- 1Department of Anthropology, The Pennsylvania State University, University Park, PA, United States
- 2African Studies Program, The Pennsylvania State University, University Park, PA, United States
Archaeologists interested in the evolution of anthropogenic landscapes have productively adopted Niche Construction Theory (NCT), in order to assess long-term legacies of human-environment interactions. Applications of NCT have especially been used to elucidate co-evolutionary dynamics in agricultural and pastoral systems. Meanwhile, foraging and/or highly mobile small-scale communities, often thought of as less intensive in terms of land-use than agropastoral economies, have received less theoretical and analytical attention from a landscape perspective. Here we address this lacuna by contributing a novel remote sensing approach for investigating legacies of human-environment interaction on landscapes that have a long history of co-evolution with highly mobile foraging communities. Our study is centered on coastal southwest Madagascar, a region inhabited by foraging and fishing communities for close to two millennia. Despite significant environmental changes in southwest Madagascar’s environment following human settlement, including a wave of faunal extinctions, little is known about the scale, pace and nature of anthropogenic landscape modification. Archaeological deposits in this area generally bear ephemeral traces of past human activity and do not exhibit readily visible signatures of intensive land-use and landscape modification (e.g., agricultural modifications, monumental architecture, etc.). In this paper we use high-resolution satellite imagery and vegetative indices to reveal a legacy of human-landscape co-evolution by comparing the characteristics – vegetative productivity and geochemical properties – of archaeological sites to those of locations with no documented archaeological materials. Then, we use a random forest (RF) algorithm and spatial statistics to quantify the extent of archaeological activity and use this analysis to contextualize modern-day human-environment dynamics. Our results demonstrate that coastal foraging communities in southwest Madagascar over the past 1,000 years have extensively altered the landscape. Our study thus expands the temporal and spatial scales at which we can evaluate human-environment dynamics on Madagascar, providing new opportunities to study early periods of the island’s human history when mobile foraging communities were the dominant drivers of landscape change.
Introduction
Among archaeologists, the identification and quantification of feedbacks between past human activities and landscape-scale transformations have historically focused on economies of scale that generate highly visible changes to landscapes (e.g., monumental architecture, intensive agriculture, shifts from forests to grasslands, etc.). In contrast, activities associated with small-scale mobile foraging economies have generally been portrayed as “low impact” (Smith, 2001; Stephens et al., 2019). In regions around the world, like the Amazon, this line of thought has been compounded by legacies of settler colonialism and the processes of industrialization of land and resource use, resulting in narratives that downplay, obscure, or erase earlier (and subtler) traces of human landscape use (Heckenberger and Neves, 2009; Douglass and Cooper, 2020). Uses of remote sensing technology (i.e., satellite images) in such contexts have successfully identified these “low-impact” signatures of human action on large geographic scales (Lombardo and Prümers, 2010). While predictive models have been used around the world to narrow the search for ephemeral archaeological deposits (McMichael et al., 2014; Kirk et al., 2016; Davis et al., 2020a), the use of automated remote sensing in archaeology remains skewed toward landscape modifications that are easy to identify because of their lasting marks and effects (Tarolli et al., 2019; Davis, 2021).
Prior remote sensing analyses on Madagascar have focused on the drivers of settlement and mobility (e.g., resource availability and social aggregation) using predictive models based on satellite-derived environmental information and statistical modeling (Davis et al., 2020a, b). To fully understand settlement patterns, however, we must also look at the ecological effects of human occupation (Figure 1). Therefore, in this paper, we focus on identifying and characterizing legacies of forager activities and coastal settlement on the landscape of the Velondriake Marine Protected Area of southwest Madagascar, a region with a history of coastal foraging that extends back at least to 2,000 cal year BP (Figure 2; Douglass et al., 2019). The climate of southwest of Madagascar is defined by a wet season and a dry season. The region is extremely arid, receiving less than 50 cm of rainfall annually, and is home to highly endemic flora and fauna which varies according to the underlying geology (Douglass and Zinke, 2015). The Velondriake region sits on a Quaternary dune system composed of sandy-shell and limestone coastal rock outcrops, while further inland the geology is characterized by Pleistocene and Eocene geological systems (Besairie, 1964). Vegetation in Velondriake is largely xerophytic and classified as a “spiny thicket” ecoregion, which contains some of the highest levels of endemic flora on the island (Gautier and Goodman, 2003). The people living in coastal Velondriake today primarily identify as Vezo (roughly translated as “to paddle”) and center their livelihood on the sea (Astuti, 1995). The area has been inhabited for thousands of years by communities practicing foraging and fishing, as well as agropastoralism over the past several 100 years (Douglass et al., 2018; Hixon et al., 2021).
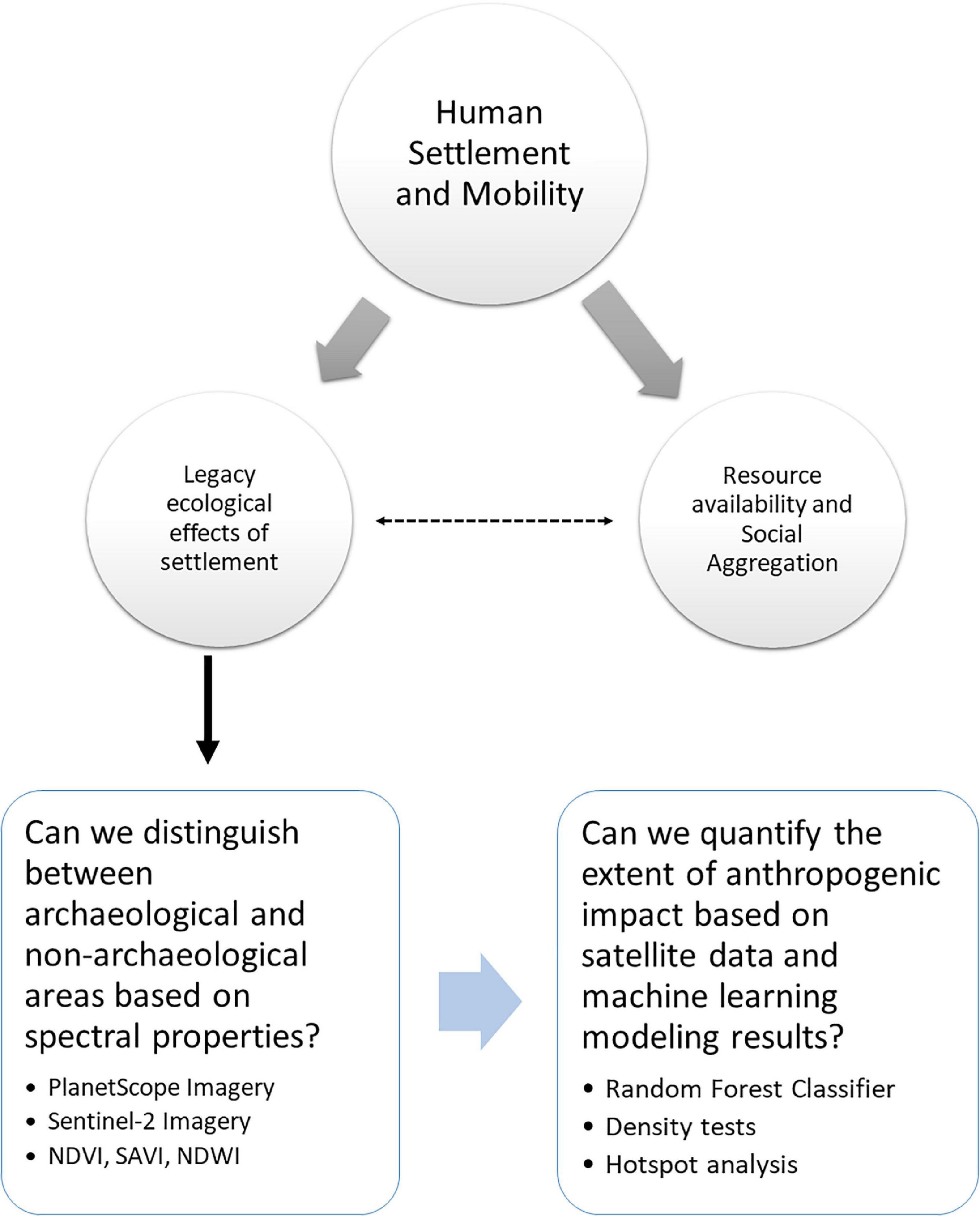
Figure 1. Illustrates the methodological workflow presented in this article. The central question of human settlement and mobility patterns has been investigated previously by Davis et al. (2020a,b) as a consequence of resource availability and social aggregation. The results of this prior work feed directly into this article, and vice versa (indicated by dashed arrow). Within this paper we evaluate whether we can distinguish between archaeological and non-archaeological areas recorded in our previous work based on spectral properties (including vegetative indices). Then, we use these results to train a machine learning classifier to quantify the extent of anthropogenic impacts.
In this article, we investigate whether the ancient communities inhabiting the Velondriake region in southwest Madagascar significantly modified their landscape in ways that persist into the present. Specifically, we hypothesize that lasting changes in vegetative communities and soil chemistry are legacies of fitness-enhancing activities that have landscape-scale effects. We further demonstrate that these legacies can be systematically characterized using remote sensing and machine learning techniques. Machine learning, specifically probability-based methods (e.g., Breiman, 2001; Malley et al., 2012), allow us to systematically evaluate the likelihood of anthropogenic disturbance across large geographic spaces based on the geophysical properties identified in remotely sensed data. We look at geophysical properties of vegetation and soil as a palimpsest resulting from movement, settlement, and resource use over thousands of years. We then compare these landscape signatures between locations with and without material culture surface scatters to assess cumulative anthropogenic effects on the landscape. Our expectation is that places where people settled or engaged in sustained land-use will exhibit significantly different patterns in vegetative and soil properties when compared to locations with no evidence of archaeological settlement.
