstruct historic diversity of flora and fauna using sediments (Willerslev et al., 2003), to construct present-day plant biodiversity using samples taken from the air (Kraaijeveld et al., 2015), fish and amphibian diversity in freshwater systems (Thomsen et al., 2012) and marine environments (Port et al., 2016), and a broad range of eukaryotes in soil (Drummond et al., 2015). The substrates from which we can amplify DNA are ever expanding and are reviewed by Bohmann et al. (2014) and Deiner et al. (2017). Moreover, metabarcoding has been performed on stomach contents and scat samples to study the diets of numerous taxa including birds (Jedlicka et al., 2013), marine fish and mammals (Leray and Knowlton, 2015; Berry et al., 2017; Jakubavièiûtë et al., 2017), freshwater fishes (Guillerault et al., 2017), terrestrial mammals (Shehzad et al., 2012; Srivathsan et al., 2015; Iwanowicz et al., 2016), and even highly cryptic species such as flying insects (Kaunisto et al., 2017) and insectivorous bats (Bohmann et al., 2011; Galan et al., 2018). As the cost of sequencing has declined, metabarcoding has been applied on massive spatial extents to study the biogeography of animal diets (Roffler et al., 2021). Some of the more interesting applications have taken diet analysis a step further and inferred resource partitioning among large-bodied African herbivores (Kartzinel et al., 2015) and population structure of vampire bats (Bohmann et al., 2018) from diet metabarcoding data (Figure 4G).
High-throughput sequencers are increasing in power at an astounding pace such that entire genomes are now readily resequenced and thousands of environmental samples can be pooled for metabarcoding on a single sequencing run. Recent advances in sequencing such as PacBio’s SMRT technology have increased the length of sequences from hundreds of base pairs to up to 100 kb using single-molecule sequencing (Heather and Chain, 2016). Miniaturization and portability are the next frontiers of sequencing. Oxford Nanopore Technologies has developed the minIONTM and smidgIONTM—portable sequencers that attach to a laptop or cellular phone, respectively—promising to bring the power of genomic sequencing to the field or rural areas with little access to electricity or infrastructure. The cost to sequence DNA has decreased by orders of magnitude over the last decade and the development of benchtop sequencers that produce rapid results allow for large-scale biomonitoring (Faria et al., 2016; Goordial et al., 2017).
While DNA metabarcoding has enabled either qualitative or quantitative inventories of ecological communities in the wider environment, this technique is now being used to describe the ecosystems found on and within organisms. For example, sequencing to identify the microbial community has revealed that microbial composition on the skin of amphibians varies among species, changes during metamorphosis (Kueneman et al., 2014; Chai et al., 2018), and can play a key role in resistance to chytridiomycosis (Batrachochytrium dendrobatidis) (Harris et al., 2009). In another system, the diversity of gut microbes of black howler monkeys (Alouatta pigra) was higher in populations living in pristine rainforests compared to those in fragmented forests, suggesting that microbiomes can be used as habitat quality indicators (Amato et al., 2013). Moreover, next-generation natural history has revealed that vultures harbor bacteria toxic to other species within their guts, suggesting co-adaptation of host and microbiome to a specific diet (Roggenbuck et al., 2014). Conversely, the giant panda retains a gut microbiota more similar to its omnivorous relatives despite its herbivorous diet indicating a strong influence of host phylogeny on microbe composition (Xue et al., 2015).
An offshoot of eDNA permits the use of invertebrate-derived DNA (iDNA) to measure vertebrate biodiversity from the gut content of hematophagous (Schnell et al., 2012; Kocher et al., 2017; Tessler et al., 2018), coprophagous, and sarcophagous (Calvignac-Spencer et al., 2013; Rodgers et al., 2017) invertebrates. In essence, this advancement allows researchers to take advantage of invertebrates to sample the vertebrate community, which can be difficult to survey directly. Once collected, processed and genetically identified, these data can be fed into occupancy models for species- and community-level monitoring (Abrams et al., 2019; Tilker et al., 2020).
The combination of traditional morphological techniques with molecular methods has enabled significant advancements in phylogenetic resolution and systematics analyses (Rokas et al., 2003; Wiens et al., 2010; Giribet, 2015; Pyron et al., 2015). Delimitation of species has been improved by the use of molecular tools (Leaché et al., 2014), and reinterpretations of the fossil record using molecular data have given us new insights into the tree of life on different taxonomic levels (Delsuc et al., 2005; Dunn et al., 2008; Meusemann et al., 2010; Hug et al., 2016). Additionally, phylogenetics, phylogeography, and population genetics have provided a more complete picture of global biodiversity (Cutter, 2013; Soltis and Soltis, 2016; Vacher et al., 2020). These next-generation genetic techniques critically rely on traditional natural history collections that provide voucher specimens that link organisms and their genomes to specific places and times.
While these genetic methods can be used to test specific hypotheses, we highlight research that helps describe what species occur where, how they relate to each other, and where they are situated on the tree of life. All such applications are curiosity-driven descriptive research that highlight the renaissance of natural history.
Often, one of the challenges with natural history research has been the collection of sufficient observations in space and time to understand large-scale patterns. By mobilizing public participation to collect observations for scientific research, community science (previously called citizen science) has emerged as a critical tool for natural history research (Bonney et al., 2009; Dickinson et al., 2010; Theobald et al., 2015). The availability of new affordable devices and advances in genetic methods have improved the validity of community science observations (Kamenova et al., 2017; Beck et al., 2018). Not only can community scientists collect their own observations, non-specialists across the globe can place and retrieve devices, such as camera traps and bioacoustic recorders, or collect samples from the environment that can be processed later by specialists to detect species or individuals. As such, community scientists have expanded the spatial (Dickinson et al., 2012; McKinley et al., 2017) and temporal (Magurran et al., 2010; Dickinson et al., 2012) scales that can be monitored. Public participation has substantially increased the number of observations and the diversity of taxonomic groups observed (Turrini et al., 2018) and thereby the resolution of natural history data at relatively minimal cost (McKinley et al., 2017). Our knowledge of natural history has increased while encouraging the public to develop connections with nature. These new technologies are expanding the pool of people engaged in natural history, which may help increase diversity within the discipline. Research dependent on community scientists has thereby raised awareness of multiple environmental problems (Dickinson et al., 2012; McCormick, 2012).
Some of the most outstanding examples of community science are the Christmas Bird Count (CBC) established in 1900 (Butcher et al., 1990), the Breeding Bird Survey (BBS) initiated in 1965 (Hudson et al., 2017), and the Garden Bird Feeding (GBF) Survey commenced in 1970. Thousands of people have participated in data collection useful to understand not only population trends of different bird species but also to improve our knowledge on natural history of parental care behavior (Zárybnická et al., 2017), habitat selection (Sauer et al., 1996; Hoffman and Smith, 2003), diet (Schmidt and Ostfeld, 2003; Boyle and Sigel, 2015), and species distributions (La Sorte and Thompson, 2007; Kirchman and Van Keuren, 2017).
New technologies have allowed better integration of public participation in research through platforms such as iNaturalist, eBird, Zooniverse, and Reef. These platforms facilitate rapid information sharing, which allows participants to manage their observations using globally accessible databases. Beginning in 2002, the Cornell Lab of Ornithology and the National Audubon Society launched eBird, which has grown such that hundreds of thousands of community scientists have entered nearly 1 billion records. iNaturalist, launched in 2008, works as a social network to which pictures of fauna and flora can be submitted, identified, and validated by other iNaturalist users. These georeferenced and dated observations from multiple platforms are shared with the Global Biodiversity Information Facility. Finally, Zooniverse launched in 2009 with the purpose of connecting volunteers with researchers to facilitate data processing in many disciplines. The diversity of disciplines and total number of users make this science web portal one of the most popular and successful community science platforms (Hecker et al., 2018; Adler et al., 2020).
Data collected through these platforms are made public and offer researchers, land managers, and educators different ways to be involved in research. Hundreds of natural history papers have used these data and filled knowledge gaps on changes in species abundance (Breed et al., 2013) and distribution (Gomes et al., 2019; Vendetti et al., 2019), biodiversity, plant and animal reproductive phenology (Chandler et al., 2017; Li et al., 2019), and have informed conservation actions (Robinson et al., 2018; Schuttler et al., 2018).
Advances in computing have also interfaced with community science to allow for the processing of large data sets. Web-based applications allow citizens to train and then tag large numbers of photos (Swanson et al., 2016) and bird calls (Priyadarshani et al., 2018). These large datasets assembled with the help of community scientists can now be processed with computer vision and deep learning to automate species identification (Trouille et al., 2019). As a result, large community science repositories like iNaturalist, Pl@ntNet, Merlin, and SongSleuth now include automated identification of photos and recordings uploaded by users. This has recently advanced toward automated identification of individual animals. For example, the non-profit organization Wild Me uses computer vision to identify unique individuals of a variety of species based on spot patterns. This technique has been integrated with community science to identify unique whale sharks (Rhincodon typus) from videos taken by scuba divers, tourists, and scientists. Wild Me is now mining YouTube videos, where the tags and text in videos are used to identify the spatial location, and computer vision identifies the unique individual. By so doing, Wild Me is able to reconstruct movement patterns and estimate survival of sharks (McKinney et al., 2017).
In addition to detecting which organisms are present in the environment, natural history research endeavors to describe how they behave. Advancements in biologging, or the “use of miniaturized animal-attached tags for logging and/or relaying of data about an animal’s movements, behavior, physiology and/or the environment” (Rutz and Hays, 2009) represents a goldmine for such descriptions. The use of biologging overcomes many of the challenges previously encountered when seeking to observe animals undisturbed in their natural environment (Rutz and Hays, 2009), particularly for cryptic or wide-ranging species (Wilmers et al., 2015). Studies utilizing biologging are often exploratory and seek to describe novel patterns or behaviors and is why natural history is a beneficiary of this advancement in technology.
A brief chronology of biologging applications indicates how much progress has been made since their inception. Among the first uses of biologgers came from Scholander (1940) who attached gauges to whales to study diving depths. Telemetry devices using radio signals to transmit information on animal locations were first used in the early 1960s on grizzly bears in Yellowstone National Park (Hebblewhite and Haydon, 2010). The advent of the Global Positioning System—first used in biologging on caribou in Ontario in the early 1990s (Rodgers, 2001)—automated the acquisition and increased the precision of animal positional data. Currently, biologgers provide much more information than animal locations (reviewed in Wilmers et al., 2015). They provide animal activity patterns and acceleration and information about the external environment, often in near real-time (Wang et al., 2015; Wilmers et al., 2017; Figure 4H).
Demonstrating the renewed appreciation for next-generation natural history in the academy, Wilson et al. (2013) published a description of cheetah hunting behavior in the journal Nature. Similarly, Williams et al. (2014) described the energetic costs of multiple puma (Puma concolor) behavior in the journal Science. Advances in biologging have also prompted several recent special features in scientific journals (Cagnacci et al., 2010; Börger et al., 2020). Thus, the importance of next-generation natural history observational research is now recognized by top-tier journals, which was not the case for the former generation of natural history. Similarly, analytical methods for biologging data have emerged; there now exist at least 58 user-contributed R packages for analyzing animal movements (Joo et al., 2020).
A continual challenge in biologging is to make products that are smaller, lighter, less expensive, and have more efficient batteries. For example, the first GPS collars were suitable only for the largest animals (Tomkiewicz et al., 2010) given the ethical rule that sensors should weigh a very small percentage of the animal’s body weight (<5% of an individual’s body mass; Sikes and Animal Care and Use Committee of the American Society of Mammalogists, 2016). Miniaturization is increasingly permitting smaller subjects to be studied, and cost reductions are allowing for larger sample sizes and more widespread use (Ripperger et al., 2020). Miniaturizing is excelling with initiatives such as the Icarus project1, which is using space-based receivers to facilitate extremely small transmitters for the study of animal movement. Sensors have now been attached to organisms ranging from bees to lions, passerines to fish, snakes to whales, and most taxa in between (reviewed in Cooke et al., 2004). Given the impressive trend of animal-borne sensors becoming smaller and more efficient in the last decade, the future of biologging seems bright.
