- Shandong Institute of Parasitic Diseases, Shandong First Medical University, Shandong Academy of Medical Sciences, Jining, China
As Jiaxiang County of Shandong province is an area with complex mosquito vector composition, it is necessary to investigate the relationship between bacterial diversity, physicochemical factors, and larval density. Therefore, the physicochemical properties of 46 breeding sites for six kinds of habitat types (small puddles, small water containers, paddy fields, large water containers, irrigation channels, and drainage ditches) were investigated by a multiparameter analyzer; the water’s bacterial diversity was analyzed by the 16S rRNA full-length sequencing method. Spearman correlation and multiple linear regression were used to analyze the correlation between larval density and variables. The variables analyzed were dissolved oxygen, pH, hardness, turbidity, conductivity, temperature, ammonia nitrogen, water depth, and distance from the nearest house. One-Way ANOVA was used to understand whether there are differences in bacterial diversity in different habitats. Pearson linear correlation model was used to analyze the effects of bacterial diversity and richness on mosquito densities in breeding sites. A total of 3291 larvae were captured, and a total of 6 species of 4 genera were identified. The identified species were Culex pipiens pallens, Aedes albopictus, Anopheles sinensis, Culex tritaeniorhynchus, Culex bitaeniorhynchus, and Mansonia uniformis. The density and species can be jointly affected by physicochemical properties and bacterial diversity, especially Shannon index and distance from the nearest house. In general, the physicochemical parameters and bacterial diversity of different habitats were significantly different. Even for the same habitat type, the physicochemical parameters varied greatly due to different environments.
Introduction
Mosquito is an important medical arthropod transmitting various mosquito-borne diseases such as malaria, dengue fever, and Japanese encephalitis. The larva and pupa stages of various mosquitoes are in water all the time, and the water body with positive larvae is regarded as their breeding sites (El-Ghani et al., 2012; Guo et al., 2017; Ferreira-de-Lima and Lima-Camara, 2018). Jiaxiang County is located in the Southwest Plain of Shandong Province, China. It has a temperate monsoon climate, numerous rivers and lakes, and a developed water system. All these conditions are suitable for breeding a variety of mosquitoes. Culex pipiens pallens and Aedes albopictus are the dominant mosquito species (Wang et al., 2020). The former is a potential vector of the West Nile virus (WNV) in Shandong Province (Jiang et al., 2014). The latter caused an outbreak of the dengue virus in Jiaxiang County in August 2017. There were 79 cases, which made the area a focus of attention for epidemic prevention personnel (Shi et al., 2019; Liu et al., 2020). In addition, Anopheles sinensis is the most important plasmodium vector in Shandong Province. In 2010, Shandong Province reported 117 malaria cases, among which 70% were imported cases. This may be related to the large rice and lotus cultivation area in southwest Shandong Province, which created a favorable breeding environment for Anopheles (Liu et al., 2015).
It is widely believed that larval source management (LSM) can effectively prevent mosquitoes’ breeding and development. Researches show that LSM has an inhibitory effect on transmitting mosquito-borne diseases, whether in the countryside or a city. However, Jiaxiang County is rich in water resources, is densely populated, and its residents have a weak awareness of prevention, applying unreasonable mosquito control methods, and inadequate sanitation management. Hence, it is difficult to manage the larval breeding sites in this area (Wang et al., 2020). The Breteau Index (BI), an indicator of the density of Aedes mosquitoes in an area, can be used to assess the risk of dengue epidemics in that area. According to reports, the average BI of Jiaxiang County in August 2017 hit a stunning 107.27, whereas, for 2017 and 2018, it was 45.30 and 15.95, respectively (Liu et al., 2020). Areas where BI>20 will be regarded as high-risk means that any importation of external cases could cause a dengue epidemic in this area (Aryaprema and Xue, 2019). These data indirectly indicate that Ae. albopictus density in Jiaxiang County is high, and there is a potential risk of mosquito-borne virus transmission. In addition, in 2018, the resistance ratio of Cx. p. pallens to DDVP, propoxur, cypermethrin and deltamethrin was 19.76, 4.6, 438, and 351, respectively. Also, over the last decade, the resistance of Cx. p. pallens to different types of insecticides has risen to varying degrees (Liu et al., 2019; Wang et al., 2020). Therefore, scientific guidance is urgently needed to carry out LSM in this county. To some extent, understanding the larval density and distribution in this area, as well as the environment of breeding sites, is the first step in this plan.
While choosing the spawning site, female mosquitoes follow specific cues such as physicochemical factors including dissolved oxygen (DO), pH, hardness, turbidity, conductivity, temperature, ammonia nitrogen (AN), water depth (WD), and several biological factors (e.g., the composition of microorganism in water) (Juliano et al., 2004). They try to avoid water bodies where competitors and/or predators are already present and prefer to choose safe water bodies with specific physicochemical properties and microorganisms (Bentley and Day, 1989; Lin et al., 2008). In addition, the distance from the nearest house (DNH) is an important factor that needed to be considered to estimate the significance of the study to public health (Killeen et al., 2001). The research demonstrated that the distance between breeding sites and the nearest house determined the level of blood uptake by a female mosquito, which in turn affected the density, emergence rate of larvae, growth cycle, and malaria transmission dynamics (Killeen et al., 2001). However, with the expansion of urban sprawl to rural areas and frequent personnel exchanges, significant changes have taken place in the environment of breeding sites (Jacob et al., 2005). This affects the density and distribution of mosquito larvae, which, to a certain degree, increases the risk of transmission of mosquito-borne diseases and boosts the pressure of its prevention and control as well.
Bacteria in the breeding sites and other biological factors jointly create the external environment in which the larvae can live, exchanging materials directly with larvae intestines (Dada et al., 2018). This can directly affect the survival status and even the density of the larvae (Mukhopadhyay and Chatterjee, 2016). Hence, it is necessary to investigate the composition of bacteria of water in breeding sites. This information will enable us to estimate whether water bacterial diversity was related to larval density. To our knowledge, habitat analysis on breeding sites is very limited, and reports on bacterial diversity and richness.
of the breeding sites are short (Molineaux, 1997; Qing et al., 2020). To enrich the research findings, we analyzed the relationship between the larval density of Jiaxiang County in Shandong Province and the physicochemical properties and bacterial diversity of water in the breeding sites, and explain the correlation between them using statistics. If water’s physicochemical properties and/or bacterial diversity vary, our results provide a rationale prediction of mosquito density and help develop scientific strategies for mosquito vector control.
Materials and Methods
Selection of the Study Area
Jiaxiang County (35°11′ N, 116°06′ E) is located in the southwest of Shandong Province. Jiaxiang County is hot and rainy from June to August, with an average rainfall of 398.5 mm in summer. Zhu Zhaoxin River passes through, and the water system is developed, providing many favorable conditions for mosquito breeding and reproduction. We selected the Jintun town as the center, which had a dengue fever outbreak in August 2017, and radiated out to the surrounding towns and villages to survey the breeding sites. We invited several epidemic prevention personnel from the local health center to assist us in sampling. Water bodies with larva or pupa activity or eggs were selected as sampling sites (Nambunga et al., 2020). Then, GPS was used to locate breeding sites. The distribution of breeding sites is shown in Figure 1.
