- 1ZooPlantLab, Department of Biotechnologies and Biosciences, University of Milano-Bicocca, Milan, Italy
- 2Quantia Consulting Srl, Milan, Italy
- 3MM Spa, Milan, Italy
- 4Institute of Biomedical Technologies, Italian National Research Council, Milan, Italy
Drinking water quality and safety is strictly regulated and constantly monitored, but little is known about the microorganisms inhabiting drinking water treatment plants (DWTPs). This lack of knowledge prevents optimization of designs and operational controls. Here we investigated the drinking water microbial community harbored by a groundwater-derived DWTP, involving mineral and vegetal granular activated carbon filters (GACs). We used 16S rRNA gene sequencing to analyze water microbiome variations through the potabilization process, considering (i) different GAC materials and (ii) time from GAC regeneration. Our results revealed the predominance of Cand. Patescibacteria, uncultivable bacteria with limited metabolic capacities and small genomes, from source to downstream water. Microbial communities clustered per sampling date, with the noteworthy exception of groundwater samples. If the groundwater microbiome showed no significant variations over time, the community structure of water downstream GACs (both mineral and vegetal) seemed to be affected by time from GAC regeneration. Looking at a finer scale, different GAC material affected microbiome assembly over time with significant variation in the relative abundances of specific taxa. The significance of our research is in identifying the environmental microorganisms intrinsic of deep groundwater and the community shift after the perturbations induced by potabilization processes. Which microorganisms colonize different GACs and become abundant after GACs regeneration and over time is a first step toward advanced control of microbial communities, improving drinking water safety and management of operational costs.
Introduction
Drinking water is the primary source of human sustenance. Unraveling the composition and dynamics underlying the bacterial communities in drinking water sources will allow us to highlight new aspects of the strict interactions occurring between water and humans. Based on this concept, it is clear the need to deeply understand the role that drinking water treatment plants (DWTPs) play in the modulation of the microbial communities.
The recent literature in this field has allowed us to describe the DWTPs as full-fledged engineered ecosystems harboring complex microbial communities (Pinto et al., 2014; Bautista-de los Santos et al., 2016; Prest et al., 2016; Zhang and Liu, 2019) and affecting the lives of 5 billion people using a safely managed drinking water service (United Nations, 2018).
However, while the strict monitoring ensures safety and quality of the distributed drinking water, most of the ecological dynamics behind the perturbations due to potabilization treatments remain to be unveiled. Recently, Zhang et al. (2017) proposed drinking water treatment and distribution processes as ecological disturbances that can be seen in the variation in the microbiome continuum, from groundwater to the tap. Groundwater is often characterized by a very low concentration of microorganisms, mainly constituted by environmental, unculturable prokaryotes (Douterelo et al., 2014; Brown et al., 2015; Bruno et al., 2018), and often characterized by very small dimensions (Luef et al., 2015; Bruno et al., 2017), affecting drinking water characteristics. At the same time, the DWTP peculiar built environment represents a unique microbial habitat shaping the native microbial diversity of pristine water.
The water treatment process consists of a draft phase from different sources (groundwater, lakes, etc.), a treatment phase for the removal of chemical contaminants, and a disinfection phase. These processes are upstream of the last step of distribution to the end-users. It is currently documented that, among these steps, a critical role in the modulation of microbial communities is played by the granular activated carbon (GAC) filtration. GAC filters are often used in the potabilization process in order to carry off natural organic matter and micropollutants and to reduce color and taste. Moreover, they are used for the removal of precursors for disinfection by-products in systems that apply chlorination and for the improvement of the biological stability of treated water. The elimination relies on the adsorption process of GACs, in which molecules are accumulated on the surface of the material composing GAC, thanks to physical and chemical interactions. Influent water quality, flow rate, and contact time also affect the removal efficiency of GAC (Crittenden et al., 2012).
Several studies suggested that bacterial biomass resides and proliferates in GACs (Hammes et al., 2008; Saccani et al., 2014), facilitating the bacterial regrowth (Hammes et al., 2008). New evidence shows that carbon filters harbor a microbial community that differs from the upstream community and that can seed the downstream microbial community (Pinto et al., 2012; Gibert et al., 2013; Bruno et al., 2018). Indeed, bacteria defined as “leaky colonizers” (e.g., Hydrogenophaga, Acidovorax and Denitratisoma) can persist in GAC/sand filters and can affect water downstream shaping the drinking water microbiome (Pinto et al., 2012). Filter-specific occurrence has also been reported (Lautenschlager et al., 2014; Oh et al., 2018; Vignola et al., 2018).
These data support the evidence that the composition of the filters may play a role in the bacterial community composition. Currently though, few studies deeply investigated the role of raw material in affecting the microbial ecosystem inside GACs filters. Activated carbon can be produced from a variety of carbonaceous materials including nutshells, coal, wood, and bone char. The most commonly used activated carbon media are the traditional granular activated carbon, derived from a mixture such as wood or coal, and coconut shell-based granular activated carbon, an emerging activated carbon. Xing et al. (2020) reported that more dissolved organic carbon (DOC) and disinfection by-products (DBPs) precursors were removed by coconut shell-based GACs, meanwhile, less microbes, less metabolites and smaller microbial clusters were detected in the effluent of coconut shell GAC compared with traditional GAC. Nevertheless, the precise dynamics involving the communities colonizing this environment currently remain an open field of research.
Our study investigates the drinking water microbiome harbored by a DWTP involving mineral (coal) and vegetal (coconut shell) GACs thanks to high-throughput DNA sequencing techniques. We focused on the microbial dynamics occurring through the potabilization process, considering in particular (i) different GAC materials and (ii) time from GAC regeneration. Disentangling the structural changes in the microbial composition of the filters is crucial, as they determine the microbiological quality of the filtered water.
Materials and Methods
Study Site and Water Sampling
Water samples were collected from a DWTP in the metropolitan area of Milan (Italy). Milan lies on an alluvial plain and the main source of drinking water is groundwater, which covers an area of about 2000 km2. We can distinguish three stratigraphic units: the deepest (the third aquifer) is about 100 m of depth, it is characterized by low or intermediate permeability sediments (silt and clay, with fractions of sand), and it is confined; the second aquifer is characterized by high or intermediate permeability sediments (gravel and sand with fine fractions and horizons composed by sandy conglomerates), ranging from 30/40 to 100 m of depth; the first aquifer is superficial, with a maximum depth of 40 m, and it is characterized by high permeability sediments (gravel and sand with fractions of silt) (Masetti et al., 2007; De Caro et al., 2017).
The DWTP we selected is one of the most modern MM DWTPs. Located in a large public green area, it came into operation in the autumn of 1980. The DWTP is fed by 21 wells, whose basic flow rate is 840 L/s overall, drawing water mainly from the second aquifer. The current configuration of the treatment scheme consists of a first adsorption phase on GAC filters placed upstream of a disinfection phase with sodium hypochlorite. The first phase is based on the subdivision of the flow pumped by the wells on 20 GACs operating in parallel, of which six of vegetable origin (coconut shell) and 14 of mineral origin (coal) (Supplementary Data S1). The GAC filters are positioned in columns with a diameter of 1.8 m and height of 3 m.
Samples were collected from four different sampling points: (i) at the source from groundwater (GW), (ii) after the passage through the granular activated carbon filters (CF), (iii) after the passage through the granular activated vegetal (based on coconut shell) carbon filters (CF_V), and (iv) bulk water downstream both types of GACs. Regarding carbon filters, we sampled water from different tanks (three from CF and three from CF_V, each time), as illustrated in Figure 1.
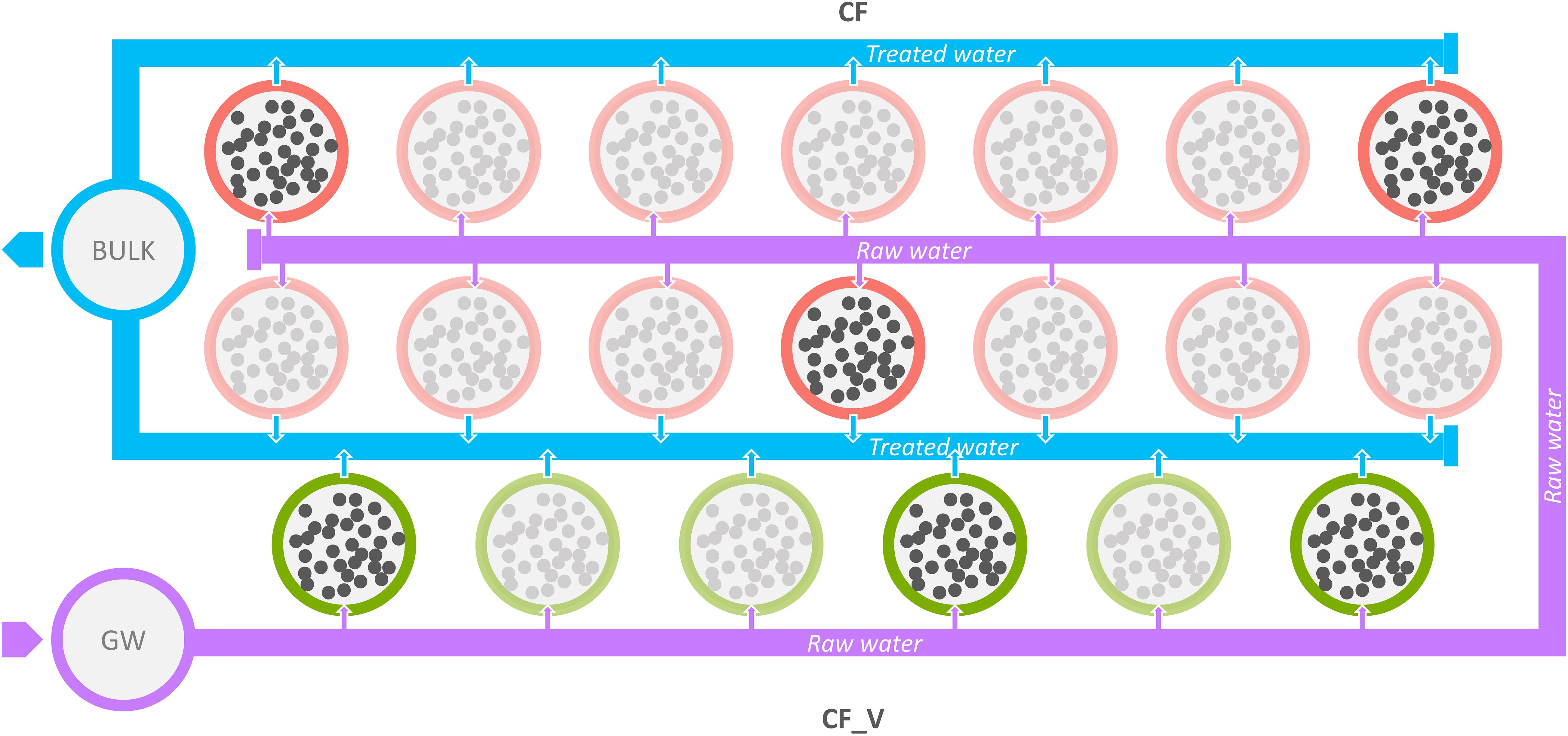
Figure 1. Schematic representation of the sampling points examined. Violet circle: inlet water from groundwater (GW); red circles: mineral GACs (CF); green circles: vegetal GACs (CF_V); light blue circles: bulk water collected from GACs, both vegetal and mineral (BULK); blurred circles indicate not sampled GACs. Raw water flux from groundwater is represented in violet, while the treated (after the passage through GAC filters) water flux is represented in light blue.