The diversity of applications of biologging to the field of natural history is impressive. The flight mechanics of insects have been studied in detail (Ando et al., 2002; Kutsch, 2002), and previously undescribed transcontinental bird migrations spanning tens of thousands of kilometers have been documented (Egevang et al., 2010; McKinnon et al., 2017). Video cameras mounted on secretive woodland caribou have provided a rare glimpse into the predator evasion strategy of an adult female protecting its young calf (Thompson et al., 2012), and the time it takes a brown bear to consume a moose calf has been recorded to the nearest minute (Brockman et al., 2017). Whale foraging behaviors, including cooperative hunting, dive patterns, and prey choice has been linked to whale morphology, prey distribution, and oceanic conditions through the use of multisensory tags attached to whales with suction cups (Goldbogen et al., 2013, 2017; Friedlaender et al., 2020). Accelerometers have taught us that pumas (Puma concolor) can mitigate the high energetic hunting costs of pouncing by using cryptic tactics such as stalking (Williams et al., 2014), but that canids gain more energy per unit effort employing a cursorial cooperative hunting strategy (Hubel et al., 2016). These illuminating examples only scratch the surface of what has already been done, let alone what the future holds. We believe biologging will continue to produce the detailed observations that underlie next-generation natural history and will only be limited by the imagination of a new generation of naturalists.
Remote sensing has been used to characterize the environment where species occur but is increasingly being used to detect species themselves. Remote sensing involves the acquisition of information about organisms and processes on Earth via aircraft or satellite including Radio Detection and Ranging (radar), Light Detection and Ranging (lidar), multispectral imagery, hyperspectral imagery, thermal imagery, and acoustic sensing. Aerial observations have long contributed to our understanding of plant community growth and succession regimes (Graham, 1997; Asner et al., 2015; Merkle et al., 2016) and animal distribution (Richard et al., 2010) and aggregation (Miller et al., 1998; Slooten et al., 2006). Within the last decade, unmanned aerial vehicle (“drone”) surveys have greatly improved the accuracy of aerial surveys (spatial resolution as high as 0.82 cm/pixel) (Jones et al., 2006; Getzin et al., 2012; Hodgson et al., 2016, 2018; Figure 4E). The advent of pole-to-pole sensing via satellites has expanded these studies from local to global analyses using multi-decadal time series (Boyd and Danson, 2005). At nearly the same spatial resolution, satellite sensing has surpassed the travel radius of a single researcher, and provides critical, frequent data, even in harsh climates (Cavalieri et al., 2003; Castañeda et al., 2005), areas of political instability, or human-wildlife conflict (De Wulf et al., 1988; Nackoney et al., 2014). In sensitive ecosystems, rare and sensitive organisms can benefit from this type of monitoring because in situ sampling is non-essential, thereby limiting the researcher footprint (Kellenberger et al., 2009). In addition, remote sensing facilitates global understanding of biotic data by placing local findings (e.g., deforestation) in a larger network of interconnected regions (Vargas Zeppetello et al., 2020).
For the last 50 years, satellite remote sensing has documented landscape-level modifications due to human encroachment (Harper et al., 2007), spread of invasive species (Pengra et al., 2007), plant and animal diseases (Colwell, 1996; Liu et al., 2006; Bruno et al., 2007), and fire (Nepstad et al., 1999). The recent availability of satellite based lidar data from the Global Ecosystem Dynamics Investigation mission now opens a new window of opportunity to understand fine scale vegetation structure at a global scale, including predictions of tropical forest tree species richness (e.g., Marselis et al., 2020). These data collected at the global scale can then be used as explanatory variables to model resource selection and identify suitable habitat for target species (Jarvis et al., 2005).
With advances in spatial resolution in satellite imagery, scientists can also detect and enumerate individual plants and animals in open environments. This has resulted in the identification of rare species (Jarvis et al., 2005), subpopulations, and global censuses (Lynch and LaRue, 2014). Automated image analysis has complemented advances in remote sensing to greatly increase the efficiency of natural history research (Brodrick et al., 2019). In cases where individual animals visually contrast with their environments, feature extraction and object-based image analysis have been used to count animals from aerial imagery, including waterbirds (Dolliver, 2019; Figure 4C), caribou (Rangifer tarandus; Laliberte and Ripple, 2003), and dugongs (Dugong dugon (Hodgson et al., 2013). Even high-resolution satellite images of the entire planet have been used to census large vertebrates, including marine mammals in vast oceanic areas (Borowicz et al., 2019). Pattern recognition methods have been used to identify individual marine mammals such as gray seals (Halichoerus grypus; Hiby and Lovell, 1990). Moreover, high-resolution imagery has documented the decline in species (Avery and Haines-Young, 1990) and identified aggregations of species in previously unknown places (Fretwell et al., 2015).
A variety of non-satellite platform technologies has enabled detection on smaller scales. These technologies have recorded the presence of individuals without dependence on visible light, including radar to map breeding habitat (Cortez et al., 2013) and aerial infrared imagery to estimate population size (Udevitz et al., 2008). Locally and globally, the availability of new remote sensing technologies continues to emerge and push the boundaries of spatial (e.g., Moxley et al., 2017) and spectral (e.g., Sandino et al., 2018) analyses. The continued advancements of these remote-sensing technologies have allowed for the extraordinarily detailed descriptions of where species are, how many of each species there are, and the habitats that influence their occurrence.
Along with the efficiency gains of recent technological advances in species detection, comparable advances in statistical approaches have allowed us to make sense of this massive quantity of data to produce robust natural history inferences. Thus, such efficiency gains, which enables the detection of hundreds to thousands of species (e.g., DNA metabarcoding) in hundreds to thousands of samples, have been followed by advances in statistical approaches. Although these methods can be used for a variety of applications including hypothetico-deductive research, they are essential to describing patterns of diversity, occurrence, and abundance of the large datasets generated by next-generation natural history.
The emergence of new statistical methods for analyzing community and metacommunity data, in particular (latent variable) joint species distribution models, now allow an explicit and flexible explanation of community composition by species’ environmental preferences, as well as biotic interactions and spatial autocorrelation (Warton et al., 2015; Bush et al., 2017; Ovaskainen et al., 2017). The ability to collect community data at broad scales and analyze such data with new statistical approaches effectively solves many of the problems around data limitation and analysis that community ecology has long struggled with, especially when using both techniques in synergy (Bohmann et al., 2014; Warton et al., 2015; Creer et al., 2016; Bush et al., 2017; Ovaskainen et al., 2017). For example, joint species distribution models require large datasets, which may limit their application to conventional, small metacommunity datasets. The “novel community data” generated by sequencers and sensors is much better suited for these complex models, especially when quantifying changing biotic interactions over scales, in space, and with the environment (Ovaskainen et al., 2016).
Beyond community-level data, statistical advances can correct for imperfect detection of species in natural history observations to produce reliable information about species occurrences. For example, the development of occupancy models (MacKenzie et al., 2018) has allowed next-generation natural history observations, such as those collected with camera traps, bioacoustics, or environmental DNA, to produce more robust inference by disentangling the probability that a species is detected from the probability that it occurs at a given spatial location or under a given set of environmental conditions. Dynamic occupancy models have extended this framework to estimate rates of patch colonization and extinction, which can be linked to biotic or abiotic predictors in long-term monitoring programs (Royle and Kéry, 2007). In addition, these models have been extended to co-occurrence models to quantify species interactions by asking how the presence of one species influences the probability that another is also present while accounting for landscape covariates that may influence each species independently (Bailey et al., 2009).
While species occupancy is an important state variable in ecology, more recent statistical approaches allow for the estimation of population density, and not just probability of occurrence, from a wide variety of natural history datasets. Perhaps the most transformative of these approaches is the class of spatial population models that include spatial capture-recapture, spatial mark-resight, and spatial correlated count models (Royle et al., 2013), which overcome the sensitivity of mark-recapture density estimates to closure assumptions previously corrected for by placing a buffer around a study area. Spatial capture-recapture is the most data-hungry of these approaches because it requires the identification of unique individuals such as through camera trapping of uniquely marked individuals (e.g., jaguars, Sollmann et al., 2011) or with non-invasive genetic sampling (Sollmann et al., 2013; Augustine et al., 2019). As the efficiency of fecal genotyping has increased with improved molecular approaches (Eriksson et al., 2020) and the use of scat detection dogs, spatial capture-recapture has become the canonical statistical framework for estimating population density when animal identity is known. Genetic data can also be incorporated using close-kin mark recapture (first proposed by Skaug, 2001) based on the principle that an individual’s genotype can be considered a recapture of its parents’ genotypes, which allows abundance to be estimated based on the number of parent-offspring pairs in a sample. If only a portion of animals can be uniquely identified, such as when combining cameras with a subset of marked animals, spatial mark-resight models can be employed to produce density estimates consistent with spatial capture-recapture analysis (Ruprecht et al., 2020; Figure 4A).
Statistical approaches purporting to estimate the population density of animals when no individuals can be uniquely identified represent the most challenging problem, but attempts at this include the random encounter model (Rowcliffe et al., 2008), N-mixture models (Royle, 2004), spatial correlated count models (Chandler and Royle, 2013), and more recently time-to-event and space-to-event models (Moeller et al., 2018). While these approaches have received some support in the literature, they can be highly sensitive to the violation of assumptions and further research is required to assess the conditions under which some of them yield robust estimates of population density (Duarte et al., 2018; Ruprecht et al., 2020).
Statistical approaches have similarly advanced for the analysis of a growing number of large animal movement datasets. Resource selection functions and their variants were first used to identify how animals used landscapes at multiple spatial scales, but have increasingly been expanded to study how prey respond to predators (Basille et al., 2015; Rayl et al., 2018) and how animal movement rates vary according to landscape properties (Avgar et al., 2016). Such estimation of animal movement parameters can now be used to infer the behavioral state of animals using state-space models that account for imperfect locations (Patterson et al., 2008), mixture-models (Morales et al., 2004), and hidden Markov models (Franke et al., 2004), which can be used, for instance, to distinguish active foraging behavior from movements between feeding patches and migration.
As new technologies in remote sensing have allowed us to measure landscape variables with heightened accuracy, advances in statistical approaches have been required to link landscapes to the occupancy, density, space use, and distribution of individual species or whole communities. The resulting datasets are largely (but not exclusively) descriptive in nature. These statistical advances applied to the large datasets afforded by new technologies produce natural history insight that can lead to new research questions and help address applied problems in conservation and management in a manner distinct from how natural history was conducted a generation ago.
The technological advances discussed above are allowing ecologists to engage in next-generation natural history. As before with traditional natural history, this natural history provides the observational data necessary for future ecologists and conservationists to develop new hypotheses pertinent to ecological theory, conservation, and management. Next-generation natural history, however, provides robust, large datasets of observations that cover large spatial and temporal extents. Unlike traditional natural history observations that produced anecdotes of what individuals saw and heard, next-generation natural history observations are auditable, verifiable, and archivable, thereby ensuring more reproducible research. The archivable nature of next-generation natural history allows for researchers to go back in time and ask and answer questions about long-term changes or apply new technology that was not available when the data were initially collected. Thus, next-generation natural history description no longer depends on the identification, interpretation of behavior, and documentation by a few observers for a small set of observations, making it less “old-fashioned” and “sloppy,” that is, more objective. With next-generation natural history, hypotheses pertinent to ecological theory, management, and conservation can be developed based on richer and more objective data, thereby promising a more robust theorization of the natural world.
In addition to serving as the jumping off point for hypothesis-driven research probing ecological theory, next-generation natural history provides greater objectivity and efficiency in conservation and management. To highlight the efficacy of next-generation natural history, we consider a case study of the famous long-term study of the federally threatened northern spotted owl (Strix occidentalis caurina) (Figure 4D). Due to precipitous population declines, increased uncertainty associated with estimates of vital rates for small populations, and because non-invasive methods can be effective for detecting and tracking population change of spotted owls (Duchac et al., 2020; Lesmeister et al., 2021), this study is now transitioning from traditional mark-resighting of individuals to passive acoustic monitoring focused on occupancy. From a long-term population monitoring standpoint there are several key advantages to using next-generation natural history methods. First, extended deployments of autonomous recording units have dramatically increased effective survey coverage from a handful of study areas to broad spatial coverage of the forests in the Pacific Northwest. Further, the transition to next-generation natural history methods allows for simultaneous monitoring of an important congeneric competitor (barred owl; S. varia) that contributes to spotted owl population declines, in addition to providing a permanent record of all vocal species at survey locations. These observations can be linked to remote sensing data to describe and then generate hypotheses about the relationships and effects of forest structure, abiotic factors, and anthropogenic disturbance. This approach would not be possible without the technological advancements that enable long-term passive acoustic monitoring of forests, hundreds of terabytes of acoustic data to be affordably stored and archived, and the advent of high-performance computers and artificial intelligence to automate the classification of hundreds of thousands of hours of acoustic recordings (Ruff et al., 2021).