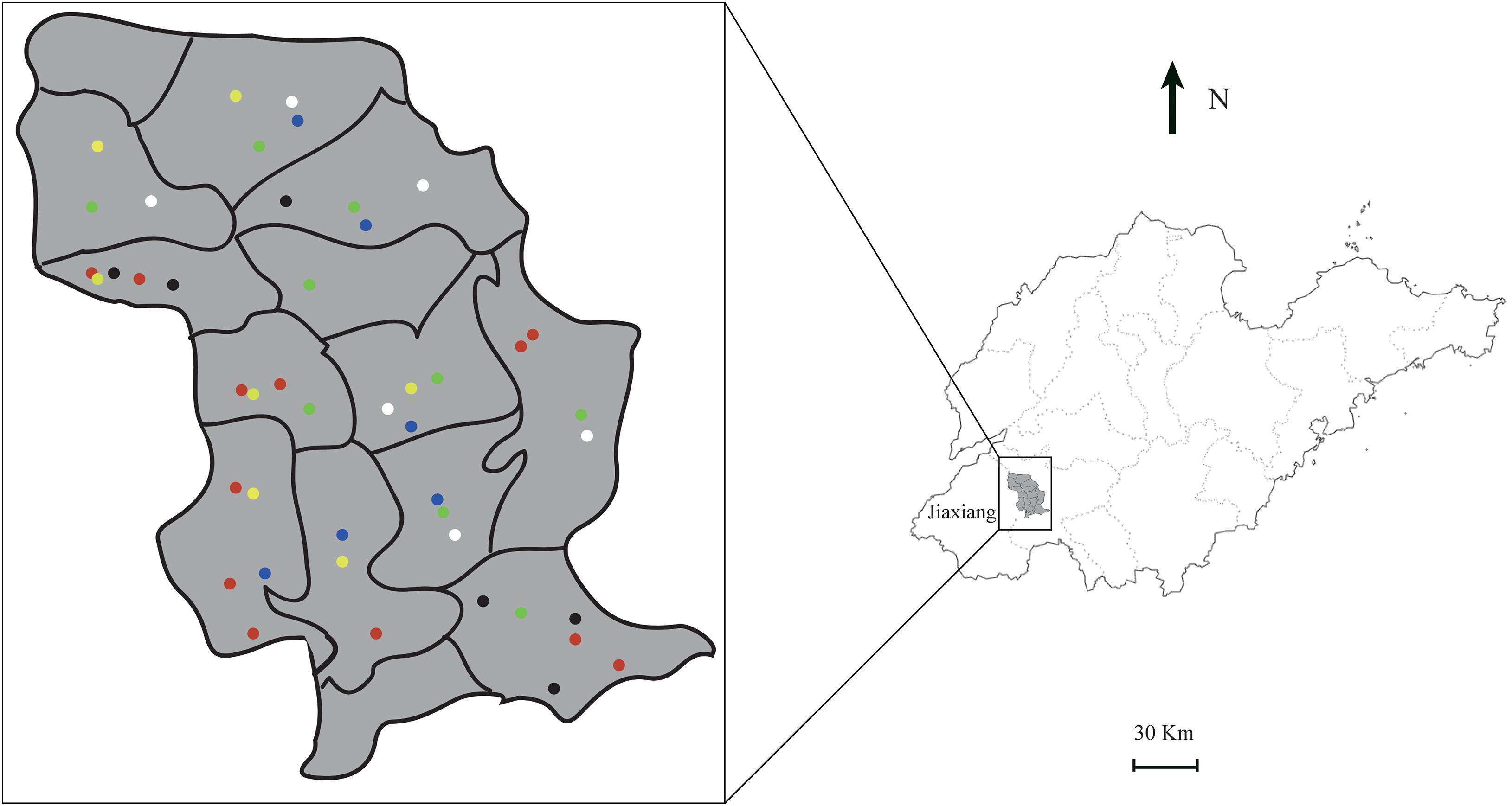
Figure 1. Locations and habitats type of sampling sites in Jiaxiang County. Six black dots represent small puddles, 12 red dots representing small water containers, seven yellow dots representing paddy fields, nine green dots representing large water containers, six white dots representing irrigation channels, and six blue dots representing drainage ditches. The location of each dot displayed a sampling site.
Larva Collection and Identification
Mosquito larva was collected from each breeding site using a standard 350 mL long handle dipper. Each breeding site was dipped four times within a collection period. When the sampling site could not be immersed, siphoning or dumping was used. According to the planned date, each sampling site was sampled five times: July 7, 2019, July 14, 2019, July 23, 2019, July 31, 2019, and August 7, 2019. In the case of thunderstorms or other exceptional circumstances, sampling was postponed. If the water at the sampling site was dry, we located a same-type breeding site nearby. Larvae collected from each breeding site were brought to the Shandong Institute of Parasitic Diseases and classified into species level using standard identification keys (Wilkerson et al., 1990). Quantification of larval density (Villarreal-Treviño et al., 2015) was as follows: D (per dipper) = , where D = mosquito density. The calculation method of mosquito species distribution was based on Kabirul’s method (Bashar et al., 2016): C = × 100%, where C = mosquito species distribution, n = number of positive habitats, and N = the total number of habitats.
Identification of the Water Physicochemical Properties
At the site, we measured the water physicochemical properties of each sampling site, including pH, turbidity (NTU: Nephelometric turbidity unit), conductivity (μS/cm), DO (mg/L), AN (mg/L), hardness (mg/L), and temperature (°C) using a portable multiparameter tester (Palintest Potacheck 2, United Kingdom). WD (cm) and DNH (m) were measured by a graduated string with a lead weight and a tapeline, respectively. After the measurement, at least 200 mL of water was taken from each sampling site and placed into a sterile conical flask. Then, the flask was placed into an icebox and brought back to the laboratory to analyze the water body’s bacterial diversity and richness. The collection time, locations, environmental status, and habitat types were recorded timely and in detail.
Analysis of Bacterial Diversity and Richness
The water samples of each breeding site were centrifuged at 12000 rpm for 10 min in 4°C, and the supernatant was discarded to obtain bacterial precipitation. A Power Soil DNA Isolation kit was used to extract the total bacterial DNA, and the primer sequence 27F (AGRGTTTGATYNTGGCTCAG) and 1492R (TASGGHTACCTTGTTASGACTT) were used to amplify the full-length 16S rRNA gene. PCR reaction conditions were 95°C for 5 min, 95°C for 30 s, 50°C for 30 s, and 72°C for 1 min, with a total of 30 cycles and a reaction system of 10 μL. After the construction of magnetic beads (MagicPure Size Selection DNA Beads) purification, preparing library, and target gene sequencing, in turn, original reads were corrected to obtain Circular Consensus Sequencing (CCS) (SMRT Link, Version 8.0). The Lima software (version 1.7.0) was used to identify the CCS sequences of different samples by a barcode sequence and a remove chimera (UCHIME, version 8.1), resulting in high-quality CCS sequences. The sequences were clustered at a similarity level of 97% (USEARCH, version 10.0) (Quast et al., 2013) and the OTU (Operational Taxonomic Units) were filtered with 0.005% of all numbers sequenced as the threshold (Kõljalg et al., 2013). Bacteria chose 16S: Silva databases (Release 132)1 (Wang et al., 2007) and RDP Classifier (version 2.2)2 (Larkin et al., 2007), and species were annotated with a confidence threshold of 0.8. Finally, the Alpha index analysis software Mothur (Version V.1.30)3 was used to analyze the microbial diversity and richness of different habitat samples.
Statistical Analysis
All variables were tested by the Shapiro–Wilk test for normality and then analyzed for homogeneity of variance. We used a simple correlation coefficient to analyze the correlation between continuous variables of physicochemical factors. DNH was not considered because there was no logical relationship between variables. Results showed that the variables of physical and chemical factors were not normally distributed and had different variances, and they were not independent of each other. Therefore, it was more reasonable to use a Spearman correlation and multiple linear regression to analyze the correlation between mosquito density and physical and chemical factors (Nikookar et al., 2017). Canonical correspondence analysis (CCA) has been performed to further explore the associations between larval densities and physicochemical factors (Bashar et al., 2016). One-way ANOVA was used to explain whether there were differences in bacterial diversity and richness among different habitat types. Pearson’s correlation model was used to analyze the effects of bacterial diversity and richness on larval densities in breeding sites.