The sampling campaign for microbiome analyses started in July 2017 and ended in May 2018, with four sampling events, every 3 months (t1: July 2017, t2: October 2017; t3: February 2018; t4: May 2018).
All samples were collected in aseptic conditions using sterile disposable bottles (LP Italia). Samples were kept at 4°C and in the dark during transport and processed within 24 hours.
Sample Processing and eDNA Extraction
Seven liters of water for each sample were filtered using a vertical filtration apparatus, set up using nitrocellulose filters of diameter = 47 mm and pore size = 0.2 μm (MCE-Membrane Filters Millipore) and a vacuum pump (ME 2 NT vacuubrand). The filtrate was recovered and further concentrated applying a tangential flow filtration system (10K MWCO, Sartorius) in order to collect the smaller fraction of microorganisms, according to Bruno et al., 2017. To increase the biomass, samples belonging to the same sampling point were further concentrated with Vivaspin (10K MWCO, Sartorius) and let absorbed on a nitrocellulose filter. All the procedures were performed in a pre-amplification room under the flow cabinet, with sterilization measures between samples using bleach and UV light. Filters were stored at −80°C until DNA extraction.
Environmental DNA (eDNA) extractions were carried out, according to the same contamination control measures, for both >0.2 μm and <0.2 μm fractions separately. The filters were separated in two halves, of which one was used for DNA extraction. The filters were cut into smaller pieces with sterile instruments, and mechanical and chemical lysis were carried out as described in the protocol of DNeasy PowerSoil Kit (Qiagen); after lysis and wash steps, DNA was eluted in 75 μL of the elution buffer. We included negative controls to verify the absence of contamination during DNA extraction steps.
Library Preparation and High-Throughput DNA Sequencing
Before library preparation, bacterial DNA presence and abundance was measured by qPCR for each sample, using the same primer pairs of amplicon PCR (without overhanging adapters) (Supplementary Data S2). The V3–V4 hypervariable regions of the 16S ribosomal RNA (rRNA) gene were amplified with S-D-Bact-0341-b-S-17, 5′-CCTACGGGNGGCWGCAG-3′ and S-D-Bact-0785-a-A-21, 5′-GACTACHVGGGTATCTAATCC-3′ primer pairs with overhanging adapters, according to the 16S Metagenomic Sequencing Library Preparation protocol (Illumina, SanDiego, CA, United States) with modifications, as described in Bruno et al. (2017) and further improvement due to the low DNA concentrations. In detail, amplicon PCR and index ligation were carried out using PuReTaq Ready-To-Go (RTG) PCR Beads (Cytiva) and reaction volumes modified accordingly. The PCR-clean up step was modified in the final resuspension volume with a twofold increase in the sample concentration.
All the procedures were carried out in the laminar flow cabinet, in order to avoid contamination with exogenous DNA and inter-samples contamination, and in separate rooms for the pre- and post- amplification steps, with dedicated personal protective equipment (PPE). Negative controls were included in library preparation.
All libraries were sequenced on a MiSeq platform (Illumina) in a single 2 × 300 bp paired-end run. The sequencing process was conducted twice by the National Research Council, Institute of Biomedical Technologies (CNR-ITB, Italy) and the Center for Omics Sciences at the IRCCS Ospedale San Raffaele (COSR).
Microbial Composition and Community Structure Analysis
The raw paired-end FASTQ reads were imported into the Quantitative Insights Into Microbial Ecology 2 program (QIIME2, ver. 2020.6; Caporaso et al., 2010; Bolyen et al., 2019) and demultiplexed native plugin. Raw reads were subsequently deposited into the European Nucleotide Archive (ENA) (see Data Availability paragraph). The Divisive Amplicon Denoising Algorithm 2 (DADA2) (Callahan et al., 2016) was used to quality filter, trim, denoise, and mergepairs the data. Chimeric sequences were removed using the consensus method. The taxonomic assignment of the ASVs calculated was carried out using the feature-classifier2 plugin (Bokulich et al., 2018) implemented in QIIME2 against the SILVA SSU non-redundant database (138 release), adopting a consensus confidence threshold of 0.8.
Rarefaction curves were calculated to evaluate if the sequencing efforts generated enough data to well represent the overall microbial diversity in samples. The corresponding plot was generated with the phyloseq R package (McMurdie and Holmes, 2013).
In order to estimate the effect of the different carbon filter treatments, the Faith phylogenetic index (Faith PD) was calculated (Faith, 2016). The Kruskal–Wallis H test for all pairwise tests was used to compare the groups (CF, CF_V, BULK, GW). When multiple tests were applied, we used Benjamini and Hochberg correction, and the obtained q-value is reported in the text.
Paired difference testing between samples from each subject implemented in q2-longitudinal plugin were used to test whether alpha diversity (Faith PD) changed significantly (Bokulich et al., 2018) across different sampling dates for both carbon treatments (CF and CF_V at t1-t2-t3-t4).
Unweighted and weighted UniFrac distances (Lozupone and Knight, 2005) were used to perform the community analyses (beta diversity), evenly sampled at 10,000 reads per sample, using the core-metrics-phylogenetic QIIME2 plugin. Samples with less than this threshold were excluded from the downstream analyses.
Statistical significance among groups (sampling point, sampling date and the interaction between the sampling point and sampling date) was determined by the ADONIS (permutation-based ANOVA, PerMANOVA) test (Anderson, 2005) with 999 permutation-based weighted UniFrac metrics. PerMANOVA Pairwise contrast was performed and the Benjamini-Hochberg FDR correction was used to calculate q-values. The test was performed using beta-group-significance QIIME2 implemented plugin based on the adonis function in vegan R package (Oksanen et al., 2007).
We decided to adopt an ordination approach to explore the structure of microbial communities and specifically, we used Non-metric Multidimensional Scaling (NMDS) implemented in phyloseq R package (McMurdie and Holmes, 2013).
In order to explore the variation of each ASV over time, a Mann-Whitney rank-sum test was carried out between percentage abundance values of all ASVs. For granular activated carbon filters (CF, CF_V), the test was carried out individually for each ASV considering (i) set of abundance values of a specific ASV computed from the samples of the first time point (t1) versus the samples of next time point (t2); (ii) set of abundance values of a specific ASV computed from the samples of t2 versus the samples of the next time point (t3); (iii) set of abundance values of a specific ASV computed from the samples of t3 time point versus the samples of the next time point (t4); (iv) set of abundance values of a specific ASV computed from the samples of t1 versus the samples of t4. The analyses were performed with our own script written in Python3. Pandas (McKinney, 2011), NumPy (Walt et al., 2011), and scipy.stats (Virtanen et al., 2020) libraries were used to build the functions. Variations with a p-value less than 0.05 were reported in a heatmap plot built using matplotlib Python library (Barrett et al., 2005), annotated at the best annotated taxonomic level. In order to allow a neutral evaluation of the abundances variation of the bacteria collected, we did not perform a p-value adjustment.
Drinking Water Monitoring
Microbial and physico-chemical analyses were conducted by the drinking water company MM Spa on water samples according to the Italian law D. Lgs. n. 31 of 2 February 2001 (implementing the European Directive 98/83/CEE). In detail, Escherichia coli and coliform bacteria (EN ISO 9308-1 or EN ISO 9308-2), Enterococci (EN ISO 7899-2), Pseudomonas aeruginosa (EN ISO 16266), and Clostridium perfringens including spores (EN ISO 14189) were monitored. Also, an extensive monitoring from MM Spa of physico-chemical parameters provided for by law generated the data reported in Supplementary Data S3. Chemical data were analyzed by using R (version 3.5.0) and all plots were generated using ggplot ver. 2.0 (Wickham, 2016).
Different variables were taken into consideration: untreated water entering carbon filters (GW), output from mineral filters (CF) and output from vegetal (coconut shell) filters (CF_V). We applied a GLMM (Generalized Linear Mixed effect Model) with negative binomial distribution of the response variable (considering the variable “sampling date” as a random effect), using lme4 R package (Bates et al., 2014).
Results
Water Quality Parameters
MM Spa and ATS (Agenzia di Tutela della Salute della città metropolitana di Milano, the Italian health protection agency for Milan metropolitan area), concurrently with our analyses, carried out the monitoring on drinking water distribution and it resulted free of pathogens and compliant with the standard about drinking water quality (European directive 98/83/CE).
The main chemical and physical measurements conducted by MM Spa are reported in Supplementary Data S3. In particular, the groundwater temperature ranged from 14.4 to 16.4°C, and pH values varied from 7.3 and 7.8.
After the installation of coconut shell-based GACs operating in parallel with traditional GACs, MM Spa extensively monitored in the period from 2015-01-01 to 2016-11-01 the concentration of the main organic contaminants (organic molecules, pesticides and herbicides) before the passage through the filters (GW) and at the exit from the two types of filters, the mineral GACs (CF), and the vegetal GACs (CF_V).
Analyzing the concentration of chloroform, tetrachloroethylene, trichloroethylene and organo-chlorinated compounds dissolved in water, our data indicated that both mineral and vegetal filters have a good capacity to reduce the concentration of organic pollutants (tetrachloroethylene p-value < 2e-16 and organo-chlorinated compounds p-value < 2e-16). Moreover, only in the concentration of trichloroethylene there was a significant difference between the effect of vegetal and mineral filters (p-value = 0.00355), showing a higher concentration of this substance in the water leaving the mineral filters compared to the vegetal ones (Figure 2 and Supplementary Table S1).
