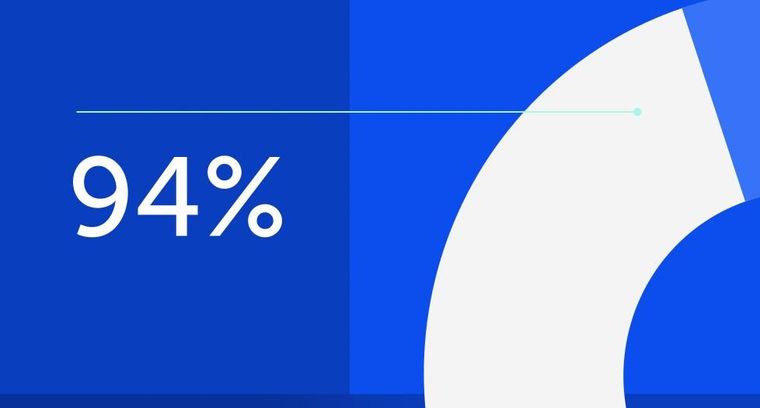
94% of researchers rate our articles as excellent or good
Learn more about the work of our research integrity team to safeguard the quality of each article we publish.
Find out more
REVIEW article
Front. Ecol. Evol., 04 March 2021
Sec. Evolutionary and Population Genetics
Volume 9 - 2021 | https://doi.org/10.3389/fevo.2021.630397
This article is part of the Research TopicLife and Death: New Perspectives and Applications in Forensic ScienceView all 13 articles
Forensic medicine has, for a long time, been relying on biochemical, anthropologic, and histopathologic evidences in solving various investigations. However, depending on the method used, lengthy sample processing time, scanty sample, and less sensitivity and accuracy pervade these procedures. Accordingly, newer arenas such as the thanatomicrobiome have come forward to aid in its quandaries; furthermore, the parallel advances in genomic and proteomic techniques have complemented and are still emerging to be used in forensic experiments and investigations. Postmortem interval (PMI) is one of the most important aspects of medico-legal investigations. The current trend in PMI estimation is toward genomic analyses of autopsy samples. Similarly, determination of cause of death, although a domain of medical sciences, is being targeted as the next level of forensic casework. With the current trend in laboratory sciences moving to the discovery of newer disease-specific markers for diagnostic and prognostic purposes, the same is being explored for the determination of the cause of death by using techniques such as Real-Time PCR, DNA micro-array, to Next-Gen Sequencing. Establishing an individual’s biological profile has been done using medicolegal methods and anthropology as well as bar-bodies/Davidson bodies (gender determination); and in cases where the determination of age/gender is a challenge using morphological characteristics; the recent advances in the field of genomics and proteomics have played a significant role, e.g., use of mitochondrial DNA in age estimation and in maternity disputes. The major hurdle forensic medical research faces is the fact that most of the studies are conducted in animal models, which are often difficult to mimic in human and real-time scenarios. Additionally, the high accuracy required in criminal investigations to be used in a court of law as evidence has prevented these results to come out of the labs and be used to the optimum. The current review aims at giving a comprehensive and critical account of the various molecular biology techniques including “thanatogenomics,” currently being utilized in the veritable fields of forensic medicine.
Death eventuates the emergence of a vast, complex ecosystem. The natural process of decomposition after death comprises of physical and biochemical changes that provide critical information about time since death, location, and manner of death. Understanding this process entails an understanding of the human genetic, physiologic, metabolic, and, most importantly, environmental diversity imparted and shared by our microbial cohabitants. The term microbiome was suggested by Lederberg “to signify the ecological community of commensal, symbiotic, and pathogenic microorganisms that share our body space” (Lederberg and McCray, 2001; NIH HMP Working Group et al., 2009). The substantially sizeable spatiotemporal diversity of the human microbiome is not only dynamic but also often predictable, which makes them potential candidates to serve as markers for various intrinsic and extrinsic factors (Grice and Segre, 2011; Human Microbiome Project Consortium, 2012a; David et al., 2014; Chevalier et al., 2015; Zhou and Bian, 2018). On the one hand, they influence our health and disease predispositions and, on the other hand, play an essential role in the decay process (Hyde et al., 2013). It has revolutionized our perception of the complex dynamics that unfold after death.
Earlier, much of our knowledge used to come from culture-based approaches which, although species-specific, cannot account for the majority of the uncultivable microbes and their diversity (Zhou et al., 2004; Bik et al., 2006). The advent of high-throughput or next-generation sequencing (NGS) has brought about the necessary alternative to study the human microbiome as well as expand its practice in forensic genetic investigations. Metagenomic sequencing studies carried out as part of the Human Microbiome Project have helped us identify several body sites harboring complex and significantly different microbial communities within and among individuals (Human Microbiome Project Consortium, 2012a).
Thanatomicrobiome is the microbial community associated with the host after its death and is named after the Greek god of death, Thanatos (Javan et al., 2016a). It characterizes the microbial succession in and around dead biomass and contributes significantly to modulating decomposition (Javan et al., 2017). In parallel, the potential of using genomic data in forensic medicine has increased manifold, giving rise to thanatogenomics. Even though the human microbiome essentially includes bacteria, fungi, viruses, and other unicellular organisms, it is the bacteria which are of utmost importance in the forensic context because of their diversity and their primary role in the decay process (American Society for Microbiology, 2013). Moreover, they are not only spread throughout the different parts of the body but are also consolidated in certain areas such as the oral cavity and intestine, creating particular niches. The internal organs such as the heart, liver, and spleen are sterile in healthy humans, but within 24 h of the postmortem period, there is a proliferation of the microbiome in the internal organs (Gevers, 1975). The overall progression of the decay process is dependent upon several external and internal factors and plays an important role in determining the accuracy of the predictive methods in forensic sciences. The thanatomicrobial community has two distinctive components: the microbiome of the internal organs of cadavers and the microbiome associated with external body surfaces, the latter also termed epinecrotic microbiome (Javan et al., 2016a). With the aid of genomic and molecular advances in the past decade, the thanatomicrobiome has emerged as a tool of enormous potential in the arsenal of forensic medicine (Alan and Sarah, 2012).
In this review, we have attempted a comprehensive account of the potential applications of the thanatomicrobiome in forensic medicine, primarily on the postmortem interval (PMI), and cause of death, focusing on the possible microbial marker profiles and their robustness as well as limitations. The estimation of time since death is fundamental in establishing useful information regarding the time of death, necessary information that needs to be presented in the court of law. It is also one of the ad rem challenges for forensic specialists all over the world as far too many variables affect the postmortem changes, making it difficult to rely on one specific method. In the early postmortem period, temperature nomogram, rigor mortis, and hypostasis are considered as the standard tools in day-to-day practice (Madea, 2016). The temperature nomograms use algor mortis as the basis for calculating time since death, the Henssge’s nomogram being one of the most popular methods (Henssge, 1988). The other methods in early postmortem period include histopathological and biochemical methods. Degenerative changes in the skin, which initiates as vacuolation of the corpus basale and spinosum, start appearing 6 h after death. The disintegration of the dermis is complete by 18 h (Bardale et al., 2012). The biochemical methods include postmortem biochemical markers in tissue and body fluids (Donaldson and Lamont, 2014). The body fluids include vitreous humor, synovial fluid, pericardial fluid, and cerebrospinal fluid. Some of these markers which are well investigated in the literature are potassium, sodium, urea, chloride, hypoxanthine, and cardiac troponin T (Meurs et al., 2019). In the late postmortem period, the body starts disintegrating, and this is known as decomposition. This period is divided into fresh, bloat, early decay, advanced decay, and skeletal remains (Metcalf, 2019). During this period, when ample time has passed after death, PMI estimates with the available methods of algor, rigor, or livor mortis may no longer be applicable, and various other methods, such as forensic entomology and molecular assessment, have to be relied upon, which all have their own limitations and weaknesses (Pittner et al., 2020). The determination of the cause of death is, again, vital in narrowing down the circumstances in which a person died or suffered an injury. In autopsy-negative cases, the DNA can be extracted from the blood and tissue samples of the cadaver to possibly analyze the cause of death, a method termed as “molecular autopsy” (Ackerman et al., 2001). Similarly, the thanatomicrobial samples can be collected and analyzed through the available high-throughput platforms to aid in forensic investigations. These methods, too, come with their own pitfalls and limitations.
Our literature search included the keywords “thanatomicrobiome,” “microbiome,” “forensic medicine,” “postmortem interval,” “postmortem submersion interval,” “cause of death,” and “gut microbiome” on the PubMed, Embase, and Scopus databases between October 2019 and December 2020. The search results were sorted for the articles which focused on the application of thanatomicrobiome in forensic medicine. Most of the articles dealt with PMI as it is the most extensively studied area for the thanatomicrobiome (Figure 1). Additionally, we have briefed upon the various technologies and methods that have been in use for microbial profiling and the newer approaches and practices that are coming up in thanatomicrobiome studies.
Forensic medicine and genomics go a long way back. Minisatellites and microsatellites were proposed for human identification by Sir Alec Jeffreys. Restriction fragment length polymorphism (RFLP), which required intact DNA in micrograms, was primarily used for human identification (Børsting and Morling, 2016). Next came the highly sensitive polymerase chain reaction (PCR)-based mitochondrial DNA (mtDNA) sequencing which could be used to type even degraded samples in trace amounts, e.g., hair shafts (Sullivan et al., 1992). This technique has been extensively in use and gave rise to the creation of the European DNA Profiling Group’s mtDNA population database project (EMPOP) (Parson and Bandelt, 2007). With further advances in sequencing methods such as Sanger’s chain termination method and capillary electrophoresis, eventually, short tandem repeat (STR) assays took over, followed by single-nucleotide polymorphism base extension (Mitchelson, 2003; Børsting and Morling, 2016). These methods have been used extensively in forensic casework (Phillips et al., 2007; Børsting et al., 2009; Walsh et al., 2014). Because of their polymorphic nature, STRs are given high weight in human identification. Overall, the current applications for DNA profiling include identification of crime suspects, identification of dead bodies or human remains, matching with profiles of criminals, e.g., the Combined DNA Indexing System (CODIS) of the Federal Bureau of Investigation (FBI), and genealogical DNA testing with microsatellites on the Y-chromosome, respectively. CODIS is a database of more than 12 million human DNA profiles and has aided in countless criminal investigations. This only emphasizes the importance of genomic technologies in forensic medicine. However, the applications of genomic technologies in forensics are not limited to human samples alone and involve the amalgamation of multiple disciplines (Arenas et al., 2017; Javan et al., 2019).
Micro-organisms show intra- and inter-individual variation in terms of number and distribution in different body sites (Human Microbiome Project Consortium, 2012a). Hence, comprehensive microbial profiling, which is an essential step in identifying relevant markers in forensic medicine, includes both characterizing the species that are present and the extent of their abundance (Human Microbiome Project Consortium, 2012b; Zhou and Bian, 2018).
In the pre-NGS era, accurate microbial profiling involved simple culturing and finding out interactions between co-cultured taxa; these methods were constrained by their limited number of taxa and interactions as well as failed to provide the dynamics of the entire community (Malla et al., 2019). Culture-independent methods have several advantages in this regard: direct extraction of the genetic material from the sample of interest, discovery and characterization of a vast number of microbial species which were otherwise unidentifiable or undefined with previous techniques, and obtaining a high-throughput profile of the microbial community by genome sequencing (Gill et al., 2006; Schloissnig et al., 2013; Adserias-Garriga et al., 2017a; Almeida et al., 2019). Massively parallel sequencing, aided further by robust bioinformatics pipelines, has evolved as a boon by allowing microbial analysis and overcoming the challenges of identifying unknown and low-abundance microorganisms (Schmedes et al., 2016). Due to its high throughput, low cost, and reduced turnaround time, it is a powerful technique to gain insight into the human thanatomicrobiome, possibly to infer into existing disease states as well as past medical conditions, human identification, PMI estimation, and decomposition analysis (Cho and Blaser, 2012; Schmedes et al., 2016).
Overall, the currently available tools for the genetic profiling of the thanatomicrobiome have shown a distinct shift toward the newer and advanced molecular biology techniques. These include DNA extraction kits as well as group-specific PCR primers and metagenomics and whole-genome sequencing (Figure 2). Some commercially available DNA isolation techniques are the QIAamp DNA stool minikit (Qiagen), Inhibitor Removal Technology column (Carlsbad), and PowerSoil® DNA isolation kit (MoBio Laboratories, Inc.) (Finley et al., 2015; Hauther et al., 2015; Lawrence et al., 2019). To identify the relative quantities of key microbial groups for the suitability of PMI estimation, group-specific primers have been developed and used successfully (Matsuki et al., 2002; Liszt et al., 2009).
There are two primary metagenomic (direct genetic analysis of entire communities of organisms) approaches (Figure 3) that have been in use: marker gene- or amplicon-based sequencing and shotgun whole genome sequencing (WGS). In the former, a genomic region is amplified, which is conserved within a taxon but variable among taxa. Common marker genes include 16S rRNA gene for prokaryotes, 18S rRNA gene for eukaryotes, and internal transcribed spacer for fungi (Zhou and Bian, 2018). The most commonly used bacterial genetic marker to identify microbial taxa present in a community is the 16S rRNA gene. It is a single phylogenetic marker which can provide a more in-depth coverage but cannot differentiate at the species level. The reasons for which it is widely used for molecular identification are as follows: (a) the relatively short 16S rRNA gene has hypervariable regions appropriately sized for the metagenomic platforms and (b) the conserved regions of this gene allow for designing universal primers to target the hypervariable regions describing the microbial diversity (Bell et al., 2018). It has been observed that the V4 hypervariable region is the most sensitive for microbial signatures. The segments juxtaposed to V2 and V4 hypervariable regions have, again, the highest accuracy for taxonomic determination (Chakravorty et al., 2007; Bell et al., 2018). However, this approach has certain limitations. 16S rRNA data profiling has low discriminatory power for some taxa, and it cannot provide higher than genus-level resolution (Mignard and Flandrois, 2006). It is also limited to the marker gene regions; hence, the functional traits of the community are left out of the investigation. Finally, there are technical issues like the purity of the isolates and primer selection bias (Janda and Abbott, 2007).
