- 1Laboratório de Coleções Zoológicas, Instituto Butantan, São Paulo, Brazil
- 2Fundação Butantan, São Paulo, Brazil
- 3Graduando em Ciências da Computação, Faculdade Anhembi Morumbi, São Paulo, Brazil
- 4Laboratório de Parasitologia, Instituto Butantan, Instituto de Medicina Tropical, Universidade de São Paulo, São Paulo, Brazil
- 5Instituto de Matemática e Estatística, Universidade de São Paulo, São Paulo, Brazil
Mosquito-borne diseases affect millions of people and cause thousands of deaths yearly. Vaccines have been hitherto insufficient to mitigate them, which makes mosquito control the most viable approach. But vector control depends on correct species identification and geographical assignment, and the taxonomic characters of mosquitoes are often inconspicuous to non-taxonomists, which are restricted to a life stage and/or even damaged. Thus, geometric morphometry, a low cost and precise technique that has proven to be efficient for identifying subtle morphological dissimilarities, may contribute to the resolution of these types of problems. We have been applying this technique for more than 10 years and have accumulated thousands of wing images with their metadata. Therefore, the aims of this work were to develop a prototype of a platform for the storage of biological data related to wing morphometry, by means of a relational database and a web system named “WingBank.” In order to build the WingBank prototype, a multidisciplinary team performed a gathering of requirements, modeled and designed the relational database, and implemented a web platform. WingBank was designed to enforce data completeness, to ease data query, to leverage meta-studies, and to support applications of automatic identification of mosquitoes. Currently, the database of the WingBank contains data referring to 77 species belonging to 15 genera of Culicidae. From the 13,287 wing records currently cataloged in the database, 2,138 were already made available for use by third parties. As far as we know, this is the largest database of Culicidae wings of the world.
Introduction
Diseases whose etiological agents are dispersed by vectors, such as mosquitoes, have been a major public health problem worldwide for years. These diseases, between the 17th and 20th centuries, caused the death of more people than all other causes combined (Gubler, 1991) and interfered with the economic development of large areas around the world (Philip and Rozenboom, 1973; Calmon, 1975; Gubler, 1991). Amongst insect Families popularly known as mosquitoes, the Culicidae family represents an important group composed of several vector species of disease-causing pathogens, including viruses, worms and protozoans. All these pathogens cause important but neglected Tropical Diseases, such as lymphatic filariasis, which is one of the leading causes of global disability. This disease affects around 120 million people in tropical and subtropical areas of the world (World Health Organization, 2020a). In 2019, it led more than 400,000 people to death, 67% of them children (World Health Organization, 2020b).
However, despite the importance of mosquitoes, their taxonomic identification based only on traditional morphology is quite complex and often difficult to conclude, i.e., Subgenus Nyssorhynchus, which is difficult to identify using only external morphology (Sallum et al., 2010). In addition, traditional taxonomy has been in crisis for decades (Godfray, 2002), with few professional specialists in the field remaining worldwide. Furthermore, frequently mosquito samples are morphologically damaged by being sampled using CDC light, BG or other mosquito traps. Even taxonomists have difficulty identifying them. Thus, the arising of new technologies has complemented the traditional technique in several studies, such as the case of geometric morphometry (GM), which is based on the fusion between geometry, biology (Bookstein, 1982) and statistics (Monteiro and Reis, 1999).
The GM allows the multivariate study of the shape of biological structures in two or three spatial dimensions. This technique allows various statistical biometric assessments, graphic representation of shape and size, preserving the physical integrity of the shape and preventing a collapse in linear measurements that do not represent the structure as a whole (Richtsmeier et al., 2002). Based on multivariate character analysis, which allows the simultaneous comparison of different characteristics in a complex body structure (Rohlf, 1993; Monteiro and Reis, 1999), this technique has been used extensively to solve different biological problems. In some cases, it is useful when applied alone, while in others its combination with any other integrative taxonomy technique can increase its accuracy (Schlick-Steiner et al., 2010; Garros and Dujardin, 2013; Lorenz et al., 2017).
In mosquitoes, the most used structure is the wings (Ruangsittichai et al., 2011; Jaramillo et al., 2015; Sumruayphol et al., 2016; Wilke et al., 2016; Chaiphongpachara and Laojun, 2019; Chaiphongpachara et al., 2019; Sauer et al., 2020; Souza et al., 2020), mainly due to its two-dimensionality, which increases precision and repeatability even when they are assembled and digitized by different operators (Lorenz and Suesdek, 2013). In general, the shape of the wing is quite informative (Klingenberg, 2010), on both hereditary, geographic, and evolutionary issues. Some studies have indicated that in Diptera, the wing shape is inheritable (Bitner-Mathé and Klaczko, 1999), has polygenic determination and is minimally influenced by epigenetic factors (Jirakanjanakit et al., 2007; Morales-Vargas et al., 2010). Although reports indicate that the shape of the wing is influenced by temperature differences and eco-geographic factors (Aytekin et al., 2009; Gómez et al., 2014), this influence is minimal (Morales Vargas et al., 2013). Therefore, the shape of the wing is presented as a more stable character than the size of the wing, which is strongly influenced by the environment (climatic and environmental factors such as larval density in the same breeding place, availability of food, temperature, etc.) (Morales Vargas et al., 2013; Gómez et al., 2014), and variations in this character should be interpreted with caution.
Any study of the Life Science field needs reliable taxonomic identification. However, every year there is a more frequent need for helpful and innovative techniques since the traditional taxonomy has been increasingly restricted to a specialized group. Thus, automated identification methods which extract and analyse informative features of species in images have emerged as a helpful tool, and consequently, the number of large databases (DBs) hosting this data has also grown. Although DBs of winged insect images are mentioned in thematic reviews in which researchers emphasize the importance of creating morphometric DBs for culicids (Dujardin, 2008; Lorenz et al., 2017) and other vectors, efforts toward building those specific to mosquitoes are still incipient. This gap has hindered classification tests or biometric identification, as well as studies of long-term biological inference in insects (Sonnenschein et al., 2015).
According to the Registry of Research Data Repositories–re3data1, there are about 1,423 databases registered in the Life Science area, and 1,311 in Natural Sciences. These numbers together correspond to approximately six times the number of records in the Engineering area (with the lowest number of records, 482)—values updated in January 2021. This can be explained by the large growth in data production in recent years, mainly the Big Data common in the area of Molecular Biology, reflecting the massive production of gene sequences (Stephens et al., 2015). However, despite the expressive number of DBs registered in re3data, Brazil contributes only with 11 records, which certainly does not reflect the amount of DBs created and used in the country.
The myth of the Tower of Babel besides serving as a metaphor for an existing problem in the systematic of insects (Caterino et al., 2000), can also be associated with the extensive “independent production” of programs and databases related to gene annotations (Drãghici et al., 2006), for example. Thus, to avoid the same inconsistency of the aforementioned metaphor, morphometric DBs created in the Culicidology area must be able to connect to other DBs, whether they are about georeferenced, genetic annotation or bibliographic data.
The wing GM, in addition to requiring computational implementations, requires a robust DB that allows the validation of this application through tests. Furthermore, according to Garros and Dujardin (2013), for a morphometric DB to have taxonomic utility, it must share, at least, the computed cartesian coordinates and/or the images of the structures. As far as we know, there are only two repositories that share useful images for alar GM on insects, ApiClass2 and XYOM (formerly CLIC bank;3). ApiClass is a specialized online system for identifying bee subspecies belonging to the species Apis mellifera Linnaeus, 1758, based on the wings, and which uses a Relational DB (RDB) with 5,763 images maintained with the MySQL database management system (DBMS). 3 (Dujardin, 2012).
In this work, we present WingBank4 a data platform developed to be a pioneer in the storage and sharing of wing images, cartesian coordinates of the images and other morphological information, and data of interest for integrative taxonomy, such as spatio-temporal data. In addition, it was conceived to serve as a basis for machine learning in the GM field, managing data from dozens of species and thousands of specimens of mosquitoes. WingBank architecture is composed of an RDB, managed through the DBMS Microsoft SQL Server (MS-SQL), and a prototype of a web system that fosters research by allowing users to retrieve the data they need and also contribute with new data.
This article describes: (a) the modeling and implementation of the WingBank’s RDB, aimed at micro and macroevolutionary studies that use the wing geometric morphometrics technique; and (b) the development of the web system for managing biological data related to wing morphometry, which is the user interface for this RDB.
Materials and Methods
Target Audience
The WingBank system is intended for undergraduate and graduate students, scientific researchers, and health professionals, such as entomological and epidemiological supervisors, and other interested parties, who need to download or upload images and data of wing geometric morphometry of mosquitoes around the world.
Taxonomic Classification
The taxonomic classification adopted in this work followed the one used by Walter Reed Biosystematics Unit (WRBU), Entomology Division, Walter Reed Research Institute (Walter Reed Army Institute of Research, WRAIR) in its Systematic Catalog of Culicidae5 and presented by Ralph Harbach (Elmasri and Navathe, 2011) in his Taxonomic Mosquito Inventory6, following the guidelines of Wiley and Liebermann (2011); Vences et al. (2013), and Wilkerson et al. (2015) for the classification of the Aedini Tribe. The informal taxonomic categories (Series, Group, Subgroup, Complex) were not adopted in the modeling and implementation of DB. Only the definitions a) sensu lato (“s.l.”) with the records of species belonging to the species complex, b) “sp.” with the records that do not have specific identification, and c) sensu stricto (“s.s.”) for records identified at a specific level were adopted.
