- 1CAS Key Laboratory of Environmental Biotechnology, Research Center for Eco-Environmental Sciences, Chinese Academy of Sciences, Beijing, China
- 2State Key Laboratory of Biogeology and Environmental Geology, China University of Geosciences, Beijing, China
- 3School of Ocean Sciences, China University of Geosciences, Beijing, China
- 4State Key Laboratory of Biogeology and Environmental Geology, China University of Geosciences, Wuhan, China
- 5School of Environment and Resource, Southwest University of Science and Technology, Sichuan, China
- 6Department of Hydraulic Engineering, Tsinghua University, Beijing, China
Dams represent the most significant anthropogenic disturbance to global rivers. Previous studies have shown that free-living and particle-attached microbes exhibited differentially in river and reservoir ecosystems. However, little is known about the dam’s effect on their co-occurrence patterns. Here, a random matrix theory (RMT)-based network approach was used to construct microbial ecological networks for free-living and particle-attached communities in the immediate vicinity of the Three Gorges Dam (TGD), based on a high-throughput sequencing of 16S rRNA gene. Microbial distribution pattern showed that differences caused by lifestyle (free-living vs. particle-attached) were greater than those caused by geographic position (upstream vs. downstream of the TGD). Network analysis revealed higher connectivity and a lower number of modules in the overall downstream networks. Furthermore, considering the lifestyle, the network structures and properties for free-living and particle-attached microbes were different between upstream and downstream of the dam. Specifically, free-living communities located upstream of the dam exhibited a more complex co-occurrence pattern than the particle-attached communities, whereas the opposite was true for those located downstream of the dam. This variation indicated a strong impact of the dam on microbial interactions for microbes with similar lifestyle in the vicinity of the dam. We identified 112 persistent operational taxonomic unit (OTU)-level species that stably coexisted regardless of lifestyle and geographic positions. These persistent species occupied 21.33–25.57% of the total nodes in each network, and together with their first neighbors, they contributed more than 50% of the nodes and edges belonging to each network. Furthermore, we found that taxonomic affiliations for central nodes (with high degree) varied in these persistent species sub-networks. Collectively, our findings expand the current understanding of the dam’s effect on species interaction variation patterns for free-living and particle-attached communities in the vicinity of the dam, which are more complex than traditional alpha and beta microbial diversity.
Introduction
Dam construction has permanent impacts from species to ecosystem levels and from local to global scales (Grill et al., 2015). River fragmentation, diminishing the longitudinal connectivity, is one of the greatest problems induced by dam. This in turn particularly influences species migration and dispersal, as well as organic and inorganic matter transport downstream. Vast efforts have been made to elucidate the dam effects on biogeochemical cycling (Maavara et al., 2020) and various biodiversity (Wu et al., 2004) such as fish (Liermann et al., 2012), bathetic diatom (Liu et al., 2013), microinvertebrate (Mihalicz et al., 2019), and microbial plankton (Yan et al., 2015; Huang et al., 2016; Liu et al., 2018). Specifically, dam significantly shapes areas upstream of the dam to lacustrine state from a free-flowing phase, trapping sediments, nutrients, and even biodiversity (Vorosmarty et al., 2003; Maavara et al., 2014, 2015). One of our recent studies showed that microbial diversity accumulates in a downstream direction in the reservoir with various contributions from tributary, mainstem, and riverbank soils (Wang S. et al., 2021). Similarly, downstream of the dam also experiences severe environmental changes due to the alteration of a river’s flow and sediment transport (Maren et al., 2013). It has shown that microbial diversity reduced suddenly after the dam (Liu et al., 2018). Collectively, damming has great impacts on river ecology in a microbiological view. Some studies have investigated the temporal patterns in microbial interactions for free-living and particle-attached plankton or specific interactions between cyanobacteria and other heterotrophic bacteria or eukaryotes in river and reservoir ecosystems (Li et al., 2015; Zhao et al., 2016; Liu L. et al., 2019), but there has been little revealed as to how microbial interactions change upstream and downstream of the dam.
It is acknowledged that river structure could leave an imprint on microbial ecological network (Widder et al., 2014), and microbial ecological network has close relationships with temporal and spatial environmental fluctuations (Li et al., 2019; Wang L. et al., 2021). Since free-living and particle-attached planktons have been reported with different compositions and activities in diverse aquatic environments (Rosel and Grossart, 2012; Li et al., 2018), they preferred differential microbial interactions (Xu et al., 2018; Wang et al., 2020), but little is known on how damming could influence these interactions. Even blocked by damming, passive dispersal of microbial species transports some species from upstream to downstream of the dam with water going through the dam. Furthermore, previous studies have found the lifestyle transition between free-living and particle-attached planktons in other aquatic ecosystems, such as reservoir (Liu M. et al., 2019) and marine environments (Teeling et al., 2012), under control of substrate availability, motility, and chemotactic behavior (Grossart, 2010). Therefore, it is reasonable to come up with a hypothesis that the co-occurring microbial species could have distinct microbial interaction patterns between upstream and downstream of the dam; lifestyle transition may contribute to this distinctness due to hydrodynamics fluctuation, accompanied with nutrient flux variation, from water releasing to water impoundment.
To investigate the potential dam impacts on microbial interactions for free-living and particle-attached planktons, we employed a random matrix theory (RMT)-based approach to build microbial ecological networks based on the high-throughput sequencing data of 16S rRNA gene from sites immediately upstream and downstream of the Three Gorges Dam (TGD). The objectives are as follows: (i) to reveal the species interaction patterns based on lifestyle (free-living vs. particle-attached) and geographic position (upstream vs. downstream of the dam) and (ii) to investigate how dam-induced changes in microbial networks influence community stability upstream and downstream of the dam.
Materials and Methods
Field Measurements and Sampling
During October 2011, a field campaign was conducted in the vicinity of the TGD (Supplementary Figure 1A). A total of 14 sites were chosen to collect water samples, seven of which were distributed upstream of the TGD and the others downstream, with the furthest site extending about 20 km up and 10 km down of the TGD, respectively. During our sampling period, the Three Gorges Reservoir started water impoundment, so water level went up to 167–170 m before the dam (Tang et al., 2018).
The geographic information including latitude, longitude, and elevation for each sampling site was recorded by GPS (eTrex Venture, Garmin, United States). For each site, two filter pore sizes (0.22 and 0.7 μm) were used. A pore size of 0.7 μm was used to separate the large particles (particle-attached) from free-living (0.22 μm) microorganisms. This is not the typical choice of 3 μm for investigating particle-attached microbes, but a previous study has shown that relatively higher similarities in GC content, effective genome size, general taxonomic composition, and functional gene categories were observed between 0.8 and 3 μm pore size cut-offs (Smith et al., 2013). Furthermore, we did find distinct community compositions between 0.22 and 0.7 μm pore sizes regarding to either overall microbial communities (Wang et al., 2012) or functional groups such as ammonia-oxidizing archaea and bacteria (Huang et al., 2016). Water samples for geochemistry measurements and microbial analysis were collected following previous procedures (Wang S. et al., 2021). In situ measurements, including depth, temperature, conductivity (COND), pH, total dissolved solids (TDS), dissolved oxygen (DO), turbidity (TURB), and oxidation-reduction potential (ORP), were conducted by using a multiple-probe set Horiba (U20D, Japan). Concentrations of ammonium (NH4–N), nitrate (NO3–N), nitrite (NO2–N), silica (SiO2), and phosphate (PO4–P, DIP) were measured by spectrophotometric Hach kits (Hach Chemical Co., IA, United States) in the field.
Water Geochemistry Measurements in Laboratory
Additional filtrate through a 0.45-μm pre-combusted (500°C for 4 h) Whatman filter was collected for laboratory measurements, including cations, anions, dissolved inorganic carbon (DIC), total dissolved nitrogen (TDN), and total dissolved phosphate (TDP). Cations and anions were measured by direct current plasma optical emission spectrometry (DCP-OES, Beckman, United States) and Dionex ion chromatography (Dionex, United States), respectively. TDN was determined by using high-temperature combustion on a Shimadzu TOC analyzer (TOC-V) interfaced with a nitrogen detector, and TDP was analyzed by persulfate oxidation (Sharp et al., 2002). Subtracting the sum of NH4–N and NOx–N from TDN was used to estimate the abundance of dissolved organic nitrogen (DON). Dissolved organic phosphorus (DOP) was calculated as the differences between TDP and DIP. Water samples for cations were acidified and stored at 4°C. Various volumes of water were filtered due to changes in turbidity.
DNA Extraction, PCR, and Sequencing
DNAs were extracted from collected 0.22- and 0.7-μm filters and then used for PCR with barcoded primer sets (515F, 5′-GTGCCAGCMGCCGCGGTAA-3′ and 806R, 5′-GGACTACHVGGGTWTCTAAT-3′). PCR was performed in triplicate for each sample, following the procedure of initial denaturation at 98°C for 1 min, 30 cycles of 30 s at 95°C, 30 s at 53°C, and 30 s at 72°C and a final 5-min extension at 72°C. Each 25-μl PCR reaction system contained 2.5 μl 10× PCR buffer, 2.0 μl of 2.5 mmol/l dNTP, 1.0 μl of each primer (10 mmol/l), 0.25 μl of DNA polymerase (TaKaRa Taq, Dalian, China), and 1.0 μl of genomic DNA (20–50 ng). The PCR products from triplicate were combined and purified by agarose electrophoresis. After quantification by Qubit fluorometer (Invitrogen, United States), equal quantities of barcoded PCR products from different samples were pooled and sent for sequencing library construction. Sequencing was performed on Illumina Miseq sequencing platform.