Figure 3. A schematic representation of amplicon-based and shotgun metagenomic sequencing procedures.
Shotgun sequencing of the metagenome, on the other hand, accounts for higher taxonomic resolution and less bias and provides a greater level of diversity (Jovel et al., 2016; Malla et al., 2019). WGS also gives a functional inference about the microbial community as it engages in a genome-wide approach using strings of genomic DNA sequences and matching them to an annotated database of known DNA sequences. It can provide strain-level characterization by producing sequence reads of strain-specific markers (Schmedes et al., 2016). For strain-specific microbial identification alongside functional profiling, the GSMer approach and the HuMiChip2 microarray platform have been developed (Tu et al., 2014, 2017). Multi locus sequence typing (MLST)-based identification methods such as MetaMLST can characterize single strains of known species (Zolfo et al., 2018) and can be applied to profile the human thanatomicrobiome communities. However, analysis of this massive scale of data usually requires the development of efficient and accurate computational pipelines and comprehensive annotation of reference databases, which pose a challenge in itself (Quince et al., 2017). The processing decisions required for the data analyses involve a series of transformations using executable standard line software. These decisions ultimately have downstream effects on the results and interpretation of the data; hence, any application of the thanatomicrobiome in the criminal justice system would require streamlined standard operating procedures (Leipzig, 2016; Kaszubinski et al., 2020a).
For the 16S amplicon sequencing analysis, taxonomy, and community characterization, the standard process uses operational taxonomic unit-based or amplicon sequence variant (ASV)-based clustering tools, such as Quantitative Insights Into Microbial Ecology 2 (QIIME2), Mothur, and Divisive Amplicon Denoising Algorithm (DADA2) (Nguyen et al., 2016; Bharti and Grimm, 2019). Current studies mainly employ 16S rRNA sequencing considering that it can provide consistent estimates, is economical and time-saving, and is suitable for a large number of samples to screen microbial markers for estimating time and location of death. Other approaches involving transcriptome and proteome analysis are also gaining popularity.
Finally, while metagenomic analyses give insights about community structure, meta-transcriptomic investigation sheds light upon the functional profiles of the community. Both of these analytic methods together, at varying stages of decomposition, have shown potential in PMI estimations (Burcham et al., 2019a, b). Moreover, total RNA sequencing can prove to be a cost-effective method compared to WGS, as it is equally reliable and has fewer chances of overestimating microbial diversity from sequencing errors (Kunin et al., 2010; Burcham et al., 2019a).
Being ubiquitous and having erectable ecologies, there is no denying the potential of microbes as physical evidence for forensic science. However, several hurdles in forensic sciences still need to be overcome. Metcalf has outlined some lacunae that need to be filled before utilizing the thanatomicrobiome in the estimation of PMI (Metcalf, 2019). The same can be extended to other potential medicolegal applications. These include the time frames in which microbes can be most informative, the external variables that need to be incorporated in the microbial models, the sample types and sample location ideal for addressing a particular problem, the stage of investigation in which the microbial sample should be collected, and the most robust modeling methods. These issues, along with particular other challenges, are discussed below.
The highly diverse human microbial community shows high inter-individual variability depending upon food habits, age and sex, ethnicity, geographical provenance, and presence of pathological conditions, among other factors, and this potentially affects the thanatomicrobiome composition and, consequently, the microbial succession (Arumugam et al., 2011; Wu et al., 2011; David et al., 2014; Dehingia et al., 2015; Rehman et al., 2016; Gupta et al., 2017; Angelakis et al., 2018; Gaulke and Sharpton, 2018; Senghor et al., 2018; de la Cuesta-Zuluaga et al., 2019). These can grossly be divided into abiotic and biotic factors. A clear understanding of these factors and their interactions will help in devising generalized models for use in forensics.
The abiotic factors include time, temperature, humidity, pH, and antemortem living habits, e.g., diet and drugs. The biotic factors, on the other hand, include insects, scavenger population, commensals, and antemortem infections (Dash and Das, 2020).
An increase in temperature considerably changes the decomposition process in dead bodies (Benbow et al., 2018). It also significantly alters the bacterial colonization of tissues (Iancu et al., 2018). Furthermore, the rate and degree of decomposition are greatly increased if the cadaver is exposed to water (Abad Santos, 2019). Generally, the decomposition process happens faster in humid climates. Hence, a colder environment and lesser relative humidity will thwart microbial proliferation to a degree (Jordan and Tomberlin, 2017).
The gut microbiota, in its composition and diversity, is closely related to dietary habits and food products. Bacteroidetes and Actinobacteria populations are positively associated with a fat-rich diet but negatively with fibers, whereas Firmicutes and Proteobacteria have a reverse association (Wu et al., 2011; Senghor et al., 2018). A comparative study of animal- and plant-based diets showed that the former led to a decrease in Firmicutes (Roseburia spp., E. rectale, and R. bromii), while the latter increased the abundance of Bacteroides spp. and Bilophila spp. (David et al., 2014). Accordingly, there is country-based diversity; the gut microbiome in western populations with a more prevalent protein- and fat-rich diet is rich in Firmicutes (Blautia, Roseburia, Ruminococcus, and Clostridium difficile), Actinobacteria (B. adolescentis and B. catenulatum), and Bacteroidetes (Bacteroides), while Asian people have an intermediate gut microbial diversity with dominant species being Prevotella, Butyrivibrio, and Staphylococcus aureus (Arumugam et al., 2011; Senghor et al., 2018). This variability extends within the different regions of the same country as well, as shown in an NGS-based study on Indian populations of distinct cultures, traditions, and diet (Dehingia et al., 2015). However, the sizeable microbial diversity among populations of different countries cannot be explained by diet alone (Nishijima et al., 2016). Other factors such as lifestyle and subsistence strategies, host genetics, and altitude of living are also involved (Nam et al., 2011; Schnorr et al., 2014; Das et al., 2018; Jha et al., 2018).
The use of antibiotics is of considerable significance in thanatomicrobiome analysis. The alteration of the gut microbiota in prolonged antibiotic use is well established. The effects are twofold: (1) it makes the host susceptible to other infections, e.g., C. difficile colitis (Norén, 2010) and (2) it causes colonization of the gut by antibiotic-resistant organisms (Keeney et al., 2014; Nogueira et al., 2019). In a recent study, Clostridium was found to be the most abundant genus in the liver and spleen of drug overdose victims (Brackett, 2018). Additionally, Bacteroides and Alistipes were found abundantly in the gut of individuals on laxatives (Vila et al., 2020). Thus, antibiotic use during the lifetime has to be taken into account while looking for thanatomicrobiome signatures.
Other environmental variables like soil type and insect activity may also affect the predictable microbial succession during decomposition. However, even though the decomposer community is derived from soil, soil type itself is not an independent factor for community development, and thus decomposition is a sufficiently reproducible process (Metcalf et al., 2016). In the presence and absence of insect populations, the microbial succession process during decomposition can be identified by specific taxa for each group (Guo et al., 2016). Finally, body mass can be a pivotal factor, as it has been seen that larger carcasses decompose slower and release higher levels of nitrogen required for the growth of microbial communities after the death of the host (Spicka et al., 2011; Deel et al., 2020). On the contrary, the characterization of soil microbial communities of four different Sus scrofa carcasses revealed no significant differences through decomposition (Weiss et al., 2016). These instances show that, even though environmental variables can influence microbial communities, they may not necessarily affect the succession all the time. However, these studies had only a handful of samples and were carried out with animal models, and further human studies are necessary to understand how these and other factors impact the succession of microbial communities and the accuracy of the microbial clock.
High-throughput sequencing technologies have opened new frontiers in a cost-effective analysis of the microbial communities. However, it is not without its share of shortcomings. Firstly, the characterization of the microbiome in most studies has been achieved at the upper taxonomic ranks. This alone cannot serve the purpose of individualistic human identification we are ultimately aiming for. Secondly, sample preparation methods involving nucleic acid extraction remain a notable area of creating a bias toward various microbial taxa (Yuan et al., 2012). Most studies cited in the latter sections of this review have used the 16S rRNA gene amplicon sequencing. The conserved regions of the gene, the available databases, and a large number of studies on this marker in the existing literature make it an attractive target (Schmedes et al., 2016). Nevertheless, it suffers from insufficient species resolution, sequence and copy number variation in a single bacterium, PCR bias, inaccurate relationships based on variability outside of the marker region, and horizontal transfer of the gene region (Fox et al., 1992; Suzuki and Giovannoni, 1996; Wang et al., 1997; Klappenbach et al., 2000; Schouls et al., 2003; Janda and Abbott, 2007; Soergel et al., 2012). These may lead to misclassified reads, inaccurate quantification, and confounded analyses. Bioinformatic tools like Phylogenetic Investigation of Communities by Reconstruction of Unobserved States (PICRUSt), which can predict functional gene profiles from the relative abundance of all known taxa, can be handy but only for known species (Langille et al., 2013). Shotgun metagenomics, on the other hand, can differentiate at the species level, but it has less depth of coverage for particular sites of the genome. Complex samples, containing thousands of species, will suffer from limited coverage of particular genomes, especially those communities with low abundance and coming from a trace amount of samples.
Additionally, the sequencing platform used can itself be a source of technical bias and hinder the accurate determination of microbial composition and functional profile (Rutty et al., 2015; Hao et al., 2017). For instance, 454 technology has a low yield, a variable length of sequence reads, and a high rate of insertion/deletion errors, whereas the ion torrent platform has a higher rate of sequencing errors (Salipante et al., 2014; Hao et al., 2017). Earlier, Illumina sequencers, although with high throughput, could produce reads only 100 bases in length and hence could not provide enough taxonomic information for reliable species-level resolution. In comparison, the 454 pyrosequencing system has a longer read length (Liu et al., 2012). Computational limitations also include high cost and sufficiently large computing power. Moreover, algorithms that are capable of analyzing the data in a biologically relevant manner are on demand (Rothberg and Leamon, 2008; Petrosino et al., 2009). Identifying the microbe of interest within a highly complex metagenomic sample also requires its resolution from its near neighbors as well as from a vast community creating background noise. A highly sensitive and accurate detection technique is imperative in this scenario. Such targeted markers or marker panels should not only provide species-level resolution but must also be developed in a cost-effective method, balancing the sequence coverage and throughput. Newly developed technologies in implementation should be validated, and sample analysis has to focus more on a molecular level to identify specific and individualized microbial signatures. Last but not least, the variability in the working knowledge of the technicians and engineers and, subsequently, the inter-laboratory variation that exists, but difficult to evaluate, have to be taken into account. The development of novel quality control metrics, especially for cases where a high-quality reference is not available, could be useful in reducing the sequencing errors (Trivedi et al., 2014).
To apply the thanatomicrobial profiles in the veritable field of forensic sciences, universally applicable models should be in place with validated protocols, which incorporate temporal and seasonal microbial changes while taking care of other sources of variation (Figure 4), such as host characteristics and environments. These models are challenging to establish due to the limited amount of available data as well as a lack in the generalization of existing model systems. A great barrier for the applicability of the thanatomicrobiome in creating such models for PMI estimation is the lack of human cadaver-associated data sets from different environments encompassing diverse populations. Many experiments are carried out using pigs as animal surrogate models as they are similar to humans in diet, body fat, and body hair coverage (Schoenly et al., 2006). However, pig and human bacterial communities are non-comparable (Furet et al., 2009). Comparative fecal metagenomics revealed that the pig fecal phyla are closer to that of cow rumen and chicken cecum (Lamendella et al., 2011). A comparison of the skeletal muscle tissue decomposition between human, pork, beef, and lamb showed that no single analog could precisely predict human decomposition in the soil, although they are all close approximations of the human decomposition dynamics (Stokes et al., 2013). Moreover, most studies on human thanatomicrobiome so far have been limited to the North American population. In the context of this enormous diversity and associated shortcomings, efforts should be focused on (a) studies including populations from diverse geographical and ethnic backgrounds, (b) individualizing the microbiome profiles while trying to incorporate the existing variables and chalking out the microbes common to humans, (c) determining the portions of these microbes which vary due to the environmental and living conditions mentioned above, and (d) selecting those bacteria which are relatively abundant to reduce stochastic sampling effects. Moreover, while building multivariate models with the thanatomicrobiome data, care should be taken not to overfit. Adding a new covariate is desirable only when it improves the model significantly over the expected natural increase in precision associated with it, or we risk losing the generalizability of the model. Overall, these advances will result in the development of a defined set of markers for the human microbiome and target genes or sequences containing sufficient variation to generate a profile that can provide a sufficient degree of individualization of the donor(s) of biological evidence for aiding in human identity testing, PMI estimation, and determination of the cause of death.
Figure 4. Development of microbial models. To address the gaps in knowledge, new technology is adopted, and research is carried out on microbial community succession and functionality. Data obtained from these experiments are used to build machine learning models (with training and validation sets), which are then fine-tuned with available best parameters to eventually be used for unknown microbial samples.
