- 1Criminalistics Direction, National Institute of Criminalistics and Criminology, Algiers, Algeria
- 2School of Health and Life Sciences, Teesside University, Middlesbrough, United Kingdom
- 3National Horizons Centre, Darlington, United Kingdom
- 4School of Pharmacy and Biomolecular Sciences, Liverpool John Moores University, Liverpool, United Kingdom
Introducing animal carbon-source to soil initiates biochemical and microbial processes that lead to its decomposition and recycling, which subsequently cause successional shifts in soil microbial community. To investigate the use of soil microbial community to inform criminal investigation, this study was designed to mimic clandestine graves. It compared the decomposition of stillborn piglets (Sus scrofa domesticus), as human analogues, to oak (Quercus robur) leaf litter and soil-only controls outdoors for 720 days. Environmental and edaphic parameters were monitored and showed soil microbial community alignment with temperature seasonality, which highlighted the importance of this abiotic factor. Denaturing gradient gel electrophoresis (DGGE) data were used to calculate Hill numbers and diversity indices of the bacterial 16S rRNA community did not distinguish mammalian- from plant-based decomposition consistently during the first or second year of the study. In contrast, the fungal 18S rRNA community allowed clear differentiation between different treatments (beta diversity) throughout the 720-day experiment and suggested the moment of the decomposing mammalian skin rupture. 16S rRNA-based NGS facilitated the identification of e.g., Pirellulaceae, Acidobacteria ii1-15_order and Candidatus xiphinematobacter as Year 2 bacterial markers of gravesoil at family, order and species taxonomic levels, respectively, and confirmed the similarity of the calculated Hill diversity metrics with those derived from DGGE profiling. Parallel soil elemental composition was measured by portable X-ray Fluorescence where calcium profiles for the piglet-associated soils were distinct from those without carrion. Also, soil calcium content and PMI correlated positively during the first year then negatively during the second. This study is one of the first to apply a multidisciplinary approach based on molecular and physicochemical analytical techniques to assess decomposition. It highlights the recognised potential of using soil microbial community in forensic investigations and provides a proof-of-concept for the application of a combined molecular and elemental approach to further understand the dynamics of decomposition. In addition, it sets the scene for further research in different conditions based on Hill numbers metrics instead of the classic ecological indices for soil necrobiome richness, diversity and evenness.
Introduction
Death is the beginning of a series of changes in the body leading to its decomposition through two major processes, autolysis and putrefaction (Shirley et al., 2011). Decomposition involves internal changes to the body together with the action of endogenous and exogenous organisms (Pinheiro, 2006). Researchers divided this environment-dependent process (Cockle and Bell, 2017) into different stages (Megyesi et al., 2005; Goff, 2009). Although these are a convenient means for decomposition stage assessment, they are not always discernible as their supposedly distinctive characteristics usually blend with one another (Pinheiro, 2006; Knobel et al., 2018). Also, decomposition rates vary significantly depending on parameters related to: (i) the environment, such as location, atmospheric conditions and presence of clothing (Voss et al., 2011); (ii) exposure to exogenous organisms such as microbes, insects, plants (Iyengar and Hadi, 2014) and scavenging animals (Behrensmeyer, 1978); (iii) funeral care including embalming, wrapping, type of burial and coffin (Pinheiro, 2006; Tumer et al., 2013; Hau et al., 2014; Lauber et al., 2014); and (iv) cadaver physical condition which includes the circumstances of death, medical treatment, and antemortem and perimortem physical conditions of the deceased (Sutherland et al., 2013).
Various animals such as different species of birds, reptiles, fish, rodents, and mammals have been used for decomposition studies as human taphonomic proxies, with swine and murine models used the most (Ralebitso-Senior and Olakanye, 2018). Domestic pigs (Sus scrofa domesticus), in particular, have high anatomical, physiological, and immunological similarities with humans (Litten-Brown et al., 2010). Also, there is similarity between swine and human cadavers in terms of decomposition where putrefaction progresses at approximately the same rate to that of human bodies of comparable weight (Campobasso et al., 2001) although their body parts surface areas to volume ratios differ (Connor et al., 2018). Without access to donated human bodies and relevant ethical guidelines, animal proxies remain the best alternative to study decomposition and develop forensic investigative tools (Ralebitso-Senior and Pyle, 2018; Williams et al., 2019).
Microorganisms play significant roles in human decomposition (Bisker and Ralebitso-Senior, 2018; Bishop, 2019) and heavily affect the decomposition rate; hence Lauber et al. (2014) reported a two to three times faster decomposition in mice placed on non-sterile soil compared to those deposited on sterile soil. In addition, there is evidence that microorganisms are essential to cadaver organic residue recycling although more knowledge is required about the structure of the associated microbial communities. Furthermore, Cobaugh et al. (2015) reported the persistence of human-associated microbial species in soil, which are very distinct and scarce in nature thus making them possible biomarkers of human decomposition. Ultimately, microbial communities associated with gravesoil have the potential to be used as a tool to estimate time since death and locate clandestine burials (Metcalf et al., 2013, 2016; Finley et al., 2015a; Olakanye et al., 2015, 2017).
Denaturing gradient gel electrophoresis (DGGE) is an economical and reliable method used in many laboratories around the world, with the generated data analysed further by calculating appropriate ecological indices for richness, diversity and evenness. To date, most published research on characterisation of soil microbial communities for forensic applications have used classic diversity metrics such as Shannon-Wiener, Simpson index of concentration or those built within software/pipeline packages (Can et al., 2014; Olakanye et al., 2017; Olakanye and Ralebitso-Senior, 2018; Procopio et al., 2019). However, these metrics have known shortcomings and generate results that are not easy to interpret. In contrast, Hill numbers (0D, 1D, 2D and Hill ratio 1D/0D) are equivalent to algebraic transformation of most other indices, and quantify diversity in units of modified species counts. For example, unlike Simpson's index of concentration, they preserve the doubling property and extract ecological meaning from complex data (Chao et al., 2014) allowing better interpretation of results (Chao et al., 2010). Next generation sequencing as a high throughput metagenome profiling technique has been applied widely in forensic microbial ecology studies. Its application is being improved further where processed sequencing data are analysed using the novel high-resolution approach that targets amplicon sequence variants (ASVs) instead of operational taxonomic units (OTUs) (Bolyen et al., 2018), and relevant statistical analysis.