All statistical analyzes were performed using Microsoft Excel 2019 for Windows and SPSS 16 (SPSS Inc., Chicago, IL, United States 2007), with a significance level of 0.05.
Results
Habitat Types
A total of 62 representative potential habitats were investigated. Out of these, 46 bred larvae. We classified these 46 breeding sites into different habitat types according to the situation on the spot, such as small puddles (6), small water containers (12), paddy fields (7), large water containers (9), irrigation channels (6), and drainage ditches (6). Figures 1, 2 show the locations and habitat types.
Larval Density and Species Distribution
A total of 3,291 larvae were collected in all breeding sites, and a total of four genera and six species were identified, including Cx. p. pallens (2026/61.56%), Ae. albopictus (732/22.24%), An. sinensis (147/4.47%), Cx. tritaeniorhynchus (319/9.69%), Cx. bitaeniorhynchus (13/0.40%), and Ma. uniformis (54/1.64%). The larvae densities of each habitat type were: small puddles (3.38), small water containers (4.55), paddy fields (2.31), large water containers (4.42), irrigation channels (2.96), and drainage ditches (2.66), respectively (see Table 1). In terms of the distribution of mosquito species, Cx. p. pallens was the highest (C = 98.70%), which could be found in all habitat types. It was followed by Ae. albopictus (C = 67.83%), Cx. tritaeniorhynchus (C = 55.65%), An. sinensis (C = 25.65%), and Ma. uniformis (C = 10.43%). The distribution of Cx. bitaeniorhynchus was the smallest (C = 4.35%). An. sinensis was only present in paddy fields and irrigation channels, Cx. bitaeniorhynchus was found only in large water containers, and Ma. uniformis was found only in irrigation channels.
The Physical and Chemical Parameters and Bacterial Diversity of Breeding Sites
Table 2 shows the means and standard deviations of various variables for each habitat. Figure 3 shows the mean and standard error of the OTU, bacterial diversity index (Shannon index), and the richness index (Chao 1) of breeding sites. The Shannon diversity index dilution curve (Figure 4A) showed each sample’s microbial diversity at different sequencing quantities. The curve was flat, indicating that the sequencing quantity was large enough, and the results of OTU clustering accurately reflected the samples’ real situation. The breeding sites with the highest Shannon index were drainage ditches, and the lowest was small water containers. Figure 4B shows the top-ten species at the richness level in each habitat. The bacteria with the highest richness in small puddles was Hydrogenophaga. The bacteria with the highest richness in small water containers, paddy fields, and irrigation channels were all Curvibacter. Polynucleobacter microbes were most abundant in large water containers. Results of one-way ANOVA showed that the Shannon index (df = 5, F = 5.841, p = 0.001) and Chao 1 (df = 5, F = 10.859, p < 0.01) were different between habitat types.
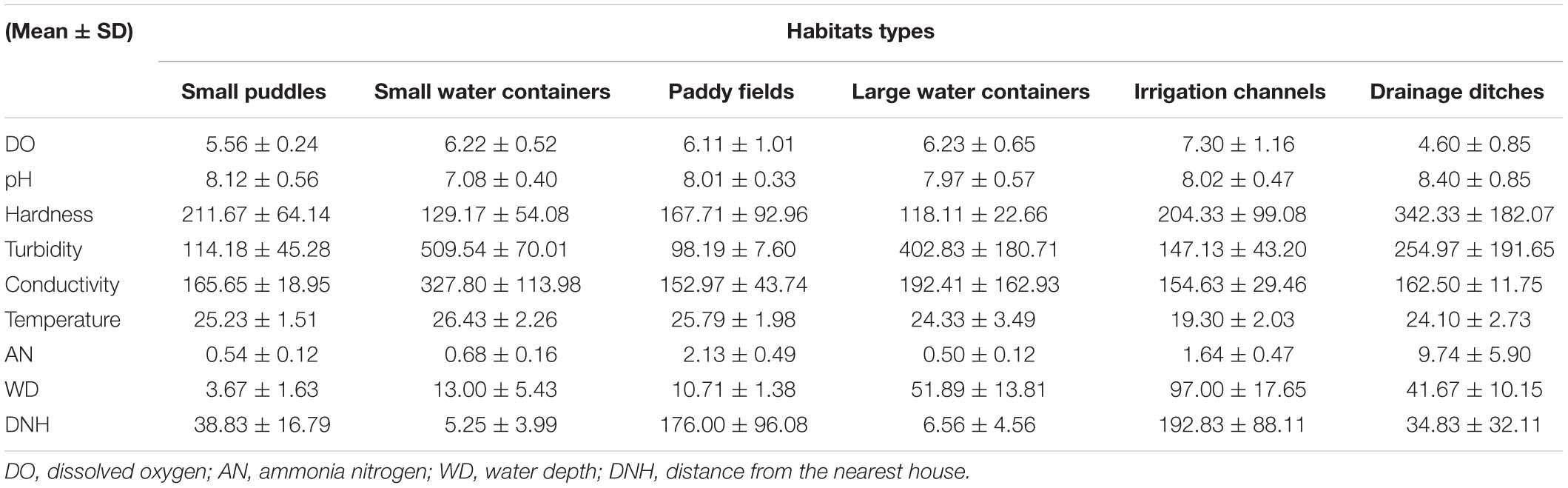
Table 2. Means and standard deviations of physicochemical characteristics in different larval habitats.
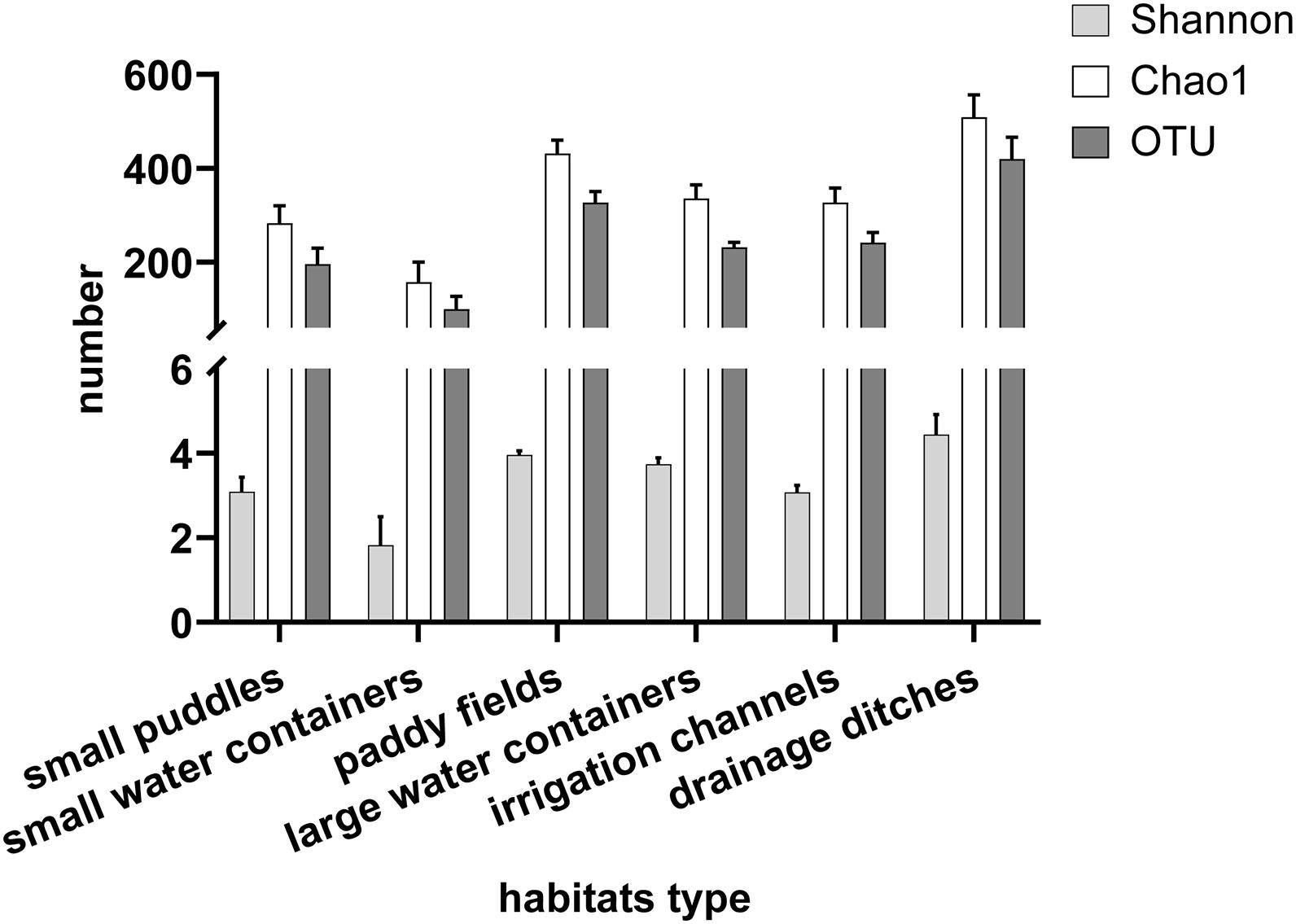
Figure 3. Mean and standard error of the OTU, Shannon Index, and Chao 1 of the 6 habitat types. Shannon index is the bacterial diversity index used to measure species diversity, which was affected by species richness and community evenness in the sample. Chao 1 is the richness index, representing the number of species.