While the exact timing of the initial peopling of Madagascar is hotly debated (e.g., Anderson et al., 2018; Douglass et al., 2019; Hansford et al., 2020; Mitchell, 2020), coastal foraging has a long history on the island (e.g., Barret, 1985; Rakotozafy, 1996; Dewar et al., 2013; Douglass, 2016; Douglass et al., 2018), and predates evidence for the introduction of farming and herding lifeways (Domic et al., under review; Godfrey et al., 2019; Hixon et al., 2021). The debate over Madagascar’s human settlement has been central to questions of anthropogenic impact on the island, notably via activities that may have contributed to the extinction of endemic fauna (Godfrey and Douglass, in press). Theories regarding anthropogenic drivers of extinction include potential overhunting, habitat modification, and forms of direct or indirect competition between endemic and introduced animals (Dewar, 1984, 1997; Burney, 1997, 1999; Hixon et al., 2018; Godfrey et al., 2019). Teasing apart drivers of extinction requires further clarification of anthropogenic landscape change, including in regions with long histories of foraging and the management of wild resources. Madagascar is also known for its long history of climatic variability (e.g., Dewar and Richard, 2007; Douglass and Zinke, 2015). As climate change impacts intensify today, studies of how past populations modified landscapes to mitigate the impacts of resource scarcity and climate variability are vital for promoting sustainability (Razanatsoa et al., in press; Douglass and Cooper, 2020; Douglass and Rasolondrainy, 2021). However, most archaeological sites that retain information about resource use and adaptation by early mobile communities of foragers and herders consist of ephemeral artifact surface scatters and are actively disappearing due to erosion and development (Parker Pearson, 2010; Davis et al., 2020b). Innovative approaches are thus particularly needed to clarify human-environment dynamics during the earliest phases of Madagascar’s human settlement and in regions vulnerable to loss or erosion of cultural landscapes.
Niche Construction and the Legacy of Land-Use Practices
Anthropologists interested in the effects that humans have on their environment and the two-way feedbacks in socio-ecological systems have productively integrated Niche Construction Theory (NCT; Laland and O’Brien, 2010; Fuentes, 2016; Zeder, 2016). NCT, which stems from evolutionary biology, stresses that organisms actively modify the selective pressures in their environment in order to increase their fitness. In doing so, all organisms contribute to feedbacks that influence and alter the niches of other organisms that share those same spaces (Odling-Smee et al., 2003; Laland and O’Brien, 2010). It should be emphasized that many of the concepts of NCT borrow from related concepts previously described by evolutionary biologists (see Spengler, 2021), such as environmental engineering (e.g., Jones et al., 1994, 2010) – central to the work presented here. NCT has guided investigations of how “low impact” human activities create niches by altering the distribution and abundance of flora and fauna in ways that enhance human livelihoods (Rowley-Conwy and Layton, 2011). Prior work shows that many such niche construction activities are identifiable through soil changes (Smith, 2001) and alterations to vegetation (e.g., modification of trees) (Mobley and Eldridge, 1992; Oliver, 2007). Investigations of cultural landscapes further demonstrate how humans intentionally manipulate soil chemistry and vegetative growth patterns (Lightfoot et al., 2013). Such niche construction activities have landscape-scale effects that are difficult to assess using site-based approaches alone. Furthermore, taphonomic processes and other destructive forces can disproportionately affect the remains of ephemeral sites (i.e., foraging camps) and evidence for landscape management by “low impact” communities with high levels of mobility (Iovita et al., n.d.; Smith, 2001). Applications of NCT and related evolutionary frameworks to landscapes shaped by foraging economies have also revealed feedbacks between human use of fire, biodiversity, and resilience to climate change (Bliege Bird et al., 2008, 2020; Bird et al., 2016; Bliege Bird and Bird, 2020). This work demonstrates the vast spatial extent and persistence through time of legacies of landscape management by foragers, including through innovative use of historical aerial photographs that permit landscape-scale analysis of the effect of previous fire regimes on contemporary settlement and land-use patterns (Bliege Bird et al., 2020).
Our paper aims to build on this previous work by combining archaeological satellite-based remote sensing and innovative computational automation approaches to reveal legacies of forager land-use in coastal Madagascar. Prior remote sensing studies of archaeological foraging societies (and recently paleoanthropological sites) have taken place around the world, and many have relied on unsupervised land-cover classifications and environmental proxies to narrow down survey areas to where ephemeral cultural deposits are likely to exist (Keeney and Hickey, 2015; Davis et al., 2020a; d’Oliveira Coelho et al., 2021; Lim et al., 2021). Here, we attempt to use multispectral satellite data to directly pinpoint ecological changes associated with archaeological activity. Innovating and expanding the use of approaches that can effectively reveal “low-impact” signatures of human-environment dynamics is critical for developing more holistic understandings of the contributions of diverse communities – including highly mobile foraging communities – to shaping landscapes (Crumley, 1979).
In leveraging NCT, we are also engaging principles of relocation and perturbation (Figure 3), wherein relocation refers to the movement of certain ideas and resources, and perturbation refers to the subsequent adaptation and changes to both human populations and environments over time (Odling-Smee et al., 2003; Quintus and Cochrane, 2018). Signatures of a modified niche include changes to biotic, abiotic, and artifactual components of the environment, and must be related to intentional, non-random processes (Odling-Smee et al., 2013; also see Jones et al., 2010). In order for remote sensing tools to identify niche construction, we must be able to recognize instances of both perturbation (to quantify landscape modifications, themselves) and relocation (to identify different kinds of niche construction and the potential movement of people across a landscape). Relocation and perturbation should be traceable via similarities in material culture between sites, but also via patterns in vegetative and soil properties; i.e., archaeological niche construction will display perturbations in the form of distinct geophysical characteristics related to vegetative and soil characteristics and these differences will be relocated as communities migrate between places.
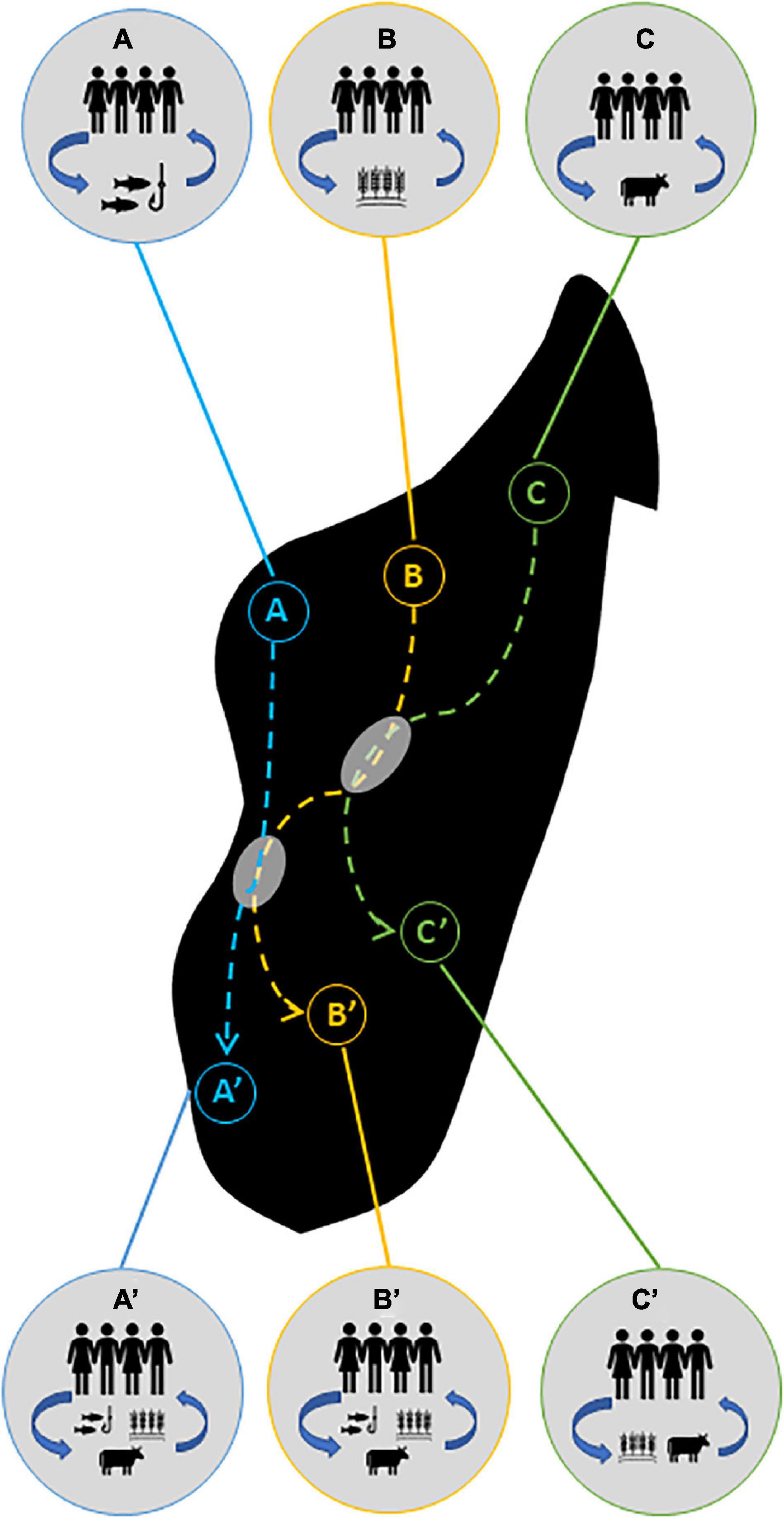
Figure 3. Illustration of perturbation and relocation components of Niche Construction Theory (NCT). Each population (A–C) performs specific activities which create feedbacks (blue arrows) between themselves and their surrounding environments. This constitutes a cultural “niche.” Relocation is represented by the dashed arrows, in which people move throughout a landscape and take their practices with them. In addition, ideas and adaptations can be spread from one community to another (gray circles) when different groups overlap in time and space, leading to the adoption of new behaviors and the formation of new niches (A’–C’).
In this study, we focus primarily on perturbation effects. Relocation will be the focus of future work and will require a robust radiocarbon dataset to evaluate settlement chronologies and temporal shifts in settlement. Nonetheless, our present study has ramifications for how archaeologists reconstruct and understand the impacts of foraging societies on a global scale, as the methods proposed here can be widely applied. It also contributes new insights into longstanding questions regarding the consequences of human colonization of the unique insular environments of the southwestern Indian Ocean, and Madagascar specifically.
