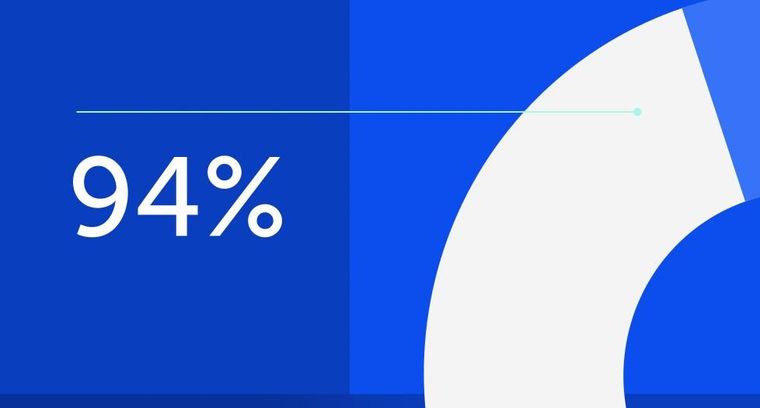
94% of researchers rate our articles as excellent or good
Learn more about the work of our research integrity team to safeguard the quality of each article we publish.
Find out more
PERSPECTIVE article
Front. Ecol. Evol., 17 December 2021
Sec. Conservation and Restoration Ecology
Volume 9 - 2021 | https://doi.org/10.3389/fevo.2021.675261
This article is part of the Research TopicConnecting the Human Dimension and Global Marine Ecosystem ServicesView all 5 articles
Populations of Atlantic salmon are crashing across most of its natural range: understanding the underlying causes and predicting these collapses in time to intervene effectively are urgent ecological and socioeconomic priorities. Current management techniques rely on phenomenological analyses of demographic population time-series and thus lack a mechanistic understanding of how and why populations may be declining. New multidisciplinary approaches are thus needed to capitalize on the long-term, large-scale population data that are currently scattered across various repositories in multiple countries, as well as marshaling additional data to understand the constraints on the life cycle and how salmon operate within the wider food web. Here, we explore how we might combine data and theory to develop the mechanistic models that we need to predict and manage responses to future change. Although we focus on Atlantic salmon—given the huge data resources that already exist for this species—the general principles developed here could be applied and extended to many other species and ecosystems.
Many of the world’s marine and inland fisheries are in a parlous state (Free et al., 2019; Food and Agriculture Organization [FAO], 2020; WWF International, 2021), and the traditional long-term focus on single stocks, population-based models and the maximum sustainable yield paradigm has failed to prevent collapses in many species across the globe (Caddy and Seijo, 2005). This is not a new problem—the United Nations Food and Agriculture Organisation (FAO) addressed global overfishing as one of its first priorities following its inception in the 1940s—but the scale and reach of these impacts is now much greater, with worrying declines of many previously dominant species. The Atlantic cod offers a salutary lesson here—it once sustained some of the most productive fisheries in the world, but overfishing pushed even these populations into collapse (Hutchings and Myers, 1994). Climate change is also a growing concern for global fish stocks, especially as it is predicted to interact with overfishing to alter the size structure and metabolic demands of both the focal species and the wider food web within which they operate (Brander, 2007). How these fundamental relationships operate across multiple scales (in space and time), organizational levels (from individuals to ecosystems), and among different genetically diverse populations and phylo-geographic groups by means of eco-genetic feedback, remains poorly understood. This lack of understanding has hindered our ability to forecast the impact of future change on fish stocks and subsequently to conserve and manage those stocks sustainably. However, we are now entering a period where we can combine new modeling approaches with extensive datasets to grapple with this challenge, and species such as the Atlantic salmon, Salmo salar (hereafter, salmon) can provide a means of advancing the field rapidly now that the foundational building blocks are within reach.
The salmon was once widespread and common on both sides of the Atlantic, but populations are now collapsing over much of its range (Soto et al., 2018; Almodóvar et al., 2019; ICES, 2020). Although there is no evidence that it faces imminent global extinction as a species, abundances are a fraction of their previous levels and local extinctions are accelerating, effectively rendering them commercially or functionally extinct in many of their previous strongholds. This species is of particular interest and concern because of its complex lifecycle—it is one of<1% of fishes that span both the marine and freshwater realms—and also because of its dual value as both a food fish (increasingly via industrial scale commercial aquaculture, as well as via direct exploitation of wild stocks) and a widespread recreational fishery important to rural economies (Butler et al., 2009; Winfield, 2016). Given that an individual salmon will move through different jurisdictional areas and food webs over its lifetime, it must run a gauntlet of multiple stressors—from the catchment to the ocean and back again—if it is to survive and reproduce (Arthington et al., 2016). If we are to forecast and manage future population change more effectively, we need to be able to understand, predict, and intervene when and where key bottlenecks arise in response to stressors that affect individuals as they grow and move through the food web (Figure 1).
Figure 1. Feeding interactions and gaps in our knowledge across the life cycle in both the marine and freshwater environment need resolving to understand population drivers for future management of Atlantic salmon. Model parameters can relate to different stages the life cycle with thermal performance curves showing the relationship between temperature and vital rates (survival and growth) across salmonids and how these may vary at each life stage (data points for Atlantic salmon are shown as solid squares). The central graphic shows the Northeast Atlantic marine food web, arranged with salmon in the center (blue star) and with species that are one, two, or three trophic links away arranged in each subsequent ring. No species in this complex food web is more than three trophic links away from salmon, highlighting the importance of the surrounding ecosystem for understanding salmon population dynamics. There are still some black boxes in our understanding of how pressures on some life stages (e.g., development of eggs in the river, survival at sea) affect population dynamics. The life cycle also presents numerous opportunities for management interventions, including egg planting, construction of salmon ladders, and altering catch-and-release quotas of returning adults.
Currently, sophisticated statistical analyses of demographic population time-series data are used to inform management policy (Massiot-Granier et al., 2014; Olmos et al., 2019, 2020), but a mechanistic framework that links resource supply through the food web to metabolic and life-history traits, and ultimately population growth, is missing (Hampton et al., 2013). Historically, fish population biologists have overlooked the wider food web and how it changes across major environmental gradients, even though we know it can be at least as important as abiotic drivers of population dynamics (Woodward et al., 2010a; Pellissier et al., 2018). The critical role of stage- and/or size-specific resource supply to (and predation on) has also been ignored in traditional population models in general Woodward et al., 2005a, 2010b; Gilljam et al., 2011; Rudolf and Rasmussen, 2013) and for salmon in particular. The consequent dearth of integrated studies that combine high-quality data with realistic models based on first principles means we still cannot effectively predict species responses to future environmental change and drivers of mortality.
