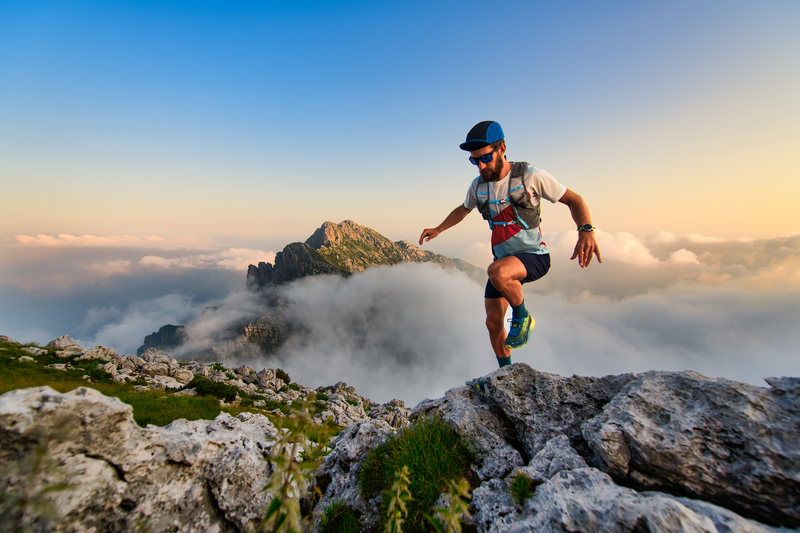
95% of researchers rate our articles as excellent or good
Learn more about the work of our research integrity team to safeguard the quality of each article we publish.
Find out more
ORIGINAL RESEARCH article
Front. Ecol. Evol. , 26 February 2021
Sec. Behavioral and Evolutionary Ecology
Volume 9 - 2021 | https://doi.org/10.3389/fevo.2021.615899
This article is part of the Research Topic Cognition and Adaptation to Urban Environments View all 6 articles
Enhanced cognitive ability has been shown to impart fitness advantages to some species by facilitating establishment in new environments. However, the cause of such enhancement remains enigmatic. Enhanced cognitive ability may be an adaptation occurring during the establishment process in response to new environments or, alternatively, such ‘enhancement’ may merely reflect a species’ characteristic. Based on previous findings that have shown ‘enhanced’ cognitive ability (i.e., higher success rate in solving novel food-extraction problems or, ‘innovation’) in Eastern gray squirrels (Sciurus carolinensis), a successful mammalian invader and urban dweller, we used an intraspecific comparative paradigm to examine the cause of their ‘enhanced’ cognitive ability. We conducted a field study to compare cognitive performance of free-ranging squirrels residing in rural and urban habitats in native (United States) and non-native environments (United Kingdom). By using established tasks, we examined squirrels’ performance in easy and difficult, novel food-extraction problems (innovation), a motor memory recall test of the difficult problem, and a spatial learning task. We found that the four groups of squirrels showed comparable performance in most measures. However, we also found that the native urban squirrels showed: (1) higher success rate on the first visit for the difficult problem than the non-native urban squirrels; (2) some evidence for higher recall latency for the difficult problem after an extended period than the non-native rural squirrels; and (3) learning when encountering the same difficult problem. These results suggest that the previously reported ‘enhanced’ performance is likely to be a general characteristic and thus, a pre-adaptive phenotypic trait that brings fitness advantages to this species in a new environment. Despite this, some cognitive abilities in gray squirrels such as solving novel problems has undergone mild variation during the adaptive process in new environments.
Enhanced cognitive ability has been shown to advance fitness measures in some contexts (Sol and Lefebvre, 2000; Sol et al., 2002, 2008; Nicolakakis et al., 2003; Keagy et al., 2009; Amiel et al., 2011; Cole et al., 2012; Cauchard et al., 2013; Webb et al., 2014; Preiszner et al., 2017). Such enhancement may be reflected as flexible behavioral adjustments to demands and challenges in environments, which may facilitate a species’ ability to adapt to urban environments (Lowry et al., 2013; Mangalam and Singh, 2013) or successfully invade and establish in novel environments (Sol and Lefebvre, 2000; Sol et al., 2002, 2005, 2008; Amiel et al., 2011). For example, a higher frequency of innovative foraging (e.g., consumption of novel food or using novel foraging techniques) are seen in successful invasive species compared with those that are not successful in the same environment (Sol and Lefebvre, 2000; Sol et al., 2002). Other examples are individuals of some species that are more successful or faster in solving novel food-extraction problems, which have been reported in urban populations compared with their rural counterparts (e.g., Liker and Bókony, 2009; Papp et al., 2015; Audet et al., 2016; but also see Preiszner et al., 2017; Prasher et al., 2019).
Despite these examples, the possible causes of enhanced cognitive ability remain enigmatic. At one level, the core idea underlying some hypotheses is that regardless of variation in ability during establishment stages, any resulting enhancement may be driven by adaptation during establishment in a new environment (e.g., skill-pooled effect by Giraldeau, 1994). Alternatively, a non-mutually exclusive possibility is that ‘enhanced’ ability may be a species’ natural characteristic which has been shown in other traits such as competitive ability (the inherent superiority hypothesis by Elton, 1958).
Previously, we employed an inter-specific comparative paradigm to compare the performance of an established population of invasive Eastern gray squirrel (Sciurus carolinensis) with a closely-related, but native, Eurasian red squirrel (Sciurus vulgaris) in solving an easy and a difficult novel food-extraction problem (Chow et al., 2018). We found that compared with the red squirrels, the gray squirrels showed higher success rates in solving the easy problem in the first visit, and higher success rates in solving the difficult problem in the first and subsequent visits to the testing apparatus. These results raise the question as to whether the resulting ‘enhanced’ problem-solving ability in the established gray squirrel population is adaptative driven during the establishment process in the new environment, or is a species characteristic that was already present in native populations, thus representing ‘inherent superiority.’ Accordingly, our aim in this study was to determine which hypothesis best explains this enhancement.
Eastern gray squirrels (Sciurus carolinensis) thrive in urban environments (Jessen et al., 2018), expand populations throughout native environments in the United States (Koprowski et al., 2016) and show high success rates of establishment and invasion (80% success rate or 59 out of 74 introductions, see Bertolino, 2009) in non-native environments such as the United Kingdom, Ireland, Italy, and South Africa (Gonzales, 2005). Because the gray squirrel is considered one of the top one-hundred invasive species in the world (Lowe et al., 2004), EU law (see EU Regulation 1143/2014 on Invasive Alien Species) prohibits translocations to another country and thus, prevents a much preferable common garden approach for our investigation. We therefore used historical information of introduction events (e.g., Middleton, 1930, 1931) and studies from genetic analysis of the origins of this species (Signorile et al., 2016) to locate one of the founding populations residing in non-native rural and urban environments in the United Kingdom since the 19th century. We then compared cognitive performance of squirrels in these regions with that of squirrels in rural and urban environments in the eastern United States from which this introduced species originated. Such sampling efforts resulted in four squirrel groups (i.e., native rural, native urban, non-native rural, and non-native urban groups) to comprehensively assess the cause of any ‘enhanced’ cognitive ability.