Materials and Methods
Previous studies, using traditional ground-based and remote sensing survey (Douglass, 2016; Davis et al., 2020a, b), have expanded our understanding of Late Holocene (∼3,000 cal year B.P.) settlements on Madagascar’s southwest coast. This work assessed whether settlement was affected by resource availability and used satellite-derived information about environmental factors (e.g. proximity to important resources) to generate a probability model to locate archaeological settlements. This work documented hundreds of new archaeological deposits and indicated that freshwater availability, marine resources, and defensibility are among the primary drivers of settlement choice and mobility in this region since the Late Holocene. Additionally, this work suggested the presence of Allee effects, or positive density dependence, wherein settlement actively modifies surrounding environments and results in improvements to habitat suitability (Fretwell and Lucas, 1969; Angulo et al., 2018).
In this paper, we are addressing whether ecological legacies of landscape modification exist and whether these constructed niches – primarily in the form of soil and vegetative properties – can allow us to predict ancient settlement locations and provide insight as to the extent of anthropogenic landscape modifications (Figure 1). In order to do this, we must first determine whether available satellite data have the spectral resolution needed to discern between known archaeological sites and places that do not contain material culture deposits (Figure 1). We use high-resolution PlanetScope satellite imagery (Planet Team, 2020) with 3 m spatial resolution and multispectral capabilities to calculate vegetative productivity and soil moisture content in surveyed areas with known archaeological sites (n = 340). In total, we averaged 6 PlanetScope images taken between 2018 and 2020 during the wet and dry seasons to create a 3-year average of the study region (Supplementary Table 1). The years chosen experienced climatic conditions within the typical range for the region (Andavadoaka Monthly Climate Averages, n.d.). We supplemented PlanetScope images with multispectral bands available from Sentinel-2 to provide additional assessment of moisture retention properties of soils and vegetation (see below). We then compared these values with ground-tested locations without any evidence of archaeological materials based on absence of surface deposits (i.e., ceramics, shells, charcoal, etc.; n = 80). Sites in this region tend to feature single occupation horizons and relatively shallow cultural deposits that are typically indicated by the presence of surface scatters (Douglass, 2016). All archaeological and non-archaeological datapoints were recorded during systematic survey operations between 2011 and 2020 (Douglass, 2016; Davis et al., 2020a).
Machine Learning Algorithm
We used PlanetScope imagery and compiled 3-year averages for the dry and wet seasons between 2018 and 2020. Seasonal differences are extreme in this region (Jury, 2003), and thus we need to account for these variations and how they affect our ability to discern archaeological materials in satellite data. As such, we conducted a pixel-by-pixel comparison in R (R Core Team, 2020) between the 3-year averaged PlanetScope images to highlight differences in environmental geophysical properties between the wet and dry season using the equation:
Where Δr, difference between images; D, the dry season image, and W, the wet season image.
Next, we assessed the spectral characteristics of a sample of known archaeological (n = 340) and non-archaeological (n = 80) deposits throughout the study area to determine their degree of separability between different image bands. Some prior studies have chosen non-archaeological sites randomly, but here we used ground-tested locations to alleviate potential errors in sample creation (Sonnemann et al., 2017). We drew 10 m buffers around each data point and the average value for each band was calculated in R (R Core Team, 2020). Then we statistically compared archaeological and non-archaeological sites using different bands of PlanetScope imagery (see Supplementary File).
Next, we used Google Earth Engine (GEE; Gorelick et al., 2017), following Orengo et al. (2020), to train a random forest (RF) probability algorithm to identify archaeological deposits in southwest Madagascar. Because the archaeological deposits in this area are primarily ephemeral artifact scatters, high resolution data are required to attempt any sort of automated identification. We ran a RF probability algorithm using 128 trees and three iterations [which were deemed optimal for archaeological purposes by Orengo et al. (2020)] to locate artifact scatters (code can be found in the Supplementary document). The RF procedure outputs a raster of values ranging from 0 to 1, wherein 1 is a perfect match to an archaeological deposit. To evaluate accuracy and performance of this model, we withheld 40 archaeological points and 17 non-archaeological points (∼12% of the training data) for validation. Then we calculated the precision (Equation 1), recall (Equation 2), and F1 (Equation 3) scores for the training and test data using thresholds of 0.60, 0.65, and 0.70.
A perfect result would have precision, recall, and F1 scores of 1.
Vegetative Indices
To evaluate ecological signatures between archaeological and non-archaeological areas, we used vegetative indices, which are mathematical formulas that provide indications of biomass and plant health/stress. First, we measured vegetative productivity using normalized difference (NDVI) and soil adjusted (SAVI) vegetative indices (Jensen, 2007). NDVI is calculated using the formula:
SAVI is calculated using the formula:
Where L is a soil adjustment factor. An optimal value for L has been demonstrated at L = 0.5 and was used here (Huete, 1988).
NDVI is one of the most commonly employed vegetative indices and measures vegetation based on a ratio of reflectance values in the near infrared (NIR) and Red wavelengths. However, NDVI has accuracy issues when faced with ecologically and geologically heterogeneous areas and high soil reflectance. The SAVI is an adjusted version of NDVI which corrects for reflectance caused by soil diversity, making it useful for geographically expansive studies with high rates of ecological diversity (Huete, 1988). Both NDVI and SAVI relay information pertaining to water absorption and retention in vegetation. After calculating vegetative index values, we extracted the average values from a 20 m buffer around each archaeological and non-archaeological datapoint used for training the RF algorithm (discussed above). Then we assessed the difference between archaeological and undisturbed locations using non-parametric tests of association (see Supplementary File). We conducted all calculations in R v. 4.0.2 (R Core Team, 2020) using the raster (Hijmans, 2019) and rgdal (Bivand et al., 2019) packages.
Next, we evaluated Sentinel-2 imagery, which contains short-wave infrared (SWIR) bands, in the same manner as described above. We compiled 5 years of Sentinel-2 imagery by month using GEE (Gorelick et al., 2017; also see Supplementary File). SWIR has increased sensitivity to moisture content and can be used to distinguish mineral compositions of soils (Davis, 2017; Thabeng et al., 2020). One limitation of the Sentinel-2 data is that it has much lower spatial resolution (20 m). The only high-resolution SWIR satellite currently available is Maxar’s Worldview-3 satellite (which has shown promise for archaeological purposes, see Davis, 2017). We did not have access to these particular data, however. To improve the utility of the Sentinel-2 SWIR, we used a pansharpening procedure – a form of data fusion whereby lower-spatial resolution imagery is enhanced using a higher-resolution dataset (Garzelli et al., 2004) – to resample the SWIR data from 20 to 3 m using PlanetScope imagery (see Supplementary Figure 1). Pan-sharpening followed a principle-component analysis (PCA) method using the RStoolbox package in R (Leutner et al., 2019). PCA pansharpening is appropriate because PlanetScope imagery is spectrally compatible with Sentinel-2 sensors (Ichikawa and Wakamori, 2018).
Using this pansharpened SWIR data, we calculated a Normalized Difference Water Index (NDWI; Gao, 1996), which is a vegetative index that reflects the biochemical metrics of plants (Sun et al., 2019). Such metrics can be used to distinguish different taxa, in addition to assessing the water content of leaves (Gao, 1996; Sun et al., 2019). NDWI uses the NIR and SWIR spectrum to measure the liquid water molecules contained within vegetative canopies (Gao, 1996). It is calculated using the formula:
Where NIR is the near-infrared band and SWIR1 corresponds to the first shortwave-infrared band of Sentinel 2 (1,610 nm).
Finally, to assess the potential bias in our samples of archaeological and non-archaeological points, we generated 1,000 random points within the study area and compared vegetative index and SWIR reflectance values between them and our ground-tested data (Supplementary Figure 2). If people have fundamentally changed the geophysical and/or geochemical properties of the landscape, we hypothesized earlier that the random locations will express different vegetative and soil properties than areas with archaeological surface scatters. Conversely, if people merely settled in areas with specific geochemical characteristics that are distributed throughout the landscape, random locations should show some similarities with archaeological areas.
Spatial Analysis
In order to quantify the impact of niche construction on different parts of the study area, we ran several spatial analyses to determine the amount of land area impacted by legacy effects and the distribution of niche construction activities across the landscape. Settlements in this region are non-randomly distributed (Davis et al., 2020b), as are niche construction activities more generally (Odling-Smee et al., 2013). Thus, anthropogenic niche construction on Madagascar should also be non-random, and likely clusters in particular places that have been used repeatedly over time. After assessing the results of the RF algorithm, the locations identified as archaeological sites with the threshold with the highest accuracy scores were converted to polygons. We calculated the area of these polygons to generate an assessment of the extent of archaeological activity within the study region. Next, we analyzed the spatial distribution of these points using the Getis–Ord General G test to compare the distribution of areas identified as archaeological by the RF algorithm with random patterns (Getis and Ord, 1992). Then we converted the polygons of anthropogenic locations into points and computed kernel density estimations for these locations. We conducted all spatial tests in in ArcGIS 10.7.1 (ESRI, 2020).
Results
Assessments of training datasets and PlanetScope imagery resulted in clear distinctions between archaeological and non-archaeological points in all four electromagnetic bands (Blue, Green, Red, and NIR; Figure 4B). The differences between the samples are also statistically significant (see Supplementary File). The RF classifier was trained using 300 archaeological points and 63 non-archaeological points and resulted in strong performance on both the training data and test data (see Figure 4 and Table 1).