Data Organization
As a basis for the creation of the WingBank DB, around 14 thousand images of mosquito wings were used, deposited in the Entomological Collection under the curatorship of Dr Flávia Virginio, Lead Investigator of Medical Entomology Study Group, located at the Laboratório de Coleções Zoológicas (LCZ) of the Instituto Butantan (IB). Voucher specimens (wing donors) are also deposited in that collection. For this purpose, the wings were extracted from mosquitoes, mounted between a slide and coverslip as described by Virginio et al. (2015). Each wing, when deposited in the Collection, received an identification tag containing a sequential code in accordance with the policies adopted by LCZ, and with additional information, such as gender, wing side and number of the individual (e.g., FD001-IBSP-Ent 1). All wings were photographed and stored in digital format together with their morphometric and biological, taxonomic, geographical, space-time, environmental, climate and human resources information.
WingBank Creation: Step by Step
The main phases for the creation of a DB are gathering of requirements, conceptual database design, choice of a DBMS, data model mapping (also called logical database design), physical database design and finally, database system implementation and tuning (Elmasri and Navathe, 2011). Thus, for the creation of the WingBank DB, firstly the requirements specification and analysis were carried out with the researchers who own the images, based on the data collection forms and guidelines proposed by Gaffigan and Pecor (Gaffigan and Pecor, 1997) and Foley et al. (2011). This step basically consisted of collecting information regarding the interests of DB users and the program applications that will interact with it, based on occasional meetings. From this, it was possible to identify the main requirements for the elaboration of the WingBank, characterize the types of data desirable for storage in WingBank and the query and data maintenance functionalities to be offered to users through the web system.
After gathering the requirements, in the second phase, the construction of the Conceptual Database Model was elaborated. Based on the Entity-Relationship Model (ERM) (Chen, 1977), an Entity-Relationship (ER) Diagram was built. This model was chosen because it assists in the modeling of relational DBs, is very expressive and easy to understand, and supplies the need for abstraction in this phase of modeling. Subsequently, in the third phase, using the software Visual Paradigm Community 14.17, the ER diagram was mapped into a relational diagram. In the relational model, data is organized “in tables, nothing but tables” (Date, 2004), in other words, the types of entities are transformed into tables, the attributes in columns of the respective tables, the multivalued attributes in different tables, the relationships in foreign keys and (possibly) new tables. In the diagram of the relational scheme, the crow’s foot notation (Everest, 1976) was used to indicate the cardinalities of the relationships established through foreign keys. This notation was created for conceptual data modeling, but it is often used (combined with other notations) to graphically represent cardinality and participation constraints on relationships expressed through foreign keys in a relational scheme. In the created relational scheme, the domain of each attribute was also defined.
At the fourth phase, it is delimiting important specifications related to stored databases, such as file storage structures and indexes. The scheme refinement is an optional step in the logical design, but it is commonly used. Through this, it is possible to identify potential problems in the created scheme and apply techniques to improve it. In the physical model of the WingBank DB, some final specifications were implemented, especially those related to storage and access to the DB. In this phase, some indexes were created, both to ensure the coherence of the connections between the data, and to improve the performance of future operations on the data (Elmasri and Navathe, 2011).
And finally, the last phase is about database and application programs implementation and testing, a continuous activity known as database tuning. To obtain useful knowledge from the data, it is necessary that it is clear. However, many databases are composed of redundant and inconsistent data, missing values, as well as values and fields that are not logically related to each other stored in the same table (Parsaye and Chignell, 1993; Savasere et al., 1995; Adriaans and Zantinge, 1996; Fayyad et al., 1996). To avoid mistakes before feeding the WingBank DB, part of the data cleaning was done manually, and the other part was performed together with the initial process of data entering, through SQL scripts, in a careful process of inspection, cleaning, standardization, completion and transformation of data. These steps refer to the Knowledge Discovery in Databases (KDD) methodology (Fayyad et al., 1996), except for the data mining phase.
As the raw data were not fully standardized, there was a small loss of information in the process. For example, the following were not uploaded to the database: (a) photographic records without information about gender, (b) geographic information of cases in which there was no relationship between individuals and localities, although there was information on the location of the collection. Data from other specific cases were kept, such as (c) photographic records with an unnoticed side, which did not compromise the integrity of the bank, (d) damaged wings, but with some preserved points.
There are different design patterns for the development of a system with access to the DB. In the WingBank web system, Domain-Driven Design (DDD) and Model View Controller (MVC) standards were used. The first is a development approach that focuses on understanding business rules and how they should be reflected in the system code and domain model (Evans, 2003). In addition to this technique being linked to the good practices of Object-Oriented Programming, it is a way of organizing the code so that the business rules are in delimited contexts and decoupled from the database. The DDD was used in the architecture of practically the entire application, from infrastructure to data access.
For the communication between the system model layer and the DB, the Object-Relational Mapping was used. It’s a technique that consists of representing a program object in a relational way so that it can be persisted in an RDB and, when necessary, be retrieved and created as an object in main memory again, without loss of information in this process.
The MVC standard was used only for the architecture of the web interface client application. In this pattern, the model layer refers to the representation of the data on which the system operates. The vision layer is the presentation of data and processing logic to end-users, even allowing their interaction with the system. Finally, the controller responds to events, manages access to the model and view, and coordinates the flow of data between them.
The WingBank web system provides features for searching, visualizing, and downloading data that are related to a scientific publication. As this data is public, that is, it can be accessed by anyone, there is no functionality to control user access in the developed prototype. The behavior of the system was described in an Activity Diagram in the Unified Modeling Language (UML).
Results
The WingBank RDB contains morphological information and their respective ecological and space-time metadata, exclusively from mosquitoes. This DB is a prototype under test and is available at https://wingbank.butantan.gov.br/. It is currently open for searching and downloading via the website and sending data via email V2luZ0JhbmtAYnV0YW50YW4uZ292LmJy. This database can also be used by users as a faithful depository of images and data of geometric morphometry, as it generates unique identifiers (see Standardization of image labeling) that can be cited in scientific publications.
This DB was inspired by the actions promoted by the National Center for Biotechnology Information (NCBI), one of the Institutes that has provided the most biological databases to date (Agarwala et al., 2015). At this moment, in WingBank, 13,287 wing records are registered, of which 12,939 are already linked to images (right and/or left wings) of mosquitoes belonging to the Culicidae family. Currently, there are 13 scientific publications registered on WingBank, of which 8 are already linked to 2,138 images which, due to this relationship with one or more publications, are already available for use by third parties (Table 1). Of these wing images available for use by third parties, 584 are left wings, 1,476 right wings and 78 with unknown sides. It is worth mentioning, as the wing images available for other users until now represent samples of totalities located in a unique time-space, we decided that instead of linking only the files used on each study, we would link all images of those specimens located in those unambiguous times and spaces. To date, WingBank stores data collected from 1998 to 2016, in 10 different Brazilian states. Forty-three people, linked to 19 different National and International Teaching and Research Institutions, have so far contributed to WingBank with the collection of information and specimens, mounting of slides, and/or photographic and morphometric records.
The main types of data (Figure 1) of this DB were from Field and/or Colony, and/or Donation; Wing images, which can be right and/or left wings and can be related to some Scientific Publication and also with landmark Notes; Taxonomic Classification (Family, Subfamily, Tribe, Genus, Subgenus, Species, Subspecies), Classification Method, which is possible to report which method was used (Molecular or Morphological); and the Person Responsible for identifying each individual. It is important to mention that the relationship between Wing Image and a Scientific Publication is a requirement for an individual’s data to be publicly available.
Conceptual Database Design
A conceptual database model must be concise, descriptive, and present the main requirements of the modeled domain. It can be expressed in different ways. The most common and that was used in this DB, is through the ER diagram. Elmasri and Navathe’s graphical notation (Elmasri and Navathe, 2011) was used, which is based on the notation of Peter Chen, creator of ERM (Codd, 1970). An ER diagram is structured in (see Figure 2): (A) types of entities (rectangles), which represent the abstraction of a real-world object in the DB, such as, Individual, Image and Taxonomic Identification; (B) types of relationships (diamond-shaped boxes), which are connected by lines to the rectangular boxes representing the participating entity types, followed by the cardinality ratio and participation constraint of each relationship type, such as: [individual] 1 < has > 2 [image], [individual] 1 < is > 1 [species], that is, each individual can have up to 2 images, and each individual corresponds to only one species; (c) attributes (ellipses), which are details, properties that characterize a type of entity, such as gender of the individual and dimension of the image.