Fortunately, we are now well-placed to start making these advances by building on a combination of institutional holders of fisheries data (e.g., contributors to International Council for Exploration of the Sea working groups; ICES, 2020) and new data-gathering initiatives, such as the recently established Missing Salmon Alliance (missingsalmonalliance.org). Given the huge investment in monitoring that has been conducted on both sides of the Atlantic, the salmon is arguably one of the most heavily studied of all the fishes on the planet, at least in terms of long-term and large-scale population demographics (Birnie-Gauvin et al., 2019). There are long-term time-series data on both catches and population parameters that often span many decades in focal systems, and there are even more extensive spatial snapshots across its range (Juanes et al., 2004). This means the salmon is uniquely well-positioned as a model species to understand how multiple pressures affect fish stocks and, potentially, to develop new predictive models that can forecast future change. The fact that its range covers huge gradients in latitude, temperature, biodiversity, and productivity can help us unravel the major underlying drivers that would otherwise be difficult to disentangle for other species (Verspoor et al., 2008; Miettinen et al., 2020).
Numerous key study systems are nested within such gradients, and these contain exceptionally detailed observational data in the freshwater environment—from the warmer, productive waters of the River Frome in southern England (Gregory et al., 2019), to the cold, less productive waters of the Vesturdalsá on the edge of the Arctic Circle in Northern Iceland (Antonsson and Gudjonsson, 2002), both of which are ICES index rivers for marine survival of salmon with over 40 years of population data (ICES, 2020; see Figure 2). Some of these ICES “index rivers” even have individual-based tagging and food web data, which can provide exceptional insights into how salmon operate within the wider ecosystem. At a second tier of sites, comparable data exist in the freshwater environment, but these still need to be collated and/or standardized to account for the different techniques used to sample algae, invertebrates, and fishes. In addition, archived samples are often collected in routine biomonitoring, which could also be worked up to boost the sample size and coverage beyond that offered by the first tier of core sites (Gray et al., 2014). There is also a third tier of sites with strong population time-series data, but which currently lack food web data or archived samples, which would therefore require de novo generation to plug key gaps in the environmental gradients (Woodward et al., 2010a; Pellissier et al., 2018). Building this third tier of data is more challenging and would require substantial additional funding, so it needs to be viewed as a more strategic goal that can be addressed beyond what can be achieved now with the existing data and samples in the first two tiers.
Figure 2. Map of rivers throughout the European range of Atlantic salmon with long-term population dynamics data (white stars), some of which contain additional high resolution food web data (yellow stars—Vesturdalsá in Iceland and River Frome in the United Kingdom—note this list is not exhaustive). Long-term time series of salmon population dynamics are shown for these two rivers, along with a third site in Spain (River Pas), which contains food web data that is not yet standardized across trophic compartments. The quantified food webs for the Vesturdalsá and River Frome highlight the consistent scaling of biomass flux through the food web, with trophic biomass pyramids shown in the insets (colors correspond to the major trophic groups: diatoms in green, invertebrates in blue, salmonids in red, and other fish in purple). Note that the width of the bars in the trophic pyramids equates to total biomass on a log scale. The final column illustrates the use of biomass pyramids to identify potential for a management intervention, with recent construction of a salmon ladder on the Miðfjarðará in Iceland aimed at converting the excess biomass of invertebrates above the waterfall into salmon biomass.
Despite these unique strengths in terms of the scale and scope of the empirical data, much of the key information is still dispersed across the published and gray literature (or even held as physical samples preserved in laboratory or museum archives). This has resulted in the largely piecemeal approach that has characterized the field to date, potentially obscuring the bigger picture and limiting our ability to characterize the mechanisms operating at large spatial and temporal scales. Uniting these different data sources and targeting key gaps in these large gradients in a tiered approach provides a way to make major advances faster and more effectively (Figure 3, Phase 1). Thus, although large amounts of data are available on catches, for instance, resolution of different life stages represents a much smaller subset of that vast data resource, and those that have resolved food webs are a smaller (and often different) subset still. Nonetheless, there are enough sites and data across the salmon’s range to start assembling the pieces of the jigsaw. Bringing together data in this way can facilitate the building and validation of new models that go beyond the phenomenological and site-specific forms that have dominated to date (Khalilian et al., 2010; Finley, 2011). A more general framework should dramatically improve on the currently very limited ability to predict population change under different future scenarios of management intervention or environmental change.
Figure 3. Projected timeline of prioritized actions to advance the field and build ecosystem-based predictive capacity over the next decade, with increasing emphasis on the marine phase to span the full life cycle as data and models evolve.
Our central premise is that significant steps can now be made via more joined-up collaborations that draw together the currently piecemeal data, and augmenting it with strategically targeted efforts to collect new data where key gaps exist, to build the next generation of mechanistic models. This will also require a shift in perspective from the population to the individual level, to identify bottlenecks in the life cycle, and also to scale up to the higher organizational levels to understand how the food web and wider ecosystem shape population dynamics. Ultimately, these two threads of investigation should eventually combine to provide a holistic understanding that is currently lacking, but major advances can still be made before we reach that point by tackling both ends of the problem, with the even bigger benefits from these synergies emerging later. It is not necessary to attempt the leap to a fully mechanistic individual-based food web model in one bound—that would be unfeasible at present and also unwieldy. Rather, the initial aim would be to build a mechanistic understanding of how key aspects of the food web and environmental drivers affect the key life stages that allow us to forecast responses to future change and for prioritizing management interventions in the most effective—and ecologically sound—manner.
