- 1State Key Joint Laboratory of Environment Simulation and Pollution Control, School of Environment, Tsinghua University, Beijing, China
- 2Shaanxi Institute of Zoology, Xi’an, China
- 3Conservation Ecology Center, Smithsonian Conservation Biology Institute, Front Royal, VA, United States
The national surveys on giant panda (Ailuropoda melanoleuca) population and habitat quality have shown a high-density population of this species in the Qinling Mountains, China. We investigated five adjacent nature reserves (NR), i.e., the key distribution area of giant pandas in the Qinling Mountains, to model and identify the potential dispersal routes for giant pandas. We hypothesized that giant pandas will spread to neighboring areas when the population of the species keeps increasing. Habitat suitability was firstly evaluated based on environmental and disturbance factors. We then identified source and sink patches for giant pandas’ dispersal. Further, Minimum Cumulative Resistance (MCR) model was applied to calculate cost of movement. Finally, the Current Theory was adopted to model linkages between source and sink patches to explore potential dispersal routes of giant pandas. Our results showed that (1) the three large source patches and eight potential sink patches were identified; (2) the 14 potential corridors were predicted for giant pandas dispersing from source patches to the neighboring areas; (3) through the predicted corridors, the giant pandas in the source patches could disperse to the west, the south and the east sink patches. Our research revealed possible directional patterns for giant pandas’ dispersal in their key distribution area of the Qinling Mountains, and can provide the strong recommendations in policy and conservation strategies for improving giant panda habitat management in those identified sink patches and also potential dispersal corridors.
Introduction
The “corridor” theory illustrates passage ways for wildlife movement including dispersal routes, which are essential in ecology and conservation, especially in areas with road disturbance and human activities (Liu et al., 1999). Protection of endangered as well as vulnerable wildlife populations and their habitat requires numerous efforts, for instance, constructing the necessary corridors, helping wildlife species buffer against various disturbances, protecting the valuable gene bank and further maintaining the overall diversity of the ecosystems. Potential corridors are based on the functions of landscape connectivity and population dynamics—such as animal migration patterns and movements for better resources (Moilanen and Hanski, 2001). Haddad et al. (2003) pointed that linkages in the landscape are connected by dispersal routes and facilitate movement and dispersal of individuals, genes, and ecological processes.
However, the current natural environment has been strongly impacted by human activities. Natural landscapes are mostly fragmented due to human disturbing. As known, landscape fragmentation can result in a decrease in habitat availability, and a sharp increase in population density among few suitable habitat patches (May, 1975; Lovejoy et al., 1984). Fahrig et al. (2019) made a great discussion on the relationship between habitat fragmentation and biodiversity. The distance between remnant habitat patches and the degree of landscape connectivity are all important determinants for biotic response to fragmentation (Meurk, 1988). Thus, some individuals in the population would migrate to other habitat patches, i.e., emigration, in order to locally reduce population density (Chetkiewicz et al., 2006). Therefore, it is essential to get clear where could be the migration corridors as well as the habitat patches which could receive the migrating individuals.
Giant Panda (Ailuropoda melanoleuca) is a rare and vulnerable mammal species which is categorized as a first-class species for protection (Schipper et al., 2008; Swaisgood et al., 2010). They mainly distribute in six mountainous regions of Sichuan, Shaanxi, and Gansu provinces, China (Hu, 2001; Songer et al., 2012). The 3rd national survey on giant panda population and its habitat showed that there are 273 individuals in Shaanxi (SFA, 2006), with 192 individuals (70% of the Qinling giant panda population) in our studied key distribution area of giant pandas in the Qinling Mountains. This key distribution area includes five nature reserves (NRs): Changqing, Foping, Guanyinshan, Laoxiancheng, and Zhouzhi. Liu and Jin (2008) found out that there are two areas with a high population density of giant pandas, which are located in Changqing NR and Foping NR. Assuming that giant pandas in these two areas keep increasing their local population, some of their individuals would disperse out to the surrounding suitable habitat patches due to competitions on food, mate and space, etc., which gives rise to a natural phenomenon that limits the growth of the wild population of giant pandas (Reid et al., 1991; Gittleman, 1994). Where could be the suitable habitat patches and the potential routes for giant pandas to disperse among those patches? Although the giant panda has been lowdown its status from endangered to vulnerable species (IUCN, 2016), this species is still important to pay attention to since it is the flag species and also umbrella species in wildlife conservation in China as well as in the world.
The Qinling Mountains have experienced commercial logging from 70s to 90s in the last century (Liu et al., 2017b, 2018; Zhang et al., 2019), started the forest restoration after 1998 when the logging was banned. It has made certain forest fragmentation in some areas on the Qinling landscape. With the strengthened natural protection, the giant panda population is increasing according to the national surveys (SFA, 2006, 2015). Therefore, if dispersing of giant panda individuals occurs, they need to find their routes to disperse to the suitable habitat patches which can make them settle down under current fragmented environment. Dispersal is an important factor for the function and dynamic state of the endangered species (Hanski, 2001). Animal individual’s dispersing out of the local source patches is also the most appropriate way for giant pandas to obtain suitable habitat, choosing the palatable bamboo as their food, therefore, slowing down the increasing rate of population in one given area (Lu et al., 2001; Pan et al., 2001). Therefore, existence of the dispersing routes for giant pandas and further for human to protect them are crucial, and some necessary restoration efforts can be made to them.
So this study aimed at exploring the potential dispersal routes in the key distribution area of giant pandas in the Qinling Mountains, on the base of identifying the suitable habitat patches as well as the sink patches for receiving the dispersing giant pandas potentially. We tried to achieve our aim by answering the following main scientific questions: (1) What is our modeling approach for identifying the potential dispersing routes for giant pandas? (2) Where are the source and sink patches of giant pandas? (3) Where are the potential dispersal routes among source and sink patches?
Materials and Methods
Study Area
Based on the reasons described in the introduction, we selected five giant panda nature reserves (107°17′–107°58′, 33°19′–33°46′) who are neighbored each other: Changqing, Foping, Guanyinshan, Laoxiancheng, and Zhouzhi NRs as the study area (Figure 1 and Table 1), which is the key distribution area of giant pandas with a high density population in the Qinling Mountains.
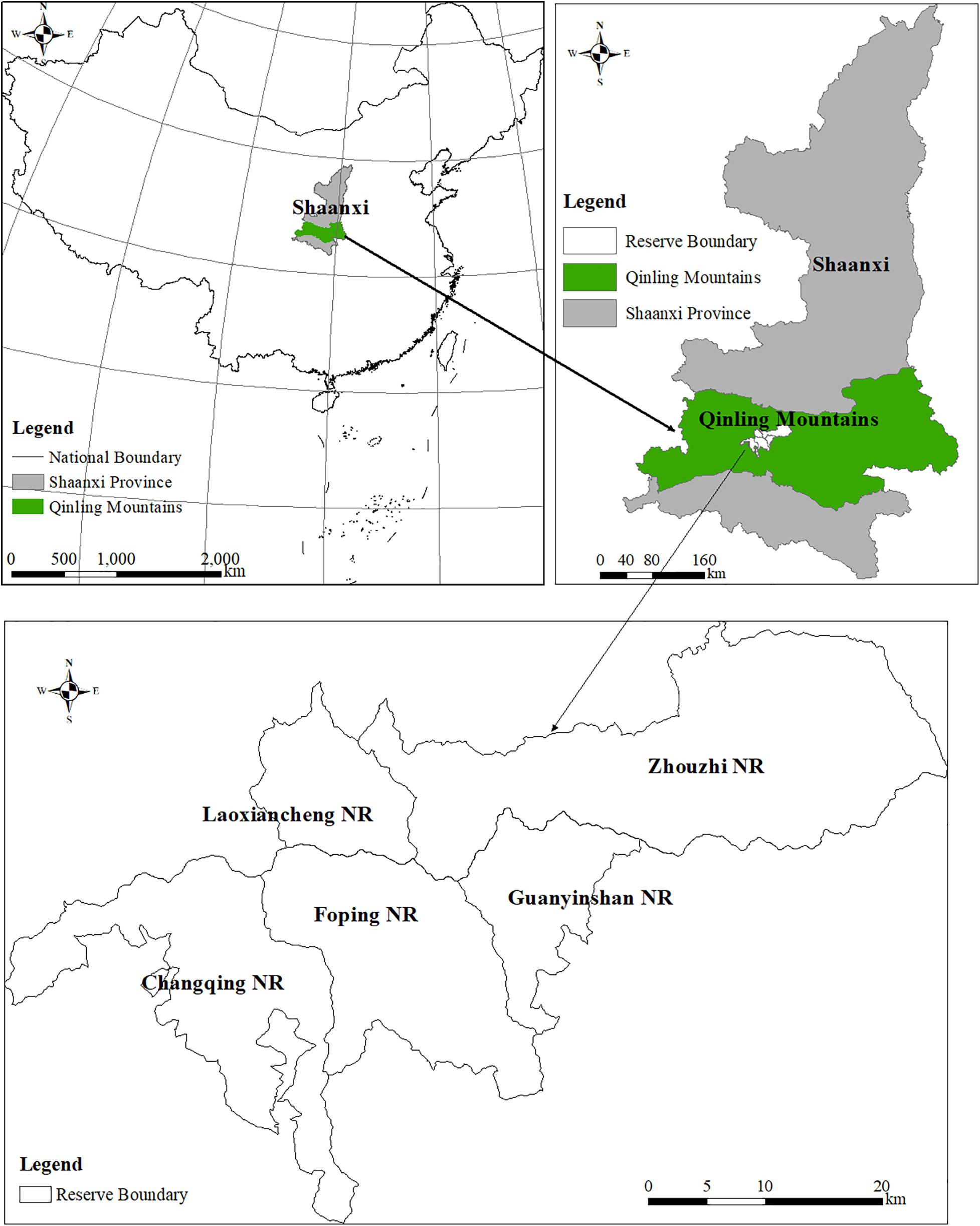
Figure 1. Five nature reserves (NR) in the key distribution area of giant pandas in the Qinling Mountains, China.