To get an overview when testing the hypotheses, we not only examined the ability of solving novel problems but also tested other cognitive performances such as learning and memory. To do so, we used established cognitive tasks in wild gray squirrels and those that were housed in the laboratory. These tasks, employed in previous studies, have shown that gray squirrels rapidly adjust their behavior under recurring changes in a spatial and color discrimination-reversal learning task (Chow et al., 2015), learn the successful solution for a difficult novel food-extraction problem and remember the solutions 22 months after first learning it (Chow et al., 2017). Gray squirrels were also able to apply effective solutions when solving a similar but different problem (Chow et al., 2017), and to apply less preferred solutions when the preferred solution becomes ineffective (Chow et al., 2019). With these observations in mind, we predicted that if enhanced cognitive ability resulted from adaptation in the novel environments, then the native rural group would show a poorer performance than their urban counterparts as well as the non-native rural and urban groups. However, if such enhancement is a typical species characteristic of gray squirrels, then comparable task performances would be observed across the four groups regardless of habitat (rural vs. urban) or environments (native vs. non-native).
Thirty-seven wild gray squirrels from the native environments (United States) and 29 squirrels from the non-native environments (United Kingdom) participated in this study. Habitat characteristics were matched as closely as possible between continents. Following Møller (2010), urban habitats included infrastructures with continuous buildings that are interspersed by roads and parks, whereas rural habitats included intermittent houses and farms, farmland or forests. In all locations, there were different types of native and exotic trees such as pin oak (Quercus palustris) and red oak (Quercus rubra). Based on field surveys to determine the number of foraging squirrels during their active time, there were typically 2–5 squirrels per site observed foraging in the rural habitats and 7–12 squirrels in the urban habitats. Both types of habitats had predators such as hawks, and domestic cats and dogs. In all sites, direct and indirect food provisioning by humans were observed, suggesting that the squirrels had the opportunity to gain experience encountering novel objects (e.g., feeder) and exposure to humans. We checked that feeders were emptied and encouraged residents to not provide additional food for squirrels prior to and during data collection. Data for the two native groups were collected in two urban (Wilkes-Barre, PA, United States, 41.25° N, 75.88° W) and two rural habitats (Marlton, NJ, United States, 39.89° N, 74.92° W) between August and November, 2016 in the United States. Data for the two non-native groups were collected in one urban (St. John’s college, University of Cambridge, 52.21° N, 0.12° E) and three rural habitats (Madingley, Cambridgeshire, 52.22° N, 0.04° E) between November, 2016 and February, 2017 in the United Kingdom. We minimized resampling the same individual by choosing sites that were between 800 m to 1 km apart, typically preventing overlap in home ranges between sites (or >5 acres larger than its home range, Koprowski, 1994).
The use of live-trapping methods prior to the main experiment appeared to deter squirrels from approaching test apparatuses, when test apparatuses were presented within a short period after trapping and thus, would have affected participation in experiments (Chow et al., 2018). Accordingly, we identified individuals using ‘mark-recaptured’ method whereby their unique characteristics were identified from video footage (Sony HDR-CX405). Video cameras were positioned 1 m away from apparatus throughout the experiment. Each video was subjected to a frame-by-frame analysis using the software PremierePro Adobe CS6. Following Chow et al. (2018), this analysis required intensive observer training (around 2 months) to identify each individual’s unique physical characteristics such as markings or colors on the face/limbs/body including body/tail/ear shape (see Supporting Information Note 1 for identification method and reliability test).
To examine cognitive performances, we used two tasks previously employed by Chow et al. (2018) in addition to two well-established tasks (Chow et al., 2015, 2017). These tasks included (1) an easy (Figure 1A, see SV1_easy_task_LukeC) and a difficult (Figure 1B, see SV2_difficult_task_JessicaJ) novel food-extraction problem (hereafter, innovation) to measure behavioral flexibility and innovative problem-solving performance, (2) a recall test to measure motor memory (or the successful and efficient solutions) for solving the difficult (an experienced) problem after an extended period (hereafter, the recall test), and (3) a spatial-learning task (Figure 1C, see SV3_Spatial Memory_Belle) to measure associative learning, or gradual behavioral changes with increased experience, between locations and rewards (hereafter, spatial learning).
Figure 1. Four tasks that are used to measure flexibility and cognitive performance: Two innovation tasks that include (A) the easy problem that requires a squirrel (X-23 is shown in the image) to lift up a transparent lid using its teeth to solve the problem, and (B) the difficult problem that required a squirrel (Iron Fist shown in the image) to use its nose to cause a lever and/or nut drop by pushing a lever-end that was close to the reward. The left image shows the transparent Plexiglas box during the habituation phase (without levers) whereas the right image shows the box during the main testing (with 10 levers). Also shown are the two cognitive tasks that include (C) the spatial-learning task in which a squirrel had to associate locations with rewards (Quick Silver is shown in the right image). The left image shows the five holes (in dark circles) at the bottom of each container where inaccessible nut pieces were used to control squirrels use of olfactory cues during locations-rewards learning. Rewarded containers were baited at diagonal corners (total 8 containers) to control side bias. The recall test was the same difficult problem in (B) presented to squirrels 25 days after their last experience it.
The easy problem was modified from a previous study (Chow et al., 2018). The apparatus was constructed with 32 interlocking plastic containers (each container: length × width × depth: 4.5 cm × 3 cm × 1.5 cm). Sixteen of 32 containers were each covered by a transparent lid which squirrels could easily lift to access a reward. The other 16 containers were turned upside down and used as a connection between containers (Figure 1A). The difficult problem was the same food-extraction problem that was used by Chow et al. (2018) who have shown that it was a more difficult problem than the easy problem for wild gray squirrels, as the solutions involved counter-intuitive methods (also see section “Comparison for the Two Innovation Tasks” below). It was a puzzle box (Figure 1B) that had a transparent cubic-shaped plexiglas top (length × width × height: 25 cm × 25 cm × 19 cm) and a pyramid-shaped wooden base (length × width × height: 25 cm × 25 cm × 3 cm). On each side of the box, there were 10 holes (length × width: 2 cm × 0.9 cm) aligned horizontally but not vertically. The holes on one side of the box were roughly aligned with the holes on the opposite side. Ten levers (1.5 cm × 29.8 cm and 0.5 cm thick) were inserted across the box through the holes, leaving 2.5 cm of both lever-ends protruding outside the box. This design allowed the squirrels to act on any lever-end using their nose, teeth or front paws. On each lever, there was a nut container (transparent back: 2 cm × 1.5 cm; opaque side: 1.5 cm × 1.5 cm) 2.5 cm away from one lever-end. This design allowed squirrels to see the nuts when they were on the opposite side of the box. The containers were positioned just inside the box so that squirrels had to cause a lever/nut to drop by pushing (instead of pulling) a lever-end if they were close to a nut container, or pulling (instead of pushing) the other lever-end if they were far from the nut container. The whole box was secured by four wooden legs, creating a 4.5 cm gap between the top and the base in which squirrels could obtain a nut (if there was a nut) upon solving the challenge. This apparatus was also used for the third task in which we measured motor memory in the recall test.