Implementing this potential roadmap will require prioritization of both ongoing and future monitoring and modeling efforts, so they can be most effectively employed to make the biggest advances quickly that can then be built upon in the next iteration. The collection and analysis of data from the marine phase of the salmon life cycle is technically challenging (Chaput, 2012; Strøm et al., 2019). Nevertheless, several large-scale international research projects have focused on the growth and survival of salmon at sea (e.g., SALSEA (salmonatsea.com), SAMARCH (samarch.org), SeaSalar (seasalar.no)]. Combining these marine data, as they become available, with detailed parameterization of the freshwater stages of the life cycle is crucial because it is becoming increasingly apparent that the freshwater phase has consequences for success in the marine realm and the eventual return of spawning adults (Russell et al., 2012; Gregory et al., 2019). Similarly, top-down control from large marine predators can have major implications for the success of repeat spawners and thus overall viability of certain salmon populations (Lacroix, 2014), highlighting the importance of improved understanding in the marine stage of the life cycle (Figure 3, Phase 2).
Local management actions have long been a feature of conservation efforts directed at increasing salmon production during the freshwater phase, but these have often been misguided and lacked a firm evidence base to quantify their perceived benefits. The next generation of mechanistic models we need to predict and manage responses to future change requires a more complete understanding of how management actions in the freshwater stage affect marine survival, and hence the overall efficacy of conservation efforts. By concentrating on the freshwater phase of the salmon life cycle, key drivers can be identified and isolated more effectively, allowing us to build toward the mechanistic understanding from the ground up, starting with the critical early life stages. Moving beyond inference toward linking cause and effect will ultimately also require both experimentation in, and manipulation of, the natural environment (e.g., river restoration and monitoring of effects), and this is also possible in the freshwater environment. Ultimately, the aim is to have a unifying general mechanistic framework that adequately predicts the growth and production of salmon, such that management actions can be judged in the context of the whole life cycle (Figures 1, 3, Phase 3).
Every ecosystem is composed of multiple individuals, each with its own set of metabolic constraints, and those individuals are generally positioned within the food web on the basis of their body size, particularly in aquatic systems (Brown et al., 2004; Woodward et al., 2005a, 2010b). We now know that simple allometric models can predict food web structure and dynamics in these systems (Petchey et al., 2008; Woodward et al., 2010b), including freshwaters that contain salmonids at the higher trophic levels (Forseth et al., 2001; O’Gorman et al., 2017, 2019). We also know that temperature plays a strong (and predictable) role in determining the abundance and size of these predators, as well as being a major driver of many ecosystem processes (McGinnity et al., 2009; O’Gorman et al., 2016; Nicola et al., 2018). Thus, where information can be gathered on the body mass and abundance of the members of the food web—including salmon—it should be possible to construct feasible trophic networks and also models that describe their key properties. If additional information can also be garnered on environmental temperature, then this can be incorporated to start to understand how (and at what rates) biomass and energy move between these nodes of different species and size classes. This provides the “trophic backbone” of the system and allows salmon to be seen as a part of these higher organizational levels. It is also important for identifying deviations from the general pattern and potential drivers, which can then help in prioritizing (and testing) future management interventions. For instance, if the slope of the mass-abundance scaling relationship is steeper than predicted by theory, this could imply that the ecosystem is under stress and that less biomass is moving from the base of the web into the higher trophic levels, including salmon (see O’Gorman et al., 2017). Similarly, a truncated size spectrum could provide another indicator that the system is under stress, resulting in reduced salmon population biomass, as large species high in the web are already rare and especially vulnerable to perturbations (Jennings and Blanchard, 2004).
It is important to note that we are not arguing that temperature is the sole variable of interest, but rather that it is a master variable, so that once it is accounted for, the residual variation due to other pressures is easier to identify and isolate (Jones et al., 2017). Another key advantage of such an approach is that it effectively involves “taxon-free” metrics that avoid the confounding contingencies of community ecology and biogeography within particular sites. For instance, the scaling and structure of the food web or size spectrum should follow similar rules, largely irrespective of the names of the species involved (e.g., Gilljam et al., 2011). This helps us escape the spatial confounds that would otherwise prevent us from seeing the bigger picture, and which have contributed to the piecemeal approach used to date.
Once this general backbone is in place, it can then be used to understand what is driving local differences that deviate from the underlying general pattern. The converse approach of trying to build from phenomenological inferences from specific case studies is unlikely to succeed and has not done so to date, largely because it is inevitably bedeviled with contingencies and the lack of a unifying general mechanistic framework. An example of the potential for these types of allometric and metabolic approaches being used to understand what shapes the abundance and biomass of fishes can be drawn from recent work on the brown trout (Salmo trutta)—a close relative of the Atlantic salmon and one that often shares the same prey and predators within the food web—and how it responds to gradients of land use change in the United Kingdom (Perkins et al., 2018) and temperature in Iceland (O’Gorman et al., 2016). Brown trout often share the same anadromous life cycle as salmon, with individuals migrating to the sea before returning to spawn in their natal rivers, although populations of brown trout that remain resident in the river throughout their life cycle are also common (Elliott, 1994). Given their similar taxonomy and position in the food web, there is every reason to expect that salmon can also be understood by employing similar tools—especially because we now have access to even larger datasets across even wider gradients than in these initial studies focused on brown trout.
Indeed, recent pilot studies in Iceland have shown that riverine food webs containing salmon do indeed follow the same general rules that are seen elsewhere, such as the highly size-structured and thus quantifiably predictable flow of energy from small, abundant resources to large, rare predators (e.g., Thompson et al., 2016; Perkins et al., 2018; see Figure 2). Intriguingly, these have offered new glimpses of how we might assess the potential biomass that a system could support but which currently lacks salmon (e.g., the “free space” in the trophic pyramid in headwaters above a waterfall barrier; see Figure 2). These types of data could be used more generally to test hypotheses about the capacity of food webs to hold salmon, by deploying often routine management interventions (e.g., relocation, supplementation, or habitat restoration) as Before-After-Control-Impact (BACI) style “field experiments” (Green, 1979; Thompson et al., 2018). For instance, the modeling framework and food web data could be used to prioritize where management interventions should be targeted, by identifying bottlenecks, and for testing hypotheses about what the response should be to those interventions. This then feeds back into the next rounds of model development and targeted data-gathering as they are challenged with more and increasingly robust “experimental” data. Embedding a more formalized and co-ordinated prediction-testing mode could help move management practices beyond a reliance on simple correlational time series by strengthening the evidence base with a more mechanistic understanding.