Soil analysis in forensic investigation has evolved considerably with various inorganic and organic techniques used. While these techniques help inform investigations, their use varies from simple and practical analysis such as portable XRF (pXRF) and spectrometry that could be performed in situ by crime scene investigators, to more technical methods requiring highly qualified staff or necessitating laboratory work (chromatography and molecular analysis). Since it is a rapid, non-destructive, simultaneous multi-element technique (Shand and Wendler, 2014), pXRF is readily available in forensic laboratories and used widely for routine analysis of trace evidence and artefacts. This makes the expansion of its use to complement microbial community profiling of gravesoil within forensic microbial ecology, viable and cost-effective for forensic units. pXRF is affected by factors such as soil moisture content and heterogeneity hence the importance of sample preparation prior to analysis (Ravansari and Lemke, 2018).
In order to enrich the knowledge base about carrion decomposition with the northeast of England as an example context, the following hypotheses were developed:
A. The subsurface decomposition of domestic piglet (S. scrofa domesticus) as a human analogue will change the surrounding soil microbial community composition distinctly from the subsurface decomposition of Quercus robur (oak leaves).
B. Soil microbial community signature associated with the decomposition of S. scrofa domesticus will persist after the decomposition of the animal proxy.
C. The composition of soil microbial community associated with decomposition is influenced by seasonal weather changes.
D. Soil elemental composition is influenced by the decomposing resource (animal vs. vegetal) and type of burial.
To test these hypotheses and explore the changes in soil microbial community biodiversity, a 2-year study was set to imitate clandestine graves. It consisted of in situ burials of piglets (Sus scrofa domesticus) as human cadaver proxies and oak (Quercus robur) leaf litter, with soil-only controls. Key atmospheric and edaphic variables, such as temperature, pH, humidity, and elemental composition were measured. Portable X-Ray Fluorescence was used to analyse the elemental composition during decomposition to illustrate the importance of physicochemical characterisation of gravesoil to complement microbial ecology techniques in a forensic context. The study aimed to enrich the knowledge base on subsurface decomposition using molecular microbial ecology methods supported by secondary analyses and environmental monitoring. In the long-term, this could enhance the forensic toolkit for post-mortem/post-burial interval (PMI/PBI) estimation and location of clandestine graves, and ultimately inform investigations and facilitate court proceedings.
Materials and Methods
Experimental Design
The open air 36 m2 study ecosystem (North Yorkshire, U.K., Lat. 53.762551°N; Long. 1.244004°W) was a woodland characterised by tree clusters of predominately oak (Quercus robur) and as loamy soil composed of clay (22%), silt (32%) and sand (46%) (Olakanye et al., 2017). It was used for forestry agriculture and not a human taphonomic facility. Therefore, there were no expected legacy effects as the ecosystem has, to our knowledge, never been used for decomposition research. To mitigate for lateral migration between pits while ensuring even spatial distribution, the study area was divided into three sections containing three pits each, which were dug ~1.9 to 2.25 m apart (Supplementary Figure 1). Two sets of three pits each were used as triplicate piglet cadaver and oak (Quercus robur) leaf litter burials, respectively, with the remaining set used for soil-only controls. To minimise scavenging activity by vertebrates, each piglet was placed in a mesh wire cage prior to burial. All nine graves were monitored at monthly intervals for the first 12 months (December 2014–December 2015; Olakanye et al., 2017) then up to 24 months (January 2016–December 2016; current report) for soil temperature, pH, and microbial (bacterial and fungal) communities. Soil core samples were collected at 20–60 cm deep from all four sides of each pit using a stainless-steel soil corer (Eijkelkamp, Netherlands), which was cleaned after each sampling with water and 99.9% (v/v) ethanol (Thermo Fisher Scientific, Loughbrough, UK). The samples were then transported to the laboratory in a cold storage box containing ice packs (ca 4°C), and combined for each pit in 25 ml sterile universal tubes prior to storage at −20°C.
Environmental Monitoring
Data for rainfall, UV index, sun exposure, cloud coverage, humidity and daily atmospheric temperatures were obtained from www.worldweatheronline.co.uk. Soil temperature was recorded at each point of sampling using a Fisher Scientific portable thermometer (Fisher Scientific, Loughborough, U.K.) and further expressed in Accumulated degree-days (ADD) (Nolan et al., 2019). The ADD for each day was calculated by adding together the average positive temperatures of each day from the start of the experiment. Similarly, cumulative precipitation was calculated for each day and consisted of the rainfall (mm) accumulated between the first (01/12/2014) and last (01/12/2016) days of the study.
The average pH of each soil sample was measured electrochemically using a Hanna pH 213 microprocessor (Hanna Instruments, Bedfordshire, UK). Each soil sample (10 g) was mixed with deionised water in a 1:5 (w/v) ratio and left to settle for 30 min prior to measurement as described by Stokes et al. (2009).
Soil Mineral Composition
Mineral composition of all soil samples was assessed by portable X-Ray Fluorescence (pXRF) using a portable Niton XL3 950 GOLDD+ series Analyser (Niton UK Limited, Winchester, U.K.). To quantify the content for phosphorus, potassium, iron, calcium, magnesium and sulphur (Hopkins et al., 2000; Carter and Tibbett, 2006; Elfstrand et al., 2007; Benninger et al., 2008), soil samples were dried overnight at 105°C, ground with a mortar and pestle, sieved to 2 mm and homogenised. XRF cups (31 mm) were filled with ~8 g of homogenised soil from each individual treatment and covered with 5 μm polypropylene film (Williams et al., 2020) before scanning at 30 s low and 60 s light beam using mining mode. Blank and standard samples were run after every 10 samples analysed. The limit of detection of pXRF used for the standard reference materials (SRM) is: P (0.03%), S (0.01%), and Mg (0.65%).
DNA Extraction
DNA was extracted from each soil sample (500 mg) from all 2 years of the study using FastDNA™ Spin Kit for Soil™ (MP Biomedicals, U.K.) according to the manufacturer's instructions and stored at −20°C until required. The concentration and purity (A260/A280) of each DNA extract was determined by UV spectrophotometry (Synergy-HT microplate reader; Biotek, Bedfordshire, UK) where a ratio of 1.8–2.0 was equivalent to high quality and high purity DNA (Torsvik, 1980; Hart et al., 2015).