To summarize, the limitations pertaining to these upcoming methods are twofold: on the one hand, they deal with extreme population variation as well as ecological diversities and, on the other, the technological aspects still have a lot of area for improvement in creating more human cadaver-associated databases and streamlining the bioinformatic pipelines. By extension, the current knowledge gaps in the applicability of the thanatomicrobiome in forensic medicine are (a) the sample types and locations most fitting to provide the best possible evidence in criminal cases, (b) the time frames in which microbial evidence is most informative, (c) the most robust predictive models, and (d) standard bioinformatic pipelines. In the following sections, these issues have been addressed in order to differentiate (1) anecdotal cases or cases based on certain environments and (2) identify those having a more general scope in forensic and medicolegal investigations.
The presence of specific microbes can act as hidden evidence or bioindicator for the cause of death. In general, if we recover a single microbial species from body fluids at autopsy, it is suggestive of an infection incurred during life, while a mixed profile points toward a postmortem invasion (Alan and Sarah, 2012). This can prove to be useful in confirming the diagnosis of an antemortem infection, identifying the etiological agent of a previously undiagnosed infectious disease, or identifying microbial markers for particular types of death (Ventura Spagnolo et al., 2019) (Table 1).
A recent multinational study focused on different anatomical sites in human cadavers with various causes of death such as accident, suicide, and homicide found that the manner of death has a significant influence on the microbial community in various postmortem organs, e.g., brain, liver, uterus, and prostate (Lutz et al., 2020). The PMI and cause of death were associated with the alpha and beta diversity of the tissue microbial profiles (Lutz et al., 2020). Similar associations were observed previously where the beta dispersion differed significantly between anatomical body sites and causes and manners of death (Kaszubinski et al., 2020b). A different microbial signature was also observed in cardiovascular disease cases. For the associations between relative bacterial abundance and manners of death, ASVs from the families MLE1-12, Enterobacteriaceae, and Chitinophagaceae were positively associated in natural deaths, whereas 10 unique ASVs from the Bacilli class were negatively associated with homicide (Lutz et al., 2020). These findings imply that microbial signature variability could be measured through beta diversity in predicting the cause or manner of death.
An extensive study on 336 body fluid samples from 129 autopsies, involving bacterial culture, 16S rRNA identification, and matrix-assisted laser desorption/ionization-time of flight (MALDI-TOF), to establish a correlation between microbial isolates and cause of death found correlations in six out of eight seawater drowning cases. Enterococcus and Enterobacter were isolated in the cardiac blood and peritoneal fluid of a patient who died of colon perforation and cardiopulmonary arrest, showing the migration of the colon communities of Enterobacteriaceae to peritoneal fluid and cardiac blood (Na et al., 2017). A kinetic postmortem survey of the microbiota in culture-positive extra-intestinal tissue found bacterial translocation to extra-intestinal compartments such as mesenteric lymph nodes (MLNs), spleen, liver, kidney, and cardiac blood 5 min after death, the highest rates being in MLNs (Heimesaat et al., 2012). The highest detection rate was observed in organs for Lactobacillus at 3 and 12 h postmortem. Such rapid translocation of intestinal bacteria could be useful in distinguishing between primary pathogenic bacteria and secondary putrefactive contaminants not only while investigating causes of unknown deaths but also for screening tissue donors before allogeneic transplantation. Investigating the dynamics of bacterial translocation and intestinal dysbiosis in contributing to the early stages of liver disease, Fouts et al. (2012) found that there is an increase in intestinal permeability independent of microbiome changes or endotoxin and translocation of families Enterobacteriaceae, Enterococcaceae, and Bacillaceae, primarily to the MLNs, in cholestatic liver injury. The postmortem transmigration of Staphylococcus aureus and Clostridium perfringens during murine decomposition can help in determining the cause of death and track down the origins associated with the geographic location of the human remains during postmortem investigations (Burcham et al., 2016). In 10 heart samples from individuals who died of cardiac arrest, Bell et al. (2018) studied the heart thanatomicrobiome and found sex-related differences in male and female corpses at the bacterial phylum (Firmicutes in males and Proteobacteria in females), class (Bacilli in males and γ-Proteobacteria in females), order (Lactobacillales and Rhizobiales only detected in males and Pseudomonadales in females), family (Streptococcaceae only detected in males), and genus (Streptococcus and Lactobacillus in males) levels, which demonstrated that microbial populations form ecological niches differently in the hearts of males and females after death (Bell et al., 2018). They also found Proteobacteria to have the highest abundance, which indicates a relationship between heart thanatomicrobiome and cardiac diseases; as Proteobacteria, usually found in the gut, can act as precursors to inflammation; it can also cause dysbiosis and an increased risk of heart disease, as it has been shown as an independent marker of risk of cardiovascular disease in previous antemortem studies (Amar et al., 2013; Metcalf et al., 2013; Shin et al., 2015). From a cross-sectional study of an urban–industrial population, decreased phylogenetic diversity was a significant predictor of heart disease in a <24-h time window, where the dominant taxa were Streptococcus, Prevotella, Fusobacterium, and Rothia (Pechal et al., 2018). Furthermore, Rothia, along with other additional community metrics, can be used as a biomarker for hosts with chronic dysbiosis (Pechal et al., 2018). Such qualitative and quantitative changes in the microbiome can pave new ways of identifying existing disease conditions of the deceased.
The presence of diatoms in blood or organs (e.g., lungs) has long since been the gold-standard indicator to diagnose drowning and whether it is antemortem or postmortem. However, in rare cases, when identification through diatoms is impractical, other microbes can aid in providing informative results. In the past, methods employed to identify these organisms have included light and electron microscopy, bacterial culture, and PCR (Lunetta et al., 1998; He et al., 2008; Lucci et al., 2008; Tie et al., 2010; Huys et al., 2012; Sitthiwong et al., 2014; Rácz et al., 2016). A culture study with 10 drowning victims and three postmortem submersions revealed fecal coliform (E. coli) and fecal streptococci (E. faecalis) to be useful markers for drowning (Lucci et al., 2008). Aeromonads in the blood can also be considered as a potential marker for freshwater drowning, as it has been shown by another culture study (Huys et al., 2012). Uchiyama et al. (2012) developed a multiplex real-time PCR protocol for assaying bacterioplankton in drowning cases. In 43 victims, they found related microbes, e.g., Aeromonas for freshwater drowning and Vibrio and Photobacterium for seawater drowning in all lung samples compared to non-drowning victims. This method was further applied to 20 cadavers, where it was found that this method can potentially be used as a high-throughput supportive diagnostic test for antemortem drowning (Rutty et al., 2015). Kakizaki et al. (2012) used the 454 pyrosequencing approach to ascertain the cause of death of two drowning cases and found that it is possible to determine diverse aquatic microbes in drowning victims that can point toward the aquatic environment in which the drowning occurred. An animal study sampled for lungs, kidney, liver, and blood, and found significant aquatic microbial presence in the drowned group compared to closed organs of postmortem immersion group (Lee et al., 2017). Aquatic bacterial communities were further dominated by Proteobacteria (seawater), Bacteroidetes (freshwater), Actinobacteria, and Bacillariophyta (Lee et al., 2017).
The risk factors for sudden infant death syndrome (SIDS), defined by the unexpected death of an otherwise healthy infant aged less than 1 year, include genetic predisposition, immunological disorder, exogenous stressors, and undiagnosed infections/sepsis. The common microbial agents associated with SIDS are human herpes virus, enterovirus, respiratory syncytial virus, adenovirus, cytomegalovirus, Epstein–Barr virus, Streptococcus pneumoniae, Bordetella pertussis, Haemophilus influenzae, and Neisseria meningitidis (Fernández-Rodríguez et al., 2006; Alvarez-Lafuente et al., 2008; Oliveira and Amorim, 2018; Ventura Spagnolo et al., 2019). SIDS has been part of many a legal investigation because of the possibility of crime in infants of this age group. Routine postmortem protocols exist in the form of serology testing and PCR assays for many bacteria and viruses in specimens from autopsies. However, comprehensive microbial profiling has not yet been developed in this arena, and it can potentially provide us with etiology-specific disease diagnoses. Such a panel, accompanying autopsy findings and antemortem clinical information, can be a handy tool to ascertain causes of death due to infection and rule out other causes in criminal investigations.
However, it is worth noting that microorganisms can be influenced by environmental conditions, and variation in microbial population will exist at different PMI; hence, considering these, utmost care must be practiced while relying on a microbial signature solely for the cause of death. Furthermore, using a combination of laser microdissection and microbial typing, the cause of death of a child from the 18th century was resolved to be osteomyelitis caused by Pseudomonas aeruginosa (D’Argenio et al., 2017). Such findings should prompt us to preserve samples of unknown clinical cases for future innovative technologies.
Reliable methods of PMI estimation is of utmost importance, as it has invaluable relevance to the inceptive phase of criminal investigations. Inferring the PMI has long been based on observatory data from direct body inspection, viz., body cooling, postmortem lividity, and rigor mortis, to name a few (Salam et al., 2012). However, beyond the compound method, many other methods have come up in the aid of PMI (Sharma et al., 2015; Madea et al., 2019). Accumulated degree days (ADD), a unit representing the amount of time an organism spends at a temperature within its development threshold, can be a useful tool in that it can be practiced for decomposition data across various environments and regions (Megyesi et al., 2005). Biochemical assessment can be advantageous after cellular death and when redistribution of electrolytes has set in. A commonly used biochemical test is the vitreous humor potassium concentration (Adjutantis and Goutselinis, 1972). A combination of multiple methods can be useful, e.g., vitreous humor potassium, combined with vitreous humor hypoxanthine and ambient temperature, has shown encouraging results (Rognum et al., 2016). However, biochemical markers come with their own limitations due to the variability in age, sex, cause of death, and other factors. Furthermore, time dependency plays a pivotal role in the usability of these markers in PMI estimation (Woydt et al., 2018). Most biochemical markers have predictive ability if the cadaver is still in the early phases of its postmortem period. As time progresses and the body enters the advanced phases of decomposition, the PMI estimation becomes more difficult.
Methods and technology that can objectively measure with high accuracy and precision are being considered (Donaldson and Lamont, 2014). Moreover, the potential of ecological succession patterns of small organisms or insect species as an indicator of PMI has been long established (Tomberlin et al., 2011). Forensic entomology is an integral part of forensic investigations when the time since death is beyond 3 days (Kashyap and Pillai, 1989). However, due to their seasonal and developmental variation, it is difficult to practically impose them in all circumstances. Information on the geographical variation of many of these forensically important insects is also unknown (Klong-Klaew et al., 2018). Henceforth, the postmortem microbiome has shown much promise as a novel, alternative, and precise method to aid in the estimation of PMI, as they are ubiquitous and have predictable ecologies, on the premise that bacterial succession changes in a predictable and clock-like manner across mammalian species within the same environment in response to the changes in a decomposing resource (Deel et al., 2020).
Research revolving around the applicability of the human microbial community in PMI includes sampling from different organs (Figure 5 and Table 2), as the pattern of community succession differs at various time points of decomposition according to the location (Deel et al., 2020).
Figure 5. Illustration of different body sites for thanatomicrobiome sampling from existing studies. Boxes indicate the dominant phyla in the microbial communities in that respective organ/body part.
External locations facilitate an easily accessible, non-invasive manner of sampling for the epinecrotic microbial community. In this regard, the skin is the most important site in forensic applications. It also provides specific locations on the human body with their own niche of microorganisms. This is important because the microbial population in a particular site, e.g., oral cavity is, at the same time, different from that of the other body locations and similar across individuals. In a study carried out at the Southeast Texas Applied Forensic Science laboratory, two human corpses each were allowed to decompose in spring and winter seasons, which revealed that a suite of bacterial and fungal groups form a reproducible network that emerges on a predictable time scale and, hence, can serve as estimators of PMI (Metcalf et al., 2016). Two other human subjects were sampled from the internal left buccal region, external left/right cheeks, external left/right biceps, torso, and rectum (Hyde et al., 2015). With the progress of decomposition, the microbial diversity and richness for both bodies were similar for the skin, mouth, and rectal samples. Besides that, Acinetobacter of γ-Proteobacteria class was abundant in both bodies in the late decomposition stages. Another study by the same group found that communities from the mouth and oral cavity differ in pre-bloat and end-bloat stages of decomposition (Hyde et al., 2013). Oral microbial community sampling from three donated individuals showed different dynamics in bacterial composition as the decomposition process progresses through fresh, bloat, active and advanced decay, and dry remains stages and had similar overall successional changes. Firmicutes and Actinobacteria were predominant in the fresh stage, while Clostridiales and Bacillaceae were the principal representatives of Firmicutes from bloat to advanced decay (Adserias-Garriga et al., 2017b). Although the sample sizes for the aforementioned studies are less and variable, the similarity in the microbial succession across the different stages of decomposition is a pivotal finding, as it reflects the possibility of using the oral microbiome in PMI estimation. An analysis of the surface communities residing in the ear and nasal canals of 17 cadavers led to the successful development of a k-nearest neighbor regression model, which could accurately predict the true PMI to within 55 ADD (Johnson et al., 2016). In a larger study involving 188 subjects, samples were collected from various sites such as the eyes, ear, nose, mouth, and rectum and analyzed to show that predictive models from composition and functional profiles, in the context of each sample location, could be used to estimate PMI within the time frame of less than as well as greater than 2 days (Pechal et al., 2018). Zhang et al. (2019) utilized the datasets from the study mentioned above and employed machine learning algorithms in an attempt to predict PMI and found that xgboost method resulted in highest accuracy compared to random forest and neural network while incorporating all anatomical areas. The xgboost method is a tree-based machine learning method which grows sequentially into a single tree by fitting on residuals. The accuracy of this method was 0.745 for PMI and 0.876 for the location of death. It is worth noting that cases do not always allow for a sample collection from all anatomical sites. Therefore, the aforementioned studies simultaneously serve the purpose of highlighting the anatomical areas which are most important in postmortem casework and the types of sample that must be collected to provide the best possible information. A meta-analysis using the QIITA open-source platform and subsequent model testing using data from the first 25 sample days of each included study indicated that skin data using the 16S rRNA genetic marker performs reasonably in accurately predicting PMI at the phylum level (Belk et al., 2018). Another recent study demonstrated that, upon repeated sampling, the postmortem skin microbiome is stable for up to 60 h (Kodama et al., 2019). They studied 16 scenes of death to see whether objects present at the scene can be linked to the decedents and found that, overall, it could be traced three-fourth of the time with single-user based items, such as smoking pipes and medical devices being the most accurate. The findings are similar to those of previous studies which identified mobile phones as having personal microbial signatures of the user (Meadow et al., 2014; Lax et al., 2015). Overall, these findings point toward the skin and the oral cavity being potentially promising sites to build an accurate microbial clock upon. Furthermore, the skin microbiome can also be extended to act as complementary evidence to establish associations and identifications, e.g., for the analysis of partial or smudged fingerprints for better comparison. Notably, skin commensals are highly resistant to humidity; hence, the epinecrotic thanatomicrobiome signatures can be utilized for deducing models of PMI estimation (Javan et al., 2016b). However, the small number of cadavers available for some of these studies, as well as the inability to account for the PMI in donated cadavers, requires further evaluation of the skin microbiome (Javan et al., 2016a). The skin microbiome is also in a state of dynamic flux due to its constant exposure to the environment. Therefore, the microbial community may not always be exclusive to the individual, and the skin bacterial population truly representative of a particular environment, e.g., coryneforms in moist environments, should also be taken into consideration.
