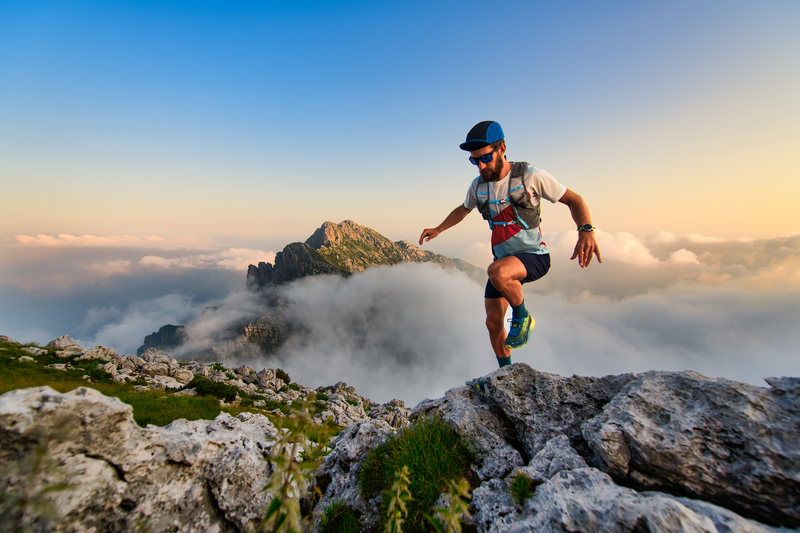
95% of researchers rate our articles as excellent or good
Learn more about the work of our research integrity team to safeguard the quality of each article we publish.
Find out more
ORIGINAL RESEARCH article
Front. Ecol. Evol. , 13 April 2021
Sec. Biogeography and Macroecology
Volume 9 - 2021 | https://doi.org/10.3389/fevo.2021.641686
This article is part of the Research Topic Invaders on the Horizon! Scanning the Future of Invasion Science and Management View all 16 articles
Invasive alien species (IAS) are a significant component of global changes, causing severe economic and biodiversity damage. In this regard, Hakea sericea is one of the most widespread IAS throughout the Mediterranean region, including Portugal. The difficulty surrounding its management is exacerbated by post-fire situations, signifying a challenging task for managers. To assist in this effort, we used a system dynamic approach to model the population dynamics of Hakea sericea regarding the combinations of wildfire risk and control scenarios, which differ in periodicity, type of interventions, and cohort age. The ultimate goal of this study was to assess the effectiveness and costs of control efforts at reducing the abundance of this IAS. A Natura 2000 site Alvão/Marão (code PTCON0003) in northern Portugal, severely invaded by Hakea sericea, served as the study site. The modeling results demonstrate that Hakea sericea is likely to continue spreading if left uncontrolled. Although it may not be possible to ensure eradication of Hakea sericea from the study, repeated control actions aimed at the entire IAS population could be very effective in reducing its area. From a practical standpoint, removing all plants 24 months after each fire event followed by subsequent monitoring appears to be the most cost-effective strategy for managing Hakea sericea. Considering the modeling results, the dynamic modeling framework developed is a versatile, instructive tool that can support decision-making aimed at effective management of Hakea sericea.
Changing weather patterns and the speed of these changes exert a direct influence on the ecosystems, including the ability of exotic species to become established and invasive when introduced into a new environment. Invasive alien species (IAS) are widespread phenomena recognized as one of the main global threats to biodiversity (Early et al., 2016), with escalating impacts at ecological, economic, and human health levels (Hulme, 2006; Simberloff et al., 2013; Marbuah et al., 2014). To tackle this increasing problem, the European Commission published a dedicated Regulation (no. 1143/2014) on IAS that focuses on the need to take management measures for IAS that are widespread (European Union, 2014). Traditionally, IAS management in natural ecosystems has focused on removing the target invader under the assumption that its impacts would dissipate and the ecosystem would recover after its removal (Pearson and Ortega, 2009), but this is not always realistic or feasible (Zavaleta et al., 2001; Marchante et al., 2011). The extent and rate of ecosystem recovery depend on the propagule pressure, type, and frequency of disturbance, among other factors (Foxcroft et al., 2011). Due to the complexity of external drivers influencing IAS spread and impact (Hulme, 2006), IAS control and management is challenging and costly, both economically and environmentally (Hyder et al., 2008; Hulme, 2009), which raises concerns for managers, conservationists, and other stakeholders working with IAS. The interaction between the most relevant ecological components during the invasion process generates significant and increasingly complex influences on the ecosystems (Le Roux et al., 2020), which further complicates IAS management.
Given that it is impractical to deal with all invasive species and invaded populations at once, prioritization of actions is an important strategy to support cost-effective resource allocation (Krug et al., 2010; Gallardo and Aldridge, 2013) and is essential for successful IAS management (McGeoch et al., 2016; Lohr et al., 2017). One of the tools applied in decision-making is ecological modeling, which can be implemented at a relatively low cost in terms of money, effort, and capacity to recreate hypothetical management scenarios (Day et al., 2018). These tools have become indispensable in better understanding, predicting, and controlling biological invasions (e.g., Broenniman and Guisan, 2008; Buchadas et al., 2017). The need for correctly addressing uncertainty, which is inherent to any invasion process (Lewis et al., 2016), has fostered the application of dynamic modeling approaches (e.g., Buchadas et al., 2017). Dynamic models can capture the complexity of interactions among key ecological components by combining environmental conditions, effects of time, and stochastic factors that are difficult to understand otherwise (Jørgensen, 1999). Therefore, this type of models has the potential to support decision-making in IAS management (Santos et al., 2015; Büyüktahtakın and Haight, 2018), for example, for risk evaluation (Guisan et al., 2013), spread dynamics (Ferrari et al., 2014; Martins et al., 2016), management effectiveness (Hyder et al., 2008; Chalak et al., 2011; Portela et al., 2020) of IAS, and restoration of invaded ecosystems (Hall et al., 2020). System dynamics (SD) is a process-based and problem-oriented modeling able to study, analyze, and visualize the behavior of complex systems, in which time is a critical component (Mashaly and Fernald, 2020). This methodology considers the relationships between variables and subsystems, providing insights into potential consequences of system perturbations and future uncertainties (Mirchi et al., 2012). In SD, the system structure is composed of state variables and flows which influence each other, including feedback mechanisms (Mashaly and Fernald, 2020) that attempt to capture the structural functioning in systems affected by long-term environmental changes, such as the impacts resulting from the IAS (Buchadas et al., 2017). In this sense, SD modeling is an excellent tool for solving a range of specific environmental problems (Turner et al., 2016), including those related to IAS management (BenDor and Metcalf, 2006).