Polymerase Chain Reaction (PCR) and Agarose Gel Electrophoresis
DNA extracts were amplified on a Primus 96 Plus thermocycler (MWG Biotech AG, Ebersberg, Germany) using Promega 2X Master Mix containing Taq DNA polymerase (50 U ml–1), dATP (400 μM), dGTP (400 μM), dCTP (400 μM), dTTP (400 μM), MgCl2 (3 mM), and BSA (10 mg ml−1). The V3 region of the 16S rRNA gene (location 341–534) was enzymatically amplified in the PCR using the nested primer set GC388f (5′-CGC CCG CCG CGC GCG GCG GGC GGG GCG GGG GCA CGG GGG GCC TAC GGG AGG CAG CAG-3′) and 530r (5′-ATT ACC GCG GCT GCT GG-3′) according to Muyzer et al. (1993).
Fungal 18S rRNA gene was amplified using the nested primer sets NS1f (5′- CCA GTA GTC ATA TGC TTG TC-3′) and NS8r (5′-TCC GCA GGT TCA CCT ACG GA-3′) for the first reaction, then NS1f and NS210-GCr (5′-CGC CCG CCG CGC GCG GCG GGC GGG GCG GGG GCA CGG GGG G GAA TTA CCG CGG CTG CTG GC-3′) as described by White et al. (1990). The thermal cycling conditions used were as described by Olakanye et al. (2015). Each of the 16S rRNA and 18S rRNA gene PCR amplicons were checked using a 1.5% (w/v) agarose gel electrophoresis with 1X TBE buffer and SYBR Safe (Invitrogen) staining.
Denaturing Gradient Gel Electrophoresis
Each of the bacterial 16S rRNA and fungal 18S rRNA gene amplicons (17 μl) were mixed with 5X loading buffer (3 μl) and electrophoresed on 10 and 8% (w/v) polyacrylamide gels (acrylamide/bis-acrylamide 37.5:1) using a 35–65% and 15–40% denaturing gradient, respectively. Electrophoresis was performed on a DCODE™ Universal Mutation Detection System (Bio-Rad, Hertfordshire, UK) at 60°C/110V for 16 h. The gels were observed under UV light on an Alpha Imager HP® (Alpha Innotech, Braintree, UK) after SYBR Gold (Invitrogen) staining.
Next-Generation Sequencing (NGS)
The DNA extracts from the first year of the in situ piglet study were processed, analysed and reported as Olakanye et al. (2017). The DNA extracts from both the first and second years of study were sequenced identically on Illumina MiSeq platform by NU-Omics (Northumbria University, Newcastle Upon Tyne, UK). The preparation and sequencing of 16S rRNA gene sequence libraries was completed according to Kozich et al. (2013). The V4 region of the bacterial 16S rRNA gene was targeted using the primers 515f (5′-GTGCCAGCMGCCGCGGTAA-3′) and 806r (5′-GGACTACHVGGGTWTCTAAT-3′) (Kozich et al., 2013; Guo et al., 2016; Pechal et al., 2018). Year 1 samples were analysed for operational taxonomic units (OTUs) with amplicon sequence variants (ASVs) assessed for year 2. Therefore, comparisons for NGS findings are made with some precaution.
Data and Statistical Analysis
pXRF readings were performed separately for each individual replicate with Microsoft Office ExcelTM Professional Plus 2016 used subsequently to calculate the averages for each set of triplicate readings, and plot graphs. Phoretix 1D software (TotalLab, Newcastle, U.K.) was used to analyse DGGE images and quantify the microbial community fingerprints (Ritz et al., 2004; Chimutsa et al., 2015). Hill numbers (0D, 1D, 2D and ratio 1D/0D), as measures of ecological diversity (Chao et al., 2010, 2014), were calculated using Equations 1–4 in Microsoft Office ExcelTM Professional Plus 2016 based on the number and volume of the DGGE gel bands (OTU) or the NGS taxonomic classification at species level (ASV):
Hill number 0D (taxa richness):
Hill number 1D (common taxa diversity):
where Pi: proportion; H': Shannon-Wiener index.
Hill number 2D (dominant taxa diversity):
Hill ratio (community evenness):
All data were evaluated by a two-way ANOVA with replication followed by Tukey HSD and Bonferroni to explore the interactions using IBM® SPSS® Statistics version 25 (IBM Corporation, New York, USA).
For multivariate analysis, data were unit vector normalised, mean centred, and scaled to standard deviation. These data were then subjected to principal component analysis (PCA) to highlight correlations between weather conditions, and demonstrate the differences between fungal and bacterial ecological indices against environmental parameters. Principal co-ordinates analysis (PCoA) identified differences in bacterial community beta-diversity in response to seasonal shifts.
The raw sequencing reads resulting from next-generation sequencing of year 2 DNA extracts, which are the focus of the current manuscript, were analysed using the Quantitative Insights Into Microbial Ecology version 2 (QIIME2) software suite version 2018.8 (Bolyen et al., 2018; Hall and Beiko, 2018; Nearing et al., 2018). Denoising and filtering of the sequences were completed using the Divisive Amplicon Denoising Algorithm 2 (DADA2). QIIME2 was used to calculate diversity metrics and assign taxonomy using the classifier artefact “gg-13-8-99-515-806-nb-classifier.qza,” trained on Greengenes database, trimmed to the V4 hyper-variable region of the 16S rRNA gene using the primers f515 and r806, and clustered at 99% sequence identity. Core metrics for alpha and beta-diversity were generated using QIIME2. Kruskal-Wallis test was used to determine significant differences in alpha-diversity between groups while PERMANOVA was used to assess significant differences in beta-diversity.