Data Processing
Paired-end reads were merged with fastq-join program (Aronesty, 2011) and then assigned to different samples based on barcode sequences using split_library.py in QIIME (Caporaso et al., 2010) with default parameters. Further quality control was carried out in Mothur (Schloss et al., 2009) using trim.seq() function with parameters as follows: maxhomop = 6, qwindowsize = 50, qwindowaverage = 25, maxambig = 0, pdiffs = 1, bdiffs = 0, and minlength = 120. The good quality sequences were sent to chimeric checking by using ChimeraSlayer (Haas et al., 2011), and then the filtered sequences were clustered at 97% sequence identity level by using UCLUST (Edgar, 2010). Taxonomy was assigned against the Greengenes database 13.8 using the ribosome database project (RDP) classifier algorithm (Cole et al., 2014). The generated operational taxonomic unit (OTU) table was resampled to 30,000 reads, and the following analyses were based on these randomized data.
The Network Construction
Microbial ecological networks were constructed for samples upstream and downstream of the TGD based on 16S rRNA gene sequencing data. Then, we further built networks for free-living and particle-attached microbes upstream (UF and UP) and downstream (DF and DP) of the TGD. An RMT-based method was employed due to its advantage to automatically identify the appropriate similarity threshold prior to network construction (Deng et al., 2012). According to Deng et al. (2012), the following steps have been involved into constructing networks based on this approach: high-throughput sequencing data collection and data transformation/standardization, pair-wise Spearman similarities between any two OTUs, and an adjacent matrix by applying a threshold to the correlation values determined by the RMT-based approach. Once the adjacency matrix is defined, an undirected network graph based on the strengths of the connections of each OTU with all of the other connected OTUs can be drawn. In our study, prior to network construction, OTUs were filtered by occurrence; only those existing in more than 50% of the total samples (that is, seven of 14 samples for overall upstream and downstream networks and four of seven samples for UF, UP, DF, and DP networks) were kept for network computation. Then, a symmetric correlation matrix is calculated based on Spearman correlation coefficient (r-value) and then converted into the similarity matrix by taking the absolute values. Thereafter, an optimal similarity threshold is determined by ramping up the similarity threshold from 0.30 to 1.00 with 0.01 intervals. This threshold was then used to filter the similarity values for adjacency matrix, and only values above the threshold kept. In this study, an identical cut-off of 0.90 was used to construct overall upstream and downstream networks and 0.96 for UF, UP, DF, and DP networks. The constructed networks were then visualized and modified in the software Gephi 0.9.1 (Bastian et al., 2009). Sub-networks for OTUs shared by UF, UP, DF, and DP networks and their first neighbors (the OTUs that are directly connected with those shared OTUs) were identified. In this study, we used the term of “persistent species” for these stably coexisting species in all networks regardless of lifestyle and geographic positions.
Positive and negative associations in the networks have specific ecological meanings. For example, positive association may represent the same taxonomic affiliation, similar preferential environmental conditions, interspecies cross-feeding, or niche overlapping, whereas negative associations indicate a competitive relationship or niche partitioning and/or a resistant ability to be grazed by predator in a food web (Deng et al., 2012). The network construction and statistical analysis were done at http://ieg4.rccc.ou.edu/mena/.
Topological Property of the Constructed Ecological Networks
Global network properties were calculated as described in Deng et al. (2012), including average connectivity (avgK), average path distance (GD), and average clustering coefficient (avgCC). Modularity was determined by using fast greedy modularity optimization (Newman, 2006). We check the scale-free property of networks by fitting network connectivity distribution with the power law model. A scale-free network means only a few nodes in the network have a large number of connections, while most of the nodes have no or few connections (Barabási, 2009). Random networks were generated by randomization procedure with 100 permutations to evaluate whether or not the constructed networks were random, with the number of nodes and links constant between random and experimental networks (Deng et al., 2012). Nodes’ topological roles were identified as previously described (Wang et al., 2018). Briefly, nodes could be classified into four categories, namely, peripheral nodes, connectors, module hubs, and network hubs.
Statistical Analyses
Clustering with Ward’s method was used to group samples by environmental parameters. Differences in microbial composition between samples were visualized using non-metric multidimensional scaling (NMDS) based on Bray–Curtis dissimilarity. NMDS and clustering were carried out via the PAST program. Permutational multivariate analysis of variance (PERMANOVA) was used to assess whether groupings apparent in NMDS plots were significantly different. The partial Mantel test was employed to figure out the significant environmental factors that drive the structure of the whole network or those sub-networks in terms of taxonomic lineages (Deng et al., 2012). The response ratio (RR) was used to evaluate the relative changes of taxonomic lineages between particle-attached and free-living networks regardless of geographic position and between upstream and downstream networks regardless of plankton lifestyle. The calculations were performed by an in-house pipeline1. The RR value greater than zero means the abundance of a certain taxonomic lineage increases in the treatment group. The result is considered significant when the 95% confidence interval (CI) does not overlap zero.
Results
Microbial Community Compositions and Environmental Conditions Upstream and Downstream of the TGD
Damming has changed hydrological conditions greatly. For instance, the average value of pH was a bit higher upstream than downstream of the dam (7.64 vs. 6.66). The fluctuation of DO was concomitant with ORP, with declining trends observed from upstream to downstream of the dam and the highest value peaking immediately downstream of the dam (site DWON-1). Turbidity was gradually decreasing when approaching to the dam (22.6 to 9.5 NTU) in the reservoir, but it sharply increased when water is going through the dam (28 NTU) and then decreased dramatically further away from the dam (13.2 NTU). Nutrients such as silicates, TN, and TP slightly changed between upstream and downstream of the dam. A clustering tree showed that environmental conditions for waters just going through the dam were more similar with those from upstream (Supplementary Figure 1B), and we noticed that downstream tributary DOWN-5 was distinctly different from other sampling sites. For microbial community composition, a clear separation between free-living and particle-attached fractions was observed on the NMDS ordination regardless of geographic positions (Supplementary Figure 1C), with a PERMANOVA significance of P < 0.01. Furthermore, the free-living fraction distributions were more scattered than their particle-attached counterpart (Supplementary Figure 1C). Proteobacteria and Actinobacteria were the major lineages for both free-living and particle-attached communities (Figure 1), but Planctomycetes, Nitrospirae, Cyanobacteria, Chlorobi, Bacteroidetes, and Acidobacteria were more abundant in the particle-attached communities.
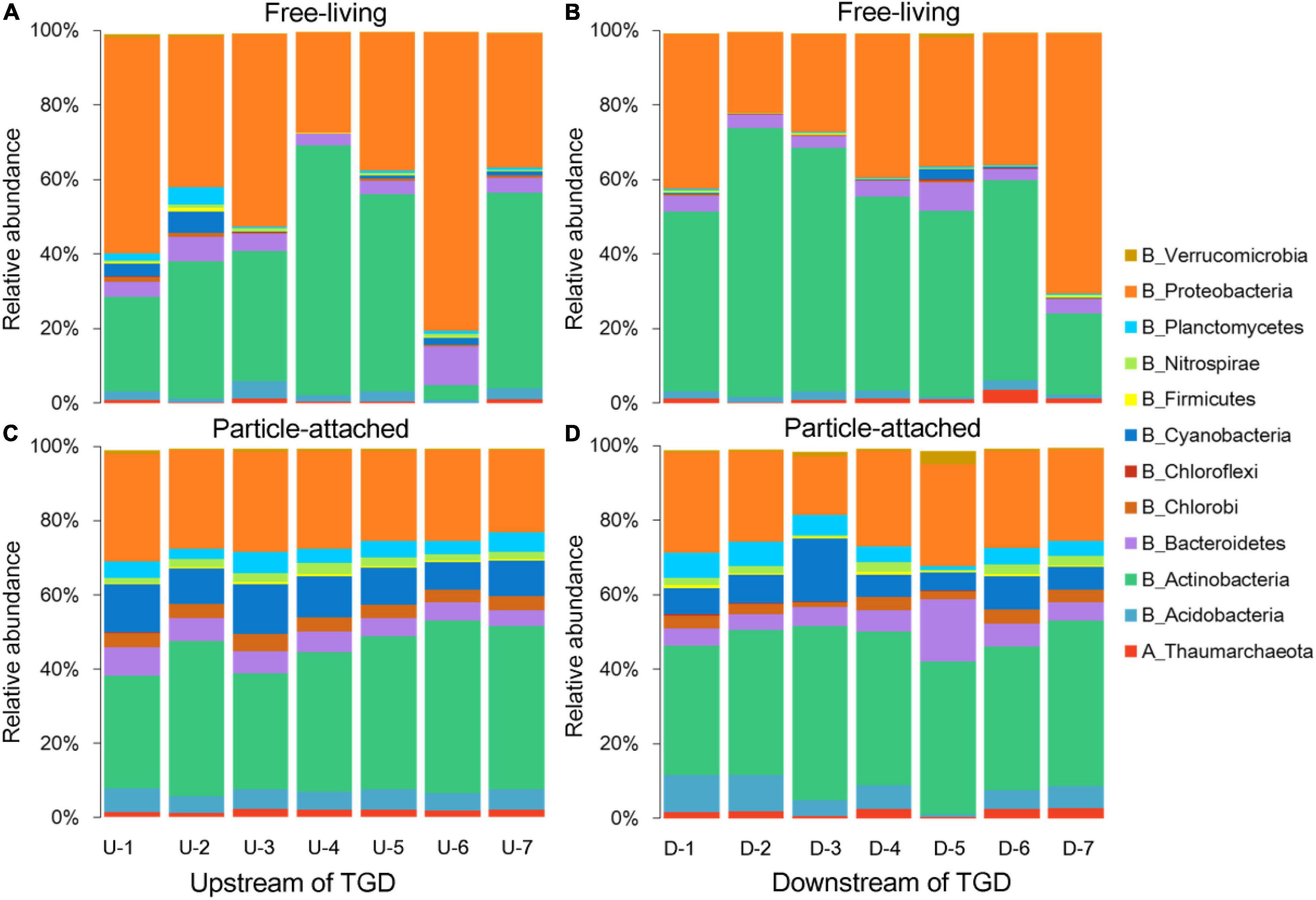
Figure 1. Taxonomic compositions for free-living (A,B) and particle-attached (C,D) communities upstream and downstream of the Three Gorges Dam (TGD), respectively.