Contrary to external body surfaces, internal organs are not directly under the influence of external environmental factors in the initial stages of decomposition. The decomposition of the various human organs also happen in a different order of progression, where organs such as the stomach, intestine, liver, and pancreas undergo decomposition first and that of tendons, bones, and nulligravid uterus happen last (Javan et al., 2016b). Characteristic microbial succession in the internal sites and organs can thus be utilized to predict PMI. In 11 cases in which the PMI ranged from 20 to 240 h, organ thanatomicrobiome analyses suggested that facultative anaerobes, such as Lactobacillus, predominate in cadavers with short PMI (29.5 h), while cadavers with long PMI (240 h) have a predominance of obligate anaerobes like Clostridium (Can et al., 2014). In a cross-sectional study involving 27 human cadavers from criminal cases with known PMI, statistically significant organ-, sex-, and time-dependent differences were found in the thanatomicribome communities of the brain, heart, liver, spleen, buccal cavity, and blood (Javan et al., 2016b). With the increase in the depth of taxonomic levels, the prediction models could better explain (65% at the species level compared to 21% at the family and genus level) the variance in PMI due to different variables. Notably, Firmicutes (e.g., Clostridium, Peptoniphilus, and Bacillus) was a stable biomarker across microbial communities derived from different body locations. Thus, organ-specific microbial signatures can possibly be useful in PMI estimation. Species-specific identification of microbes must also be emphasized upon when going for thanatomicrobiome signatures. However, ambient-temperature exposure, which imparts significant influence on the microbial community, was not accounted for in this study (Javan et al., 2016b).
A postmortem microbiome of liver and spleen revealed Clostridium sp. in 95% of the samples. In the 16S rRNA gene, the V4 hypervariable region could sufficiently discriminate Clostridium sp. in postmortem tissue, where it predominates in both short (4 h) and long (10 days) PMIs (Javan et al., 2016b, 2017). This ubiquity of Clostridium sp. is explained by its rapid generation times, the proteolytic activity resulting in transmigration to other tissues, and hypoxic environment, together known as the postmortem clostridium effect. In line with this finding, Clostridium spp. was the most abundant organism isolated from the postmortem liver and spleen tissues of drug overdose subjects (Brackett, 2018). Moreover, the preponderance of Pseudomonas sp. was detected exclusively in internal organs such as the brain, heart, liver, spleen as well as the blood of female cadavers, while males contained the facultative anaerobe Rothia (Javan et al., 2016b). This organ- as well as sex-specific difference is also important for pathologists working on decomposing human bodies for location, identity, and possible cause of death.
A targeted qPCR study from human large intestinal microflora showed that Bacteroides and Lactobacillus declined exponentially with increasing PMI (Hauther et al., 2015). In contrast, Bifidobacterium did not show any significant trends, pointing out the potential of the former two microbes as quantitative indicators of PMI. This was further validated by an amplicon sequencing experiment, which revealed a microbial succession over time toward a common decay community in four bodies, wherein Bacteroides and Parabacteroides decreased, while Clostridium and Anaerosphaera, as well as Ignatzschineria and Wohlfahrtiimonas, increased significantly (DeBruyn and Hauther, 2017). Notably, the latter two are fly associated γ-Proteobacteria, and therefore they could provide a better understanding of PMI estimation by augmenting the existing options. Overall, these findings may help build predictive models of different thanatomicrobiome communities according to the internal organ microbial signatures.
As a potential extension of the microbial clock from internal organs to skeletal remains, Damann et al. (2015) collected the lower rib from 12 decomposing bodies. An interstage taxonomic succession of Firmicutes, Bacteroidetes, Actinobacteria, and Acidobacteria from partially skeletonized remains to dry remains was observed. For each successive decay stage, α-Proteobacteria increased, while γ-Proteobacteria decreased. The communities associated with the partially skeletonized remains (PMI of 27–284 days) were closer to those of the human gut (Firmicutes and Bacteroidetes), while those of the dry remains (PMI of 554–1,692 days) mimicked that of the soil (Actinobacteria and Acidobacteria). Similar findings were obtained from the soil samples of three cadavers: Proteobacteria and Bacteroidetes dominated the first 6 days of decomposition, after which there was a sudden shift to an increase in Firmicutes, with a concomitant decrease in Proteobacteria (Adserias-Garriga et al., 2017a).
The decomposition soil of 18 human subjects showed increased taxon richness and decreased evenness and a consistent diversity with buried remains, whereas surface cadavers showed a decrease in all parameters, alluding to the importance of the depth of burial as it can affect the microbial community composition and, subsequently, PMI estimations (Finley et al., 2016). Moreover, the microbial succession of the soil communities is complete by at least 420 days after burial (Thomas et al., 2017). Hence, in longer PMI or dry remains stage, the soil communities are analogous with varying depths. It is still well to remember that the decomposition process varies widely depending on hot or cold environmental conditions, as a colder climate can preserve intact cadavers for longer periods of time. Hence, the anatomical location of sampling is crucial as is the surrounding environment, as external body sites which allow for non-invasive sample collections, despite providing an accurate microbial clock, are more prone to environmental changes, especially in hotter climates, whereas internal body sites, although less impacted by the environment, require invasive sampling. A 2013 study demarcated liver and pericardial fluid as best sampling sites and concluded that postmortem sampling is to be done within 5 days (Tuomisto et al., 2013). This is because pericardial fluid aspiration needs no handling of organs in the abdominal cavity and can be easily carried out with a sterile needle. For estimating longer PMI, the microbial successions of bones or burial soil are, again, better choices. A combination of all these parameters can be taken into account after careful consideration of the location of death, time frame, and convenience of sampling.
Many research groups have employed animal models such as swine (Dickson et al., 2011) and mice models (Dong et al., 2019) (Table 3), as these are similar to human bodies to the extent that they can provide a better understanding of the decomposition process in humans whereas being more readily available at the same time compared to human bodies (Javan et al., 2016a; Zhou and Bian, 2018). They are also low in cost compared to carrying out experiments on human cadavers.
Metcalf et al. (2013) in five dead and decomposing mice over 48 days at eight time-points each, used high-throughput sequencing to study samples taken from the abdominal cavity, skin of head and torso, and associated grave soil to infer that the succession of prokaryotic and eukaryotic microbial communities may be useful in the estimation of PMI in early stages of decomposition, especially within 3 days (Metcalf et al., 2013). They suggested a microbial clock to provide an accurate PMI estimate as shifts in the presence and abundance of specific bacterial and eukaryotic taxa in skin and soil sites were consistent during the decomposition stages. Epinecrotic community composition and succession analyses showed that relatively abundant phyla like Proteobacteria, Firmicutes, Bacteroidetes, and Actinobacteria, under their notable variation during the decomposition process, can aid in PMI estimation (Guo et al., 2016). Indeed, metagenomic sequencing of epinecrotic bacterial communities showed that phylum- and family-level taxonomic resolution could explain 62.1 and 78.5% variation in physiological time, respectively, throughout decomposition (Pechal et al., 2014). A phylum-level taxonomic resolution in a random forest model with Campylobacteraceae, Moraxellaceae, Aerococcaceae, Micrococcaceae, Clostridiales Incertae Sedis XI, Comamonadaceae, and Fusobacteriaceae was the better choice to distinguish between days of decomposition (Pechal et al., 2014). The oral bacterial community, in a study on adult mice, showed distinct characteristic features at different taxonomic levels. Within 10 days after death, Proteobacteria and Firmicutes dominate, with γ-Proteobacteria showing a strong positive linear correlation with PMI. At the family and genus levels, the relative abundance of Enterobacteriaceae and Proteus increased with an increase in PMI (Dong et al., 2019). Furthermore, the postmortem microbiome in 20 Dutch rabbits showed organ- and temperature-specific changes in the community, which is in line with the findings in human cadavers. The intestinal thanatomicrobiome was also different in composition compared to those of the kidneys and lungs (Lawrence et al., 2019). Another study on the postmortem structure and function dynamics of Staphylococcus aureus by whole-body fluorescent imaging and culture-based methods showed that it reaches its highest concentration on 5–7 days postmortem and, thus, can potentially be tracked to determine PMI ranges (Burcham et al., 2016).
In gnotobiotic mice associated with human microbiota (hfa), enterococci and enterobacteria thrived in ileal lumen 3 and 24 h postmortem, respectively, pointing toward the fact that these microbes can possibly be used to precisely define PMI under specific ambient conditions (Heimesaat et al., 2012). The intestinal flora diversity in SD rats reduced again up to the 30th day postmortem, whereas the intragroup similarity showed a downward trend, indicating that the succession of intestinal flora is related to PMI (Li et al., 2018). With a T-RFLP approach, decomposing pork loin showed a stable abundance of Enterobacteriaceae 24 h onward (Handke et al., 2017). β-Proteobacteria was present within the first few days, while α-Proteobacteria became abundant 15 days onward. Firmicutes were present in the beginning as well as on 40-, 50-, and 60-day samples, thus making them significant in the estimation of prolonged PMI. However, Comamonadaceae and Clostridiaceae were only present up to 24 h, which was contradictory to previous findings from other groups. This difference can either be attributed to environmental variation or the different techniques used by other groups, primarily 454 pyrosequencing or Illumina sequencing technologies. The proteomic analysis of the same sample could identify several bacterial subspecies and strains, namely Rhodopseudomonas palustris strain BisB18 and Baizongia pistaciae strain Bp (Handke et al., 2017). However, high signals from muscle peptides prevent the possibility of appropriately identifying bacteria-specific peaks. Overall, these studies indicate that Proteobacteria and Firmicutes show significant variability in abundance with time after death, and may be considered as potentially useful in the estimation of PMI. Finally, an RNA-based denaturing gradient gel electrophoresis analysis of an isolated pig leg bacterial community in the context of soil bacterial profile revealed that soil communities were significantly different compared to control soil (fresh sandy loam garden soil without any carcass), especially in the advanced phase of decay, implying the importance of soil bacterial community profiling to identify secret burials (Bergmann et al., 2014). The terrestrial communities’ analysis in the form of transcriptome or proteome of the microbes can also prove to be a useful tool in PMI estimation. However, accuracy can be badly affected by the mode of sample collection and environmental temperature.
Postmortem submersion interval (PMSI), which is the amount of time human remains have been partially or fully submerged in an aquatic environment, demands a different approach as the decomposition process in water bodies is slower with adipocere formation due to anaerobic environment when compared with that of a terrestrial environment due to the effect of water temperature, salinity, tides, depth, mobility of the carcass, and scavengers (Caruso, 2016; He et al., 2019). Epinecrotic community from S. scrofa carcasses revealed not only significant temporal shifts in the bacterial communities at the phyletic and generic levels with the progression of the decomposition process but also significant seasonal variation between summer and winter (Benbow et al., 2015). Furthermore, using automated ribosomal intergenic spacer analysis method on S. scrofa carcasses, epinecrotic biofilms were shown to exhibit significant succession during the first 2 weeks, implying its possible use to estimate PMSI (Lang et al., 2016). An experiment on three swine heads for the bacterial succession data across two seasons, five stages of decomposition, and bacterial communities showed site- and season-specific preponderance and colonization (Dickson et al., 2011). Proteobacteria, Firmicutes, Bacteroidetes, and Actinobacteria were found to be the predominant phyla in epinecrotic rat samples, which showed dynamic variation, with Proteobacteria decreasing while Firmicutes increasing during decomposition, the latter becoming the dominant phylum on days 5 and 6 (He et al., 2019).
Furthermore, Clostridiaceae and Comamonadaceae were the most abundant families which displayed different succession patterns, as the former was abundant on days 1, 4, 5, and 6, while the latter decreased from day 4 onward. Thus, the detection of a combination of Clostridiaceae with Comamonadaceae could help narrow down the PMSI estimation (He et al., 2019). Currently, PMSI estimation is mainly derived from the inspection of the gross morphological findings of the recovered remains, like ADDs and total aquatic decomposition score (De Donno et al., 2014). Nevertheless, the abovementioned studies point toward the possibilities of novel methods which can bring about PMSI estimation not only for whole bodies but also for separated body parts as a result of pre- or post-submersion dismemberment.