To examine whether squirrels were learning by associating locations with rewards that would not be affected by side bias, we relied on the square-shaped box design from our previous study, in which gray squirrels in a laboratory were required to learn that two (out of four) diagonally positioned corners were rewarded (Chow et al., 2015). To do this, our square-shaped box in the easy problem was modified so that the new apparatus had a top and a bottom layer (Figure 1C). The top layer of the box had four corners, with each corner formed by four containers (2 × 2 containers to form a right angle). The bottom layer was a plexiglas container (3 cm depth) that had five holes (diameter: 0.2 mm) at the bottom of each container. It served for two functions: (1) we filled these bottom containers with inaccessible hazelnut pieces and replaced the nut pieces regularly to refresh the scent; this aimed to control squirrels using the scents of nuts as cues during learning; (2) the bottom layer increased the height of the box so that the squirrels had to lift up a lid completely (i.e., the lid stood still vertically), which prevented the squirrels from re-approaching a (rewarded) well that was empty.
Our experimental procedure generally followed that of Chow et al. (2018), which maximized the measure of individual performance and minimized confounding variables such as the effect of weather or social interference and social learning on performance (see Supplementary Information Note 2). The procedures involved presenting the apparatus in the field from dawn to dusk daily for 6–8 weeks at each site, regardless of weather conditions. This approach allowed individuals to participate in the experiment during their active time, as well as allowing this species, an opportunistic food generalist, to exploit new resources. The fact that the squirrels were allowed to freely visit and leave a task at all times also ensured high motivation to participate in the tasks. In addition, we used the inter-trial interval protocol set by Chow et al. (2018) as a guide to include subordinates when dominant individuals were at rest (i.e., individuals of this species have slightly different active times and thus, both subordinates and dominants can freely participate in the tasks). The increased number of squirrels in urban parks has been shown to positively correlate with increased intraspecific aggression (Parker and Nilon, 2008), leading to dominant individuals monopolizing an apparatus. Accordingly, we placed two identical apparatuses in urban habitats to minimize social disturbance from conspecifics or social learning. However, this arrangement did not prevent natal dispersal (Koprowski, 1996) which led to variation in total number of squirrels participating in all tasks.
Two days before the main experiment, we selected a location either covered by bushes or close to a tree, some distance from major roads to minimize predation risk and incidents of road kills. Once a location was selected, we presented whole intact hazelnuts and hazelnut kernels to attract squirrels. We checked and rebaited each location three times per day. The main experiment started once we observed squirrels visiting the location regularly and emptying the nuts in that location. All tasks were set at the same location throughout the experiment. We conducted the same number of trials across sites, but the inter-trial interval varied depending on the available daylight hours (see Table 1). A trial started after checking the apparatus and ended with the next check. A video camera was used to record both individual identity and all task performances.
Table 1 summarizes the details of the main experimental protocol. We first presented squirrels with the two innovation tasks, followed by the spatial-learning task and finally the recall test. We counterbalanced the presentation of the easy and the difficult problems across sites. Before the main experiment started, we conducted a habituation phase for each task, aiming to minimize neophobic responses to novel objects. For the easy problem, we placed 8 hazelnut kernels on the top of the apparatus. For the difficult problem, we carried out two trials of 2 h (total 4 h) of habitation in which we placed 10 hazelnuts with shells on each side of the transparent box without levers (Left image of Figure 1B). As the setup of the video camera could not capture the squirrels approaching from behind the camera, we did not have a standardized way to measure neophobia. Nevertheless, the experimenter (PKYC) stood at least 25 away from the apparatus and noted the squirrels in all locations approaching the apparatus or retrieving a nut under a minute upon spotting the apparatus from a tree or on the ground. The main experiment for each task started when squirrels obtained all nuts from the apparatus for two consecutive trials (determined for each trial from video footages). For the easy problem, we placed a hazelnut kernel in each container during the main experiment. We randomised the orientation of the box between trials. For the difficult problem, we inserted 10 levers across the box (Figure 1B). Five levers contained shelled nuts, whereas the other five levers were empty (which served as a control). For each trial, we randomized the facing direction of the levers and which levers contained a nut. These randomizations were also used for the recall test of this difficult problem, 25 days after their last experience. For the spatial-learning task, we baited rewards at diagonal corners (total 8 containers) and used a rock to secure the apparatus. The position and orientation of the apparatus and the rock was set in the same way in each trial so that squirrels would be able to use any cues to locate a reward (e.g., the relative distance between the rock and a rewarded container, or the relative distance between a tree and the rewarded container).
We analyzed behavioral data using Adobe PremierePro CS6. We quantified task performance as a proxy for proficiency (proportion of success), latency or efficiency and accuracy. See below for definitions of each index, for each task.
For the two innovation tasks, proficiency could be defined as the ability to apply existing behaviors when solving novel problems (Kummer and Goodall, 1985; Reader and Laland, 2003). We measured such proficiency as the solving outcome for each task: ‘innovators’ were those that successfully solved a problem once or more, whereas ‘non-innovators’ were those that failed to solve the problem. Success in the easy problem was determined when squirrels lifted the transparent lid of a container. Success in the difficult problem was indicated when squirrels made a lever/nut drop. We measured whether the squirrels successfully solved each problem on their first visit or on their subsequent visit. A visit was defined when a squirrel first appeared in the video and manipulated either problem until it was out of the video for ≥2 min. A subsequent visit was recorded when the same squirrels reappeared in the video and manipulated the problem until it left the video for ≥2 min. In the case of a successful innovation, we also considered the speed (or efficiency) to innovate. We measured the total latency of an individual to obtain a success (hereafter, innovation latency). Because not every manipulation would lead to an immediate success, this innovation latency consisted of several bouts of unsuccessful manipulations (or solving latencies). We measured a bout of manipulation for the easy problem as the time from when a squirrel started using any of its body parts to manipulate a lid, until it either stopped manipulating that part of the apparatus (in the case of unsuccessful solving) or lifted the lid (in the case of successful solving). Likewise, we measured a bout of manipulation for the difficult problem as the duration from when a squirrel started using any part of its body to manipulate a lever, until it either stopped manipulating that lever in unsuccessful cases or made a lever/nut drop in successful cases. Lower solving latency reflected a higher degree of efficiency.
For each individual that had participated in the difficult problem and also turned up for the recall test, we used the same measurement defined above in innovation latency to determine the total latency to obtain success in the recall test. To minimize the confusion between the two total latencies, we referred to the total latency in the recall test as ‘recall latency.’ This latency may be used to determine whether the squirrel used a similar amount of time to solve the difficult problem after an extended period. We further recorded the successful solution that each innovator used to solve the problem on their very last success with the solution it used on its first return.
For the spatial-learning task, we included squirrels that had participated in the task on a daily basis, because learning induces progressive behavioral changes (van Schaik, 2013). Due to the proximity between containers, squirrels tended to open a container that was adjacent to the previous one. Accordingly, we examined learning performance by focusing on the first container (rewarded or unrewarded) that a squirrel opened in each visit (i.e., when it arrived at the task until it left the box and moved >5 m away). The learning criterion was six (out of eight) or more successive first openings of rewarded containers and these openings included both rewarded corners. This criterion minimized perfect performance (i.e., choices on four adjacent rewarded wells located on one corner would be explained by side bias/preference). We measured proficiency as the total number of first openings that squirrels made before reaching the criterion (i.e., excluding the six openings to reach criterion), with fewer first openings made to reach the criterion indicating higher proficiency. To further understand whether the squirrels showed similar learning performance before reaching the criterion, we analyzed efficiency and accuracy (e.g., two individuals used comparable numbers of first openings to reach criterion, but one individual made a lot of openings in the unrewarded wells whereas the other individual made an equal number of openings in the rewarded and unrewarded wells). Efficiency was measured as the number of first openings on unrewarded containers (or errors) that squirrels made before reaching the learning criterion, with fewer number of errors indicating higher efficiency. Accuracy was recorded as the number of first openings on rewarded (or correct) containers that squirrels made before reaching the learning criterion, with a higher number of correct indicating higher accuracy.