Contrasting Features for the Free-Living and Particle-Attached Networks Both Upstream and Downstream of the TGD
We created four networks based on their relative position to the TGD and lifestyle, namely, UF, UP, DF, and DP (Figure 2). The global properties for each network were calculated (Table 1). High R2 values of power law models, ranging from 0.818 to 0.884, indicated that these four networks are scale-free. Module structure could be considered as distinct groups of OTUs, those within a module having more connections among themselves than OTUs outside the module. High modularity values (0.671–0.858) were detected in the free-living (UF and DF) and particle-attached (UP and DP) networks both upstream and downstream of the TGD (Table 1), with particularly higher values in UP (0.858 vs. 0.781 in UF) and DF (0.811 vs. 0.671 in DP) networks. For networks upstream of the TGD, we found greater avgK (UF: 4.77 vs. UP: 2.506) and avgCC (0.381 vs. 0.237) and more inter-module connections (10.78 vs. 5.48%) in the free-living network than the particle-attached network (Table 1). The opposite was true for networks downstream of the TGD, with lower values in DF than DP network (avgK: 3.489 vs. 6.637; avgCC: 0.285 vs. 0.369; inter-module connections: 3.40% vs. 12.40%) (Table 1). A randomization procedure detected that the constructed networks were significantly distinct from the randomly generated networks.
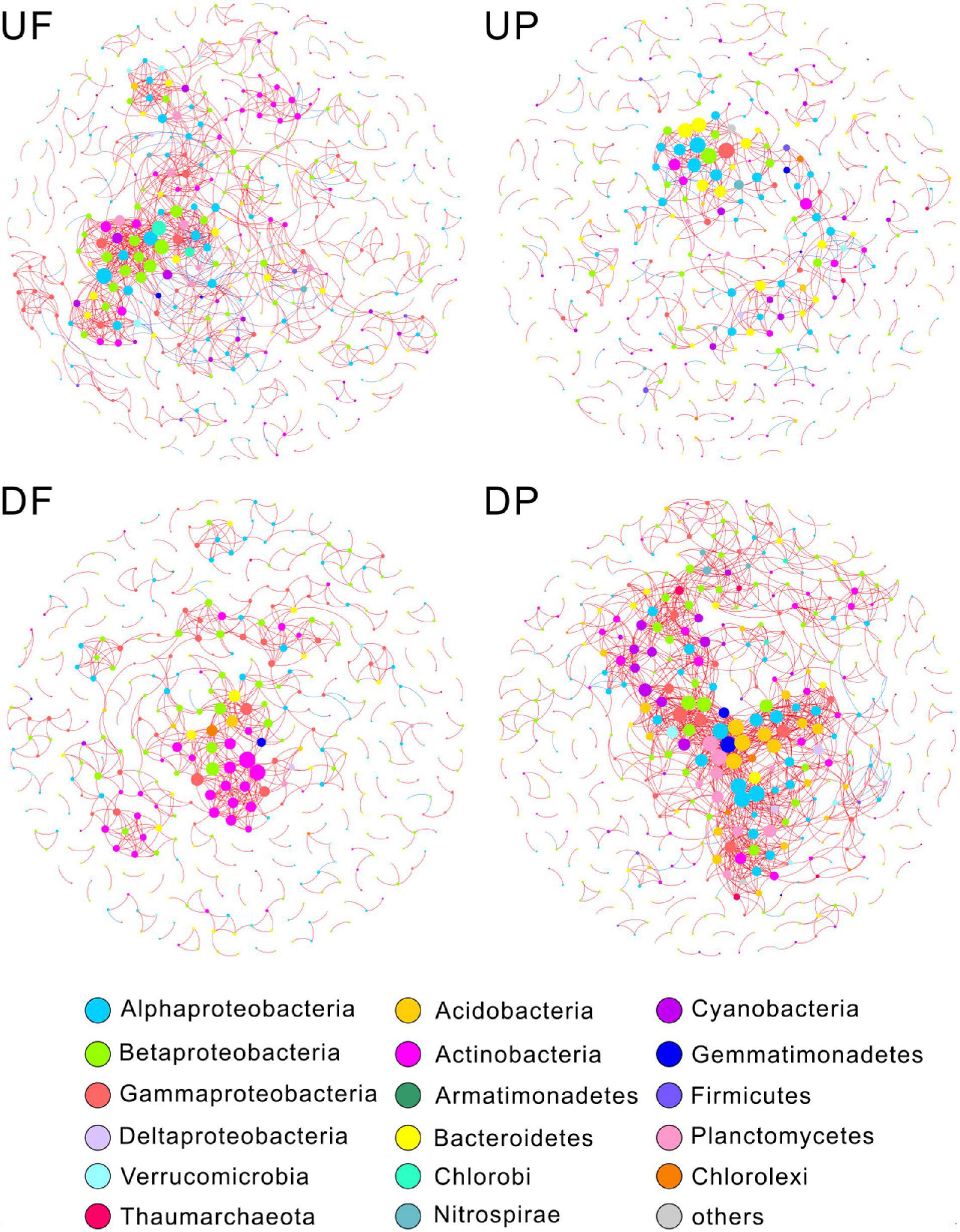
Figure 2. Microorganisms with different lifestyles also differ in their response to the influence of the dam. Nodes are colored by phylogenetic phyla (class for Proteobacteria). Node sizes are proportional to degree. A red link indicates positive correlation, and a blue link indicates negative correlation. UF, free-living network for upstream; UP, particle-attached network for upstream; DF, free-living network for downstream; DP, particle-attached network for downstream.
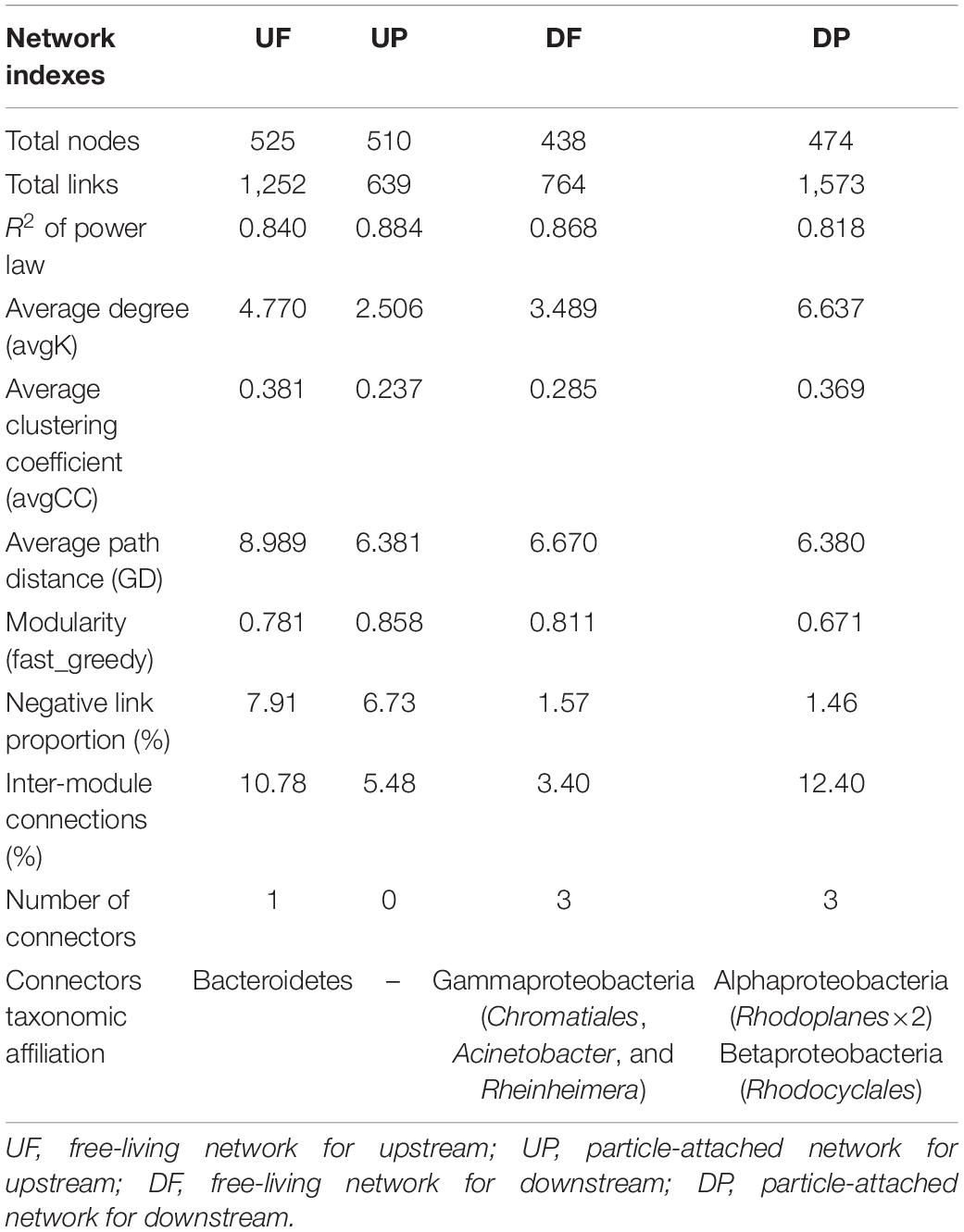
Table 1. Topological properties of free-living and particle-attached networks upstream and downstream of the TGD.