The northern spotted owl case study is interesting not only for the shift to using next-generation natural history to increase the spatial extent and scope of the project; the basic ecological knowledge about this species gained by Forsman et al. (1984) prompted one of the greatest changes to federal land management through the Northwest Forest Plan of 1994. Thus, natural history and next-generation natural history can play a crucial role in conservation and management by addressing one of the key limitations to conserving biodiversity–a dearth of basic ecological knowledge and a poor understanding of community ecology.
Such a lack of understanding persists with other taxa and continues to restrict our ability to respond to disturbances (e.g., timber harvest and wildfire) at local, regional, and global scales. Despite protection of remaining old-growth forests on federal lands through the Northwest Forest Plan, numerous species became imperiled in the Pacific Northwest (e.g., marbled murrelet, Brachyramphus marmoratus; red tree vole, Arborimus longicaudus; Humboldt marten, Martes caurina humboldtensis). Following next-generation natural history studies that described the population size using mark-resight models (Linnell et al., 2018) and diet of the species through DNA metabarcoding (Eriksson et al., 2019) among other studies, the Humboldt marten was formally listed as a threatened species under the Endangered Species Act, thereby increasing the protections for the species and increasing effort toward long-term conservation of the species.
Another example of next-generation natural history informing management and conservation at the local scale is possible from describing “what killed this animal?,” which can have significant consequences for public perception, management, and future policy in cases of livestock depredations and crop damage. This question can be answered simply by swabbing carcasses for DNA in a timely fashion (Williams et al., 2003; Hopken et al., 2016) or through biologging the predator (Bacon et al., 2011; Johansson et al., 2015). More globally, describing “which tusks belong to the same elephant and where did it come from?” in illegally trafficked ivory tusks can reveal transnational criminal networks (Wasser et al., 2018) because genotypes from numerous source populations spanning entire continents were described through next-generation natural history. Next-generation natural history is therefore increasingly producing data streams that are allowing for robust evidence-based conservation and management. Used effectively, next-generation natural history can rapidly inform managers, organizations, and nations that currently have limited data concerning which organisms are present, how organisms are distributed, and why organisms are where they are. This rapid infusion of data to data-poor areas can help identify mechanisms of species decline and propel conservation efforts.
As ecologists are once again doing detailed descriptive research, the distinction between natural history and ecology have become blurred. We argue that researchers should embrace detailed descriptions of nature as a critically important scientific endeavor. Without the need for hypotheses to drive data analyses, natural historians can make exciting discoveries by looking for patterns that may have been overshadowed by the results of hypothesis testing (Yanai and Lercher, 2020). Further, unique natural history insights are finding their way into journals of the highest caliber, suggesting that descriptive natural history research is experiencing a renaissance through the uniquely detailed observations made possible by next-generation natural history.
As dedicated natural historians, we deplore the decline in taxon-specific biology and systematics courses. Through these formative courses, students learn how to interpret and understand the natural world with direct first-hand experience. Even more tragically, this decline has not been replaced with adequate training in the requisite technical skillsets necessary to conduct next-generation natural history. We are failing to train the next generation of ecologists in both regards. Both observation-based taxon-specific biology courses and technical skillsets such as genetics wet lab techniques, proficiency in computer programming languages, and a more robust understanding of statistics, are necessary for future ecologists to push the boundaries of next-generation natural history.
We acknowledge that a possible result of these technological advances is a growing gap between natural history conducted in developing and economically wealthy nations. Even though new technologies have greatly reduced the costs of obtaining each sample, much of the equipment necessary to acquire and process data (e.g., sequencers, LiDAR sensors mounted on planes, high-performance computers) remains quite expensive and requires regular maintenance. Thus, next-generation natural history must involve collaboration and scientists should strive to conduct ethical science and leverage interests in these technologies to enhance the diversity in our science.
Naturalists often lament that the technological advances above are detrimental to developing connections between humans and nature, despite their promise of more rigorous science and useful applications. We do not dispute that, for some people, this is a possible consequence, and these technologies may exacerbate the division of labor between fieldwork, data analysis, and interpretation. But many modern ecologists are increasingly bound to the office anyway. For example, post-doctoral scholars are typically handed datasets that they did not collect themselves, and principal investigators often desk-manage multiple projects and their personal life at the expense of extensive field seasons. In addition, the competition to secure an academic job and the drive toward increasing citation metrics for career advancement unfortunately disincentivize years-long hard-won field studies where the data are collected by the person leading the research. We, however, acknowledge that a solid amount of direct field experience can be invaluable if not irreplaceable—particularly in the formative years of many ecologists—as a source of inspiration, intuition, and baseline information that can serve as a “reality-check” in building sensible biological hypotheses for many years to come. Acquisition of direct anecdotal or systematic observational data, even if the observer is armed with nothing more than a pen and paper, are also an important step to encouraging participation and gaining a robust gut-feeling about how nature works. These anecdotal field experiences importantly guide what to measure and which metrics to use (i.e., study design) and help guide more effective evaluation of patterns, generation of realistic models, and testing of hypotheses. While collecting field data is still time consuming and labor intensive, next-generation natural history can greatly boost this field investment into hyper-dimensional datasets that often span very large spatial scales with substantial replication.
The higher-quality datasets generated by next-generation natural history motivate landscape to regional scale eDNA or community-wide DNA surveys, biologgers on animals, camera traps, or audio recorders. While there are consequences to using new technologies to detect and identify species, the use of these new technologies does not necessitate a disconnect between humans and nature and between field intuition and data analyses. Although implementing next-generation natural history takes advantage of technological advancements, it continues to require researchers to intentionally spend time in nature to deploy devices through which observations can accrue and intuition can develop. We argue that these technologies allow early engagement of students to see, hear, or otherwise detect animals in the wild and inspire engagement with nature.
Next-generation natural history also allows ecologists to bring the experience of nature to humans without having to invest in long field seasons. Data collectors can share photographs of rare and cryptic animals, sounds of the forest, and movement of animals without observation bias. With the use of the internet, these experiences can be shared across the globe with millions of people, which allows ecology to be more inclusive while having minimal impact on wild places. No longer is a 4-year botany degree needed; kids can at least play with apps like iNaturalist and have their observations auto-identified through computer algorithms and verified by other observers. While these virtual experiences are valuable for science communication and engagement, they are a complement but not a substitute for directly connecting with nature. This inclusivity allows people with disabilities or those in an increasingly urbanized world to gain new avenues for the appreciation and understanding of nature. Those without an entry point for hiking and camping (e.g., people from urban environments), international travel experience, money, or freedom from family obligations to spend months away from home can contribute to research and develop a connection with the flora, fauna, and places that are otherwise inaccessible. Even non-experts can help collect data for analyses by placing devices on their property or by tagging photos and sounds (e.g., via Zooniverse). By including these traditionally excluded groups, next-generation natural history can diversify, increase its stakeholders, and influence nature conservation policy.
We argue that an ongoing development of next-generation technologies for natural history is deepening our connections with nature instead of eroding them. As Wilmers et al. (2015) state in their review of biologging, “Ecologists often lament that increased use of technology is removing ecologists from the field and so causing a general decline in natural history skills. Our experience, however, has been the opposite. The more multidimensional and fine-scaled animal sensors become, the more time and effort is required by biologists in the field to collect the environmental covariates that are used to predict sensor output.” In our experience, next-generation natural history projects require a different approach to fieldwork. Although initially researchers may spend less time in each location, the benefits of next-generation natural history research facilitate robust landscape-scale studies that continue to require extensive time spent in the field. The devices deployed and samples collected allow us to multiply our sampling locations while increasing the duration of sampling time, thereby increasing our sampling effort and area of inference. These new technologies allow us to process samples quickly and efficiently, sometimes even in real time. What has changed in natural history is that in addition to extensive collection of field data, ecologists now implement advanced statistical, computational, and molecular methods when returning from the field to answer the question at hand. We argue that the result is a deeper understanding of nature that can build a greater appreciation for nature and motivate its conservation.
MT, ED, CA, JU, AM, JR, CE, JD, and TL conceptualized the manuscript and performed the literature search. MT, ED, and TL produced figures. MT, ED, CA, JU, AM, JR, CE, JD, DL, MB, CP, and TL wrote sections of the manuscript. MT and TL led synthesis of written sections. All authors were significant contributors to content and revisions of the manuscript.
Support for this project was provided by the Oregon State University, the USDA Forest Service, the Oregon Department of Fisheries and Wildlife, the Pittman-Robertson Wildlife Restoration Act, and the National Science Foundation H. J. Andrews Long-Term Ecological Research grant (NSF # DEB-0823380).
The authors declare that the research was conducted in the absence of any commercial or financial relationships that could be construed as a potential conflict of interest.
We thank D. Yu, S. Sparrow, E. Dymit, K. Loonam, and reviewers for their thoughtful comments and constructive feedback.
Abrams, J. F., Hörig, L. A., Brozovic, R., Axtner, J., Crampton-Platt, A., Mohamed, A., et al. (2019). Shifting up a gear with iDNA: from mammal detection events to standardised surveys. J. Appl. Ecol. 56, 1637–1648. doi: 10.1111/1365-2664.13411
Adler, F. R., Green, A. M., and Şekercioğlu, ÇH. (2020). Citizen science in ecology: a place for humans in nature. Ann. N. Y. Acad. Sci. doi: 10.1111/nyas.14340 [Epub ahead of print].
Amato, K. R., Yeoman, C. J., Kent, A., Righini, N., Carbonero, F., Estrada, A., et al. (2013). Habitat degradation impacts black howler monkey (Alouatta pigra) gastrointestinal microbiomes. ISME J. 7, 1344–1353. doi: 10.1038/ismej.2013.16
Ando, N., Shimoyama, I., and Kanzaki, R. (2002). A dual-channel FM transmitter for acquisition of flight muscle activities from the freely flying hawkmoth, Agrius convolvuli. J. Neurosci. Methods 115, 181–187. doi: 10.1016/S0165-0270(02)00013-4
Asner, G. P., Martin, R. E., Anderson, C. B., and Knapp, D. E. (2015). Quantifying forest canopy traits: imaging spectroscopy versus field survey. Remote Sens. Environ. 158, 15–27. doi: 10.1016/j.rse.2014.11.011
Augustine, B. C., Royle, J. A., Murphy, S. M., Chandler, R. B., Cox, J. J., and Kelly, M. J. (2019). Spatial capture-recapture for categorically marked populations with an application to genetic capture-recapture. Ecosphere 10:e02627. doi: 10.1002/ecs2.2627
Avery, M. I., and Haines-Young, R. H. (1990). Population estimates for the dunlin Calidris alpina derived from remotely sensed satellite imagery of the Flow Country of northern Scotland. Nature 344, 860–862. doi: 10.1038/344860a0
Avgar, T., Potts, J. R., Lewis, M. A., and Boyce, M. S. (2016). Integrated step selection analysis: bridging the gap between resource selection and animal movement. Methods Ecol. Evol. 7, 619–630. doi: 10.1111/2041-210X.12528
Bacon, M. M., Becic, G. M., Epp, M. T., and Boyce, M. S. (2011). Do GPS clusters really work? Carnivore diet from scat analysis and GPS telemetry methods. Wildl. Soc. Bull. 35, 409–415. doi: 10.1002/wsb.85
Bailey, L. L., Reid, J. A., Forsman, E. D., and Nichols, J. D. (2009). Modeling co-occurrence of northern spotted and barred owls: accounting for detection probability differences. Biol. Conserv. 142, 2983–2989. doi: 10.1016/j.biocon.2009.07.028
Barrows, C. W., Murphy-Mariscal, M. L., and Hernandez, R. R. (2016). At a crossroads: the nature of natural history in the twenty-first century. BioScience 66, 592–599. doi: 10.1093/biosci/biw043
Basille, M., Fortin, D., Dussault, C., Bastille-Rousseau, G., Ouellet, J.-P., and Courtois, R. (2015). Plastic response of fearful prey to the spatiotemporal dynamics of predator distribution. Ecology 96, 2622–2631. doi: 10.1890/14-1706.1
Beck, S., Berner, A. M., Bignell, G., Bond, M., Callanan, M. J., Chervova, O., et al. (2018). Personal Genome Project UK (PGP-UK): a research and citizen science hybrid project in support of personalized medicine. BMC Med. Genomics 11:108. doi: 10.1186/s12920-018-0423-1
Berry, T. E., Osterrieder, S. K., Murray, D. C., Coghlan, M. L., Richardson, A. J., Grealy, A. K., et al. (2017). DNA metabarcoding for diet analysis and biodiversity: a case study using the endangered Australian sea lion (Neophoca cinerea). Ecol. Evol. 7, 5435–5453. doi: 10.1002/ece3.3123
Betke, M., Hirsh, D. E., Makris, N. C., McCracken, G. F., Procopio, M., Hristov, N. I., et al. (2008). Thermal imaging reveals significantly smaller brazilian free-tailed bat colonies than previously estimated. J. Mammal. 89, 18–24. doi: 10.1644/07-MAMM-A-011.1
Betts, M. G., Hadley, A. S., Frey, D. W., Frey, S. J. K., Gannon, D., Harris, S. H., et al. (2021). When are hypotheses useful in ecology and evolution? Ecol. Evol. doi: 10.1002/ece3.7365 [Epub ahead of print].