It is widely recognized that IAS control and management are costly endeavors, and in the majority of situations, it is an important barrier to successful IAS control (Kettenring and Adams, 2011; Dana et al., 2019). Therefore, a better understanding of using the scarcity of resources is essential in the decision-making process. The difficulty in measuring costs, its context-dependence (IUCN, 2018), associated with the complex nature of invasion dynamics (Epanchin-Niell, 2017) make it a challenging (Kerr et al., 2016; Reyns et al., 2018), but necessary task for improving long-term management of IAS. Here, we develop a SD model for the analysis of the effectiveness and costs of control efforts of Hakea sericea (silky hakea), one of the worst woody IAS in Portugal. In South Africa, where this IAS has been problematic for over 100 years, biological control with several agents (Gordon and Fourie, 2011) has proven to be the most successful method. Still, it is not yet an option in Europe. In the country, the use of the “fell and burn” technique, which comprises felling of adult plants, leaving them for 12–18 months and then burning the vegetation, also proved to be a very effective control method, leaving minimal follow-up (Esler et al., 2010). However, in Portugal, the use of this methodology is scarce due to the limited knowledge on the interactions between fire, ecosystem, and the IAS. Control of this IAS relies almost exclusively on mechanical removal (e.g., chainsaws, brush cutters, skid steer brush cutters, and bulldozers), which is hugely expensive. To help managers design effective and efficient allocation of resources, we model the impact of wildfires associated with several control options on the abundance of Hakea sericea and control costs. Although previous studies have documented that fire is a key driver Hakea sericea invasion, its influence on the success of control efforts is not explored, and this is the primary focus of the present work. A sensitivity analysis (SA) was also carried out to explore the effect of uncertainty on the abundance of Hakea sericea. We apply the SD model to the management of Hakea sericea in a Natura 2000 site (Alvão/Marão, Northern Portugal), where invasion by this IAS is particularly relevant due to being a management priority. We expect that the outputs of the proposed modeling framework will provide crucial information on the potential magnitude of Hakea sericea invasion in a study area and contribute to the implementation of spatial-temporal management scenarios and strategies aimed at effective long-term management of Hakea sericea.
Hakea sericea (Proteaceae) is native to south-eastern Australia and has become invasive in South Africa, New Zealand, and several European countries, such as Portugal, France, and Spain (EPPO, 2017). This serotinous species can form dense, extensive, almost monospecific stands that can alter vegetation composition and structure (Richardson et al., 1989). In South Africa, in Cape fynbos vegetation, dense stands of Hakea sericea lead to significant reductions in cover (van Wilgen and Richardson, 1985) and richness (Richardson et al., 1989) of native species. Its impacts also include alterations of fuel properties and abundance, which can modify the fire regime (van Wilgen and Richardson, 1985). The expansion of this IAS is intimately linked to fire, which stimulates the release and scatters of winged seeds that germinate in the post-fire environment (Wilson et al., 2020). In Portugal, Hakea sericea generally flowers in its third year of life, during winter, between December and February. Fruit development, which becomes visible in late February, continues for several months and mature fruits emerge in June (personal observations).
The Alvão/Marão Natura 2000 network, located in the north of mainland Portugal (41°39′N, 7°83′W), was selected as the study area. The vegetation in this area is predominated by oaks (Quercus robur and Quercus pyrenaica), pines (Pinus pinaster), and a variety of shrub and herb species, mainly belonging to the genera Erica and Ulex. At the beginning of the 20th century, Hakea sericea seedlings were purposefully planted in the area to form mature hedges. The occurrence of wildfires and insufficient management practices facilitate its spread. Major increases in the Hakea sericea invasion occurred after 2013 as a consequence of an intense wildfire. Nowadays, this IAS occupies different altitudinal zones, especially those previously dominated by Pinus pinaster. It occurs in dense stands (>75% cover) and as isolated individuals dispersed in the area.
The climate in the study area is of Mediterranean type with an Atlantic influence (Csb in the Köppen classification). Data from the closest weather station (41°18′N, 7°44′W) indicates that the mean annual precipitation during 1981-2010 was 1,023 mm, being more abundant in autumn and winter. The average temperatures range from 6.3 (January) to 21.7°C (August), and the mean maximum (28.6°C) and minimum (2.8°C) temperature occur in August and January, respectively.
A SD framework focusing on the post-fire dynamics of Hakea sericea was developed within the software STELLA (iSEE systems Inc., Version 9.0.3). The model comprises five interactive sub-models, respectively, pertaining to the vegetation ecological succession, population dynamics of Hakea sericea, fire events and burnt area dynamics, management control efforts, and cost estimates, as shown on the conceptual diagram (Figure 1). The model runs on a monthly time step for 50 years to assist the long-term management of this IAS. The fire and control efforts sub-models can be included (turned-on) or excluded (turned-off) in each simulation run.