Four ecological roles, namely, peripherals, module hubs, network hubs, and connectors, were assigned to each node based on connectivity within and among modules. In this study, we did not detect network hubs and module hubs for UF, UP, DF, and DP networks, but we found some connectors. One was distributed in the UF network, assigned to Bacteroidetes; three in the DF network, belonging to different genera (Acinetobacter, Rheinheimera, and an unknown genus in Chromatiales) in Gammaproteobacteria; and three in the DP network, two of which affiliated with Rhodoplanes in Alphaproteobacteria and one with Rhodocyclales in Betaproteobacteria (Table 1). Notably, although fewer total species were involved in the co-occurrence networks downstream of the TGD (total nodes of 438 for DF and 474 for DP) than those upstream (total nodes of 525 for UF and 510 for UP), more connectors were identified (DF: three connectors + DP: three connectors vs. UF: one connector + UP: none) (Table 1). The UF connector was found in the UP network as a peripheral node. One of the three DF connectors was persistent in UF, UP, and DP networks and the other two occurred in the UF network. Two of the DP connectors (belonging to Alphaproteobacteria) were found as UP nodes, and the other one in Betaproteobacteria was detected as a DF node.
Greater Variation in Species Interactions With Regard to Plankton Lifestyle Than to Geographic Position
We then simplified networks by grouping nodes belonging to the same taxonomic affiliation to show the interaction differences at high taxonomic level (Supplementary Figure 2). Similar trends were detected that UF and DP interactions were more complex than UP and DF. Response ratio results showed that a total of seven phyla were detected significantly different between free-living and particle-attached networks (Figure 3), including an archaeal phylum Thaumarchaeota and five bacterial phyla Nitrospirae, Planctomycetes, Cyanobacteria, Acidobacteria, and Bacteroidetes and a bacterial class Gammaproteobacteria. However, when considering geographic position regardless of lifestyle, only two bacterial classes (Alphaproteobacteria and Deltaproteobacteria) were significantly different between overall upstream and downstream networks (Supplementary Figure 3).
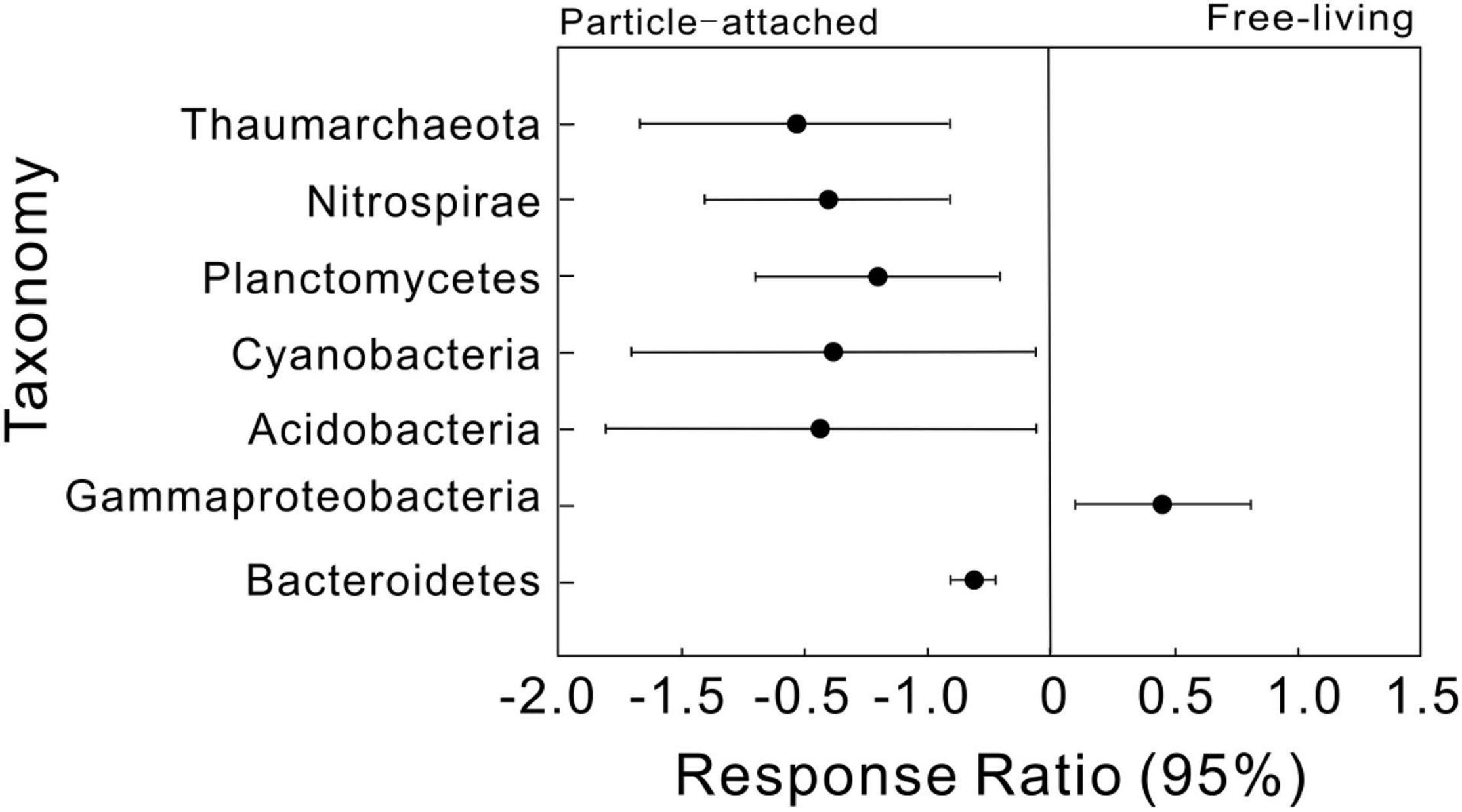
Figure 3. Response ratio analysis of taxonomic changes in particle-attached (UP and DP) and free-living networks (UF and DF). Values greater than zero indicate increased relative abundances in the free-living networks, while those less than zero indicate microbes enriched in particle-attached networks.
Although the relative abundances of taxa have less variation between overall upstream and downstream networks, we did detect great changes in network structures (Supplementary Table 1). More complex and denser network was observed downstream of the TGD, characterized by higher avgK (7.781 vs. 5.391 in upstream network), shorter GD (3.528 vs. 4.681), fewer modules (27 vs. 36), and lower modularity (0.570 vs. 0.692).
Persistent Species Co-occurred in the Free-Living and Particle-Attached Networks Both Upstream and Downstream of the TGD
Although distinctly different network structures were detected for free-living and particle-attached communities both upstream and downstream of the TGD (Figure 2 and Table 1), a total of 112 OTUs were identified to persist in UF, UP, DF, and DP networks, and we extracted them to generate sub-networks (Figure 4). These co-occurring OTUs occupied 21.96–25.57% of the total nodes involved in those four networks, but when considering the first neighbors, they together occupied 48.04–67.09% of the total nodes and 62.60–89.57% of the total links (Figure 5). The relative abundances of these species were generally lower than 1% except for five OTUs. These first neighbors are all identified as peripheral nodes, but they occupied a high proportion and some of them have high degree and high betweenness, which could be hub nodes. Species interactions in all the four networks were mainly dominated by positive associations. Despite of low abundance of negative edges, the proportion is higher in networks upstream (negative proportion: 4.85–6.55%) than downstream (0.105–0.764%) (Table 1).