Bohmann, K., Evans, A., Gilbert, M. T. P., Carvalho, G. R., Creer, S., Knapp, M., et al. (2014). Environmental DNA for wildlife biology and biodiversity monitoring. Trends Ecol. Evol. 29, 358–367. doi: 10.1016/j.tree.2014.04.003
Bohmann, K., Gopalakrishnan, S., Nielsen, M., Nielsen, L., dos, S. B., Jones, G., et al. (2018). Using DNA metabarcoding for simultaneous inference of common vampire bat diet and population structure. Mol. Ecol. Resour. 18, 1050–1063. doi: 10.1111/1755-0998.12891
Bohmann, K., Monadjem, A., Lehmkuhl Noer, C., Rasmussen, M., Zeale, M. R. K., Clare, E., et al. (2011). Molecular diet analysis of two african free-tailed bats (molossidae) using high throughput sequencing. PLoS One 6:e21441. doi: 10.1371/journal.pone.0021441
Bonney, R., Cooper, C. B., Dickinson, J., Kelling, S., Phillips, T., Rosenberg, K. V., et al. (2009). Citizen science: a developing tool for expanding science knowledge and scientific literacy. BioScience 59, 977–984. doi: 10.1525/bio.2009.59.11.9
Börger, L., Bijleveld, A. I., Fayet, A. L., Machovsky-Capuska, G. E., Patrick, S. C., Street, G. M., et al. (2020). Biologging special feature. J. Anim. Ecol. 89, 6–15. doi: 10.1111/1365-2656.13163
Borowicz, A., Le, H., Humphries, G., Nehls, G., Höschle, C., Kosarev, V., et al. (2019). Aerial-trained deep learning networks for surveying cetaceans from satellite imagery. PLoS One 14:e0212532. doi: 10.1371/journal.pone.0212532
Boyd, D. S., and Danson, F. M. (2005). Satellite remote sensing of forest resources: three decades of research development. Prog. Phys. Geogr. Earth Environ. 29, 1–26. doi: 10.1191/0309133305pp432ra
Boyle, W. A., and Sigel, B. J. (2015). Ongoing changes in the avifauna of La Selva biological station, costa rica: twenty-three years of christmas bird counts. Spec. Issue Ecol. Conserv. Avian Insectivores Rainfor. Understory Pan Trop. Perspect. 188, 11–21. doi: 10.1016/j.biocon.2015.01.004
Breed, G. A., Stichter, S., and Crone, E. E. (2013). Climate-driven changes in northeastern US butterfly communities. Nat. Clim. Change 3, 142–145. doi: 10.1038/nclimate1663
Brockman, C. J., Collins, W. B., Welker, J. M., Spalinger, D. E., and Dale, B. W. (2017). Determining kill rates of ungulate calves by brown bears using neck-mounted cameras. Wildl. Soc. Bull. 41, 88–97. doi: 10.1002/wsb.733
Brodrick, P. G., Davies, A. B., and Asner, G. P. (2019). Uncovering ecological patterns with convolutional neural networks. Trends Ecol. Evol. 34, 734–745. doi: 10.1016/j.tree.2019.03.006
Bruno, J. F., Selig, E. R., Casey, K. S., Page, C. A., Willis, B. L., Harvell, C. D., et al. (2007). Thermal stress and coral cover as drivers of Coral Disease Outbreaks. PLoS Biol. 5:e124. doi: 10.1371/journal.pbio.0050124
Burton, A. C., Neilson, E., Moreira, D., Ladle, A., Steenweg, R., Fisher, J. T., et al. (2015). Wildlife camera trapping: a review and recommendations for linking surveys to ecological processes. J. Appl. Ecol. 52, 675–685. doi: 10.1111/1365-2664.12432
Bury, R. B. (2006). Natural history, field ecology, conservation biology and wildlife management: time to connect the dots. Herpetol. Conserv. Biol. 1, 56–61.
Bush, A., Sollmann, R., Wilting, A., Bohmann, K., Cole, B., Balzter, H., et al. (2017). Connecting Earth observation to high-throughput biodiversity data. Nat. Ecol. Amp Evol. 1:0176.
Butcher, G. S., Fuller, M. R., McAllister, L. S., and Geissler, P. H. (1990). An evaluation of the christmas bird count for monitoring population trends of selected Species. Wildl. Soc. Bull. 18, 129–134.
Buxton, R. T., McKenna, M. F., Mennitt, D., Fristrup, K., Crooks, K., Angeloni, L., et al. (2017). Noise pollution is pervasive in U.S. protected areas. Science 356:531. doi: 10.1126/science.aah4783
Cagnacci, F., Boitani, L., Powell, R. A., and Boyce, M. S. (2010). Preface. Philos. Trans. R. Soc. B Biol. Sci. 365, 2155–2155. doi: 10.1098/rstb.2010.0098
Calvignac-Spencer, S., Merkel, K., Kutzner, N., Kühl, H., Boesch, C., Kappeler, P. M., et al. (2013). Carrion fly-derived DNA as a tool for comprehensive and cost-effective assessment of mammalian biodiversity. Mol. Ecol. 22, 915–924. doi: 10.1111/mec.12183
Castañeda, C., Herrero, J., and Casterad, M. A. (2005). Facies identification within the playa-lakes of the Monegros desert, Spain, from field and satellite data. CATENA 63, 39–63. doi: 10.1016/j.catena.2005.05.011
Cavalieri, D. J., Parkinson, C. L., and Vinnikov, K. Y. (2003). 30-Year satellite record reveals contrasting Arctic and Antarctic decadal sea ice variability. Geophys. Res. Lett. 30:e018031. doi: 10.1029/2003GL018031
Chai, L., Dong, Z., Chen, A., and Wang, H. (2018). Changes in intestinal microbiota of Bufo gargarizans and its association with body weight during metamorphosis. Arch. Microbiol. 200, 1087–1099. doi: 10.1007/s00203-018-1523-1
Chandler, M., See, L., Copas, K., Bonde, A. M. Z., López, B. C., Danielsen, F., et al. (2017). Contribution of citizen science towards international biodiversity monitoring. SIMeasures Biodivers. 213, 280–294. doi: 10.1016/j.biocon.2016.09.004
Chandler, R. B., and Royle, J. A. (2013). Spatially explicit models for inference about density in unmarked or partially marked populations. Ann. Appl. Stat. 7, 936–954. doi: 10.1214/12-AOAS610
Colwell, R. R. (1996). Global climate and infectious disease: the cholera paradigm. Science 274:2025. doi: 10.1126/science.274.5295.2025
Cooke, S. J., Hinch, S. G., Wikelski, M., Andrews, R. D., Kuchel, L. J., Wolcott, T. G., et al. (2004). Biotelemetry: a mechanistic approach to ecology. Trends Ecol. Evol. 19, 334–343. doi: 10.1016/j.tree.2004.04.003
Cortez, J. D., Henke, S. E., Redeker, E., Fulbright, T. E., Riddle, R., and Young, J. (2013). Demonstration of ground-penetrating radar as a useful tool for assessing pocket gopher burrows. Wildl. Soc. Bull. 37, 428–432. doi: 10.1002/wsb.279
Creer, S., Deiner, K., Frey, S., Porazinska, D., Taberlet, P., Thomas, W. K., et al. (2016). The ecologist’s field guide to sequence-based identification of biodiversity. Methods Ecol. Evol. 7, 1008–1018. doi: 10.1111/2041-210X.12574
Cutter, A. D. (2013). Integrating phylogenetics, phylogeography and population genetics through genomes and evolutionary theory. Mol. Phylogenet. Evol. 69, 1172–1185. doi: 10.1016/j.ympev.2013.06.006
Dandois, J. P., and Ellis, E. C. (2013). High spatial resolution three-dimensional mapping of vegetation spectral dynamics using computer vision. Remote Sens. Environ. 136, 259–276. doi: 10.1016/j.rse.2013.04.005
De Wulf, R. R., Goossens, R. E., MacKinnon, J. R., and Cai, W. S. (1988). Remote sensing for wildlife management: giant panda habitat mapping from LANDSAT MSS images. Geocarto Int. 3, 41–50. doi: 10.1080/10106048809354132
Deiner, K., Bik, H. M., Mächler, E., Seymour, M., Lacoursière-Roussel, A., Altermatt, F., et al. (2017). Environmental DNA metabarcoding: transforming how we survey animal and plant communities. Mol. Ecol. 26, 5872–5895. doi: 10.1111/mec.14350
Dejean, T., Valentini, A., Miquel, C., Taberlet, P., Bellemain, E., and Miaud, C. (2012). Improved detection of an alien invasive species through environmental DNA barcoding: the example of the American bullfrog Lithobates catesbeianus. J. Appl. Ecol. 49, 953–959. doi: 10.1111/j.1365-2664.2012.02171.x
Delsuc, F., Brinkmann, H., and Philippe, H. (2005). Phylogenomics and the reconstruction of the tree of life. Nat. Rev. Genet. 6, 361–375. doi: 10.1038/nrg1603
Dickinson, J. L., Shirk, J., Bonter, D., Bonney, R., Crain, R. L., Martin, J., et al. (2012). The current state of citizen science as a tool for ecological research and public engagement. Front. Ecol. Environ. 10, 291–297. doi: 10.1890/110236
Dickinson, J. L., Zuckerberg, B., and Bonter, D. N. (2010). Citizen science as an ecological research tool: challenges and benefits. Annu. Rev. Ecol. Evol. Syst. 41, 149–172. doi: 10.1146/annurev-ecolsys-102209-144636
Diete, R. L., Meek, P. D., Dickman, C. R., and Leung, L. K.-P. (2014). Burrowing behaviour of the northern hopping-mouse (Notomys aquilo): field observations. Aust. Mammal. 36, 242–246.
Disney, R. H. L. (1968). The terms ecology and natural history. J. Biol. Educ. 2, 235–237. doi: 10.1080/00219266.1968.9653538
Dolliver, J. E. (2019). Using Satellite Imagery to Count Nesting Albatross From Space. Corvallis, OR: Oregon State University.