Although mammalian models provide an idea of the applicability of the microbiome in PMI estimation, these experiments are carried out in tightly controlled, predictable environments, which means that the data have to be validated on human cadavers before making its way into the forensic toolbox. It is, therefore, well to remember that studies on animal models can only be able to support certain proposals made on humans and must not be treated as equivalent to human studies.
We have to pay attention to adopting the new methods into the medicolegal system, the path to which will not be a smooth one. Currently, no laws exist as such regarding the use of thanatomicrobiome evidence in criminal investigations. The collection of microbial evidence from the crime scene or the individual for comparison is sure to bring newer legal and ethical concerns. The first steps in incorporating the thanatomicrobiome into the courtroom are the admissibility of microbial evidence and collection of samples. This depends largely upon the existing laws and regulations holding up the standards of the criminal justice system in a country, but as long as the method (a) has been widely tested, (b) has widely accepted operation standards, (c) is generally accepted in the scientific community, and (d) has known potential quantifiable error rates, the thanatomicrobiome could be made admissible as expert testimony. One other issue likely to present itself as a hurdle, as microbial evidence starts being introduced into the courtroom, is the complexity of the statistical analysis, as it can potentially confuse and distract the jury. The standards for statistical analyses, therefore, need to be stricter, accompanied by sound theory, but long before these issues can be delved into in depth, solid groundwork needs to be carried out to regularize the entire process.
Metcalf has suggested a step-by-step approach on how this can be achieved—by identifying the needs, primary research, prototype development, and validation and acceptance, leading to adoption (Metcalf, 2019). This requires communication between the researcher and the medicolegal community to identify the main factors that need to be addressed by microbial technologies. The stakeholders, in this case, are the forensic community including law enforcers and medical examiners, the research community, the technology manufacturers, and the decision-makers. Basic research in collaboration with the forensic community and connecting to the legal system by the proper use of technology will help overcome many limitations. First, practical needs such as the sample collection stage or collection site need to be identified to be incorporated into the forensic workflow. Then, the research community can connect to the end-users in the justice and legal system by communicating their findings and help develop newer, robust, and cost-effective technologies. Currently, coordinated efforts, such as the American Gut Project, Earth Microbiome Project, QIIME2 platform with options for machine learning analysis, rapid drop in sequencing costs, and more and more standardized datasets coming up, can help the transition of a prototype from an academic lab to a practitioner a lot easier, taking into consideration that sampling and data generation methods undergo rigorous quality control, which is still challenging. Validation is essential in the development of any new technology, more so in forensic medicine since these methods affect the conviction or acquittal of individuals, policy decisions, and government actions on a larger scale (Schmedes et al., 2016). Every method, therefore, must be validated, including but not limited to its sensitivity, specificity, accuracy, the limit of detection, and error rate, before implementation. Moreover, this must happen on a local level, in connection with the end-users and legal community, since thanatomicrobial tools are always likely to be developed based on data from a particular population and specific geolocation. Hence, the need of the hour is the validation of large, population-, ethnicity-, and location-based datasets.
To realize the potential of human thanatomicrobiome characterization in forensic sciences, we should incorporate additional methods already available to or recently coming up to expand the horizons. More advanced sequencing methods can be developed and streamlined for data elucidation from complex metagenomic samples. Genomic data alone is not sufficient to get the picture beyond the species richness of the microbial communities. The level of gene transcription and the functional protein profiles are unknown even when we identify the genomic DNA of a species. Other “omic” studies, such as transcriptomics, proteomics, and metabolomics, have become far more accessible than before, and these areas should be further ventured into thanatomicrobiome research. Whole transcriptome analysis of the microbes is an exciting prospect, as it provides insights into gene expression and function in an organ-, tissue-, or condition-specific manner (D’Argenio, 2018; Heintz-Buschart and Wilmes, 2018). In fact, mRNA transcripts degrade differently for different tissues depending upon postmortem gene regulation and expression signatures (Scott et al., 2020). Publicly available bioinformatic pipelines like SAMSA have been developed for meta-transcriptomic data analysis (Westreich et al., 2016). The high sensitivity, rapidity, and its broad spectrum in characterizing microorganisms make mass spectrometry an intriguing option to be utilized in thanatomicrobiome work. Bottom-up proteomics approaches like MALDI-TOF and LC-MS/MS have already been used successfully in identifying bacteria (Fenselau, 2013; Handke et al., 2017). Proteomics can also rule out causes of infection by investigating the presence of bacterial protein components. Finally, it can help in elucidating the functionality, understanding, and succession of the gut microbiome in disease states and postmortem (Toscano et al., 2018). These prospects make metaproteomics and thanatoproteomics potential and forthcoming major areas in forensic microbiology research.
Microbial succession after death is a complex process, and different independent estimates could throw light on the same forensic variable. These estimates, then, can be combined within a Bayesian framework to improve the accuracy of the said variable. Such statistical frameworks can draw from several observations and need not necessarily be confined to the thanatomicrobiome alone to resolve the problem at hand, such as time since death. Furthermore, machine learning methods are being widely employed, keeping in pace with the massive amount of data that is being generated and will only grow with time. These methods can help in the prediction of pre-existing pathological conditions; as we know, many diseases affect human colonies. They can also link microbial communities’ host phenotypes and other environmental parameters (Qu et al., 2019). As we incorporate multiple “omic” data types and diverse human thanatomicrobiome datasets and continue to grow upon our computational arsenal, we will improve our understanding of the succession of the thanatomicrobial communities.
Determining the time of death within a narrow window has always been of utmost importance and equally challenging. We have often pondered as death investigators, as we are always coerced to do a retrospective search from tremendously variable and often incomplete medical reliquiae, whether it is even possible to develop foolproof technologies. Nevertheless, the promise of applicability is there, in PMI estimation through different phases of decomposition, in studying aquatic microbial succession as a tool for PMSI, as well as to differentiate between antemortem and postmortem and between freshwater and saltwater drowning, in quantifying the human epinecrotic communities, and in diagnosing or ruling out infectious causes of death. Newly developing technologies and the parallel development of computational pipelines are promising and have made it possible for the facilitation of generalizable microbial models, specific for each environment, into the medicolegal system and aid in forensic investigations, to exist in our foreseeable future.
PS and PP conceived the manuscript. DR and ST conducted the literature and designed the tables and figures. PP, DR, and ST drafted the manuscript. PS and PP edited the manuscript. All the authors contributed to the article and approved the submitted version.
The authors declare that the research was conducted in the absence of any commercial or financial relationships that could be construed as a potential conflict of interest.
Ackerman, M. J., Tester, D. J., and Driscoll, D. J. (2001). Molecular autopsy of sudden unexplained death in the young. Am. J. Forensic Med. Pathol. 22, 105–111. doi: 10.1097/00000433-200106000-00001
Adjutantis, G., and Goutselinis, A. (1972). Estimation of the time of death by potassium levels in the vitreous humour. Forensic Sci. 1, 55–60. doi: 10.1016/0300-9432(72)90147-1
Adserias-Garriga, J., Hernández, M., Quijada, N. M., Rodríguez Lázaro, D., Steadman, D., and Garcia-Gil, J. (2017a). Daily thanatomicrobiome changes in soil as an approach of postmortem interval estimation: an ecological perspective. Forensic Sci. Int. 278, 388–395. doi: 10.1016/j.forsciint.2017.07.017
Adserias-Garriga, J., Quijada, N. M., Hernandez, M., Rodríguez Lázaro, D., Steadman, D., and Garcia-Gil, L. J. (2017b). Dynamics of the oral microbiota as a tool to estimate time since death. Mol. Oral Microbiol. 32, 511–516. doi: 10.1111/omi.12191
Almeida, A., Mitchell, A. L., Boland, M., Forster, S. C., Gloor, G. B., Tarkowska, A., et al. (2019). A new genomic blueprint of the human gut microbiota. Nature 568, 499–504. doi: 10.1038/s41586-019-0965-1
Alvarez-Lafuente, R., Aguilera, B., Suárez-Mier, M. A., Morentin, B., Vallejo, G., Gómez, J., et al. (2008). Detection of human herpesvirus-6, Epstein-Barr virus and cytomegalovirus in formalin-fixed tissues from sudden infant death: a study with quantitative real-time PCR. Forensic Sci. Int. 178, 106–111. doi: 10.1016/j.forsciint.2008.02.007
Amar, J., Lange, C., Payros, G., Garret, C., Chabo, C., Lantieri, O., et al. (2013). Blood microbiota dysbiosis is associated with the onset of cardiovascular events in a large general population: the D.E.S.I.R. study. PloS One 8:e54461. doi: 10.1371/journal.pone.0054461
American Society for Microbiology (2013). FAQ: Human Microbiome. Washington, DC. Available online at: https://www.ncbi.nlm.nih.gov/books/NBK562894/ (accessed January 7, 2021).
Angelakis, E., Bachar, D., Yasir, M., Musso, D., Djossou, F., Melenotte, C., et al. (2018). Comparison of the gut microbiota of obese individuals from different geographic origins. New Microbes New Infect. 27, 40–47. doi: 10.1016/j.nmni.2018.11.005
Arenas, M., Pereira, F., Oliveira, M., Pinto, N., Lopes, A. M., Gomes, V., et al. (2017). Forensic genetics and genomics: much more than just a human affair. PLoS Genet. 13:e1006960. doi: 10.1371/journal.pgen.1006960
Arumugam, M., Raes, J., Pelletier, E., Le Paslier, D., Yamada, T., Mende, D. R., et al. (2011). Enterotypes of the human gut microbiome. Nature 473, 174–180. doi: 10.1038/nature09944
Bardale, R. V., Tumram, N. K., Dixit, P. G., and Deshmukh, A. Y. (2012). Evaluation of histologic changes of the skin in postmortem period. Am. J. Forensic. Med. Pathol. 33, 357–361. doi: 10.1097/PAF.0b013e31822c8f21
Belk, A., Xu, Z. Z., Carter, D. O., Lynne, A., Bucheli, S., Knight, R., et al. (2018). Microbiome data accurately predicts the postmortem interval using random forest regression models. Genes 9:104. doi: 10.3390/genes9020104
Bell, C. R., Wilkinson, J. E., Robertson, B. K., and Javan, G. T. (2018). Sex-related differences in the thanatomicrobiome in postmortem heart samples using bacterial gene regions V1-2 and V4. Lett. Appl. Microbiol. 67, 144–153. doi: 10.1111/lam.13005
Benbow, M. E., Barton, P. S., Ulyshen, M. D., Beasley, J. C., DeVault, T. L., Strickland, M. S., et al. (2018). Necrobiome framework for bridging decomposition ecology of autotrophically and heterotrophically derived organic matter. Ecol. Monogr. 89:e01331. doi: 10.1002/ecm.1331
Benbow, M. E., Pechal, J. L., Lang, J. M., Erb, R., and Wallace, J. R. (2015). The potential of high-throughput metagenomic sequencing of aquatic bacterial communities to estimate the postmortem submersion interval. J. Forensic Sci. 60, 1500–1510. doi: 10.1111/1556-4029.12859
Bergmann, R. C., Ralebitso-Senior, T. K., and Thompson, T. J. (2014). An RNA-based analysis of changes in biodiversity indices in response to Sus scrofa domesticus decomposition. Forensic Sci. Int. 241, 190–194. doi: 10.1016/j.forsciint.2014.06.001
Bharti, R., and Grimm, D. G. (2019). Current challenges and best-practice protocols for microbiome analysis. Brief Bioinform. 22, 178–193. doi: 10.1093/bib/bbz155
Bik, E. M., Eckburg, P. B., Gill, S. R., Nelson, K. E., Purdom, E. A., Francois, F., et al. (2006). Molecular analysis of the bacterial microbiota in the human stomach. Proc. Natl. Acad. Sci. U.S.A. 103, 732–737. doi: 10.1073/pnas.0506655103
Børsting, C., and Morling, N. (2016). “Genomic applications in forensic medicine,” in Medical and Health Genomics, 1st edn (Elsevier), 295–309. doi: 10.1016/b978-0-12-420196-5.00022-8
Børsting, C., Rockenbauer, E., and Morling, N. (2009). Validation of a single nucleotide polymorphism (SNP) typing assay with 49 SNPs for forensic genetic testing in a laboratory accredited according to the ISO 17025 standard. Forensic Sci. Int. Genet. 4, 34–42. doi: 10.1016/j.fsigen.2009.04.004
Brackett, E. (2018). Thanatomicrobiome Signatures in Drug Overdose Cases. Thesis, Honors College of Middle Tennessee State University, Murfreesboro, TN.