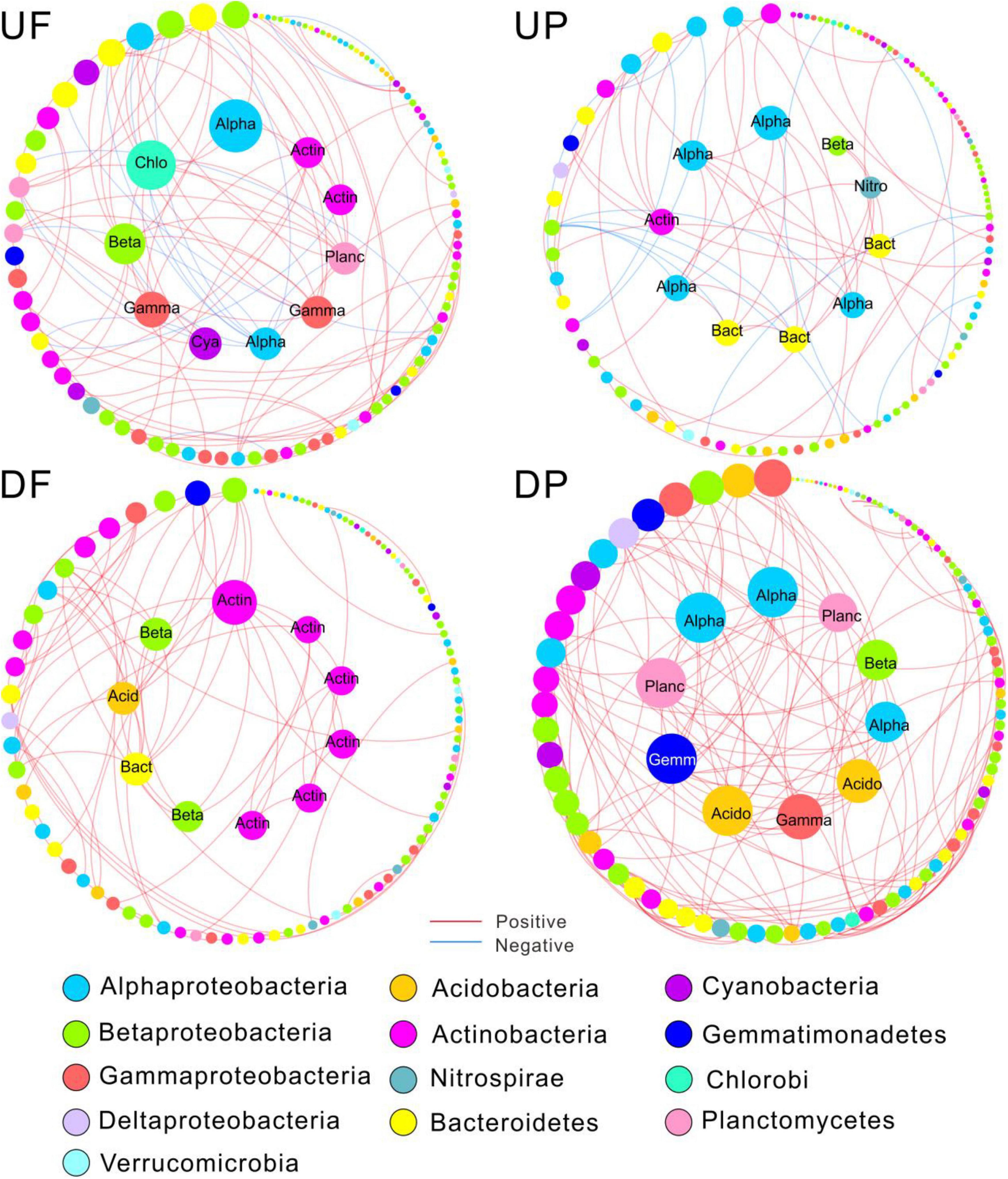
Figure 4. Sub-networks for the 112 shared operational taxonomic units (OTUs) persistent in the four networks. The node size was constant with the overall networks, proportional to node degree. Edges were only kept those among the shared nodes. The top 10 OTUs with the highest node connectivity are shown in inner circles and marked with taxonomic affiliation. Alpha, Alphaproteobacteria; Beta, Betaproteobacteria; Gamma, Gammaproteobacteria; Chlo, Chloroflexi; Cya, Cyanobacteria; Acid, Acidobacteria; Actin, Actinobacteria; Bact, Bacteroidetes; Planc, Planctomycetes; Gemm, Gemmatimonadetes; Nitro, Nitrospirae; UF, free-living network for upstream; UP, particle-attached network for upstream; DF, free-living network for downstream; DP, particle-attached network for downstream.
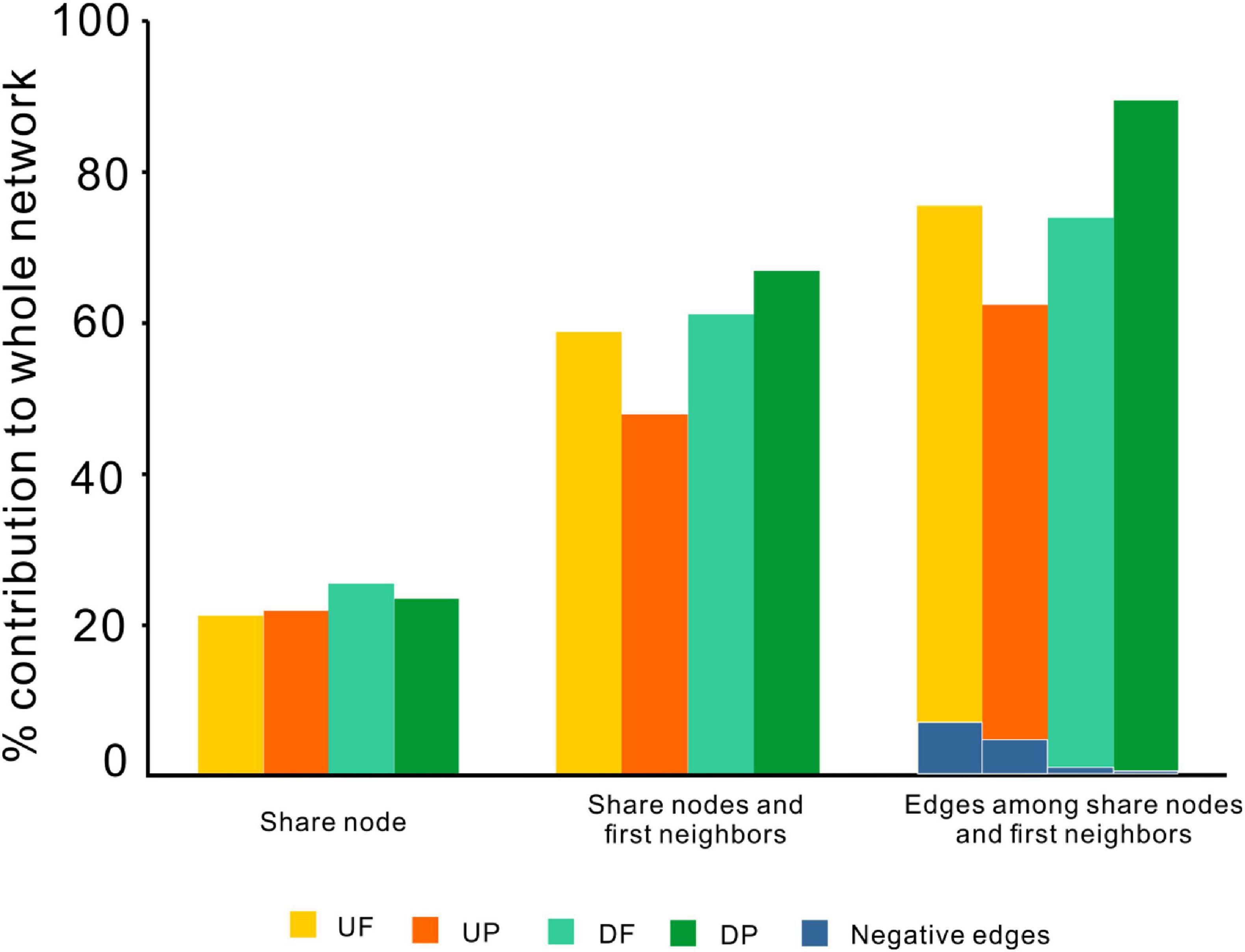
Figure 5. The contributions of shared OTUs and their first neighbors to the whole networks. UF, free-living network for upstream; UP, particle-attached network for upstream; DF, free-living network for downstream; DP, particle-attached network for downstream.
Nodes with higher connectivity are considered as central nodes, so we focused on the top 10 nodes for each sub-network and found that species involved shifted among sub-networks (Figure 4). Specifically, the central nodes for UF sub-network contained Proteobacteria (Alpha-, Beta-, and Gamma-), Chloroflexi, Cyanobacteria, Planctomycetes, and Actinobacteria. The number of central nodes belonging to Alphaproteobacteria and Bacteroidetes increased in UP sub-network and Nitrospirae occurred. A total of six central nodes affiliated with Actinobacteria were found in DF sub-network, indicating Actinobacteria played critical roles in the free-living community downstream of the TGD. The predominant position of Actinobacteria was replaced by Proteobacteria (Alpha-, Beta-, and Gamma-), Planctomycetes, Acidobacteria, and Gemmatimonadetes in DP sub-network.
The Correlations Between the Network Structures and Environmental Conditions
To explore the effects of water geochemistry- and nutrient-related factors on variation of network structures, partial Mantel tests were employed (Table 2). The whole network structures did not show any correlation with either geochemistry or nutrient factors. Then, we considered nodes (OTUs) in different taxonomic categories, and some categories showed close relationships with geochemistry, while others were significantly controlled by nutrients (Table 2). For example, in UF network, significant correlations with geochemistry mainly occurred for OTUs belonging to Acidobacteria and Opitutae, and Planctomycea with nutrients. In UP network, Nitrospirae and Verrucomicrobiae were marginally significantly correlated with geochemistry and nutrients, respectively. For DF network, more taxa were correlated with nutrients (Acidobacteria, Planctomycea, and Verrucomicrobiae) than geochemistry (Deltaproteobacteria). In DP network, Deltaproteobacteria and Gemmatimonadetes were correlated with geochemistry and Clostridia with nutrients.