Given that empirical evidence has implied that ‘enhanced’ cognitive ability may well be a species characteristic of gray squirrels (Parker et al., 2013), we analyzed data using frequentist model testing and Bayesian null hypothesis testing. Given that the tasks presented to the squirrels here were established, the Bayesian approach allowed us to incorporate prior findings and assess data from a probability distribution (i.e., credible interval) as well as to assess the strength of our data in supporting the null hypothesis relative to the alternative hypothesis (i.e., Bayes factor) (Jarosz and Wiley, 2014). Such an approach is useful when sample size is small and when examining results that appear to support null hypotheses, as in our case (Ellison, 2004; Garamszegi, 2016). We used packages ‘lme4’ (Bates et al., 2015), ‘brms’ (Bürkner, 2017, 2018) and ‘bayestestR’ (Makowski et al., 2019) in RStudio version 1.1.463 (RStudio Team, 2016) to analyze these data.
The Shapiro–Wilks test was used to examine the normality of data and, in turn, determine the appropriate link function for the distribution of response variables. Because the two innovation tasks have been shown to vary in their level of difficulty (Chow et al., 2018), we first examined whether the level of task difficulty (easy or difficult problem) had an effect on innovation latency. For this analysis, we included the squirrels that solved both the easy and the difficult problems, and conducted a Generalized Linear Mixed Model (GLMM) with gamma log-link distribution that included task (easy and difficult) as a fixed factor, the total latency to achieve the first success for each food-extraction problem as the response variable, and site as a random variable.
For frequentist model testing, we used Tukey’s HSD tests for P-value adjustments for multiple group comparisons. We considered the significance level of a two-tailed test when P ≤ 0.05. We report descriptive statistics including means with standard deviations and the two-tailed significance tests. For Bayesian null hypothesis testing, we included informative prior distributions based on the findings for wild and laboratory gray squirrels using the same or similar tasks (see details below). We report median estimate, lower and upper bound of highest posterior distribution (HPD), credible interval (CI) and Bayes factor (BF10). Bayesian statistics included 4 chains, each with 10,000 iterations and 1,000 warmup samples (totalling 36,000 for post-warmup samples).
For each innovation task, squirrel group (i.e., native rural, native urban, non-native rural, and non-native urban) was a fixed factor to assess group differences in solving outcome (success/failure). We used Generalized Linear Model (GLM) with binominal distribution and logit link function to compare the number of squirrels that were innovators or non-innovators on their first visit and subsequent visits. To examine whether the innovators in the four habitats differed in the mean solving latency with increased experience (i.e., learning rate) of each innovation task, we used our previous findings (Chow et al., 2018) as a guide for the number of successes required to reach the asymptote in each task. We included squirrels that had completed 4 blocks of 5 successes (20 successes) for the easy problem and 7 blocks of 5 successes (35 successes) for the difficult problem. A block of mean solving latency was the average of five total solving latencies to achieve success. To accommodate variables with numerical values that were positively skewed but not strongly centered on zero, we used GLM with gamma distribution and log link function to model solving latency of the first block of success for each of the innovation tasks. A GLMM with gamma distribution and log link function was used to assess whether solving latency in subsequent blocks of success was affected by group, success block number, and their interaction. Due to convergence or single fit issues, all models included random effect squirrel identity (see additional analyses including site as a random variable in Supplementary Tables 1,2). In Bayesian model testing, information for prior distribution of the solving latency in each innovation task (the easy task: M = 4.32s; SD = 4.51; the difficult problem: M = 12.66s; SD = 21.22) was obtained from Chow et al. (2018) who used the same task in another population of non-native gray squirrels.
For the recall test, we analyzed innovators that had participated in the difficult problem and returned to the recall test. Each innovator varied in the number of successes for the difficult problem. Thus, we obtained the mean of the very last five total solving latencies in the difficult problem for each innovator as their “last block of mean solving latency.” We then used a GLMM to compare this mean latency with the mean recall latency of the first block (i.e., the mean of the very first five total solving latencies to achieve success) for the recall test. We also used a GLMM to examine whether additional learning occurred during the recall test. We obtained a lower number of successes per innovator for this test than the difficult problem because the recall test was presented to the squirrels for 1 day only (see Table 1 for experimental protocol). Nevertheless, 18 (out of 21 innovators) completed 2 blocks of 5 successes (10 successes), which allowed us to conduct between-group analyses. In all GLMM models, group, success block number, and their interaction were included as fixed variables. We additionally controlled for the number of previously successful experiences that each innovator obtained as a covariate, whereas individual identity was set as a random variable to simplify the model structure (see additional analyses including site as a random variable in Supplementary Table 3). In Bayesian analyses, we took the average of the first five successes (i.e., a block; M = 4.60; SD = 2.53) in a study that assessed laboratory squirrels’ long-term memory (Chow et al., 2017) for the prior distribution.
For the spatial-learning task, we examined three performances. These included: (1) the total number of first openings that the squirrels made before reaching the criterion (i.e., proficiency); (2) the number of first openings on unrewarded containers (or errors) that the squirrels made before reaching the learning criterion (i.e., efficiency); and (3) the number of first openings on rewarded containers (or correct openings) that the squirrels made before reaching the learning criterion (i.e., accuracy). In model testing for the spatial-learning task, we used GLMM Poisson distribution with log link function to accommodate count data. Group was the fixed factor whereas squirrel identity was the random effect (see additional analyses including site as a random variable in Supplementary Table 4). For Bayesian analyses, we used a design similar to that of Chow et al. (2015) for a study of gray squirrels in the laboratory; this included the mean (M) and standard deviation (SD) for the total number of first openings before reaching the criterion (M = 29.4; SD = 11.13), the number of errors in first openings before reaching the criterion (M = 6.20; SD = 3.19) and the number of correct first openings before reaching the criterion (M = 22.8; SD = 10.45).
Twenty-six squirrels participated in both tasks. The squirrels took significantly less time to solve the easy problem than the difficult problem (GLMM: χ21 = 7.38, P = 0.007). On average, squirrels used 10 s (SD ± 11.30) to solve the easy problem and 21.13 s (SD ± 20.98) to solve the difficult problem.
Fifty-one squirrels attempted to solve the easy problem (see SV1_easy_task_LukeC in Supplementary Materials). Figure 2A shows the proportion of innovators in each group on the first visit and in the subsequent visits. The difference between the number of innovators in the four groups was not significant on the first visit (GLM: χ23 = 4.24, P = 0.237) or the subsequent visits (χ23 = 5.54, P = 0.137). Thirty-two innovators completed 4 blocks of 5 successes. The four groups did not differ significantly in solving latency on the first block (χ21 = 7.29, P = 0.063, Figure 2B). The innovators in all four groups significantly decreased their mean solving latency with increased experience (GLMM: χ21 = 8.42, P = 0.004, Figure 2B). The interaction between group and blocks of successes were also significant (group∗success blocks: χ21 = 9.72, P = 0.021). Post hoc analyses in the interaction between groups and success blocks revealed marginal significance between the native rural group and the native urban group (P = 0.055, Table 2 and Figure 2B) and between the native rural group and the non-native rural group (P = 0.064, Table 2). Bayesian inference tests showed that significant lower solving latency across success blocks was observed in the native rural group than the native urban group and the non-native rural group. Despite this, the Bayes factor showed that these results remained anecdotal. Aside from these two results, all other results provide moderate to very strong support for the null hypothesis over the alternative hypothesis (Table 2).