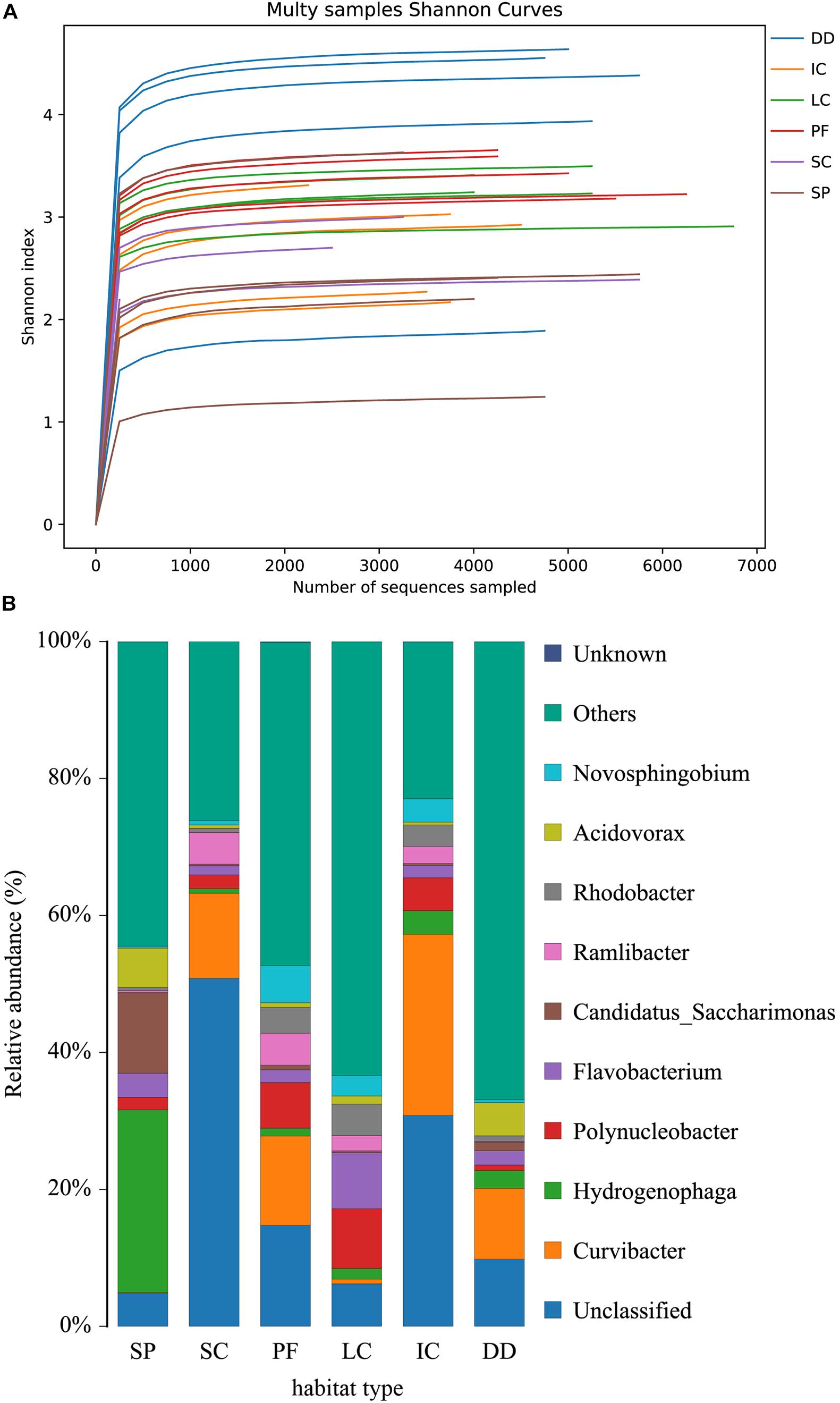
Figure 4. Alpha diversity analysis: (A) Shannon diversity index dilution curve. It shows the microbial diversity of each sample at different sequencing quantities. The curve is flat, indicating that the sequencing quantity is large enough, and the results of Shannon index accurately reflected the real situation of the samples. (B) The top-ten species at the richness level in each habitat. DD, drainage ditches; IC, irrigation channels; LC, large water containers; PF, paddy fields; SC, small water containers; SP, small puddles.
Correlations Between Variables
Table 3 shows the correlation coefficients between the variables of each physicochemical factor. Among the 36 correlation coefficients, 7 (19.44%) were statistically significant, indicating a non-random correlation between the variables with physicochemical properties. pH was negatively correlated with turbidity (r = − 0.442, p = 0.002) and conductivity (r = − 0.502, p < 0.01). A significant negative and positive correlation existed between hardness with turbidity (r = − 0.325, p = 0.028) and with AN (r = 0.339, p = 0.021), respectively. Turbidity was positively correlated with conductivity (r = 0.357, p = 0.015) and negatively correlated with AN (r = − 0.375, p = 0.01). Temperature was positively correlated with WD (r = − 0.407, p = 0.005).
Relationship Between Larval Density and Variables
Spearman correlation analysis (Table 4) shows the correlations between larval densities and physical and chemical parameters. In the multiple linear regression model analysis, the larvae densities of various types were taken as dependent variables, and then 9 physical and chemical factors, as independent variables respectively, were incorporated into the model. The variables were fitted into the regression equation according to the stepwise selection method, and the independent variables with a large contribution to the regression equation were reserved (p < 0.05) were retained. According to the results of multiple linear regression analyzes (Table 5), Cx. p. pallens (F = 18.30, p < 0.001) required low DNH, whereas An. sinensis (F = 72.388, p < 0.001) required high DNH. Ae. albopictus (F = 16.239, p < 0.001) required less DNH, AN, and pH, but a higher temperature. These variables explained the density of Ae. albopictus more strongly than other variables. Cx. tritaeniorhynchus (F = 7.929, p = 0.001) required high AN and WD. Compared to other variables, high WD and DNH and low pH contributed the most to Ma. uniformis (F = 33.498, p <0.001). Our results showed that Cx. bitaeniorhynchus did not fit the regression equation as the P value was greater than 0.05.