Drummond, A. J., Newcomb, R. D., Buckley, T. R., Xie, D., Dopheide, A., Potter, B. C., et al. (2015). Evaluating a multigene environmental DNA approach for biodiversity assessment. GigaScience 4:46. doi: 10.1186/s13742-015-0086-1
Duarte, A., Adams, M. J., and Peterson, J. T. (2018). Fitting N-mixture models to count data with unmodeled heterogeneity: bias, diagnostics, and alternative approaches. Ecol. Model. 374, 51–59. doi: 10.1016/j.ecolmodel.2018.02.007
Duchac, L. S., Lesmeister, D. B., Dugger, K. M., Ruff, Z. J., and Davis, R. J. (2020). Passive acoustic monitoring effectively detects Northern Spotted Owls and Barred Owls over a range of forest conditions. Condor 122:duaa017. doi: 10.1093/condor/duaa017
Dunn, C. W., Hejnol, A., Matus, D. Q., Pang, K., Browne, W. E., Smith, S. A., et al. (2008). Broad phylogenomic sampling improves resolution of the animal tree of life. Nature 452, 745–749. doi: 10.1038/nature06614
Egevang, C., Stenhouse, I. J., Phillips, R. A., Petersen, A., Fox, J. W., and Silk, J. R. D. (2010). Tracking of Arctic terns Sterna paradisaea reveals longest animal migration. Proc. Natl. Acad. Sci. U.S.A. 107:2078. doi: 10.1073/pnas.0909493107
Eriksson, C. E., Moriarty, K. M., Linnell, M. A., and Levi, T. (2019). Biotic factors influencing the unexpected distribution of a Humboldt marten (Martes caurina humboldtensis) population in a young coastal forest. PLoS One 14:e0214653. doi: 10.1371/journal.pone.0214653
Eriksson, C. E., Ruprecht, J., and Levi, T. (2020). More affordable and effective noninvasive single nucleotide polymorphism genotyping using high-throughput amplicon sequencing. Mol. Ecol. Resour. 20, 1505–1516. doi: 10.1111/1755-0998.13208
Faria, N. R., Sabino, E. C., Nunes, M. R. T., Alcantara, L. C. J., Loman, N. J., and Pybus, O. G. (2016). Mobile real-time surveillance of Zika virus in Brazil. Genome Med. 8:97. doi: 10.1186/s13073-016-0356-2
Ficetola, G. F., Miaud, C., Pompanon, F., and Taberlet, P. (2008). Species detection using environmental DNA from water samples. Biol. Lett. 4, 423–425. doi: 10.1098/rsbl.2008.0118
Fleischner, T. L., Espinoza, R. E., Gerrish, G. A., Greene, H. W., Kimmerer, R. W., Lacey, E. A., et al. (2017). Teaching biology in the field: importance, challenges, and solutions. BioScience 67, 558–567. doi: 10.1093/biosci/bix036
Forsman, E. D., Meslow, E. C., and Wight, H. M. (1984). Distribution and biology of the spotted owl in Oregon. Wildl. Monogr. 12, 3–64.
Franke, A., Caelli, T., and Hudson, R. J. (2004). Analysis of movements and behavior of caribou (Rangifer tarandus) using hidden Markov models. Ecol. Model. 173, 259–270. doi: 10.1016/j.ecolmodel.2003.06.004
Fretwell, P. T., Phillips, R. A., Brooke, M., de, L., Fleming, A. H., and McArthur, A. (2015). Using the unique spectral signature of guano to identify unknown seabird colonies. Remote Sens. Environ. 156, 448–456. doi: 10.1016/j.rse.2014.10.011
Frick, W. (2013). Acoustic monitoring of bats, considerations of options for long-term monitoring. Therya 4, 69–78. doi: 10.12933/therya-13-109
Friedlaender, A. S., Bowers, M. T., Cade, D., Hazen, E. L., Stimpert, A. K., Allen, A. N., et al. (2020). The advantages of diving deep: fin whales quadruple their energy intake when targeting deep krill patches. Funct. Ecol. 34, 497–506. doi: 10.1111/1365-2435.13471
Furnas, B. J., and Callas, R. L. (2015). Using automated recorders and occupancy models to monitor common forest birds across a large geographic region. J. Wildl. Manag. 79, 325–337. doi: 10.1002/jwmg.821
Galan, M., Pons, J.-B., Tournayre, O., Pierre, É, Leuchtmann, M., Pontier, D., et al. (2018). Metabarcoding for the parallel identification of several hundred predators and their prey: application to bat species diet analysis. Mol. Ecol. Resour. 18, 474–489. doi: 10.1111/1755-0998.12749
Getzin, S., Wiegand, K., and Schöning, I. (2012). Assessing biodiversity in forests using very high-resolution images and unmanned aerial vehicles. Methods Ecol. Evol. 3, 397–404. doi: 10.1111/j.2041-210X.2011.00158.x
Giribet, G. (2015). Morphology should not be forgotten in the era of genomics-a phylogenetic perspective. Spec. Issue Proc. Int. Congr. Invertebr. Morphol. 256, 96–103. doi: 10.1016/j.jcz.2015.01.003
Goldbogen, J. A., Cade, D. E., Calambokidis, J., Friedlaender, A. S., Potvin, J., Segre, P. S., et al. (2017). How baleen whales feed: the biomechanics of engulfment and filtration. Annu. Rev. Mar. Sci. 9, 367–386. doi: 10.1146/annurev-marine-122414-033905
Goldbogen, J. A., Friedlaender, A. S., Calambokidis, J., McKenna, M. F., Simon, M., and Nowacek, D. P. (2013). Integrative approaches to the study of baleen whale diving behavior, feeding performance, and foraging ecology. BioScience 63, 90–100. doi: 10.1525/bio.2013.63.2.5
Gomes, V. H. F., Vieira, I. C. G., Salomão, R. P., and ter Steege, H. (2019). Amazonian tree species threatened by deforestation and climate change. Nat. Clim. Change 9, 547–553. doi: 10.1038/s41558-019-0500-2
Goordial, J., Altshuler, I., Hindson, K., Chan-Yam, K., Marcolefas, E., and Whyte, L. G. (2017). In situ field sequencing and life detection in remote (79°26′N) Canadian high arctic permafrost ice wedge microbial communities. Front. Microbiol. 8:2594. doi: 10.3389/fmicb.2017.02594
Graham, M. H. (1997). Factors determining the upper limit of giant kelp, Macrocystis pyrifera Agardh, along the Monterey Peninsula, central California, USA. J. Exp. Mar. Biol. Ecol. 218, 127–149. doi: 10.1016/S0022-0981(97)00072-5
Green, A. M., Chynoweth, M. W., and Şekercioğlu, ÇH. (2020). Spatially explicit capture-recapture through camera trapping: a review of benchmark analyses for wildlife density estimation. Front. Ecol. Evol. 8:473. doi: 10.3389/fevo.2020.563477
Guazzo, R. A., Weller, D. W., Europe, H. M., Durban, J. W., D’Spain, G. L., and Hildebrand, J. A. (2019). Migrating eastern North Pacific gray whale call and blow rates estimated from acoustic recordings, infrared camera video, and visual sightings. Sci. Rep. 9:12617. doi: 10.1038/s41598-019-49115-y
Guillerault, N., Bouletreau, S., Iribar, A., Valentini, A., and Santoul, F. (2017). Application of DNA metabarcoding on faeces to identify European catfish Silurus glanis diet. J. Fish Biol. 90, 2214–2219. doi: 10.1111/jfb.13294
Harper, G. J., Steininger, M. K., Tucker, C. J., Juhn, D., and Hawkins, F. (2007). Fifty years of deforestation and forest fragmentation in Madagascar. Environ. Conserv. 34, 325–333. doi: 10.1017/S0376892907004262
Harrer, L. E. F., and Levi, T. (2018). The primacy of bears as seed dispersers in salmon-bearing ecosystems. Ecosphere 9:e02076. doi: 10.1002/ecs2.2076
Harris, R. N., Brucker, R. M., Walke, J. B., Becker, M. H., Schwantes, C. R., Flaherty, D. C., et al. (2009). Skin microbes on frogs prevent morbidity and mortality caused by a lethal skin fungus. ISME J. 3, 818–824. doi: 10.1038/ismej.2009.27
Hatch, L. T., Clark, C. W., Van Parijs, S. M., Frankel, A. S., and Ponirakis, D. W. (2012). Quantifying loss of acoustic communication space for right whales in and around a U.S. National Marine Sanctuary. Conserv. Biol. 26, 983–994. doi: 10.1111/j.1523-1739.2012.01908.x
Heather, J. M., and Chain, B. (2016). The sequence of sequencers: the history of sequencing DNA. Genomics 107, 1–8. doi: 10.1016/j.ygeno.2015.11.003
Hebblewhite, M., and Haydon, D. T. (2010). Distinguishing technology from biology: a critical review of the use of GPS telemetry data in ecology. Philos. Trans. R. Soc. B Biol. Sci. 365, 2303–2312. doi: 10.1098/rstb.2010.0087
Hecker, S., Haklay, M., Bowser, A., Makuch, Z., and Vogel, J. (2018). Citizen Science: Innovation in Open Science, Society and Policy. London: UCL Press.
Hiby, L., and Lovell, P. (1990). Computer aided matching of natural markings: a prototype system for grey seals. Rep. Int. Whal. Comm. 12, 57–61.
Hodgson, A., Kelly, N., and Peel, D. (2013). Unmanned Aerial Vehicles (UAVs) for surveying marine fauna: a dugong case study. PLoS One 8:e79556. doi: 10.1371/journal.pone.0079556
Hodgson, J. C., Baylis, S. M., Mott, R., Herrod, A., and Clarke, R. H. (2016). Precision wildlife monitoring using unmanned aerial vehicles. Sci. Rep. 6:22574. doi: 10.1038/srep22574
Hodgson, J. C., Mott, R., Baylis, S. M., Pham, T. T., Wotherspoon, S., Kilpatrick, A. D., et al. (2018). Drones count wildlife more accurately and precisely than humans. Methods Ecol. Evol. 9, 1160–1167. doi: 10.1111/2041-210X.12974
Hoffman, S. W., and Smith, J. P. (2003). Population trends of migratory raptors in Western North America, 1977-2001. Condor 105, 397–419. doi: 10.1093/condor/105.3.397
Hofmeester, T. R., Cromsigt, J. P. G. M., Odden, J., Andrén, H., Kindberg, J., and Linnell, J. D. C. (2019). Framing pictures: a conceptual framework to identify and correct for biases in detection probability of camera traps enabling multi-species comparison. Ecol. Evol. 9, 2320–2336. doi: 10.1002/ece3.4878
Hopken, M. W., Orning, E. K., Young, J. K., and Piaggio, A. J. (2016). Molecular forensics in avian conservation: a DNA-based approach for identifying mammalian predators of ground-nesting birds and eggs. BMC Res. Notes 9:14. doi: 10.1186/s13104-015-1797-1
Hubel, T. Y., Myatt, J. P., Jordan, N. R., Dewhirst, O. P., McNutt, J. W., and Wilson, A. M. (2016). Additive opportunistic capture explains group hunting benefits in African wild dogs. Nat. Commun. 7:11033. doi: 10.1038/ncomms11033
Hudson, M.-A. R., Francis, C. M., Campbell, K. J., Downes, C. M., Smith, A. C., and Pardieck, K. L. (2017). The role of the North American Breeding Bird Survey in conservation. Condor 119, 526–545. doi: 10.1650/CONDOR-17-62.1
Hug, L. A., Baker, B. J., Anantharaman, K., Brown, C. T., Probst, A. J., Castelle, C. J., et al. (2016). A new view of the tree of life. Nat. Microbiol. 1:16048. doi: 10.1038/nmicrobiol.2016.48
Iwanowicz, D. D., Vandergast, A. G., Cornman, R. S., Adams, C. R., Kohn, J. R., Fisher, R. N., et al. (2016). Metabarcoding of fecal samples to determine herbivore diets: a case study of the endangered pacific pocket mouse. PLoS One 11:e0165366. doi: 10.1371/journal.pone.0165366
Jakubavièiûtë, E., Bergström, U., Eklöf, J. S., Haenel, Q., and Bourlat, S. J. (2017). DNA metabarcoding reveals diverse diet of the three-spined stickleback in a coastal ecosystem. PLoS One 12:e0186929. doi: 10.1371/journal.pone.0186929
Jarvis, A., Williams, K., Williams, D., Guarino, L., Caballero, P. J., and Mottram, G. (2005). Use of GIS for optimizing a collecting mission for a rare wild pepper (Capsicum flexuosum Sendtn.) in Paraguay. Genet. Resour. Crop Evol. 52, 671–682. doi: 10.1007/s10722-003-6020-x
Jedlicka, J. A., Sharma, A. M., and Almeida, R. P. P. (2013). Molecular tools reveal diets of insectivorous birds from predator fecal matter. Conserv. Genet. Resour. 5, 879–885. doi: 10.1007/s12686-013-9900-1
Jerde, C. L., Chadderton, W. L., Mahon, A. R., Renshaw, M. A., Corush, J., Budny, M. L., et al. (2013). Detection of Asian carp DNA as part of a Great Lakes basin-wide surveillance program. Can. J. Fish. Aquat. Sci. 70, 522–526. doi: 10.1139/cjfas-2012-0478
Johansson, Ö, McCarthy, T., Samelius, G., Andrén, H., Tumursukh, L., and Mishra, C. (2015). Snow leopard predation in a livestock dominated landscape in Mongolia. Biol. Conserv. 184, 251–258. doi: 10.1016/j.biocon.2015.02.003
Jones, G. P., Pearlstine, L. G., and Percival, H. F. (2006). An assessment of small unmanned aerial vehicles for wildlife research. Wildl. Soc. Bull. 34, 750–758.