Results
Environmental Conditions
Key environmental parameters were monitored and/or measured in the current study (Supplementary Figure 2) as they have a direct impact on decomposition rate, total necrobiome and microbial community functions/dynamics, in particular. Soil temperatures, which were recorded at each sampling time (Figure 1A) and expressed further in accumulated degree-days (Figure 1B), reflected the seasonal weather fluctuations. There was no statistically significant difference between the control and experimental treatments (p = 0.87) based on two-way ANOVA with replication. The average (n = 3) pH values showed that, unlike the soils associated with S. scrofa domesticus decomposition, control and leaf litter samples had very similar pH trends over the 24-month period (Figure 2), and two-way ANOVA showed significant differences between the treatments (p = 0.04). Cumulative precipitation was calculated at the different sampling intervals and recorded an average range of 0 to 1,400 mm over the 720 days of study (Supplementary Figure 2A). Overall, Principal component analysis (PCA) of the recorded weather conditions at the study site (Figure 3) showed that most of these parameters clustered to the right axis and correlated positively at the different sampling time points. The exceptions were snowfall and days of snow, which correlated negatively.
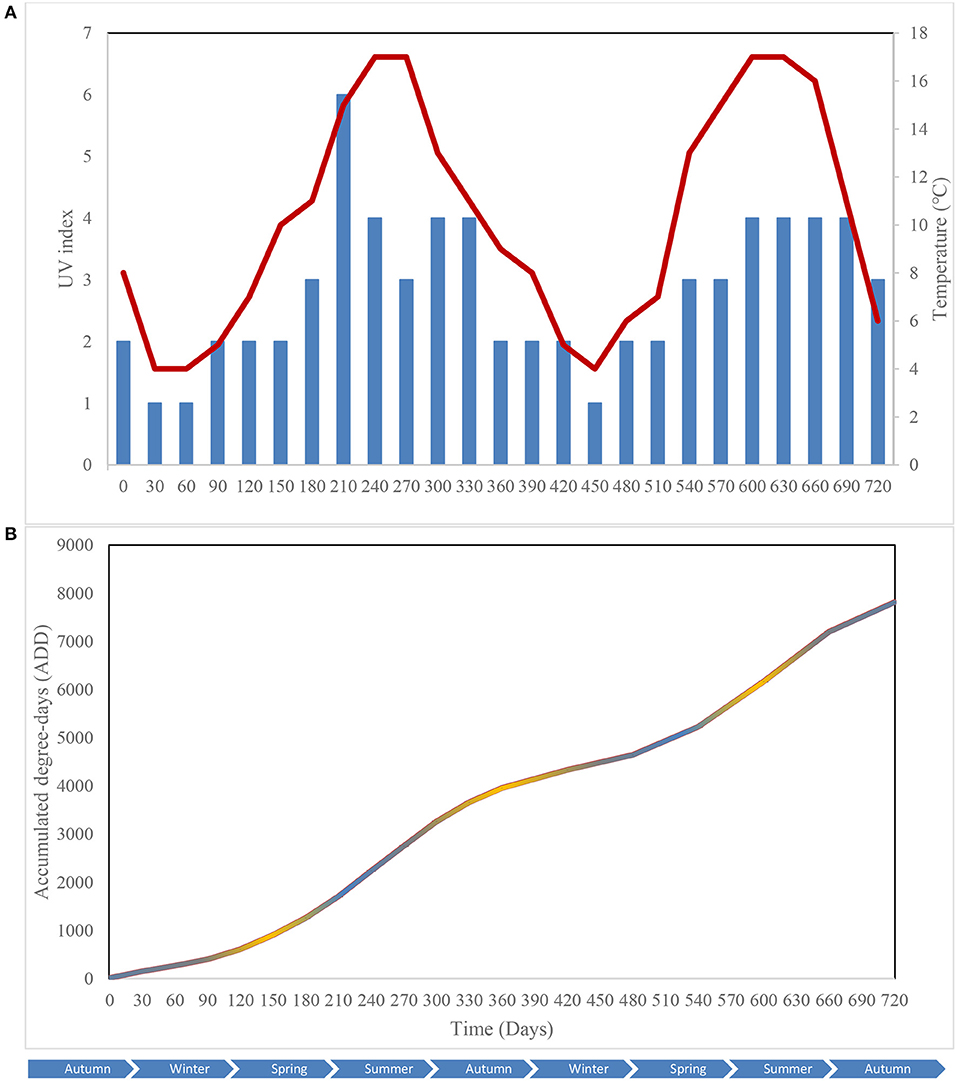
Figure 1. Atmospheric temperature () and UV index () at the study site (A); Accumulated degree-days (ADD) of the control (), leaf litter () and S. scrofa domesticus () treatments during a 720-day in situ study (B). Each data point is the mean of triplicate samples.
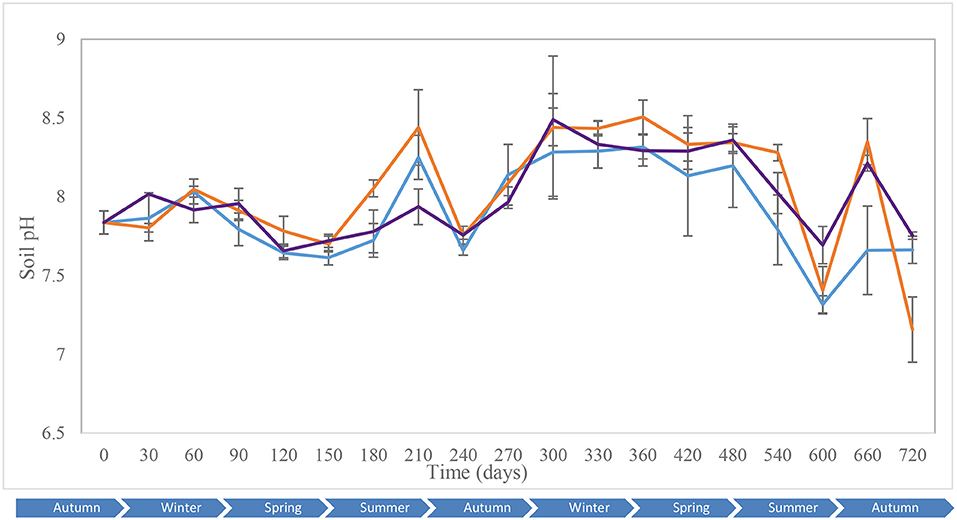
Figure 2. Average (n = 3) soil pH of the control (), leaf litter () and S. scrofa domesticus () treatments during a 720-day in situ study. Error bars are SEM.