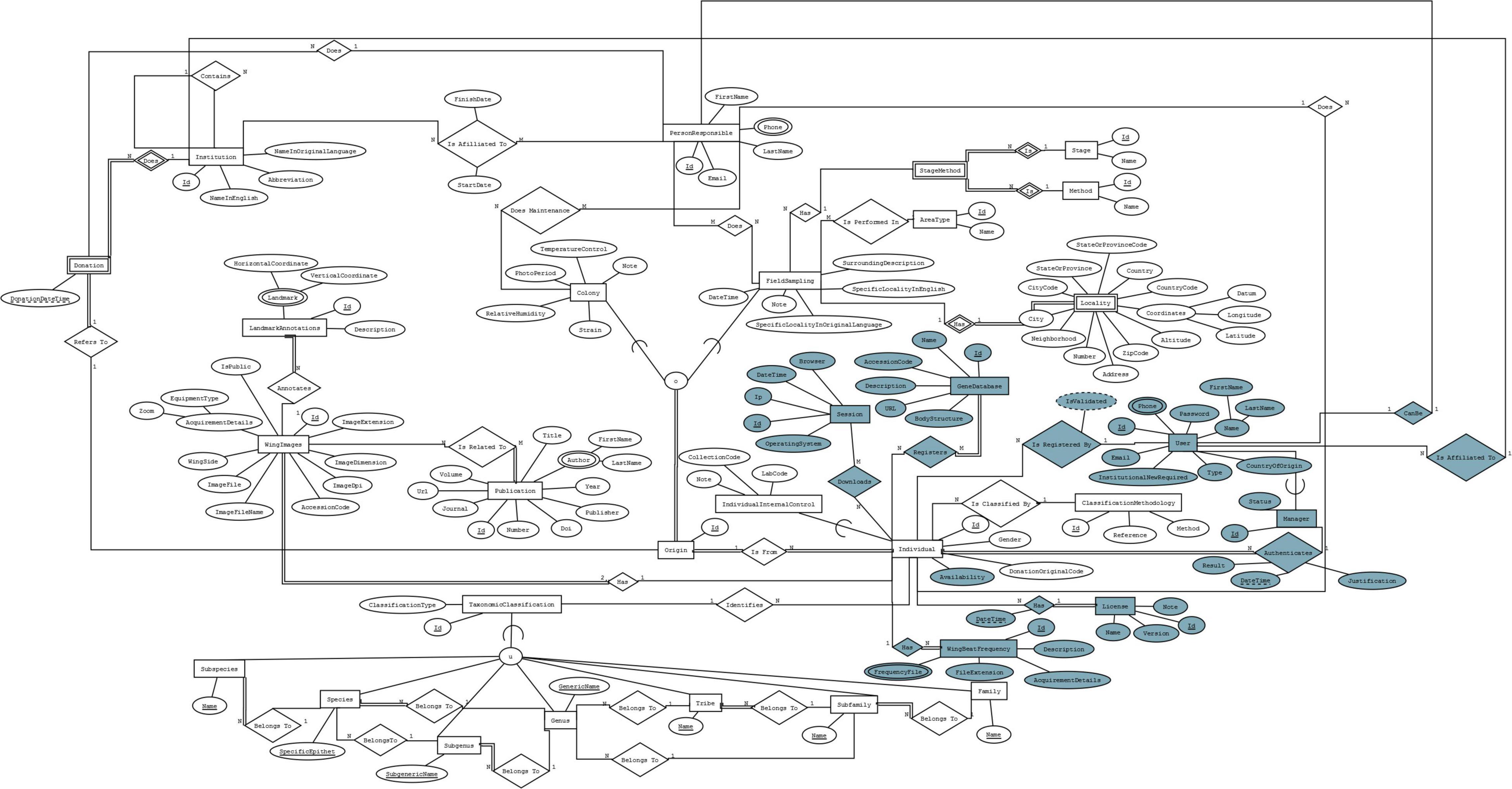
Figure 2. Representation of the Conceptual Database Design through an Enhanced Entity-Relationship (EER) diagram. Rectangles: types of entities, which represent the abstraction of a real-world object. Ellipses: Attributes, which are details, properties that characterize a type of entity. Diamonds: types of relationships, which represent associations between entities, accompanied by their participation restrictions and cardinalities. Filled geometric forms: Entity, Attributes and Relationships which were planned but not yet implemented (Phase II).
Entities are typified as strong (defined in the diagram by a simple outline, e.g., Publication) or weak (double outline, e.g., Donation). Attributes can be compound (subdivided into more than one component attribute, e.g., Coordinates); multivalued (with double contour, e.g., Phone in PersonResponsible); complex (multivalued and compound at the same time; e.g., Author in Publication); derivative (with dashed outline; e.g., Is validated). When the participation constraints of an entity in a relationship are partial, the line between them is simple; when the participation is total, the line is double (Elmasri and Navathe, 2011).
For a better understanding of the diagram that will be presented below, it is worth mentioning that this database has specializations. Specialization is the definition of subsets of entities (subclasses) of an entity type (in this case, considered a superclass), in which the subclasses have their own attributes and/or relationships and, at the same time, inherit the attributes (including, the keys) of the superclass. Therefore, an entity in a subclass is always an entity in its superclass. Specialization can be of the overlapping type (denoted by the symbol “o” inside a circle) or disjunctional type (symbol “d” inside a circle). In the disjunctional type, a superclass entity can be at most in one of the subclasses of the specialization; in the overlapping type, an entity of the superclass can be in several subclasses. A category (denoted by the symbol “u” in a circle) can be seen as a set of entities of different types that do not necessarily have attributes in common between them (Elmasri and Navathe, 2011).
In general, the symbol ∩ is added to the relationship in which you want to represent the specialization or category. The opening of this symbol is directed at the entity on which the restriction is being applied. For example, if the intention is to represent that an Individual can originate from a Field Collection, a Laboratory Colony, or that he can be collected in the Field, but then be Colonized/Maintained in the laboratory, this can be represented by directing the opening of the symbol to its Origin (which is the superclass of an overlapping specialization) as shown in Figure 2.
Key attributes of entity types (solely identifying their entities) are underlined (e.g., Id in most entities), while those that are partial keys are denoted by a dashed line (as in DonationDateTime). The WingBank is represented by an Enhanced Entity-Relationship (EER) diagram, as some specific restrictions have been applied, such as the total overlapping specialization of Origin (Colony and FieldSampling), the Individual specialization (IndividualInternalControl); and the Taxonomic Classification category, which is a subset of the union of entity classes: family, Subfamily, Tribe, Genus, Subgenus, Species and Subspecies. The weak entity type StageMethod has no attributes (something unusual) because it was created to represent a binary relationship between Stage and Method and, therefore, to make it possible its association with FieldSampling. Weak entity types, like Locality, did not receive a partial key, as they participate in a 1:1 relationship with Sampling, that is, if each Sampling can have only 1 Locality, then the Locality key can be the Sampling key itself.
The Conceptual Model represents the following situation: An individual (female or male) is taxonomically classified using a method and based on a specific reference. Taxonomic information for each individual can be presented at different levels: Family, Subfamily, Tribe, Gender, Species and Subspecies. Each individual, when registered in the system, automatically receives a unique and sequential code (“WingBankCode”), which will be with it as part of the DB. The specimen may originate from a field data collection, from a Laboratory Colony, or even being collected and then colonized. In either case, it may also have come from a Donation. If the individual is the result of a collection, it must have information regarding the collected stage, collection method, date of collection, general and specific location, information about the surroundings of the collection area, type of area, etc. If the individual comes from a laboratory colony, it must have information regarding laboratory conditions, such as temperature, relative humidity, etc. If the individual originated from a donation and has a “DonationOriginalCode,” this code is kept in the records. If this individual is an internal property of the Instituto Butantan, it will have a “LabCode” and if it is already deposited at the Entomological Collection of LCZ, it will receive a “CollectionCode.” In addition, there are people responsible for each step individuals go through, such as collection, colonization, donation, and taxonomic classification. These people are linked to one or more institutions.
Considering the importance of sharing computed coordinates in morphometric DBs, as mentioned by Garros and Dujardin (2013), WingBank DB modeling included the types of entities LandmarkAnnotation and Landmark, which make it possible to store raw computed coordinates (Landmarks and/or Semilandmarks of the wings). Some types of entities have attributes with predefined domains (Table 2), as the case of the type of entity AreaType, in which the values of the Name attribute were restricted to Park, which represents Urban Park or Linear Park; Urban, which refers to areas distributed by cities; Preserved, which represents Forested Areas, Environmental Protection Areas, Conservation Units, or Permanent Preservation Areas; and Rural, which represents Agriculture and Livestock.
Other types of entities such as Stage and Method, at first, received restricted values for the “Name” attribute, such as: Immature, Egg, Larva (1st stage), Larva (2nd stage), Larva (3rd stage), Larva (4th stage), Larva, Pupa, Adult, Immature/Adult and Manual Sampling, Manual Aspiration, Metal/Plastic Scoop, Metal Ladle, Fine Mesh, Pasteur Pipette/Dropper, Manual suction pump, Entomological Manual Aspirator, Entomological Automatic Aspirator, Trap, Egg Trap, Larva Trap, Adult Trap, CDC, Shannon, New Jersey, MoquitoMagnet, Manual Sampling/Manual Aspiration, respectively. Finally, the entity type ClassificationMethodology received only two different values for the ClassificationMethod attribute: Morphological or Molecular.
As the data currently stored on WingBank has been collected in the past and with less homogeneity, part of the attributes may receive the special value NULL, since the values of these attributes for some of the previously collected records are unknown. It is worth mentioning that this does not mean that these fields will be permanently null. The new records are expected to be more complete. Furthermore, in order to complement the implicit and explicit restrictions expressed in the DB scheme, the semantic restrictions that apply to the data have been described in a data dictionary (Supplementary Table 1).
Logical Database Design
The relational data model was created by Edgar F. Codd (1970) and was considered a revolutionary idea in the 1970s (Seltzer, 2008). The main motivation was an observation made about the workflow of programmers from the company IBM (International Business Machines) where Codd worked. He needed to rewrite a large number of application programs manually whenever the content or physical organization of a database changed (IBM, 2011). Relational DBs were originally proposed to separate the physical storage of data from its conceptual representations and provide mathematical foundations for the representation and search for data (see Figure 3).