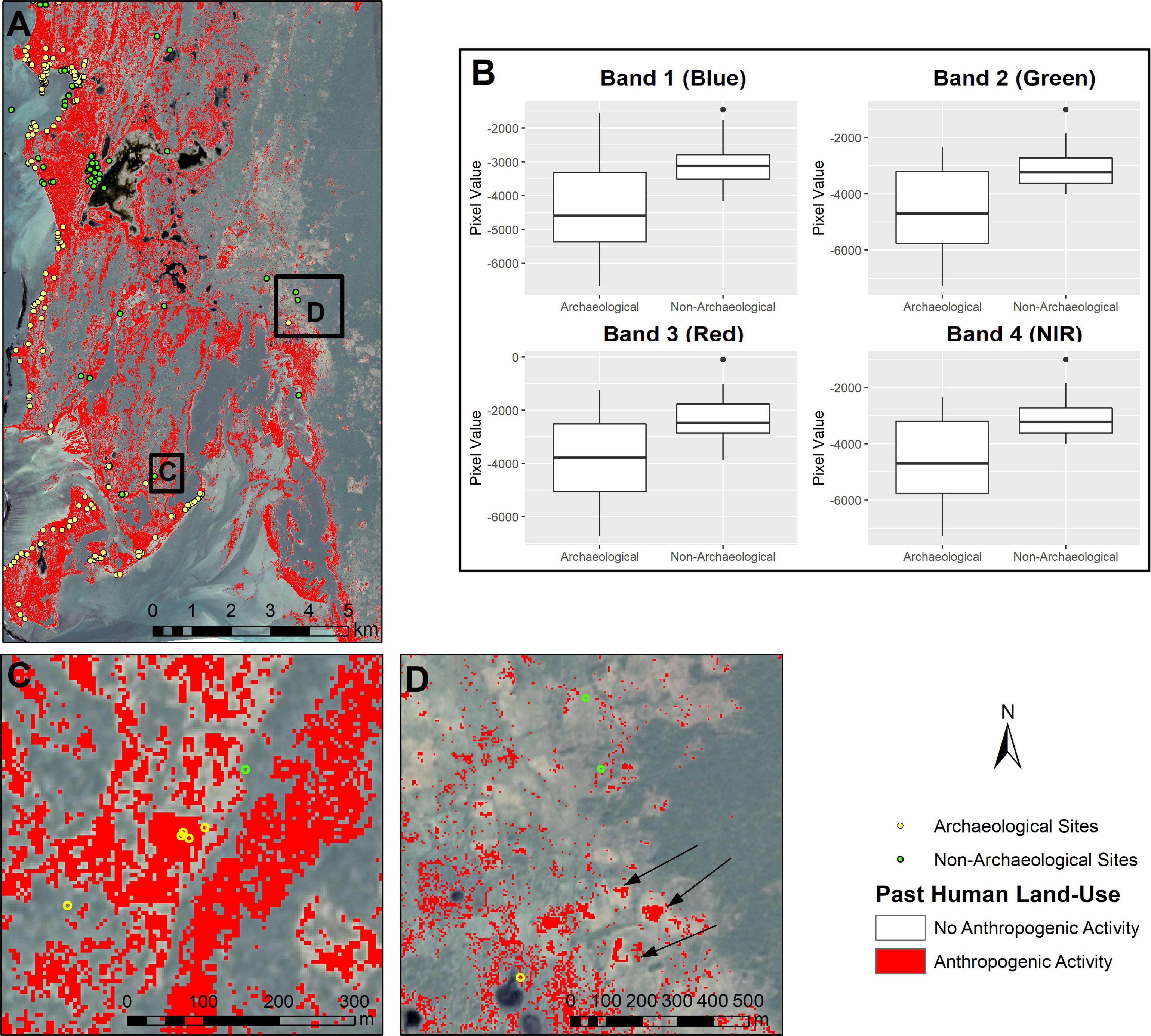
Figure 4. (A) Results of random forest (RF) algorithm (red) using a threshold of 0.7 compared with known archaeological deposits (yellow circles) and non-archaeological areas (green circles). (B) Visualization of band separability between archaeological and non-archaeological training data. All band differences are statistically significant (p < 0.001). (C) Close up of known archaeological and non-archaeological sites. All but one are correctly identified. (D) Another area with anthropogenic activity (forest clearings indicated by arrows) and known archaeological sites. Imagery © 2020 Planet Labs. Inc.
Analyses of annually averaged NDVI and SAVI show a significant difference (W = 8639, p < 0.05) between vegetation in archaeological and non-archaeological contexts, where archaeological sites have higher mean vegetative index values than locations without archaeological activity (Figure 5; mean of −0.02 and −0.03, respectively). NDWI results show the same pattern (W = 8468, p-value = 0.042). This suggests that archaeological sites exhibit vegetative index values that are distinct from non-archaeological areas. Furthermore, archaeological sites are noticeably different in their vegetative index value distribution from randomly generated points throughout the study region (see Supplementary Figures 2–5).
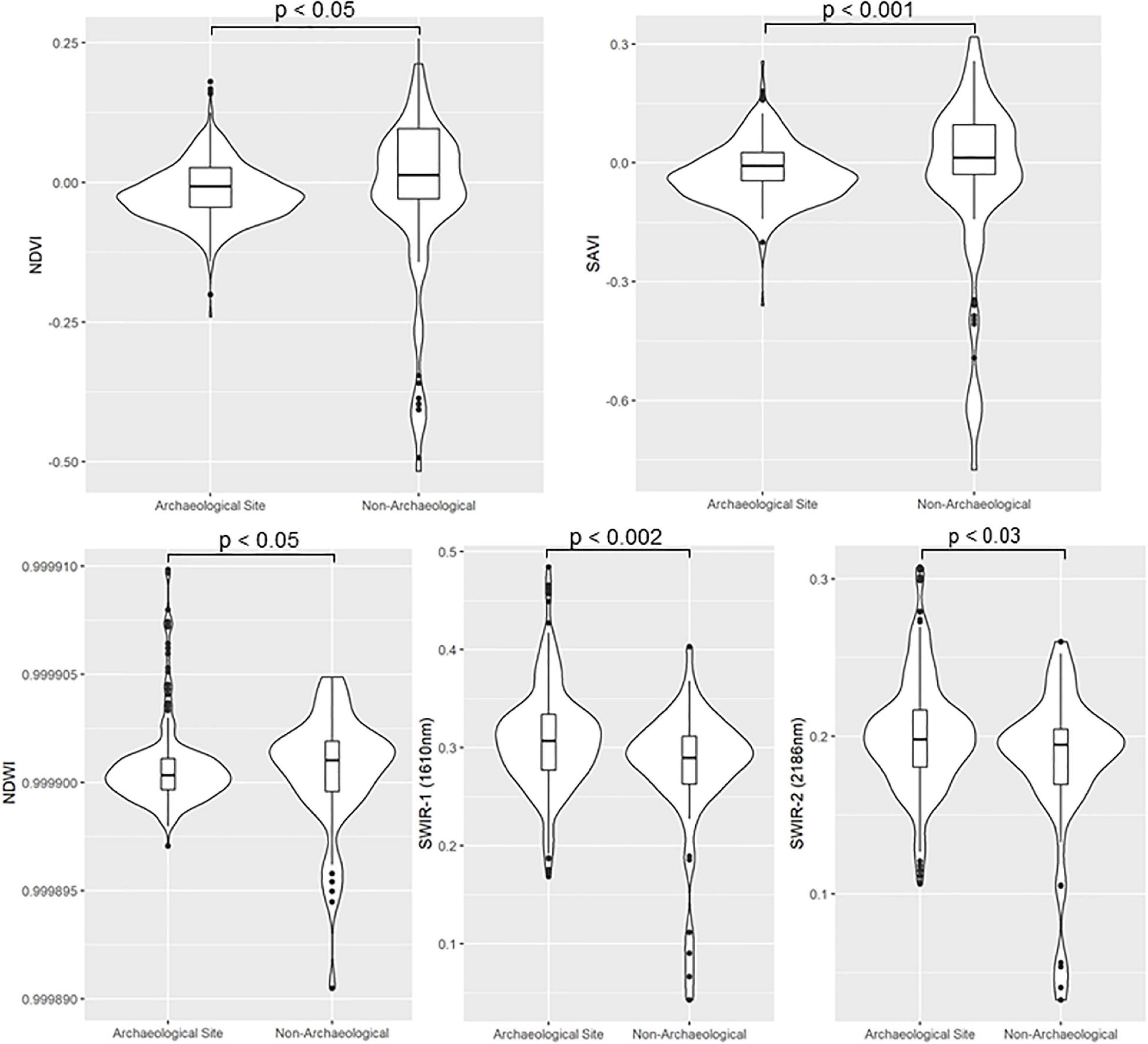
Figure 5. Vegetation index values for archaeological and non-archaeological locations. NDVI and SAVI are annual averages over 3 years of PlanetScope imagery (Planet Team, 2020). Normalized Difference Water Index (NDWI) uses the annually averaged PlanetScope and Sentinel-2 short-wave infrared (SWIR) bands.
SWIR analysis indicates that there is a tendency for areas surrounding archaeological sites to have slightly higher reflectance values in the SWIR spectrum (SWIR1: W = 12713, p-value < 0.002; SWIR2: W = 11999, p-value = 0.024). Most archaeological deposits express higher reflectance in the SWIR spectrum, indicating mineralogical differences and vegetation that might be distinct from surrounding non-archaeological areas.
Seasonally, we find that NDVI and SAVI values during the wet season, show a significant difference (W = 8282, p < 0.02) between vegetation in archaeological and non-archaeological contexts, where archaeological sites have higher mean vegetative index values than locations without archaeological activity (mean of −0.005 and −0.008, respectively; see Supplementary Figure 2). During the dry season, however, there is no significant difference (W = 10230, p = 0.925) between locations.
Using the RF results with a threshold of 0.65, we calculated the area of all identified potential archaeological deposits within the study region. Approximately 38.6 km2 (∼17%) of the study region exhibits differences in soil and vegetative properties which are likely linked to human activities. Spatial analyses demonstrate that anthropogenic areas are clustered and non-randomly distributed throughout the landscape (p > 0.0001; Supplementary Figure 6). The density of anthropogenic areas appears highest 3–5 km inland from the coast (Figure 6), where there are known to be an abundance of seasonal freshwater ponds.