Figure 1. General conceptual diagram with the structure and organization of the sub-models: (A) fire occurrence and extent, (B) vegetation ecological succession, (C) population dynamics of Hakea sericea, (D) management of control efforts, and (E) cost estimates. The fire and control sub-models influence interactively the competitive process between the Hakea sericea and the other vegetation cover dynamics, as indicated by bold arrows.
The dynamics of vegetation and Hakea sericea sub-models aim to recreate the changes in vegetation structure (i.e., herbs, shrubs, and trees) and composition of the invaded population [seedlings, young plants with no fruits (age < 3 years old), and adult plants (age > 3 years old)] over time in response to fire and control efforts. In both sub-models, the post-fire succession was based on the temporal rates that reproduce the number of months needed by each class to reach the respective dominance (Bastos et al., 2016). Data used for parameterization of the post-fire succession of vegetation was compiled from Moreira et al. (2001) and Mouillot et al. (2005), whereas the parameterization of the post-fire succession of Hakea sericea population was based on EPPO (2017) and Le Maitre et al. (2008).
The sub-model of fire occurrence and extent characterizes the likelihood of fire occurrence based on temperature and precipitation as the two most crucial weather determinants, obtained from the closest weather station for the 1981–2010 period. In the model, we assumed that mean monthly temperatures exceeding 15°C and monthly precipitation below 20 mm create conditions favorable to fire, according to the fire statistics made available by the ICNF (2017). This sub-model also had in account the fire frequency based on the average number of fire events of the study area, and fire extent, determined using a random number between 0.1 (low fire extent) and 1.0 (extreme fire extent).
In the design of the management of control efforts sub-model, we considered the technique commonly used to control Hakea sericea in the study area, which consists of mechanical felling of young and adult plants. The sub-model also considers the timing of occurrence (before or after a fire), frequency (i.e., removal interval), intensity (i.e., the proportion of plants removed per control event, which can assume values between 0 and 1.0, where 1.0 means that all plants were removed), and additional follow-up monitoring.
The cost estimates sub-model addresses the expense invested in each control effort per hectare. It was determined by the product of the area subjected to control (after proper conversion to ha) and the cost/ha of the control method used. For simplicity, we considered four control methods (initial removal of adult plants using heavy equipment, removal of young plants and dead plant matter after a fire event using brushcutters, removal of young plants using brushcutters, and hand-pulling of young plants). The costs of each method, in Euros/ha, were based on actual quotes provided by local contractors.
In total, the proposed modeling framework includes fourteen dynamic state variables (Table 1), divided into five main groups, with one variable related to the occurrence of fire, three variables related to the vegetation structure (herbs, shrubs, and trees), three variables based on Hakea sericea age stratification, represented by three life stage cohorts (seedlings, young plants, and adult plants), two variables associated to the control of young and adult plants, and, finally, three variables related to the cost estimates. Specification of the state variables included in the model is presented in Table 1. The initial values of these variables were based on literature knowledge and pre-existing field data of the study area. The full explanation of processes (Supplementary Appendix 1), equations (Supplementary Table 1), and variables (Supplementary Table 2) included in the model construction are available as Supplementary Electronic Material.
Table 1. Specification of the state variables included into the model construction, their description, initial values, and measure units.
In order to reduce the computational complexity of the simulation exercise and to accommodate more realistic management practices, we assumed the following conditions: (i) control of adult plants of Hakea sericea occurs only once, at the beginning of the simulation period or 12, 24, or 36 months after each fire event; (ii) control of young plants of Hakea sericea occurs only after a fire event; (iii) control of young and adult plants occur at an intensity of 1.0; (iv) follow-up monitoring occurs 10 months after each post-fire control. We also included a management scenario where no control option was applied (scenario 1). In total, twelve management scenarios were simulated, consisting of combinations of age cohorts (young plants vs. adult plants), frequencies of post-fire removal (0, 12, 24, and 36 months after a fire event), and the execution or not of follow-up monitoring (Table 2).
For each scenario, 25 independent stochastic simulations were carried out for the simulation period, and the average values of the abundance of Hakea sericea (%) and costs were calculated.
Statistical analysis was performed using the software package IBM SPSS version 26 for Windows (Orchard Road-Armonk, NY, United States). Management scenarios were compared in terms of efficacy and costs using a one-way analysis of variance (ANOVA), followed by Duncan’s multiple range test, at a 95% confidence level.
To evaluate how changes in the main parameters affected the estimated efficacy of each management scenario, a local SA by one-parameter-at-a-time technique (OAT) (Czitrom, 1999) was performed. For this, the different plant cover conversion rates and number estimates of fire events were adjusted with changes of ±10 and ±50% from the original values (Ligmann-Zielinska, 2013) and the results with and without variation (reference results) were expressed in percentage of each state variable variation. The results are positive or negative, considering the response trend of the selected state variables, representing the percentages of change in the Hakea sericea abundance between simulations with and without variation in the parameter under study. The percentage absolute value represents the distance to the state variables’ reference results. Implementation of the OAT technique in the current work required 14 model simulations for each management scenario.
The results of scenario 1 showed that unplanned fires altered the structure and composition of the vegetation, creating favorable conditions for the spread of Hakea sericea. At the beginning of the simulation period, Hakea sericea comprised 20% of the total vegetation of a sampling area and, after 25 simulations throughout 50 years, this proportion increased by 30% (Table 3). In the same period, herbaceous vegetation increased from 20 to 34%, at the expense of the other growth forms, whose relative abundance decreased. The occurrence of wildfires caused significant tree abundance loss, which almost disappeared within 50 post-fire years.
Table 3. Proportion of plant cover areas (abundance) at the beginning and at the end of the simulation period (50 years) under the occurrence of wildfires.