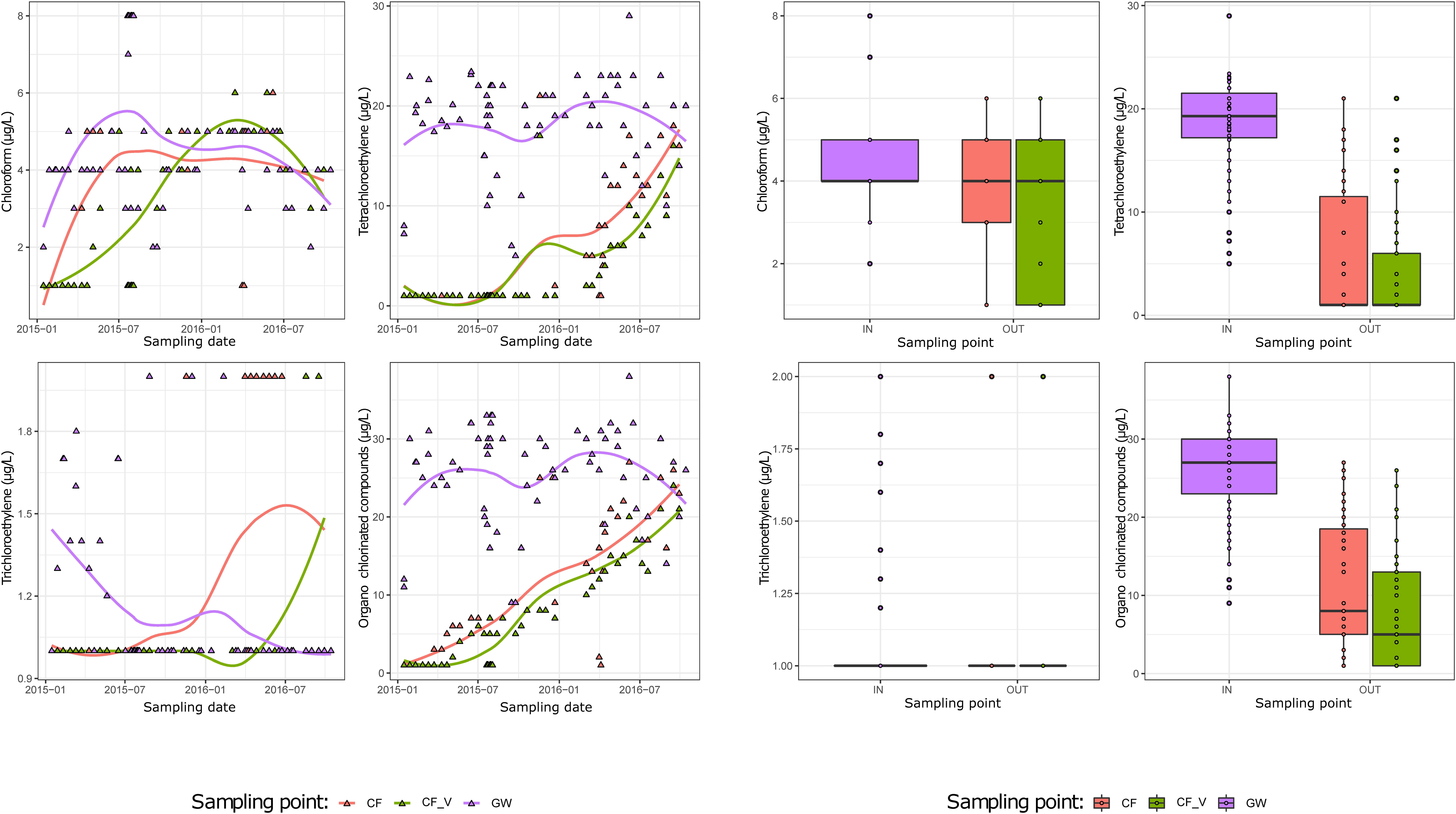
Figure 2. Main organic molecules (chloroform, tetrachloroethylene, trichloroethylene and organo-chlorinated compounds) measured in GW, CF, and CF_V samples, showed per sampling date (left panel) and per sampling point (right panel).
Measuring the concentration of the main pesticides and herbicides (dichlorobenzamide, atrazine, terbuthylazine desethyl, total pesticides, 3,6-dichloropyridazine, tris(2-chlorethyl) phosphate), it emerged that both types of GACs can significantly reduce the concentration of these substances, with the exception of pesticides whose concentration did not vary significantly between untreated and treated (after the passage through GACs) water (Figure 3 and Supplementary Table S2).
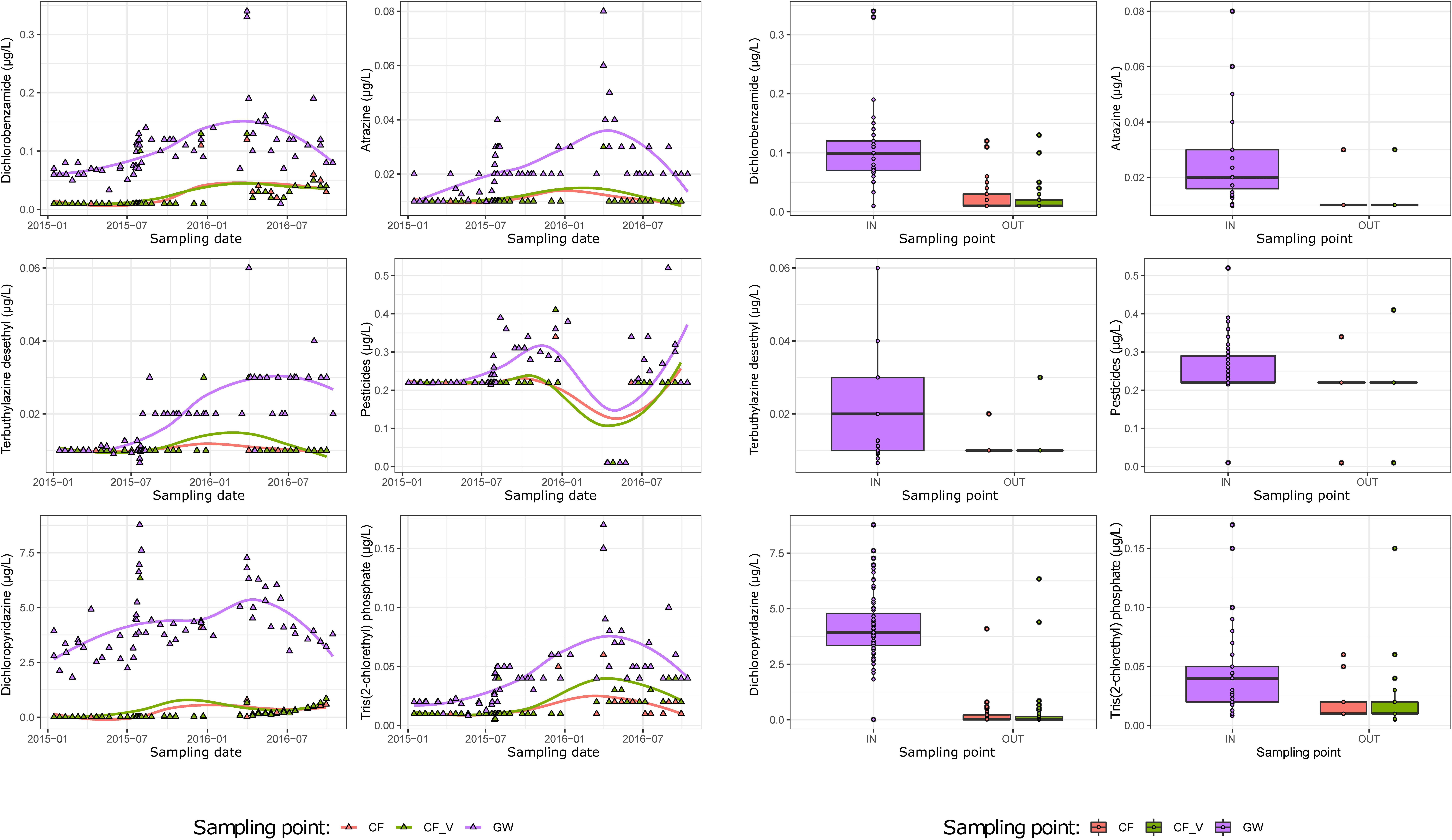
Figure 3. Main pesticides and herbicides (dichlorobenzamide, atrazine, terbuthylazine desethyl, total pesticides, 3,6-dichloropyridazine, tris(2-chlorethyl) phosphate) measured in GW, CF and CF_V samples, showed per sampling date (left panel) and per sampling point (right panel).
Supplementary analyses are described in Supplementary Data S4, Supplementary Table S3, and Supplementary Figure S1.
Sequence Analysis
About 6,290,337 reads were obtained from 33 samples. After quality filtering, merging reads and chimera removal of the two Illumina runs, we got 1,692,048 sequences, with a median frequency of 51,274 reads per sample (were the max number of reads per samples obtained was 104,765 and minimum was 1713). We obtained 5594 ASVs (amplicon sequence variants, Callahan et al., 2017).
Microbiome Diversity Composition and Distribution
An exploratory analysis of bacterial 16S rDNA copies based qPCR showed that, if GW samples had a remarkably low 16S copies/L, no significant differences in 16S rDNA copies existed between CF and CF_V samples (ANOVA, Tukey post hoc test, p-value = 0.42) (Supplementary Table S4 and Supplementary Figure S2). Microbiome biodiversity and composition were evaluated via alpha and beta diversity analyses.
Rarefaction curves showed converging alpha diversity with increasing sequencing depth, confirming sampling effort consistency (Supplementary Figure S3).
Alpha diversity was calculated to evaluate the existence of DWTP component environments and their effects on microbial communities.
Considering all the different sampling points (CF, CF_V, BULK, GW), the Kruskal–Wallis H test based on Faith PD was significant (H = 13.987, p-value = 0.003). The Kruskal–Wallis pairwise test was used to compare the values of alpha diversity among the groups.
The greater diversity was measured between CF and CF_V (H = 8.3, q-value = 0.02) with higher alpha diversity values for CF than for CF_V. Both the carbon filter types were significantly different from the groundwater in terms of microbial diversity, with GW showing higher values of diversity with respect to all the other groups, also with respect to BULK. There was no significant difference in measured species richness between both CF and CF_V and BULK (q-value > 0.05) (Figure 4A and Supplementary Figure S4). Kruskal–Wallis H tests based on Faith PD are reported in Supplementary Table S5. However, we underline that, being n = 3 both for GW and for BULK samples, the H does not fully follow a chi-squared distribution, and the results of the test should be used with caution.