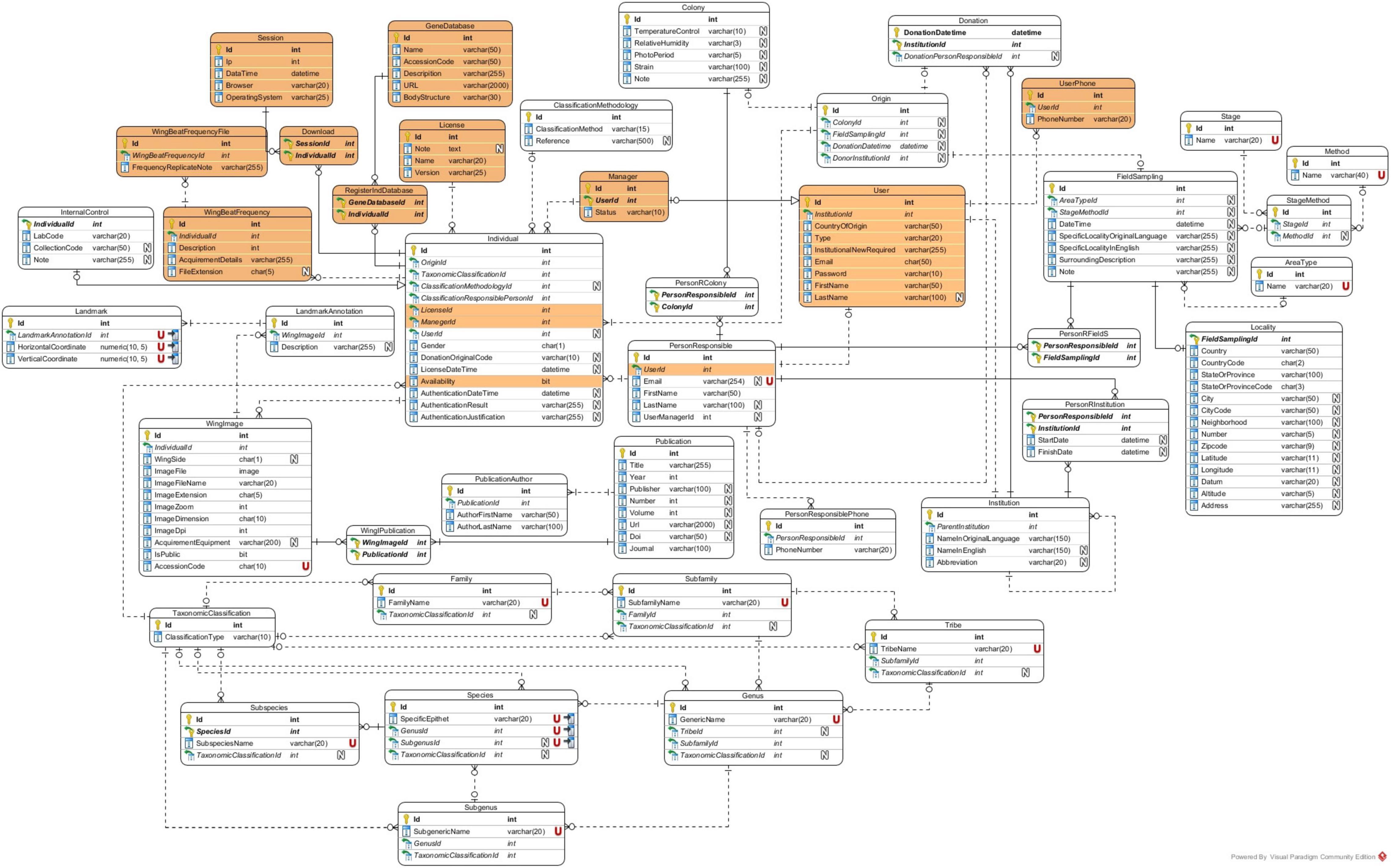
Figure 3. Representation of the Logical Database Design by mapping a conceptual schema in the EER model into a relational representation. In this model, the types of entities are transformed into tables, the attributes in columns of the respective tables, the multivalued attributes in other tables, the relationships in foreign keys and (possibly) new tables. This diagram also shows the crow’s foot notation which indicates the cardinalities of the relationships established by means of foreign keys. Filled boxes: Entity, Attributes and Relationships which were planned but not yet implemented (Phase II).
Standardization of Image Labeling
The images of each wing stored in the WingBank are fundamental pieces for this DB. With its implementation, they were functionally linked to other information which enables data validation and recovery, considering that MorphoJ (Klingenberg, 2011), one of the programs commonly used in GM analysis, has a feature called “Extract New Classifier from ID String.” This feature allows the classification of samples to be analyzed based on each character present in the file label (input). A nomenclature standard for wing image files is proposed here, which applies to all files submitted to this DB.
Each record on the WingBank (image of the wing + other metadata), when registered in the system, automatically receives a unique and sequential access code (“WingBankCode”), which will be with it as part of the DB, and will facilitate the creation of a reference in a scientific publication, for example. This identifier consists of an alphanumeric string containing a letter (prefix) representing the gender (F or M) of the individual, a sequence of 8 non-variable fixed digits, filled with leading zeros, a letter (suffix), which represents the side (R or L, right or left); and finally, when the information related to the side is non-existent, the insertion of the letter U (Unknown) is adopted, as in “F00000001R,” “F00000001L,” and “F00000001U,” respectively.
Implementation of the Web System
Figure 4 is a diagram in UML that describes the behavior of the web search system developed for WingBank. This Activity Diagram represents the flows of the system processes, whether they are business processes or internal operations. This type of diagram also shows deviations for alternative processes, such as displaying an error message. It is then possible to observe the lanes which were used to organize the actors and components of the system at different stages of the flow, to make it more readable and show the importance of each actor in the flow. The actors of the flow arranged in the lanes are User and Google API. The components are Web and Server. As shown in the diagram, the search process always starts and ends with the user (User).
Currently, on the WingBank platform, there are two types of searches (Figure 5) the simple one, where the user performs the search by keywords, and (Figure 6) the advanced one, which allows the user a combined search in the most diverse ways. The user can filter the results by characteristics related to the collection (Sampling), that is, space-time information such as Specific Locality, which refers to specifications such as breeding type; Locality referring to the most generalized location such as an address, City, State/Province, Country or Geographic coordinates (Latitude and Longitude). It is also possible to filter searches by information related to animal biology, such as Gender and/or Wing Side.
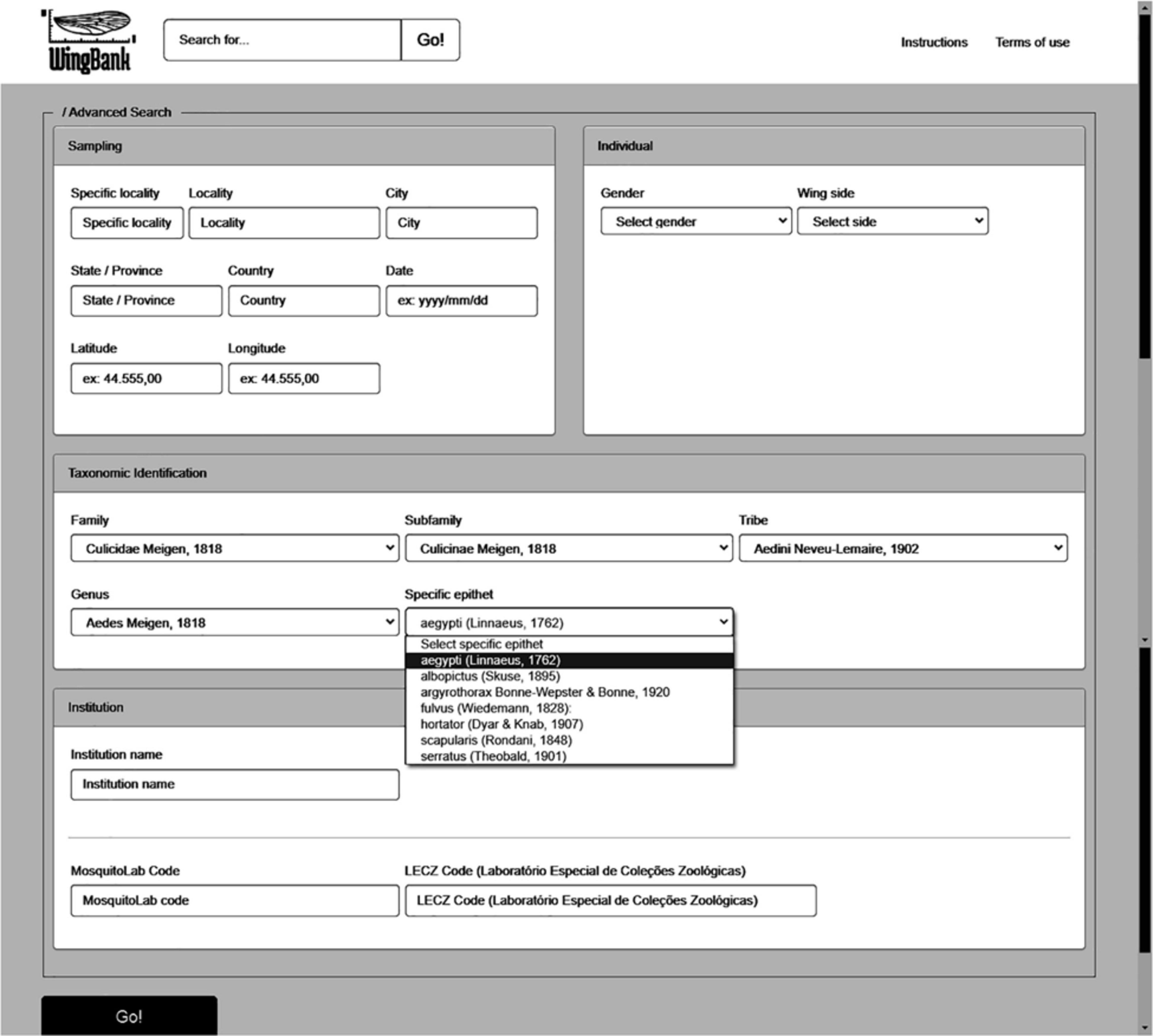
Figure 6. Layout interface of advanced search of WingBank website. A detailed search for “Taxonomic Identification.”