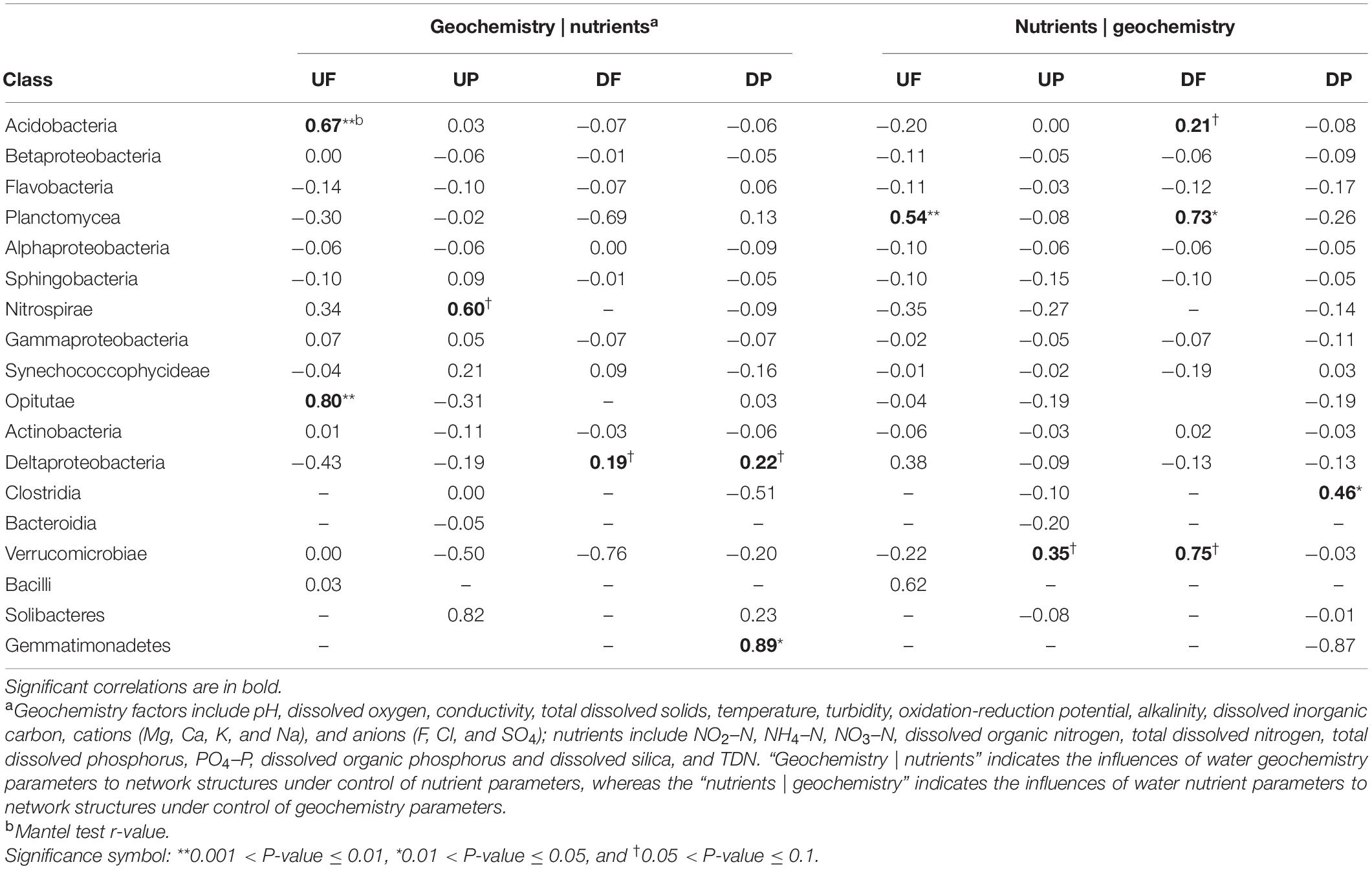
Table 2. The partial Mantel test to reveal environmental parameters that are correlated with class-level sub-network topology.
Discussion
The dam effect on species interactions for free-living and particle-attached planktons provides a new aspect for our understanding about microbial responses to anthropogenic disturbance (i.e., dam construction) in river ecosystems. In this study, an RMT-based approach was employed to reveal the microbial interactions upstream and downstream of the TGD regarding to different lifestyles (free-living vs. particle-attached). Our results revealed that the dam’s impact on microbial interactions was more significant than microbial community composition. Specifically, lifestyle was the predominant factor in determining microbial community structures regardless of geographic positions relative to the dam (Figure 1 and Supplementary Figure 1), but the situation for microbial interactions was more complex. Both lifestyles and geographic positions influenced the species co-occurrence patterns (Figure 2 and Table 1). A previous study reported decreased microbial diversity immediately downstream of the TGD (Liu et al., 2018), and our findings added that hydrodynamic conditions downstream of the dam favored overall denser species interactions, but free-living and particle-attached communities performed differentially upstream and downstream of the dam.
Contrasting microbial co-occurrence patterns for free-living and particle-attached planktons between upstream and downstream of the dam could be a sign of dam impact. A previous study demonstrated that microbial interactions in particle-attached community were more complex than those in free-living community (Wang et al., 2020), and we indeed found such phenomena for samples downstream of the dam (Figure 2 and Table 1). An opposite situation was displayed for samples upstream of the dam, the free-living network showed more complex interactions than the particle-attached network, as evidenced by higher avgK and avgCC, weakened modular structure, and more inter-module connections (Table 1). Dam-induced hydrological and geochemical changes between upstream and downstream of the dam should be the primary reason for this discrepancy. Lower flow velocity or longer water resident time (WRT) was characterized in the TGD reservoir (Tang et al., 2018), especially during water impoundment in dry season. During our sampling time (October 2011), the suspended sediment inflow dramatically decreased as compared to September 2011 (Tang et al., 2016). Furthermore, long WRT allowed more particles to settle down, as evidenced by the lower turbidity immediately upstream of the dam. As the physical space for particle-attached community reduced, the opportunity of microbe–microbe interactions may be weakened. In contrast, serious sediment scouring just before our sampling time increased the suspended particles in the water immediately downstream of the dam and further enhanced the microbial interactions in particle-attached community. Therefore, the relatively static conditions immediately upstream of the dam favored free-living community, but the more dynamic conditions downstream of the dam favored particle-attached community. Despite these differences, some taxonomic lineages were favored in both particle-attached networks (Figure 3). Their co-occurrence might reflect similar eco-physiological responses to varying environmental conditions or metabolic interactions. For instance, the enrichment of heterotrophic bacteria such as Bacteroidetes may imply their roles in dissolved organic matter transformation (Fernandez-Gomez et al., 2013), and the co-occurrence of Thaumarchaeota and Nitrospirae potentially indicates the co-metabolic interaction between ammonia-oxidizing and nitrite-oxidizing bacteria (Reji et al., 2019).
Dispersal of microbes from upstream to downstream of the dam could explain the existence of the shared species co-occurring in UF and DF networks, as well as in UP and DP networks (Figure 4). Since these shared OTUs and their first neighbors contributed more than 50% of the nodes and edges in each network (Figure 5), the loss of them could cause severe impacts and even network structure collapse, indicating these persistent species greatly influenced the network structure stability. Furthermore, most of these species are rare species that occupied extremely low abundances (usually <1%). However, increasing evidence showed that rare species could have disproportionately great effects on ecosystem function relative to their low abundance (Lynch and Neufeld, 2015; Avolio et al., 2019). Besides, those shared species between free-living and particle-attached communities also indicated the transition of plankton lifestyle occurring during the dispersal process, and we also found that some peripheral nodes could become central nodes such as connectors in other networks. Typical freshwater microbes such as Actinobacteria, Bacteroidetes, Firmicutes, and Gammaproteobacteria had significant lifestyle variations during the Microcystis bloom in the Three Gorges Reservoir (Liu M. et al., 2019). One possible explanation for this kind of transition is that they occupied unique niches with distinct combinations of resources and microenvironments, which provide their ability to directly respond to environmental change (Yenni et al., 2017). In our case, under the variable hydrological and physicochemical conditions, the shift between free-living and particle-associated lifestyle may potentially help them to bear and utilize the fluctuant nutrient influx over time (e.g., before vs. after water impoundment) and space (e.g., upstream vs. downstream of the TGD). In addition, the taxonomic affiliation of the central nodes for sub-networks related to these shared species varied by lifestyle and geographic positions, indicating the potential function changes.