Given the rationale for an individual-to-ecosystem approach, the next obvious step will be to prioritize how best to collate existing data and where to target new data acquisition—both in terms of selecting the best geographical locations for site selection and spanning the major environmental gradients of temperature, biodiversity, and productivity as a first step and then filling in the gaps to resolve and refine the data and models iteratively. If this were an experimental study, it would be akin to making planned contrasts that can control as many variables as possible whilst manipulating the major axis of interest—but when dealing with observations in the real world this needs to be tempered by capitalizing on existing time-series data. As such, this idealized design will inevitably need to be adapted pragmatically and examples of potential candidate sites on the European seaboard that could be targeted for generating the first rounds of data and models are highlighted in Figure 2, from Spain to the Arctic. These sites already have broadly comparable data on salmon life-stages as well as data or archived samples for the wider food web, which could be used to facilitate building and testing of the metabolic-based population models and assembling well-resolved food webs (Figure 3, Phase 1).
Food web data can be time-consuming to collect (Gray et al., 2014), so in the first instance, biomass and abundance data from basal resources to higher consumers could be used to build coarse trophic biomass and abundance pyramids (Trebilco et al., 2013; see Figure 2). Ultimately, additional data collation and sampling effort could yield more sophisticated measures, such as size spectra (Jennings and Blanchard, 2004), or “trivariate food webs,” whereby the network of feeding links is overlain on an X-Y plot of body mass and abundance for each species, from diatoms to fishes (Cohen et al., 2003; Woodward et al., 2005a,b; see Figure 2). These more highly resolved data could provide deeper insights into the flux of energy and biomass through particular species and size classes (including salmon) within the food web. They may also help to anticipate the indirect effects of fish competitors, such as the Pacific pink salmon that have recently become a prominent invader in European waters (Armstrong et al., 2018; Mo et al., 2018; Sandlund et al., 2019). The use of body size and temperature data and how they combine to shape individual metabolic demands and energy flux through the ecosystem lies at the core of both the theory and the data that will be needed and can provide an overarching conceptual framework.
Classical Matrix Population Models (MPMs) use empirical data on fecundities and probabilities of survival of successive life stages in a “transition matrix” to project the growth or decline of a population in discrete life-stage categories (Caswell, 2000). A new class of MPM can be developed by expanding the matrix to include the body size of salmon at each life stage, thus enabling the use of Ecological Metabolic Theory (EMT) to link body size and thermal physiology to fundamental life-history traits (Brown et al., 2004; Figure 1). Predictions from metabolic theory can then be nested inside each cell of the expanded matrix to parameterize survival, growth, and fecundity mechanistically as a function of temperature. For example, we already know that salmon growth rate differs with temperature across its life cycle in the absence of predation or resource supply constraints (Nicieza and Metcalfe, 1997; Handeland et al., 2008; Jonsson, 2012; Figure 1), and this allows us to predict the time spent in different life-history stages as well as stage-specific intrinsic mortality. Food web theory can then start to be incorporated into such a Metabolic MPM by altering growth rates via restricted resource supply and survival via predation on different life stages, to model the consequences for population abundances across systems. Ultimately, such a mechanistic stage- and size-structured population model for salmon could then be developed to predict population dynamics for multiple sites across its range, and to forecast responses within sites to future change.
Such a general mechanistic framework goes far beyond classical MPMs, which still rely on detailed parameterization using locally collected data (Kendall et al., 2019). Current models may be able to predict abundances in short time-frames in specific systems, but they lack a mechanistic basis, so they cannot address changes in population dynamics under different (and future) scenarios (Smallegange et al., 2017). Conventional MPMs can only capture population dynamics within the time window that the life-history parameters are measured, but a Metabolic MPM can overcome this by allowing parameters to change over time as a function of body size and temperature using EMT. Model parameters could also be collated or collected in a coordinated approach to allow data from across the species range to be useful for parameterization and the resulting model(s) could be used to explore the relative importance of management interventions that target particular parts of the life cycle in freshwater and marine environments for sustaining stocks, by partitioning life stages in both realms based upon metabolic and resource supply constraints.
Although marine mortality is often cited as a major concern for salmon stocks, many studies show that body size at migration from the river ultimately determines survival rates at sea (Russell et al., 2012; Gregory et al., 2019). Consequently, the freshwater and marine phases are intimately coupled through metabolic and food web constraints—different marine survival rates can be simulated to gauge how much survival is needed at sea to maintain a viable population. Some recent European salmonid declines have indeed been attributed to marine mortality, with changing sea temperatures and reduced resource availability invoked as leading candidates (Todd et al., 2011; Oke et al., 2020; Olmos et al., 2020): although the empirical data are still sparse, ongoing initiatives like SAMARCH will increasingly shed light on this part of the life cycle, allowing the next generations of models and data to be refined and improved iteratively (Figure 3, Phase 2).
These new models can be parameterized by combining existing data from focal sites such as those highlighted by yellow stars in Figure 2, plus new data gathered at key junctures in these large-scale gradients. For instance, building new food webs in a subset of sites (e.g., white stars in Figure 2 highlight some candidate sites) would enable highly resolved long-term population data to be matched to data describing the wider trophic network, just as is already available for the first tier of other focal sites. The food web approaches envisaged have already been road-tested and published for > 100 other ecosystems (e.g., Woodward et al., 2005b, 2012; Layer et al., 2010, 2011; O’Gorman and Emmerson, 2010; Ledger et al., 2013; Perkins et al., 2018; O’Gorman et al., 2019), and employing individual size-based data in this way will enable salmonids to be tracked as they grow and move through the food web. Although often seemingly complex, these trophic networks are governed by relatively simple rules: even in the most biodiverse food webs in the current catalog, with>100 species and>1,000 feeding links (e.g., United Kingdom chalk rivers, as well as the NE Atlantic food web), salmon sit within just three trophic links of every other species (Figure 1). They are even more tightly coupled in the simpler freshwater systems than in the marine realm, and in Icelandic rivers salmon are separated by just two links from every other species. As such, changes in abundance of their prey or predators can ripple through the food web rapidly, and this can have strong effects on the stage-specific development, fecundity, mortality, and ultimately population dynamics of the salmon.
Strategically enriching existing data with new focal sites, particularly from rivers where stock data are available (e.g., ICES, 2020), would enable new food web data to be mapped onto extensive population-level time-series data as efficiently as possible, and would inform a mechanistic modeling framework that could be used to explore new or future scenarios. This would be a huge undertaking if starting from scratch, but by capitalizing on the many decades of work done to date it represents an attainable goal that involves (relatively) little financial outlay. Generating such data would give unprecedented breadth and depth for testing hypotheses across the broadest possible scale, over potentially hundreds of rivers, and beyond what is available for almost any other wild species.