The five NRs in our study were established in different years according to the conservation progress and steps of China. Guanyinshan NR, originally a commercial logging bureau, was established lastly among these five NRs directly due to the logging ban to be enacted in 1998 in order to protect the forest and restore the habitat for giant pandas. The five NRs have similar environmental and climatic conditions. The summer season has a warm humid climate accompanied by the south-east ward monsoon, which makes the area has a northern sub-tropical floras with rich species composition (Liu, 2001). The study area grows a mixture of forest types including warm-temperate deciduous broadleaf forest and sub-tropical evergreen broadleaf forest. Mountainous terrain showed that vegetation changes with elevation increasing, such as deciduous broadleaf forest at low elevation, beech forest at middle elevation, and conifer forest at high elevation (Liu et al., 2017a). This area provides adequate habitat for wildlife survival (Liu and Li, 2008).
Data
This study used multi sources data including:
(1) Distribution data of giant panda signs (including feces, tracks, feeding signs, and individual occurring sites) from the 3rd national survey of giant panda population and habitat provided by Shanxi Forestry Department, used for deriving the density map of giant panda signs with a resolution of 30 m × 30 m for later spatial calculation;
(2) Vegetation map with a resolution of 30 m × 30 m provided by Shanxi Forestry Department;
(3) Bamboo distribution maps with a resolution of 30 m × 30 m provided by five nature reserves related to this study;
(4) Terrain data including digital elevation data layer and its derived slope data layer and slope aspect data layer with also a resolution of 30 m × 30 m from Institute of Geographic Science, China Academic Sciences;
(5) Hydrological data with river distribution map from Shanxi Forestry Department, used for deriving the map of distance to rivers with a resolution of 30 m × 30 m;
(6) Human disturbance data including locations of medicinal-herbs collection, lacquer collection, bamboo shoot collection, hunting, road construction, and farming. from the 3rd national survey of giant panda population and habitat provided by Shanxi Forestry Department, used for deriving the map of distance to human disturbance with a resolution of 30 m × 30 m;
(7) Road distribution map provided by Shanxi Forestry Department, used for deriving the map of distance to roads with a resolution of 30 m × 30 m.
We used all these data to analysis habitat suitability, identify important patch areas as source patches where giant pandas dispersing from and sink patches where giant pandas dispersing into, and find potential dispersal routes between source and sink patches.
Software
Linkages Mapper toolbox in ArcGIS was a software program used in our study that needed proper conditions to be applied generally. Linkage design models such as isolation-by-resistance (IBR) model (McRae, 2006), least-cost path (LCP) model (Adriaensen et al., 2003) combine with the Current Theory were widely applied to predict pathways connecting population pairs or gene flow (McRae and Paul, 2007; St-Louis et al., 2014).
Integrative Modeling Approach
The whole modeling approach of our study contained four submodels (Figure 2). These submodels were taken to predict the giant pandas’ dispersing pattern through analyzing habitat suitability (submodel 1), identifying source and sink patches (submodel 2), calculating the habitat resistance patterns (submodel 3), and final mapping the potential dispersal routes (submodel 4).
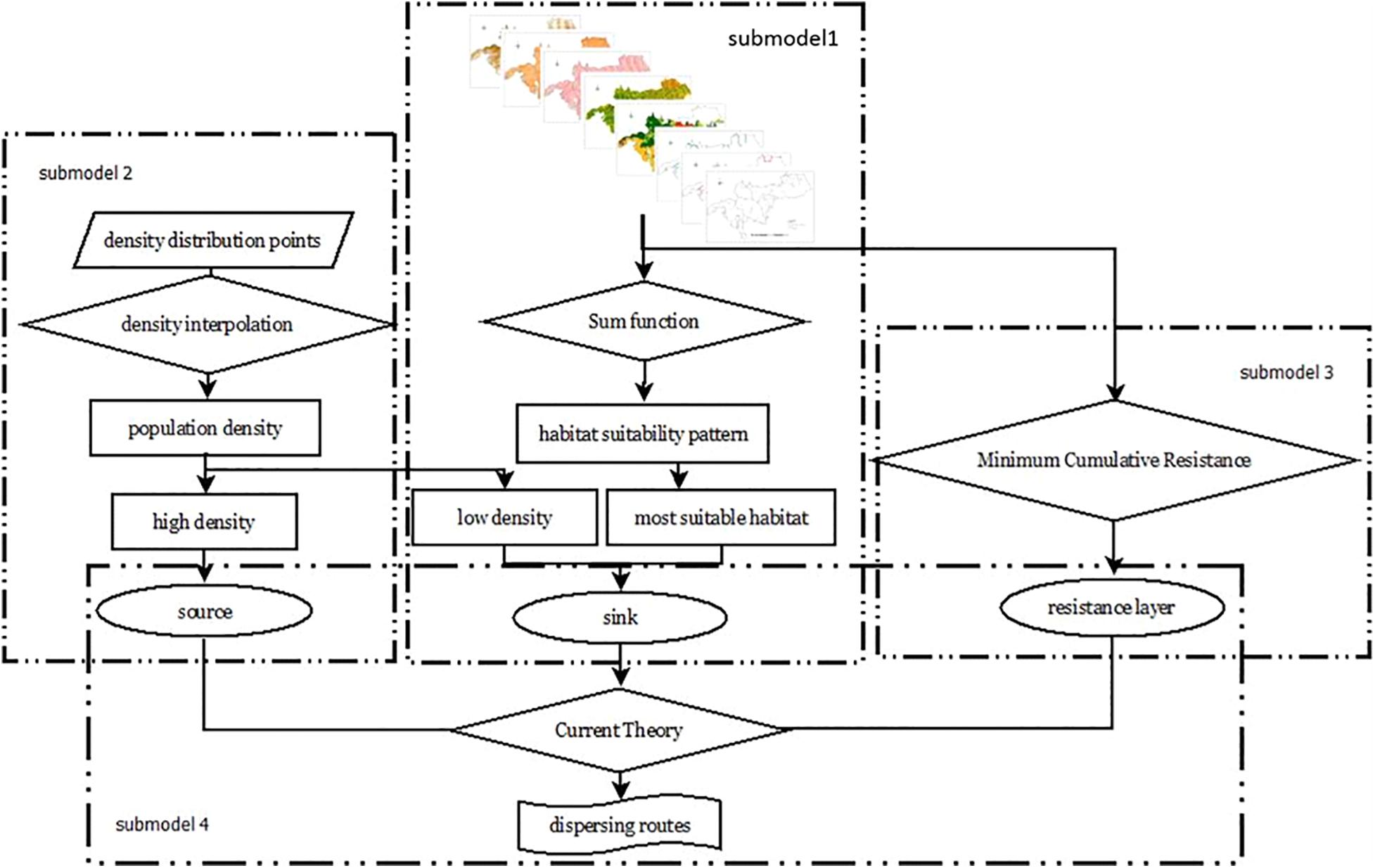
Figure 2. Integrative modeling approach to map the dispersal routes for giant pandas in their key distribution area of the Qinling Mountains, China.
Submodel 1: Analyzing Habitat Suitability
An efficient method for modeling the individuals’ dispersal was habitat suitability analysis to understand the nature conditions that the species confronted during migrating. The main eight habitat factors used were elevation, slope, aspect, vegetation, bamboo as well as distances to rivers, roads, and human disturbances (Supplementary Figure 1), similar with the research work done by Wu et al. (2013). In order to implement the spatial calculation, the same map resolution and projection is required. So, we standardized all GIS data layers in the same spatial projection and resampled these layers into 30 m × 30 m resolution to match with the resolution of vegetation map (30 m × 30 m). To obtain the suitability value, we applied expert assessment approach by which the weight assigning to different habitat factors has been often applied in giant panda habitat assessment, such as Ouyang et al. (2000); Li et al. (2005), Xu et al. (2005), and Wu et al. (2013). For map calculation, we used values of 0, 1, 2, and 3 to represent unsuitable, marginal suitable, suitable, and most suitable (Supplementary Material).