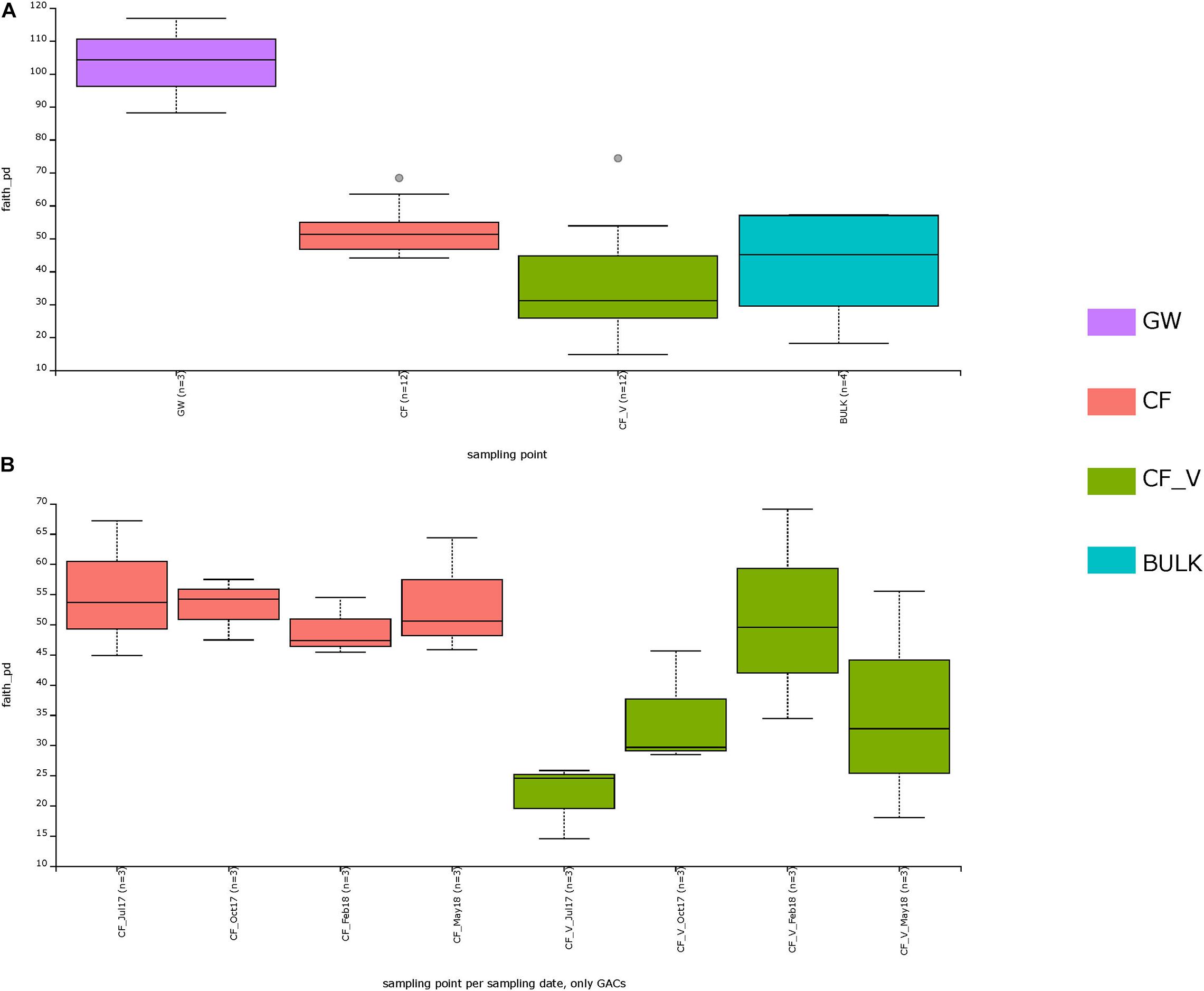
Figure 4. Alpha diversity based on Faith PD metric. In the upper panel (A), sampling points measured diversity was indicated. In the lower panel (B), a focus on GACs (CF and CF_V) for different sampling dates (July17: t1; Oct17: t2; Feb18: t3; May18: t4) was plotted.
Focusing exclusively on GACs, alpha diversity measures based on Faith PD (Supplementary Table S6) exhibited similar values for CF samples in different sampling dates (and q-values > 0.5), in contrast to CF_V samples, which showed increasing values from the first to the third sampling date (t1–t3) and then a decrease in the last sampling date (t4) (Figure 4B).
After taxonomy assignment, we identified 48 bacterial phyla and 107 classes (plus seven Archaea phyla and nine Archaea classes) (Supplementary Data S5).
Taxonomic analysis revealed that most of the sequences in all the samples were associated with the phyla Patescibacteria (46%) and Proteobacteria (26%). Patescibacteria was the most abundant phylum in GW samples (23%), as well as in CF (51%), CF_V (47%), and BULK (40%) samples. Interestingly, members (Diapherotrites, Aenigmarchaeota, Nanoarchaeota) of the Archaea DPANN superphylum accounted for about 9% of GW reads, 2% of CF reads, 1% CF_V reads, 2% of BULK reads.
Looking inside Class rank, we found members belonging to Parcubacteria (Patescibacteria, 35%), followed by GammaProteobacteria (Proteobacteria, 20%), ABY1 (Patescibacteria, 7%) and AlphaProteobacteria (Proteobacteria, 6%); 0.1% of sequences resulted in Unassigned.
Focusing on specific ASVs, we observed that the most abundant ASV was assigned to Candidatus Nomurabacteria (Patescibacteria). A microorganism belonging to Nitrosopumilaceae (Crenarchaeota, Archaea) was detected in 31 out of 33 samples, as well as Candidatus Omnitrophus (Verrucomicrobia), bacteriap25 (Myxococcota), and Woesearchaeales (Nanoarchaeota, Archaea).
N97 - MIX_NOV_GW, deriving from the smaller fraction (<0.2 μm) of GW samples, was constituted by 100% Candidatus Nomurabacteria, possibly an ultra-small bacterium (10 reads, not considered in further analyses).
Groundwater samples were characterized by the predominance (>3.5%) of Omnitrophaceae, Woesearchaeales, Leptospirillaceae, Parcubacteria, ABY1. Mineral carbon filters samples showed a switch in composition, and the most abundant families identified belonged to Candidatus Adlerbacteria, Candidatus Nomurabacteria, Gallionellaceae, Comamonadaceae, Xanthobacteraceae, Beggiatoaceae, Candidatus Uhrbacteria, Bdellovibrionaceae. A similar behavior was observed in vegetal carbon filters: the most abundant families reported were assigned to Candidatus Nomurabacteria, Acidiferrobacteraceae, Candidatus Adlerbacteria, Comamonadaceae, Xanthobacteraceae, Gallionellaceae, Candidatus Uhrbacteria. In downstream GACs bulk, Candidatus Nomurabacteria, Candidatus Adlerbacteria, Simkaniaceae, Candidatus Uhrbacteria, Gallionellaceae, Obscuribacteraceae, Xanthobacteraceae, Comamonadaceae, Omnitrophaceae represented the most abundant families (in the case of candidate phyla, we reported the most accurate taxonomic assignment).
Bar chart representation highlights the distribution of the 50 most abundant ASV across sampling points, assigned to the taxonomic rank of Family (Figure 5).
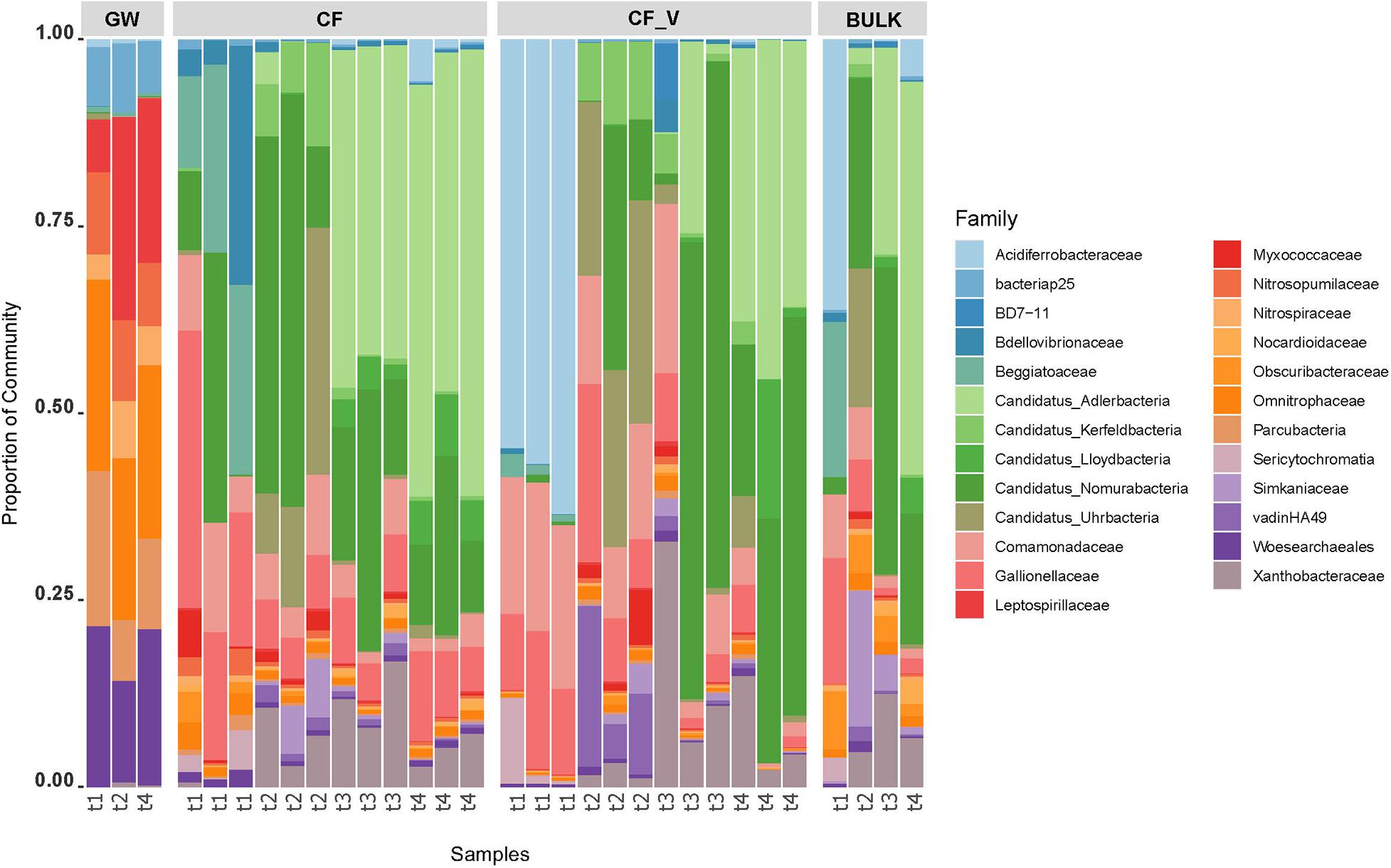
Figure 5. Barchart visualization depicting the relative abundance and distribution of the most abundant ASVs assigned to the Family taxonomic rank. GW: groundwater samples; CF_V: samples collected after the passage through granular activated vegetal carbon filters; CF: samples collected after the passage through granular activated vegetal carbon filters; BULK: downstream bulk GACs samples; t1–t4: sampling dates.