Figure 2. Comparisons of the performance in the easy problem among the four squirrel groups (native rural, native urban, non-native rural, and non-native urban group). (A) Success rate (the number of innovators that lifted a lid) on the first visit (First) and in the subsequent visit (Final), and (B) mean total solving latency to obtain a success (in seconds) across 4 blocks of 5 successes (20 successes).
Table 2. GLMM and Bayesian test on the effect of squirrel group (native rural, native urban, non-native rural, and non-native urban group), success block number, and their interaction (Group*Block) on solving latency of the easy problem.
Sixty-seven squirrels participated in the difficult problem (see SV2_difficult_task_JessicaJ in Supplementary Material). Figure 3A showed the proportion of innovators for the difficult problem on the first visit and in the subsequent visits. There was a significant difference in the number of squirrels successfully solving the problem between the four groups (GLM χ23 = 10.07, P = 0.018, Figure 3A). Post hoc analyses revealed that the native urban group had a higher success rate than the non-native urban group in solving the difficult problem on the first visit (P = 0.022; Figure 3A). In the subsequent visit, the difference in the success rate between the number of innovators in the four groups was not significant (χ23 = 7.32, P = 0.062, Figure 3A). Eighteen innovators completed 7 blocks of 5 successes. Neither group nor the interaction between group and blocks of successes were significant (GLMM group: χ23 = 1.10, P = 0.776, group∗block: χ23 = 2.14, P = 0.543, Table 3 and Figure 3B). The innovators significantly decreased their mean solving latency to achieve success with increased experience (χ21 = 5.13, P = 0.023, Figure 3B). Bayes factor values indicated moderate to extremely strong results favoring the null hypothesis over the alternative hypothesis (Table 3).
Figure 3. Comparison of the performance in the difficult problem among the four squirrel groups (native rural, native urban, non-native rural, and non-native urban group). (A) Success rate (the number of innovators causing a lever/nut drop) on the first visit (First) and in the subsequent visits (Final), and (B) mean total latency to obtain a success (in seconds) across 7 blocks of 5 successes (35 successes).
Table 3. GLMM and Bayesian test on the effect of squirrel group (native rural, native urban, non-native rural, and non-native urban group), success block number, and their interaction (Group*Block) on solving latency of the difficult problem.
Twenty-five days after the innovators had experienced the difficult problem, 21 innovators participated in the recall test (Figure 4A) and all innovators successfully solved the same problem after the extended period. The solution that the innovators used to solve the problem on their first return was the same as the solution used on their very last success, indicating that all squirrels remembered the learned successful solution for this problem.
Figure 4. Comparison of the performance in the recall test among the four squirrel groups (native rural, native urban, non-native rural, and non-native urban group). (A) The very last block (i.e., mean total latency of the last five successes) of the difficult problem and the first block (i.e., mean total latency of the first five successes) of the recall test for each group. (B) The first block and the second block of the recall test across 2 blocks of 5 successes (10 successes) for each group.
At the within-group level, the innovators did not show a significant difference between their mean innovation latency in the very last five successes of the difficult problem and the mean recall latency in the first block of the recall test (last_first: GLMM χ23 ≤ 0.01, P = 0.957, Figure 4A and Table 4). The interaction between group and blocks was also not significant (group∗last_first: χ23 = 1.40, P = 0.706, Table 4). Bayes factors indicated moderate to strong evidence favoring the null over the alternative hypothesis. At the between-group level, we found that groups significantly differed in their mean recall latency on the first block of the recall test (group: χ23 = 8.20, P = 0.042, Figure 4B). However, pairwise comparisons indicated no significant difference between groups. Whereas Bayesian inference test showed a significant difference between the native urban and non-native rural group, the Bayes factor indicated the significant difference was anecdotal (Table 4). As the difficult problem required 40 or more successes to reach asymptote, we examined whether the highly-experienced innovators would be able to retrieve the learned solution for the difficult problem (as opposed to the less experienced innovators who were still learning the solution). To do so, we further analyzed the innovators that were highly experienced with the task (i.e., innovation latency reached a plateau) by including those that had completed 7 blocks of 5 successes (35 successes) in the difficult problem and that had returned to the recall test (Nnativerural = 2, Nnativeurban = 4, Nnon–nativerural = 5, Nnon–nativeurban = 5). When we compared the innovators’ very last 5 successes’ of innovation latency with their first block of recall latency, the four groups showed comparable results (group: χ23 = 5.47, P = 0.140; last_first: χ21 = 0.10, P = 0.758; group∗last first: χ23 = 1.91, P = 0.592).
Table 4. The recall test: GLMM and Bayesian approach testing the effect of squirrel group (native rural, native urban, non-native rural, and non-native urban group) on success block number, and their interaction on recall latency.
For the innovators that had completed two blocks of success in the recall test, the four groups significantly differed in their solving latency (χ23 = 8.32, P = 0.040); post hoc analyses showed that the native urban group had a significantly higher solving latency than the other three groups (Figure 4B). Recall latency across the two blocks of successes was not significant (χ21 = 1.21, P = 0.271). However, the interaction between group and success blocks was significant (first_second: χ23 = 17.06, P < 0.001); the native urban group showed a decrease in solving latency across the two blocks (P < 0.001, Table 4). When we included the innovators that had completed 35 successes (Nnativerural = 3, Nnativeurban = 6, Nnon–nativerural = 5, Nnon–nativeurban = 4), further learning at the group level became non-significant (group: χ23 = 6.38, P = 0.094; first_second: χ21 = 0.20, P = 0.658; group∗first_second: χ23 = 4.20, P = 0.240).
Forty-six squirrels participated in the spatial-learning task (see SV3_spatial_learning_Arrow in Supplementary Material). Table 5 shows the results for between-group comparisons. The difference in the number of total openings between the four groups was not significant (GLMM χ23 = 1.22, P = 0.748, Figure 5A). Bayes factors ranged from <0.001 to 0.024, thus providing evidence favoring the null hypothesis over the alternative hypothesis. The four groups also showed comparable numbers of first openings on unrewarded containers (χ23 = 3.13, P = 0.372, Figure 5B) and rewarded containers (χ23 = 2.00, P = 0.572, Figure 5C) before reaching the learning criterion. Bayes factors ranged from <0.001 to 0.229, indicating moderate to strong support for the null over the alternative hypothesis.
Table 5. The spatial learning task: results of GLMM and Bayesian approach testing the effect of squirrel group (native rural, native urban, non-native rural, and non-native urban group), success block number, and their interaction on the performance of the spatial learning task.
Figure 5. Comparison of the performance of a spatial-learning task across the four squirrel groups (native rural, native urban, non-native rural, and non-native urban group). (A) Proficiency, the total number of first openings in rewarded and non-rewarded containers before reaching the learning criterion. (B) Efficiency, the number of non-rewarded containers opened or error openings before reaching the learning criterion. (C) Accuracy, the number of rewarded containers opened or correct openings before reaching the learning criterion.