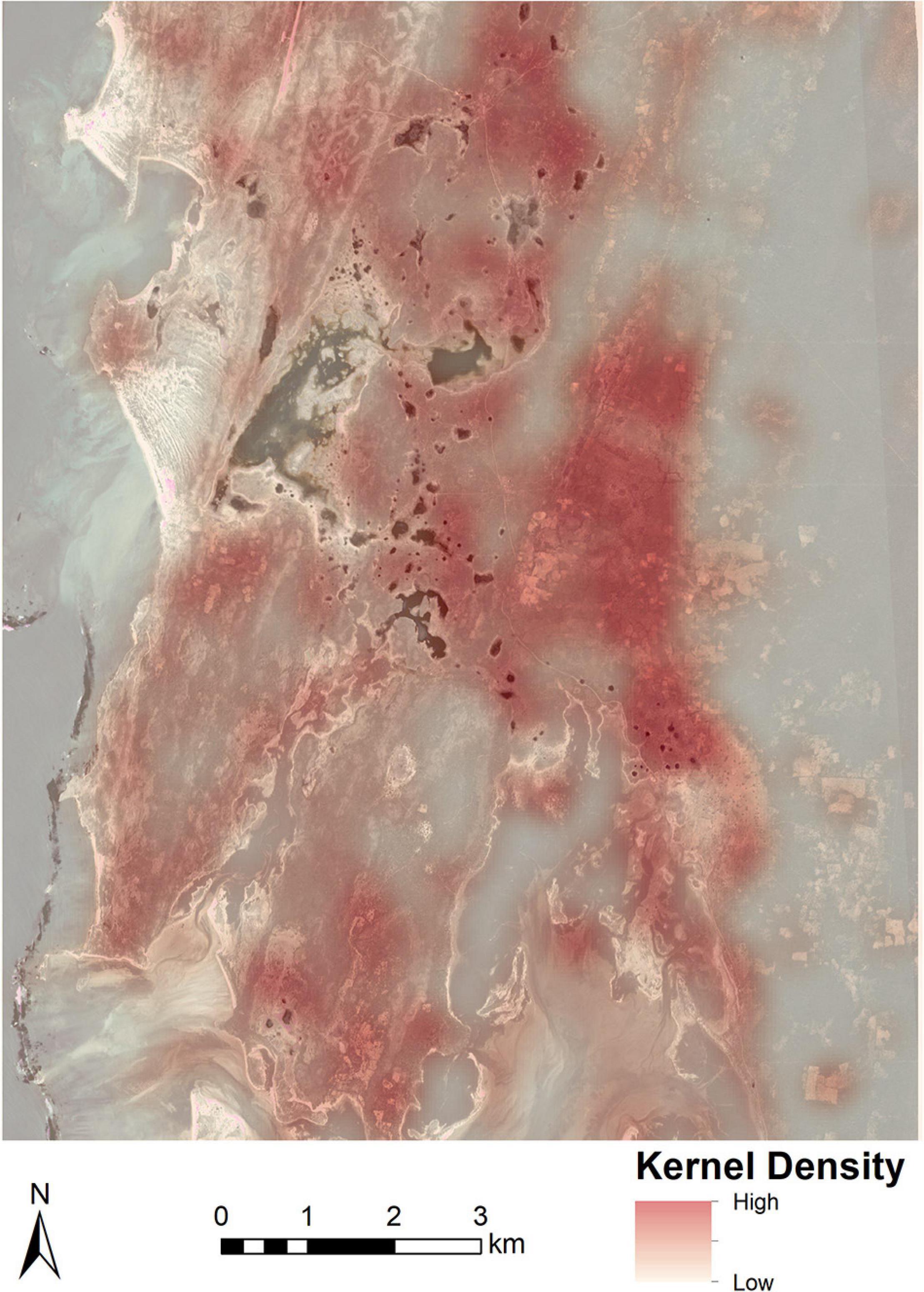
Figure 6. Density of anthropogenic modifications within the study region identified by the machine learning algorithm. Imagery © 2020 Planet Labs. Inc.
Discussion
The results of this analysis suggest that ancient coastal communities in southwest Madagascar – including highly mobile foraging and herding populations – have contributed to shaping the modern landscape in important ways. Our previous work contributed directly to this present study by allowing us to investigate settlement patterns and landscape change from two different, but complementary, angles. Our prior investigations focused on the factors that influence settlement choice (e.g., resource availability and social cohesion), while this study focuses on the long-lasting, landscape-scale effects of settlement. In fact, prior work (Davis et al., 2020a, b) indicated the presence of Allee effects, which suggests that ancient communities actively modified their ecological surroundings in ways that increased the suitability of previously settled areas. Here, we used machine learning and vegetative indices to further investigate the possibility that Allee effects were present in coastal Madagascar and resulted in legacy effects via cultural niche construction. The results of this study largely complement earlier investigations, pinpointing specific areas that contain landscape modifications, many of which overlap with previous predictive modeling results (Figure 7). Archaeological deposits exhibit significantly different spectral characteristics when compared to non-archaeological locations. While the potential of multispectral and hyperspectral imagery has long been established for archaeology, the detection of scant artifact scatters is not the norm (c.f., Orengo and Garcia-Molsosa, 2019). Rather, most literature focuses on the detection of highly visible landscape modifications, like architecture and remains of intensive agricultural activity (e.g., Tarolli et al., 2019; also see Davis, 2021). As such, this work suggests that the development of machine learning and cloud-based computational processing provides the ability to detect even the most ephemeral archaeological deposits and use these to reveal patterns of human activity and impact on the wider landscape.
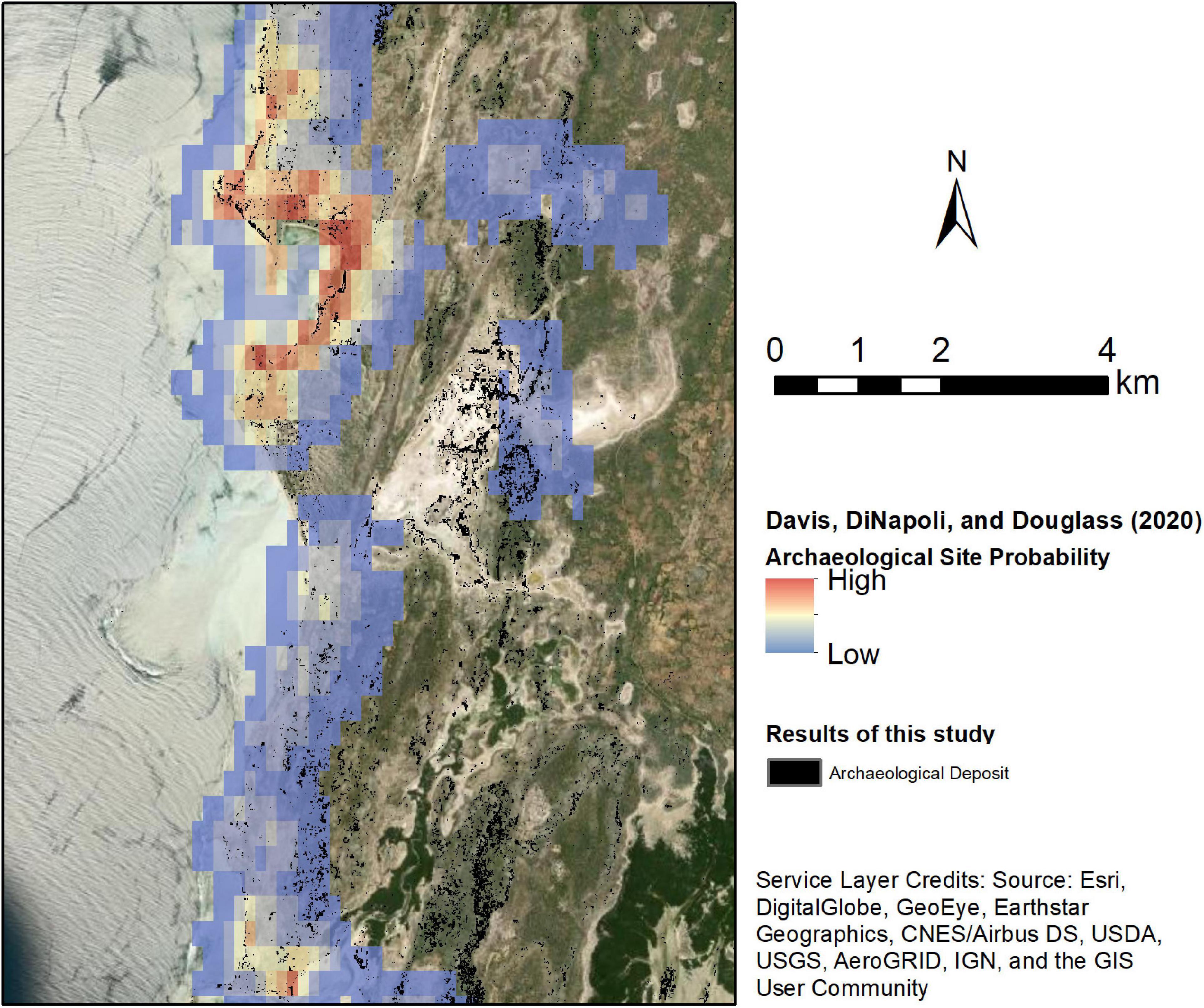
Figure 7. Comparison between predictive modeling results of Davis et al. (2020b) and this study. The previous study investigated settlement distribution via environmental and social drivers, while this study looked at long-term ecological effects of settlement activity. Notice that both models detect or predict archaeological activity in many of the same locations. The areas ranked with the highest likelihood by Davis et al. (2020b) also contain some of the oldest recorded evidence of human occupation in this region (∼2,500–3,000 cal year B.P.).
Our analysis shows that the overall health and abundance of extant vegetation (defined by vegetative index scores) on and around archaeological deposits exhibits a statistically significant different when compared to areas lacking any archaeological materials. The exact difference is not clear, however, as assessments of median values suggest vegetative values are higher in non-archaeological locations while evaluations of mean values suggest that vegetative values are higher in archaeological locations. The contrast between these two averages may be indicative of the wide range of environmental contexts within the study area and could suggest that non-archaeological localities exhibit wider range of environments while archaeological areas are more limited in their vegetative diversity. This, itself, could be the result of active settlement choices or the effects of human landscape modification. This hypothesis is supported by Figure 5, which shows a larger range of values among non-archaeological areas compared with archaeological locations.