The search for Taxonomic Classification can also be filtered by Family, Tribe, Genus and Specific Epithet. The Institution field, although it can at first be used by any user, is of greater interest to the internal users of the Instituto Butantan, as it involves searching for related Institutions (InstitutionName), Internal Code of the laboratory (LabCode) and the Collection depository (LCZCode). The results are displayed in a table, in which the user can sort the results by any of the table headings, just dragging it to the indicated field.
Discussion
In recent publications referring to biological databases, Dujardin et al. (2010); Garros and Dujardin (2013) report on the importance of creating DBs related to the use of the mosquito wing geometric morphometry (WGM) technique. In addition, Lorenz et al. (2017); Jaramillo et al. (2015), and Wilke et al. (2016), among other several authors (Calle et al., 2002; Jirakanjanakit and Dujardin, 2005; Dujardin, 2008; Jirakanjanakit et al., 2008; Henry et al., 2010; Vidal et al., 2011; Motoki et al., 2012; Vidal and Suesdek, 2012; Yeap et al., 2013; Börstler et al., 2014; Laurito et al., 2015; Phanitchat et al., 2019; Chaiphongpachara and Laojun, 2020; Sauer et al., 2020; Carvajal et al., 2021) who have already used to solve biological problems in Culicidae, directly and indirectly, reinforce the importance of gathering morphological, ecological and space-time data related to mosquitoes in a DB. The WingBank can enable studies of reanalysis and meta-analysis, micro and macroevolutionary and that can also contribute to integrative taxonomy, a multidisciplinary approach defended by several authors as the best approach for the diagnosis of species (Schlick-Steiner et al., 2010; Garros and Dujardin, 2013). Garros and Dujardin (2013) suggest that the need for a DB is underestimated, since the very power of morphometry to identify rates is underestimated, reinforcing that the success of the identification of rates through WGM depends on the relevance of the reference images in their level of form divergence, as well as in the classification technique. In addition, a program of automatic digitization of points, such as WINGMACHINE (Houle et al., 2003), or morphometric analysis in an agile way such as XYOM (XYOM-CLIC) can optimize the process.
However, despite all entries about the importance, as far as we know, until the creation of the WingBank in 2018 (Virginio-Fonseca, 2018), there was no relational database to raw material for analysis of alar geometric morphometry for mosquitoes with worldwide reach. The only project closest to what was expected from WingBank was MoMe-CLIC (Morphometrics in Medical Entomology—Collection of Landmark for Identification and Characterization) which had an image repository “CLIC bank”—currently migrated to XYOM (XYOM-CLIC), created by Dujardin et al. (2010). It differs from WingBank by being a broad spectrum repository of Arthropod wing images covering different Classes and Orders, including Culicidae, Tephiritidae, Braconidae, Glossinidae, Ceratopogonidae, Psychodidae (Insecta; Diptera); Reduviidae (Insecta; Hemiptera) and Mummuciidae (Arachnida; Solifugae). With the changes that have taken place on its website over the years, XYOM became an interesting web application that dismisses downloads, installation, configuration and urdergo automatic updating. Although XYOM and WingBank have been distinctly conceived, their main goal allows them to be mutually complementary.
Currently, WingBank has data of 77 species belonging to 15 genera, as shown in Table 3. The WingBank shelters several mosquito species of medical and veterinary importance in Brazil and worldwide, such as Cx. nigripalpus, a species from which the Saint Louis virus has already been isolated (Belle et al., 1964; Chamberlain et al., 1964; Dow et al., 1964); Cx. coronator which has had specimens found naturally infected with several viruses, such as Saint Louis in Brazil and Trinidad and Tobago, the Venezuelan Equine Encephalitis virus in Mexico (Mackay et al., 2010) and the West Nile virus in the United States (Unlu et al., 2010); Cx. quinquefasciatus, species with records of participation in the transmission of West Nile fever, and lymphatic filariasis (Fernandes et al., 2016; Guedes et al., 2017).
In addition, it is possible to find in the WingBank data from Ae. aegypti, which participates in the transmission of urban yellow fever, Zika, chikungunya, dengue, besides being able to transmit microfilariae in urban areas (Jowett, 1986; Cirio, 2005; Lee and Rohani, 2005; Noridah et al., 2007; Chouin-Carneiro et al., 2016; Costa-Da-Silva et al., 2017a,b); Ae. scapularis, a species from which Ilhéus, Melao and yellow fever viruses were isolated (Spence et al., 1962; Vasconcelos et al., 2001; Pauvolid-Correa et al., 2013), and their participation in the transmission of the Rocio virus is suspected, due to their abundance in the Vale do Ribeira region at the time the epidemy occurred (Jowett, 1986). Another group present on WingBank is Psorophora ferox, which already was found naturally infected with Rocio virus (Lopes et al., 1981); Haemagogus leucocelaenus, which has specimens found naturally infected with the yellow fever virus (Shannon et al., 1938); Coquilettidia venezuelensis, related to the transmission of Oropouche virus (Forattini, 1965); and Mansonia titillans, from which Venezuelan Equine Encephalitis viruses have been isolated (Aitken, 1972; Sudia, 1972).
Furthermore, WingBank has data of several species of the Anopheles genus, such as Anopheles darlingi, An. aquasalis, An. nuneztovari sl, An. oswaldoi, An. triannulatus sl, An. albitarsis sl, An. cruzii sl, An. bellator and An. homunculus, which are related to the transmission of malaria (Ramirez and Dessen, 1994; Tadei and Thatcher, 2000; Bourke et al., 2013). More than that, WingBank stores images and information from many other species, which may not have been studied for vectorial capacity yet, but which can be considered for future studies. Currently, data from 11 species are available for search on WingBank, because they are already related to some scientific publication: Ae. aegypti, Ae. albopictus, Ae. scapularis, An. albitarsis s.l., An. arthuri s.l., An. cruzii s.l., An. homunculus, An. strodei s.l., An. triannulatus s.l., Cx. quinquefasciatus and Cx. nigripalpus. This number may increase rapidly from the beginning of the use of the DB by external users, who will be able to contribute with images and information of species collected around the world.
Finally, it is known that the predictive power of this data to compose an automated identification system based on machine learning (Weeks et al., 1999; Gurgel-Gonçalves et al., 2017; Khalighifar et al., 2019; Valan et al., 2019; Motta et al., 2020) depends largely on the quality and quantity of the training sample (Kalayeh and Landgrebe, 1983; Nigam et al., 2000; Mukherjee et al., 2003; Tam et al., 2006; Dobbin et al., 2008; Kim, 2009). In this context, WingBank is a pioneer on several fronts: exclusivity regarding the alar structure and the Family Culicidae, extremely important for public health worldwide; quantitative and qualitative awareness of the data; accessibility and credibility in making the material available in a friendly interface and reliable hosting at one of the most recognized research institutions in the world: the Instituto Butantan. Therefore, it is expected that this DB will be an important ally, and possibly a watershed event in the field of creating new technologies in the area of public health.
WingBank is the largest database of Culicidae wings that we are aware of, and the first of the relational type and with the proposed intentions. The database facilitates the search for information. It already has 13,287 wing records, of which 12,939 are already linked to images, and 2,138 images are already available for use by third parties, which makes it possible to carry out future meta-analysis and reanalysis studies. Furthermore, each record received an access code (WingBankCode), which makes it more efficient and reliable to cite the records of wings into scientific publications. In addition, with WingBank it was possible to contemplate the South American culicidofauna, which stands out for its biodiversity, as well as for the great number of mosquito-related diseases present in this region.
In this context, the WingBank composed of thousands of information with important richness and density about the diversity of the Brazilian Culicid fauna can be used as a basis for programs to digitize the landmarks of the wings of mosquitoes. Making automatic identification through the use of this DB should be the next step, which will be further strengthened with each contribution made by professional colleagues from around the world. New studies with micro and macroevolutionary objectives will also be possible, and the work of employees of health fields will be facilitated, mainly if they do not know with which species they are handling. As geometric morphometrics increasingly rises, the launch of WingBank may revolutionize Medical Entomology, bringing benefits to students, professional workers and civil society. We are not waiting in the wings, this collaborative work is only beginning.
Data Availability Statement
The original contributions presented in the study are included in the article/Supplementary Material, further inquiries can be directed to the corresponding author/s.
Author Contributions
LS had the first insight into the creation of the database. FV wrote the first draft of the manuscript and materialized the idea by building the basilar structure. All authors contributed to the subsequent study conception and design. FV and LS performed the material preparation and the data collection. FV, LA, VD, and KB designed and implemented the database. LA performed the data cleaning and SQL process. All authors contributed to the versions of the manuscript. All authors read and approved the final manuscript.
Funding
This research was funded by Coordenação de Aperfeiçoamento de Pessoal de Nível Superior (CAPES) grant 23038.005.274/2011-24, grant 032/2010—23038.001614/2016-52, and by Fundação de Amparo à Pesquisa do Estado de São Paulo (FAPESP) grants 13/05521-9, 11/18962-8, 10/15039-1, 10/14479-8, 07/01665-5, 06/05164-8 and 06/02622-5. LS has been fellow of Conselho Nacional de Desenvolvimento Científico e Tecnológico (CNPq) grants 311805/2014-0 and 311984/2018-5, and fellow of Fundação Butantan (FB).