Enhanced cognitive ability may result from the adaptation process in new environments in urban or non-native areas, but the ‘enhancement’ may as well reflect a typical species characteristic. Here, we have followed up on previous findings demonstrating that a higher success rate in innovation was observed in non-native gray squirrels compared with native red squirrels residing in the foreign environment. We used an intra-specific comparative paradigm to determine the likely cause of ‘enhanced’ cognitive ability by comparing cognitive task performances (in innovation, spatial learning and motor memory) of gray squirrels residing in rural and urban habitats in both native and non-native environments.
We found that the innovators in the four groups showed comparable proficiency and latency in solving the easy problem (Figures 2A,B). Differences were also observed in the number of innovators successfully solving the difficult problem on their first visit between the non-native urban group and the native urban group, but not between the rural and urban groups (Figure 3A). These findings appear to contradict the assumption that novel environments, as in habitats that are out of a species’ native range or urban environments, would enhance performance. The fact that success rate was comparable among the four groups in subsequent visits may have suggested that other traits such as persistence, which are key to innovation success, differ between populations, and thus, influence the success rate in the first visit (see Supplementary Information Note 3). Variation in these traits may be moderated by more subtle and variable environmental characteristics (e.g., green canopy coverage, direct human disturbance) in urban habitats.
The fact that the squirrels in the native urban group outperformed the non-native urban group on their first visit in the difficult problem may reveal the readiness of individuals exploiting novel resources in their (new) environments. Our findings appear to suggest that a lower degree of readiness (or high neophobic response to novel objects) in utilizing novel resources is seen in the non-native urban group. Higher levels of neophobia to food and novel objects has been shown to have undergone adaptation in non-native cane toads (Rhinella marina) compared with their native counterparts (Candler and Bernal, 2015). However, this explanation is unlikely because when we observed squirrels in the field (e.g., hiding behind a tree in rural sites or standing 25 m away from the apparatus in urban sites), all groups would more-or-less readily approach or manipulate novel objects. In addition to this, within 1–2 min after setting up the apparatus, the squirrels either took the freely available nuts during the habituation phase or attempted to solve the problem when they first observed each food-extraction task, even though our set up was not the ideal method for measuring neophobia (the setup of the video camera may introduce measurement bias as squirrels may approach the apparatus behind the video camera). Nevertheless, other reports have suggested that gray squirrels efficiently exploit new resources in urban environments. For example, gray squirrels living in cities use different artificial structures such as chimneys, attics, or roofs as dreys (Clark, 1994) and consume different types of food such as sunflower seeds that are not naturally present in their habitats (Bowers and Breland, 1996) or artificially-processed food from trash cans (PKY. Chow, personal observation). Accordingly, an alternative explanation for such differences may follow from traits that are important for innovation performance as discussed above.
Despite evidence of a higher success rate in solving the difficult problem in the native urban group than the non-native urban group, we also found that the squirrels in the native urban group showed additional learning in the recall test. This suggests that the squirrels in the native urban group invest less time for learning when they encounter new food sources. However, this result should be interpreted with caution because this is more likely related to the fixed number of trials presented to squirrels (Table 1). A limited number of rewards was shared among a higher number of innovators from the first visits, leading the native urban group to obtain a lower mean number of successful experiences than the non-native urban group before the recall test. This could be further supported by the analysis on the highly experienced innovators across the four groups. They showed no further improvement in the recall test, which indicates the innovators in the native urban group invest in learning and securing new food sources (Table 4).
Thus far, we have highlighted the similarities and differences between groups. Our results overall indicate that the four groups mostly show comparable performance, thus favoring the hypothesis that the ‘enhancement’ of this trait is likely a species characteristics. However, our results in solving novel problems also suggests that these cognitive abilities may have undergone mild modifications during the adaptive process when invading new environments, but are not relate to urbanization. Here, we consider other possibilities that may explain the comparable performance between the four groups in the cognitive tasks. One possibility is that our sampling effort in only one non-native urban population may limit the detection of differences between the groups. However, it seems unlikely because another non-native founding population that had solved the same easy and difficult problems also showed comparable success rate and efficiency (Chow et al., 2018). This indicates that some of the results are replicated. In addition to this, our use of Bayesian analyses allowed us to assess the distribution of these data in relation to the previous results. The fact that Bayes factors of comparable task performances indicate strong to extremely strong evidence for the null hypothesis over the alternative hypothesis strengthens the conclusion that the previously observed ‘enhanced’ problem-solving performance in gray squirrels is likely an inherited trait or species characteristics.
Another possibility that would have limited the detection of differences between groups may have been related to our test procedure. For example, the gray squirrel is a scatter-hoarder (Jacobs and Liman, 1991; Macdonald, 1997) that has excellent spatial learning memory as a result of this specialization (Shettleworth, 1990; Vander Wall, 1990, p.3; Pravosudov and Roth, 2013). Perhaps, our recall test could follow the test duration of a previous study in which the non-native laboratory gray squirrels dealt with the same difficult problem after nearly 2 years instead of 25 days in the present study. However, the fact that less than half of the innovators returned to solve the difficult problem, possibly as a result of dispersal (Koprowski, 1996), reflects our practical constraint for assessing long-term memory in wild populations. Nevertheless, we were able to show that all four groups of innovators are capable of retaining relevant information for at least 25 days despite differences in the recall latency observed in the four groups.
As a final note, we emphasize that because the present study has not examined the variation in cognitive ability at different invasion stages, our findings here in this long-established population should not be taken to suggest that there is no variation in ability and performance at other invasion stages. For example, enhanced performance and flexibility may be particularly important for a species at the early stage of establishment (i.e., ‘the adaptive flexibility’ hypothesis, see Wright et al., 2010) and may decrease to its original level when a species successfully establishes in a novel environment. If this hypothesis is true, then better performance would be observed in populations that are expanding than those that are established in foreign environments. Indeed, there is evidence that there are differences in phenotypic traits such as reproductive rate of gray squirrels which is higher in frontier populations than those in established populations (Goldstein et al., 2015). Accordingly, we suggest future studies should test the ‘adaptive flexibility hypothesis’ by comparing flexibility (alongside other cognitive performances of squirrels) between frontier populations with established populations. One might also examine whether there is a general pattern across cognitive tasks (‘g’) and whether such patterns would be similar or differ in new environments (e.g., rural vs. urban area). However, if performance of the frontier population is comparable with the established population, then the findings will further strengthen the conclusion that this trait is likely a species characteristic as has been suggested in other traits that have been proposed to render ‘inherent superiority’ (e.g., competitive ability, see Elton, 1958).
We used an intra-specific comparative paradigm to compare cognitive performance in rural and urban squirrels in both their native and non-native environments. Our results suggest that although some cognitive abilities such as solving novel food-extraction problems may have undergone mild variation during the adaptive process in novel environments (but less in urban areas), comparable task performance suggests that the previously observed ‘enhanced’ cognitive performance of gray squirrels is a pre-adaptive characteristic. This pre-adaptive characteristic may be related to their habitat and lifestyle as a food generalist. This, alongside, other pre-adaptive biological and reproductive traits (Parker et al., 2013) may help to explain why they are well-adapted in both rural and urban habitats (Bonnington et al., 2014), and why few numbers of introduced gray squirrels (see Wood et al., 2007) often result in a high rate of establishment (80% or 59 out of 74 introductions, see Bertolino, 2009) and invasion (Lowe et al., 2004).