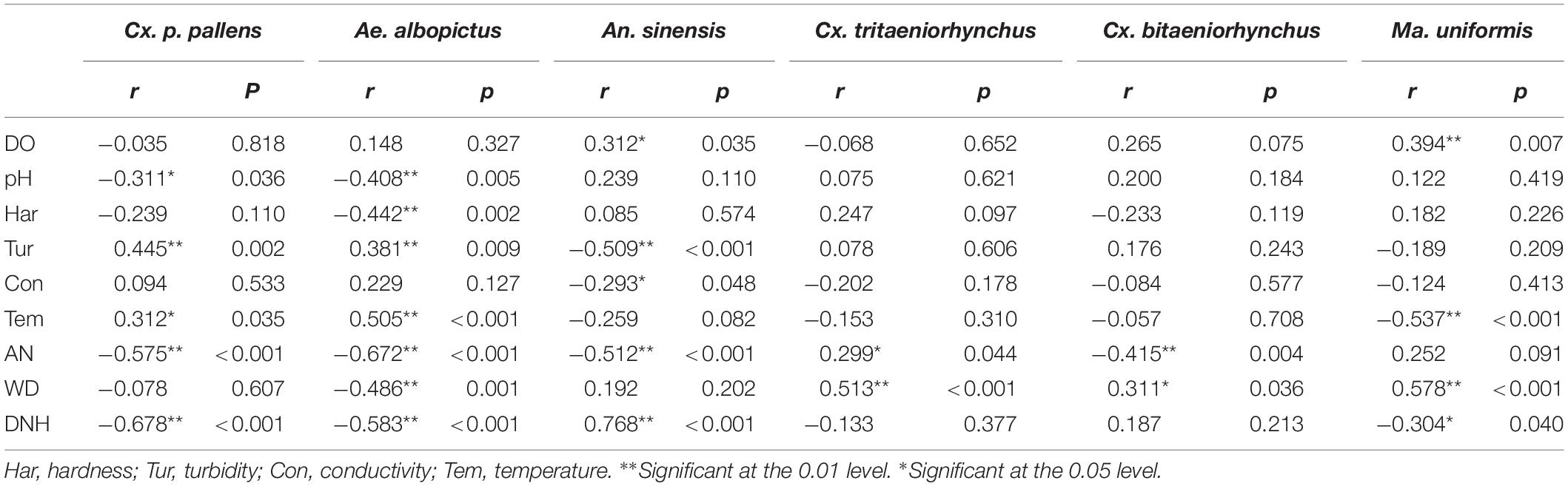
Table 4. Spearman correlation coefficient between larval density and physicochemical characteristics of larval habitats.

Table 5. The multiple regression equation shows the contribution rate of each variable to mosquito density.
Table 6 showed that the density of Cx. p. pallens negatively correlated with the Shannon index (r = − 0.430, p = 0.018) and the richness index (r = − 0.644, p < 0.001). The density of Ae. albopictus negatively correlated with the bacterial diversity index (r = − 0.411, p = 0.024) and the richness index (r = − 0.572, p = 0.001). Cx. tritaeniorhynchus positively correlated with the Shannon index (r = 0.493, p = 0.006), while other mosquito species did not significantly correlate with bacterial diversity. Figure 5 visually showed the correlation between larval density and variables based on the Canonical correspondence analysis (CCA).

Table 6. Pearson correlation coefficient between larval density and bacterial diversity of larval habitats.
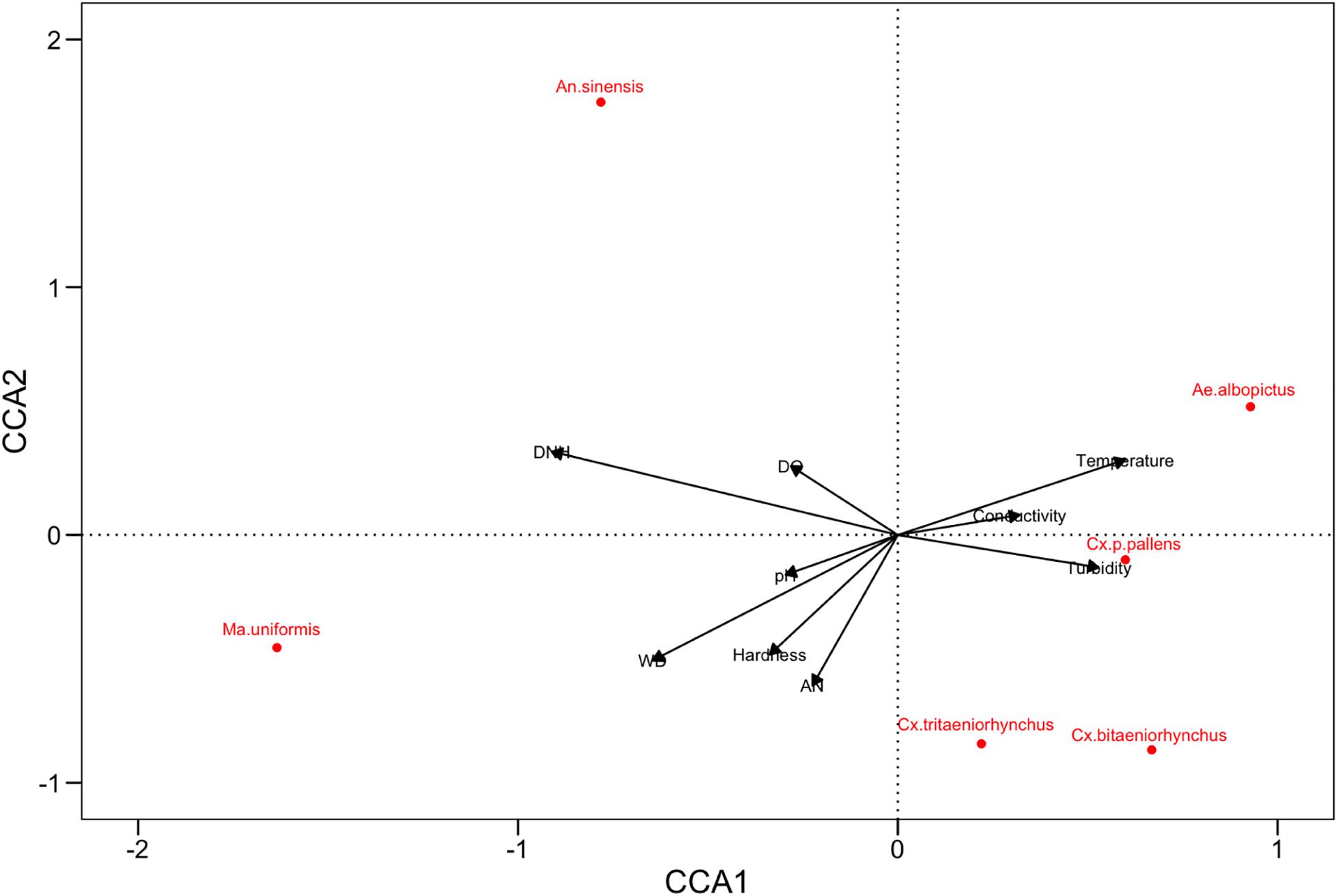
Figure 5. Biplot representing the results of CCA, the relationship between the larval density (red labels) and the physicochemical variables (black arrows). The constrained inertia explained by the CCA is 31.18%, and the proportion explained by CCA1 axis and CCA2 axis was 35.35% and 10.27%, respectively.