Noteworthy, a temporal trend was observed in the relative abundances of some microbial taxa. The first sampling date (t1, July 2017, after GACs renewal) was characterized by the presence of Acidiferrobacteraceae in vegetal GACs, whereas Beggiatoaceae family was predominant in mineral GACs, and then both dropped in the later dates. If Candidatus Adlerbacteria and Xhantobacteraceae showed an increase in relative abundance in GACs over time from the second sampling date, during the whole sampling campaign, other taxa seemed to increase in relative abundances in GACs only in the second sampling date (Candidatus Kerfeldbacteria, Candidatus Uhrbacteria). Sericytochromatia showed a higher relative abundance in the first sampling date in GACs and BULK samples.
To better explore the microbial differences among sampling points, we computed beta diversity metrics (unweighted and weighted UniFrac) and generated Non-metric Multidimensional Scaling (NMDS) plots. Global ADONIS test was significant for both unweighted and weighted UniFrac metrics (complete results for all tests performed are reported in Supplementary Tables S7–S12). Our analyses showed a clear cluster representing the GW samples, for both the metrics considered (Figure 6). The result is supported by the higher values of pseudo-F obtained in the comparison between the GW samples and the other samples, both from a qualitative and quantitative point of view (Weighted UniFrac: CF-GW pseudo-F = 10.52705, p-value = 0.009; CF_V-GW pseudo-F = 6.242822, p-value = 0.009; BULK-GW pseudo-F = 8.271299, p-value = 0.052. Unweighted UniFrac: CF-GW pseudo-F = 4.335064, p-value = 0.0075; CF_V-GW pseudo-F = 3.985224, p-value = 0.006; BULK-GW pseudo-F = 2.956413, p-value = 0.042).
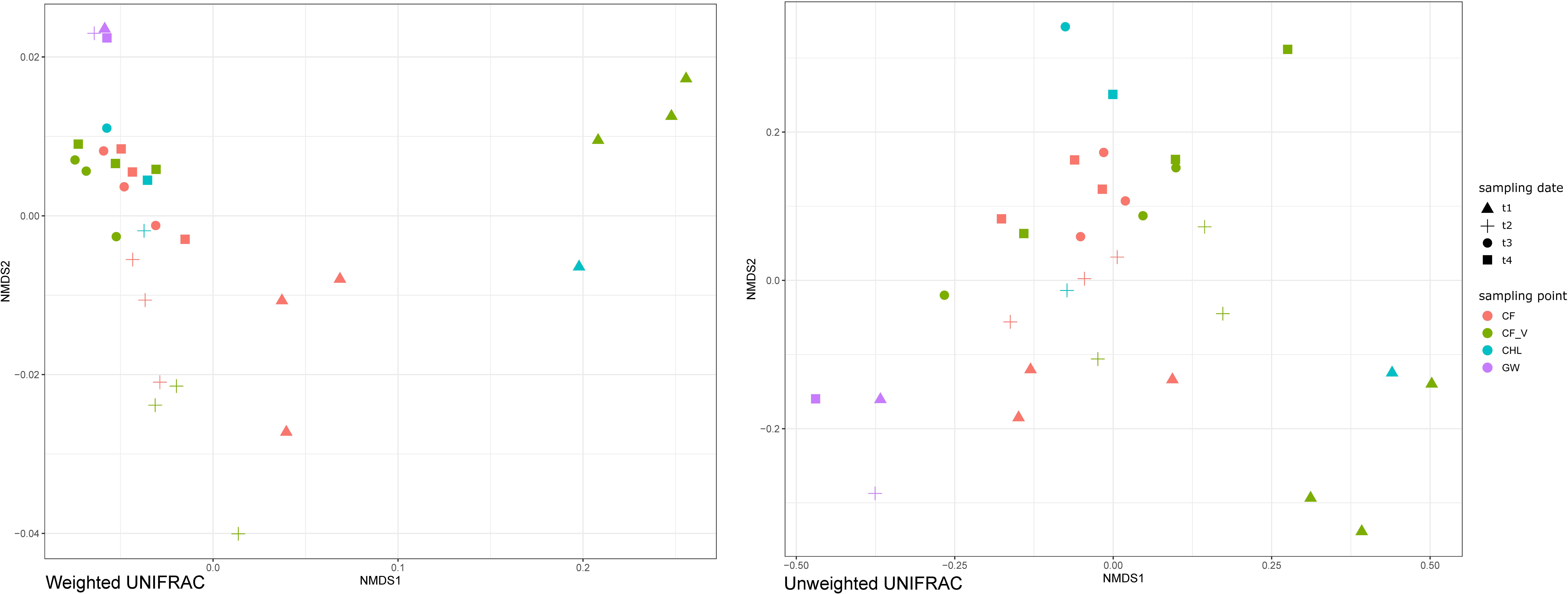
Figure 6. NMDS based on weighted and unweighted UniFrac distance metric. Water samples were colored by sampling point (GW: groundwater; CF: mineral GACs; CF_V: vegetal GACs; BULK: downstream GACs samples); shapes indicate sampling date (t1–t4).
Regarding the comparison between the two different types of carbon, no significant difference emerged between CV and CF_V considering the quantitative aspect (p-value > 0.005). Only from the qualitative analysis a low but significant variation appeared between the two groups (Unweighted UniFrac pseudo F = 2.379772, p-value = 0.006).
Our analyses showed that there was a significant effect of time that emerged from both metrics. One small specific cluster was constituted by samples collected after the passage of mineral GACs (CF) of the first sampling date (July 2017), together with the corresponding BULK sample (Figure 6). When we focused our attention exclusively on GACs samples during different sampling points, pairwise comparisons showed no significant difference among samples (Supplementary Tables S11, S12).
GACs Differential Abundances Over Time
Thus, to figure out the contribution of specific taxa to the GAC microenvironment over time, we evaluated the differential abundances of ASVs, which significantly differ between sampling dates, considering mineral and vegetal GACs. To do that, we calculated the significant variations in relative abundances between July 2017 and October 2017 (t2-1), October 2017 and February 2018 (t3-2), February 2018 and May 2018 (t4-3), July 2017 and May 2018 (t4-1). All the p-values obtained are reported in Supplementary Data S6. In general, we noted that different ASVs belonging to the same phylogenetic group showed different trends (Supplementary Figure S5). Focusing on Patescibacteria phylum, four ASVs assigned to Candidatus Adlerbacteria significantly diminished in the second sampling date compared to the first one (t2-1), and a significant increase was evident in the subsequent time span (t3-2) for two of these ASVs. Conversely, a different ASV assigned to the same taxon showed the same behavior in mineral GACs. Considering Candidatus Kerfeldbacteria, Candidatus Nomurabacteria, Candidatus Peregrinibacteria, and Candidatus Uhrbacteria ASVs, we could see a similar heterogeneity in temporal variation in the same taxonomic rank, with significant variations in GACs in different time spans for different ASVs. Taking into account other phyla, we observed a comparable behavior. Candidatus Omnitropus (Omnitrophaceae, Verrucomicrobia), highly abundant in all the groundwater samples (9% of GW reads), exhibited a not uniform temporal dynamic, considering different ASVs. However, eight out of twenty-one ASVs had an overall (considering the temporal range from July 2017 to May 2018, t4-1) significant increase in mineral GACs, in spite of one ASV significantly increasing for the vegetal ones for t4-1. Looking inside the Proteobacteria phylum and focusing on the two families that appeared to have a pattern in vegetal versus mineral GACs (i.e., Beggiaotoaceae and Acidiferrobacteraceae), we reported that several ASVs significantly contributed to the GACs microbial composition in a not homogenous way. Two Acidiferrobacteraceae ASVs significantly decreased in CF_V in t2-1, thus contributing to the peculiar temporal pattern of the vegetal GACs. In Beggiatoaceae family, only one ASV significantly decreased in mineral GACs in t2-1. Considering Xanthobacteraceae, only one ASVs showed a significant differential abundance, with an increase in t3-2 in mineral GACs. One specific ASV (out of two) belonging to Rokubacteriales (Methylomirabilota) showed a significant decrease in mineral GACs for all the sampling time spans considered, but in vegetal GACs never had substantial variation in relative abundance.
Overall, if we sum the contribution of positive and negative differential abundances of ASVs for each time span, considering mineral and vegetal GACs, we can gather differences in the temporal trends between the two types of carbon filters (Figure 7). Mineral GAC had higher variation in the positive and negative values (t2-1: + 25, -24; t3-2: + 51, -14; t4-3: + 29, -38 significant ASVs) of differential abundances than vegetal GAC. Vegetal GAC, after the first time span, which had a strong drop in ASVs with a significant differential abundance (t2-1: + 9, -40), exhibited a certain stability in the following time spans (t3-2: + 29, -4; t4-3: + 20, -1).

Figure 7. Significant differential ASVs abundances considering mineral GACs (CF) and vegetal GACs (CF_V), comparing different sampling dates. Positive and negative variations are shown.
Finally, to resume the variations in relative abundances that significantly occurred from the starting condition to the end of the sampling campaign, we computed the ASVs that were significantly enriched or diminished in vegetal and mineral carbon filters from t1 to t4 (t4-1). Candidatus Adlerbacteria and Candidatus Nomurabacteria had a significant decrease in vegetal GACs, whereas 17 different ASVs, plus Candidatus Adlerbacteria, in mineral GACs. The enriched ASVs in mineral GACs from t1 to t4 were 58, 28 in vegetal GACs, with in common eleven taxa. Noteworthy, CF_V unique ASVs were assigned to Acidiferrobacteraceae (uncultured), Leptospirillaceae (Leptospirillum), Comamonadaceae (unassigned), Bdellovibrionaceae (Bdellovibrio), Parachlamydiaceae (unassigned) and Candidatus Nomurabacteria. Conversely, CF unique ASVs were assigned to 30 different taxa and mainly belonged to Rokubacteriales, Blfdi19 (Myxococcota), Paracaedibacteraceae, Elusimicrobiota, Nanoarchaeota, Patescibacteria, Omnitrophaceae, Woesearchaeales, Caenarcaniphilales (Melainabacteria), Parcubacteria, Legionellaceae, Obscuribacteraceae, Candidatus Staskawiczbacteria, Candidatus Yanofskybacteria. No shared taxa were found between the groups of significantly enriched and significantly diminished, in vegetal GACs (Supplementary Data S6).