DGGE-Based Profiling of Soil Necrobiome
To achieve a protracted 24-month study of microbial community shifts in the subsurface necrobiome, soil samples from the first year as published by Olakanye et al. (2017) were reprocessed with new DGGE of the fungal 18S and bacterial 16S rRNA gene profiles. Denaturing gradient gel electrophoresis was used as an economical, rapid, and reliable technology that can be accessible to laboratories globally for forensic profiling of soil microbial communities. To facilitate direct temporal comparisons between treatments and gels, the fungal 18S and bacterial 16S rRNA DGGE profiles were analysed to calculate and interpret the Hill ecological indices for taxa richness (0D), common taxa diversity (1D), dominant taxa diversity (2D) and evenness (1D/0D) (Supplementary Figures 3–6) with subsequent statistical analyses (Table 1).
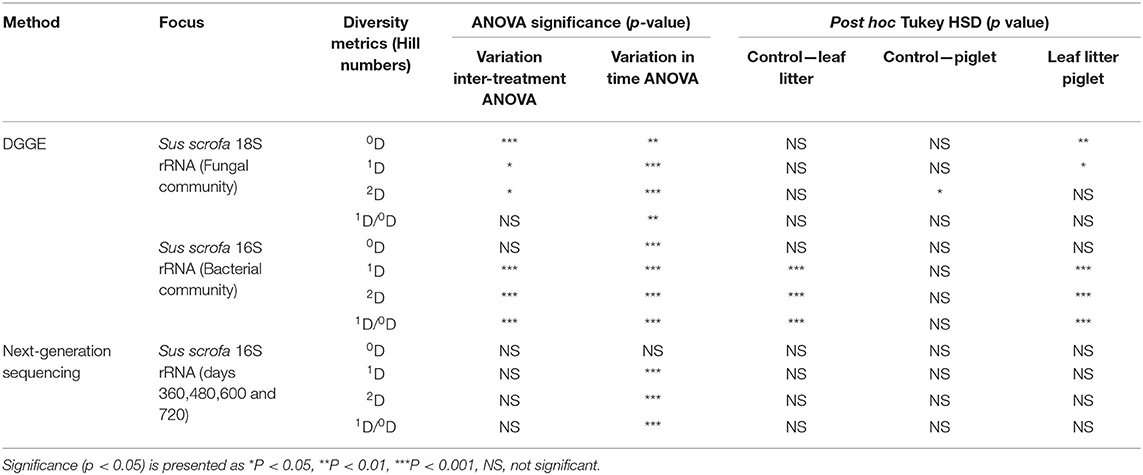
Table 1. Statistical significance for richness (0D), common taxa diversity (1D), dominant taxa diversity (2D), and evenness (1D/0D) during Sus scrofa domesticus and oak leaf litter decomposition.
Taxa Richness (0D) Diversity (1D, 2D) and Evenness (1D/0D)
Hill number 0D is the sum of the different taxa in the community and allows the assessment of community richness. The profiles of the fungal 18S rRNA gene richness (0D) showed temporal differences between the control, leaf litter and piglet soil samples (Supplementary Figure 3A). For example, Tukey HSD and Bonferroni post hoc tests revealed a significant decrease in fungal community richness between day 60/ADD 258 and day 90/ADD 396. Overall, the changes in fungal richness coincided with variations in seasonal weather with a considerable decrease starting in late autumn (day 330/ ADD 3631) and lasting until the end of winter 2015 (day 360/ADD 3921). Two-way ANOVA with replication showed a significant temporal difference in fungal community richness between the control, S. scrofa and oak leaf litter soils (p = 0.0002) and between the S. scrofa and oak leaf litter treatments (p = 0.01). There were no significant differences observed between the control and experimental samples (Table 1). In contrast to the fungal communities, there was no significant difference in bacterial richness between the three different soils (p = 0.179), although significant temporal differences were recorded within each treatment (p < 0.00).
Hill number 1D is the exponential of Shannon-Wienner index that facilitates the estimation of the community common taxa diversity, which decreased for the fungal communities in the piglet and control soils on day 30/ADD 144 and in the leaf litter treatments on day 60/ADD 258. Two-way ANOVA with replication highlighted a significant difference in fungal common taxa diversity (1D) between the treatments at different sampling times (-p < 0.000). Also, there was a significant difference in common taxa diversity between the experimental treatments (p = 0.014, Tukey HSD; p = 0.015, Bonferroni), however, no significant differences were observed between the control and experimental samples (Table 1). A significant difference in bacterial common taxa diversity was detected between the treatments at different time points during the second year of study (p < 0.000). Subsequently, post hoc analysis with Tukey (HSD) revealed statistical differences in the bacterial 1D on days 360, 480, 660, and 720 between leaf litter and control, and leaf litter and piglet soils, but no difference between control and piglet soils. In addition, there were season-based statistically significant differences in common taxa diversity between winter 2015/day 360 and end of winter 2016/day 420, and between summer/day 600 and winter/day 720.
Hill number 2D is the reciprocal of Simpson index that allows the evaluation of the dominant taxa diversity in a community. Similar to fungal 1D, 2D for this clade recorded a maximum increase in Sus scrofa soils on day 60. Two-way ANOVA with replication indicated a significant difference in fungal dominant taxa diversity between the treatments (p = 0.018) (Table 1). Unlike for 0D and 1D, there was a significant difference in 2D between the control and piglet soil samples (p = 0.024, Tukey HSD; p = 0.27, Bonferroni), however, no significant differences were observed between the control and leaf litter, or between piglet and leaf litter soils. Similar patterns in bacterial dominant taxa diversity resulted generally between control and piglet soils, which contrasted with the leaf litter treatments. Nevertheless, significant differences were detected between the treatments at the different time points (p = 0.0001). Specifically, post hoc analysis with Tukey HSD showed statistical differences in bacterial 2D on days 360, 480, 660, and 720 between leaf litter and control, and leaf litter and piglet soils, but no difference between control and piglet soils.
Community evenness (Hill ratio 1D/0D) gives an indication of how close in number each taxon is in the community. Two-way ANOVA with replication showed no significant difference in fungal community evenness between the treatments at the different time points during the second year of the study (p = 0.68) (Table 1). However, the difference was significant for bacterial community evenness (p < 0.00) as reflected by post hoc Tukey HSD and Bonferroni tests which showed differences in the 1D/0D index between days 360, 420, and 540, and then days 600 and 660. Furthermore, significant differences were revealed between leaf litter and control, and leaf litter and piglet soils, but not between control and piglet soils.