Joo, R., Boone, M. E., Clay, T. A., Patrick, S. C., Clusella-Trullas, S., and Basille, M. (2020). Navigating through the r packages for movement. J. Anim. Ecol. 89, 248–267. doi: 10.1111/1365-2656.13116
Kamenova, S., Bartley, T. J., Bohan, D. A., Boutain, J. R., Colautti, R. I., Domaizon, I., et al. (2017). “Chapter three - invasions toolkit: current methods for tracking the spread and impact of invasive species,” in Advances in Ecological Research, eds D. A. Bohan, A. J. Dumbrell, and F. Massol (Boston: Academic Press), 85–182.
Kartzinel, T. R., Chen, P. A., Coverdale, T. C., Erickson, D. L., Kress, W. J., Kuzmina, M. L., et al. (2015). DNA metabarcoding illuminates dietary niche partitioning by African large herbivores. Proc. Natl. Acad. Sci. U.S.A. 112:8019. doi: 10.1073/pnas.1503283112
Kaunisto, K. M., Roslin, T., Sääksjärvi, I. E., and Vesterinen, E. J. (2017). Pellets of proof: first glimpse of the dietary composition of adult odonates as revealed by metabarcoding of feces. Ecol. Evol. 7, 8588–8598. doi: 10.1002/ece3.3404
Kellenberger, R., Kneubühler, M., and Kellenberger, T. (2009). “Spectral characterisation and mapping of Welwitschia mirabilis in Namibia,” in Proceedings of the 2009 IEEE International Geoscience and Remote Sensing Symposium, Cape Town, 354–362.
Kirchman, J. J., and Van Keuren, A. E. (2017). Altitudinal Range Shifts of Birds At the Southern Periphery of the Boreal Forest: 40 Years of change in the Adirondack Mountains. Wilson J. Ornithol. 129, 742–753. doi: 10.1676/16-164.1
Kocher, A., de Thoisy, B., Catzeflis, F., Valière, S., Bañuls, A.-L., and Murienne, J. (2017). iDNA screening: disease vectors as vertebrate samplers. Mol. Ecol. 26, 6478–6486. doi: 10.1111/mec.14362
Kraaijeveld, K., de Weger, L. A., Ventayol García, M., Buermans, H., Frank, J., Hiemstra, P. S., et al. (2015). Efficient and sensitive identification and quantification of airborne pollen using next-generation DNA sequencing. Mol. Ecol. Resour. 15, 8–16. doi: 10.1111/1755-0998.12288
Kueneman, J. G., Parfrey, L. W., Woodhams, D. C., Archer, H. M., Knight, R., and McKenzie, V. J. (2014). The amphibian skin-associated microbiome across species, space and life history stages. Mol. Ecol. 23, 1238–1250. doi: 10.1111/mec.12510
Kutsch, W. (2002). Transmission of muscle potentials during free flight of locusts. Comput. Electron. Agric. 35, 181–199. doi: 10.1016/s0168-1699(02)00018-2
La Sorte, F. A., and Thompson, F. R. I. I. I. (2007). Poleward shifts in winter ranges of north american birds. Ecology 88, 1803–1812. doi: 10.1890/06-1072.1
Laiolo, P. (2010). The emerging significance of bioacoustics in animal species conservation. Conserv. Plan. Emerg. Glob. Clim. Econ. Realities 143, 1635–1645. doi: 10.1016/j.biocon.2010.03.025
Laliberte, A. S., and Ripple, W. J. (2003). Automated wildlife counts from remotely sensed imagery. Wildl. Soc. Bull. 31, 362–371.
Larson, E. R., Renshaw, M. A., Gantz, C. A., Umek, J., Chandra, S., Lodge, D. M., et al. (2017). Environmental DNA (eDNA) detects the invasive crayfishes Orconectes rusticus and Pacifastacus leniusculus in large lakes of North America. Hydrobiologia 800, 173–185. doi: 10.1007/s10750-017-3210-7
Leaché, A. D., Fujita, M. K., Minin, V. N., and Bouckaert, R. R. (2014). Species delimitation using genome-wide SNP data. Syst. Biol. 63, 534–542. doi: 10.1093/sysbio/syu018
Leray, M., and Knowlton, N. (2015). DNA barcoding and metabarcoding of standardized samples reveal patterns of marine benthic diversity. Proc. Natl. Acad. Sci. U.S.A. 112:2076. doi: 10.1073/pnas.1424997112
Lesmeister, D. B., Appel, C. L., Davis, R. J., Yackulic, C. B., and Ruff, Z. J. (2021). Simulating the Effort Necessary to Detect Changes in Northern Spotted Owl (Strix Occidentalis Caurina) Populations Using Passive Acoustic Monitoring. Portland: U.S. Department of Agriculture, Forest Service, Pacific Northwest Research Station. p.55.
Lesmeister, D. B., Nielsen, C. K., Schauber, E. M., and Hellgren, E. C. (2015). Spatial and temporal structure of a mesocarnivore guild in midwestern north America. Wildl. Monogr. 191, 1–61. doi: 10.1002/wmon.1015
Levi, T., Allen, J. M., Bell, D., Joyce, J., Russell, J. R., Tallmon, D. A., et al. (2019). Environmental DNA for the enumeration and management of Pacific salmon. Mol. Ecol. Resour. Resour. 19, 597–608. doi: 10.1111/1755-0998.12987
Li, E., Parker, S. S., Pauly, G. B., Randall, J. M., Brown, B. V., and Cohen, B. S. (2019). An urban biodiversity assessment framework that combines an urban habitat classification scheme and citizen science data. Front. Ecol. Evol. 7:277. doi: 10.3389/fevo.2019.00277
Linnell, M. A., and Lesmeister, D. B. (2020). Predator-prey interactions in the canopy. Ecol. Evol. 10, 8610–8622. doi: 10.1002/ece3.6518
Linnell, M. A., Moriarty, K., Green, D. S., and Levi, T. (2018). Density and population viability of coastal marten: a rare and geographically isolated small carnivore. PeerJ 6:e4530. doi: 10.7717/peerj.4530
Liu, D., Kelly, M., and Gong, P. (2006). A spatial-temporal approach to monitoring forest disease spread using multi-temporal high spatial resolution imagery. Remote Sens. Environ. 101, 167–180. doi: 10.1016/j.rse.2005.12.012
Llusia, D., Márquez, R., Beltrán, J. F., Benítez, M., and do Amaral, J. P. (2013). Calling behaviour under climate change: geographical and seasonal variation of calling temperatures in ectotherms. Glob. Change Biol. 19, 2655–2674. doi: 10.1111/gcb.12267
Lynch, H. J., and LaRue, M. A. (2014). First global census of the Adélie Penguin. Auk 131, 457–466. doi: 10.1642/AUK-14-31.1
MacKenzie, D. I., Nichols, J. D., Royle, J. A., Pollock, K. H., Bailey, L. L., and Hines, J. E. (eds) (2018). Occupancy Estimation and Modeling: Inferring Patterns and Dynamics of Species Occurence, 2nd Edn. Boston: Academic Press.
Maffei, L., Noss, A. J., Cuéllar, E., and Rumiz, D. I. (2005). Ocelot (Felis pardalis) population densities, activity, and ranging behaviour in the dry forests of eastern Bolivia: data from camera trapping. J. Trop. Ecol. 21, 349–353. doi: 10.1017/S0266467405002397
Magurran, A. E., Baillie, S. R., Buckland, S. T., Dick, J. M. C. P., Elston, D. A., Scott, E. M., et al. (2010). Long-term datasets in biodiversity research and monitoring: assessing change in ecological communities through time. Spec. Issue Long Term Ecol. Res. 25, 574–582. doi: 10.1016/j.tree.2010.06.016
Marselis, S. M., Abernethy, K., Alonso, A., Armston, J., Baker, T. R., Bastin, J.-F., et al. (2020). Evaluating the potential of full-waveform lidar for mapping pan-tropical tree species richness. Glob. Ecol. Biogeogr. 29, 1799–1816. doi: 10.1111/geb.13158
McCallum, M., and McCallum, J. (2006). Publication trends of natural history and field studies in herpetology. Herpetol. Conserv. Biol. 1, 62–67.
McDowall, P., and Lynch, H. J. (2017). Ultra-fine scale spatially-integrated mapping of habitat and occupancy using structure-from-motion. PLoS One 12:e0166773. doi: 10.1371/journal.pone.0166773
McKinley, D. C., Miller-Rushing, A. J., Ballard, H. L., Bonney, R., Brown, H., Cook-Patton, S. C., et al. (2017). Citizen science can improve conservation science, natural resource management, and environmental protection. Role Citiz. Sci. Biol. Conserv. 208, 15–28. doi: 10.1016/j.biocon.2016.05.015
McKinney, J. A., Hoffmayer, E. R., Holmberg, J., Graham, R. T., Driggers, W. B. III, de la Parra-Venegas, R., et al. (2017). Long-term assessment of whale shark population demography and connectivity using photo-identification in the Western Atlantic Ocean. PLoS One 12:e0180495. doi: 10.1371/journal.pone.0180495
McKinnon, E. A., Artuso, C., and Love, O. P. (2017). The mystery of the missing warbler. Ecology 98, 1970–1972. doi: 10.1002/ecy.1844
Merkle, J. A., Monteith, K. L., Aikens, E. O., Hayes, M. M., Hersey, K. R., Middleton, A. D., et al. (2016). Large herbivores surf waves of green-up during spring. Proc. Biol. Sci. 283:20160456. doi: 10.1098/rspb.2016.0456
Meusemann, K., von Reumont, B. M., Simon, S., Roeding, F., Strauss, S., Kück, P., et al. (2010). A phylogenomic approach to resolve the arthropod tree of life. Mol. Biol. Evol. 27, 2451–2464. doi: 10.1093/molbev/msq130
Miller, K. E., Ackerman, B. B., Lefebvre, L. W., and Clifton, K. B. (1998). An evaluation of strip-transect aerial survey methods for monitoring manatee populations in Florida. Wildl. Soc. Bull. 26, 561–570.