The topology properties of microbial network could be used to imply community stability under various environmental stress (Hernandez et al., 2021) or under climatic change conditions (Wang et al., 2018). More complex microbial interactions could be beneficial for higher efficiency of resource utilizing (Morrien et al., 2017), better information transferring and disturbance buffering (Layeghifard et al., 2017), and consequently strengthened ecosystem stability (Olesen et al., 2007). Previous studies have shown that a relatively stable microenvironment could be supplied by particles (or substrates) in the fluctuant and dynamic ecosystem (Simon et al., 2002; Garneau et al., 2009), thus leading to more active particle-attached plankton community than the free-living counterpart (Li et al., 2018). Species interactions could be considered as an endogenous regulator of community, as different combinations of microorganism can be recruited to occupy the central positions in networks (Konopka et al., 2015). That is to say, more complex interactions among diverse microbes ensured the buffering capacity against environmental disturbance. Based on the above reasoning, more complex microbial interactions in particle-attached community downstream of the TGD may indicate a more efficient nutrient transferring and substrate transformation.
Conclusion
In summary, this study demonstrated that microbial interaction patterns in the vicinity of the TGD and their relationships to environmental factors varied dependent on both geographic position relative to the dam (upstream vs. downstream of the dam) and plankton lifestyle (free-living vs. particle-attached). Regardless of geographic position, particle-attached plankton community showed more complex and closer interactions, enriching taxa such as Thaumarchaeota, Nitrospirae, Bacteroidetes, and Gammaproteobacteria, which usually form co-metabolic interactions. When considering both geographic position and lifestyle, the common notion that particle-attached species generate more complex interactions than free-living counterpart only held true for communities downstream of the dam, whereas the opposite is true for upstream communities. More complex microbial interactions in particle-attached community downstream of the TGD may indicate higher community stability, which was beneficial for efficient nutrient transferring and substrate transformation. Most interestingly, we found persistent species co-occurred in free-living and particle-attached communities both upstream and downstream of the dam, primarily triggered by both water dispersal (from upstream to downstream) and lifestyle transition (between free-living and particle-attached). These persistent species and their first neighbors in each network contributed more than 50% of the total nodes and edges, indicating their potential great influence on maintaining the network structure in the vicinity of the dam in the river ecosystems. Our results revealed that microbial interactions offered a novel insight into understanding damming-induced impacts on microbial community beyond regular compositions and diversity. In further studies, it is imperative to investigate how dam-induced changes in microbial interactions influence river ecosystem process.
Data Availability Statement
The datasets presented in this study can be found in online repositories. The names of the repository/repositories and accession number(s) can be found below: NCBI BioProject (accession: PRJNA598282).
Author Contributions
SW performed the analysis and prepared the manuscript with the help of YD. WH and YD helped with interpreting the data. HJ and HD conceived the research idea. LH, SC, BW, YC, and BL collected the samples and performed field geochemistry measurements. All authors contributed to the article and approved the submitted version.
Funding
This project was supported by the National Natural Science Foundation of China (Nos. U1906223, 41672331, and 41807316) and the National Key R&D Program of China (No. 2016YFC0502204).
Conflict of Interest
The authors declare that the research was conducted in the absence of any commercial or financial relationships that could be construed as a potential conflict of interest.
Supplementary Material
The Supplementary Material for this article can be found online at: https://www.frontiersin.org/articles/10.3389/fevo.2021.624476/full#supplementary-material
Supplementary Figure 1 | Samples grouped by microbial community composition and environmental conditions.
Supplementary Figure 2 | Simplified networks by grouping nodes belong to the same taxonomic affiliation.
Supplementary Figure 3 | Response ratio analysis revealing taxa enriched in downstream networks as compared to upstream.
Supplementary Table 1 | Global properties for overall upstream and downstream networks regardless of lifestyle.
Footnotes
References
Aronesty, E. (2011). ea-utils : “Command-Line Tools for Processing Biological Sequencing Data”. Available online at: https://github.com/ExpressionAnalysis/ea-utils (accessed April 15, 2016).
Avolio, M. L., Forrestel, E. J., Chang, C. C., La Pierre, K. J., Burghardt, K. T., and Smith, M. D. (2019). Demystifying dominant species. New Phytol. 223, 1106–1126. doi: 10.1111/nph.15789
Barabási, A.-L. (2009). Scale-free networks: a decade and beyond. Science 325, 412–413. doi: 10.1126/science.1173299
Bastian, M., Heymann, S., and Jacomy, M. (2009). “Gephi: an open source software for exploring and manipulating networks,” in Proceedings of the 3rd International AAAI Conference on Weblogs and Social Media, North America, 361–362.
Caporaso, J. G., Kuczynski, J., Stombaugh, J., Bittinger, K., Bushman, F. D., Costello, E. K., et al. (2010). QIIME allows analysis of high-throughput community sequencing data. Nat. Methods 7, 335–336.
Cole, J. R., Wang, Q., Fish, J. A., Chai, B. L., McGarrell, D. M., Sun, Y. N., et al. (2014). Ribosomal Database Project: data and tools for high throughput rRNA analysis. Nucleic Acids Res. 42, D633–D642.
Deng, Y., Jiang, Y. H., Yang, Y. F., He, Z. L., Luo, F., and Zhou, J. Z. (2012). Molecular ecological network analyses. BMC Bioinformatics 13:113. doi: 10.1186/1471-2105-13-113
Edgar, R. C. (2010). Search and clustering orders of magnitude faster than BLAST. Bioinformatics 26, 2460–2461. doi: 10.1093/bioinformatics/btq461
Fernandez-Gomez, B., Richter, M., Schuler, M., Pinhassi, J., Acinas, S. G., Gonzalez, J. M., et al. (2013). Ecology of marine Bacteroidetes: a comparative genomics approach. ISME J. 7, 1026–1037. doi: 10.1038/ismej.2012.169
Garneau, M. -È, Vincent, W. F., Terrado, R., and Lovejoy, C. (2009). Importance of particle-associated bacterial heterotrophy in a coastal Arctic ecosystem. J. Mar. Syst. 75, 185–197. doi: 10.1016/j.jmarsys.2008.09.002
Grill, G., Lehner, B., Lumsdon, A. E., MacDonald, G. K., Zarfl, C., and Reidy Liermann, C. (2015). An index-based framework for assessing patterns and trends in river fragmentation and flow regulation by global dams at multiple scales. Environ. Res. Lett. 10:015001. doi: 10.1088/1748-9326/10/1/015001
Grossart, H. P. (2010). Ecological consequences of bacterioplankton lifestyles: changes in concepts are needed. Environ. Microbiol. Rep. 2, 706–714. doi: 10.1111/j.1758-2229.2010.00179.x
Haas, B. J., Gevers, D., Earl, A. M., Feldgarden, M., Ward, D. V., Giannoukos, G., et al. (2011). Chimeric 16S rRNA sequence formation and detection in Sanger and 454-pyrosequenced PCR amplicons. Genome Res. 21, 494–504. doi: 10.1101/gr.112730.110
Hernandez, D. J., David, A. S., Menges, E. S., Searcy, C. A., and Afkhami, M. E. (2021). Environmental stress destabilizes microbial networks. ISME J. doi: 10.1038/s41396-020-00882-x [Epub ahead of print].
Huang, L., Dong, H., Jiang, H., Wang, S., and Yang, J. (2016). Relative importance of advective flow versus environmental gradient in shaping aquatic ammonium oxidizers near the Three Gorges Dam of the Yangtze River, China. Environ. Microbiol. Rep. 8, 667–674. doi: 10.1111/1758-2229.12420
Konopka, A., Lindemann, S., and Fredrickson, J. (2015). Dynamics in microbial communities: unraveling mechanisms to identify principles. ISME J. 9, 1488–1495. doi: 10.1038/ismej.2014.251
Layeghifard, M., Hwang, D. M., and Guttman, D. S. (2017). Disentangling interactions in the microbiome: a network perspective. Trends Microbiol. 25, 217–228. doi: 10.1016/j.tim.2016.11.008
Li, J., Zhang, J., Liu, L., Fan, Y., Li, L., Yang, Y., et al. (2015). Annual periodicity in planktonic bacterial and archaeal community composition of eutrophic Lake Taihu. Sci. Rep. 5:15488.
Li, J. L., Salam, N., Wang, P. D., Chen, L. X., Jiao, J. Y., Li, X., et al. (2018). Discordance between resident and active bacterioplankton in free-living and particle-associated communities in estuary ecosystem. Microb. Ecol. 76, 637–647. doi: 10.1007/s00248-018-1174-4
Li, Y., Gao, Y., Zhang, W., Wang, C., Wang, P., Niu, L., et al. (2019). Homogeneous selection dominates the microbial community assembly in the sediment of the three gorges reservoir. Sci. Total Environ. 690, 50–60. doi: 10.1016/j.scitotenv.2019.07.014
Liermann, C. R., Nilsson, C., Robertson, J., and Ng, R. Y. (2012). Implications of dam obstruction for global freshwater fish diversity. Bioscience 62, 539–548. doi: 10.1525/bio.2012.62.6.5
Liu, J., Soininen, J., Han, B.-P., Declerck, S. A. J., and Whittaker, R. (2013). Effects of connectivity, dispersal directionality and functional traits on the metacommunity structure of river benthic diatoms. J. Biogeogr. 40, 2238–2248. doi: 10.1111/jbi.12160
Liu, L., Chen, H., Liu, M., Yang, J. R., Xiao, P., Wilkinson, D. M., et al. (2019). Response of the eukaryotic plankton community to the cyanobacterial biomass cycle over 6 years in two subtropical reservoirs. ISME J. 13, 2196–2208. doi: 10.1038/s41396-019-0417-9
Liu, M., Liu, L., Chen, H., Yu, Z., Yang, J. R., Xue, Y., et al. (2019). Community dynamics of free-living and particle-attached bacteria following a reservoir Microcystis bloom. Sci. Total Environ. 660, 501–511. doi: 10.1016/j.scitotenv.2018.12.414
Liu, T., Zhang, A. N., Wang, J., Liu, S., Jiang, X., Dang, C., et al. (2018). Integrated biogeography of planktonic and sedimentary bacterial communities in the Yangtze River. Microbiome 6:16.