The difference in vegetative index scores is statistically significant in the wet season (p = 0.01). Additionally, the SWIR wavelength displays differences in the soils around archaeological sites and non-archaeological locations, suggesting that there are underlying differences in the mineralogical composition and moisture retention properties between areas with and without archaeological surface materials. NDWI index assessments further demonstrate that areas with archaeological materials have vegetation with different water retention properties than non-archaeological or random locations. This may signal healthier vegetation, overall, or might relate to the presence of introduced taxa that are not xerophytic and thus retain more water than endemic taxa. Because this region is arid and rainfall is highly variable (Jury, 2003), even small increases in vegetation moisture content could have significant implications for human livelihoods and the biota that sustain them. This, coupled with spatial tests of identified archaeological deposits, indicates the presence of a distinctive human niche on Madagascar resulting from a variety of economic activities – ranging from foraging to pastoralism and agriculture – since the Late Holocene. The inland areas of the study area may have been preferred by pastoralists who have been present for the past several 100 years (Parker Pearson, 2010), and the anthropogenic signatures found here may therefore reflect the activities of pastoral and foraging community activities. While the precise geochemical composition of soils requires further ground-based studies, human activity has likely played a role in changing these components of the landscape.
Some caution is necessary in interpreting these results, however. While it is possible that these distinctive differences between archaeological and non-archaeological locations are due to human activities (e.g., Storozum et al., 2021), it is also possible that these soils were inherently different prior to human occupation. Our prior work demonstrates that human settlement choice is not random in this region (Davis et al., 2020a, b), and thus communities may have chosen areas already possessing specific soil and vegetation properties. To definitively establish the nature of human impact on soil chemistry, and to understand the feedbacks between soil and vegetation dynamics in this region, future studies will need to evaluate stratigraphic and geochemical changes in soil composition through time at archaeological and non-archaeological localities. This will involve ground-based survey as well as a program of radiometric dating to improve settlement chronologies.
Nonetheless, based on this analysis, we can definitively state that archaeological deposits in southwest Madagascar have distinct geophysical and vegetation profiles compared with non-archaeological locations. Likewise, the difference in vegetation between archaeological and non-archaeological areas is inherently linked to the geochemical properties of these locations, as well as to modern ecological variables. Because the primary difference between our study locations is the presence or absence of archaeological deposits, vegetative differences are likely linked to human activity (Lasaponara and Masini, 2007; Bennett et al., 2012). Furthermore, our results indicate that ephemeral archaeological deposits composed of artifact scatters can be distinguished from surrounding environments using high-resolution multispectral imagery and machine learning. Thus, we can conclude that underlying differences in geophysical properties of the landscape and vegetative composition are impacted by a legacy of human landscape use over the past several 1,000 years. The results presented here demonstrate that archaeological sites dated to as early as ca. 3,000 cal year B.P. by Douglass (2016) are identifiable based on their ecological impact. However, a robust settlement chronology for the study region is needed to investigate whether the age of archaeological deposits impacts their signature or detectability via multispectral remote sensing.
More broadly, we demonstrate how a focus on areas with “low-impact” human activities can facilitate greater understanding of the extent of human occupation and land-use on a landscape shaped by diverse socioeconomic systems and exhibiting an ephemeral archaeological record (Parker Pearson, 2010; Douglass and Zinke, 2015). The impacts of early communities of foragers on Madagascar’s diverse ecosystems is widely debated, but more evidence of the (in)direct effects of human settlement are needed (Davis et al., 2020a; Domic et al., under review; Douglass et al., 2019). The same can be said for many regions around the world where the availability and quality of archaeological data is lower for periods of time when foraging was the dominant livelihood strategy shaping landscapes (Stephens et al., 2019). By extending our focus to these understudied components we can re-evaluate and more fully appreciate the extent to which diverse peoples have modified the Earth’s environments.
A shift in research attention toward understanding the environmental impacts of small-scale and mobile subsistence communities can also fundamentally change how we approach sustainability and conservation. One of anthropology’s longstanding goals has been to understand the dynamics between human and environmental systems (Steward and Setzler, 1938; Steward, 1955; Carneiro, 1970; Davis, 2020). Long-term perspectives on human-environment dynamics offered by archaeology provide context for understanding contemporary land-use and sustainability issues. A goal of historical ecology and ecological anthropology is to derive lessons from these long-term perspectives and eco dynamics to inform present and future management decisions (Altschul et al., 2017, 2020; Rick and Sandweiss, 2020). By viewing anthropogenic landscape modifications at multiple scales and levels of intensity, as well as via a range of (in)direct impacts, we can improve our understanding of human niche construction in diverse societies, ranging from small-scale, mobile communities to large urban centers.
This paper makes contributes to Malagasy archaeology by illuminating the scale and extent of human traces on the landscape, in a way that is time and cost-effective, allowing us to lay the critical foundation needed for further work on coupled human-natural systems. The archaeological data contained within this space has implications for our understanding of human niche construction on Malagasy landscapes over time. Beyond Madagascar, this study also holds importance for studying the ecological legacies of foraging societies and detecting ephemeral archaeological sites in semi-arid environments using high resolution satellite images and machine learning techniques.
Data Availability Statement
The PlanetScope imagery analyzed for this study is proprietary and unfortunately cannot be shared. However, access to PlanetScope imagery is free for educators and researchers via Planet’s Education and Research Program: https://www.planet.com/markets/education-and-research/. The datasets generated from PlanetScope image analysis (i.e., density tests) for this study can be found in Penn State’s ScholarSphere Repository at https://doi.org/10.26207/zmsr-tc92. Data can also be replicated using GEE. Compilation of Sentinel data in GEE can also be run using the following link: https://code.earthengine.google.com/67eeee7b0e7cb49beac1237aff1f5f53 and the Random Forest analysis can be run in GEE using the following link: https://code.earthengine.google.com/99ccb498ea588d44a0b3300841163405.
Author Contributions
DD designed and performed the research. DD and KD analyzed data, wrote and revised the manuscript, and acquired the funding. Both the authors contributed to the article and approved the submitted version.
Funding
This project was supported by a National Science Foundation Dissertation Improvement Grant (BCS-2039927, to KD and DD), the National Geographic Society (NGS-77912R-21, to KD and DD), a Lewis and Clark Fund Grant from the American Philosophical Society (to DD), an Explorers Club Mamont Scholar Grant (to DD), the NASA Pennsylvania Space Grant Consortium (to DD), a Sigma Xi Grant in Aid of Research (to DD), and the Institute for Computational and Data Sciences at Penn State University (to KD and DD).
Conflict of Interest
The authors declare that the research was conducted in the absence of any commercial or financial relationships that could be construed as a potential conflict of interest.
Publisher’s Note
All claims expressed in this article are solely those of the authors and do not necessarily represent those of their affiliated organizations, or those of the publisher, the editors and the reviewers. Any product that may be evaluated in this article, or claim that may be made by its manufacturer, is not guaranteed or endorsed by the publisher.
Acknowledgments
We are indebted to the Morombe Archaeological Project (MAP) team and to the communities of Commune de Befandefa and the Velondriake Marine Protected Area. In particular, we wish to honor the tremendous contributions of the late Zafy Maharesy Dieu Donné Chrisostome, a founding member of the MAP team who led many of the archaeological surveys this study builds on. We also want to thank Juliana Algava for her help with illustrations. PlanetScope Imagery was provided through Planet’s Education and Research Program. Additional thanks to the two reviewers who provided valuable feedback on an earlier version of this article.
Supplementary Material
The Supplementary Material for this article can be found online at: https://www.frontiersin.org/articles/10.3389/fevo.2021.689399/full#supplementary-material
References
Altschul, J. H., Kintigh, K. W., Aldenderfer, M., Alonzi, E., Armit, I., Barceló, J. A., et al. (2020). Opinion: to understand how migrations affect human securities, look to the past. Proc. Natl. Acad. Sci. U.S.A. 117, 20342–20345. doi: 10.1073/pnas.2015146117
Altschul, J. H., Kintigh, K. W., Klein, T. H., Doelle, W. H., Hays-Gilpin, K. A., Herr, S. A., et al. (2017). Opinion: fostering synthesis in archaeology to advance science and benefit society. Proc. Natl. Acad. Sci. U.S.A. 114, 10999–11002. doi: 10.1073/pnas.1715950114
Andavadoaka Monthly Climate Averages (n.d.). WorldWeatherOnline.Com. Available online at: https://www.worldweatheronline.com/andavadoaka-weather/toliara/mg.aspx (accessed October 23, 2020).
Anderson, A., Clark, G., Haberle, S., Higham, T., Nowak-Kemp, M., Prendergast, A., et al. (2018). New evidence of megafaunal bone damage indicates late colonization of Madagascar. PLoS One 13:e0204368. doi: 10.1371/journal.pone.0204368
Angulo, E., Luque, G. M., Gregory, S. D., Wenzel, J. W., Bessa-Gomes, C., Berec, L., et al. (2018). Review: allee effects in social species. J. Anim. Ecol. 87, 47–58. doi: 10.1111/1365-2656.12759
Astuti, R. (1995). The People of the Sea: Identity and Decent Among the Vezo of Madagascar. Cambridge: Cambridge University Press.
Barret, J.-B. (1985). Sarodrano: Étude d’un Site Archéologique Côtier Du Sud-Ouest Malgache. Ph.D. thesis. Paris: Université de Paris.
Bennett, R., Welham, K., Hill, R. A., and Ford, A. L. J. (2012). The application of vegetation indices for the prospection of archaeological features in grass-dominated environments. Archaeol. Prospect. 19, 209–218. doi: 10.1002/arp.1429
Besairie, H. (1964). Carte Géologique de Madagascar: 1:1,000,000 (color), 3 sheets. Antananarivo: Service Geologique de Madagascar.
Bird, D. W., Bird, R. B., Codding, B. F., and Taylor, N. (2016). A landscape architecture of fire: cultural emergence and ecological pyrodiversity in Australia’s Western Desert. Curr. Anthropol. 57, S65–S79. doi: 10.1086/685763
Bivand, R., Keitt, T., and Rowlingson, B. (2019). Rgdal: Bindings for the “Geospatial” Data Abstraction Library (version R package version 1.4-4).