Discussion
Groundwater-Derived Drinking Water Biodiversity at a Glance
Groundwater ecosystem is characterized by low oxygen levels plus lack of sunlight and external energy sources. Microorganisms inhabiting this ecosystem strongly affect nutrient cycling by turning over matter and energy, showing a strong impact in water quality downstream (Kirchman, 2012; Long et al., 2016).
High-throughput DNA sequencing techniques, not relying on cultivation methods, have allowed the uncovering of previously unknown microorganisms, recalcitrant to growth in laboratory conditions. Among them, the candidate bacterial superphylum Patescibacteria (also known as Candidate Phyla Radiation, CPR) and archaeal superphylum DPANN (Diapherotrites, Parvarchaeota, Aenigmarchaeota, Nanoarchaeota, and Nanohaloarchaeota, Castelle and Banfield, 2018) are noticeable examples. However, the relevant expansion of our view of the tree of life (Brown et al., 2015; Hug et al., 2016; Dombrowski et al., 2019) included microorganisms from subsurface and groundwater environments still far from being completely understood. Metagenomic data (Luef et al., 2015; Castelle and Banfield, 2018) suggested they had limited metabolic capacities, thus probably taking advantage of other microorganisms for complete redox transformations, suggesting a possible syntrophic nature of their interactions (a sort of “metabolic handoff”). Beam et al. (2020) hypothesized that, rather than be solely attributable to symbiotic lifestyle, the divergent coding potential, small genomes and small cell sizes of Patescibacteria and DPANN may be a result of an ancestral, primitive energy metabolism based on fermentation. The limited metabolic potential of Patescibacteria and DPANN, not only could provide an explanation of the difficulties to cultivate them in laboratory conditions, but also highlight the interdependencies among microorganisms in these ecosystems. The presence of DPANN, especially in GW samples (9%), and the striking dominance in our sequencing data of Patescibacteria, from the source water to downstream water (46% of total reads), agrees with our previous studies (Bruno et al., 2018) on a different DWTP in the same area (43% of total reads). Remarkably, the primers we used for 16S-based sequencing, even if showing a certain grade of affinity for Archaea sequences, were designed to amplify the bacterial domain. Thus, the presence of Archaea sequences was not completely unexpected, but it deserves further investigation. The presence of a vast majority of unculturable environmental microorganisms shifts the focus of the investigations from target opportunistic pathogens to the overall microbial community: deciphering microbial dynamics in DWTPs is rather challenging and it involves the understanding of the complete picture of the ecology of this environment. The persistence of opportunistic pathogens in DWTP despite disinfection practices highlights the limitation of bacterial indicators, and strengthens the need of implementing omics analyses to differentiate “healthy”/“unhealthy” microbiome signatures (Zhang and Liu, 2019). Moreover, the putative presence of ultra-small bacteria deserves further studies, given the possible co-occurrence relationships among microorganisms in this ecosystem. Recent studies (Herrmann et al., 2019) suggested that the predominance of Patescibacteria in groundwater is caused by their preferential mobilization from soils and flourishing under oligotrophic conditions. Probably, groundwater seeds in turn carbon filters and such high abundance of Patescibacteria in GACs could find explanation on the particular microenvironment, and probably in the carbon filters characteristics of surface area and porosity.
Due to the oligotrophic environment, which selected the microbial communities inhabiting groundwaters, stressors applied to this peculiar ecosystem, such as potabilization treatments, can have a strong impact on microbial community structure, with downstream effects to water characteristics. This is what we inferred from HTS results: groundwater samples clustered together in NMDS plot, with no influence of sampling date, suggesting a stable condition over time and an equilibrium in the nutrients cycle. On the other hand, GACs samples (both mineral and vegetal) seemed to be affected by time, with changes in microbial community balances throughout the sampling campaign. It is necessary to investigate if this shift was due to the need of different metabolic functions in relation to the filter substrate changes over time.
GAC Microenvironment Under the Lens
There is growing agreement in the role exerted by filtration in the microbial community structure, seeding water downstream (Pinto et al., 2012; Gibert et al., 2013; Bruno et al., 2018; Oh et al., 2018) and a clear demonstration that filter medium is a deterministic factor that can drive the assembly of different microbial communities (Vignola et al., 2018).
Our previous data (Bruno et al., 2018) suggested an increase in bacterial DNA copies in GACs, supporting the role of GAC in harboring a microbial community able to seed water downstream. Nevertheless, in vitro cultivation of these environmental bacteria was not conclusive in this and in the previous sampling campaign. This was not completely unexpected, in the light of sequencing results, showing a microbial community composed by a large amount of uncultivable bacteria.
If our exploratory analyses based on 16S rDNA qPCR quantification showed no significant differences in bacterial load between vegetal and mineral GAC, our data all converged in depicting the subtle but relevant differences in vegetal and mineral GACs. Although time (sampling date) appeared to be the main trigger of GACs (and downstream bulk, as a consequence) microbiota, analyzing the data in depth, differences in abundances of specific taxa and in the “colonization dynamics” can be seen considering different GAC raw material. Time-dependent colonization exhibited its effects on the different alpha-diversity trends considering different raw materials, as well on the differences in relative abundances of specific taxa.
Our result showed differential abundances of Acidiferrobacteraceae and Beggiatoaceae families, considering vegetal and mineral carbon filters of the first sampling date (after carbon filters renewal, July 2017): Acidiferrobacteraceae were enriched in the vegetal filters, while Beggiatoaceae in the mineral ones, without being mutually exclusive. Moreover, both were present in the downstream bulk sample of the same sampling date.
Acidiferrobacteraceae are a family recently described belonging to GammaProteobacteria (Kojima et al., 2015). They are autotroph, sulfur-oxidizing bacteria via the SOX system. Recently, the presence of dissimilatory sulfate reduction and sulfide oxidation genes were also reported (Umezawa et al., 2016; Wegner et al., 2019). On the other hand, Beggiatoaceae are large nitrate-accumulating, sulfur-oxidizing bacteria that span the range from obligate sulfur-based chemolithoautotrophy to heterotrophic growth supplemented by sulfur oxidation (Teske and Salman, 2014). Some taxa are capable of both denitrification and dissimilatory nitrate reduction to ammonium (Schutte et al., 2018). Beggiatoaceae thrive in microbial mats, surficial sediments, and sediment–water interfaces since they require access to reduced sulfur species and oxidants such as oxygen or nitrate (Teske and Salman, 2014). This could explain the presence of members of the Beggiatoaceae family in granular filters, where the water-carbon interface has a wide area and the substrates are available.
The shift in composition that we clearly observed over time can be due to the changing conditions of the GAC microenvironment, because of the accumulation of organic compounds and/or the competition with other taxonomic groups. To a lesser extent, other groups showed temporal variations, probably participating in the dynamic equilibrium of nutrient cycles. Members belonging to the Gallionellaceae family, which have been reported mainly in GACs, could play a role in oxidative processes from Fe3+ to ferric hydroxide (Fe(OH)3) during autotrophic growth and therefore be involved in carbon and iron cycles. AmongProteobacteria, we also detected ASVs assigned to the Xanthobacteraceae family. These chemoheterotrophic aerobic bacteria were found mainly in treated water (downstream GAC filtration) and significantly enriched in t2 of vegetal GACs. Since the nitrogen fixation pathway is found in many species and some species are capable of growing on unusual substrates, such as alkenes, halogenated aromatic and aliphatic compounds, terpenes, thiophenes, and polyaromatic compounds and can be found in various environments (soil, water, polluted sites) (Oren, 2014), the changed environmental conditions may have favored the growth of members of this family.
The characteristic of being predators of Gram-negative bacteria is known from the Bdellovibrionaceae (Pineiro et al., 2004), one of the most abundant families in GACs.
Furthermore, the presence of the only recently described phylum of Rokubacteria (Methylomirabilota), a group phylogenetically close to the Nitrospirae, was detected and exhibited a significant increase uniquely in mineral GACs, from t1 to t4. Unlike Nitrospirae, however, Rokubacteria genomes are large (6–8 Mbps), with a high GC percentage (66–71%) and potentially a mixotrophic metabolism (both autotrophic and heterotrophic), despite the small cell size (0.3–0.4 μm; the smallest bacteria, belonging to the CPR, measure 0.15–0.2 μm). The combination of a large genome, which codes for a “generalist” metabolism, in an oligotrophic environment, and the small size of the organism, makes these bacteria unique. Furthermore, their high intra-phylum genetic heterogeneity is another unique characteristic (Becraft et al., 2017).
On the whole, our results revealed an overall similar microbial community comparing vegetal and mineral GACs. This is in agreement with the chemical analyses carried out during this sampling campaign and in the past, which indicated a similar efficiency in removing the main chemical contaminants. Nevertheless, trichloroethylene concentration showed an improved reduction after the passage through vegetal GACs. Moreover, previous data (Crippa, 2016) suggested that vegetal GACs had improved removal efficiencies of chloroform and tetrachloroethylene, in terms of filter life before the breakthrough time was reached.
Organohalide-respiring bacteria (OHRB) are involved in the biodegradation of organohalides, such as trichloroethylene, and are distributed among several distinct phyla (ranging from Chloroflexi to Firmicutes and Proteobacteria) (Löffler et al., 2013; Chen et al., 2020). In our samples, no OHRB sequences were found, but sequences assigned to Dehalococcoidia SAR202 clade (phylum Chloroflexi) were reported in all but one GACs samples (<0.6% for each sample) with no significant differences in relative abundances. Recent studies (Patil et al., 2014; Yu et al., 2016; Jin et al., 2020) indicated that non-Dehalococcoides Chloroflexi may play a role in the microbial dechlorination processes, but further investigations are needed to clarify all the actors involved in these pathways.
Noteworthy, microbial analyses highlighted finer scale differences and different colonization dynamics, indicating a higher stability of vegetal GACs compared to mineral GACs, in terms of relative abundances of specific taxa after the initial filter colonization. Coconut shells are a renewable resource made of high-grade carbon and they are ideal for filtration due to their high percentage of micro-pores on their surface, making it the most promising option for removing a wide variety of particles and pollutants.
Considering environmental and economic impact for the DWTP examined, the use of vegetal GAC instead of mineral GAC was estimated in an average reduction of environmental impacts of about 20% and in economic savings (Crippa, 2016). Given these evidences, it is worth pursuing the microbial dynamics in filters considering the properties of raw material, such as high specific surface area (i.e., porosity) and specific chemical surface characteristics including hydrophobicity and presence of functional groups (Crittenden et al., 2012; Knezev, 2015).
As a final remark, molecular techniques allowed us to take a set of snapshots depicting the microbial community structure in this peculiar ecosystem. Further efforts are needed to critically address the issues of microbial live/dead ratio and microbial functions, overcoming methodological biases and current challenges that actually limit their application (for an exhaustive discussion see Zhang and Liu, 2019). Implementing bacterial activity measurements in drinking water research and monitoring will provide new perspectives and cope with current and future needs.