To integrate the eight habitat factors, we applied Analytic Hierarchy Process (AHP) which is based on the judgment of importance of each factor. The AHP method was developed by Saaty (1980), and has been often applied, such as Li et al. (2005); Nekhay et al. (2009), and Liu and Sun (2010). We divided the factors into three groups which are biotic factors (vegetation and bamboo), abiotic factors (elevation, slope, aspect, and distance to rivers), and disturbing factors (distances to roads and human disturbances). We then made comparison of importance between every two factors and formed the matrix of importance values. Then the weights of the eight factors were obtained by computing the maximum latent roots and corresponding eigenvectors of the matrix (Liu and Sun, 2010). The weights were 0.492 for bamboo, 0.240 for human disturbances, 0.093 for vegetation, 0.080 for roads, 0.068 for rivers, 0.036 for slope, 0.012 for elevation, and 0.006 for aspect. For creating the final habitat suitability map, each pixel was calculating its suitability value by summing the eight layers through each habitat factor’s suitability value multiplied by AHP weights (maintained two numbers behind decimal and then changed to integer by timing 100 for running GIS calculation). This map has a value range from 0 to 835. We reclassified this map into four categories with thresh hold values by using Natural Breaks (Jenks) method: (0∼208.75) as unsuitable, (208.75∼417.5) as marginal suitable, (417.5∼626.25) as suitable, (626.25∼835) as most suitable.
Submodel 2: Identifying Source and Sink Patches
In our research, source patches are the most suitable habitat patches with high-density signs of giant pandas to indicate more animal individuals, where the individuals disperse from and to other habitat patches. Sink patches are the suitable habitat patches with low-density signs of giant pandas, where can receive those dispersing individuals. Linkages between sources and sinks for dispersal have been described as landscape processes, where the matter and energy of source patches can outflow to sinks under viable conditions. Giant pandas disperse and spread to areas with abundant bamboo food, water, and other environment resources for its survival and reproduction.
We first created the density map of giant panda signs and further we used this map to indicate the density of giant panda population. Using the home range of the giant panda, about 6 km2 (Hu et al., 1985), density maps were calculated using point density tool in ArcGIS with a searching radius of 1.38 km (home range area of 6 km2). According to Liu and Jin (2008), two high-density areas all have giant panda sings of ≥4 sites/km2. So we maintained to use these criteria to determine source patches. We further defined sink patches with giant panda sings of <4 sites/km2 and continuous suitable habitat of ≥20 km2.
Submodel 3: Calculating Habitat Resistance by Using MCR Model
When a species moves through various landscapes, it normally encounters obstacles on the way (Gabelli et al., 2006). The Minimum Cumulative Resistance (MCR) model was used for calculating the habitat resistance, which reflects the accessibility and possibility for the species to disperse and find suitable habitat. The MCR model was first proposed by Knaapen et al. (1992) and we applied the formula (1) which was modified by Yu (1998).
Dij is the spatial distance from source j to landscape unit i. Ri is the resistance of pixel i in landscape. We assigned the resistance in Table 2 with values of 1, 4, 7, and 10 for eight habitat factors to represent four classes of habitat resistance, i.e., smallest, small, strong, and strongest. We then summed up the resistance values by using AHP weights to obtain Ri for each pixel. Min was the minimum resistance of the assessed pixel to all different sources, and f was the function coefficient between MCR and dispersing process. Habitat resistance surface was derived from cost distance toolbox in ArcGIS using resistance layer.
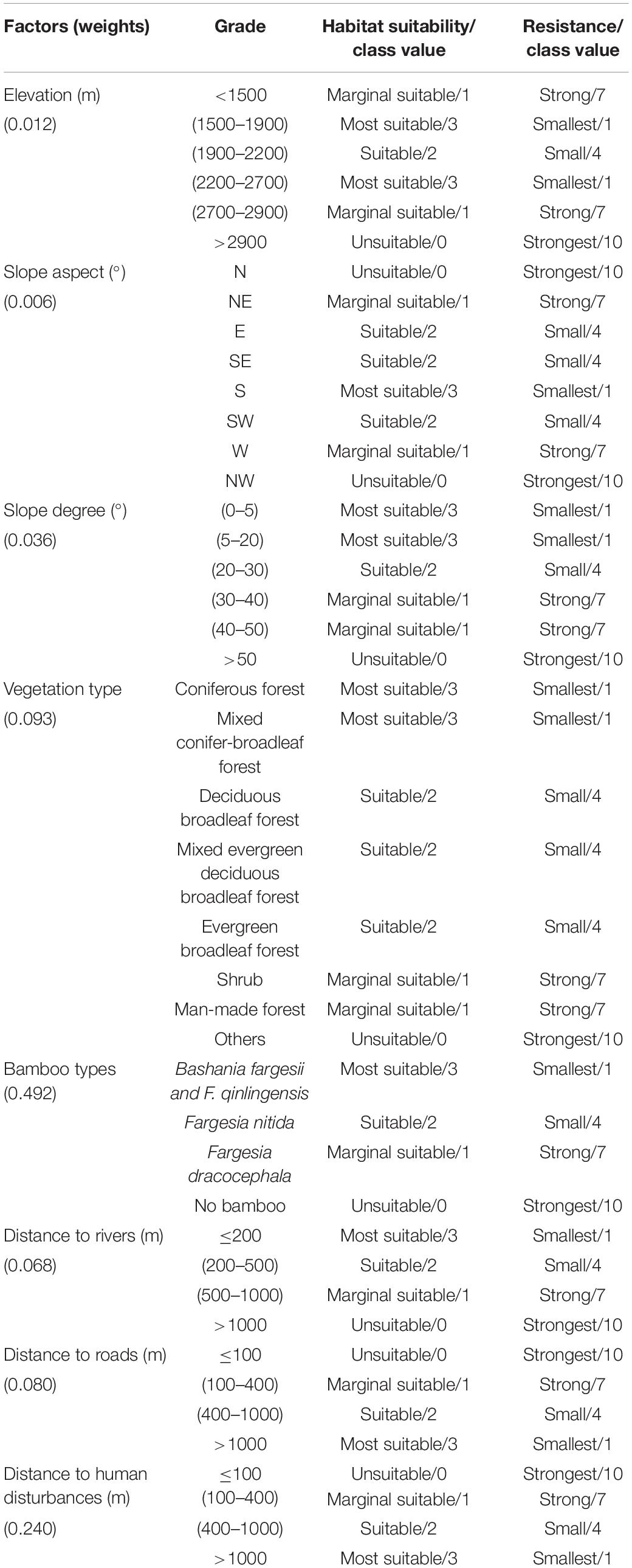
Table 2. Value assignment for habitat suitability classes and resistance analysis for the selected eight habitat factors in the key distribution area of giant pandas in the Qinling Mountains, China.
Submodel 4: Predicting Dispersal Routes by Applying the Current Theory
The Current Theory belongs to physics, in which circuits is defined as networks of nodes connected by resistors. When a voltage, V, applied across a resistor, the amount of current, I, flowing through the resistor depends on the voltage applied and the resistance, R (McRae et al., 2008). Nowadays, ecologists are thinking that ecological process like individual movement and gene flow can be related to resistance, current, and voltage in raster grids, which is like what the Current Theory described. Based on the calculated habitat resistance in submodel 3, the Current Theory was applied to predict giant pandas’ dispersal routes by using formula (2).
Longer distance giant pandas disperse, the higher habitat resistance they would encounter. In order to simulate the density of individual movement like the current, the Current Theory was used to simulate the resistance layer. The greater the resistance is, the smaller the current (the dispersing flow of giant pandas) is. We regarded source patches as the starting locations and sink patches as the terminal locations of migration. The habitat resistance determines corridors between any pairs of source and sink patches. The implementation of the Current Theory was applied by the Linkage Mapper Toolkit in ArcGIS.
Results
Reclassified Eight Habitat Factors
Table 2 showed the eight habitat factors and their detailed classes for habitat suitability assigned based on the expert knowledge. Based on this constructed expert knowledge system, the eight habitat factors were all reclassified with four classes: unsuitable, marginal suitable, suitable and most suitable with values of 0, 1, 2, 3, respectively. The eight new maps of these factors were obtained and shown in Supplementary Figure 2. They are ready for being used in spatial calculation of habitat suitability of giant pandas.
Suitability Pattern of Giant Panda Habitat
Habitat suitability map (Figure 3) showed that the area of the most suitable habitat was 101.8 km2 in total and shown by three main patches: the largest one 68 km2, the second largest one 24 km2, and the third largest one 10 km2. The most suitable and suitable habitats were distributed in the western part of the study area. The most suitable patches were mainly in Foping, Changqing and Laoxiancheng NRs, while marginal suitable and unsuitable areas were in Zhouzhi NR and the east part of Guanyinshan NR.