Discussion
Mosquito problems have been categorized as medical care problems and environmental issues with the progress of human society, urban sprawl, and intensification of environmental changes (Ahmed et al., 2007). Sole dependence on insecticides can no longer completely eliminate the hazards that mosquitoes had brought to us. Luckily, LSM is environment friendly and does not lead governments and inhabitants to too much economic burden and time cost. These could be rapidly realized utilizing eliminating breeding sites or using chemical or biological methods. However, developing efficient and safe strategies required a scientific understanding of the control area’s larval density and environmental factors.
In recent years, due to the vigorous development in modern agriculture, the mode of agriculture in Jiaxiang County has changed. For example, paddy fields have been replaced by citrus orchards, which directly resulted in the change of some breeding sites’ environment. We suspect that this may be a factor that led to the failure to obtain mosquitoes such as An. dirus, which prefer open water (Service, 1991; Norris, 2004). In addition, agricultural activities such as the use of pesticides and fertilizers changed the richness of microbiota and algae in the water, which are the main food sources for larvae (Noori et al., 2016). This disturbed selecting suitable sites for oviposition and incubation by specific mosquitoes.
Most of the microbiota in the breeding sites form parasitic or symbiotic relationships with the larvae, and their impact on the larvae can be classified in the following three aspects: regulating the internal and external environment and immune response of the mosquito host, promoting the developments and growth of larvae, and causing pathogenicity to larvae such as infection of the filamentous fungi Beauveria bassiana and Bacillus thuringiensis (Bti). In addition, studies have shown that bacterial diversity might be related to larval density (Dida et al., 2018). Therefore, we used molecular biology methods, namely the 16S rRNA sequencing technology, to sequence and analyze the bacterial diversity and richness of breeding sites. Results showed that different habitats have large differences in bacterial diversity and richness, even in the same habitat, because bacterial diversity and richness in water are always dynamic and affected by their environment, such as light, temperature, vegetation cover, and human activities. In general, Cx. p. pallens and Ae. albopictus, the dominant species in Jiaxiang County, both showed lower tolerance to bacteria, which also seems to explain the lower diversity of intestinal symbiotic bacteria in these two species (Coon et al., 2016). There was no statistical correlation between the density of other species and the bacterial diversity, which we hypothesized might be due to the small number of samples we tested from water bodies or the large variation in the diversity index of different habitat types. Cx. p. pallens has a wide range of fitness for physical and chemical properties such as pH, hardness, turbidity, and AN, which is consistent with the results of Nikookar et al., (Nikookar et al., 2017). In other words, Cx. p. pallens have no strict requirements regarding the environment they breed in, and they can even breed in less hospitable environments. This seems to explain the dominance of Cx. p. pallens in the less sanitary areas of northern China. In addition, Gabriel et al., have shown that Culex (especially Cx. p. pallens and Cx. tritaeniorhynchus) are widely distributed in a variety of water bodies, providing intuitive evidence that they are highly adaptable to different habitats. Urbanization, human activities, and other factors have affected the breeding environment’s physicochemical properties and water bodies (Ndaruga et al., 2004). Despite this, the density of Culex in Jiaxiang County remains high. Previous reports and our study give a reasonable explanation for this phenomenon.
Ae. albopictus is the main vector of the dengue virus. Our study showed that Ae. albopictus density was negatively correlated with WD. This may be because they prefer to lay eggs in small water containers such as junked tires, plastic bottles, and surface-gathered water and have strong adaptability to low water levels (Li et al., 2014; Petric et al., 2014). There is a negative correlation between Ae. albopictus and DNH. In other words, the farther the breeding site is from the house, the lower the density of Ae. albopictus, and vice versa. This characteristic is observed in all species except An. sinensis. WHO has reported that Ae. albopictus prefers to lay eggs in small containers having stagnant water in people’s yards or houses (World Health Organization, 1983). Based on such characteristics, dumping stagnant water to reduce the incidence of dengue fever by reducing the BI could be an effective and environment-friendly method. Moreover, Ae. albopictus, just like Cx. p. pallens showed a positive correlation with turbidity and temperature, while a negative correlation with pH and AN. They showed a wide range of adaptation to the abovementioned physical and chemical properties. As Thavara and Bashar reported, Ae. albopictus preferred to inhabit human-made habitats inside and outside houses, where the habitats’ physical and chemical properties were more fluctuating (Thavara et al., 2004).
The increase in salt compounds and turbidity had a significant effect on the density of An. sinensis, and they showed low tolerance to AN levels. Kenawa found a strong positive correlation between its density and dissolved oxygen (El-Ghani et al., 2012), which is consistent with our results (r = 0.312, p = 0.035). In comparison with Aedes and Culex, Anopheles mosquitoes were more sensitive to other physical and chemical changes in water (El-Ghani et al., 2012). Minakawa et al. (2005) suggested that Anopheles preferred sunny open waters such as lotus ponds, paddy fields, and irrigation channels where breeding sites are far from houses. Our results also confirm that Anopheles mosquito density is positively correlated with DNH. We suspect that this may be related to the strong long-range flight ability of An. sinensis. The rice-growing areas and lotus ponds in Jiaxiang County are widely distributed, which provides the conditions for the breeding of An. sinensis in the area. Applying agricultural insecticides seem to be the most effective way to control An. sinensis. However, the epidemic prevention department should instruct farmers in the region to spray insecticides scientifically and rationally to slow the development of mosquito resistance. On the basis of the existing rice-planting pattern in Jiaxiang County, humid irrigation replacing the current irrigation regime can effectively eliminate the breeding sites for irrigation channels, which is also an effective method to control An. Sinensis (Tusting et al., 2013). Minakawa found that Anopheles in western Kenya were not found in sunny open water and preferred lakeside swamps. This difference suggests that even mosquitoes from the same genus may show differences in habitat selection. The primary reason for this phenomenon was geographical isolation and ecological differences (Varela and Yadav, 2020), suggesting that we should first understand their habits and adapt to local conditions to prevent and control larvae.
Culex tritaeniorhynchus is the primary medium carrying the Japanese encephalitis virus and the dominant mosquito species in Shandong Province, especially near the South-to-North Water Diversion Project. In terms of distribution alone, they are similar to Cx. p. pallens, as both of them, were found in all habitat types. However, the density of Cx. tritaeniorhynchus that we collected was significantly lower than that of Cx. p. pallens. They can survive in deep water with low oxygen content, so the density and WD showed a strong positive correlation. From the results of the regression equation, it is evident that AN and WD had the highest density contribution to Cx. tritaeniorhynchus, which is the same as the research results of Wang et al., (Wang et al., 2020). Cx. bitaeniorhynchus and Ma. uniformis showed a low distribution, and they were only found in large water containers and irrigation channels, respectively. Combined with previous surveys, we found that the density of these two species had been low in Jiaxiang County, which did not bring trouble or danger to humans. Therefore, this is not discussed in this article.
Although the CCA results were consistent with the Spearman, it may be able to predict larval density in a much more intuitive way. We can intuitively receive such results from the biplot: the larval density of Ae. albopictus and Cx. p. pallens can be better predicted by low WD, DNH, and high temperature. Alternatively, high DNH and DO may be more useful in predicting larval density of An. sinensis than other variables. The larval density of Ma. uniformis can be affected by high WD and low temperature, while the density of Cx. bitaeniorhynchus and Cx. tritaeniorhynchus can hardly be predicted from the results of CCA. The CCA biplot indicated that Anopheles, Aedes, Culex, and Mansonia were plotted in different axis which may explain different requirement for different variables (Bashar et al., 2016).