The primary goal of any management plan for invasive species is to eliminate or reduce its population. The results yielded by the twelve management scenarios considered in this study (that differ in time between control efforts, cohort age, and inclusion of follow-up monitoring) were very heterogeneous. The majority of them did not produce desired outcomes (Figure 2).
Figure 2. Box and whisker plot of the relative abundance of Hakea sericea (%) after 50 independent simulations for the tested management scenarios. Different lowercase letters indicate significant differences (P < 0.05) between management scenarios. The dashed line at 20% indicates the initial abundance of Hakea sericea.
The absence of control measures (scenario 1), as well as the low frequency of rounds of control (scenarios 2 and 5), had counterproductive effects since it allowed the IAS to increase in abundance (Figure 2). Removal of all plants 36 months after a fire event (scenario 10) caused a minimal decline in the relative abundance of Hakea sericea. In contrast, increasing the frequency of control efforts to 12 of 24 months after a fire event resulted in pronounced decreases in Hakea sericea abundance (Figure 2). Of the eight possible management strategies, half of them focused only on the control of young plants to prevent them from reaching reproductive maturity (scenarios 3, 4, 6, and 7). In comparison, the remaining four strategies also included control of adult plants (scenarios 8, 9, 11, and 12). When control efforts were only employed on young plants, the Hakea sericea abundance decreased by around 90%, stand about 3–4%, at the final of the simulation period. In turn, removing young and adult plants of Hakea sericea 12 or 24 months after a fire event and follow-up monitoring at 10 months after the first intervention (scenarios 11 and 12) significantly increased control efficacy, keeping its abundance below 0.5%.
The estimated costs associated with each management scenario were also determined, and the results are shown in Figure 3. The economic costs of controlling Hakea sericea were highly variable, ranging from 650 €/ha to approximately 11,000 €/ha. Less expensive strategies included those referred in scenarios 2 (650 €/ha), 5 (3,100 €/ha), 4 and 7 (around 6,000 €/ha), but had limited controlling effects on Hakea sericea. In contrast, scenarios 11 and 12, which successfully brought the species down to abundances lower than 1%, presented control costs around 10,000 euros/ha. Similar economic costs (P < 0.001) were observed in scenarios 6, 8, and 9 but with less efficiency.
Figure 3. Estimated costs (Euros/ha) of the tested management scenarios. Data are means ± standard deviation. Different lowercase letters indicate significant differences (P < 0.05) between management scenarios.
The results from the OAT SA (Supplementary Appendix) showed that, without control management, the parameters related to the temporal conversion rates of seedlings and young plants of Hakea sericea cause the main changes in the Hakea sericea abundance. In turn, the number estimates of fire events were the parameter with the primary influence on the outputs of almost all selected management scenarios.
The dynamic model developed in this study allowed us to determine the best management strategy for minimizing the Hakea sericea abundance in the presence of stochastic unplanned fires and different management strategies. According to the current fire regime in the study area and in the absence of control measures aimed at Hakea sericea, the abundance of this IAS may increase in the coming decades. The large increase in relative abundance yielded by the model (about 30% more than the initial value) suggests that Hakea sericea will take advantage of fire disturbances. This finding can be partially explained by the extraordinary capacity of this species to release its seeds after a fire event. Hakea sericea possesses a large aerial seedbank composed of heat-resistant fruits accumulated throughout its lifetime (Brown and Whelan, 1999), resulting in high propagule pressure, typical of successful invaders (Simberloff, 2009). Fire occurrence generally results in the opening of fruits and the release of abundant seeds within a few days (personal observations), facilitating its establishment and invasion in the absence of canopy cover and/or ground layer vegetation. Moreover, the seeds can also be dispersed by the wind across a wide area, supporting a quick spread of the species (Richardson et al., 1987; EPPO, 2017). Similar IAS expansion after a fire has been documented for other invasive species, such as Acacia sp. (Souza-Alonso et al., 2017), Pinus radiata (Richardson and Brown, 1986), Arundo donax (Coffman et al., 2010), Chromolaena odorata (Dew et al., 2017), or Cenchrus ciliaris L. syn Pennisetum ciliare (L.) Link (Jarnevich et al., 2019). From an economic point of view, curbing such expansion will require more resources, thus increasing management costs. The increasing post-fire abundance of Hakea sericea will also have detrimental effects on the habitat composition and structure and native plants’ succession. In particular, our results highlighted a reduction of shrub vegetation and a poor representation of tree species after the simulation period, reflecting long recovery times for this lifeform. In addition, it creates favorable opportunities for Hakea sericea to establish and spread. The existence of undesired synergies between disturbances, such as fire and IAS, supports the importance of incorporating such stochastic effects when making management decisions.
In our study, none of the management strategies tested resulted in the total eradication of Hakea sericea. This fact is not surprising since complete eradication has been most successful only at early invasion stages or in small islands (Gherardi and Angiolini, 2007). For IAS that are widespread and abundant, as Hakea sericea is in Portugal, the management goal is generally to reduce their populations to a level that would substantially reduce their ecological impact (Prior et al., 2018; Nunes et al., 2020). The management strategies tested here yielded different outcomes depending upon the frequency of control efforts, and the age of the plants controlled. Our findings indicate that it is more effective to concentrate efforts on controlling the entire population rather than focusing only on a specific age cohort. Similarly, controlling only IAS at the early stages of development (young plants), although helpful in preventing the development of a seedbank and thus the establishment and spread of this IAS, was not effective in reducing the overall abundance of Hakea sericea at the end of the simulation period. As a consequence of poor treatment efficacy, future management resources will be needed. This goal is more likely to be achieved when the entire invasive population (young and adult plants) is controlled at short time intervals after a fire event.