Burcham, Z. M., Cowick, C. A., Baugher, C. N., Pechal, J. L., Schmidt, C. J., Rosch, J. W., et al. (2019a). Total RNA analysis of bacterial community structural and functional shifts throughout vertebrate decomposition. J. Forensic Sci. 64, 1707–1719. doi: 10.1111/1556-4029.14083
Burcham, Z. M., Pechal, J. L., Schmidt, C. J., Bose, J. L., Rosch, J. W., Benbow, M. E., et al. (2019b). Bacterial community succession, transmigration, and differential gene transcription in a controlled vertebrate decomposition model. Front. Microbiol. 10:745. doi: 10.3389/fmicb.2019.00745
Burcham, Z. M., Hood, J. A., Pechal, J. L., Krausz, K. L., Bose, J. L., Schmidt, C. J., et al. (2016). Fluorescently labeled bacteria provide insight on post-mortem microbial transmigration. Forensic Sci. Int. 264, 63–69. doi: 10.1016/j.forsciint.2016.03.019
Can, I., Javan, G. T., Pozhitkov, A. E., and Noble, P. A. (2014). Distinctive thanatomicrobiome signatures found in the blood and internal organs of humans. J. Microbiol. Methods 106, 1–7. doi: 10.1016/j.mimet.2014.07.026
Caruso, J. L. (2016). Decomposition changes in bodies recovered from water. Acad. Forensic Pathol. 6, 19–27. doi: 10.23907/2016.003
Chakravorty, S., Helb, D., Burday, M., Connell, N., and Alland, D. (2007). A detailed analysis of 16S ribosomal RNA gene segments for the diagnosis of pathogenic bacteria. J. Microbiol. Methods 69, 330–339. doi: 10.1016/j.mimet.2007.02.005
Chevalier, C., Stojanović, O., Colin, D. J., Suarez-Zamorano, N., Tarallo, V., Veyrat-Durebex, C., et al. (2015). Gut microbiota orchestrates energy homeostasis during cold. Cell 163, 1360–1374. doi: 10.1016/j.cell.2015.11.004
Cho, I., and Blaser, M. J. (2012). The human microbiome: at the interface of health and disease. Nat. Rev. Genet. 13, 260–270. doi: 10.1038/nrg3182
Damann, F. E., Williams, D. E., and Layton, A. C. (2015). Potential use of bacterial community succession in decaying human bone for estimating postmortem interval. J. Forensic Sci. 60, 844–850. doi: 10.1111/1556-4029.12744
D’Argenio, V. (2018). Human microbiome acquisition and bioinformatic challenges in metagenomic studies. Int. J. Mol. Sci. 19:383. doi: 10.3390/ijms19020383
D’Argenio, V., Torino, M., Precone, V., Casaburi, G., Esposito, M. V., Iaffaldano, L., et al. (2017). The cause of death of a child in the 18th century solved by bone microbiome typing using laser microdissection and next generation sequencing. Int. J. Mol. Sci. 18:109. doi: 10.3390/ijms18010109
Das, B., Ghosh, T. S., Kedia, S., Rampal, R., Saxena, S., Bag, S., et al. (2018). Analysis of the Gut microbiome of rural and urban healthy indians living in sea level and high altitude areas. Sci. Rep. 8:10104. doi: 10.1038/s41598-018-28550-3
Dash, H. R., and Das, S. (2020). Thanatomicrobiome and epinecrotic community signatures for estimation of post-mortem time interval in human cadaver. Appl. Microbiol. Biotechnol. 104, 9497–9512. doi: 10.1007/s00253-020-10922-3
David, L. A., Maurice, C. F., Carmody, R. N., Gootenberg, D. B., Button, J. E., Wolfe, B. E., et al. (2014). Diet rapidly and reproducibly alters the human gut microbiome. Nature 505, 559–563. doi: 10.1038/nature12820
De Donno, A., Campobasso, C. P., Santoro, V., Leonardi, S., Tafuri, S., and Introna, F. (2014). Bodies in sequestered and non-sequestered aquatic environments: a comparative taphonomic study using decompositional scoring system. Sci. Justice 54, 439–446. doi: 10.1016/j.scijus.2014.10.003
de la Cuesta-Zuluaga, J., Kelley, S. T., Chen, Y., Escobar, J. S., Mueller, N. T., Ley, R. E., et al. (2019). Age- and sex-dependent patterns of gut microbial diversity in human adults. mSystems 4:e261-19. doi: 10.1128/mSystems.00261-19
DeBruyn, J. M., and Hauther, K. A. (2017). Postmortem succession of gut microbial communities in deceased human subjects. PeerJ 5:e3437. doi: 10.7717/peerj.3437
Deel, H., Bucheli, S., Belk, A., Ogden, S., Lynne, A., Carter, D. O., et al. (2020). “Using microbiome tools for estimating the postmortem interval,” in Microbial Forensics, 3rd edn. (Elsevier), 171–191. doi: 10.1016/B978-0-12-815379-6.00012-X
Dehingia, M., Devi, K. T., Talukdar, N. C., Talukdar, R., Reddy, N., Mande, S. S., et al. (2015). Gut bacterial diversity of the tribes of India and comparison with the worldwide data. Sci. Rep. 5:18563. doi: 10.1038/srep18563
Dickson, G. C., Poulter, R. T., Maas, E. W., Probert, P. K., and Kieser, J. A. (2011). Marine bacterial succession as a potential indicator of postmortem submersion interval. Forensic Sci. Int. 209, 1–10. doi: 10.1016/j.forsciint.2010.10.016
Donaldson, A. E., and Lamont, I. L. (2014). Estimation of post-mortem interval using biochemical markers. Aust. J. Forensic Sci. 46, 8–26. doi: 10.1080/00450618.2013.784356
Dong, K., Xin, Y., Cao, F., Huang, Z., Sun, J., Peng, M., et al. (2019). Succession of oral microbiota community as a tool to estimate postmortem interval. Sci. Rep. 9:13063. doi: 10.1038/s41598-019-49338-z
Fenselau, C. C. (2013). Rapid characterization of microorganisms by mass spectrometry—what can be learned and how? J. Am. Soc. Mass Spectrom. 24, 1161–1166. doi: 10.1007/s13361-013-0660-7
Fernández-Rodríguez, A., Ballesteros, S., de Ory, F., Echevarría, J. E., Alvarez-Lafuente, R., Vallejo, G., et al. (2006). Virological analysis in the diagnosis of sudden children death: a medico-legal approach. Forensic Sci. Int. 161, 8–14. doi: 10.1016/j.forsciint.2005.10.012
Finley, S. J., Lorenco, N., Mulle, J., Robertson, B. K., and Javan, G. T. (2015). Assessment of microbial DNA extraction methods of cadaver soil samples for criminal investigations. Aust. J. Forensic Sci. 48, 265–272. doi: 10.1080/00450618.2015.1063690
Finley, S. J., Pechal, J. L., Benbow, M. E., Robertson, B. K., and Javan, G. T. (2016). Microbial signatures of cadaver gravesoil during decomposition. Microb. Ecol. 71, 524–529. doi: 10.1007/s00248-015-0725-1
Fouts, D. E., Torralba, M., Nelson, K. E., Brenner, D. A., and Schnabl, B. (2012). Bacterial translocation and changes in the intestinal microbiome in mouse models of liver disease. J. Hepatol. 56, 1283–1292. doi: 10.1016/j.jhep.2012.01.019
Fox, G. E., Wisotzkey, J. D., and Jurtshuk, P. Jr. (1992). How close is close: 16S rRNA sequence identity may not be sufficient to guarantee species identity. Int. J. Syst. Bacteriol. 42, 166–170. doi: 10.1099/00207713-42-1-166
Furet, J. P., Firmesse, O., Gourmelon, M., Bridonneau, C., Tap, J., Mondot, S., et al. (2009). Comparative assessment of human and farm animal faecal microbiota using real-time quantitative PCR. FEMS Microb. Ecol. 68, 351–362. doi: 10.1111/j.1574-6941.2009.00671.x
Gaulke, C. A., and Sharpton, T. J. (2018). The Influence of ethnicity and geography on human gut microbiome composition. Nat. Med. 24, 1495–1496. doi: 10.1038/s41591-018-0210-8
Gevers, W. (1975). Biochemical aspects of cell death. Forensic Sci. 6, 25–29. doi: 10.1016/0300-9432(75)90220-4
Gill, S. R., Pop, M., Deboy, R. T., Eckburg, P. B., Turnbaugh, P. J., Samuel, B. S., et al. (2006). Metagenomic analysis of the human distal gut microbiome. Science 312, 1355–1359. doi: 10.1126/science.1124234
Grice, E. A., and Segre, J. A. (2011). The skin microbiome. Nat. Rev. Microbiol. 9, 244–253. doi: 10.1038/nrmicro2537
Guo, J., Fu, X., Liao, H., Hu, Z., Long, L., Yan, W., et al. (2016). Potential use of bacterial community succession for estimating post-mortem interval as revealed by high-throughput sequencing. Sci. Rep. 7:24197. doi: 10.1038/srep24197
Gupta, V. K., Paul, S., and Dutta, C. (2017). Geography, ethnicity or subsistence-specific variations in human microbiome composition and diversity. Front. Microbiol. 8:1162. doi: 10.3389/fmicb.2017.01162
Handke, J., Procopio, N., Buckley, M., van der Meer, D., Williams, G., Carr, M., et al. (2017). Successive bacterial colonisation of pork and its implications for forensic investigations. Forensic Sci. Int. 281, 1–8. doi: 10.1016/j.forsciint.2017.10.025
Hao, Y., Pei, Z., and Brown, S. M. (2017). Bioinformatics in microbiome analysis. Methods Microbiol. 44, 1–18. doi: 10.1016/bs.mim.2017.08.002
Hauther, K. A., Cobaugh, K. L., Jantz, L. M., Sparer, T. E., and DeBruyn, J. M. (2015). Estimating time since death from postmortem human gut microbial communities. J. Forensic Sci. 60, 1234–1240. doi: 10.1111/1556-4029.12828
He, F., Huang, D., Liu, L., Shu, X., Yin, H., and Li, X. (2008). A novel PCR-DGGE-based method for identifying plankton 16S rDNA for the diagnosis of drowning. Forensic Sci. Int. 176, 152–156. doi: 10.1016/j.forsciint.2007.08.005
He, J., Guo, J., Fu, X., and Cai, J. (2019). Potential use of high-throughput sequencing of bacterial communities for postmortem submersion interval estimation. Braz. J. Microbiol. 50, 999–1010. doi: 10.1007/s42770-019-00119-w
Heimesaat, M. M., Boelke, S., Fischer, A., Haag, L. M., Loddenkemper, C., Kühl, A. A., et al. (2012). Comprehensive postmortem analyses of intestinal microbiota changes and bacterial translocation in human flora associated mice. PLoS One 7:e40758. doi: 10.1371/journal.pone.0040758
Heintz-Buschart, A., and Wilmes, P. (2018). Human gut microbiome: function matters. Trends Microbiol. 26, 563–574. doi: 10.1016/j.tim.2017.11.002
Henssge, C. (1988). Death time estimation in case work. I. The rectal temperature time of death nomogram. Forensic Sci. Int. 38, 209–236. doi: 10.1016/0379-0738(88)90168-5
Human Microbiome Project Consortium (2012a). A framework for human microbiome research. Nature 486, 215–221. doi: 10.1038/nature11209
Human Microbiome Project Consortium (2012b). Structure, function and diversity of the healthy human microbiome. Nature 486, 207–214. doi: 10.1038/nature11234
Huys, G., Coopman, V., Van Varenbergh, D., and Cordonnier, J. (2012). Selective culturing and genus-specific PCR detection for identification of Aeromonas in tissue samples to assist the medico-legal diagnosis of death by drowning. Forensic Sci. Int. 221, 11–15. doi: 10.1016/j.forsciint.2012.03.017
Hyde, E. R., Haarmann, D. P., Lynne, A. M., Bucheli, S. R., and Petrosino, J. F. (2013). The living dead: bacterial community structure of a cadaver at the onset and end of the bloat stage of decomposition. PLoS One 8:e77733. doi: 10.1371/journal.pone.0077733
Hyde, E. R., Haarmann, D. P., Petrosino, J. F., Lynne, A. M., and Bucheli, S. R. (2015). Initial insights into bacterial succession during human decomposition. Int. J. Legal Med. 129, 661–671. doi: 10.1007/s00414-014-1128-4
Iancu, L., Dean, D. E., and Purcarea, C. (2018). Temperature influence on prevailing Necrophagous diptera and bacterial taxa with forensic implications for postmortem interval estimation: a review. J. Med. Entomol. 55, 1369–1379. doi: 10.1093/jme/tjy136
Janda, J. M., and Abbott, S. L. (2007). 16S rRNA gene sequencing for bacterial identification in the diagnostic laboratory: pluses, perils, and pitfalls. J Clin. Microbiol. 45, 2761–2764. doi: 10.1128/JCM.01228-07
Javan, G. T., Finley, S. J., Abidin, Z., and Mulle, J. G. (2016a). The thanatomicrobiome: a missing piece of the microbial puzzle of death. Front. Microbiol. 7:225. doi: 10.3389/fmicb.2016.00225
Javan, G. T., Finley, S. J., Can, I., Wilkinson, J. E., Hanson, J. D., and Tarone, A. M. (2016b). Human thanatomicrobiome succession and time since death. Sci. Rep. 6:29598. doi: 10.1038/srep29598
Javan, G. T., Finley, S. J., Smith, T., Miller, J., and Wilkinson, J. E. (2017). Cadaver thanatomicrobiome signatures: the ubiquitous nature of clostridium species in human decomposition. Front. Microbiol. 8:2096. doi: 10.3389/fmicb.2017.02096
Javan, G. T., Finley, S. J., Tuomisto, S., Hall, A., Benbow, M. E., and Mills, D. (2019). An interdisciplinary review of the thanatomicrobiome in human decomposition. Forensic Sci. Med. Pathol. 15, 75–83. doi: 10.1007/s12024-018-0061-0
Jha, A. R., Davenport, E. R., Gautam, Y., Bhandari, D., Tandukar, S., Ng, K. M., et al. (2018). Gut microbiome transition across a lifestyle gradient in Himalaya. PLoS Biol. 16:e2005396. doi: 10.1371/journal.pbio.2005396
Johnson, H. R., Trinidad, D. D., Guzman, S., Khan, Z., Parziale, J. V., DeBruyn, J. M., et al. (2016). A machine learning approach for using the postmortem skin microbiome to estimate the postmortem interval. PLoS One 11:e0167370. doi: 10.1371/journal.pone.0167370
Jordan, H. R., and Tomberlin, J. K. (2017). Abiotic and biotic factors regulating inter-kingdom engagement between insects and microbe activity on vertebrate remains. Insects 8:54.