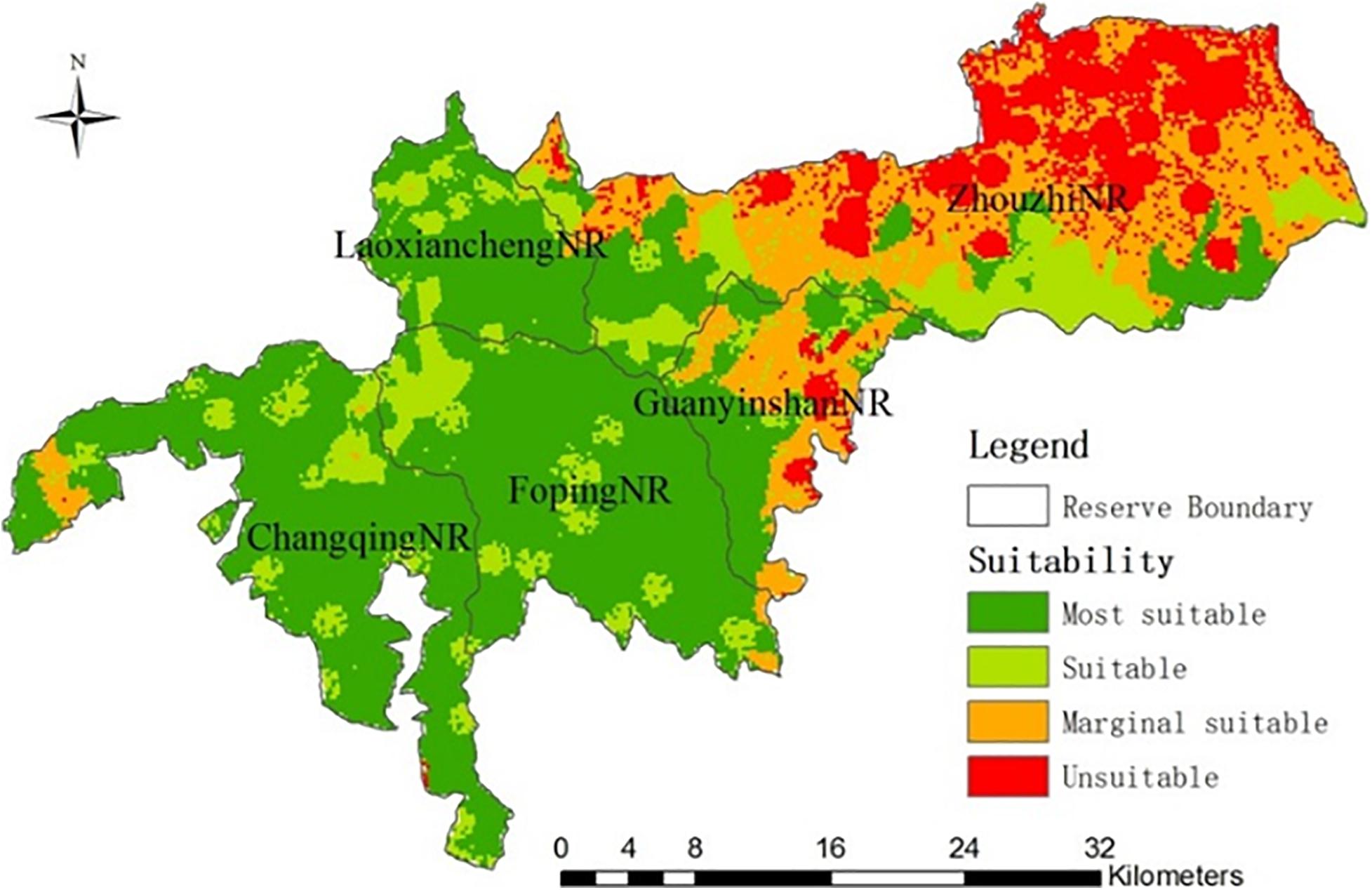
Figure 3. The suitability map of the key distribution area of giant pandas in the Qinling Mountains, China.
Source and Sink Patches
We used the created density map of giant panda activity signs to reflect the density of giant panda population. From this sign density map, we mapped the source patches and the sink patches (Figure 4). Figure 4A showed that the largest population patches were located in Changqing and Foping NRs, and the highest density of giant panda signs reached 8 sites/km2. Three identified crucial large source patches contained two in the north of Changqing with areas of 7.5 km2 and 11.7 km2, and one in the middle of Foping with an area of 19.0 km2. Some small source patches appeared separately in the different locations: two of them in Changqing with areas of 2.1 km2 and 0.7 km2, and two of them in Foping with areas of 1.5 km2 and 1.8 km2. Zhouzhi and Laoxiancheng NRs had one smaller source patch for each with areas of 0.8 km2 and 1.9 km2.
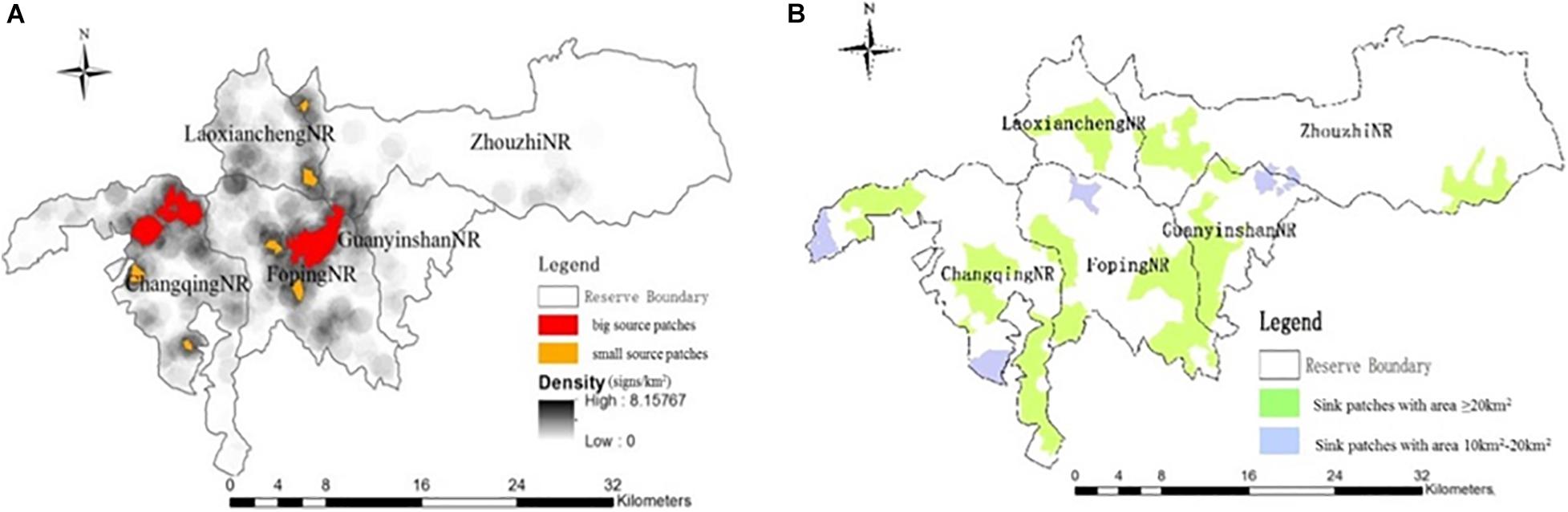
Figure 4. Maps of source patches (A) and sink patches (B) in the key distribution area of giant pandas in the Qinling Mountains, China.
Figure 4B showed the identified sink patches and 8 big patches with an area of ≥20 km2 were found in the study area, including three patches in Changqing with areas of 28.7 km2, 34.8 km2, 47.5 km2, one patch in Foping with an area of 22.1 km2, one patch in Laoxiancheng with an area of 32 km2, two patches in Zhouzhi with areas of 24 km2 and 39.1 km2, and one patch at the junction area of Foping and Guanyinshan with an area of 97.8 km2. Meanwhile, we found four smaller sink patches with areas all less than 20 km2 where giant pandas can also potentially disperse to.
The total areas of the source and sink patches are 47.3 km2 and 325.8 km2, respectively. The large sink areas indicates that the population of giant pandas in the study area does have the space to increase potentially.
Resistance Patterns for the Dispersal of Giant Pandas
The final resistance cost pattern calculated by MCR, to indicate the difficulty for giant panda to pass through the landscape, was shown in Figure 5 in which each pixel reflected the cost of migration to the nearest habitat sources. It shows that there exists less cost for giant panda to disperse in the west part of the study area, while the high resistance occurs in the eastern part such as Zhouzhi. Based on this resistance patterns, it was possible to identify areas where giant pandas would potentially disperse to.