Bliege Bird, R., Bird, D. W., Codding, B. F., Parker, C. H., and Jones, J. H. (2008). The ‘Fire Stick Farming’ hypothesis: Australian aboriginal foraging strategies, biodiversity, and anthropogenic fire mosaics. Proc. Natl. Acad. Sci. U.S.A. 105, 14796–14801. doi: 10.1073/pnas.0804757105
Bliege Bird, R., and Bird, D. W. (2020). Climate, landscape diversity, and food sovereignty in arid australia: the firestick farming hypothesis. Am. J. Hum. Biol. 33:e23527. doi: 10.1002/ajhb.23527
Bliege Bird, R., McGuire, C., Bird, D. W., Price, M. H., Zeanah, D., and Nimmo, D. G. (2020). Fire mosaics and habitat choice in nomadic foragers. Proc. Natl. Acad. Sci. U.S.A. 117, 12904–12914. doi: 10.1073/pnas.1921709117
Burney, D. A. (1997). Tropical islands as paleoecological laboratories: gauging the consequences of human arrival. Hum. Ecol. 25, 437–457.
Burney, D. A. (1999). “Rates, patterns, and processes of landscape transformation and extinction in Madagascar,” in Extinctions in Near Time, ed. R. D. E. MacPhee (Boston, MA: Springer US), 145–164. doi: 10.1007/978-1-4757-5202-1_7
Carneiro, R. L. (1970). A theory of the origin of the state: traditional theories of state origins are considered and rejected in favor of a new ecological hypothesis. Science 169, 733–738. doi: 10.1126/science.169.3947.733
d’Oliveira Coelho, J., Anemone, R. L., and Carvalho, S. (2021). Unsupervised learning of satellite images enhances discovery of late miocene fossil sites in the urema rift, gorongosa, mozambique. PeerJ 9:e11573. doi: 10.7717/peerj.11573
Crumley, C. L. (1979). Three locational models: an epistemological assessment for anthropology and archaeology. Adv. Archaeol. Method Theory 2, 141–173.
Davis, D. S. (2017). The applicability of short-wave infrared (SWIR) imagery for archaeological landscape classification on rapa nui (Easter Island), Chile. Alpenglow 3:7.
Davis, D. S. (2020). Studying human responses to environmental change: trends and trajectories of archaeological research. Environ. Archaeol. 25, 367–380. doi: 10.1080/14614103.2019.1639338
Davis, D. S. (2021). Theoretical repositioning of automated remote sensing archaeology: shifting from features to ephemeral landscapes. J. Comp. Appl. Archaeol. 4, 94–109. doi: 10.5334/jcaa.72
Davis, D. S., Andriankaja, V., Carnat, T. L., Chrisostome, Z. M., Colombe, C., Fenomanana, F., et al. (2020a). Satellite-based remote sensing rapidly reveals extensive record of holocene coastal settlement on Madagascar. J. Archaeol. Sci. 115:105097. doi: 10.1016/j.jas.2020.105097
Davis, D. S., DiNapoli, R. J., and Douglass, K. (2020b). Integrating point process models, evolutionary ecology, and traditional knowledge improves landscape archaeology: a case from Southwest Madagascar. Geosciences 10:267. doi: 10.3390/geosciences10080287
Dewar, R. E., Radimilahy, C., Wright, H. T., Jacobs, Z., Kelly, G. O., and Berna, F. (2013). Stone tools and foraging in Northern Madagascar challenge holocene extinction models. Proc. Natl. Acad. Sci. U.S.A. 110, 12583–12588. doi: 10.1073/pnas.1306100110
Dewar, R. E., and Richard, A. F. (2007). Evolution in the hypervariable environment of Madagascar. Proc. Natl. Acad. Sci. U.S.A. 104, 13723–13727. doi: 10.1073/pnas.0704346104
Dewar, R. E. (1984). “Extinctions in Madagascar: the loss of the subfossil fauna,” in Quarternary Extinctions, eds P. S. Martin and R. G. Klein (Tuscon, AZ: University of Arizona Press), 574–593.
Douglass, K. (2016). An Archaeological Investigation of Settlement and Resource Exploitation Patterns in the Velondriake Marine Protected Area, Southwest Madagascar, ca. 900 BC to AD 1900. Ph.D. Dissertation. New Haven, CT: Yale University.
Douglass, K., Antonites, A. R., Quintana Morales, E. M., Grealy, A., Bunce, M., Bruwer, C., et al. (2018). Multi-analytical approach to zooarchaeological assemblages elucidates late holocene coastal lifeways in Southwest Madagascar. Quat. Int. 471, 111–131. doi: 10.1016/j.quaint.2017.09.019
Douglass, K., and Cooper, J. (2020). Archaeology, environmental justice, and climate change on islands of the Caribbean and Southwestern Indian Ocean. Proc. Natl. Acad. Sci. U.S.A. 117, 8254–8262. doi: 10.1073/pnas.1914211117
Douglass, K., Hixon, S., Wright, H. T., Godfrey, L. R., Crowley, B. E., Manjakahery, B., et al. (2019). A critical review of radiocarbon dates clarifies the human settlement of Madagascar. Quat. Sci. Rev. 221:105878. doi: 10.1016/j.quascirev.2019.105878
Douglass, K., and Rasolondrainy, T. (2021). Social memory and niche construction in a hypervariable environment. Am. J. Hum. Biol. 33:e23557. doi: 10.1002/ajhb.23557
Douglass, K., and Zinke, J. (2015). Forging ahead by land and by sea: archaeology and paleoclimate reconstruction in Madagascar. Afr. Archaeol. Rev. 32, 267–299. doi: 10.1007/s10437-015-9188-5
Fretwell, S. D., and Lucas, H. L. (1969). On territorial behavior and other factors influencing habitat distribution in birds: I. theoretical development. Acta Biotheor. 19, 16–36. doi: 10.1007/BF01601953
Fuentes, A. (2016). The extended evolutionary synthesis, ethnography, and the human niche: toward an integrated anthropology. Curr. Anthropol. 57, S13–S26. doi: 10.1086/685684
Gao, B. C. (1996). NDWI—a normalized difference water index for remote sensing of vegetation liquid water from space. Remote Sens. Environ. 58, 257–266. doi: 10.1016/s0034-4257(96)00067-3
Garzelli, A., Nencini, F., Alparone, L., Aiazzi, B., and Baronti, S. (2004). “Pan-sharpening of multispectral images: a critical review and comparison,” in Proceedings of the IGARSS 2004: 2004 IEEE International Geoscience and Remote Sensing Symposium, Anchorage, AK. doi: 10.1109/IGARSS.2004.1368950
Gautier, L., and Goodman, S. M. (2003). “Introduction to the flora of Madagascar,” in The Natural History of Madagascar, eds S. M. Goodman and J. P. Benstead (Chicago, IL: University of Chicago Press), 221–250.
Getis, A., and Ord, J. K. (1992). The analysis of spatial association by use of distance statistics. Geogr. Anal. Adv. Spat. Sci. 24, 189–206. doi: 10.1007/978-3-642-01976-0_10
Godfrey, L. R., and Douglass, K. (in press). “New insights into the relationship between human ecological pressures and the vertebrate extinctions,” in The New Natural History of Madagascar, ed. S. M. Goodman (Princeton, NJ: Princeton University Press).
Godfrey, L. R., Scroxton, N., Crowley, B. E., Burns, S. J., Sutherland, M. R., Pérez, V. R., et al. (2019). A new interpretation of Madagascar’s megafaunal decline: the ‘subsistence shift hypothesis. J. Hum. Evol. 130, 126–140. doi: 10.1016/j.jhevol.2019.03.002
Gorelick, N., Hancher, M., Dixon, M., Ilyushchenko, S., Thau, D., and Moore, R. (2017). Google earth engine: planetary-scale geospatial analysis for everyone. Remote Sens. Environ. 202, 18–27. doi: 10.1016/j.rse.2017.06.031
Hansford, J. P., Wright, P. C., Pérez, V. R., Muldoon, K. M., Turvey, S. T., and Godfrey, L. R. (2020). Evidence for early human arrival in Madagascar is robust: a response to mitchell. J. Isl. Coast. Archaeol. 15, 1–7. doi: 10.1080/15564894.2020.1771482
Heckenberger, M., and Neves, E. (2009). Amazonian archaeology. Annu. Rev. Anthropol. 38, 251–266. doi: 10.1146/annurev-anthro-091908-164310
Hijmans, R. J. (2019). Raster: Geographic Data Analysis and Modeling (version R package version 2.8-19).
Hixon, S. W., Douglass, K. G., Crowley, B. E., Rakotozafy, L. M. A., Clark, G., Anderson, A., et al. (2021). Late holocene spread of pastoralism coincides with endemic megafaunal extinction in Madagascar. Proc. R. Soc. B 288:20211204. doi: 10.1098/rspb.2021.1204
Hixon, S. W., Elliott Smith, E. A., Crowley, B. E., Perry, G. H., Randrianasy, J., Ranaivoarisoa, J. F., et al. (2018). Nitrogen isotope (δ 15 N) patterns for amino acids in lemur bones are inconsistent with aridity driving megafaunal extinction in South-Western Madagascar. J. Quat. Sci. 33, 958–968. doi: 10.1002/jqs.3073
Huete, A. R. (1988). A soil-adjusted vegetation index (SAVI). Remote Sens. Environ. 25, 295–309. doi: 10.1016/0034-4257(88)90106-X
Ichikawa, D., and Wakamori, K. (2018). “The integrated use of landsat, sentinel-2 and planetscope satellite data for crop monitoring,” in Proceedings of the IGARSS 2018-2018 IEEE International Geoscience and Remote Sensing Symposium, Valencia (Piscataway, NJ: IEEE), 7707–7710.
Iovita, R., Braun, D. R., Douglass, M. J., Holdaway, S. J., Lin, S. C., Olszewski, D. I., et al. (n.d.). Operationalizing niche construction theory with stone tools. Evol. Anthropol. Issues News Rev. Available online at: https://doi.org/10.1002/evan.21881 (accessed January 21, 2021).