Conflict of Interest
The authors declare that the research was conducted in the absence of any commercial or financial relationships that could be construed as a potential conflict of interest.
The handling editor declared a shared affiliation with several of the authors, LS and KB, at time of review.
Acknowledgments
We would like to thank the Instituto Butantan for allowing us to develop this work-study and allowing us to deposit the WingBank website on its domain, and for allowing the several mosquitoes samplings on its green area. We would also like to thank all workers and students from the Laboratório de Parasitologia and Laboratório de Coleções Zoológicas, from the same institution, for their support during sampling to provide the data. A special acknowledgment to Fernanda Almeida and Karina Zanatta (assistants from the Laboratório de Parasitologia) and Gabrielle R. de Andrade (Technologist from the Laboratório de Coleções Zoológicas) for hours of assistance on data organizing, spreadsheeting, paper diagramming and logistics, and Eliane Campos de Oliveira (Technologist from the Laboratório de Coleções Zoológicas) for years of dedication to support in curating the Entomological Collection from Instituto Butantan. Finally, we thank all taxonomists who identified the massive number of mosquitoes that we collected in the samplings.
Supplementary Material
The Supplementary Material for this article can be found online at: https://www.frontiersin.org/articles/10.3389/fevo.2021.660941/full#supplementary-material
Supplementary Table 1 | Data dictionary of the semantic restrictions applied to WingBank.
Footnotes
- ^ https://www.re3data.org/
- ^ http://apiclass.mnhn.fr
- ^ http://xyom-clic.eu/clic-bank/
- ^ https://wingbank.butantan.gov.br/
- ^ http://www.mosquitocatalog.org/
- ^ http://mosquitotaxonomic-inventory.info/
- ^ https://www.visual-paradigm.com/
References
Agarwala, R., Barrett, T., Beck, J., Benson, D. A., Bollin, C., Bolton, E., et al. (2015). Database resources of the national center for biotechnology information. Nucleic Acids Res. 43, D6–D17.
Aitken, T. H. G. (1972). “Habits of some mosquito hosts of VEE virus from northeastern South America, in cluding trinidad,” in Proceedings Workshop-Symposium on Venezuelan encephalitis virus. Pan American Health Organization, 243, (Washington DC: Scientific Publ), 254–256.
Aytekin, S., Aytekin, A. M., and Alten, B. (2009). Effect of different larval rearing temperatures on the productivity (Ro) and morphology of the malaria vector anopheles superpictus grassi (Diptera: Culicidae) using geometric morphometrics. J. Vector Ecol. 34, 32–42. doi: 10.1111/j.1948-7134.2009.00005.x
Belle, E., Grant, L., and Page, W. (1964). The isolation of st. louis encephalitis virus from culex nigripalpus mosquitoes in jamaica. Am. J. Trop. Med. Hygiene 13, 452–454. doi: 10.4269/ajtmh.1964.13.452
Bitner-Mathé, B. C., and Klaczko, L. B. (1999). Heritability, phenotypic and genetic correlations of size and shape of drosophila mediopunctata wings. Heredity 83, 688–696. doi: 10.1046/j.1365-2540.1999.00606.x
Bookstein, F. L. (1982). Foundations of morphometrics. Ann. Rev. Ecol. Systemat. 13, 451–470. doi: 10.1146/annurev.es.13.110182.002315
Börstler, J., Lühken, R., Rudolf, M., Steinke, S., Melaun, C., Becker, S., et al. (2014). The use of morphometric wing characters to discriminate female Culex pipiens and Culex torrentium. J. Vector Ecol. 39, 204–212.
Bourke, B. P., Oliveira, T. P., Suesdek, L., Bergo, E. S., and Sallum, M. A. M. (2013). A multi-locus approach to barcoding in the anopheles strodei subgroup (Diptera: Culicidae). Parasites Vectors 6:111.
Calle, L. D. A., Qui?ones, M. L., Erazo, H. F., and Jaramillo, O. N. (2002). Morphometric discrimination of females of five species of Anopheles of the subgenus Nyssorhynchus from Southern and Northwest Colombia. Memórias do Instituto Oswaldo Cruz. 97, 1191–1195. doi: 10.1590/s0074-02762002000800021
Carvajal, T. M., Amalin, D. M., and Watanabe, K. (2021). Wing geometry and genetic analyses reveal contrasting spatial structures between male and female Aedes aegypti (L.) (Diptera: Culicidae) populations in metropolitan Manila. Philippines. Infect. Genet. Evol. 87:104676. doi: 10.1016/j.meegid.2020.104676
Caterino, M. S., Cho, S., and Sperling, F. A. H. (2000). The current state of insect molecular systematics: a thriving tower of babel. Ann. Rev. Entomol. 45, 1–54. doi: 10.1146/annurev.ento.45.1.1
Chaiphongpachara, T., and Laojun, S. (2020). Wing morphometric variability of the malaria vector Anopheles (Cellia) epiroticus linton et harbach (diptera: culicidae) for the duration of the rainy season in coastal areas of samut songkhram. Thailand. Folia Parasitologica. (Praha). 67:2020.007.
Chaiphongpachara, T., Sriwichai, P., Samung, Y., and Ruangsittichai, J. (2019). Geometric morphometrics approach towards discrimination of three member species of Maculatus group in Thailand. Acta Tropica 192, 66–74. doi: 10.1016/j.actatropica.2019.01.024
Chaiphongpachara, T., and Laojun, S. (2019). Comparative analysis of the internal and external outlines of wings for an outline-based geometric morphometric approach to distinguish three Aedes mosquitoes (Diptera: Culicidae) in Thailand. J. Entomol. Acarol. Res. 51, 1–10.
Chamberlain, R., Sudia, W., Coleman, P., and Beadle, L. (1964). Vector studies in the St. Louis encephalitis epidemic, tampa bay area, Florida, 1962. Am. J. Trop. Med. Hygiene 13, 456–461. doi: 10.4269/ajtmh.1964.13.456
Chen, P. P.-S. (1977). The Entity-Relationship Model: Toward a Unified View of Data. Massachusetts, MA: Library Of The Massachusetts Institute Of Technology.
Chouin-Carneiro, T., Vega-Rua, A., Vazeille, M., Yebakima, A., Girod, R., Goindin, D., et al. (2016). Differential susceptibilities of aedes aegypti and aedes albopictus from the americas to Zika Virus. PLoS Negl. Trop. Dis. 10:e0004543. doi: 10.1371/journal.pntd.0004543
Cirio, S. M. (2005). Epidemiologia E Clínica De Cães Portadores De Dirofilariose Em Espaços Urbanos De Município Do Litoral Do Paraná E Aspectos Da Histologia De Culex quinquefasciatus (Say, 1823) (Diptera, Culicidae). Curitiba: Universidade Federal Do Paraná.
Codd, E. F. (1970). A relational model of data for large shared data banks. Commun. ACM 13:377. doi: 10.1145/362384.362685
Costa-Da-Silva, A. L., Ioshino, R. S., De Araujo, H. R. C., Kojin, B. B., Zanotto, P. M. D., Oliveira, D. B. L., et al. (2017a). Laboratory strains of aedes aegypti are competent to Brazilian zika virus. PLos One 12:e0171951. doi: 10.1371/journal.pone.0171951
Costa-Da-Silva, A. L., Ioshino, R. S., Petersen, V., Lima, A. F., Cunha, M. D. P., Wiley, M. R., et al. (2017b). First report of naturally infected aedes aegypti with chikungunya virus genotype ECSA in the Americas. PLoS Negl. Trop. Dis. 11:e0005630. doi: 10.1371/journal.pntd.0005630
Dobbin, K. K., Zhao, Y., and Simon, R. M. (2008). How large a training set is needed to develop a classifier for microarray data? Clin. Cancer Res. 14, 108–114. doi: 10.1158/1078-0432.ccr-07-0443
Dow, R., Coleman, P., Meadows, K., and Work, T. (1964). Isolation of St. Louis encephalitis viruses from mosquitoes in the tampa bay area of florida during the epidemic Of 1962. Am. J. Trop. Med. Hygiene 13, 462–468. doi: 10.4269/ajtmh.1964.13.462
Drãghici, S., Sellamuthua, S., and Khatria, P. (2006). Babel’s tower revisited: a universal resource for crossreferencing across annotation databases. Bioinformatics 22, 2934–2939. doi: 10.1093/bioinformatics/btl372
Dujardin, J. P. (2008). Morphometrics applied to medical entomology. Infect. Genet. Evol. 8, 875–890. doi: 10.1016/j.meegid.2008.07.011
Dujardin, J.-P. (2012). Morphometrics in Medical Entomology – Collection of Landmark for Identification and Characterization. Available online at: https://xyom-clic.eu/posts/ (accessed April 5, 2021).
Dujardin, J. P., Kaba, D., and Henry, A. B. (2010). The exchangeability of shape. BMC Res. Notes 3:266. doi: 10.1186/1756-0500-3-266
Evans, E. (2003). Domain-Driven Design: Tackling Complexity In The Heart Of Software. Boston MA: Addison Wesley.
Everest, G. (1976). Basic Data Structure Models Explained With A Common Example. Austin, TX: IEEE Computer Society Publications Office.
Fayyad, U., Piatetsky-Shapiro, G., and Smyth, A. P. (1996). From data mining toknowledge discovery indatabases. AI Magazine 17:37. Available online at: https://www.aaai.org/ojs/index.php/aimagazine/article/view/1230/1131 (accessed April 5, 2021).