Looking further ahead, we anticipate the ability to embed Bayesian approaches in such models for a more rigorous comparison to empirical data. For instance, the mizer package in R would enable multi-species, trait-based, and community size spectrum modeling (Scott et al., 2013), potentially allowing changes in food-web structure to be projected along broadscale environmental gradients, in addition to changes in salmon population dynamics (Spence et al., 2016; Reum et al., 2019; Woodworth-Jefcoats et al., 2019). Future change and management scenarios related to natural and anthropogenic drivers could be exploited by adjusting key life stage parameter values to forecast the potential success of different management interventions, which could be validated against real-world conservation efforts, such as planting salmon eggs in artificial gravel reds (i.e., spawning nests), building salmon ladders to give access to upstream habitat (Figure 2), and altering catch-release quotas of returning adults. Empirically grounding the modeling of conservation measures in this way can help prioritize and evaluate conservation efforts and expenditure more efficiently, and by using more standardized approaches and general frameworks we can start to leverage the (largely unexploited) statistical power of these “experiments” being done at large scales (Figure 3, Phase 3).
These approaches in combination will be beneficial for informing future policy and management: for Atlantic salmon, and similar taxa, we need to start considering not just the focal species but also the wider food web and ecosystems that support it. Focusing on a species in isolation will never be able to reveal its full population behavior and dynamics, so we need a more ecosystem-based approach to management that incorporates food web interactions and considers differences across locations (National Marine Fisheries Service [NMFS], 2016) and among evolutionarily distinct populations (Verspoor et al., 2008). This is especially important for a species whose range not only spans jurisdictions but also different aquatic realms (marine and freshwater) and ecosystems—which will themselves be under many different pressures (Arthington et al., 2016). The integration of science into management and policy requires the coordination of existing efforts as well as the direction of new research to fill the knowledge gaps, but much of this can be done via investment in and alignment with both established and emerging networks of researchers and stakeholders. Collating and disseminating scientific advances in the field will be vital to ensure a thorough understanding of a species and its environment, essentially leading to more effective policy surrounding the species. For salmon in particular, we can enhance the long-term datasets and biomonitoring by targeting the insertion of the key information we are lacking on the life cycle and the surrounding food web, to design policy and interventions that consider both the freshwater and marine environment and any future changes within them (Figures 1–3). Developing this more holistic approach moves us closer to the more mechanistic understanding that will allow better forecasting of future change and, by extension, more effective management.
GW produced a first draft of the manuscript. OM and EO’G played a key role in editing the final version. All authors contributed to the writing of the manuscript.
OM was supported by the Falkathing, as part of the Icelandic Six Rivers Project.
The authors declare that the research was conducted in the absence of any commercial or financial relationships that could be construed as a potential conflict of interest.
All claims expressed in this article are solely those of the authors and do not necessarily represent those of their affiliated organizations, or those of the publisher, the editors and the reviewers. Any product that may be evaluated in this article, or claim that may be made by its manufacturer, is not guaranteed or endorsed by the publisher.
Almodóvar, A., Ayllón, D., Nicola, G. G., Jonsson, B., and Elvira, B. (2019). Climate-driven biophysical changes in feeding and breeding environments explain the decline of southernmost European Atlantic salmon populations. Can. J. Fish. Aquat. Sci. 76, 1581–1595. doi: 10.1139/cjfas-2018-0297
Antonsson, T., and Gudjonsson, S. (2002). Variability in timing and characteristics of Atlantic salmon smolt in Icelandic rivers. Trans. Am. Fish. Soc. 131, 643–655. doi: 10.1577/1548-8659(2002)131<0643:VITACO>2.0.CO;2
Armstrong, J. D., Bean, C. W., and Wells, A. (2018). The Scottish invasion of pink salmon in 2017. J. Fish Biol. 93, 8–11. doi: 10.1111/jfb.13680
Arthington, A. H., Dulvy, N. K., Gladstone, W., and Winfield, I. J. (2016). Fish conservation in freshwater and marine realms: status, threats and management. Aquat. Conserv. Mar. Freshw. Ecosyst. 26, 838–857. doi: 10.1002/aqc.2712
Birnie-Gauvin, K., Thorstad, E. B., and Aarestrup, K. (2019). Overlooked aspects of the Salmo salar and Salmo trutta lifecycles. Rev. Fish Biol. Fish. 29, 749–766. doi: 10.1007/s11160-019-09575-x
Brander, K. M. (2007). Global fish production and climate change. Proc. Natl. Acad. Sci. U.S.A. 104, 19709–19714. doi: 10.1073/pnas.0702059104
Brown, J. H., Gillooly, J. F., Allen, A. P., Savage, V. M., and West, G. B. (2004). Toward a metabolic theory of ecology. Ecology 85, 1771–1789. doi: 10.1890/03-9000
Butler, J. R., Radford, A., Riddington, G., and Laughton, R. (2009). Evaluating an ecosystem service provided by Atlantic salmon, sea trout and other fish species in the River Spey, Scotland: the economic impact of recreational rod fisheries. Fish. Res. 96, 259–266. doi: 10.1016/j.fishres.2008.12.006
Caddy, J. F., and Seijo, J. C. (2005). This is more difficult than we thought! The responsibility of scientists, managers and stakeholders to mitigate the unsustainability of marine fisheries. Philos. Trans. R. Soc. Lond. B Biol. Sci. 360, 59–75. doi: 10.1098/rstb.2004.1567
Chaput, G. (2012). Overview of the status of Atlantic salmon (Salmo salar) in the North Atlantic and trends in marine mortality. ICES J. Mar. Sci. 69, 1538–1548. doi: 10.1093/icesjms/fss013
Cohen, J. E., Jonsson, T., and Carpenter, S. R. (2003). Ecological community description using the food web, species abundance, and body size. Proc. Natl. Acad. Sci. U.S.A. 100, 1781–1786. doi: 10.1073/pnas.232715699
Finley, C. (2011). All the Fish in the Sea: Maximum Sustainable Yield and the Failure of Fisheries Management. Chicago, IL: University of Chicago Press. doi: 10.7208/chicago/9780226249681.001.0001
Food and Agriculture Organization [FAO] (2020). The State of World Fisheries and Aquaculture 2020. Sustainability in action. Rome: FAO. doi: 10.4060/ca9229en
Forseth, T., Hurley, M. A., Jensen, A. J., and Elliott, J. M. (2001). Functional models for growth and food consumption of Atlantic salmon parr, Salmo salar, from a Norwegian river. Freshw. Biol. 46, 173–186. doi: 10.1046/j.1365-2427.2001.00631.x
Free, C. M., Thorson, J. T., Pinsky, M. L., Oken, K. L., Wiedenmann, J., and Jensen, O. P. (2019). Impacts of historical warming on marine fisheries production. Science 363, 979–983. doi: 10.1126/science.aau1758
Gilljam, D., Thierry, A., Edwards, F. K., Figueroa, D., Ibbotson, A. T., Jones, J. I., et al. (2011). Seeing double:: size-based and taxonomic views of food web structure. Adv. Ecol. Res. 45, 67–133. doi: 10.1016/B978-0-12-386475-8.00003-4
Gray, C., Baird, D. J., Baumgartner, S., Jacob, U., Jenkins, G. B., O’Gorman, E. J., et al. (2014). Ecological networks: the missing links in biomonitoring science. J. Appl. Ecol. 51, 1444–1449. doi: 10.1111/1365-2664.12300
Green, R. H. (1979). Sampling Design and Statistical Methods for Environmental Biologists. Hoboken, NJ: John Wiley & Sons.