The raw data supporting the conclusions of this article will be made available by the authors, without undue reservation.
The animal study was reviewed and approved by Institutional Animal Care and Use Committee, Wilkes University and the Ethics Committee, University of Cambridge.
PKYC designed the study and conducted statistical analysis. PKYC wrote the first draft of the manuscript. All authors contributed to the article and approved the submitted version.
This study was funded by the Association for the Study in Animal Behaviour (research grants to PKYC). MAS recognizes support of the United States National Science Foundation (DEB 15556707), and the H. Fenner Research Endowment of Wilkes University for support during the study.
The authors declare that the research was conducted in the absence of any commercial or financial relationships that could be construed as a potential conflict of interest.
We are grateful to St. John’s College, University of Cambridge (United Kingdom), Wilkes University (United States) and Kings Grant Association (United States) granted access to the study areas. We also thank Robert T., Davies J., Temizyürek T., and two reviewers for their feedbacks on the manuscript, D. Taylor for building a puzzle box, and G. Chang, Mr. A. Yuen and Mrs. A. Yuen, and J. Curry for logistic supports during the data collection in the United States.
The Supplementary Material for this article can be found online at: https://www.frontiersin.org/articles/10.3389/fevo.2021.615899/full#supplementary-material
Amiel, J. J., Tingley, R., and Shine, R. (2011). Smart moves: effects of relative brain size on establishment success of invasive amphibians and reptiles. PLoS One 6:e18277. doi: 10.1371/journal.pone.0018277
Audet, J. -N., Ducatez, S., and Lefebvre, L. (2016). The town bird and the country bird: problem solving and immunocompetence vary with urbanization. Behav. Ecol. 27, 637–644. doi: 10.1093/beheco/arv201
Bates, D., Mächler, M., Bolker, B., and Walker, S. (2015). Fitting linear mixed-effects models using ‘lme4’. J. Statist. Softw. 67, 1–48.
Bertolino, S. (2009). Animal trade and non-indigenous species introduction: the world-wide spread of squirrels. ıDiver. Distribut. 15, 701–708. doi: 10.1111/j.1472-4642.2009.00574.x
Bonnington, C., Gaston, K. J., and Evans, K. L. (2014). Squirrels in suburbia: influence of urbanisation on the occurrence and distribution of a common exotic mammal. Urban Ecosyst. 17, 533–546. doi: 10.1007/s11252-013-0331-2
Bowers, M. A., and Breland, B. (1996). Foraging of gray squirrels on an urban-rural gradient: use of the gud to assess anthropogenic Impact. Ecol. Applic. 6, 1135–1142. doi: 10.2307/2269597
Bürkner, P. -C. (2017). brms: an R package for bayesian multilevel models using stan. J. Statist. Softw. 80, 1–28. doi: 10.18637/jss.v080.i01
Bürkner, P. -C. (2018). Advanced Bayesian Multilevel Modeling with the R Package brms. R J. 10, 395–411. doi: 10.32614/RJ-2018-017
Candler, S., and Bernal, X. E. (2015). Differences in neophobia between cane toads from introduced and native populations. Behav. Ecol. 26, 97–104. doi: 10.1093/beheco/aru162
Cauchard, L., Boogert, N. J., Lefebvre, L., Dubois, F., and Doligez, B. (2013). Problem-solving performance is correlated with reproductive success in a wild bird population. Anim. Behav. 85, 19–26. doi: 10.1016/j.anbehav.2012.10.005
Chow, P. K. Y., Lea, S. E. G., Hempel de Ibarra, N., and Robert, T. (2017). How to stay perfect: the role of memory and behavioural traits in an experienced problem and a similar problem. Anim. Cogn. 20, 941–952. doi: 10.1007/s10071-017-1113-7
Chow, P. K. Y., Lea, S. E. G., Hempel de Ibarra, N., and Robert, T. (2019). Inhibitory control and memory in the search process for a modified problem in grey squirrels, Sciurus carolinensis. Anim. Cogn. 22, 645–655. doi: 10.1007/s10071-019-01261-6
Chow, P. K. Y., Leaver, L. A., Wang, M., and Lea, S. E. G. (2015). Serial reversal learning in gray squirrels: learning efficiency as a function of learning and change of tactics. J. Exper. Psychol. 41, 343–353. doi: 10.1037/xan0000072
Chow, P. K. Y., Lurz, P. W. W., and Lea, S. E. G. (2018). A battle of wits? Problem-solving abilities in invasive eastern grey squirrels and native Eurasian red squirrels. Anim. Behav. 137, 11–20. doi: 10.1016/j.anbehav.2017.12.022
Clark, K. D. (1994). “Managing raccoons, skunks, and opossums in urban settings,” in Proceedings of the 16th Vertebrate Pest Conference, Davis, CA.
Cole, E. F., Morand-Ferron, J., Hinks, A. E., and Quinn, J. L. (2012). Cognitive ability influences reproductive life history variation in the wild. Curr. Biol. 22, 1808–1812. doi: 10.1016/j.cub.2012.07.051
Garamszegi, L. Z. (2016). A simple statistical guide for the analysis of behaviour when data are constrained due to practical or ethical reasons. Anim. Behav. 120, 223–234. doi: 10.1016/j.anbehav.2015.11.009
Giraldeau, L. A. (1994). Group foraging: the skill pool effect and frequency-dependent learning. Am. Naturalist 124, 72–79. doi: 10.1086/284252
Goldstein, E. A., Butler, F., and Lawton, C. (2015). Frontier population dynamics of an invasive squirrel species: do introduced populations function differently than those in the native range? Biol. Invas. 17, 1181–1197. doi: 10.1007/s10530-014-0787-x
Gonzales, E. K. (2005). The distribution and habitat selection of introduced eastern grey squirrels, Sciurus carolinensis, in British Columbia. Can. Field Naturalist 119, 343–350. doi: 10.22621/cfn.v119i3.143
Jacobs, L. F., and Liman, E. R. (1991). Grey squirrels remember the locations of buried nuts. Anim. Behav. 41, 103–110. doi: 10.1016/s0003-3472(05)80506-8
Jarosz, A. F., and Wiley, J. (2014). What are the odds? A practical guide to computing and reporting bayes factors. J. Probl. Solv. 7:2.
Jessen, T., Wang, Y., and Wilmers, C. C. (2018). Habitat fragmentation provides a competitive advantage to an invasive tree squirrel, Sciurus carolinensis. Biol. Invas. 20, 607–618. doi: 10.1007/s10530-017-1560-8
Keagy, J., Savard, J.-F., and Borgia, G. (2009). Male satin bowerbird problem-solving ability predicts mating success. Anim. Behav. 78, 809–817. doi: 10.1016/j.anbehav.2009.07.011
Koprowski, J. (1996). Natal philopatry, communal nesting, and kinship in fox squirrels and gray squirrels. J. Mammal. 77, 1006–1016. doi: 10.2307/1382781
Koprowski, J., Munroe, K. E., and Edelman, A. (2016). “Gray not grey: ecology of Sciurus carolinensis in their native range in North America,” in The Grey Squirrel: Ecology and Management of an Invasive Species in Europe, eds C. M. Shuttleworth, P. W. W. Lurz, and J. Gurnell (Bangor: European Squirrel Initiative).