Generally, and in contrast to those for the fungal 18S clade, all the DGGE-derived Hill numbers for bacterial 16S communities showed highly similar trends between Sus scrofa domesticus and control soils with pronounced increases and decreases at some specific sampling points, particularly in Year 2 of the study.
Principal Component Analysis of Hill Ecological Indices
The principal component analysis (PCA) of the weather conditions at the site of study showed that principal component 1 (PC1) accounted for 42.98% of the variations in weather conditions while PC2 accounted for 34.14%. Temperature and UV index clustered strongly on PC1. Similarly, humidity, cloud cover and rainfall clustered and correlated strongly on PC2 at the different time points. Snowfall and days of snow correlated negatively on PC1. Principal component analysis (PCA) of the 18S rRNA fungal gene Hill ecological indices showed close clustering except for the Hill ratio 1D/0D (Figure 4A). Thus, 0D, 1D, and 2D for the control and experimental treatments clustered positively on PC1. Also, PC1 accounted for 42.61% of the variations in ecological indices while PC2 accounted for 14.21%. The different treatments' ecological indices correlated strongly and clustered together on PC1 except Hill ratio 1D/0D (Figure 4A). Cloud coverage, rainfall and humidity clustered positively on PC2.
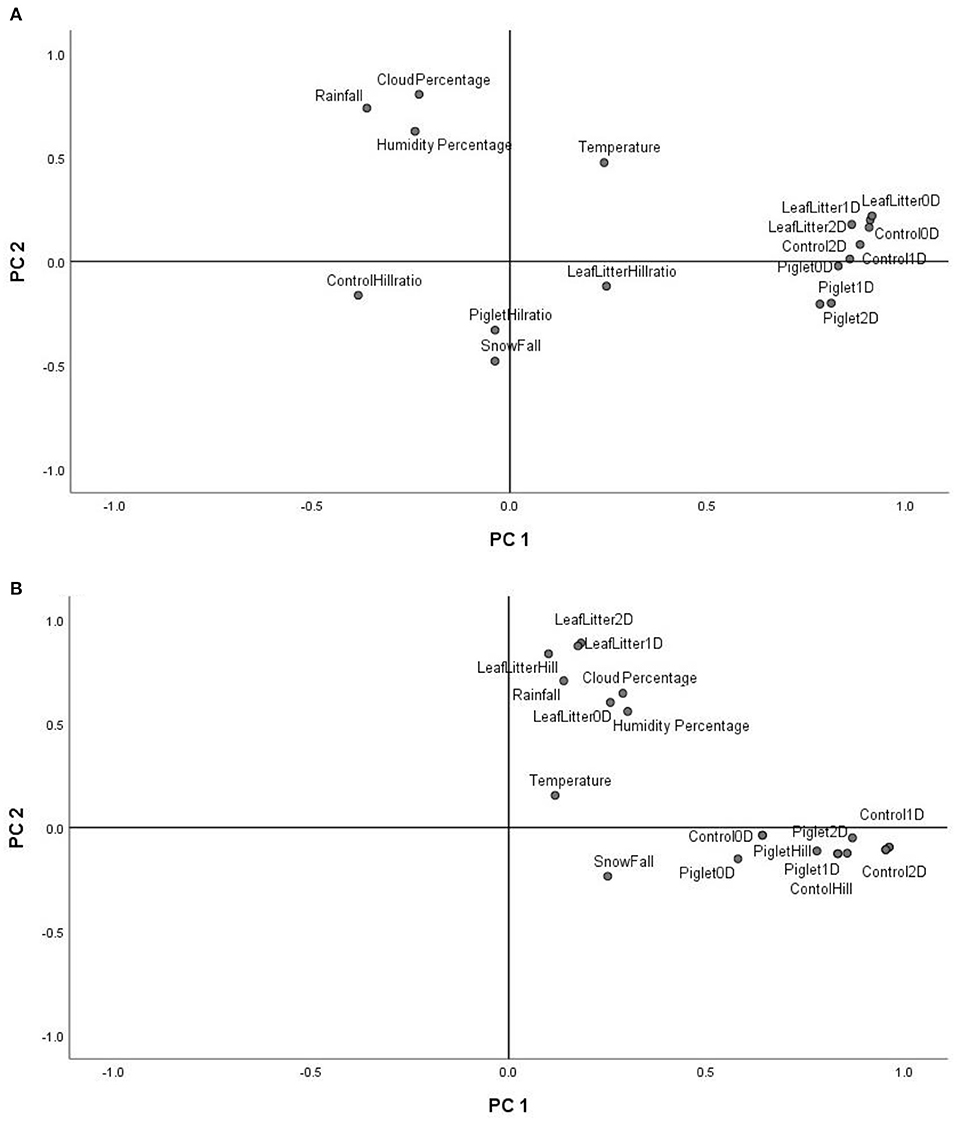
Figure 4. PCA biplot of18S fungal (A) and 16S bacterial community (B) ecological indices (Hill number 0D, 1D, 2D and Hill ratio 1D/0D) of the control, leaf litter and piglet soils.
The 16S rRNA bacterial gene PCA revealed a positive clustering of the environmental factors and all four ecological parameters for the different treatments along PC1 (Figure 4B). Also, control and piglet ecological indices clustered positively on PC1 and negatively on PC2, while leaf litter clustered positively on PC1 and PC2. Principal component 1 accounted for 41.56% of the variations while PC2 accounted for 19.21%. The diversity indices of piglet and control samples clustered strongly and positively on PC1, while leaf litter diversity indices clustered positively with rainfall and humidity on PC2.
Soil Bacterial Necrobiome Metabarcoding
High-throughput next generation sequencing, with the novel approach of targeting amplicon sequence variants (ASVs) instead of operational taxonomic units, has facilitated high-resolution metabarcoding of the soil necrobiome in forensic studies. The current report focuses on the second year (from day 360/month 12 to day 720/month 24) of our in situ piglet study, although reference is made to the results of the first year as published by Olakanye et al. (2017). Following sequencing of the bacterial 16S rRNA gene on an Illumina MiSeqTM platform, sequences were denoised, reads were rarified to 4 000 and amplicon sequence variants produced by DADA2, while taxonomy was assigned based on GreenGenes database. Only the most predominant taxa were plotted while unclassified ASVs were omitted.