Gregory, S. D., Ibbotson, A. T., Riley, W. D., Nevoux, M., Lauridsen, R. B., Russell, I. C., et al. (2019). Atlantic salmon return rate increases with smolt length. ICES J. Mar. Sci. 76, 1702–1712. doi: 10.1093/icesjms/fsz066
Hampton, S. E., Strasser, C. A., Tewksbury, J. J., Gram, W. K., Budden, A. E., Batcheller, A. L., et al. (2013). Big data and the future of ecology. Front. Ecol. Environ. 11:156–162. doi: 10.1890/120103
Handeland, S. O., Imsland, A. K., and Stefansson, S. O. (2008). The effect of temperature and fish size on growth, feed intake, food conversion efficiency and stomach evacuation rate of Atlantic salmon post-smolts. Aquaculture 283, 36–42. doi: 10.1016/j.aquaculture.2008.06.042
Hutchings, J. A., and Myers, R. A. (1994). What can be learned from the collapse of a renewable resource? Atlantic cod, Gadus morhua, of Newfoundland and Labrador. Can. J. Fish. Aquat. Sci. 51, 2126–2146. doi: 10.1139/f94-214
ICES. (2020). Working group on North Atlantic Salmon (WGNAS). ICES Sci. Rep. 2:358. doi: 10.17895/ices.pub.5973
Jennings, S., and Blanchard, J. L. (2004). Fish abundance with no fishing: predictions based on macroecological theory. J. Anim. Ecol. 73, 632–642. doi: 10.1111/j.0021-8790.2004.00839.x
Jones, L. A., Muhlfeld, C. C., and Hauer, F. R. (2017). “Chapter 6 – Temperature,” in Methods in Stream Ecology, 3rd Edn, Vol. Volume 1, eds F. R. Hauer and G. A. Lamberti (Cambridge, MA: Academic Press), 109–120. doi: 10.1016/B978-0-12-416558-8.00006-8
Jonsson, A. G. F. B. (2012). Effect of incubation temperature on growth performance in Atlantic salmon. Mar. Ecol. Prog. Ser. 454, 75–82. doi: 10.3354/meps09643
Juanes, F., Gephard, S., and Beland, K. F. (2004). Long-term changes in migration timing of adult Atlantic salmon (Salmo salar) at the southern edge of the species distribution. Can. J. Fish. Aquat. Sci. 61, 2392–2400. doi: 10.1139/f04-207
Kendall, B. E., Fujiwara, M., Diaz-Lopez, J., Schneider, S., Voigt, J., and Wiesner, S. (2019). Persistent problems in the construction of matrix population models. Ecol. Modell. 406, 33–43. doi: 10.1016/j.ecolmodel.2019.03.011
Khalilian, S., Froese, R., Proelss, A., and Requate, T. (2010). Designed for failure: a critique of the common fisheries policy of the European Union. Mar. Policy 34, 1178–1182. doi: 10.1016/j.marpol.2010.04.001
Lacroix, G. L. (2014). Large pelagic predators could jeopardize the recovery of endangered Atlantic salmon. Can. J. Fish. Aquat. Sci. 71, 343–350. doi: 10.1139/cjfas-2013-0458
Layer, K., Hildrew, A. G., Jenkins, G. B., Riede, J. O., Rossiter, S. J., Townsend, C. R., et al. (2011). Long-term dynamics of a well-characterised food web: four decades of acidification and recovery in the Broadstone Stream model system. Adv. Ecol. Res. 44, 69–117. doi: 10.1016/B978-0-12-374794-5.00002-X
Layer, K., Riede, J. O., Hildrew, A. G., and Woodward, G. (2010). Food web structure and stability in 20 streams across a wide ph gradient. Adv. Ecol. Res. 42, 265–299. doi: 10.1016/B978-0-12-381363-3.00005-8
Ledger, M. E., Brown, L. E., Edwards, F. K., Milner, A. M., and Woodward, G. (2013). Drought alters the structure and functioning of complex food webs. Nat. Clim. Change 3, 223–227. doi: 10.1038/nclimate1684
Massiot-Granier, F., Prévost, E., Chaput, G., Potter, T., Smith, G., White, J., et al. (2014). Embedding stock assessment within an integrated hierarchical Bayesian life cycle modelling framework: an application to Atlantic salmon in the Northeast Atlantic. ICES J. Mar. Sci. 71, 1653–1670. doi: 10.1093/icesjms/fst240
McGinnity, P., Jennings, E., DeEyto, E., Allott, N., Samuelsson, P., Rogan, G., et al. (2009). Impact of naturally spawning captive-bred Atlantic salmon on wild populations: depressed recruitment and increased risk of climate-mediated extinction. Proc. R. Soc. B Biol. Sci. 276, 3601–3610. doi: 10.1098/rspb.2009.0799
Miettinen, A., Palm, S., Dannewitz, J., Lind, E., Primmer, C. R., Romakkaniemi, A., et al. (2020). A large wild salmon stock shows genetic and life history differentiation within, but not between, rivers. Conserv. Genet. 22, 35–51. doi: 10.1007/s10592-020-01317-y
Mo, T. A., Thorstad, E. B., Sandlund, O. T., Berntsen, H. H., Fiske, P., and Uglem, I. (2018). The pink salmon invasion: a Norwegian perspective. J. Fish Biol. 93, 5–7. doi: 10.1111/jfb.13682
National Marine Fisheries Service [NMFS] (2016). National Marine Fisheries Service Policy Directive – Ecosystem-Based Fisheries Management Policy. Silver Spring, MD: National Marine Fisheries Service Policy Directive.