Fernandes, R. S., Campos, S. S., Ferreira-de-Brito, A., Miranda, R. M., da Silva, K. A. B., de Castro, M. G., et al. (2016). Culex quinquefasciatus from Rio de Janeiro is not competent to transmit the local Zika Virus. PLoS Negl. Trop. Dis. 10:e0004993. doi: 10.1371/journal.pntd.0004993
Foley, D., Rueda, P., and Wilkerson, R. (2011). Vectormap. Available online at: http://vectormap.si.edu/ (accessed April 5, 2021).
Gaffigan, T., and Pecor, J. (1997). Collecting, Rearing, Mounting and Shipping Mosquitoes. Available online at: https://wrbu.si.edu/resources/protocols (accessed April 5, 2021).
Garros, C., and Dujardin, J.-P. (2013). “Genetic and phenetic approaches to anopheles systematics, anopheles mosquitoe – new insights into malaria vectors,” in Anopheles Mosquitoes – New insights Into Malaria Vectors Available online at: https://www.intechopen.com/books/anopheles-mosquitoes-new-insights-into-malaria-vectors/genetic-and-phenetic-approaches-to-anopheles-systematics (accessed April 5, 2021).
Gómez, G. F., Márquez, E. J., Gutiérrez, L. A., Conn, J. E., and Correa, M. M. (2014). Geometric morphometric analysis of colombian Anopheles albimanus (Diptera: Culicidae) reveals significant effect of environmental factors on wing traits and presence of a metapopulation. Acta Tropica 135, 75–85. doi: 10.1016/j.actatropica.2014.03.020
Gubler, D. J. (1991). “Insects in disease transmission,” in Hunter Tropical Medicine, 7th Edn, ed. G. T. Strickland (Philadelphia (PA): W. B. Saunders), 981–1000.
Guedes, D., Paiva, M., Donato, M., Barbosa, P., Krokovsky, L., Rocha, S., et al. (2017). Zika Virus replication in the mosquito culex quinquefasciatus in Brazil. Emerg. Microbes Infect. 6:e69.
Gurgel-Gonçalves, R., Komp, E., Campbell, L. P., Khalighifar, A., Mellenbruch, J., Mendonça, V. J., et al. (2017). Automated identification of insect vectors of chagas disease in Brazil and Mexico: the virtual vector lab. PeerJ 18:e3040. doi: 10.7717/peerj.3040
Henry, A., Thongsripong, P., Fonseca-Gonzalez, I., Jaramillo-Ocampo, N., and Dujardin, J. P. (2010). Wing shape of dengue vectors from around the world. Infect. Genet. Evol. 10, 207–214. doi: 10.1016/j.meegid.2009.12.001
Houle, D., Mezey, J., Galpern, P., and Carter, A. (2003). BMC Evol. Biol. 3:25. doi: 10.1186/1471-2148-3-25
IBM (2011). IBM’s 100 Icons of Progress – Relational Database. Available online at: https://www.ibm.com/ibm/history/ibm100/us/en/icons/reldb/ (accessed April 5, 2021).
Jaramillo, N., Dujardin, J. P., Calle-Londono, D., and Fonseca-Gonzalez, I. (2015). Geometric morphometrics for the taxonomy of 11 species of anopheles (Nyssorhynchus) mosquitoes. Med. Vet. Entomol. 29, 26–36. doi: 10.1111/mve.12091
Jirakanjanakit, N., and Dujardin, J. P. (2005). Discrimination of Aedes aegypti (Diptera: Culicidae) laboratory lines based on wing geometry. Southeast Asian J. Trop. Med. Public Health 36, 858–861.
Jirakanjanakit, N., Leemingsawat, S., and Dujardin, J. P. (2008). The geometry of the wing of Aedes (Stegomyia) aegypti in isofemale lines through successive generations. Infect. Genet. Evol. 8, 414–421. doi: 10.1016/j.meegid.2007.05.004
Jirakanjanakit, N., Leemingsawat, S., Thongrungkiat, S., Apiwathnasorn, C., Singhaniyom, S., Bellec, C., et al. (2007). Influence of larval density or food variation on the geometry of the wing of Aedes (Stegomyia) aegypti. Trop. Med. Int. Health 12, 1354–1360. doi: 10.1111/j.1365-3156.2007.01919.x
Jowett, T. (1986). “Preparation of nucleic acids,” in Drosophila A Practical Approach, ed. D. B. Roberts (Oxford: Roberts DB Press).
Kalayeh, H. M., and Landgrebe, D. A. (1983). Predicting the required number of training samples. IEEE Trans. Pattern Anal. Mach. Intell. 5, 664–667. doi: 10.1109/tpami.1983.4767459
Khalighifar, A., Komp, E., Ramsey, J. M., Gurgel-Gonçalves, R., and Peterson, A. T. (2019). Deep learning algorithms improve automated identification of chagas disease vectors. J. Med. Entomol. 56, 1404–1410. doi: 10.1093/jme/tjz065
Kim, S. Y. (2009). Effects of sample size on robustness and prediction accuracy of a prognostic gene signature. BMC Bioinform. 10:147. doi: 10.1186/1471-2105-10-147
Klingenberg, C. P. (2010). Evolution and development of shape: integrating quantitative approaches. Nat. Rev. Genet. 11, 623–635. doi: 10.1038/nrg2829
Klingenberg, C. P. (2011). Morphoj: an integrated software package for geometric morphometrics. Mol. Ecol. Resources 11, 353–357. doi: 10.1111/j.1755-0998.2010.02924.x
Laurito, M., Almirón, W. R., and Ludueña-Almeida, F. F. (2015). Discrimination of four culex (Culex) species from the Neotropics based on geometric morphometrics. Zoomorphology 1611, 447–455. doi: 10.1007/s00435-015-0271-x
Lee, H. L., and Rohani, A. (2005). Transovarial transmission of dengue virus in aedes aegypti and aedes albopictus in relation to dengue outbreak in an urban area in Malaysia. Dengue Bull. 29, 106–111.
Lopes, O. D., Sacchetta, L. D., Francy, D. B., Jakob, W. L., and Calisher, C. H. (1981). Emergence of a new arbovirus disease in Brazil. 3. isolation of rocio virus from Psorophora ferox (Humboldt, 1819). Am. J. Epidemiol. 113, 122–125. doi: 10.1093/oxfordjournals.aje.a113075
Lorenz, C., Almeida, F., Almeida-Lopes, F., Louise, C., Pereira, S. N., Petersen, V., et al. (2017). Geometric morphometrics in mosquitoes: what has been measured? Infect. Genet. Evol. 54, 205–215. doi: 10.1016/j.meegid.2017.06.029
Lorenz, C., and Suesdek, L. (2013). Short report: evaluation of chemical preparation on insect wing shape for geometric morphometrics. Am. J. Trop. Med. Hygiene 89, 928–931. doi: 10.4269/ajtmh.13-0359
Mackay, A., Kramer, W., Meece, J., Brumfield, R., and Foil, L. (2010). Host feeding patterns of culex mosquitoes (Diptera: Culicidae) in east baton rouge parish, louisiana. J. Med. Entomol. 47, 238–248. doi: 10.1603/me09168
Morales Vargas, R., Phumala-Morales, N., Tsunoda, T., Apiwathnasorn, C., and Dujardin, J. (2013). The phenetic structure of Aedes albopictus. Infect. Genet. Evol. 13, 242–251. doi: 10.1016/j.meegid.2012.08.008
Morales-Vargas, R. E., Ya-Umphan, P., Phumala-Morales, N., Komalamisra, N., and Dujardin, J. P. (2010). Climate associated size and shape changes in Aedes aegypti (Diptera: Culicidae) populations from Thailand. Infect. Genet. Evol. 10, 580–585. doi: 10.1016/j.meegid.2010.01.004
Motoki, M. T., Suesdek, L., Bergo, E. S., and Sallum, M. A. M. (2012). Wing geometry of Anopheles darlingi root (Diptera:Culicidae) in five major Brazilian ecoregions. Infect. Genet. Evol. 12, 1246–1252. doi: 10.1016/j.meegid.2012.04.002
Motta, D., Santos, A. ÁB., Machado, B. A. S., Ribeiro-Filho, O. G. V., Camargo, L. O. A., Valdenegro-Toro, M. A., et al. (2020). Optimization of convolutional neural network hyperparameters for automatic classification of adult mosquitoes. PLoS One 15:e0234959. doi: 10.1371/journal.pone.0234959
Mukherjee, S., Tamayo, P., Rogers, S., Rifkin, R., Engle, A., Campbell, C., et al. (2003). Estimating dataset size requirements for classifying DNA microarray data. J. Comput. Biol. 10, 119–142. doi: 10.1089/106652703321825928
Nigam, K., Mccallum, A. K., Thrun, S., and Mitchell, T. (2000). Text classification from labeled and unlabeled documents using EM. Machine Learn. 39, 103–134.
Noridah, O., Paranthaman, V., Nayar, S., Masliza, M., Ranjit, K., Norizah, I., et al. (2007). Outbreak of chikungunya due to virus of central/east african genotype in Malaysia. Med. J. Malaysia 62, 323–328.
Parsaye, K., and Chignell, M. (1993). Intelligent Database Tools And Applications: Hyperin-Formation Access, Data Quality, Visualization, Automatic Discovery, 1st Edn. Wiley Professional Computing; John Wiley & Sons. 560.