Tang et al. (2010) concluded that longer periods between control efforts result in lower management efficacy, and our results corroborated this assertion. Our 50-years simulation showed that Hakea sericea abundance was significantly reduced with more frequent control efforts, i.e., at 12 to –24 month intervals. Hakea sericea generally produces viable seeds at 3-year-old. For this reason, it is reasonable to argue that differences in management efficiency between 1 or 2 and 3-year intervals should coincide with the maturity of plants, indicating that understanding the biology of the target IAS is vital for making the right management decisions. The same conclusion was drawn by Dew et al. (2017) when evaluated the seasonal efficacy of clearing Chromolaena odorata. These authors concluded that clearing efficacy was higher when executed during flowering season than during seed dispersal.
Hakea sericea quickly germinates after fires, emphasizing the importance of monitoring the growth of the new generation of plants. Our results indicate that when follow-up monitoring was included in the model, the control efficacy increased. This finding is in accordance with other studies (Lindenmayer et al., 2015; Dew et al., 2017), which have also shown that follow-up control is essential for maintaining the IAS at low levels. In our work, complete eradication of Hakea sericea is unlikely to occur, suggesting that additional follow-up treatments will be necessary. Generally, this operation would involve more resource allocation (Marais and Wannenburgh, 2008), which, in some situations, could be neglected, leading to the proliferation of the IAS with consequent unsuccessful results of management plans (Cheney et al., 2019). According to our results, when follow-up monitoring was done as a complement activity of post-fire control of Hakea sericea, the estimated costs tend to be slightly higher. However, it is also expected that the economic impact of successive follow-up treatments will dissipate over time (Marais and Wannenburgh, 2008).
Our simulation results clearly show that the economic resources’ requirements for the control of Hakea sericea are directly dependent on the control efficacy of the management strategy. Therefore, it is crucial that resources are allocated effectively. Strategies focused on a single control event, although less expensive, were ineffective and could contribute to perpetuating Hakea sericea persistence in the study area. On the contrary, strategies focused on controlling burnt areas within 12 or 24 months after fire with follow-up operations declined significantly the abundance of the IAS, but required more resources. The SA demonstrated that an increment of fires in the study area was strongly associated with decreases in Hakea sericea abundance, highlighting the importance of adjusting the management strategies with the particularities of the study area. However, due to the fire-prone characteristics of this IAS, continuous follow-up monitoring will be necessary.
When analyzing the overall performance and costs of all scenarios, control efforts implemented 24 months after a fire event followed by subsequent monitoring seemed to be the most cost-effective way of managing Hakea sericea, since it yielded the same benefits as the annual control, at a similar cost.
The SD framework developed as a part of this investigation can be adopted to support decision-making in IAS management since the simulation results reproduce realistically the dynamics of plant structural composition and are respond with credibility throughout contrasting scenarios. It provides crucial information about the temporal dynamics of the invaded population and the efficacy of several management strategies under the risk of unplanned fires. Complete eradication of Hakea sericea is unlikely within the next 50 years. Nonetheless, combining control efforts with the occurrence of fires can greatly improve the control efficacy of this problematic IAS. Control efforts performed at 2-years intervals followed by continuous monitoring can substantially reduce the abundance of this IAS, to very low levels, below 0.5%, compared to the current situation of 25% of abundance. Due to the simplicity of the framework, it can be easily adapted to other areas by adjusting its parameters to the peculiarities of each study site. Therefore, we highlight the interplay between model-based research and ecological monitoring to anticipate, with scientific credibility, the ecological responses associated with the control of IAS and test the effectiveness of ongoing management programs.
The original contributions presented in the study are included in the article/Supplementary Material, further inquiries can be directed to the corresponding author.
MM and JC conceived and designed the simulation model. MM analysed the data and wrote the manuscript with contributions from all other authors. All authors have read and approved the submitted version of the manuscript.
This work was supported by national funds by Portuguese Foundation for Science and Technology (FCT), under the project UIDB/04033/2020. MM is supported by a post-doctoral grant (SFRH/BPD/103604/2016) from FCT.
The authors declare that the research was conducted in the absence of any commercial or financial relationships that could be construed as a potential conflict of interest.
We would like to thank Paula Morais from Instituto de Conservação da Natureza e Florestas (ICNF) for providing field data for the work. We also grateful to Rita Bastos for her assistance with the sensitivity analysis and to three reviewers for their helpful comments.