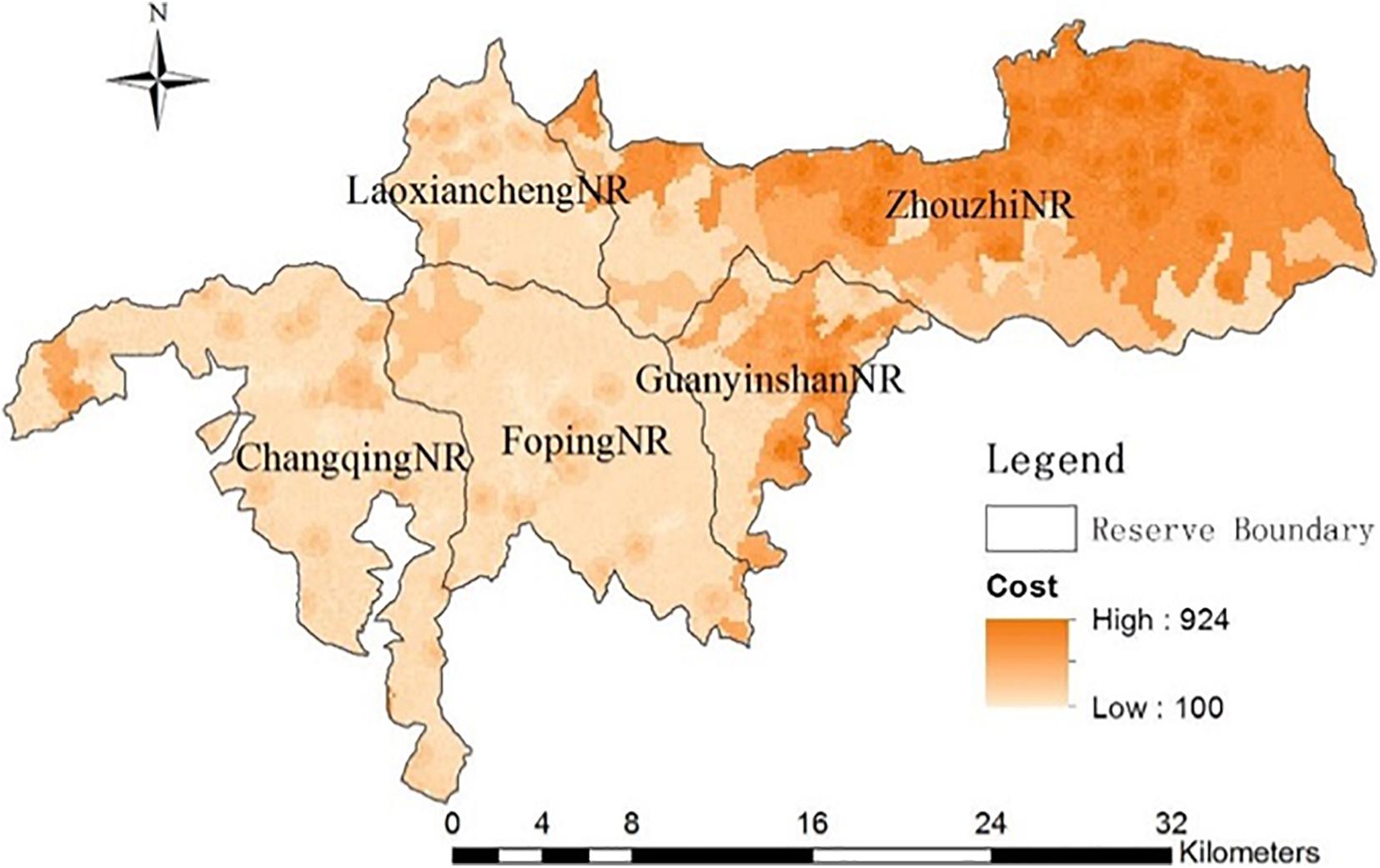
Figure 5. The summed minimum resistance cost pattern in the key distribution area of giant pandas in the Qinling Mountains, China.
Dispersal Routes for Giant Pandas
Figure 6 showed the spatial distribution of our predicted dispersal routes by the Current Theory model. We found the potential dispersal routes between three large source patches and eight large sink patches where the least distance and cost exist. Between source and sink patches, we found 14 routes in the study area in the key distribution area of giant pandas in the Qinling Mountains. Based on three large source patches, the most important potential dispersal routes for giant pandas were from the east of Changqing to the west, to the middle, to the south of Changqing, to the west of Foping and to the northeast to Laoxiancheng. The second-most important routes were from Foping to the west of Foping, to the southwest of Changqing, to the junction area of Foping and Guanyinshan, to the south of Laoxiancheng, and to the west of Zhouzhi, to the west of Guanyinshan and further to the east of Zhouzhi. The center part (with blue color in Figure 6) of the predicted potential dispersing routes has the high “current with circuits” with an ecological meaning of that giant pandas are easy to disperse in these center parts. This showed the possible intensity of giant pandas’ dispersal in the corridors with blue and orange representing high and low intensity of animal dispersing, respectively.
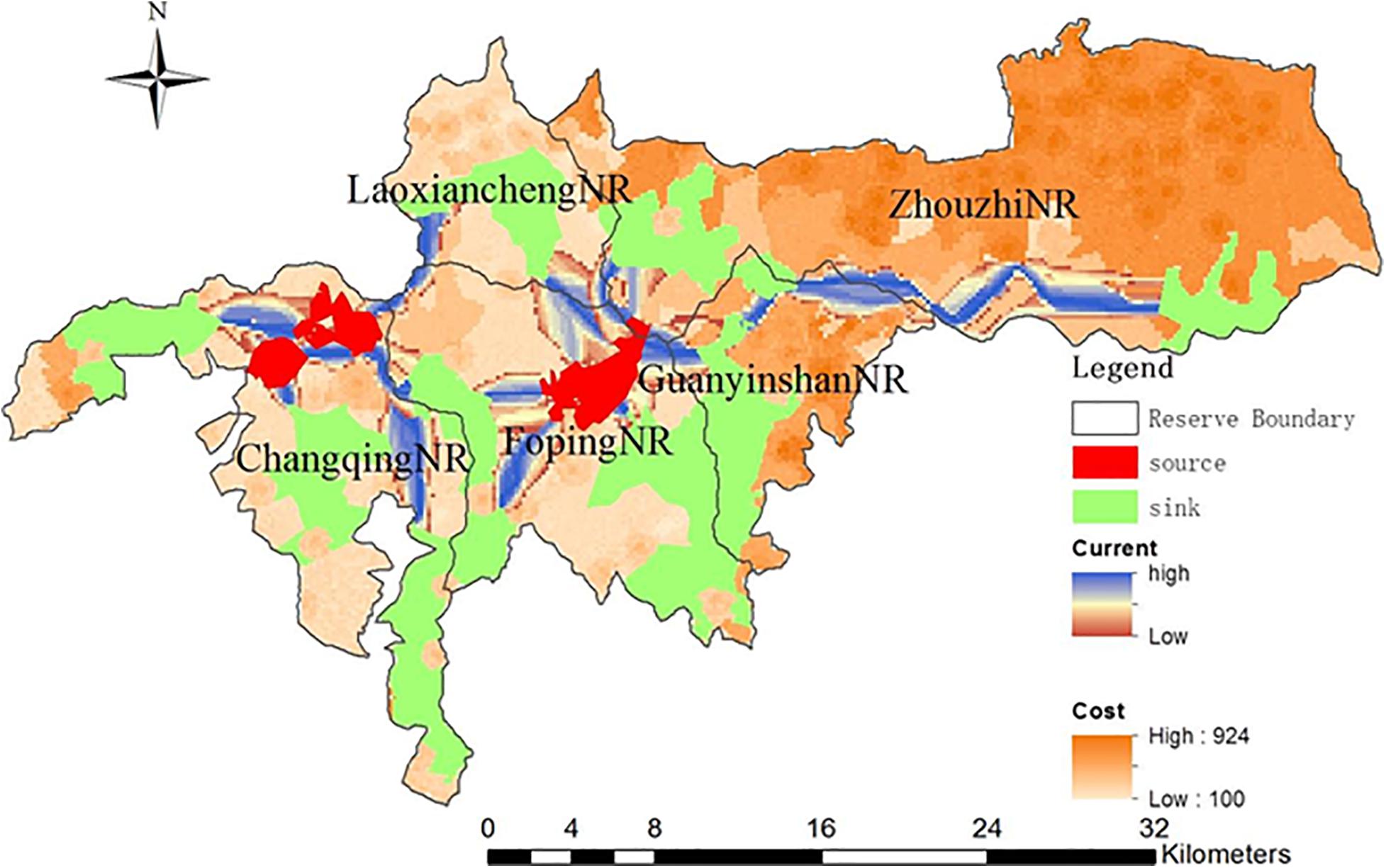
Figure 6. Giant pandas’ dispersal routes in the key distribution area of giant pandas in the Qinling Mountains, China.
Discussion
We obtained three major findings: (1) an integrative modeling approach established with applying three submodels to predict giant pandas’ dispersal routes; (2) the three large source patches and eight sink patches mapped by this integrative model; and (3) the 14 dispersal routes identified by the Current Theory model. We reached our proposed three research objectives.