Nicieza, A. G., and Metcalfe, N. B. (1997). Growth compensation in juvenile Atlantic salmon: responses to depressed temperature and food availability. Ecology 78, 2385–2400. doi: 10.1890/0012-9658(1997)078[2385:GCIJAS]2.0.CO;2
Nicola, G. G., Elvira, B., Jonsson, B., Ayllón, D., and Almodóvar, A. (2018). Local and global climatic drivers of Atlantic salmon decline in southern Europe. Fish. Res. 198, 78–85. doi: 10.1016/j.fishres.2017.10.012
O’Gorman, E. J., Ólafsson, ÓP., Demars, B. O., Friberg, N., Guðbergsson, G., Hannesdóttir, E. R., et al. (2016). Temperature effects on fish production across a natural thermal gradient. Glob. Change Biol. 22, 3206–3220. doi: 10.1111/gcb.13233
O’Gorman, E. J., Petchey, O. L., Faulkner, K. J., Gallo, B., Gordon, T. A., Neto-Cerejeira, J., et al. (2019). A simple model predicts how warming simplifies wild food webs. Nat. Clim. Change 9, 611–616. doi: 10.1038/s41558-019-0513-x
O’Gorman, E. J., Zhao, L., Pichler, D. E., Adams, G., Friberg, N., Rall, B. C., et al. (2017). Unexpected changes in community size structure in a natural warming experiment. Nat. Clim. Change 7, 659–663. doi: 10.1038/nclimate3368
O’Gorman, E. J., and Emmerson, M. C. (2010). Manipulating interaction strengths and the consequences for trivariate patterns in a marine food web. Adv. Ecol. Res0 42, 301–419. doi: 10.1016/B978-0-12-381363-3.00006-X
Oke, K. B., Cunningham, C. J., Westley, P. A. H., Baskett, M. L., Carlson, S. M., Clark, J., et al. (2020). Recent declines in salmon body size impact ecosystems and fisheries. Nat. Commun. 11:4155. doi: 10.1038/s41467-020-17726-z
Olmos, M., Massiot-Granier, F., Prévost, E., Chaput, G., Bradbury, I. R., Nevoux, M., et al. (2019). Evidence for spatial coherence in time trends of marine life history traits of Atlantic salmon in the North Atlantic. Fish Fish. 20, 322–342. doi: 10.1111/faf.12345
Olmos, M., Payne, M. R., Nevoux, M., Prévost, E., Chaput, G., Du Pontavice, H., et al. (2020). Spatial synchrony in the response of a long range migratory species (Salmo salar) to climate change in the North Atlantic Ocean. Glob. Change Biol. 26, 1319–1337. doi: 10.1111/gcb.14913
Pellissier, L., Albouy, C., Bascompte, J., Farwig, N., Graham, C., Loreau, M., et al. (2018). Comparing species interaction networks along environmental gradients. Biol. Rev. 93, 785–800. doi: 10.1111/brv.12366
Perkins, D. M., Durance, I., Edwards, F. K., Grey, J., Hildrew, A. G., Jackson, M., et al. (2018). Bending the rules: exploitation of allochthonous resources by a top-predator modifies size-abundance scaling in stream food webs. Ecol. Lett. 21, 1771–1780. doi: 10.1111/ele.13147
Petchey, O. L., Beckerman, A. P., Riede, J. O., and Warren, P. H. (2008). Size, foraging, and food web structure. Proc. Natl. Acad. Sci. U.S.A. 105, 4191–4196. doi: 10.1073/pnas.0710672105
Reum, J. C., Blanchard, J. L., Holsman, K. K., Aydin, K., and Punt, A. E. (2019). Species-specific ontogenetic diet shifts attenuate trophic cascades and lengthen food chains in exploited ecosystems. Oikos 128, 1051–1064. doi: 10.1111/oik.05630
Rudolf, V. H., and Rasmussen, N. L. (2013). Ontogenetic functional diversity: size structure of a keystone predator drives functioning of a complex ecosystem. Ecology 94, 1046–1056. doi: 10.1890/12-0378.1
Russell, I. C., Aprahamian, M. W., Barry, J., Davidson, I. C., Fiske, P., Ibbotson, A. T., et al. (2012). The influence of the freshwater environment and the biological characteristics of Atlantic salmon smolts on their subsequent marine survival. ICES J. Mar. Sci. 69, 1563–1573. doi: 10.1093/icesjms/fsr208
Sandlund, O. T., Berntsen, H. H., Fiske, P., Kuusela, J., Muladal, R., Niemelä, E., et al. (2019). Pink salmon in Norway: the reluctant invader. Biol. Invasions 21, 1033–1054. doi: 10.1007/s10530-018-1904-z
Scott, F., Blanchard, J. L., and Andersen, K. H. (2013). Multispecies, trait and community size spectrum ecological modelling in R (mizer). MIZER Vignette. Methods Ecol. Evol. 5, 1121–1125. doi: 10.1111/2041-210X.12256
Smallegange, I. M., Caswell, H., Toorians, M. E., and de Roos, A. M. (2017). Mechanistic description of population dynamics using dynamic energy budget theory incorporated into integral projection models. Methods Ecol. Evol. 8, 146–154. doi: 10.1111/2041-210X.12675
Soto, D. X., Trueman, C. N., Samways, K. M., Dadswell, M. J., and Cunjak, R. A. (2018). Ocean warming cannot explain synchronous declines in North American Atlantic salmon populations. Mar. Ecol. Prog. Ser. 601, 203–213. doi: 10.3354/meps12674
Spence, M. A., Blackwell, P. G., and Blanchard, J. L. (2016). Parameter uncertainty of a dynamic multispecies size spectrum model. Can. J. Fish. Aquat. Sci. 73, 589–597. doi: 10.1139/cjfas-2015-0022
Strøm, J. F., Rikardsen, A. H., Campana, S. E., Righton, D., Carr, J., Aarestrup, K., et al. (2019). Ocean predation and mortality of adult Atlantic salmon. Sci. Rep. 9:7890. doi: 10.1038/s41598-019-44041-5