The Supplementary Material for this article can be found online at: https://www.frontiersin.org/articles/10.3389/fevo.2021.641686/full#supplementary-material
Bastos, R., D’Amen, M., Vicente, J., Santos, M., Yu, H., Eitelberg, D., et al. (2016). A multi-scale looping approach to predict spatially dynamic patterns of functional species richness in changing landscapes. Ecol. Indic. 64, 92–104. doi: 10.1016/j.ecolind.2015.12.025
BenDor, T. K., and Metcalf, S. S. (2006). The spatial dynamics of invasive species spread. Syst. Dyn. Rev. 22, 27–50. doi: 10.1002/sdr.328
Broenniman, O., and Guisan, A. (2008). Predicting current and future biological invasions: Both native and invaded ranges matter. Biol. Lett. 4, 585–589. doi: 10.1098/rsbl.2008.0254
Brown, C. L., and Whelan, R. J. (1999). Seasonal occurrence of fire and availability of germinable seeds in Hakea sericea and Petrophile sessilis. J. Ecol. 87, 932–941. doi: 10.1046/j.1365-2745.1999.00401.x
Buchadas, A., Vaz, A. S., Honrado, J. P., Alagador, D., Bastos, R., Cabral, J. A., et al. (2017). Dynamic models in research and management of biological invasions. J. Environ. Manag. 196, 594–606. doi: 10.1016/j.jenvman.2017.03.060
Büyüktahtakın, I. E., and Haight, R. G. (2018). A review of operations research models in invasive species management: state of the art, challenges, and future directions. Ann. Oper. Res. 271, 357–403. doi: 10.1007/s10479-017-2670-5
Chalak, M., Ruijs, A., and vand an Ierland, E. C. (2011). Biological control of invasive plant species: A stochastic analysis. Weed Biol. Manag. 11, 137–151. doi: 10.1111/j.1445-6664.2011.00412.x
Cheney, C., Esler, K. J., Foxcroft, L. C., and van Wilgen, N. J. (2019). Scenarios for the management of invasive Acacia species in a protected area: Implications of clearing efficacy. J. Environ. Manage. 238, 274–282. doi: 10.1016/j.jenvman.2019.02.112
Coffman, G. C., Ambrose, R. F., and Rundel, P. W. (2010). Wildfire promotes dominance of invasive giant reed (Arundo donax) in riparian ecosystems. Biol. Invasions 12, 2723–2734. doi: 10.1007/s10530-009-9677-z
Czitrom, V. (1999). One-factor-at-a-time versus designed experiments. Am. Stat. 53, 126–131. doi: 10.1080/00031305.1999.10474445
Dana, E. D., García-de-Lomas, J., Verloove, F., and Vilà, M. (2019). Common deficiencies of actions for managing invasive alien species: a decision-support checklist. NeoBiota 48, 97–112. doi: 10.3897/neobiota.48.35118
Day, C. C., Landguth, E. L., Bearbin, A., Holden, Z. A., and Whiteley, A. R. (2018). Using simulation modeling to inform management of invasive species: A case study of eastern brook trout suppression and eradication. Biol. Conserv. 221, 10–22. doi: 10.1016/j.biocon.2018.01.017
Dew, L. A., Rozen-Rechels, D., le Roux, E., Cromsigt, J. P. G. M., and te Beest, M. (2017). Evaluating the efficacy of invasive plant control in response to ecological factors. S. Afr. J. Bot. 109, 203–213. doi: 10.1016/j.sajb.2016.12.007
Early, R., Bradley, B., Dukes, J., Lawler, J. J., Olden, J. D., Blumenthal, D. M., et al. (2016). Global threats from invasive alien species in the twenty-first century and national response capacities. Nat. Commun. 7, 12485. doi: 10.1038/ncomms12485
Epanchin-Niell, R. S. (2017). Economics of invasive species policy and management. Biol. Invasions 19, 3333–3354. doi: 10.1007/s10530-017-1406-4
Esler, K. J., van Wilgen, B. W., Te Roller, K. S., Wood, A. R., and van der Merwe, J. H. (2010). A landscape−scale assessment of the long−term integrated control of an invasive shrub in South Africa. Biol. Invasions 12, 211. doi: 10.1007/s10530-009-9443-2
European Union (2014). Regulation (EU) No 1143/2014 of the European Parliament and of the Council of 22 October 2014 on the prevention and management of the introduction and spread of invasive alien species. Off. J. Eur. Union 57, 35–55.
Ferrari, J. R., Preisser, E. L., and Fitzpatrick, M. C. (2014). Modeling the spread of invasive species using dynamic network models. Biol. Invasions 16, 949–960. doi: 10.1007/s10530-013-0552-6
Foxcroft, L. C., Pickett, S. T. A., and Cadenasso, M. L. (2011). Expanding the conceptual frameworks of plant invasion ecology. Perspect. Plant Ecol. Evol. Syst. 13, 89–100. doi: 10.1016/j.ppees.2011.03.004
Gallardo, B., and Aldridge, D. C. (2013). Priority setting for invasive species management: risk assessment of Ponto-Caspian invasive species into Great Britain. Ecol. Appl. 23, 352–364. doi: 10.1890/12-1018.1
Gherardi, F., and Angiolini, C. (2007). “Eradication and control of invasive species,” in Biodiversity conservation and habitat management, Encyclopaedia of life support systems, eds F. Gherardi, C. Corti, and M. Gualtieri (Oxford: Eolss Publishers), 274–302.
Gordon, A. J., and Fourie, A. (2011). Biological control of Hakea sericea Schrad. & J.C. Wendl. and Hakea gibbosa (Sm.) Cav. (Proteaceae) in South Africa. Afr. Entomol. 19, 303–314.
Guisan, A., Tingley, R., Baumgartner, J. B., Naujokaitis-Lewis, I., Sutcliffe, P. R., Tullock, A. I. T., et al. (2013). Predicting species distributions for conservation decisions. Ecol. Lett. 16, 1424–1435. doi: 10.1111/ele.12189
Hall, S. A., Bastos, R., Vicente, J. R., Holmes, P. M., Gaertner, M., Esler, K. J., et al. (2020). A dynamic modelling tool to anticipate the effectiveness of invasive plant control and restoration recovery trajectories in South African Fynbos. Restor. Ecol. 29, 13324. doi: 10.1111/rec.13324
Hulme, P. E. (2006). Beyond control: Wider implications for the management of biological invasions. J. Appl. Ecol. 43, 835–847. doi: 10.1111/j.1365-2664.2006.01227.x
Hulme, P. E. (2009). Trade, transport and trouble: managing invasive species pathways in an era of globalization. J. Appl. Ecol. 46, 10–18. doi: 10.1111/j.1365-2664.2008.01600.x
Hyder, A., Leung, B., and Miao, Z. (2008). Integrating data, biology, and decision models for invasive species management: application to leafy spurge (Euphorbia esula). Ecol. Soc. 13, 12. doi: 10.5751/ES-02485-130212
IUCN (2018). “Compilation of costs of prevention and management of invasive alien species in the EU,” in Technical note prepared by IUCN for the European Commission, (Gland: IUCN), 73.