Kummer, H., and Goodall, J. (1985). Conditions of innovative behaviour in primates. Philos. Trans. R. Soc. Lond. B Biol. Sci. 308, 203–214. doi: 10.1098/rstb.1985.0020
Liker, A., and Bókony, V. (2009). Larger groups are more successful in innovative problem solving in house sparrows. Proc. Natl. Acad. Sci. U.S.A. 106, 7893–7898. doi: 10.1073/pnas.0900042106
Lowe, S., Browne, M., Boudjelas, S., and De Poorter, M. (2004). Hundred of the World’s Worst Invasive Alien Species: A Selection from the Global Invasive Species Database. Invasive Species Specialist Group of the IUCN-World Conservation Union. The Invasive Species Specialist Group.
Lowry, H., Lill, A., and Wong, B. B. M. (2013). Behavioural responses of wildlife to urban environments. Biol. Rev. 88, 537–549. doi: 10.1111/brv.12012
Macdonald, I. M. V. (1997). Field experiments on duration and precision of grey and red squirrel spatial memory. Anim. Behav. 54, 879–891. doi: 10.1006/anbe.1996.0528
Makowski, D., Ben-Shachar, M. S., and Lüdecke, D. (2019). bayestestR: describing effects and their uncertainty, existence and significance within the Bayesian framework. J. Open Source Softw. 40:1541. doi: 10.21105/joss.01541
Mangalam, M., and Singh, M. (2013). Flexibility in food extraction techniques in urban free-ranging bonnet macaques, Macaca radiata. PLoS One 8:e85497. doi: 10.1371/journal.pone.0085497
Middleton, A. D. (1930). The ecology of the American grey squirrel (Sciurus carolinensis Gmelin) in the British Isles. Proc. Zool. Soc. Lond. 100, 810–843.
Møller, A. P. (2010). Interspecific variation in fear responses predicts urbanization in birds. Behav. Ecol. 21, 365–371. doi: 10.1093/beheco/arp199
Nicolakakis, N., Sol, D., and Lefebvre, L. (2003). Behavioural flexibility predicts species richness in birds, but not extinction risk. Anim. Behav. 65, 445–452. doi: 10.1006/anbe.2003.2085
Papp, S., Vincze, E., Preiszner, B., Liker, A., and Bókony, V. (2015). A comparison of problem-solving success between urban and rural house sparrows. Behav. Ecol. Sociobiol. 69, 471–480. doi: 10.1007/s00265-014-1859-8
Parker, J. D., Torchin, M. E., Hufbauer, R. A., and Lemoine, N. P. (2013). Do invasive species perform better in their new ranges? Ecology 94, 985–994. doi: 10.1890/12-1810.1
Parker, T. S., and Nilon, C. H. (2008). Grey squirrel density, habitat suitability, and behavior in urban parks. Urban Ecosyst. 11, 243–255. doi: 10.1007/s11252-008-0060-0
Prasher, S., Evans, J. C., Thompson, M. J., and Morand-Ferron, J. (2019). Characterizing innovators: ecological and individual predictors of problem-solving performance. PLoS One 14:e0217464. doi: 10.1371/journal.pone.0217464
Pravosudov, V. V., and Roth, T. C. I. I. (2013). Cognitive ecology of food hoarding: the evolution of spatial memory and the hippocampus. Annu. Rev. Ecol. Evol. Syst. 44, 173–193. doi: 10.1146/annurev-ecolsys-110512-135904
Preiszner, B., Papp, S., Pipoly, I., Seress, G., Vincze, E., Liker, A., et al. (2017). Problem-solving performance and reproductive success of great tits in urban and forest habitats. Anim. Cogn. 20, 53–63. doi: 10.1007/s10071-016-1008-z
Shettleworth, S. J. (1990). Spatial memory in food-storing birds. Philos. Trans. R. Soc. Lond. B Biol. Sci. 329, 143–151. doi: 10.1098/rstb.1990.0159
Signorile, A. L., Lurz, P. W. W., Wang, J., Reuman, D. C., and Carbone, C. (2016). Mixture or mosaic? Genetic patterns in UK grey squirrels support a human-mediated ‘long-jump’ invasion mechanism. Divers. Distribut. 22, 566–577. doi: 10.1111/ddi.12424
Sol, D., Bacher, S., Reader, S. M., and Lefebvre, L. (2008). Brain size predicts the success of mammal species introduced into novel environments. Am. Naturalist 172, (Suppl. 1), S63–S71.
Sol, D., Duncan, R. P., Blackburn, T. M., Cassey, P., and Lefebvre, L. (2005). Big brains, enhanced cognition, and response of birds to novel environments. Proc. Natl. Acad. Sci. U.S.A. 102, 5460–5465. doi: 10.1073/pnas.0408145102
Sol, D., and Lefebvre, L. (2000). Behavioural flexibility predicts invasion success in birds introduced to New Zealand. Oikos 90, 599–605. doi: 10.1034/j.1600-0706.2000.900317.x
Sol, D., Timmermans, S., and Lefebvre, L. (2002). Behavioural flexibility and invasion success in birds. Anim. Behav. 63, 495–502. doi: 10.1006/anbe.2001.1953
van Schaik, C. P. (2013). The costs and benefits of flexibility as an expression of behavioural plasticity: a primate perspective. Philos. Trans. R. Soc. Lond. B Biol. Sci. 368:20120339. doi: 10.1098/rstb.2012.0339
Webb, J. K., Letni, M., Jessop, T. S., and Dempster, T. (2014). Behavioural flexibility allows an invasive vertebrate to survive in a semi-arid environment. Biol. Lett. 10:20131014. doi: 10.1098/rsbl.2013.1014
Wood, D. J. A., Koprowski, J. L., and Lurz, P. W. W. (2007). Tree squirrel introduction: a theoretical approach with population viability analysis. J. Mammal. 88, 1271–1279. doi: 10.1644/06-mamm-a-303.1
Keywords: problem solving, innovation and flexibility, cognitive ecology, invasive rodents, urbanization, invasion biology, behavioral plasticity
Citation: Chow PKY, Clayton NS and Steele MA (2021) Cognitive Performance of Wild Eastern Gray Squirrels (Sciurus carolinensis) in Rural and Urban, Native, and Non-native Environments. Front. Ecol. Evol. 9:615899. doi: 10.3389/fevo.2021.615899
Received: 10 October 2020; Accepted: 01 February 2021;
Published: 26 February 2021.
Edited by:
Simon Ducatez, University of Cambridge, United KingdomReviewed by:
Julie Morand-Ferron, University of Ottawa, CanadaCopyright © 2021 Chow, Clayton and Steele. This is an open-access article distributed under the terms of the Creative Commons Attribution License (CC BY). The use, distribution or reproduction in other forums is permitted, provided the original author(s) and the copyright owner(s) are credited and that the original publication in this journal is cited, in accordance with accepted academic practice. No use, distribution or reproduction is permitted which does not comply with these terms.
*Correspondence: Pizza Ka Yee Chow, cGl6emEuY2hvd0Bvcm4ubXBnLmRl; a3ljMjAyLnBjQGdtYWlsLmNvbQ==
Disclaimer: All claims expressed in this article are solely those of the authors and do not necessarily represent those of their affiliated organizations, or those of the publisher, the editors and the reviewers. Any product that may be evaluated in this article or claim that may be made by its manufacturer is not guaranteed or endorsed by the publisher.
Research integrity at Frontiers
Learn more about the work of our research integrity team to safeguard the quality of each article we publish.