Moeller, A. K., Lukacs, P. M., and Horne, J. S. (2018). Three novel methods to estimate abundance of unmarked animals using remote cameras. Ecosphere 9:e02331. doi: 10.1002/ecs2.2331
Morales, J. M., Haydon, D. T., Frair, J., Holsinger, K. E., and Fryxell, J. M. (2004). Extracting more out of relocation data: building movement models as mixtures of random walks. Ecology 85, 2436–2445. doi: 10.1890/03-0269
Moxley, J. H., Bogomolni, A., Hammill, M. O., Moore, K. M. T., Polito, M. J., Sette, L., et al. (2017). Google haul out: earth observation imagery and digital aerial surveys in coastal wildlife management and abundance estimation. BioScience 67, 760–768. doi: 10.1093/biosci/bix059
Nackoney, J., Molinario, G., Potapov, P., Turubanova, S., Hansen, M. C., and Furuichi, T. (2014). Impacts of civil conflict on primary forest habitat in northern Democratic Republic of the Congo, 1990-2010. Biol. Conserv. 170, 321–328. doi: 10.1016/j.biocon.2013.12.033
Nakayama, K., Goto, S., Kuraoka, K., and Nakamura, K. (2005). Decrease in nasal temperature of rhesus monkeys (Macaca mulatta) in negative emotional state. Physiol. Behav. 84, 783–790. doi: 10.1016/j.physbeh.2005.03.009
Nepstad, D. C., Verssimo, A., Alencar, A., Nobre, C., Lima, E., Lefebvre, P., et al. (1999). Large-scale impoverishment of Amazonian forests by logging and fire. Nature 398, 505–508. doi: 10.1038/19066
Newson, S. E., Bas, Y., Murray, A., and Gillings, S. (2017). Potential for coupling the monitoring of bush-crickets with established large-scale acoustic monitoring of bats. Methods Ecol. Evol. 8, 1051–1062. doi: 10.1111/2041-210X.12720
Norouzzadeh, M. S., Nguyen, A., Kosmala, M., Swanson, A., Palmer, M. S., Packer, C., et al. (2018). Automatically identifying, counting, and describing wild animals in camera-trap images with deep learning. Proc. Natl. Acad. Sci. U.S.A. 115:E5716. doi: 10.1073/pnas.1719367115
Ovaskainen, O., Roy, D. B., Fox, R., and Anderson, B. J. (2016). Uncovering hidden spatial structure in species communities with spatially explicit joint species distribution models. Methods Ecol. Evol. 7, 428–436. doi: 10.1111/2041-210X.12502
Ovaskainen, O., Tikhonov, G., Norberg, A., Guillaume Blanchet, F., Duan, L., Dunson, D., et al. (2017). How to make more out of community data? A conceptual framework and its implementation as models and software. Ecol. Lett. 20, 561–576. doi: 10.1111/ele.12757
Patterson, T. A., Thomas, L., Wilcox, C., Ovaskainen, O., and Matthiopoulos, J. (2008). State-space models of individual animal movement. Trends Ecol. Evol. 23, 87–94. doi: 10.1016/j.tree.2007.10.009
Pengra, B. W., Johnston, C. A., and Loveland, T. R. (2007). Mapping an invasive plant, Phragmites australis, in coastal wetlands using the EO-1 Hyperion hyperspectral sensor. Remote Sens. Environ. 108, 74–81. doi: 10.1016/j.rse.2006.11.002
Pijanowski, B. C., Villanueva-Rivera, L. J., Dumyahn, S. L., Farina, A., Krause, B. L., Napoletano, B. M., et al. (2011). Soundscape ecology: the science of sound in the landscape. BioScience 61, 203–216. doi: 10.1525/bio.2011.61.3.6
Pochardt, M., Allen, J. M., Hart, T., Miller, S. D. L., Yu, D. W., and Levi, T. (2020). Environmental DNA facilitates accurate, inexpensive, and multiyear population estimates of millions of anadromous fish. Mol. Ecol. Resour. 20, 457–467. doi: 10.1111/1755-0998.13123
Port, J. A., O’Donnell, J. L., Romero-Maraccini, O. C., Leary, P. R., Litvin, S. Y., Nickols, K. J., et al. (2016). Assessing vertebrate biodiversity in a kelp forest ecosystem using environmental DNA. Mol. Ecol. 25, 527–541. doi: 10.1111/mec.13481
Priyadarshani, N., Marsland, S., and Castro, I. (2018). Automated birdsong recognition in complex acoustic environments: a review. J. Avian Biol. 49:01447. doi: 10.1111/jav.01447
Pyron, R. A., Costa, G. C., Patten, M. A., and Burbrink, F. T. (2015). Phylogenetic niche conservatism and the evolutionary basis of ecological speciation. Biol. Rev. 90, 1248–1262. doi: 10.1111/brv.12154
Rayl, N. D., Bastille-Rousseau, G., Organ, J. F., Mumma, M. A., Mahoney, S. P., Soulliere, C. E., et al. (2018). Spatiotemporal heterogeneity in prey abundance and vulnerability shapes the foraging tactics of an omnivore. J. Anim. Ecol. 87, 874–887. doi: 10.1111/1365-2656.12810
Rich, L. N., Furnas, B. J., Newton, D. S., and Brashares, J. S. (2019). Acoustic and camera surveys inform models of current and future vertebrate distributions in a changing desert ecosystem. Divers. Distrib. 25, 1441–1456. doi: 10.1111/ddi.12952
Richard, P. R., Laake, J. L., Hobbs, R. C., Heide-Jørgensen, M. P., Asselin, N. C., and Cleator, H. (2010). Baffin Bay narwhal population distribution and numbers: aerial surveys in the Canadian high arctic, 2002-04. Arctic 63, 85–99.
Ripperger, S. P., Carter, G. G., Page, R. A., Duda, N., Koelpin, A., Weigel, R., et al. (2020). Thinking small: next-generation sensor networks close the size gap in vertebrate biologging. PLoS Biol. 18:e3000655. doi: 10.1371/journal.pbio.3000655
Robinson, O. J., Ruiz-Gutierrez, V., Fink, D., Meese, R. J., Holyoak, M., and Cooch, E. G. (2018). Using citizen science data in integrated population models to inform conservation. Biol. Conserv. 227, 361–368. doi: 10.1016/j.biocon.2018.10.002
Rodgers, A. R. (2001). “Tracking animals with GPS: the first 10 years,” in Proceedings of the Conference on Tracking Animals With GPS, Aberdeen, 1–10.
Rodgers, T. W., Xu, C. C. Y., Giacalone, J., Kapheim, K. M., Saltonstall, K., Vargas, M., et al. (2017). Carrion fly-derived DNA metabarcoding is an effective tool for mammal surveys: evidence from a known tropical mammal community. Mol. Ecol. Resour. 17, e133–e145. doi: 10.1111/1755-0998.12701
Roffler, G. H., Allen, J. M., Massey, A., and Levi, T. (2021). Metabarcoding of fecal DNA shows dietary diversification in wolves substitutes for ungulates in an island archipelago. Ecosphere 12:e03297. doi: 10.1002/ecs2.3297
Roggenbuck, M., Bærholm Schnell, I., Blom, N., Bælum, J., Bertelsen, M. F., Sicheritz-Pontén, T., et al. (2014). The microbiome of New World vultures. Nat. Commun. 5:5498. doi: 10.1038/ncomms6498
Rokas, A., Williams, B. L., King, N., and Carroll, S. B. (2003). Genome-scale approaches to resolving incongruence in molecular phylogenies. Nature 425, 798–804. doi: 10.1038/nature02053
Ross, S. R. P.-J., Friedman, N. R., Dudley, K. L., Yoshimura, M., Yoshida, T., and Economo, E. P. (2018). Listening to ecosystems: data-rich acoustic monitoring through landscape-scale sensor networks. Ecol. Res. 33, 135–147. doi: 10.1007/s11284-017-1509-5
Rowcliffe, J. M., Field, J., Turvey, S. T., and Carbone, C. (2008). Estimating animal density using camera traps without the need for individual recognition. J. Appl. Ecol. 45, 1228–1236. doi: 10.1111/j.1365-2664.2008.01473.x
Royle, J. A. (2004). N-mixture models for estimating population size from spatially replicated counts. Biometrics 60, 108–115. doi: 10.1111/j.0006-341X.2004.00142.x
Royle, J. A., Chandler, R. B., Sollmann, R., and Gardner, B. (2013). Spatial Capture-Recapture. Cambridge, MA: Academic Press.
Royle, J. A., and Kéry, M. (2007). A bayesian state-space formulation of dynamic occupancy models. Ecology 88, 1813–1823. doi: 10.1890/06-0669.1
Ruff, Z. J., Lesmeister, D. B., Appel, C. L., and Sullivan, C. M. (2021). Workflow and convolutional neural network for automated identification of animal sounds. Ecol. Indic. 124:107419. doi: 10.1016/j.ecolind.2021.107419
Ruff, Z. J., Lesmeister, D. B., Duchac, L. S., Padmaraju, B. K., and Sullivan, C. M. (2020). Automated identification of avian vocalizations with deep convolutional neural networks. Remote Sens. Ecol. Conserv. 6, 79–92. doi: 10.1002/rse2.125
Ruprecht, J. S., Eriksson, C. E., Forrester, T. D., Clark, D. A., Wisdom, M. J., Rowland, M. M., et al. (2020). Evaluating and integrating spatial capture-recapture models with data of variable individual identifiability. Ecol. Appl. doi: 10.1101/2020.03.27.010850 [Epub ahead of print].
Rutz, C., and Hays, G. C. (2009). New frontiers in biologging science. Biol. Lett. 5, 289–292. doi: 10.1098/rsbl.2009.0089
Salamon, J., and Bello, J. P. (2017). Deep convolutional neural networks and data augmentation for environmental sound classification. IEEE Signal Process. Lett. 24, 279–283. doi: 10.1109/LSP.2017.2657381
Sandino, J., Gonzalez, F., Mengersen, K., and Gaston, K. J. (2018). UAVs and machine learning revolutionising invasive grass and vegetation surveys in remote arid lands. Sensors 18:605. doi: 10.3390/s18020605
Sauer, J. R., Pendleton, G. W., and Peterjohn, B. G. (1996). Evaluating causes of population change in north american insectivorous songbirds. Conserv. Biol. 10, 465–478. doi: 10.1046/j.1523-1739.1996.10020465.x
Schmidt, K. A., and Ostfeld, R. S. (2003). Songbird populations in fluctuating environments: predator responses to pulsed resources. Ecology 84, 406–415.
Schneider, S., Taylor, G. W., Linquist, S., and Kremer, S. C. (2019). Past, present and future approaches using computer vision for animal re-identification from camera trap data. Methods Ecol. Evol. 10, 461–470. doi: 10.1111/2041-210X.13133
Schnell, I. B., Thomsen, P. F., Wilkinson, N., Rasmussen, M., Jensen, L. R. D., Willerslev, E., et al. (2012). Screening mammal biodiversity using DNA from leeches. Curr. Biol. 22, R262–R263. doi: 10.1016/j.cub.2012.02.058
Scholander, P. F. (1940). Experimental Investigations on the Respiratory Function in Diving Mammals and Birds. Frankfurt: Universitätsbibliothek Johann Christian Senckenberg.