Jarnevich, C. S., Thomas, C., Young, N. E., Backer, D., Cline, S., Frid, L., et al. (2019). Developing an expert elicited simulation model to evaluate invasive species and fire management alternatives. Ecosphere 10, e02730. doi: 10.1002/ecs2.2730
Jørgensen, S. E. (1999). State-of-the-art of ecological modelling with emphasis on development of structural dynamic models. Ecol. Modell. 120, 75–96. doi: 10.1016/S0304-3800(99)00093-9
Kerr, N. Z., Baxter, P. W., Salguero-Gómez, R., Wardle, G. M., and Buckley, Y. M. (2016). Prioritizing management actions for invasive populations using cost, efficacy, demography and expert opinion for 14 plant species world-wide. J. Appl. Ecol. 53, 305–316. doi: 10.1111/1365-2664.12592
Kettenring, K. M., and Adams, C. R. (2011). Lessons learned from invasive plant control experiments: a systematic review and meta-analysis. J. Appl. Ecol. 48, 970–979. doi: 10.1111/j.1365-2664.2011.01979.x
Krug, R. M., Roura, N., and Richardson, D. (2010). Clearing of invasive alien plants under different budget scenarios: Using a simulation model to test efficiency. Biol. Invasions 12, 4099–4112. doi: 10.1007/s10530-010-9827-3
Le Maitre, D. C., Krug, R. M., Hoffman, J. H., Gordon, A. J., and Mgidi, T. N. (2008). Hakea sericea: Development of a model of the impacts of biological control on population dynamics and rates of spread of an invasive species. Ecol. Model. 212, 342–358. doi: 10.1016/j.ecolmodel.2007.11.011
Le Roux, J. J., Clusella-Trullas, S., Mokotjomela, T. M., Mairal, M., Richardson, D. M., Skein, L., et al. (2020). “Biotic Interactions as mediators of biological invasions: Insights from South Africa,” in in Biological invasions in South Africa, eds B. van Wilgen, J. Measey, D. Richardson, J. Wilson, and T. Zengeya (Cham: Springer International Publishing), 357–427.
Lewis, M., Petrovskii, S., and Potts, J. (2016). The mathematics behind biological invasions. Berlin: Springer International Publishing.
Ligmann-Zielinska, A. (2013). Spatially-explicit sensitivity analysis of an agent-based model of land use change. Int. J. Geogr. Inf. Sci. 27, 1764–1781. doi: 10.1080/13658816.2013.782613
Lindenmayer, D. B., Wood, J., MacGregor, C., Buckley, Y. M., Dexter, N., Fortescue, M., et al. (2015). A long-term experimental case study of the ecological effectiveness and cost effectiveness of invasive plant management in achieving conservation goals: Bitou bush control in Booderee National Park in eastern Australia. PLoS ONE 10:e0128482. doi: 10.1371/journal.pone.0128482
Lohr, C. A., Hone, J., Bode, M., Dickman, C. R., Wenger, A., and Pressey, R. L. (2017). Modeling dynamics of native and invasive species to guide prioritization of management actions. Ecosphere 8, e01822.
Marais, C., and Wannenburgh, A. (2008). Restoration of water resources (natural capital) through the clearing of invasive alien plants from riparian areas in South Africa - costs and water benefits. S. Afr. J. Bot. 74, 526–537. doi: 10.1016/j.sajb.2008.01.175
Marbuah, G., Gren, I.-M., and McKie, B. (2014). Economics of harmful invasive species: A review. Diversity 6, 500–523. doi: 10.3390/d6030500
Marchante, H., Freitas, H., and Hoffmann, J. H. (2011). Post-clearing recovery of coastal dunes invaded by Acacia longifolia: Is duration of invasion relevant for management success? J. Appl. Ecol. 48, 1295–1304. doi: 10.1111/j.1365-2664.2011.02020.x
Martins, J., Richardson, D. M., Henriques, R., Marchante, E., Marchante, H., Alves, P., et al. (2016). A multi-scale modelling framework to guide management of plant invasions in a transboundary context. For. Ecosyst. 3, 17. doi: 10.1186/s40663-016-0073-8
Mashaly, A. F., and Fernald, A. G. (2020). Identifying capabilities and potentials of system dynamics in hidrolohy and water resources as a promising modelling approach for water management. Water. 12, 1432. doi: 10.3390/w12051432
McGeoch, M. A., Genovesi, P., Bellingham, P. J., Costello, M. J., McGrannachan, C., and Sheppard, A. (2016). Prioritizing species, pathways, and sites to achieve conservation targets for biological invasion. Biol. Invasions 18, 299–314. doi: 10.1007/s10530-015-1013-1
Mirchi, A., Madani, K., Watkins, D., and Ahmad, S. (2012). Synthesis of system dynamics tools for holistic conceptualization of water resources problems. Water Resour. Manag. 26, 2421–2442. doi: 10.1007/s11269-012-0024-2
Moreira, F., Rego, F. C., and Ferreira, P. G. (2001). Temporal (1958–1995) pattern of change in a cultural landscape of northwestern Portugal: implications for fire occurrence. Landscape Ecology 16, 557–567.
Mouillot, F., Ratte, J. P., Joffre, R., Mouillot, D., and Rambal, S. (2005). Long-term forest dynamic after land abandonment in a fire prone Mediterranean landscape (central Corsica, France). Landsc. Ecol. 20, 101–112. doi: 10.1007/s10980-004-1297-5
Nunes, L., Raposo, M., Meireles, C., Gomes, C., and Ribeiro, N. (2020). Control of invasive forest species through the creation of a value chain: Acacia dealbata biomass recovery. Environments 7, 39. doi: 10.3390/environments7050039
Pearson, D., and Ortega, Y. (2009). “Managing invasive plants in natural areas: Moving beyond weed control,” in Weeds: Management, economic impacts and biology, ed. R. V. Kingely (New York: Nova Science Publishers Inc), 1–21.