Importance of Predicting the Potential Dispersal Routes for Giant Pandas
Fragmentation generally results in a discontinues landscape that consists of remnant areas of native vegetation surrounded by a matrix of agricultural or human developed land (Saunders et al., 1991). As known land use change can lead to conversion of ecological processes and reduction of biological diversity (Graham et al., 2006). Human disturbances changed the landscape of our research area, where there were increasingly more human residents in the northeast Zhouzhi and there was a national-level road (G108) in the east of Guanyinshan. So the resistance value was high in the east part of study area. Along with the essential dispersal route (from the source of Foping NR to the east sink in Zhouzhi NR), human disturbances have an impact in both sides of this route, thus, a necessity for conservation and restoration such as decreasing traffic flow, conducting more tunnels or ecological corridors in giant panda habitat should get more attention.
Dispersal route construction has been proposed as a measure to enhance the long-term genetic diversity (Schonewald-Cox et al., 1983), as well as viability of the regional panda population growth (Yin et al., 2006). Establishing, enlarging, and networking among nature reserves, and strengthening supervision and management in the predicted potential dispersal routes with populations of giant pandas were essential for decision-makers (Diamond, 1975; Diamond et al., 1976; Xu et al., 2006, 2014). From findings of our study, government planning departments should find out the low resistance dispersal routes especially in the boundary regions of NRs (like the junctions of Laoxiancheng-Foping-Zhouzhi and Foping-Laoxiancheng-Changqing), and further strengthen the effort of bamboo restoration and continuous supervision.
Rather than find corridors by adding linkages among sinks and sources, it is necessary to compare relative conservation priority of all linkages through conservation planning research (Possingham et al., 2000). Other researchers only found the cost of dispersal throughout different habitats and a linear path for giant pandas (Li et al., 2010). However, we found 14 polygon dispersal routes, where more complex spatial suitability analysis model and the physical theory were applied to effectively weight the importance of each route. Through these efforts, the influence from disturbing factors can be minimized (Zeller et al., 2012), and the structural and functional quality of ecosystem could be improved (Crooks and Sanjayan, 2006).
Conserving regional habitat connectivity could facilitate recovery and reproduction for many biological population (Bian and West, 1997; Carroll et al., 2012). Thus, we can implement ecological restoration of the damaged and threatened ecosystem; therefore, enhance the capacity and stability of the ecological system (Liu et al., 2015). More nature reserves and conservation stations should be established in the north and east of Changqing, meanwhile, we must reduce the human disturbance in Zhouzhi. Furthermore, improving habitat quality shouldn’t be ignored because this measure could get higher conservation returns than only increase the size and optimized the components of patches (Prugh et al., 2009).
Key Work in Predicting the Dispersal Routes
Source patches, sink patches, and resistance are necessary to be defined based on empirical environmental data. Source patches represent the starting locations of an animal’s movement, which can be expressed in GIS as discrete points, lines and polygons (Oh and Jeong, 2007; Liu and Li, 2008). Sink patches have overall good habitat suitability, and can act as potential sites for occupancy of animals. Resistance can be reflected through calculation of cost, which is quantified by ranking the pixels in GIS.
The MCR model, a way to calculate cost, has been applied in a number of fields, such as landscape eco-planning, landscape ecological suitability assessment (Yu et al., 2005; Liu et al., 2010), landscape pattern analysis (Nelson et al., 2009), and landscape accessibility analysis (Wang et al., 2007; Gan and Hu, 2008). Liu and Li (2008) used an applied least cost paths model, similar to the MCR model, to make a functional division for Laoxiancheng NR. MCR model was also applied to calculate the amount of ecological land that meets the demand of socioeconomic development and ecological protection (Li F. et al., 2015). The results of our study illustrated the initial dispersing directions with a low resistance cost, whereby they stop dispersing further when encountering areas with high resistance (Sawyer et al., 2011). So MCR model is aiming at quantifying multiple environmental factors and find an optimal solution for conservation or restoration of migration corridors.
For two sub-models’ processing, i.e., submodel 1 and submodel 3, we applied AHP approach to develop a system for classifying the habitat factors in order to evaluate the suitability and also the resistance of the habitat. AHP model is a very experienced approach and has been applied in many different fields which tried to calculate the weights based on the eigenvalues (Liu and Sun, 2010) for various indicators or factors through comparison the importance between any two indicators. While we admit that such comparison could be a subjective process, experienced experts will guarantee the robustness of the results as only two factors were included in the comparison. To invite more knowledgable experts might further improve the model results and give a proper pattern of giant panda dispersal routs, whereas trade-off needs to be considered between the model output and extra time cost.
The Current Theory is a reliable, efficient method to identify, further protect, restore, and construct connectivity among landscapes (Lacher and Mares, 1996). It can simulate the random movement of population by taking into account routes between patches through a connectivity parameter called resistance distance (McRae et al., 2008; Walpole et al., 2012). Qing et al. (2016) created corridors among Xiaoxiangling Mountains, which research combined the Maxent distribution model and the Current Theory and took spatial distribution of giant panda into consideration. Wang et al. (2014) conducted least-cost and circuit models to identify giant panda dispersal paths among Huangbaiyuan NR and Niuweihe NR, and compared the dispersal cost under different scenarios. Comparing with previous study, we not only identified corridors among the patches as well as reserves, but also predicted possible intensity of dispersal in the corridors.
Limitations From Small Habitat Patches for Animal Dispersal
From all source patches obtained, we easily found six small source patches (Figure 4A), which were not able to calculate out the optimal linkages between sources and sink patches. Two reasons are (1) the source patches were too small to react to any changes in the landscape; in addition, negative calculations confirmed that the least cost paths and corridors between small source and sink patches were unobtainable, (2) habitat and resistance around small source patches have a low spatial heterogeneity, and consequently no suitable corridors occur among sources and sink patches. Therefore, giant pandas’ capability and probability of moving among these six small habitat patches was unfortunately limited. These two reason were found and explained for the first time.
The Evidence of Giant Panda Using the Predicted Potential Dispersal Routes
Data from the 4th national survey of giant panda provided a very strong evidence on giant pandas’ using our predicted potential dispersal routes (Supplementary Figure 3). From this spatial pattern, dispersal trend could be revealed through comparing distribution in two periods of the 3rd and 4th national surveys. The 7.6% signs from the 4th national survey in the Qinling Mountains (Unpublished) appeared in the identified dispersal routes. This indicated giant pandas used to minimalize the resistance in their dispersal process and tried to control the distance they move and the energy they spend. From the signs occurred eastward in the dispersal routes in Supplementary Figure 3, the 38% signs appeared in the long distance routes, which evidenced they had used this long distance route from source patches in Foping NR and headed to sink patches in the east of Zhouzhi NR. So the 4th national survey’s data helped us verify the utilization of dispersal routes. Lack of distribution data, further researches by applying the infrared cameras are necessary to record the giant pandas’ activity patterns and so to provide strong proof to their utilization of dispersal routes. Due to limitation of environment data, giant pandas’ habitat environmental maps can be supplemented in the future by remote sensing inversion models developed to provide spatial continuous, high-resolution environmental layers (Li et al., 2013; Li Z. et al., 2015).
Conclusion and Management Implications
This study successfully applied MCR and Current Theory models to predict giant pandas’ potential dispersal routes on the southern slope of the Qinling Mountains. The results showed that giant pandas in high-density areas may disperse multi-directionally to neighboring sink patches from the source patches. The three large sources, eight sinks, and 14 dispersal routes between sources and sinks were identified among five NRs. We showed the potential linkages between five NRs, and mapped the intensity and direction of giant pandas’ dispersing.
Our research revealed possible directional patterns for giant panda dispersal in the key distribution area of the Qinling Mountains. This can provide the scientific support to facilitate planning and managing of giant panda NRs, encourage them to reduce the impacts from human disturbance directly on the predicted dispersal corridors and sink patches, for instance, either planting suitable bamboos in the sites where need more food resources for giant panda or thinning the bamboo stems in the places where provide some space for giant panda to pass and get new bamboo shoots. In these cases, more suitable habitat can be maintained for giant pandas.
Data Availability Statement
The datasets presented in this article are not readily available because since the elevation-related data are not allowed to share publicly. Requests to access the datasets should be directed to the corresponding author.
Author Contributions
XL, LmL, and LnL completed the research and the manuscript drafting. XL kept editing on the manuscript. XJ has provided us the valuable dataset and some ideas on the giant panda dispersing. MS helped with the manuscript quality improving. All authors contributed to the article and approved the submitted version.
Funding
We acknowledge the financial supports from the National Natural Science Foundation of China (NSFC) project (41271194) and International Project of Giant Panda Conservation Founded by State Forestry Administration (CM1424).
Conflict of Interest
The authors declare that the research was conducted in the absence of any commercial or financial relationships that could be construed as a potential conflict of interest.
Acknowledgments
We thank all people who contributed to make these valuable datasets available for further scientific researches. We also thank Ms. Eve Bohnett to enhance the language in the manuscript and Dr. Wanlong Sun to make Figure 1.
Supplementary Material
The Supplementary Material for this article can be found online at: https://www.frontiersin.org/articles/10.3389/fevo.2021.636937/full#supplementary-material
Supplementary Figure 1 | The raw data layers used for modeling: (A) elevation, (B) slope degree, (C) slope aspect, (D) vegetation, (E) bamboo types, (F) rivers, (G) roads, (H) human disturbances.