Conclusion
The water samples we tested resulted free of pathogens, according to the European Directive 98/83/CEE. In order to assure drinking water high quality and to efficiently manage water treatments, however, it is imperative to accept that DWTP are complex ecosystems, harboring an astounding biodiversity of microorganisms. Knowing their behavior and understanding their dynamics is pivotal to maintain the intrinsic biodiversity at the equilibrium and avoid opportunistic pathogens overgrowth (Read et al., 2011; Proctor and Hammes, 2015). Indeed, the functional potential of drinking water microbiome could be exploited to improve water treatment efficiency.
Our data depicted the peculiar groundwater-derived microbiome underlying Milan metropolitan area and used for drinking water distribution. Patescibacteria candidate superphylum, characterized by limited metabolic capacities and small genomes, dominate raw water and water passed through GACs, in agreement with our previous studies (Bruno et al., 2017, 2018). Moreover, our results suggested that a strong link between microbial population traits and the specific environment in which they grow exists. Due to the molecular techniques applied in our analysis, the viability of the microorganisms detected cannot be proved and their metabolic activity cannot be measured. However, HTS results revealed that if time-dependent colonization of regenerated GACs has a significant effect on microbial community structure, GACs material shapes the microbial community of DWTP at a finer scale: the nature of the filter material has an impact on the microbial community (and probably activity) in the filter niche. We suppose that deterministic factors, such as niche differentiation and competition, together with stochastic factors (Sloan et al., 2006; Vignola et al., 2018), could be the drivers of the complex dynamics occurring in GACs.
In conclusion, two main elements seem to have a role in the downstream water quality: inlet water characteristics, with the presence of Patescibacteria, seeding water downstream; and time-dependent changes of GACs water microbiome. Looking at a deeper level, also filter raw material affects microbiome assembly over time with significant variation in the relative abundances of specific taxa. This could provide the chance to purposely engineered filter microbial communities, to produce biologically stable drinking water.
Data Availability Statement
The datasets generated for this study can be found in the European Nucleotide Archive (ENA) (http://www.ebi.ac.uk/ena/data/view/PRJEB40727).
Author Contributions
AB, AS, MB, BP, MC, and ML conceived and designed the experiments. AB, DM, CC, and TC performed the experiments. AS and DM analyzed the data. AB, AS, and DM drafted the manuscript and figures. All authors contributed to the revision of the final manuscript.
Funding
The research reported in this publication was supported by funding from Fondazione Cariplo, grant no. 2015-0275.
Conflict of Interest
AS was employed by Quantia Consulting Srl. MB and BP were employed by MM Spa.
The remaining authors declare that the research was conducted in the absence of any commercial or financial relationships that could be construed as a potential conflict of interest.
Acknowledgments
We thank Dejan Lazarevic and Fabio Simeoni (COSR) for their invaluable support in sequencing. We also would like to thank the Bicocca cEnter of Science and Technology for FOOD (BEST4FOOD) of the University of Milano-Bicocca, Milan, Italy.
Supplementary Material
The Supplementary Material for this article can be found online at: https://www.frontiersin.org/articles/10.3389/fevo.2021.615513/full#supplementary-material
References
Anderson, M. J. (2005). PERMANOVA: A FORTRAN Computer Program for Permutational Multivariate Analysis of Variance. Auckland: University of Auckland.
Barrett, P., Hunter, J., Miller, J. T., Hsu, J. C., and Greenfield, P. (2005). “matplotlib–a portable python plotting package,” in Astronomical Data analysis Software and Systems XIV ASP Conference Series, Vol. 347, eds P. L. Shopbell, M. C. Britton, and R. Ebert (San Francisco: Astronomical Society of the Pacific), 91.
Bates, D., Mächler, M., Bolker, B., and Walker, S. (2014). Fitting linear mixed-effects models using lme4. arXiv [Preprint]. arXiv:1406.5823.
Bautista-de los Santos, Q. M., Schroeder, J. L., Sevillano-Rivera, M. C., Sungthong, R., Ijaz, U. Z., Sloan, W. T., et al. (2016). Emerging investigators series: microbial communities in full-scale drinking water distribution systems–a meta-analysis. Environ. Sci. Water Res. 2, 631–644. doi: 10.1039/c6ew00030d
Beam, J. P., Becraft, E. D., Brown, J. M., Schulz, F. P., Jarett, J. K., Bezuidt, O., et al. (2020). Ancestral absence of electron transport chains in Patescibacteria and DPANN. Front. Microbiol. 10:1848. doi: 10.3389/fmicb.2020.01848
Becraft, E. D., Woyke, T., Jarett, J., Ivanova, N., Godoy-Vitorino, F., Poulton, N., et al. (2017). Rokubacteria: genomic giants among the uncultured bacterial phyla. Front. Microbiol. 8:2264. doi: 10.3389/fmicb.2017.02264
Bokulich, N. A., Kaehler, B. D., Rideout, J. R., Dillon, M., Bolyen, E., Knight, R., et al. (2018). Optimizing taxonomic classification of marker-gene amplicon sequences with QIIME 2’s q2-feature-classifier plugin. Microbiome 6:90. doi: 10.1186/s40168-018-0470-z
Bolyen, E., Rideout, J. R., Dillon, M. R., Bokulich, N. A., Abnet, C. C., Al-Ghalith, G. A., et al. (2019). Reproducible, interactive, scalable and extensible microbiome data science using QIIME 2. Nat. Biotechnol. 37, 852–857. doi: 10.1038/s41587-019-0209-9
Brown, C. T., Hug, L. A., Thomas, B. C., Sharon, I., Castelle, C. J., Singh, A., et al. (2015). Unusual biology across a group comprising more than 15% of domain bacteria. Nature 523, 208–211. doi: 10.1038/nature14486
Bruno, A., Sandionigi, A., Bernasconi, M., Panio, A., Labra, M., and Casiraghi, M. (2018). Changes in the drinking water microbiome: effects of water treatments along the flow of two drinking water treatment plants in a urbanized area, Milan (Italy). Front. Microbiol. 9:2557. doi: 10.3389/fmicb.2018.02557
Bruno, A., Sandionigi, A., Rizzi, E., Bernasconi, M., Vicario, S., Galimberti, A., et al. (2017). Exploring the under-investigated “microbial dark matter” of drinking water treatment plants. Sci. Rep. 14:44350. doi: 10.1038/srep3044350
Callahan, B. J., McMurdie, P. J., and Holmes, S. P. (2017). Exact sequence variants should replace operational taxonomic units in marker-gene data analysis. ISME J. 11, 2639–2643. doi: 10.1038/ismej.2017.119
Callahan, B. J., McMurdie, P. J., Rosen, M. J., Han, A. W., Johnson, A. J. A., and Holmes, S. P. (2016). DADA2: high-resolution sample inference from illumina amplicon data. Nat. Methods 13, 581–583. doi: 10.1038/nmeth.3869
Caporaso, J. G., Kuczynski, J., Stombaugh, J., Bittinger, K., Bushman, F. D., Costello, E. K., et al. (2010). QIIME allows analysis of high-throughput community sequencing data. Nat. Methods 7, 335–336. doi: 10.1038/nmeth.f.303
Castelle, C. J., and Banfield, J. F. (2018). Major new microbial groups expand diversity and alter our understanding of the tree of life. Cell 172, 1181–1197. doi: 10.1016/j.cell.2018.02.016
Chen, W. Y., Wu, J. H., and Chu, S. C. (2020). Deciphering microbiomes in anaerobic reactors with superior trichloroethylene dechlorination performance at low pH conditions. Environ. Pollut. 257:113567. doi: 10.1016/j.envpol.2019.113567
Crippa, F. (2016). Adsorbimento su Carbone Attivo Granulare di Microinquinanti Organici: Simulazione di Processo e Ottimizzazione Delle Prestazioni. Available online at: http://hdl.handle.net/10589/120084 (accessed September 4, 2020).
Crittenden, J. C., Trussell, R. R., Hand, D. W., Howe, K. J., and Tchobanoglous, G. (2012). MWH’s Water Treatment: Principles and Design. Hoboken, NJ: John Wiley & Sons. doi: 10.1002/9781118131473
De Caro, M., Crosta, G. B., and Frattini, P. (2017). Hydrogeochemical characterization and natural background levels in urbanized areas: milan metropolitan area (Northern Italy). J. Hydrol. 547, 455–473. doi: 10.1016/j.jhydrol.2017.02.025
Dombrowski, N., Lee, J. H., Williams, T. A., Offre, P., and Spang, A. (2019). Genomic diversity, lifestyles and evolutionary origins of DPANN archaea. FEMS Microbiol. Lett. 366:fnz008. doi: 10.1093/femsle/fnz008
Douterelo, I., Boxall, J. B., Deines, P., Sekar, R., Fish, K. E., and Biggs, C. A. (2014). Methodological approaches for studying the microbial ecology of drinking water distribution systems. Water Res. 65, 134–156. doi: 10.1016/j.watres.2014.07.008
Faith, D. P. (2016). “The PD phylogenetic diversity framework: linking evolutionary history to feature diversity for biodiversity conservation,” in Biodiversity Conservation and Phylogenetic Systematics, eds R. Pellens and P. Grandcolas (Cham: Springer), 39–56. doi: 10.1007/978-3-319-22461-9
Gibert, O., Lefèvre, B., Fernández, M., Bernat, X., Paraira, M., Calderer, M., et al. (2013). Characterising biofilm development on granular activated carbon used for drinking water production. Water Res. 47, 1101–1110. doi: 10.1016/j.watres.2012.11.026
Hammes, F., Berney, M., Wang, Y., Vital, M., Köster, O., and Egli, T. (2008). Flow-cytometric total bacterial cell counts as a descriptive microbiological parameter for drinking water treatment processes. Water Res. 42, 269–277. doi: 10.1016/j.watres.2007.07.009
Herrmann, M., Wegner, C. E., Taubert, M., Geesink, P., Lehmann, K., Yan, L., et al. (2019). Predominance of cand. Patescibacteria in groundwater is caused by their preferential mobilization from soils and flourishing under oligotrophic conditions. Front. Microbiol. 10:1407. doi: 10.3389/fmicb.2019.01407
Hug, L. A., Baker, B. J., Anantharaman, K., Brown, C. T., Probst, A. J., Castelle, C. J., et al. (2016). A new view of the tree of life. Nat. Microbiol. 1:16048. doi: 10.1038/nmicrobiol.2016.48
Jin, D., Zhang, F., Shi, Y., Kong, X., Xie, Y., Du, X., et al. (2020). Diversity of bacteria and archaea in the groundwater contaminated by chlorinated solvents undergoing natural attenuation. Environ. Res. 185:10945. doi: 10.1016/j.envres.2020.109457
Kirchman, D. L. (2012). Degradation of Organic Material. Processes in Microbial Ecology. New York, NY: Oxford University Press Inc, 79–98.