Jovel, J., Patterson, J., Wang, W., Hotte, N., O’Keefe, S., Mitchel, T., et al. (2016). Characterization of the Gut microbiome using 16S or shotgun metagenomics. Front. Microbiol. 7:459. doi: 10.3389/fmicb.2016.00459
Kakizaki, E., Ogura, Y., Kozawa, S., Nishida, S., Uchiyama, T., Hayashi, T., et al. (2012). Detection of diverse aquatic microbes in blood and organs of drowning victims: first metagenomic approach using high-throughput 454-pyrosequencing. Forensic Sci. Int. 220, 135–146. doi: 10.1016/j.forsciint.2012.02.010
Kashyap, V. K., and Pillai, V. V. (1989). Efficacy of entomological method in estimation of post mortem interval: a comparative analysis. Forensic Sci. Int. 40, 245–250. doi: 10.1016/0379-0738(89)90182-5
Kaszubinski, S. F., Pechal, J. L., Schmidt, C. J., Jordan, H. R., Benbow, M. E., and Meek, M. H. (2020a). Evaluating bioinformatic pipeline performance for forensic microbiome analysis. J. Forensic Sci. 65, 513–525. doi: 10.1111/1556-4029.14213
Kaszubinski, S. F., Pechal, J. L., Smiles, K., Schmidt, C. J., Jordan, H. R., Meek, M. H., et al. (2020b). Dysbiosis in the dead: human postmortem microbiome beta-dispersion as an indicator of manner and cause of death. Front. Microbiol. 11:555347. doi: 10.3389/fmicb.2020.555347
Keeney, K. M., Yurist-Doutsch, S., Arrieta, M. C., and Finlay, B. B. (2014). Effects of antibiotics on human microbiota and subsequent disease. Annu. Rev. Microbiol. 68, 217–235. doi: 10.1146/annurev-micro091313-103456
Klappenbach, J. A., Dunbar, J. M., and Schmidt, T. M. (2000). rRNA operon copy number reflects ecological strategies of bacteria. Appl. Environ. Microbiol. 66, 1328–1333. doi: 10.1128/aem.66.4.1328-1333.2000
Klong-Klaew, T., Ngoen-Klan, R., Moophayak, K., Sukontason, K., Irvine, K. N., Tomberlin, J. K., et al. (2018). Predicting geographic distribution of forensically significant blow flies of subfamily chrysomyinae (Diptera: Calliphoridae) in Northern Thailand. Insects 9:106. doi: 10.3390/insects9030106
Kodama, W. A., Xu, Z., Metcalf, J. L., Song, S. J., Harrison, N., Knight, R., et al. (2019). Trace evidence potential in postmortem skin microbiomes: from death scene to morgue. J. Forensic Sci. 64, 791–798. doi: 10.1111/1556-4029.13949
Kunin, V., Engelbrektson, A., Ochman, H., and Hugenholtz, P. (2010). Wrinkles in the rare biosphere: pyrosequencing errors can lead to artificial inflation of diversity estimates. Environ. Microbiol. 12, 118–123. doi: 10.1111/j.1462-2920.2009.02051.x
Lamendella, R., Domingo, J. W., Ghosh, S., Martinson, J., and Oerther, D. B. (2011). Comparative fecal metagenomics unveils unique functional capacity of the swine gut. BMC Microbiol. 11:103. doi: 10.1186/1471-2180-11-103
Lang, J. M., Erb, R., Pechal, J. L., Wallace, J. R., McEwan, R. W., and Benbow, M. E. (2016). Microbial biofilm community variation in flowing habitats: potential utility as bioindicators of postmortem submersion intervals. Microorganisms 4:1. doi: 10.3390/microorganisms4010001
Langille, M. G., Zaneveld, J., Caporaso, J. G., McDonald, D., Knights, D., Reyes, J. A., et al. (2013). Predictive functional profiling of microbial communities using 16S rRNA marker gene sequences. Nat. Biotechnol. 31, 814–821. doi: 10.1038/nbt.2676
Lawrence, K. E., Lam, K. C., Morgun, A., Shulzhenko, N., and Löhr, C. V. (2019). Effect of temperature and time on the thanatomicrobiome of the cecum, ileum, kidney, and lung of domestic rabbits. J. Vet. Diagn. Invest. 31, 155–163. doi: 10.1177/1040638719828412
Lax, S., Hampton-Marcell, J. T., Gibbons, S. M., Colares, G. B., Smith, D., Eisen, J. A., et al. (2015). Forensic analysis of the microbiome of phones and shoes. Microbiome 3:21. doi: 10.1186/s40168-015-0082-9
Lederberg, J., and McCray, A. T. (2001). Ome sweet ‘omics– a genealogical treasury of words. Scientist 15:8.
Lee, S. Y., Woo, S. K., Lee, S. M., Ha, E. J., Lim, K. H., Choi, K. H., et al. (2017). Microbiota composition and pulmonary surfactant protein expression as markers of death by drowning. J. Forensic Sci. 62, 1080–1088. doi: 10.1111/1556-4029.13347
Leipzig, J. (2016). A review of bioinformatic pipeline frameworks. Brief. Bioinform. 18, 530–536. doi: 10.1093/bib/bbw020
Li, H., Liu, R. N., Zhang, S. R., Yuan, L., and Xu, J. R. (2018). Succession law of intestinal flora after death in SD rats. Fa Yi Xue Za Zhi 34, 482–486. doi: 10.12116/j.issn.1004-5619.2018.05.005
Liszt, K., Zwielehner, J., Handschur, M., Hippe, B., Thaler, R., and Haslberger, A. G. (2009). Characterization of bacteria, clostridia and Bacteroides in faeces of vegetarians using qPCR and PCR-DGGE fingerprinting. Ann. Nutr. Metab. 54, 253–257. doi: 10.1159/000229505
Liu, L., Li, Y., Li, S., Hu, N., He, Y., Pong, R., et al. (2012). Comparison of next-generation sequencing systems. J. Biomed. Biotechnol. 2012:251364. doi: 10.1155/2012/251364
Lucci, A., Campobasso, C. P., Cirnelli, A., and Lorenzini, G. (2008). A promising microbiological test for the diagnosis of drowning. Forensic Sci. Int. 182, 20–26. doi: 10.1016/j.forsciint.2008.09.004
Lunetta, P., Penttilä, A., and Hällfors, G. (1998). Scanning and transmission electron microscopical evidence of the capacity of diatoms to penetrate the alveolo-capillary barrier in drowning. Int. J. Legal Med. 111, 229–237. doi: 10.1007/s004140050159
Lutz, H., Vangelatos, A., Gottel, N., Osculati, A., Visona, S., Finley, S. J., et al. (2020). Effects of extended postmortem interval on microbial communities in organs of the human cadaver. Front. Microbiol. 11:569630. doi: 10.3389/fmicb.2020.569630
Madea, B. (2016). Methods for determining time of death. Forensic Sci. Med. Pathol. 12, 451–485. doi: 10.1007/s12024-016-9776-y
Madea, B., Ortmann, J., and Doberentz, E. (2019). Estimation of the time since death-Even methods with a low precision may be helpful in forensic casework. Forensic Sci. Int. 302:109879. doi: 10.1016/j.forsciint.2019.109879
Malla, M. A., Dubey, A., Kumar, A., Yadav, S., Hashem, A., and Abd Allah, E. F. (2019). Exploring the human microbiome: the potential future role of next-generation sequencing in disease diagnosis and treatment. Front. Immunol. 9:2868. doi: 10.3389/fimmu.2018.02868
Matsuki, T., Watanabe, K., Fujimoto, J., Miyamoto, Y., Takada, T., Matsumoto, K., et al. (2002). Development of 16S rRNA-genetargeted group-specific primers for the detection and identification of predominant bacteria in human feces. Appl. Environ. Microbiol. 68, 5445–5451. doi: 10.1128/aem.68.11.5445-5451.2002
Meadow, J. F., Altrichter, A. E., and Green, J. L. (2014). Mobile phones carry the personal microbiome of their owners. PeerJ. 2:e447. doi: 10.7717/peerj.447
Megyesi, M. S., Nawrocki, S. P., and Haskell, N. H. (2005). Using accumulated degree-days to estimate the postmortem interval from decomposed human remains. J. Forensic Sci. 50, 618–626.
Metcalf, J. L. (2019). Estimating the postmortem interval using microbes: knowledge gaps and a path to technology adoption. Forensic Sci. Int. Genet. 38, 211–218. doi: 10.1016/j.fsigen.2018.11.004
Metcalf, J. L., Wegener Parfrey, L., Gonzalez, A., Lauber, C. L., Knights, D., Ackermann, G., et al. (2013). A microbial clock provides an accurate estimate of the postmortem interval in a mouse model system. eLife 2:e01104. doi: 10.7554/eLife.01104
Metcalf, J. L., Xu, Z. Z., Weiss, S., Lax, S., Van Treuren, W., Hyde, E. R., et al. (2016). Microbial community assembly and metabolic function during mammalian corpse decomposition. Science 351, 158–162. doi: 10.1126/science.aad2646
Meurs, J., Krap, T., and Duijst, W. (2019). Evaluation of postmortem biochemical markers: completeness of data and assessment of implication in the field. Sci. Justice 59, 177–180. doi: 10.1016/j.scijus.2018.09.002
Mignard, S., and Flandrois, J. P. (2006). 16S RRNA sequencing in routine bacterial identification: a 30-month experiment. J. Microbiol. Methods 67, 574–581. doi: 10.1016/j.mimet.2006.05.009
Mitchelson, K. R. (2003). The use of capillary electrophoresis for DNA polymorphism analysis. Mol. Biotechnol. 24, 41–68. doi: 10.1385/MB:24:1:41
Na, J. Y., Park, J. H., Kim, S. H., and Park, J. T. (2017). Bacteria as normal flora in postmortem body fluid samples. Korean J. Leg. Med. 41:87. doi: 10.7580/kjlm.2017.41.4.87
Nam, Y. D., Jung, M. J., Roh, S. W., Kim, M. S., and Bae, J. W. (2011). Comparative analysis of Korean human gut microbiota by barcoded pyrosequencing. PLoS One 6:e22109. doi: 10.1371/journal.pone.0022109
Nguyen, N. P., Warnow, T., Pop, M., and White, B. (2016). A perspective on 16S rRNA operational taxonomic unit clustering using sequence similarity. NPJ Biofilms Microbiomes 2:16004. doi: 10.1038/npjbiofilms.2016.4
NIH HMP Working Group, Peterson, J., Garges, S., Giovanni, M., McInnes, P., Wang, L., et al. (2009). The NIH human microbiome project. Genome Res. 19, 2317–2323. doi: 10.1101/gr.096651.109
Nishijima, S., Suda, W., Oshima, K., Kim, S. W., Hirose, Y., Morita, H., et al. (2016). The gut microbiome of healthy Japanese and its microbial and functional uniqueness. DNA Res. 23, 125–133. doi: 10.1093/dnares/dsw002
Nogueira, T., David, P. H. C., and Pothier, J. (2019). Antibiotics as both friends and foes of the human gut microbiome: the microbial community approach. Drug Dev. Res. 80, 86–97. doi: 10.1002/ddr.21466
Norén, T. (2010). Clostridium difficile and the disease it causes. Methods Mol. Biol. 646, 9–35. doi: 10.1007/978-1-60327-365-7_2
Oliveira, M., and Amorim, A. (2018). Microbial forensics: new breakthroughs and future prospects. Appl. Microbiol. Biotechnol. 102, 10377–10391. doi: 10.1007/s00253-018-9414-6
Parson, W., and Bandelt, H. J. (2007). Extended guidelines for mtDNA typing of population data in forensic science. Forensic Sci. Int. Genet. 1, 13–19. doi: 10.1016/j.fsigen.2006.11.003
Pechal, J. L., Crippen, T. L., Benbow, M. E., Tarone, A. M., Dowd, S., and Tomberlin, J. K. (2014). The potential use of bacterial community succession in forensics as described by high throughput metagenomic sequencing. Int. J. Legal Med. 128, 193–205. doi: 10.1007/s00414-013-0872-1
Pechal, J. L., Schmidt, C. J., Jordan, H. R., and Benbow, M. E. (2018). A large-scale survey of the postmortem human microbiome, and its potential to provide insight into the living health condition. Sci. Rep. 8:5724. doi: 10.1038/s41598-018-23989-w
Petrosino, J. F., Highlander, S., Luna, R. A., Gibbs, R. A., and Versalovic, J. (2009). Metagenomic pyrosequencing and microbial identification. Clin. Chem. 55, 856–866. doi: 10.1373/clinchem.2008.107565
Phillips, C., Salas, A., Sánchez, J. J., Fondevila, M., Gómez-Tato, A., Alvarez-Dios, J., et al. (2007). Inferring ancestral origin using a single multiplex assay of ancestry-informative marker SNPs. Forensic Sci. Int. Genet. 1, 273–280. doi: 10.1016/j.fsigen.2007.06.008
Pittner, S., Bugelli, V., Weitgasser, K., Zissler, A., Sanit, S., Lutz, L., et al. (2020). A field study to evaluate PMI estimation methods for advanced decomposition stages. Int. J. Legal Med. 134, 1361–1373. doi: 10.1007/s00414-020-02278-0
Qu, K., Guo, F., Liu, X., Lin, Y., and Zou, Q. (2019). Application of machine learning in microbiology. Front. Microbiol. 10:827. doi: 10.3389/fmicb.2019.00827
Quince, C., Walker, A. W., Simpson, J. T., Loman, N. J., and Segata, N. (2017). Shotgun metagenomics, from sampling to analysis. Nat. Biotechnol. 35, 833–844. doi: 10.1038/nbt.3935