Supplementary Figure 2 | The reclassified data layers with suitability: (A) elevation, (B) slope degree, (C) slope aspect, (D) vegetation, (E) bamboo type, (F) distance to rivers, (G) distance to roads, (H) distance to human disturbances.
Supplementary Figure 3 | The eastward giant pandas’ dispersal routes used by giant panda based on data from the 4th national survey.
References
Adriaensen, F., Chardon, J. P., Blust, G. D., Swinnen, E., Villalba, S., Gulinck, H., et al. (2003). The application of ‘least-cost’ modelling as a functional landscape model. Landscape Urban Plan 64, 233–247. doi: 10.1016/s0169-2046(02)00242-6
Bian, L., and West, E. (1997). GIS modeling of elk calving habitat in a prairie environment with statistics. Photogramm. Eng. Rem. S. 63, 161–167.
Carroll, C., McRae, B. H., and Brookes, A. (2012). Use of linkage mapping and centrality analysis across habitat gradients to conserve connectivity of gray wolf populations in western north america. Conserv. Biol. 26, 78–87. doi: 10.1111/j.1523-1739.2011.01753.x
Chetkiewicz, C. L. B., St. Clair, C. C., and Boyce, M. S. (2006). Corridors for conservation: integrating pattern and process. Annu. Rev. Ecol. Evol. Syst. 37, 317–342. doi: 10.1146/annurev.ecolsys.37.091305.110050
Crooks, K. R., and Sanjayan, M. (2006). Connectivity conservation.. Cambridge, UK: Cambridge University Press.
Diamond, J. M. (1975). The island dilemma: lessons of modern biogeographic studies for the design of natural reserves. Biol. Conserv. 7, 129–146. doi: 10.1016/0006-3207(75)90052-X
Diamond, J. M., Terborgh, J., Whitcomb, R. F., Lynch, J. F., Opler, P. A., Robbins, C. S., et al. (1976). Island biogeography and conservation: strategy and limitations. Science 193, 1027–1032. doi: 10.1126/science.193.4257.1027
Fahrig, L., Arroyo-Rodríguez, V., Bennett, J. R., Boucher-Lalonde, V., Cazetta, E., Currie, D. J., et al. (2019). Is habitat fragmentation bad for biodiversity? Biol. Conserv. 230, 179–186. doi: 10.1016/j.biocon.2018.12.026
Gabelli, J., Fève, G., Berroir, J. M., Plaçais, B., Cavanna, A., Etienne, B., et al. (2006). Violation of Kirchhoff’s laws for a coherent RC circuit. Science 313, 499–502. doi: 10.1126/science.1126940
Gan, H., and Hu, H. (2008). Biodiversity conservation corridor design based on habitat selection of gaur (bos gaurus): a case study from xishuangbanna, china. Chinese J. Ecol. 27, 2153–2158.
Gittleman, J. L. (1994). Are the pandas successful specialists or evolutionary failures? BioScience 44, 456–464. doi: 10.2307/1312297
Graham, C. H., Moritz, C., and Williams, S. E. (2006). Habitat history improves prediction of biodiversity in rainforest fauna. PNAS 103, 632–636. doi: 10.1073/pnas.0505754103
Haddad, N. M., Bowne, D. R., Cunningham, A., Danielson, B. J., Levey, D. J., Sargent, S., et al. (2003). Corridor use by diverse taxa. Ecology 84, 609–615.
Hanski, I. (2001). Population dynamic consequences of dispersal in local populations and in metapopulations. Dispersal. Oxford, UK: Oxford University Press, 283–298.
Hu, J. (2001). Research on the giant panda. Shanghai, China: Shanghai Publishing House of Science and Technology.
Hu, J., Schaller, G. B., Pan, W., and Zhu, J. (1985). Wolong’s giant panda. Chengdu, China: Sichuan Publishing House of Science and Technology.
Knaapen, J. P., Scheffer, M., and Harms, B. (1992). Estimating habitat isolation in landscape planning. Landscape Urban Plan. 23, 1–16. doi: 10.1016/0169-2046(92)90060-D
Lacher, T. E., and Mares, M. A. (1996). Availability of resources and use of space in eastern chipmunks, tamias striatus. J. Mammal. 77, 833–849. doi: 10.2307/1382689
Li, F., Ye, Y., Song, B., and Wang, R. S. (2015). Evaluation of urban suitable ecological land based on the minimum cumulative resistance model: a case study from changzhou, china. Ecol. Model. 318, 194–203. doi: 10.1016/j.ecolmodel.2014.09.002
Li, H., Li, D., Li, T., Qiao, Q., Yang, J., and Zhang, H. (2010). Application of least-cost path model to identify a giant panda dispersal corridor network after the wenchuan earthquake—case study of wolong nature reserve in china. Ecol. Model. 221, 944–952. doi: 10.1016/j.ecolmodel.2009.12.006
Li, J., Li, T., Jin, X., Liu, X., and Tang, G. (2005). The quality evaluation of giant panda’s habitat based on analytic hierarchy process. J. Mt. Res. 23, 694–701. doi: 10.1007/s10971-005-6694-y
Li, Z., Liu, X., Ma, T., Kejia, D., Zhou, Q., Yao, B., et al. (2013). Retrieval of the surface evapotranspiration patterns in the alpine grassland–wetland ecosystem applying SEBAL model in the source region of the yellow river, china. Ecol. Model. 270, 64–75. doi: 10.1016/j.ecolmodel.2013.09.004
Li, Z., Liu, X., Niu, T., Kejia, D., Zhou, Q., Ma, T., et al. (2015). Ecological restoration and its effects on a regional climate: the source region of the yellow river, china. Environ. Sci. Tech. 49, 5897–5904. doi: 10.1021/es505985q
Liu, G., Yang, Z., Chen, B., Zhang, L., Zhang, Y., and Su, M. (2015). An ecological network perspective in improving reserve design and connectivity: a case study of wuyishan nature reserve in china. Ecol. Model. 306, 185–194. doi: 10.1016/j.ecolmodel.2014.10.004
Liu, J., Ouyang, Z., Taylor, W. W., Groop, R., Tan, Y., and Zhang, H. (1999). A framework for evaluating the effects of human factors on wildlife habitat: the case of giant pandas. Conserv. Biol. 13, 1360–1370. doi: 10.1046/j.1523-1739.1999.98418.x
Liu, L., Liu, X., Jin, X., Wand, Z., and Gong, M. (2017a). Research on the change of giant pandas’spatial utilization and road impacts in the qinling mountains. Acta Ecol. Sin. 37, 215–225.
Liu, X., Wu, P., Shao, X., Songer, M., Cai, Q., Zhu, Y., et al. (2017b). Spatiotemporal monitoring forest landscape for giant panda habitat through high learning-sensitive neural network in guanyinshan nature reserve in the qinling mountains, china. Environ. Earth Sci. 76:589. doi: 10.1007/s12665-017-6926-9
Liu, X. (2001). Mapping and modelling the habitat of giant pandas in Foping Nature Reserve, China. Enschede, NL: Febodruk BV.
Liu, X., and Jin, X. (2008). Habitat feature of giant pandas’ high-frequency activity area on southern slope of the qinling mountains and habitat selection of giant panda. Chinese J. Ecol. 27, 2123–2128.
Liu, X., and Li, J. (2008). Scientific solutions for the functional zoning of nature reserves in china. Ecol. Model. 215, 237–246. doi: 10.1016/j.ecolmodel.2008.02.015
Liu, X., Liu, X., Shao, X., Songer, M., He, B., He, X., et al. (2018). Plant diversity patterns of temperate forests with logging and restoration practices in northwest china. Eco. Eng. 124, 116–122. doi: 10.1016/j.ecoleng.2018.09.017
Liu, X., Shu, J., and Zhang, L. (2010). Research on applying minimal cumulative resistance model in urban land ecological suitability assessment: as an example of xiamen city. Acta Ecol. Sin. 30, 421–428. doi: 10.1115/1.859599.paper52
Liu, X., and Sun, Y. (2010). Evaluating and structuring indicators for wetland assessment. F. Environ. Sci. Eng. China 4, 221–227. doi: 10.1007/s11783-010-0029-0
Lovejoy, T. E., Rankin, J. M., Bierregaard, R. Jr., Brown, K. S. Jr., Emmons, L. H., and Van der Voort, M. E. (1984). Ecosystem decay of Amazon forest remnants. Extinctions. Chicago: University of Chicago Press, 295–325.