Phylum level resolution revealed the most predominant phyla in descending order as: Acidobacteria (19.44–35.75%), Proteobacteria (13.16–27.59%), Actinobacteria (7.00–16.54%), Planctomycetes (7.32–12.08%), Verucomicrobia (4.49–11.47%), Chloroflexi (5.61–10.00%), Bacteroidetes (3.49–11.23%), Nitrospira (0.775–2.74%), WS3_phylum (0.53–2.76%), and Firmicutes (0.34–2.22%) (Figure 5A). Similar patterns in Acidobacteria, Proteobacteria, Firmicutes, and Chloroflexi abundance were observed in piglet and leaf litter soils as compared to controls. However, two-way ANOVA did not detect any significant temporal differences between piglet or leaf litter soils. At order level, two-way ANOVA with replication showed a significant difference in abundance with time for the control and leaf litter treatments while there was no significant difference in piglet soils.
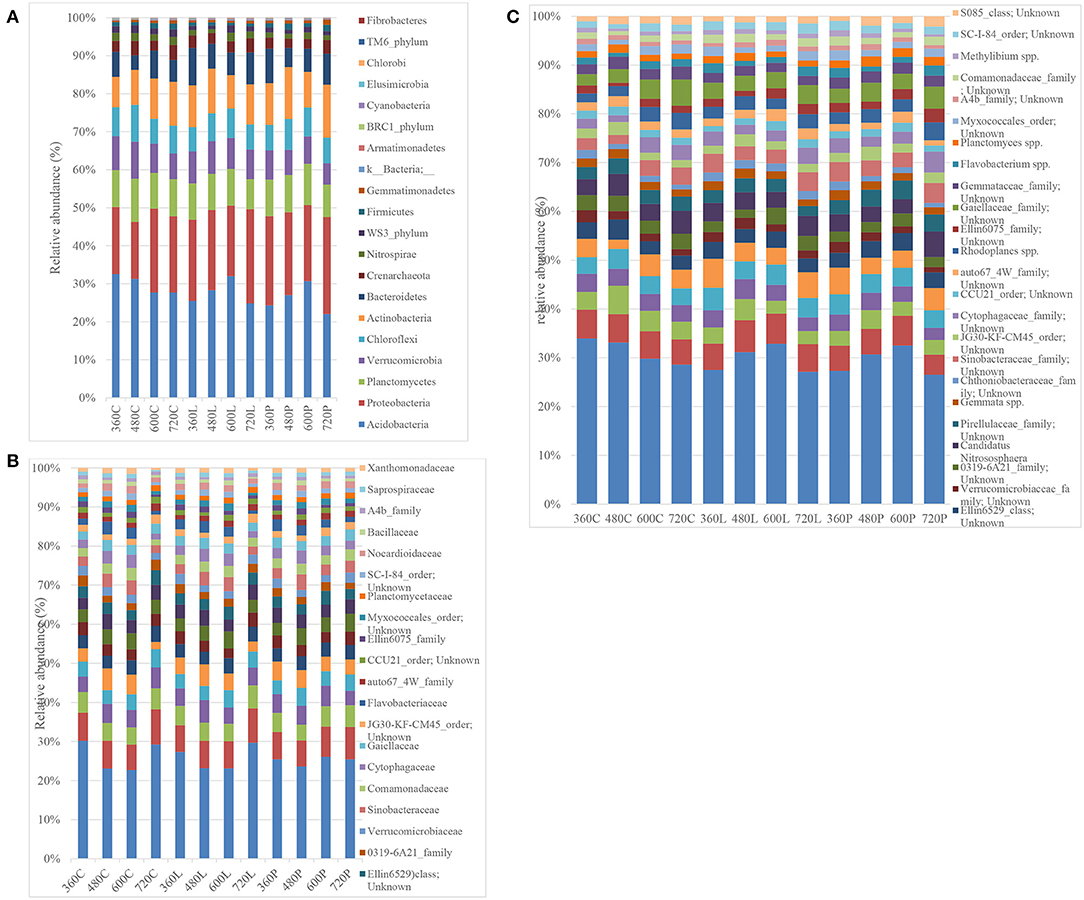
Figure 5. Bacterial phylum-level (A), family-level (B), and species-level (C) resolution bar-plots of the control (C), leaf litter (L), and piglet (P) associated soils on days 360, 480, 600, and 720.
At family level, different members from Acidobacteria (RB40_family and mn2424_family), Chloroflexi, Chtoniobacteraceae (2.43–7.44%), Hyphomacrobiaceae (1.73–4.14%), Pirellulaceae (1.79–4.11%), Chitinophagaceae (1.12–4.61%), Gemmataceae (1.38–3.23%), Sinobacteraceae (1.23–3.09%), Gaiellaceae (1.06–3.02%), and Cytophagaceae (0.99–2.78%) were the most abundant (Figure 5B). Two-way ANOVA did not show any significant differences between the different treatment groups while there were significant temporal differences within the respective treatment groups, which corresponded to seasonal variation. For example, some bacterial families showed seasonal shifts for the control and leaf litter soils, although their abundances correlated negatively with temperature for piglet soils. These include Pirellulaceae, Chitinophagaceae, Gemmataceae, Hyphomicrobiaceae, Verrumicrobiaceae, Sinobacteraceae, and Commamonadaceae. Other families such as [Chthoniobacteraceae], Nitrososphaeraceae, Chitinophagaceae, Hyphomicrobiaceae, Sinobacteraceae, Commamonadaceae, Cytophagaceae, Flavobacteriaceae, Ellin6075, Myxococcales, Bacillaceae, and Saprospiraceae showed a strong positive correlation between leaf litter and piglet soil samples. In contrast, Nitrososphaeraceae, Pirellulaceae, Chitinophagaceae, Gemmataceae, Hyphomicrobiaceae, Verrumicrobiaceae, Sinobacteraceae, Commamonadaceae, Cytophagaceae, Gaiellaceae, and Myxococcales correlated negatively between control and piglet soil samples.