Jensen, J. R. (2007). Remote Sensing of the Environment: An Earth Resource Perspective, 2nd Edn. Upper Saddle River, NJ: Pearson Prentice Hall.
Jones, C. G., Gutiérrez, J. L., Byers, J. E., Crooks, J. A., Lambrinos, J. G., and Talley, T. S. (2010). A framework for understanding physical ecosystem engineering by organisms. Oikos 119, 1862–1869. doi: 10.1111/j.1600-0706.2010.18782.x
Jones, C. G., Lawton, J. H., and Shachak, M. (1994). “Organisms as ecosystem engineers,” in Ecosystem Management eds F. B. Samson and F. L. Knopf (New York, NY: Springer), 130–147. doi: 10.1007/978-1-4612-4018-1_14
Jury, M. R. (2003). “The climate of Madagascar,” in The Natural History of Madagascar, eds S. M. Goodman and J. P. Benstead (Chicago, IL: University of Chicago Press), 75–87.
Keeney, J., and Hickey, R. (2015). Using satellite image analysis for locating prehistoric archaeological sites in alaska’s central brooks range. J. Archaeol. Sci. Rep. 3, 80–89. doi: 10.1016/j.jasrep.2015.05.022
Kirk, S. D., Thompson, A. E., and Lippitt, C. D. (2016). Predictive modeling for site detection using remotely sensed phenological data. Adv. Archaeol. Pract. 4, 87–101. doi: 10.7183/2326-3768.4.1.87
Laland, K. N., and O’Brien, M. J. (2010). Niche construction theory and archaeology. J. Archaeol. Method Theory 17, 303–322. doi: 10.1007/s10816-010-9096-6
Lasaponara, R., and Masini, N. (2007). Detection of archaeological crop marks by using satellite quickbird multispectral imagery. J. Archaeol. Sci. 34, 214–221. doi: 10.1016/j.jas.2006.04.014
Leutner, B., Horning, N., and Schwalb-Willmann, J. (2019). RStoolbox: Tools for Remote Sensing Data Analysis (version 0.2.6).
Lightfoot, K. G., Cuthrell, R. Q., Striplen, C. J., and Hylkema, M. G. (2013). Rethinking the study of landscape management practices among hunter-gatherers in North America. Am. Antiq. 78, 285–301. doi: 10.7183/0002-7316.78.2.285
Lim, J. S., Gleason, S., Jones, W., and Church, W. (2021). Nuna nalluyuituq (the land remembers): remembering landscapes and refining methodologies through community-based remote sensing in the yukon-kuskokwim delta, Southwest Alaska. Archaeol. Prospect. Available online at: https://doi.org/10.1002/arp.1840 (accessed July 21, 2021).
Lombardo, U., and Prümers, H. (2010). Pre-columbian human occupation patterns in the eastern plains of the llanos de Moxos, Bolivian Amazonia. J. Archaeol. Sci. 37, 1875–1885. doi: 10.1016/j.jas.2010.02.011
Malley, J. D., Kruppa, J., Dasgupta, A., Malley, K. G., and Ziegler, A. (2012). Probability machines: consistent probability estimation using nonparametric learning machines. Methods Inf. Med. 51, 74–81. doi: 10.3414/ME00-01-0052
McMichael, C. H., Palace, M. W., Bush, M. B., Braswell, B., Hagen, S., Neves, E. G., et al. (2014). Predicting Pre-Columbian anthropogenic soils in Amazonia. Proc. R. Soc. B Biol. Sci. 281, 20132475. doi: 10.1098/rspb.2013.2475
Mitchell, P. (2020). Debating Madagascar: a reply to Hansford et al.’s response. J. Isl. Coast. Archaeol. 15, 603–608. doi: 10.1080/15564894.2020.1771483
Mobley, C. M., and Eldridge, M. (1992). Culturally modified trees in the Pacific Northwest. Arctic Anthropol. 29, 91–110.
Odling-Smee, F. J., Laland, K. N., and Feldman, M. W. (2003). Niche Construction: The Neglected Process in Evolution. Princeton, NJ: Princeton University Press.
Odling-Smee, J., Erwin, D. H., Palkovacs, E. P., Feldman, M. W., and Laland, K. N. (2013). Niche construction theory: a practical guide for ecologists. Q. Rev. Biol. 88, 3–28. doi: 10.1086/669266
Oliver, J. (2007). Beyond the water’s edge: towards a social archaeology of landscape on the Northwest Coast. Can. J. Archaeol. 31, 1–27.
Orengo, H. A., and Garcia-Molsosa, A. (2019). A brave new world for archaeological survey: automated machine learning-based potsherd detection using high-resolution drone imagery. J. Archaeol. Sci. 112:105013. doi: 10.1016/j.jas.2019.105013
Orengo, H. A., Conesa, F. C., Garcia-Molsosa, A., Lobo, A., Green, A. S., Madella, M., et al. (2020). Automated detection of archaeological mounds using machine-learning classification of multisensor and multitemporal satellite data. Proc. Natl. Acad. Sci. U.S.A. 117, 18240–18250. doi: 10.1073/pnas.2005583117
Parker Pearson, M. (2010). Pastoralists, Warriors and Colonists: The Archaeology of Southern Madagascar. Oxford: Archaeopress.
Planet Team (2020). Planet Application Program Interface: In Space for Life on Earth. San Francisco, CA: Planet.
Quintus, S., and Cochrane, E. E. (2018). The prevalence and importance of niche construction in agricultural development in polynesia. J. Anthropol. Archaeol. 51, 173–186. doi: 10.1016/j.jaa.2018.06.007
R Core Team (2020). R: A Language and Environment for Statistical Computing (version 4.0.2). Vienna: R Foundation for Statistical Computing.
Rakotozafy, L. M. A. (1996). Etude de La Constitution Du Régime Alimentaire Des Habitants Du Site de Mahilaka Du XIème Au XIVème Siècle à Partir Des Produits de Fouilles Archéologiques. Ph.D. thesis. Antananarivo: Université d’Antananarivo.
Razanatsoa, E., Virah-Sawmy, M., Woodborne, S., Callanan, C., and Gillson, L. (in press). Adaptation of subsistence strategies of the Southwestern Malagasy in the face of climate change. Malagasy Nat. 15, 1–31.
Rick, T. C., and Sandweiss, D. H. (2020). Archaeology, Climate, and Global Change in the Age of Humans. Proc. Natl. Acad. Sci. U.S.A. 117, 8250–8253. doi: 10.1073/pnas.2003612117
Rowley-Conwy, P., and Layton, R. (2011). Foraging and farming as niche construction: stable and unstable adaptations. Philos. Trans. R. Soc. B Biol. Sci. 366, 849–862. doi: 10.1098/rstb.2010.0307
Smith, B. D. (2001). Low-level food production. J. Archaeol. Res. 9, 1–43. doi: 10.1177/156482658500700306
Sonnemann, T., Comer, D., Patsolic, J., Megarry, W., Malatesta, E. H., and Hofman, C. (2017). Semi-automatic detection of indigenous settlement features on hispaniola through remote sensing data. Geosciences 7:127. doi: 10.3390/geosciences7040127
Spengler, R. N. (2021). Niche construction theory in archaeology: a critical review. J. Archaeol. Method Theory doi: 10.1007/s10816-021-09528-4
Stephens, L., Fuller, D., Boivin, N., Rick, T., Gauthier, N., Kay, A., et al. (2019). Archaeological assessment reveals earth’s early transformation through land use. Science 365, 897–902. doi: 10.1126/science.aax1192
Steward, J. (1955). “Smallholders, householders: farm families and the ecology of intensive, sustainable agriculture,” in Anthropological Theory: An Introductory History, eds N. Haenn and R. Wilk (New York, NY: New York University Press), 1–9.
Steward, J. H., and Setzler, F. M. (1938). Function and configuration in archaeology. Am. Antiq. 4, 4–10. doi: 10.2307/275356
Storozum, M. J., Goldstein, S. T., Contreras, D. A., Gidna, A. O., Mabulla, A. Z. P., Grillo, K. M., et al. (2021). The influence of ancient herders on soil development at Luxmanda, Mbulu Plateau, Tanzania. CATENA 204:105376. doi: 10.1016/j.catena.2021.105376
Sun, C., Bian, Y., Zhou, T., and Pan, J. (2019). Using of multi-source and multi-temporal remote sensing data improves crop-type mapping in the subtropical agriculture region. Sensors 19:2401. doi: 10.3390/s19102401
Tarolli, P., Cao, W., Sofia, G., Evans, D., and Ellis, E. C. (2019). From features to fingerprints: a general diagnostic framework for anthropogenic geomorphology. Prog. Phys. Geogr. Earth Environ. 43, 95–128. doi: 10.1177/0309133318825284
Thabeng, O. L., Merlo, S., and Adam, E. (2020). From the bottom up: assessing the spectral ability of common multispectral sensors to detect surface archaeological deposits using field spectrometry and advanced classifiers in the shashi-limpopo confluence area. Afr. Archaeol. Rev. 37, 25–49. doi: 10.1007/s10437-020-09372-z
Keywords: foraging, landscape archaeology, remote sensing, niche construction, ecological legacies, Madagascar
Citation: Davis DS and Douglass K (2021) Remote Sensing Reveals Lasting Legacies of Land-Use by Small-Scale Foraging Communities in the Southwestern Indian Ocean. Front. Ecol. Evol. 9:689399. doi: 10.3389/fevo.2021.689399
Received: 31 March 2021; Accepted: 16 August 2021;
Published: 03 September 2021.
Edited by:
Geoffrey Clark, Australian National University, AustraliaReviewed by:
Triin Reitalu, Tallinn University of Technology, EstoniaXu Chen, China University of Geosciences, China
Copyright © 2021 Davis and Douglass. This is an open-access article distributed under the terms of the Creative Commons Attribution License (CC BY). The use, distribution or reproduction in other forums is permitted, provided the original author(s) and the copyright owner(s) are credited and that the original publication in this journal is cited, in accordance with accepted academic practice. No use, distribution or reproduction is permitted which does not comply with these terms.
*Correspondence: Dylan S. Davis, dsd40@psu.edu