Lu, Z., Johnson, W. E., Menotti-Raymond, M., Yuhki, N., Martenson, J. S., Mainka, S., et al. (2001). Patterns of genetic diversity in remaining giant panda populations. Conserv. Biol. 15, 1596–1607. doi: 10.1046/j.1523-1739.2001.00086.x
May, R. M. (1975). Islands biogeography and the design of wildlife preserves. Nature 254, 177–178. doi: 10.1038/254177a0
McRae, B. H. (2006). Isolation by resistance. Evolution 60, 1551–1561. doi: 10.1111/j.0014-3820.2006.tb00500.x
McRae, B. H., Dickson, B. G., Keitt, T. H., and Shah, V. B. (2008). Using circuit theory to model connectivity in ecology, evolution, and conservation. Ecology 89, 2712–2724. doi: 10.1890/07-1861.1
McRae, B. H., and Paul, B. (2007). Circuit theory predicts Gene flow in plant and animal populations. PNAS 104, 19885–19890. doi: 10.1073/pnas.0706568104
Meurk, C. D. (1988). Nature conservation: the role of remnants of native vegetation. J. Roy. Soc. New Zeal. 18, 344–346. doi: 10.1080/03036758.1988.10429159
Moilanen, A., and Hanski, I. (2001). On the use of connectivity measures in spatial ecology. Oikos 95, 147–151. doi: 10.1034/j.1600-0706.2001.950116.x
Nekhay, O., Arriaza, M., and Guzmán-Álvarez, J. R. (2009). Spatial analysis of the suitability of olive plantations for wildlife habitat restoration. Comput. Electron. Agr. 65, 49–64. doi: 10.1016/j.compag.2008.07.012
Nelson, E., Mendoza, G., Regetz, J., Polasky, S., Tallis, H., Cameron, D., et al. (2009). Modeling multiple ecosystem services, biodiversity conservation, commodity production, and tradeoffs at landscape scales. F. Ecol. Environ. 7, 4–11. doi: 10.1890/080023
Oh, K., and Jeong, S. (2007). Assessing the spatial distribution of urban parks using GIS. Landscape Urban Plan 82, 25–32. doi: 10.1016/j.landurbplan.2007.01.014
Ouyang, Z., Liu, J., Xiao, H., Tan, Y., and Zhang, H. (2000). An assessment of giant panda habitat in wolong nature reserve. Acta Ecol. Sin. 21, 1869–1874.
Pan, W., Lü, Z., Wang, D., and Wang, H. (2001). The opportunity for the Giant Panda to exist. Beijing, China: Peking University Press.
Possingham, H., Ball, I., and Andelman, S. (2000). “Mathematical methods for identifying representative reserve networks,” in Quantitative Methods for Conservation Biology, eds S. Ferson and M. A. Burgman, (Berlin: Springer), 291–306.
Prugh, L. R., Hodges, K. E., Sinclair, A. R. E., and Brashares, J. S. (2009). Effect of habitat area and isolation on fragmented animal populations. PNAS 105, 20770–20775. doi: 10.1073/pnas.0806080105
Qing, J., Xu, C., Yang, B., Yang, Z., Qi, D., Yang, X., et al. (2016). Corridors designed for the giant panda in xiaoxiangling mountains. Acta Ecol. Sin. 36, 1–9. doi: 10.5846/stxb201406131225
Reid, D. G., Taylor, A. H., Hu, J. C., and Qin, Z. S. (1991). Environmental influences on bamboo bashania fangiana growth and implications for giant panda conservation. J. Appl. Ecol. 28, 855–868. doi: 10.2307/2404212
Saunders, D. A., Hobbs, R. J., and Margules, C. R. (1991). Biological consequences of ecosystem fragmentation: a review. Conserv. Biol. 5, 18–32. doi: 10.1111/j.1523-1739.1991.tb00384.x
Sawyer, S. C., Epps, C. W., and Brashares, J. S. (2011). Placing linkages among fragmented habitats: do least-cost models reflect how animals use landscapes? J. Appl. Ecol. 48, 668–678. doi: 10.1111/j.1365-2664.2011.01970.x
Schipper, J., Chanson, J. S., Chiozza, F., Cox, N. A., Hoffmann, M., Katariya, V., et al. (2008). The status of the world’s land and marine mammals: diversity, threat, and knowledge. Science 322, 225–230. doi: 10.1126/science.1165115
Schonewald-Cox, C. M., Stephen, M. C., MacBryde, B., and Thomas, L. (1983). Genetics and conservation: a reference for managing wild animal and plant populations. Menlo Park, CA: The Benjamin-Cummings Publishing Company.
SFA. (2006). The third national survey report on the giant panda in China. Beijing, China: Science Press.
SFA. (2015). The fourth national survey report on the giant panda in China. Beijing, China: Science Press.
Songer, M., Delion, M., Biggs, A., and Huang, Q. (2012). Modeling impacts of climate change on giant panda habitat. Int. J. Ecol. 4872, 1–12. doi: 10.1155/2012/108752
St-Louis, V., Forester, J. D., Pelletier, D., Be’lisle, M., Desrochers, A., Rayfield, B., et al. (2014). Circuit theory emphasizes the importance of edge-crossing decisions in dispersal-scale movements of a forest passerine. Landscape Ecol. 29, 831–841. doi: 10.1007/s10980-014-0019-x
Swaisgood, R. R., Wei, F., Wildt, D. E., Kouba, A. J., and Zhang, Z. (2010). Giant panda conservation science: how far we have come. Biol. Lett. 6, 143–145. doi: 10.1098/rsbl.2009.0786
Walpole, A. A., Bowman, J., Murray, D. L., and Wilson, P. J. (2012). Functional connectivity of lynx at their southern range periphery in ontario, canada. Landscape Ecol. 27, 761–773. doi: 10.1007/s10980-012-9728-1
Wang, F., Mcshea, W. J., Wang, D. J., Li, S., Zhao, Q., Wang, H., et al. (2014). Evaluating landscape options for corridor restoration between giant panda reserves. PLoS One 9:e105086. doi: 10.1371/journal.pone.0105086
Wang, Y., Gong, H., and Li, X. (2007). Analysis of the culture landscape accessibility based on minimum cumulative resistance model. Geos. Inform. 5, 45–47. doi: 10.3969/j.issn.1672-4623.2007.04.017
Wu, P., Liu, X., Shao, X., Zhu, Y., and Cai, Q. (2013). GIS application in evaluating the potential habitat of giant pandas in guanyinshan nature reserve, shaanxi province. J. Environ. Inform. 21, 55–62. doi: 10.3808/jei.201300232
Xu, W., Ouyang, Z., Jiang, Z., Zheng, H., and Liu, J. (2005). Assessment of giant panda habitat in the daxiangling mountain range, sichuan, china. Chinese Biod. 14, 223–231. doi: 10.1360/biodiv.050191
Xu, W., Ouyang, Z., Viña, A., Zheng, H., Liu, J., and Xiao, Y. (2006). Designing a conservation plan for protecting the habitat for giant pandas in the qionglai mountain range, china. Divers. Distrib. 12, 610–619. doi: 10.1111/j.1366-9516.2006.00236.x
Xu, W., Viña, A., Qi, Z., Ouyang, Z., Liu, J., Liu, W., et al. (2014). Evaluating conservation effectiveness of nature reserves established for surrogate species: case of a giant panda nature reserve in qinling mountains, china. Chinese Geog. Sci. 24, 60–70. doi: 10.1007/s11769-014-0656-7
Yin, K., Xie, Y., and Wu, N. (2006). Corridor connecting giant panda habitats from north to south in the min mountains, sichuan, china. Integ. Zool. 1, 170–178. doi: 10.1111/j.1749-4877.2006.00032.x
Yu, K. J. (1998). Ecologically strategic points in landscape and surface model. Acta Geog. Sin. 53, 11–20. doi: 10.11821/xb1998s1002
Yu, K. J., Li, W., Li, D. H., Li, C. B., Huang, G., and Liu, H. L. (2005). Suitability analysis of heritage corridor in rapidly urbanizing region: a case study of taizhou city. Geog. Res. 24, 69–76. doi: 10.11821/yj2005010008
Zeller, K. A., McGarigal, K., and Whiteley, A. R. (2012). Estimating landscape resistance to movement: a review. Landscape Ecol. 27, 777–797. doi: 10.1007/s10980-012-9737-0
Keywords: giant panda, the Qinling Mountains, dispersal route, modeling, minimum cumulative resistance, Current Theory
Citation: Liu X, Liu L, Liu L, Jin X and Songer M (2021) Modeling Potential Dispersal Routes for Giant Pandas in Their Key Distribution Area of the Qinling Mountains, China. Front. Ecol. Evol. 9:636937. doi: 10.3389/fevo.2021.636937
Received: 03 December 2020; Accepted: 23 March 2021;
Published: 29 April 2021.
Edited by:
Yi Zou, Xi’an Jiaotong-Liverpool University, ChinaReviewed by:
Songtao Guo, Northwest University, ChinaDamber Bista, The University of Queensland, Australia
Copyright © 2021 Liu, Liu, Liu, Jin and Songer. This is an open-access article distributed under the terms of the Creative Commons Attribution License (CC BY). The use, distribution or reproduction in other forums is permitted, provided the original author(s) and the copyright owner(s) are credited and that the original publication in this journal is cited, in accordance with accepted academic practice. No use, distribution or reproduction is permitted which does not comply with these terms.
*Correspondence: Xuehua Liu, eHVlaHVhLWhqeEB0c2luZ2h1YS5lZHUuY24=