There are several aspects that need to be improved in this study. We did not conduct a survey of algae, protists, and rotifers in breeding grounds. However, protists are the main source of nutrition for larvae (Blaustein and Chase, 2007; Addicott, 2008), and Coelastrum, Scenedesmus, Selenastrum, and Tetrallantos that are algae for Aedes and Culex are difficult to digest, which can reduce the survival rate of larvae (Howland, 1930). Microbial diversity and physicochemical parameters of water bodies change dynamically, and a large number of survey results are needed to develop a scientific and rigorous model to predict larval density. Thus, our sample number is far from enough.
Conclusion
This research showed that the physicochemical parameters and bacterial diversity of different habitat types were significantly different. Even for the same habitat type, the physicochemical parameters varied greatly due to different environments. The variable that contributed the most to the density of Cx. p. pallens was DNH. Parameters such as DNH, AN, pH, and temperature contributed significantly to the density of Ae. albopictus. These two kinds of mosquitoes are distributed in almost all kinds of habitats in Jiaxiang County, and their density is also the highest. This suggests that we should be vigilant against the control of mosquito-borne viruses in this area, especially the dengue virus carried by Ae. albopictus.
Data Availability Statement
The original contributions presented in the study are included in the article/supplementary material, further inquiries can be directed to the corresponding author.
Author Contributions
MQG and HYW designed the study. HFW and HWW participated in field surveys with support from PC. HYW extracted the data and carried out the data analysis with support from YW. HYW and YW wrote and reviewed the article. All authors read and approved the final manuscript.
Funding
This work was supported by grants from the National Natural Science Foundation of China [81871685 (MQG)] and Shandong Provincial Natural Science Foundation [ZR2020MC048 (PC) and ZR2020KH001 (HML)].
Conflict of Interest
The authors declare that the research was conducted in the absence of any commercial or financial relationships that could be construed as a potential conflict of interest.
Acknowledgments
We would like to thank the population of BMKCloud for their collaboration during the analysis of bacterial diversity. We would also like to thank local villagers to help us searching for breeding sites.
Footnotes
References
Addicott, J. F. (2008). Predation and prey community structure: an experimental study of the E. Communication 55:41. doi: 10.2307/1935141
Ahmed, T. U., Rahman, K. B. M. S., Shamsuzzaman, M., Samajpati, S., Sultana, S., Hossain, M. I., et al. (2007). Some socio-demographic factors related to dengue outbreak in Dhaka City. Bangladesh Bangladesh. J. Zool. 35, 213–222. doi: 10.1002/anie.200461295
Aryaprema, V. S., and Xue, R. D. (2019). Breteau index as a promising early warning signal for dengue fever outbreaks in the colombo district. Sri Lanka. Acta Trop. 199:105155. doi: 10.1016/j.actatropica.2019.105155
Bashar, K., Sayfur Rahman, M. D., Nodi, I. J., and Howlader, A. J. (2016). Species composition and habitat characterization of mosquito (Diptera: Culicidae) larvae in semi-urban areas of Dhaka. Bangladesh. Pathogens Global Health 110:48. doi: 10.1080/20477724.2016.1179862
Bentley, M. D., and Day, J. F. (1989). Chemical ecology and behavioral aspects of mosquito oviposition. Annu. Rev. Entomol. 34, 401–421. doi: 10.1146/annurev.en.34.010189.002153
Blaustein, L., and Chase, J. M. (2007). Interactions between mosquito larvae and species that share the same trophic level. Annu. Rev. Entomol. 52, 489–507. doi: 10.1146/annurev.ento.52.110405.091431
Coon, K. L., Brown, M. R., and Strand, M. R. (2016). Mosquitoes host communities of bacteria that are essential for development but vary greatly between local habitats. Mol. Ecol. 25, 5806–5826. doi: 10.1111/mec.13877
Dada, N., Sheth, M., Liebman, K., Pinto, J., and Lenhart, A. (2018). Whole metagenome sequencing reveals links between mosquito microbiota and insecticide resistance in malaria vectors. Sci. Rep. 8:2084. doi: 10.1038/s41598-018-20367-4
Dida, G. O., Anyona, D. N., Abuom, P. O., Akoko, D., Adoka, S. O., Matano, A. S., et al. (2018). Spatial distribution and habitat characterization of mosquito species during the dry season along the Mara River and its tributaries, in Kenya and Tanzania. Infect Dis. Poverty 7, 2–18. doi: 10.1186/s40249-017-0385-0
El-Ghani, M. A., Shehata, M. N., Mobarak, A., and Bakr, R. (2012). Factors affecting the diversity and distribution of synanthropic vegetation in urban habitats of the Nile Delta. Egypt Rendiconti Lincei 23, 327–337. doi: 10.1007/s12210-012-0179-z
Ferreira-de-Lima, V. H., and Lima-Camara, T. N. (2018). Natural vertical transmission of dengue virus in Aedes aegypti and Aedes albopictus: a systematic review. Parasit Vectors 11:77. doi: 10.1186/s13071-018-2643-9
Guo, C., Zhou, Z., Wen, Z., Liu, Y., Zeng, C., Xiao, D., et al. (2017). Global epidemiology of dengue outbreaks in 1990-2015: a systematic review and meta-analysis. Front. Cell Infect. Microbiol. 7:317. doi: 10.3389/fcimb.2017.00317
Howland, L. J. (1930). The nutrition of mosquito larvae, with special reference to their algal food. B. Entomol. Res. 21, 431–439. doi: 10.1017/S0007485300024779
Jacob, B. G., Arheart, K. L., Griffith, D. A., Mbogo, C. M., Githeko, A. K., Regens, J. L., et al. (2005). Evaluation of environmental data for identification of anopheles (Diptera: Culicidae) aquatic larval habitats in kisumu and malindi. Kenya. J. Med. Entomol. 22, 751–755. doi: 10.1093/jmedent/42.5.751
Jiang, S., Wang, Z., Guo, X., Zhang, Y., Li, C., Dong, Y., et al. (2014). Infection and dissemination of west nile virus in china by the potential vector. culex pipiens pallens, J. Vector Ecol. 39, 78–82. doi: 10.1111/j.1948-7134.2014.12073.x
Juliano, S. A., Lounibos, L. P., and O’Meara, G. F. (2004). A field test for competitive effects of Aedes albopictus on A. aegypti in South Florida: differences between sites of coexistence and exclusion? Oecologia 139, 583–593. doi: 10.1007/s00442-004-1532-4
Killeen, G. F., McKenzie, F. E., Foy, B. D., Bøgh, C., and Beier, J. C. (2001). The availability of potential hosts as a determinant of feeding behaviours and malaria transmission by African mosquito populations. Trans. R. Soc. Trop. Med. Hyg. 95, 469–476. doi: 10.1016/s0035-9203(01)90005-7
Kõljalg, U., Nilsson, R. H., Abarenkov, K., Tedersoo, L., Taylor, A. F., Bahram, M., et al. (2013). Towards a unified paradigm for sequence-based identification of fungi. Mol. Ecol. 22, 5271–5277. doi: 10.1111/mec.12481
Larkin, M. A., Blackshields, G., Brown, N. P., Chenna, R., McGettigan, P. A., McWilliam, H., et al. (2007). Clustal W and Clustal X version 2.0. Bioinformatics 23, 2947–2948. doi: 10.1093/bioinformatics/btm404