Portela, R., Vicente, J. R., Roiloa, S. R., and Cabral, J. A. (2020). A dynamic model-based framework to test the effectiveness of biocontrol targeting a new plant invader– the case of Alternanthera philoxeroides in the Iberian Peninsula. J. Environ. Manag. 264, 110349. doi: 10.1016/j.jenvman.2020.110349
Prior, K., Adams, D., Klepzig, K., and Hulcr, J. (2018). When does invasive species removal lead to ecological recovery? Implications for management success. Biol. Invasions 20, 267–283. doi: 10.1007/s10530-017-1542-x
Reyns, N., Casaer, J., De Smet, L., Devos, K., Huysentruyt, F., Robertson, P. A., et al. (2018). Cost-benefit analysis for invasive species control: the case of greater Canada goose Branta canadensis in Flanders (northern Belgium). PeerJ 6, e4283. doi: 10.7717/peerj.4283
Richardson, D. M., and Brown, P. J. (1986). Invasion of mesic mountain fynbos by Pinus radiata. S. Afr. J. Bot. 52, 529–536. doi: 10.1016/S0254-6299(16)31486-7
Richardson, D. M., Macdonald, I. A. W., and Forsyth, G. G. (1989). Reductions in plant species richness under stands of alien trees and shrubs in the fynbos biome. South Afr. For. J. 149, 1–8. doi: 10.1080/00382167.1989.9628986
Richardson, D. M., Van Wilgen, B. W., and Mitchell, D. T. (1987). Aspects of the reproductive ecology of four Australian Hakea species (Proteaceae) in South Africa. Oecologia. 71, 345–354. doi: 10.1007/BF00378706
Santos, M., Bastos, R., Vicente, J., Berger, U., Soares-Filho, B. S., Rodrigues, H., et al. (2015). “Anticipating invasions and managing Impacts: A review of recent spatiotemporal modelling approaches,” in Biological invasions in changing ecosystems vectors, ecological impacts, management and predictions, ed. J. Canning-Clode (Warsaw: De Gruyter Open Ltd), 389–410.
Simberloff, D. (2009). We can eliminate invasions or live with them. Successful management projects. Biol. Invasions 11, 149–157. doi: 10.1007/s10530-008-9317-z
Simberloff, D., Martin, J., Genovesi, P., Maris, V., Wardle, D. A., Aronson, J., et al. (2013). Impacts of biological invasions: What’s what and the way forward. Trends Ecol. Evol. 28, 58–66. doi: 10.1016/j.tree.2012.07.013
Souza-Alonso, P., Rodríguez, J., González, L., and Lorenzo, P. (2017). Here to stay. Recent advances and perspectives about Acacia invasion in Mediterranean areas. Ann. For. Sci. 74, 55. doi: 10.1007/s13595-017-0651-0
Tang, L., Gao, Y., Wang, C., Wang, J., Li, B., Chen, J., et al. (2010). How tidal regime and treatment timing influence the clipping frequency for controlling invasive Spartina alterniflora: Implications for reducing management costs. Biol. Invasions. 12, 593–601. doi: 10.1007/s10530-009-9465-9
Turner, B. L., Menendez, H. M., Gates, R., Tedeschi, L. O., and Atzori, A. S. (2016). System dynamics modeling for agricultural and natural Resource management issues: Review of some past cases and forecasting future roles. Resources. 5, 40. doi: 10.3390/resources5040040
van Wilgen, B. W., and Richardson, D. M. (1985). The effects of alien shrub invasions on vegetation structure and fire behaviour in South African fynbos shrublands: A simulation study. J. Appl. Ecol. 22, 955–966. doi: 10.2307/2403243
Wilson, J. R., Foxcroft, L. C., Geerts, S., Hoffman, M. T., Macfadyen, S., Measey, J., et al. (2020). “The role of environmental factors in promoting and limiting biological invasions in South Africa,” in Biological invasions in South Africa, eds B. van Wilgen, J. Measey, D. Richardson, J. Wilson, and T. Zengeya (Cham: Springer International Publishing), 355–385.
Keywords: system dynamics, silky hakea, biological invasions, invasive species management, post-fire vegetation dynamics
Citation: Morais MC, Gonçalves B and Cabral JA (2021) A Dynamic Modeling Framework to Evaluate the Efficacy of Control Actions for a Woody Invasive Plant, Hakea sericea. Front. Ecol. Evol. 9:641686. doi: 10.3389/fevo.2021.641686
Received: 14 December 2020; Accepted: 23 March 2021;
Published: 13 April 2021.
Edited by:
Ross Taylor Shackleton, University of Lausanne, SwitzerlandReviewed by:
Franklin Panetta, The University of Melbourne, AustraliaCopyright © 2021 Morais, Gonçalves and Cabral. This is an open-access article distributed under the terms of the Creative Commons Attribution License (CC BY). The use, distribution or reproduction in other forums is permitted, provided the original author(s) and the copyright owner(s) are credited and that the original publication in this journal is cited, in accordance with accepted academic practice. No use, distribution or reproduction is permitted which does not comply with these terms.
*Correspondence: Maria C. Morais, Y21vcmFpc0B1dGFkLnB0
Disclaimer: All claims expressed in this article are solely those of the authors and do not necessarily represent those of their affiliated organizations, or those of the publisher, the editors and the reviewers. Any product that may be evaluated in this article or claim that may be made by its manufacturer is not guaranteed or endorsed by the publisher.
Research integrity at Frontiers
Learn more about the work of our research integrity team to safeguard the quality of each article we publish.