Rácz, E., Könczöl, F., Tóth, D., Patonai, Z., Porpáczy, Z., Kozma, Z., et al. (2016). PCR-based identification of drowning: four case reports. Int. J. Legal Med. 130, 1303–1307. doi: 10.1007/s00414-016-1359-7
Rehman, A., Rausch, P., Wang, J., Skieceviciene, J., Kiudelis, G., Bhagalia, K., et al. (2016). Geographical patterns of the standing and active human gut microbiome in health and IBD. Gut 65, 238–248. doi: 10.1136/gutjnl-2014-308341
Rognum, T. O., Holmen, S., Musse, M. A., Dahlberg, P. S., Stray-Pedersen, A., Saugstad, O. D., et al. (2016). Estimation of time since death by vitreous humor hypoxanthine, potassium, and ambient temperature. Forensic Sci. Int. 262, 160–165. doi: 10.1016/j.forsciint.2016.03.001
Rothberg, J. M., and Leamon, J. H. (2008). The development and impact of 454 sequencing. Nat. Biotechnol. 26, 1117–1124. doi: 10.1038/nbt1485
Rutty, G. N., Bradley, C. J., Biggs, M. J., Hollingbury, F. E., Hamilton, S. J., Malcomson, R. D., et al. (2015). Detection of bacterioplankton using PCR probes as a diagnostic indicator for drowning; the Leicester experience. Leg. Med. 17, 401–408. doi: 10.1016/j.legalmed.2015.06.001
Salam, H. F. A., Shaat, E. A., Aziz, M. H. A., MoneimSheta, A. A., and Hussein, H. A. S. M. (2012). Estimation of postmortem interval using thanatochemistry and postmortem changes. Alex. J. Med. 48, 335–344. doi: 10.1016/j.ajme.2012.05.004
Salipante, S. J., Kawashima, T., Rosenthal, C., Hoogestraat, D. R., Cummings, L. A., Sengupta, D. J., et al. (2014). Performance comparison of Illumina and ion torrent next-generation sequencing platforms for 16S rRNA-based bacterial community profiling. Appl. Environ. Microbiol. 80, 7583–7591. doi: 10.1128/AEM.02206-14
Schloissnig, S., Arumugam, M., Sunagawa, S., Mitreva, M., Tap, J., Zhu, A., et al. (2013). Genomic variation landscape of the human gut microbiome. Nature 493, 45–50. doi: 10.1038/nature11711
Schmedes, S. E., Sajantila, A., and Budowle, B. (2016). Expansion of microbial forensics. J. Clin. Microbiol. 54, 1964–1974. doi: 10.1128/JCM.00046-16
Schnorr, S. L., Candela, M., Rampelli, S., Centanni, M., Consolandi, C., Basaglia, G., et al. (2014). Gut microbiome of the Hadza hunter-gatherers. Nat Commun. 5:3654. doi: 10.1038/ncomms4654
Schoenly, K. G., Haskell, N. H., Mills, D. K., Bieme-Ndi, C., Larsen, K., and Lee, Y. (2006). recreating death’s acre in the school yard: using pig carcasses as model corpses, to teach concepts of forensic entomology & ecological succession. Am. Biol. Teach. 68, 402–410. doi: 10.2307/4452028
Schouls, L. M., Schot, C. S., and Jacobs, J. A. (2003). Horizontal transfer of segments of the 16S rRNA genes between species of the Streptococcus anginosus group. J. Bacteriol. 185, 7241–7246. doi: 10.1128/jb.185.24.7241-7246.2003
Scott, L., Finley, S. J., Watson, C., and Javan, G. T. (2020). Life and death: a systematic comparison of antemortem and postmortem gene expression. Gene 731:144349. doi: 10.1016/j.gene.2020.144349
Senghor, B., Sokhna, C., Ruimy, R., and Lagier, J. C. (2018). Gut microbiota diversity according to dietary habits and geographical provenance. Hum. Microbiome J. 7, 1–9. doi: 10.1016/j.humic.2018.01.001
Sharma, R., Garg, R. K., and Gaur, J. R. (2015). Various methods for the estimation of the post mortem interval for Calliphoridae: a review. Egyp. J. Forensic Sci. 5, 1–12. doi: 10.1016/j.ejfs.2013.04.002
Shin, N. R., Whon, T. W., and Bae, J. W. (2015). Proteobacteria: microbial signature of dysbiosis in gut microbiota. Trends Biotechnol. 33, 496–503. doi: 10.1016/j.tibtech.2015.06.011
Sitthiwong, N., Ruangyuttikarn, W., Vongvivach, S., and Peerapornpisal, Y. (2014). Detection and identification of diatoms in tissue samples of drowning victims. Chiang Mai J. Sci. 41, 1020–1031.
Soergel, D. A., Dey, N., Knight, R., and Brenner, S. E. (2012). Selection of primers for optimal taxonomic classification of environmental 16S rRNA gene sequences. ISME J. 6, 1440–1444. doi: 10.1038/ismej.2011.208
Spicka, A., Johnson, R., Bushing, J., Higley, L. G., and Carter, D. O. (2011). Carcass mass can influence rate of decomposition and release of ninhydrin-reactive nitrogen into gravesoil. Forensic Sci. Int. 209, 80–85. doi: 10.1016/j.forsciint.2011.01.002
Stokes, K. L., Forbes, S. L., and Tibbett, M. (2013). Human versus animal: contrasting decomposition dynamics of mammalian analogues in experimental taphonomy. J. Forensic Sci. 58, 583–591. doi: 10.1111/1556-4029.12115
Sullivan, K. M., Hopgood, R., and Gill, P. (1992). Identification of human remains by amplification and automated sequencing of mitochondrial DNA. Int. J. Legal Med. 105, 83–86. doi: 10.1007/BF02340829
Suzuki, M. T., and Giovannoni, S. J. (1996). Bias caused by template annealing in the amplification of mixtures of 16S RRNA genes by PCR. Appl. Environ. Microbiol. 62, 625–630. doi: 10.1128/AEM.62.2.625-630.1996
Thomas, T. B., Finley, S. J., Wilkinson, J. E., Wescott, D. J., Gorski, A., and Javan, G. T. (2017). Postmortem microbial communities in burial soil layers of skeletonized humans. J. Forensic Leg. Med. 49, 43–49. doi: 10.1016/j.jflm.2017.05.009
Tie, J., Uchigasaki, S., Haseba, T., Ohno, Y., Isahai, I., and Oshida, S. (2010). Direct and rapid PCR amplification using digested tissues for the diagnosis of drowning. Electrophoresis 31, 2411–2415. doi: 10.1002/elps.200900754
Tomberlin, J. K., Mohr, R., Benbow, M. E., Tarone, A. M., and VanLaerhoven, S. (2011). A roadmap for bridging basic and applied research in forensic entomology. Annu. Rev. Entomol. 56, 401–421. doi: 10.1146/annurev-ento-051710-103143
Toscano, M., de Grandi, R., and Drago, L. (2018). Proteomics: the new era of microbiology. Microbiol. Med. 32, 183–184. doi: 10.4081/mm.2017.7348
Trivedi, U. H., Cézard, T., Bridgett, S., Montazam, A., Nichols, J., Blaxter, M., et al. (2014). Quality control of next-generation sequencing data without a reference. Front. Genet. 5:111. doi: 10.3389/fgene.2014.00111
Tu, Q., He, Z., and Zhou, J. (2014). Strain/species identification in metagenomes using genome-specific markers. Nucleic Acids Res. 42:e67. doi: 10.1093/nar/gku138
Tu, Q., Li, J., Shi, Z., Chen, Y., Lin, L., Li, J., et al. (2017). HuMiChip2 for strain level identification and functional profiling of human microbiomes. Appl. Microbiol. Biotechnol. 101, 423–435. doi: 10.1007/s00253-016-7910-0
Tuomisto, S., Karhunen, P. J., Vuento, R., Aittoniemi, J., and Pessi, T. (2013). Evaluation of postmortem bacterial migration using culturing and real-time quantitative PCR. J. Forensic Sci. 58, 910–916. doi: 10.1111/1556-4029.12124
Uchiyama, T., Kakizaki, E., Kozawa, S., Nishida, S., Imamura, N., and Yukawa, N. (2012). A new molecular approach to help conclude drowning as a cause of death: simultaneous detection of eight bacterioplankton species using real-time PCR assays with TaqMan probes. Forensic Sci. Int. 222, 11–26. doi: 10.1016/j.forsciint.2012.04.029
Ventura Spagnolo, E., Stassi, C., Mondello, C., Zerbo, S., Milone, L., and Argo, A. (2019). Forensic microbiology applications: a systematic review. Leg. Med. 36, 73–80. doi: 10.1016/j.legalmed.2018.11.002
Vila, A. V., Collij, V., Sanna, S., Sinha, T., Imhann, F., Bourgonje, A. R., et al. (2020). Impact of commonly used drugs on the composition and metabolic function of the gut microbiota. Nat. Commun. 11:362. doi: 10.1038/s41467-019-14177-z
Walsh, S., Chaitanya, L., Clarisse, L., Wirken, L., Draus-Barini, J., Kovatsi, L., et al. (2014). Developmental validation of the HIrisPlex system: DNA-based eye and hair colour prediction for forensic and anthropological usage. Forensic Sci. Int. Genet. 9, 150–161. doi: 10.1016/j.fsigen.2013.12.006
Wang, Y., Zhang, Z., and Ramanan, N. (1997). The actinomycete Thermobispora bispora contains two distinct types of transcriptionally active 16S rRNA genes. J. Bacteriol. 179, 3270–3276. doi: 10.1128/jb.179.10.3270-3276.1997
Weiss, S., Carter, D. O., Metcalf, J. L., and Knight, R. (2016). Carcass mass has little influence on the structure of gravesoil microbial communities. Int. J. Leg. Med. 130, 253–263. doi: 10.1007/s00414-015-1206-2
Westreich, S. T., Korf, I., Mills, D. A., and Lemay, D. G. (2016). SAMSA: a comprehensive metatranscriptome analysis pipeline. BMC Bioinformatics 17:399. doi: 10.1186/s12859-016-1270-8
Woydt, L., Bernhard, M., Kirsten, H., Burkhardt, R., Hammer, N., Gries, A., et al. (2018). Intra-individual alterations of serum markers routinely used in forensic pathology depending on increasing post-mortem interval. Sci. Rep. 8:12811. doi: 10.1038/s41598-018-31252-5
Wu, G. D., Chen, J., Hoffmann, C., Bittinger, K., Chen, Y.-Y., Keilbaugh, S. A., et al. (2011). Linking long-term dietary patterns with gut microbial enterotypes. Science 334, 105–108. doi: 10.1126/science.1208344
Yuan, S., Cohen, D. B., Ravel, J., Abdo, Z., and Forney, L. J. (2012). Evaluation of methods for the extraction and purification of DNA from the human microbiome. PLoS One 7:e33865. doi: 10.1371/journal.pone.0033865
Zhang, Y., Pechal, J. L., Schmidt, C. J., Jordan, H. R., Wang, W. W., Benbow, M. E., et al. (2019). Machine learning performance in a microbial molecular autopsy context: a cross-sectional postmortem human population study. PLoS One 14:e0213829. doi: 10.1371/journal.pone.0213829
Zhou, W., and Bian, Y. (2018). Thanatomicrobiome composition profiling as a tool for forensic investigation. Forensic Sci. Res. 3, 105–110. doi: 10.1080/20961790.2018.1466430
Zhou, X., Bent, S. J., Schneider, M. G., Davis, C. C., Islam, M. R., and Forney, L. J. (2004). Characterization of vaginal microbial communities in adult healthy women using cultivation-independent methods. Microbiology 150, 2565–2573. doi: 10.1099/mic.0.26905-0
Keywords: postmortem interval (PMI), next-generation sequencing, thanatomicrobiome, thanatogenomics, thanatoproteomics, postmortem submersion interval, microbial clock, gut microbiome
Citation: Roy D, Tomo S, Purohit P and Setia P (2021) Microbiome in Death and Beyond: Current Vistas and Future Trends. Front. Ecol. Evol. 9:630397. doi: 10.3389/fevo.2021.630397
Received: 17 November 2020; Accepted: 29 January 2021;
Published: 04 March 2021.
Edited by:
Gulnaz T. Javan, Alabama State University, United StatesReviewed by:
Matteo Moretti, University of Pavia, ItalyCopyright © 2021 Roy, Tomo, Purohit and Setia. This is an open-access article distributed under the terms of the Creative Commons Attribution License (CC BY). The use, distribution or reproduction in other forums is permitted, provided the original author(s) and the copyright owner(s) are credited and that the original publication in this journal is cited, in accordance with accepted academic practice. No use, distribution or reproduction is permitted which does not comply with these terms.
*Correspondence: Purvi Purohit, ZHIucHVydmlwdXJvaGl0QGdtYWlsLmNvbQ==; Puneet Setia, cHVuZWV0c2V0aWFAZ21haWwuY29t
Disclaimer: All claims expressed in this article are solely those of the authors and do not necessarily represent those of their affiliated organizations, or those of the publisher, the editors and the reviewers. Any product that may be evaluated in this article or claim that may be made by its manufacturer is not guaranteed or endorsed by the publisher.
Research integrity at Frontiers
Learn more about the work of our research integrity team to safeguard the quality of each article we publish.