Schuttler, S. G., Sears, R. S., Orendain, I., Khot, R., Rubenstein, D., Rubenstein, N., et al. (2018). Citizen science in schools: students collect valuable mammal data for science, conservation, and community engagement. BioScience 69, 69–79. doi: 10.1093/biosci/biy141
Shakeri, Y. N., White, K. S., and Levi, T. (2018). Salmon-supported bears, seed dispersal, and extensive resource subsidies to granivores. Ecosphere 9:e02297. doi: 10.1002/ecs2.2297
Shehzad, W., Riaz, T., Nawaz, M. A., Miquel, C., Poillot, C., Shah, S. A., et al. (2012). Carnivore diet analysis based on next-generation sequencing: application to the leopard cat (Prionailurus bengalensis) in Pakistan. Mol. Ecol. 21, 1951–1965. doi: 10.1111/j.1365-294X.2011.05424.x
Shonfield, J., and Bayne, E. M. (2017). Autonomous recording units in avian ecological research: current use and future applications. Avian Conserv. Ecol. 12:14. doi: 10.5751/ACE-00974-120114
Sikes, R. S., and Animal Care and Use Committee of the American Society of Mammalogists (2016). 2016 Guidelines of the American Society of Mammalogists for the use of wild mammals in research and education. J. Mammal. 97, 663–688. doi: 10.1093/jmammal/gyw078
Skaug, H. J. (2001). Allele-sharing methods for estimation of population size. Biometrics 57, 750–756. doi: 10.1111/j.0006-341X.2001.00750.x
Slooten, E., Dawson, S., Rayment, W., and Childerhouse, S. (2006). A new abundance estimate for Maui’s dolphin: what does it mean for managing this critically endangered species? Biol. Conserv. 128, 576–581. doi: 10.1016/j.biocon.2005.10.013
Sollmann, R., Furtado, M. M., Gardner, B., Hofer, H., Jácomo, A. T. A., Tôrres, N. M., et al. (2011). Improving density estimates for elusive carnivores: accounting for sex-specific detection and movements using spatial capture-recapture models for jaguars in central Brazil. New Conserv. Debate Parks Vs People 144, 1017–1024. doi: 10.1016/j.biocon.2010.12.011
Sollmann, R., Tôrres, N. M., Furtado, M. M., de Almeida Jácomo, A. T., Palomares, F., Roques, S., et al. (2013). Combining camera-trapping and noninvasive genetic data in a spatial capture-recapture framework improves density estimates for the jaguar. Biol. Conserv. 167, 242–247. doi: 10.1016/j.biocon.2013.08.003
Soltis, D. E., and Soltis, P. S. (2016). Mobilizing and integrating big data in studies of spatial and phylogenetic patterns of biodiversity. Dedic. 100 Years Wu Zhengyi 38, 264–270. doi: 10.1016/j.pld.2016.12.001
Srivathsan, A., Sha, J. C. M., Vogler, A. P., and Meier, R. (2015). Comparing the effectiveness of metagenomics and metabarcoding for diet analysis of a leaf-feeding monkey (Pygathrix nemaeus). Mol. Ecol. Resour. 15, 250–261. doi: 10.1111/1755-0998.12302
Stokstad, E. (2003). Nebraska husks research to ease budget squeeze. Science 300:35. doi: 10.1126/science.300.5616.35b
Sugai, L. S. M., Silva, T. S. F., Ribeiro, J. W. Jr., and Llusia, D. (2018). Terrestrial passive acoustic monitoring: review and perspectives. BioScience 69, 15–25. doi: 10.1093/biosci/biy147
Swanson, A., Kosmala, M., Lintott, C., and Packer, C. (2016). A generalized approach for producing, quantifying, and validating citizen science data from wildlife images. Conserv. Biol. 30, 520–531. doi: 10.1111/cobi.12695
Tabak, M. A., Norouzzadeh, M. S., Wolfson, D. W., Sweeney, S. J., Vercauteren, K. C., Snow, N. P., et al. (2018). Machine learning to classify animal species in camera trap images: applications in ecology. Methods Ecol. Evol. 10, 585–590. doi: 10.1111/2041-210X.13120
Tegeler, A. K., Morrison, M. L., and Szewczak, J. M. (2012). Using extended-duration audio recordings to survey avian species. Wildl. Soc. Bull. 36, 21–29. doi: 10.1002/wsb.112
Teixeira, D., Maron, M., and van Rensburg, B. J. (2019). Bioacoustic monitoring of animal vocal behavior for conservation. Conserv. Sci. Pract. 1:e72. doi: 10.1111/csp2.72
Tessler, M., Weiskopf, S. R., Berniker, L., Hersch, R., Mccarthy, K. P., Yu, D. W., et al. (2018). Bloodlines: mammals, leeches, and conservation in southern Asia. Syst. Biodivers. 16, 488–496. doi: 10.1080/14772000.2018.1433729
Tewksbury, J. J., Anderson, J. G. T., Bakker, J. D., Billo, T. J., Dunwiddie, P. W., Groom, M. J., et al. (2014). Natural history’s place in science and society. BioScience 64, 300–310. doi: 10.1093/biosci/biu032
Theobald, E. J., Ettinger, A. K., Burgess, H. K., DeBey, L. B., Schmidt, N. R., Froehlich, H. E., et al. (2015). Global change and local solutions: tapping the unrealized potential of citizen science for biodiversity research. Biol. Conserv. 181, 236–244. doi: 10.1016/j.biocon.2014.10.021
Thompson, I. D., Bakhtiari, M., Rodgers, A. R., Baker, J. A., Fryxell, J. M., and Iwachewski, E. (2012). Application of a high-resolution animal-borne remote video camera with global positioning for wildlife study: observations on the secret lives of woodland caribou. Wildl. Soc. Bull. 36, 365–370. doi: 10.1002/wsb.130
Thomsen, P. F., Kielgast, J., Iversen, L. L., Wiuf, C., Rasmussen, M., Gilbert, M. T. P., et al. (2012). Monitoring endangered freshwater biodiversity using environmental DNA. Mol. Ecol. 21, 2565–2573. doi: 10.1111/j.1365-294X.2011.05418.x
Tilker, A., Abrams, J. F., Nguyen, A., Hörig, L., Axtner, J., Louvrier, J., et al. (2020). Identifying conservation priorities in a defaunated tropical biodiversity hotspot. Divers. Distrib. 26, 426–440. doi: 10.1111/ddi.13029
Tomkiewicz, S. M., Fuller, M. R., Kie, J. G., and Bates, K. K. (2010). Global positioning system and associated technologies in animal behaviour and ecological research. Philos. Trans. R. Soc. B Biol. Sci. 365, 2163–2176. doi: 10.1098/rstb.2010.0090
Trouille, L., Lintott, C. J., and Fortson, L. F. (2019). Citizen science frontiers: efficiency, engagement, and serendipitous discovery with human-machine systems. Proc. Natl. Acad. Sci. U.S.A. 116, 1902–1909. doi: 10.1073/pnas.1807190116
Turrini, T., Dörler, D., Richter, A., Heigl, F., and Bonn, A. (2018). The threefold potential of environmental citizen science - generating knowledge, creating learning opportunities and enabling civic participation. Biol. Conserv. 225, 176–186. doi: 10.1016/j.biocon.2018.03.024
Udevitz, M. S., Burn, D. M., and Webber, M. A. (2008). Estimation of walrus populations on sea ice with infrared imagery and aerial photography. Mar. Mammal Sci. 24, 57–70. doi: 10.1111/j.1748-7692.2007.00169.x
Vacher, J.-P., Chave, J., Ficetola, F. G., Sommeria-Klein, G., Tao, S., Thébaud, C., et al. (2020). Large-scale DNA-based survey of frogs in Amazonia suggests a vast underestimation of species richness and endemism. J. Biogeogr. 47, 1781–1791. doi: 10.1111/jbi.13847
Vargas Zeppetello, L. R., Parsons, L. A., Spector, J. T., Naylor, R. L., Battisti, D. S., Masuda, Y. J., et al. (2020). Large scale tropical deforestation drives extreme warming. Environ. Res. Lett. 15:084012. doi: 10.1088/1748-9326/ab96d2
Vendetti, J. E., Burnett, E., Carlton, L., Curran, A. T., Lee, C., Matsumoto, R., et al. (2019). The introduced terrestrial slugs Ambigolimax nyctelius (Bourguignat, 1861) and Ambigolimax valentianus (Férussac, 1821) (Gastropoda: Limacidae) in California, with a discussion of taxonomy, systematics, and discovery by citizen science. J. Nat. Hist. 53, 1607–1632. doi: 10.1080/00222933.2018.1536230
Wäldchen, J., and Mäder, P. (2018). Plant species identification using computer vision techniques: a systematic literature review. Arch. Comput. Methods Eng. 25, 507–543. doi: 10.1007/s11831-016-9206-z
Wang, Y., Nickel, B., Rutishauser, M., Bryce, C. M., Williams, T. M., Elkaim, G., et al. (2015). Movement, resting, and attack behaviors of wild pumas are revealed by tri-axial accelerometer measurements. Mov. Ecol. 3:2. doi: 10.1186/s40462-015-0030-0
Warton, D. I., Blanchet, F. G., O’Hara, R. B., Ovaskainen, O., Taskinen, S., Walker, S. C., et al. (2015). So many variables: joint modeling in community ecology. Trends Ecol. Evol. 30, 766–779. doi: 10.1016/j.tree.2015.09.007
Wasser, S. K., Torkelson, A., Winters, M., Horeaux, Y., Tucker, S., Otiende, M. Y., et al. (2018). Combating transnational organized crime by linking multiple large ivory seizures to the same dealer. Sci. Adv. 4:eaat0625. doi: 10.1126/sciadv.aat0625
Wiens, J. J., Kuczynski, C. A., Hua, X., and Moen, D. S. (2010). An expanded phylogeny of treefrogs (Hylidae) based on nuclear and mitochondrial sequence data. Mol. Phylogenet. Evol. 55, 871–882. doi: 10.1016/j.ympev.2010.03.013
Willerslev, E., Hansen, A. J., Binladen, J., Brand, T. B., Gilbert, M. T. P., Shapiro, B., et al. (2003). Diverse plant and animal genetic records from holocene and pleistocene sediments. Science 300:791. doi: 10.1126/science.1084114
Williams, C. L., Blejwas, K., Johnston, J. J., and Jaeger, M. M. (2003). A coyote in sheep’s clothing: predator identification from saliva. Wildl. Soc. Bull. 31, 926–932.
Williams, T. M., Wolfe, L., Davis, T., Kendall, T., Richter, B., Wang, Y., et al. (2014). Instantaneous energetics of puma kills reveal advantage of felid sneak attacks. Science 346:81. doi: 10.1126/science.1254885
Willson, M. F., and Armesto, J. J. (2006). Is natural history really dead? Toward the rebirth of natural history. Rev. Chil. Hist. Nat. 79, 279–283.
Wilmers, C. C., Isbell, L. A., Suraci, J. P., and Williams, T. M. (2017). Energetics-informed behavioral states reveal the drive to kill in African leopards. Ecosphere 8:e01850. doi: 10.1002/ecs2.1850
Wilmers, C. C., Nickel, B., Bryce, C. M., Smith, J. A., Wheat, R. E., and Yovovich, V. (2015). The golden age of bio-logging: how animal-borne sensors are advancing the frontiers of ecology. Ecology 96, 1741–1753. doi: 10.1890/14-1401.1
Wilson, A. M., Lowe, J. C., Roskilly, K., Hudson, P. E., Golabek, K. A., and McNutt, J. W. (2013). Locomotion dynamics of hunting in wild cheetahs. Nature 498, 185–189. doi: 10.1038/nature12295
Willson, M. F., and Armesto, J. J. (2006). Is natural history really dead? Toward the rebirth of natural history. Rev. Chil. Hist. Nat 79, 279–283.
Wood, C. M., Klinck, H., Gustafson, M., Keane, J. J., Sawyer, S. C., Gutiérrez, R. J., et al. (2021). Using the ecological significance of animal vocalizations to improve inference in acoustic monitoring programs. Conserv. Biol. 35, 336–345. doi: 10.1111/cobi.13516
Wrege, P. H., Rowland, E. D., Keen, S., and Shiu, Y. (2017). Acoustic monitoring for conservation in tropical forests: examples from forest elephants. Methods Ecol. Evol. 8, 1292–1301. doi: 10.1111/2041-210X.12730
Xue, Z., Zhang, W., Wang, L., Hou, R., Zhang, M., Fei, L., et al. (2015). The bamboo-eating giant panda harbors a carnivore-like gut microbiota, with excessive seasonal variations. mBio 6:e00022-15. doi: 10.1128/mBio.00022-15
Yanai, I., and Lercher, M. (2020). A hypothesis is a liability. Genome Biol. 21:231. doi: 10.1186/s13059-020-02133-w
Keywords: conservation, ecology, field studies, nature, environmental management
Citation: Tosa MI, Dziedzic EH, Appel CL, Urbina J, Massey A, Ruprecht J, Eriksson CE, Dolliver JE, Lesmeister DB, Betts MG, Peres CA and Levi T (2021) The Rapid Rise of Next-Generation Natural History. Front. Ecol. Evol. 9:698131. doi: 10.3389/fevo.2021.698131
Received: 20 April 2021; Accepted: 29 June 2021;
Published: 21 July 2021.
Edited by:
David Jack Coates, Department of Biodiversity, Conservation and Attractions (DBCA), AustraliaReviewed by:
William Ernest Magnusson, National Institute of Amazonian Research (INPA), BrazilCopyright © 2021 Tosa, Dziedzic, Appel, Urbina, Massey, Ruprecht, Eriksson, Dolliver, Lesmeister, Betts, Peres and Levi. This is an open-access article distributed under the terms of the Creative Commons Attribution License (CC BY). The use, distribution or reproduction in other forums is permitted, provided the original author(s) and the copyright owner(s) are credited and that the original publication in this journal is cited, in accordance with accepted academic practice. No use, distribution or reproduction is permitted which does not comply with these terms.
*Correspondence: Marie I. Tosa, dG9zYS5tYXJpZUBnbWFpbC5jb20=
Disclaimer: All claims expressed in this article are solely those of the authors and do not necessarily represent those of their affiliated organizations, or those of the publisher, the editors and the reviewers. Any product that may be evaluated in this article or claim that may be made by its manufacturer is not guaranteed or endorsed by the publisher.
Research integrity at Frontiers
Learn more about the work of our research integrity team to safeguard the quality of each article we publish.