Knezev, A. (2015). Microbial Activity in Granular Activated Carbon Filters in Drinking Water Treatment. Ph.D. Thesis, Wageningen University, Wageningen.
Kojima, H., Shinohara, A., and Fukui, M. (2015). Sulfurifustis variabilis gen. nov., sp. nov., a sulfur oxidizer isolated from a lake, and proposal of Acidiferrobacteraceae fam. nov. and Acidiferrobacterales ord. nov. Int. J. Syst. Evol. Micr. 65, 3709–3713. doi: 10.1099/ijsem.0.000479
Lautenschlager, K., Hwang, C., Ling, F., Liu, W. T., Boon, N., Köster, O., et al. (2014). Abundance and composition of indigenous bacterial communities in a multi-step biofiltration-based drinking water treatment plant. Water Res. 62, 40–52. doi: 10.1016/j.watres.2014.05.035
Löffler, F. E., Yan, J., Ritalahti, K. M., Adrian, L., Edwards, E. A., Konstantinidis, K. T., et al. (2013). Dehalococcoides mccartyi gen. nov., sp. nov., obligately organohalide-respiring anaerobic bacteria relevant to halogen cycling and bioremediation, belong to a novel bacterial class, Dehalococcoidia classis nov., order Dehalococcoidales ord. nov. and family Dehalococcoidaceae fam. nov., within the phylum Chloroflexi. Int. J. Syst. Evol. Micr. 63, 625–635. doi: 10.1099/ijs.0.034926-0
Long, P. E., Williams, K. H., Hubbard, S. S., and Banfield, J. F. (2016). Microbial metagenomics reveals climate-relevant subsurface biogeochemical processes. Trends Microbiol. 24, 600–610. doi: 10.1016/j.tim.2016.04.006
Lozupone, C., and Knight, R. (2005). UniFrac: a new phylogenetic method for comparing microbial communities. Appl. Environ. Microb. 71, 8228–8235. doi: 10.1128/AEM.71.12.8228-8235.2005
Luef, B., Frischkorn, K. R., Wrighton, K. C., Holman, H. Y. N., Birarda, G., Thomas, B. C., et al. (2015). Diverse uncultivated ultra-small bacterial cells in groundwater. Nat. Commun. 6:6372. doi: 10.1036/ncomms7372
Masetti, M., Poli, S., and Sterlacchini, S. (2007). The use of the weights-of-evidence modeling technique to estimate the vulnerability of groundwater to nitrate contamination. Nat. Resour. Res. 16, 109–119. doi: 10.1007/s11053-007-9045-6
McKinney, W. (2011). “pandas: a foundational python library for data analysis and statistics,” in Python for High Performance and Scientific Computing, Vol. 14, eds W. Scullin and N. Banglawala (Rochester, NY: University of Rochester). doi: 10.1007/978-1-4842-5839-2_5
McMurdie, P. J., and Holmes, S. (2013). phyloseq: an R package for reproducible interactive analysis and graphics of microbiome census data. PLoS One 8:e61217. doi: 10.1371/journal.pone.0061217
Oh, S., Hammes, F., and Liu, W. T. (2018). Metagenomic characterization of biofilter microbial communities in a full-scale drinking water treatment plant. Water Res. 128, 278–285. doi: 10.1016/j.watres.2017.10.054
Oksanen, J., Kindt, R., Legendre, P., O’Hara, B., Stevens, M. H. H., Oksanen, M. J., et al. (2007). The vegan package. Community Ecol. Package 10:719.
Oren, A. (2014). “The family Xanthobacteraceae,” in The Prokaryotes, eds E. Rosenberg, E. F. DeLong, S. Lory, E. Stackebrandt, and F. Thompson (Berlin: Springer), doi: 10.1007/978-3-642-30197-1_258
Patil, S. S., Adetutu, E. M., Rochow, J., Mitchell, J. G., and Ball, A. S. (2014). Sustainable remediation: electrochemically assisted microbial dechlorination of tetrachloroethene-contaminated groundwater. Microb. Biotechnol. 7, 54–63. doi: 10.1111/1751-7915.12089
Pineiro, S. A., Sahaniuk, G. E., Romberg, E., and Williams, H. N. (2004). Predation pattern and phylogenetic analysis of Bdellovibrionaceae from the Great Salt Lake, Utah. Curr. Microbiol. 48, 113–117. doi: 10.1007/s00284-003-4136-z
Pinto, A. J., Schroeder, J., Lunn, M., Sloan, W., and Raskin, L. (2014). Spatial-temporal survey and occupancy-abundance modeling to predict bacterial community dynamics in the drinking water microbiome. mBio 5:e01135–14. doi: 10.1128/mBio.01135-14
Pinto, A. J., Xi, C., and Raskin, L. (2012). Bacterial community structure in the drinking water microbiome is governed by filtration processes. Environ. Sci. Technol. 46, 8851–8859. doi: 10.1021/es302042t
Prest, E. I., Weissbrodt, D. G., Hammes, F., Van Loosdrecht, M. C. M., and Vrouwenvelder, J. S. (2016). Long-term bacterial dynamics in a full-scale drinking water distribution system. PLoS One 11:e0164445. doi: 10.1371/journal.pone.0164445
Proctor, C. R., and Hammes, F. (2015). Drinking water microbiology—from measurement to management. Curr. Opin. Biotech. 33, 87–94. doi: 10.1016/j.copbio.2014.12.014
Read, S., Marzorati, M., Guimarães, B. C., and Boon, N. (2011). Microbial resource management revisited: successful parameters and new concepts. Appl. Microbiol. Biot. 90:861. doi: 10.1007/s00253-011-3223-5
Saccani, G., Bernasconi, M., and Antonelli, M. (2014). Optimization of low energy sonication treatment for granular activated carbon colonizing biomass assessment. Environ. Technol. 35, 851–858. doi: 10.1080/09593330.2013.853698
Schutte, C. A., Teske, A., MacGregor, B. J., Salman-Carvalho, V., Lavik, G., Hach, P., et al. (2018). Filamentous giant Beggiatoaceae from the Guaymas Basin are capable of both denitrification and dissimilatory nitrate reduction to ammonium. Appl. Environ. Microb. 84:e02860–17. doi: 10.1128/AEM.02860-17
Sloan, W. T., Lunn, M., Woodcock, S., Head, I. M., Nee, S., and Curtis, T. P. (2006). Quantifying the roles of immigration and chance in shaping prokaryote community structure. Environ. Microbiol. 8, 732–740. doi: 10.1111/j.1462-2920.2005.00956.x
Teske, A., and Salman, V. (2014). “The family Beggiatoaceae,” in The Prokaryotes, eds E. Rosenberg, E. F. DeLong, S. Lory, E. Stackebrandt, and F. Thompson (Berlin: Springer), doi: 10.1007/978-3-642-38922-1_290
Umezawa, K., Watanabe, T., Miura, A., Kojima, H., and Fukui, M. (2016). The complete genome sequences of sulfur-oxidizing Gammaproteobacteria Sulfurifustis variabilis skN76T and Sulfuricaulis limicola HA5T. Stand. Genomic Sci. 11:71. doi: 10.1186/s40793-016-0196-0
United Nations (2018). Sustainable Development Goal 6 Synthesis Report on Water and Sanitation. New York, NY: United Nations, 10017.
Vignola, M., Werner, D., Wade, M. J., Meynet, P., and Davenport, R. J. (2018). Medium shapes the microbial community of water filters with implications for effluent quality. Water Res. 129, 499–508. doi: 10.1016/j.watres.2017.09.042
Virtanen, P., Gommers, R., Oliphant, T. E., Haberland, M., Reddy, T., Cournapeau, D., et al. (2020). SciPy 1.0: fundamental algorithms for scientific computing in Python. Nat. Methods 17, 261–272. doi: 10.1038/s41592-019-0686-2
Walt, S. V. D., Colbert, S. C., and Varoquaux, G. (2011). The NumPy array: a structure for efficient numerical computation. Comput. Sci. Eng. 13, 22–30. doi: 10.1109/MCSE.2011.37
Wegner, C. E., Gaspar, M., Geesink, P., Herrmann, M., Marz, M., and Küsel, K. (2019). Biogeochemical regimes in shallow aquifers reflect the metabolic coupling of the elements nitrogen, sulfur, and carbon. Appl. Environ. Microbiol. 85:e02346–18. doi: 10.1128/AEM.02346-18
Xing, X., Li, T., Bi, Z., Qi, P., Li, Z., Wang, H., et al. (2020). Efficient removal of disinfection by-products precursors and inhibition of bacterial detachment by strong interaction of EPS with coconut shell activated carbon in ozone/biofiltration. J. Hazard. Mater. 392:122077. doi: 10.1016/j.jhazmat.2020.122077
Yu, J., Park, Y., Nguyen, V. K., and Lee, T. (2016). PCE dechlorination by non-Dehalococcoides in a microbial electrochemical system. J. Ind. Microbiol. Biot. 43, 1095–1103. doi: 10.1007/s10295-016-1791-1
Zhang, Y., and Liu, W. T. (2019). The application of molecular tools to study the drinking water microbiome–current understanding and future needs. Crit. Rev. Environ. Sci. Technol. 49, 1188–1235. doi: 10.1080/10643389.2019.1571351
Keywords: drinking water, microbiome, HTS, Patescibacteria, granular activated carbon
Citation: Bruno A, Sandionigi A, Magnani D, Bernasconi M, Pannuzzo B, Consolandi C, Camboni T, Labra M and Casiraghi M (2021) Different Effects of Mineral Versus Vegetal Granular Activated Carbon Filters on the Microbial Community Composition of a Drinking Water Treatment Plant. Front. Ecol. Evol. 9:615513. doi: 10.3389/fevo.2021.615513
Received: 09 October 2020; Accepted: 01 March 2021;
Published: 25 March 2021.
Edited by:
Denis Baurain, University of Liège, BelgiumReviewed by:
Bernard Taminiau, University of Liège, BelgiumLuigimaria Borruso, Free University of Bozen-Bolzano, Italy
Copyright © 2021 Bruno, Sandionigi, Magnani, Bernasconi, Pannuzzo, Consolandi, Camboni, Labra and Casiraghi. This is an open-access article distributed under the terms of the Creative Commons Attribution License (CC BY). The use, distribution or reproduction in other forums is permitted, provided the original author(s) and the copyright owner(s) are credited and that the original publication in this journal is cited, in accordance with accepted academic practice. No use, distribution or reproduction is permitted which does not comply with these terms.
*Correspondence: Antonia Bruno, YW50b25pYS5icnVub0B1bmltaWIuaXQ=