Lynch, M. D. J., and Neufeld, J. D. (2015). Ecology and exploration of the rare biosphere. Nat. Rev. Microbiol. 13, 217–229. doi: 10.1038/nrmicro3400
Maavara, T., Chen, Q., Van Meter, K., Brown, L. E., Zhang, J., Ni, J., et al. (2020). River dam impacts on biogeochemical cycling. Nat. Rev. Earth Environ. 1, 103–116. doi: 10.1038/s43017-019-0019-0
Maavara, T., Durr, H. H., and Van Cappellen, P. (2014). Worldwide retention of nutrient silicon by river damming: from sparse data set to global estimate. Glob. Biogeochem. Cycles 28, 842–855. doi: 10.1002/2014gb004875
Maavara, T., Parsons, C. T., Ridenour, C., Stojanovic, S., Durr, H. H., Powley, H. R., et al. (2015). Global phosphorus retention by river damming. Proc. Natl. Acad. Sci. U.S.A. 112, 15603–15608. doi: 10.1073/pnas.1511797112
Maren, D. S., Yang, S.-L., and He, Q. (2013). The impact of silt trapping in large reservoirs on downstream morphology: the Yangtze River. Ocean Dyn. 63, 691–707. doi: 10.1007/s10236-013-0622-4
Mihalicz, J. E., Jardine, T. D., Baulch, H. M., and Phillips, I. D. (2019). Seasonal effects of a hydropeaking dam on a downstream benthic macroinvertebrate community. River Res. Appl. 35, 714–724. doi: 10.1002/rra.3434
Morrien, E., Hannula, S. E., Snoek, L. B., Helmsing, N. R., Zweers, H., de Hollander, M., et al. (2017). Soil networks become more connected and take up more carbon as nature restoration progresses. Nat. Commun. 8:14349.
Newman, M. E. J. (2006). Modularity and community structure in networks. Proc. Natl. Acad. Sci. U.S.A. 103, 8577.
Olesen, J. M., Bascompte, J., Dupont, Y. L., and Jordano, P. (2007). The modularity of pollination networks. Proc. Natl. Acad. Sci. U.S.A. 104, 19891–19896. doi: 10.1073/pnas.0706375104
Reji, L., Tolar, B. B., Smith, J. M., Chavez, F. P., and Francis, C. A. (2019). Differential co-occurrence relationships shaping ecotype diversification within Thaumarchaeota populations in the coastal ocean water column. ISME J. 13, 1144–1158. doi: 10.1038/s41396-018-0311-x
Rosel, S., and Grossart, H. P. (2012). Contrasting dynamics in activity and community composition of free-living and particle-associated bacteria in spring. Aquat. Microb. Ecol. 66, 169–181. doi: 10.3354/ame01568
Schloss, P. D., Westcott, S. L., Ryabin, T., Hall, J. R., Hartmann, M., Hollister, E. B., et al. (2009). Introducing mothur: open-source, platform-independent, community-supported software for describing and comparing microbial communities. Appl. Environ. Microbiol. 75, 7537–7541. doi: 10.1128/aem.01541-09
Sharp, J. H., Hansell, D. A., and Carlson, C. A. (2002). Analytical Methods for Total DOM Pools, Biogeochemistry of Marine Dissolved Organic Matter, eds. San Diego, USA: Academic Press, 35–58.
Simon, M., Grossart, H. P., Schweitzer, B., and Ploug, H. (2002). Microbial ecology of organic aggregates in aquatic ecosystems. Aquat. Microb. Ecol. 28, 175–211. doi: 10.3354/ame028175
Smith, M. W., Zeigler Allen, L., Allen, A. E., Herfort, L., and Simon, H. M. (2013). Contrasting genomic properties of free-living and particle-attached microbial assemblages within a coastal ecosystem. Front. Microbiol. 4:120. doi: 10.3389/fmicb.2013.00120
Tang, Q., Bao, Y., He, X., Fu, B., Collins, A. L., and Zhang, X. (2016). Flow regulation manipulates contemporary seasonal sedimentary dynamics in the reservoir fluctuation zone of the Three Gorges Reservoir, China. Sci. Total Environ. 548-549, 410–420. doi: 10.1016/j.scitotenv.2015.12.158
Tang, Q., Fu, B., Collins, A. L., Wen, A., He, X., and Bao, Y. (2018). Developing a sustainable strategy to conserve reservoir marginal landscapes. Natl. Sci. Rev. 5, 10–14. doi: 10.1093/nsr/nwx102
Teeling, H., Fuchs, B. M., Becher, D., Klockow, C., Gardebrecht, A., Bennke, C. M., et al. (2012). Substrate-controlled succession of marine bacterioplankton populations induced by a phytoplankton bloom. Science 336, 608–611. doi: 10.1126/science.1218344
Vorosmarty, C. J., Meybeck, M., Fekete, B., Sharma, K., Green, P., and Syvitski, J. P. M. (2003). Anthropogenic sediment retention: major global impact from registered river impoundments. Glob. Planet. Change 39, 169–190. doi: 10.1016/s0921-8181(03)00023-7
Wang, L., Han, M., Li, X., Yu, B., Wang, H., Ginawi, A., et al. (2021). Mechanisms of niche-neutrality balancing can drive the assembling of microbial community. Mol. Ecol. 30, 1492–1504. doi: 10.1111/mec.15825
Wang, S., Dong, R. M., Dong, C. Z., Huang, L., Jiang, H., Wei, Y., et al. (2012). Diversity of microbial plankton across the Three Gorges Dam of the Yangtze River, China. Geosci. Front. 3, 335–349. doi: 10.1016/j.gsf.2011.11.013
Wang, S., Hou, W., Jiang, H., Huang, L., Dong, H., Chen, S., et al. (2021). Microbial diversity accumulates in a downstream direction in the Three Gorges Reservoir. J. Environ. Sci. 101, 156–167. doi: 10.1016/j.jes.2020.08.006
Wang, S., Wang, X., Han, X., and Deng, Y. (2018). Higher precipitation strengthens the microbial interactions in semi-arid grassland soils. Glob. Ecol. Biogeogr. 27, 570–580. doi: 10.1111/geb.12718
Wang, Y., Pan, J., Yang, J., Zhou, Z., Pan, Y., and Li, M. (2020). Patterns and processes of free-living and particle-associated bacterioplankton and archaeaplankton communities in a subtropical river-bay system in South China. Limnol. Oceanogr. 65, S161–S179.
Widder, S., Besemer, K., Singer, G. A., Ceola, S., Bertuzzo, E., Quince, C., et al. (2014). Fluvial network organization imprints on microbial co-occurrence networks. Proc. Natl. Acad. Sci. U.S.A. 111, 12799–12804. doi: 10.1073/pnas.1411723111
Wu, J. G., Huang, J. H., Han, X. G., Gao, X. M., He, F. L., Jiang, M. X., et al. (2004). The Three Gorges Dam: an ecological perspective. Front. Ecol. Environ. 2, 241–248.
Xu, H., Zhao, D., Huang, R., Cao, X., Zeng, J., Yu, Z., et al. (2018). Contrasting network features between free-living and particle-attached bacterial communities in Taihu Lake. Microb. Ecol. 76, 303–313. doi: 10.1007/s00248-017-1131-7
Yan, Q., Bi, Y., Deng, Y., He, Z., Wu, L., Van Nostrand, J. D., et al. (2015). Impacts of the Three Gorges Dam on microbial structure and potential function. Sci. Rep. 5:8605.
Yenni, G., Adler, P. B., and Ernest, S. K. M. (2017). Do persistent rare species experience stronger negative frequency dependence than common species? Glob. Ecol. Biogeogr. 26, 513–523. doi: 10.1111/geb.12566
Keywords: microbial interaction, the Three Gorges Dam, free-living, particle-attached, persistent species, RMT-based network
Citation: Wang S, Hou W, Jiang H, Dong H, Huang L, Chen S, Wang B, Chen Y, Lin B and Deng Y (2021) The Lifestyle-Dependent Microbial Interactions Vary Between Upstream and Downstream of the Three Gorges Dam. Front. Ecol. Evol. 9:624476. doi: 10.3389/fevo.2021.624476
Received: 31 October 2020; Accepted: 16 April 2021;
Published: 24 May 2021.
Edited by:
Haipeng Wu, Changsha University of Science and Technology, ChinaReviewed by:
Yonghong Bi, Institute of Hydrobiology, Chinese Academy of Sciences, ChinaZhang Min, China Institute of Water Resources and Hydropower Research, China
Copyright © 2021 Wang, Hou, Jiang, Dong, Huang, Chen, Wang, Chen, Lin and Deng. This is an open-access article distributed under the terms of the Creative Commons Attribution License (CC BY). The use, distribution or reproduction in other forums is permitted, provided the original author(s) and the copyright owner(s) are credited and that the original publication in this journal is cited, in accordance with accepted academic practice. No use, distribution or reproduction is permitted which does not comply with these terms.
*Correspondence: Ye Deng, yedeng@rcees.ac.cn