Pauvolid-Correa, A., Kenney, J. L., Couto-Lima, D., Campos, Z. M. S., Schatzmayr, H. G., Nogueira, R. M. R., et al. (2013). Ilheus virus isolation in the pantanal, west-central Brazil. PLoS Negl. Trop. Dis. 7:e2318. doi: 10.1371/journal.pntd.0002318
Phanitchat, T., Apiwathnasorn, C., Sungvornyothin, S., Samung, Y., Dujardin, S., Dujardin, J. P., et al. (2019). Geometric morphometric analysis of the effect of temperature on wing size and shape in Aedes albopictus. Med. Vet. Entomol. 33, 476–484. doi: 10.1111/mve.12385
Philip, C. B., and Rozenboom, L. E. (1973). “Medico-veterinary entomology: a generation of progress. history of entomology,” in History Of Entomology, eds R. F. Smith, T. E. Mittler, and C. N. Smith (Palo Alto (CA): Annual Reviews), 333–359.
Ramirez, C. C. L., and Dessen, E. M. B. (1994). Cytogenetic analysis of a natural-population of Anopheles cruzii. Revista Brasileira De Genetica 17, 41–46.
Richtsmeier, J. T., Deleon, V. B., and Lele, S. R. (2002). The promise of geometric morphometrics. Yearbook Phys. Anthropol. 45, 63–91.
Rohlf, F. J. (1993). Morphometric tools for landmark data - geometry and biology -bookstein, FL. J. Classif. 10, 133–136.
Ruangsittichai, J., Apiwathnasorn, C., and Dujardin, J. P. (2011). Interspecific and sexual shape variation in the filariasis vectors Mansonia dives and Ma. bonneae. Infect. Genet. Evol. 11, 2089–2094. doi: 10.1016/j.meegid.2011.10.002
Sallum, M. A. M., Foster, P. G., Dos Santos, C. L. S., Flores, D. C., Motoki, M. T., and Bergo, E. S. (2010). Resurrection of two species from synonymy of anopheles (Nyssorhynchus) strodei root, and characterization of a distinct morphological form from the strodei complex (Diptera: Culicidae). J. Med. Entomol. 47, 504–526. doi: 10.1093/jmedent/47.4.504
Sauer, F. G., Jaworski, L., Erdbeer, L., Heitmann, A., Schmidt-Chanasit, J., Kiel, E., et al. (2020). Geometric morphometric wing analysis represents a robust tool to identify female mosquitoes (Diptera: Culicidae) in Germany. Sci. Rep. 19:17613.
Savasere, A., Omiecinski, E., and Navathe, S. (1995). “An efficient algorithm for mining association rules in large databases,” in Proceedings of the 21st International Conference On Very Large Data Bases, New York NY: ACM 432–444.
Schlick-Steiner, B., Steiner, F., Seifert, B., Stauffer, C., Christian, E., and Crozier, R. (2010). Integrative taxonomy: a multisource approach to exploring biodiversity. Ann. Rev. Entomol. 55, 421–438. doi: 10.1146/annurev-ento-112408-085432
Shannon, R. C., Whitman, L., and Franca, M. (1938). Yellow fever virus in jungle mosquitoes. Science 88, 101–110.
Sonnenschein, A., Vanderzee, D., Pitchers, W. R., Chari, S., and Dworkin, I. (2015). An image database of drosophila melanogaster wings for phenomic and biometric analysis. Gigascience 4:25.
Souza, A. L., da, S., Multini, L. C., Marrelli, M. T., and Wilke, A. B. B. (2020). Wing geometric morphometrics for identification of mosquito species (Diptera: Culicidae) of neglected epidemiological importance. Acta Tropica. 211:105593. doi: 10.1016/j.actatropica.2020.105593
Spence, L., Anderson, C. R., Aitken, T. H., and Downs, W. G. (1962). Melao Virus, a new agent isolated from trinidadian mosquitoes. Am. J. Trop. Med. Hygiene 11, 687–690. doi: 10.4269/ajtmh.1962.11.687
Stephens, Z. D., Lee, S. Y., Faghri, F., Campbell, R. H., Zhai, C., Efron, M. J., et al. (2015). Big data: astronomical or genomical? PLoS Biol. 13:e1002195. doi: 10.1371/journal.pbio.1002195
Sudia, W. D. (1972). Arthropod Vectors Of Venezuelan Equine Encephalitis. Washington, DC: Pan American Healt Organization.
Sumruayphol, S., Apiwathnasorn, C., Ruangsittichai, J., Sriwichai, P., Attrapadung, S., Samung, Y., et al. (2016). DNA barcoding and wing morphometrics to distinguish three Aedes vectors in Thailand. Acta Tropica 159, 1–10. doi: 10.1016/j.actatropica.2016.03.010
Tadei, W. P., and Thatcher, B. D. (2000). Malaria vectors in the brazilian amazon: anopheles of the subgenus nyssorhynchus. Revista Do Instituto De Medicina Tropical De São Paulo 42, 87–94. doi: 10.1590/s0036-46652000000200005
Tam, V. H., Kabbara, S., Yeh, R. F., and Leary, R. H. (2006). Impact of sample size on the performance of multiple-model pharmacokinetic simulations. Antimicrobial Agents Chemotherapy 50, 3950–3952. doi: 10.1128/aac.00337-06
Unlu, I., Kramer, W., Roy, A., and Foil, L. (2010). Detection of west nile virus rna in mosquitoes and identification of mosquito blood meals collected at alligator farms in Louisiana. J. Med. Entomol. 47, 625–633. doi: 10.1093/jmedent/47.4.625
Valan, M., Makonyi, K., Maki, A., Vondráček, D., and Ronquist, F. (2019). Automated taxonomic identification of insects with expert-level accuracy using effective feature transfer from convolutional networks. Systematic Biol. 68, 876–895. doi: 10.1093/sysbio/syz014
Vasconcelos, P. F. C., Costa, Z. G., Travassos, Da Rosa, E. S., Luna, E., Rodrigues, S. G., et al. (2001). Epidemic of jungle yellow fever in Brazil, 2000: implications of climatic alterations in disease spread. J. Med. Virol. 65, 598–604. doi: 10.1002/jmv.2078
Vences, M., Guayasamin, J. M., Miralles, A., and De la Riva, I. (2013). To name or not to name: criteria to promote economy of change in linnaean classification schemes. Zootaxa 3636, 201–244. doi: 10.11646/zootaxa.3636.2.1
Vidal, P. O., Peruzin, M. C., and Suesdek, L. (2011). Wing diagnostic characters for Culex quinquefasciatus and Culex nigripalpus (Diptera, Culicidae). Revista Brasileira de Entomologia. 55, 134–137. doi: 10.1590/s0085-56262011000100022
Vidal, P. O., and Suesdek, L. (2012). Comparison of wing geometry data and genetic data for assessing the population structure of Aedes aegypti. Infect. Genet. Evol. 12, 591–596. doi: 10.1016/j.meegid.2011.11.013
Virginio-Fonseca, F. (2018). Morfometria geométrica e banco de dados na investigação de problemas biológicos em Culicidae [tese]. São Paulo: Instituto de Ciências Biomédicas, doi: 10.11606/T.42.2019.tde-06062018-6150917
Virginio, F., Vidal, P. O., and Suesdek, L. (2015). Wing sexual dimorphism of pathogen-vector culicids. Parasites Vectors 8:769.
Weeks, P. J. D., O’Neill, M. A., Gaston, K. J., and Gauld, I. D. (1999). Automating insect identification: exploring the limitations of a prototype system. J. Appl. Entomol. 123, 1–8. doi: 10.1046/j.1439-0418.1999.00307.x
Wiley, E. O., and Liebermann, B. S. (2011). Phylogenetic Systematics, 2 Edn. 432. Hoboken, NJ: Wiley online library
Wilke, A. B. B., Christe, R. D., Multini, L. C., Vidal, P. O., Wilk-Da-Silva, R., De Carvalho, G. C., et al. (2016). Morphometric wing characters as a tool for mosquito identification. PLoS One 11:e0161643. doi: 10.1371/journal.pone.0161643
Wilkerson, R. C., Linton, Y. M., Fonseca, D. M., Schultz, T. R., Price, D. C., and Strickman, D. A. (2015). Making mosquito taxonomy useful: a stable classification of tribe aedini that balances utility with current knowledge of evolutionary relationships. PLos One 10:e0133602. doi: 10.1371/journal.pone.0133602
World Health Organization (2020b). World Malaria Report 2020: 20 Years of Global Progress and Challenges. Geneva: World Health Organization.
Keywords: relational database, open source, vector-borne disease, public health, medical entomology, geometric morphometric approach, integrative taxonomic approach
Citation: Virginio F, Domingues V, da Silva LCG, Andrade L, Braghetto KR and Suesdek L (2021) WingBank: A Wing Image Database of Mosquitoes. Front. Ecol. Evol. 9:660941. doi: 10.3389/fevo.2021.660941
Received: 30 January 2021; Accepted: 22 March 2021;
Published: 16 April 2021.
Edited by:
Jader Oliveira, University of São Paulo, BrazilReviewed by:
Tanawat Chaiphongpachara, Suan Sunandha Rajabhat University, ThailandRodrigo Gurgel-Gonçalves, University of Brasilia, Brazil
Copyright © 2021 Virginio, Domingues, da Silva, Andrade, Braghetto and Suesdek. This is an open-access article distributed under the terms of the Creative Commons Attribution License (CC BY). The use, distribution or reproduction in other forums is permitted, provided the original author(s) and the copyright owner(s) are credited and that the original publication in this journal is cited, in accordance with accepted academic practice. No use, distribution or reproduction is permitted which does not comply with these terms.
*Correspondence: Flávia Virginio, ZmxhdmlhLnZpcmdpbmlvQGJ1dGFudGFuLmdvdi5icg==
†These authors have contributed equally to this work and share first authorship
‡These authors have contributed equally to this work and share last authorship