Thompson, M. S., Bankier, C., Bell, T., Dumbrell, A. J., Gray, C., Ledger, M. E., et al. (2016). Gene-to-ecosystem impacts of a catastrophic pesticide spill: testing a multilevel bioassessment approach in a river ecosystem. Freshw. Biol. 61, 2037–2050. doi: 10.1111/fwb.12676
Thompson, M. S., Brooks, S. J., Sayer, C. D., Woodward, G., Axmacher, J. C., Perkins, D. M., et al. (2018). Large woody debris “rewilding” rapidly restores biodiversity in riverine food webs. J. Appl. Ecol. 55, 895–904. doi: 10.1111/1365-2664.13013
Todd, C. D., Friedland, K. D., MacLean, J. C., Hazon, N., and Jensen, A. J. (2011). “Getting into hot water? Atlantic salmon responses to climate change in freshwater and marine environments,” in Atlantic Salmon Ecology, eds O. Aas, S. Einum, A. Klemetsen, and J. Skurdal (Chichester: Wiley-Blackwell), 409–444. doi: 10.1002/9781444327755.ch16
Trebilco, R., Baum, J. K., Salomon, A. K., and Dulvy, N. K. (2013). Ecosystem ecology: size-based constraints on the pyramids of life. Trends Ecol. Evol. 28, 423–431. doi: 10.1016/j.tree.2013.03.008
Verspoor, E., Stradmeyer, L., and Nielsen, J.L. (eds). (2008). The Atlantic salmon: Genetics, Conservation and Management. Hoboken, NJ: John Wiley & Sons. doi: 10.1002/9780470995846
Winfield, I. J. (2016). Recreational fisheries in the UK: natural capital, ecosystem services, threats, and management. Fish. Sci. 82, 203–212. doi: 10.1007/s12562-016-0967-y
Woodward, G., Benstead, J. P., Beveridge, O. S., Blanchard, J., Brey, T., Brown, L. E., et al. (2010a). Ecological networks in a changing climate. Adv. Ecol. Res. 42, 71–138. doi: 10.1016/B978-0-12-381363-3.00002-2
Woodward, G., Blanchard, J., Lauridsen, R. B., Edwards, F. K., Jones, J. I., Figueroa, D., et al. (2010b). Individual-based food webs: species identity, body size and sampling effects. Adv. Ecol. Res. 43, 211–266. doi: 10.1016/B978-0-12-385005-8.00006-X
Woodward, G., Brown, L. E., Edwards, F. K., Hudson, L. N., Milner, A. M., Reuman, D. C., et al. (2012). Climate change impacts in multispecies systems: drought alters food web size structure in a field experiment. Philos. Trans. R. Soc. Lond. B Biol. Sci. 367, 2990–2997. doi: 10.1098/rstb.2012.0245
Woodward, G., Ebenman, B., Emmerson, M., Montoya, J. M., Olesen, J. M., Valido, A., et al. (2005a). Body size in ecological networks. Trends Ecol. Evol. 20, 402–409. doi: 10.1016/j.tree.2005.04.005
Woodward, G., Speirs, D. C., Hildrew, A. G., and Hal, C. (2005b). Quantification and resolution of a complex, size-structured food web. Adv. Ecol. Res. 36, 85–135. doi: 10.1016/S0065-2504(05)36002-8
Woodworth-Jefcoats, P. A., Blanchard, J. L., and Drazen, J. C. (2019). Relative impacts of simultaneous stressors on a pelagic marine ecosystem. Front. Mar. Sci0 6:383. doi: 10.3389/fmars.2019.00383
WWF International (2021). The World’s Forgotten Fishes. Available online at: https://europe.nxtbook.com/nxteu/wwfintl/freshwater_fishes_report/index.php (accessed December 1, 2021). doi: 10.3389/fmars.2019.00383
Keywords: Atlantic salmon (Salmo salar), marine and freshwater fisheries, ecosystem-based management (EBM), matrix projection models, metabolic theory of ecology (MTE), life-stage models, size structure
Citation: Woodward G, Morris O, Barquín J, Belgrano A, Bull C, de Eyto E, Friberg N, Guðbergsson G, Layer-Dobra K, Lauridsen RB, Lewis HM, McGinnity P, Pawar S, Rosindell J and O’Gorman EJ (2021) Using Food Webs and Metabolic Theory to Monitor, Model, and Manage Atlantic Salmon—A Keystone Species Under Threat. Front. Ecol. Evol. 9:675261. doi: 10.3389/fevo.2021.675261
Received: 02 March 2021; Accepted: 22 November 2021;
Published: 17 December 2021.
Edited by:
David Jack Coates, Department of Biodiversity, Conservation and Attractions (DBCA), AustraliaReviewed by:
Frederick Gilbert Whoriskey, Dalhousie University, CanadaCopyright © 2021 Woodward, Morris, Barquín, Belgrano, Bull, de Eyto, Friberg, Guðbergsson, Layer-Dobra, Lauridsen, Lewis, McGinnity, Pawar, Rosindell and O’Gorman. This is an open-access article distributed under the terms of the Creative Commons Attribution License (CC BY). The use, distribution or reproduction in other forums is permitted, provided the original author(s) and the copyright owner(s) are credited and that the original publication in this journal is cited, in accordance with accepted academic practice. No use, distribution or reproduction is permitted which does not comply with these terms.
*Correspondence: Eoin J. O’Gorman, ZS5vZ29ybWFuQGVzc2V4LmFjLnVr
†These authors have contributed equally to this work and share first authorship
Disclaimer: All claims expressed in this article are solely those of the authors and do not necessarily represent those of their affiliated organizations, or those of the publisher, the editors and the reviewers. Any product that may be evaluated in this article or claim that may be made by its manufacturer is not guaranteed or endorsed by the publisher.
Research integrity at Frontiers
Learn more about the work of our research integrity team to safeguard the quality of each article we publish.