Li, Y., Kamara, F., Zhou, G., Puthiyakunnon, S., Li, C., Liu, Y., et al. (2014). Urbanization increases Aedes albopictus larval habitats and accelerates mosquito development and survivorship. PLoS Negl. TropDis. 8:e3301. doi: 10.1371/journal.pntd.0003301
Lin, Y. S., Richard, M., Lehtinen, and Kam, Y. C. (2008). Time- and context-dependent oviposition site selection of a phytotelm-breeding frog in relation to habitat characteristics and conspecific cues. Herpetologica 64, 413–421. doi: 10.2307/27669731
Liu, H., Liu, L., Cheng, P., Yang, L., Chen, J., Lu, Y., et al. (2020). Bionomics and insecticide resistance of Aedes albopictus in Shandong, a high latitude and high-risk dengue transmission area in China. Parasit Vectors 13:11. doi: 10.1186/s13071-020-3880-2
Liu, H., Xie, L., Cheng, P., Xu, J., Huang, X., Wang, H., et al. (2019). Trends in insecticide resistance in Culex pipiens pallens over 20 years in Shandong, China. Parasit Vectors 12:167. doi: 10.1186/s13071-019-3416-9
Liu, H. M., Yang, P. P., Cheng, P., Wang, H. F., Liu, L. J., Huang, X., et al. (2015). Resistance Level of mosquito species (Diptera: Culicidae) from shandong province, China. Int. J. Insect Sci. 7, 47–52. doi: 10.4137/IJIS.S24232
Minakawa, N., Sonye, G., and Yan, G. (2005). Relationships between occurrence of Anopheles gambiae s.l. (Diptera: Culicidae) and size and stability of larval habitats’. J. Med. Entomol. 42, 295–300. doi: 10.1093/jmedent/42.3.295
Molineaux, L. (1997). Malaria and mortality: some epidemiological considerations. Ann. Trop. Med. Parasitol. 91, 811–825. doi: 10.1080/00034989760572
Mukhopadhyay, P., and Chatterjee, S. (2016). Characterization and control of symbiotic Bacillus cereus isolated from the mid gut of Anopheles subpictus Grassi. J. Parasit Dis. 40, 1414–1421. doi: 10.1007/s12639-015-0704-y
Nambunga, I. H., Ngowo, H. S., Mapua, S. A., Hape, E. E., Msugupakulya, B. J., Msaky, D. S., et al. (2020). Aquatic habitats of the malaria vector Anopheles funestus in rural south-eastern Tanzania. Malar J. 19:219. doi: 10.1186/s12936-020-03295-5
Ndaruga, A. M., George, G., Ndiritu, Nathan, N., Gichuki, and Wamicha, W. N. (2004). Impact of water quality on macroinvertebrate assemblages along a tropical stream in Kenya. African J. Ecol. 42, 208–216. doi: 10.1111/j.1365-2028.2004.00516.x
Nikookar, S. H., Fazeli-Dinan, M., Azari-Hamidian, S., Mousavinasab, S. N., Aarabi, M., Ziapour, S. P., et al. (2017). Correlation between mosquito larval density and their habitat physicochemical characteristics in mazandaran province, northern Iran. PLoS Negl. Trop. Dis. 11:e0005835. doi: 10.1371/journal.pntd.0005835
Noori, N., Lockaby, B. G., and Kalin, L. (2016). Larval development of culex quinquefasciatus in water with low to moderate. J. Vector Ecol. 40, 208–220. doi: 10.1111/jvec.12156
Norris, D. (2004). Mosquito-borne diseases as a consequence of land use change. Ecohealth 1, 19–24. doi: 10.1007/s10393-004-0008-7
Petric, D., Bellini, R., Scholte, E. J., Rakotoarivony, L. M., and Schaffner, F. (2014). Monitoring population and environmental parameters of invasive mosquito species in Europe. Parasit Vectors 7:187. doi: 10.1186/1756-3305-7-187
Qing, W., Zhijing, X., Guangfu, Y., Fengxia, M., Qiyong, L., Zhong, Z., et al. (2020). Variation in the microbiota across different developmental stages of Aedes albopictus is affected by ampicillin exposure. Microbiologyopen 9, 1162–1174. doi: 10.1002/mbo3.1026
Quast, C., Pruesse, E., Yilmaz, P., Gerken, J., Schweer, T., Yarza, P., et al. (2013). The SILVA ribosomal RNA gene database project: improved data processing and web-based tools. Nucleic Acids Res. 41, D590–D596. doi: 10.1093/nar/gks1219
Service, M. W. (1991). Agricultural development and arthropod-borne diseases: a review. Rev. Saú Pública 25, 165–178. doi: 10.1590/s0034-89101991000300002
Shi, Q., Song, X., Lv, Y., Huang, X., Kou, J., Wang, H. W., et al. (2019). Potential risks associated with Japanese encephalitis prevalence in shandong province. China. Vector Borne Zoonotic Dis. 19, 640–645. doi: 10.1089/vbz.2018.2416
Thavara, U., Tawatsin, A., and Chompoosri, J. (2004). Evaluation of attractants and egg-laying substrate preference for oviposition by Aedes albopictus (Diptera: Culicidae). J. Vector Ecol. 29, 66–72.
Tusting, L. S., Thwing, J., Sinclair, D., Fillinger, U., Gimnig, J., Bonner, K., et al. (2013). Mosquito larval source management for controlling malaria. Cochrane Database Syst. Rev. 8:CD008923. doi: 10.1002/14651858.CD008923.pub2
Varela, J. N., and Yadav, V. G. (2020). A Pichia biosensor for high-throughput analyses of compounds that can influence mosquito behavior. Microbiologyopen 10:e1139. doi: 10.1002/mbo3.1139
Villarreal-Treviño, C. R., Penilla-Navarro, R. P., Vázquez-Martínez, M. G., Moo-Llanes, D. A., Moo-Llanes, D. A., Ríos-Delgado, J. C., et al. (2015). Larval habitat characterization of Anopheles darlingi from its northernmost geographical distribution in Chiapas, Mexico. Malar J. 14:517. doi: 10.1186/s12936-015-1037-0
Wang, Q., Garrity, G. M., Tiedje, J. M., and Cole, J. R. (2007). Naive Bayesian classifier for rapid assignment of rRNA sequences into the new bacterial taxonomy. Appl. Environ. Microbiol. 73, 5261–5267. doi: 10.1128/AEM.00062-07
Wang, Y., Cheng, P., Jiao, B., Song, X., Wang, H., Wang, H., et al. (2020). Investigation of mosquito larval habitats and insecticide resistance in an area with a high incidence of mosquito-borne diseases in Jining. shandong province. PLoS One 15:e0229764. doi: 10.1371/journal.pone.0229764
Wilkerson, R. C., Strickman, D., and Litwak, T. R. (1990). Illustrated key to the female anopheline mosquitoes of Central America and Mexico. J. Am. Mosq. Control Assoc. 6, 7–34.
Keywords: full-length sequencing, habitat types, density, mosquito, bacterial diversity
Citation: Wang H, Wang Y, Cheng P, Wang H, Wang H, Liu H, Zhang C and Gong M (2021) The Larval Density of Mosquitos (Diptera: Culicidae) in Jiaxiang County, Shandong Province, China: Influence of Bacterial Diversity, Richness, and Physicochemical Factors. Front. Ecol. Evol. 9:616769. doi: 10.3389/fevo.2021.616769
Received: 09 November 2020; Accepted: 02 February 2021;
Published: 26 February 2021.
Edited by:
Mauro Marrelli, University of São Paulo, BrazilReviewed by:
Hassan Vatandoost, Tehran University of Medical Sciences, IranAntônio Ralph Medeiros De Sousa, University of São Paulo, Brazil
Copyright © 2021 Wang, Wang, Cheng, Wang, Wang, Liu, Zhang and Gong. This is an open-access article distributed under the terms of the Creative Commons Attribution License (CC BY). The use, distribution or reproduction in other forums is permitted, provided the original author(s) and the copyright owner(s) are credited and that the original publication in this journal is cited, in accordance with accepted academic practice. No use, distribution or reproduction is permitted which does not comply with these terms.
*Correspondence: Maoqing Gong, bXFnb25nQHNkZm11LmVkdS5jbg==