Similar trends in genera abundance were observed between piglet and leaf litter soils from members in the iii1-15_family, mb2424_family, Chitinophagaceae_family, JG30-KF-CM45_order, CCU21_order, Candidatus, and Flavobacterium. There were significant temporal and seasonal differences in predominant genera abundances for control and oak leaf litter samples. However, no significant temporal differences were recorded in piglet soil using two-way ANOVA. As with genus level analysis, some species (Figure 5C) showed similar trends in abundance between piglet and leaf litter soils. These included species from iii1-15_order, RB40_family, mb2424_family, Chitinophagaceae_family, JG30-KF-CM45_order, CCU21_order, Candidatus, and Flavobacterium. Species from Ellin6529_class and Candidatus nitrosphaera had distinct trends in each of the piglet, leaf litter and control soils while species from Verrucomicrobiaceae and Chthoniobacteraceae family had distinct temporal trends in leaf litter soils as compared to control and piglet soils. Species from the Pirellulaceae, Gaiellaceae, Gemmataceae and Ellin6075 family reported distinct trends in piglet treatments when compared with control and leaf litter. Nonetheless, two-way ANOVA did not detect any significant temporal (seasonal) variation in the control and experimental treatments.
One-way ANOVA on ranks (Kruskal-Wallis H test) based on the alpha-diversity Faith phylogenetic distances (Supplementary Figure 7) indicated no significant differences between the control and experimental treatments during Year 2 of the study. However, principal co-ordinates analysis (PCoA) based on the beta-diversity Bray-Curtis distances (Figure 6A) revealed the clustering of most samples at the end of autumn (day 360; month 12) and during summer (day 600; month 20), which were more distanced by the end of winter (day 480; month 16). Also, PCoA based on weighted UNIFRAC distances (Figure 6B) indicated seasonal variation in the soils, with clustering toward the left for winter (day 360; month 12) and end of autumn (day 720; month 24) while most samples from spring (day 480; month 16) and summer (day 600; month 20) clustered in the centre.
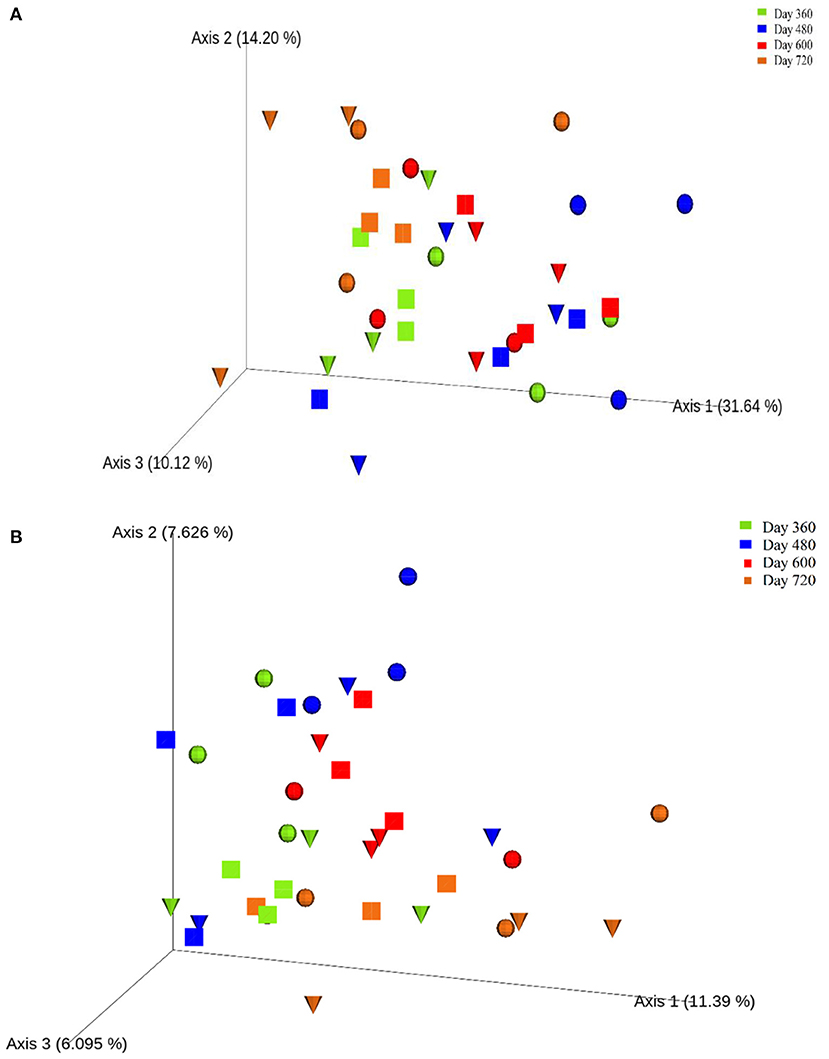
Figure 6. Principal co-ordinates analysis (PCoA) ordination of the control (), leaf litter () and piglet () soils samples based on Bray-Curtis (A) and weighted UNIFRAC (B) distances of the observed species ASVs.
Overall, dissimilarity test ANOSIM indicated significant temporal (seasonal) differences in the treatments. Likewise, PERMANOVA identified significant temporal differences while the test of homogeneity of dispersion PERMDISP did not detect any significant temporal differences in the treatments (Table 2), which suggests a location but no dispersion effect.

Table 2. Comparison of 16S rRNA community seasonal variation using three statistical methods (p-value).
NGS-Based Hill Ecological Indices
Hill numbers determined from the Year 2 NGS data were based on the numbers of species identified after assigning taxonomy. As recorded for DGGE-based bacterial profiles (Supplementary Figure 3B), species richness (Hill number 0D) showed similar patterns for the control and piglet soils, which contrasted the leaf litter soils (Figure 7A). Overall, two-way ANOVA with replication showed no significant difference in species richness between the control and experimental treatments during the second year of the study (p = 0.36) (Table 1). This statistical tool was applied also to the trends in Figure 7B and indicated a significant difference in common species diversity (1D) between the control and experimental treatments at different time points (p < 0.03), but no significant differences between the piglet and leaf litter treatments. Comparable patterns in the diversity of dominant species (2D) resulted for the S. scrofa domesticus and Quercus robur leaf litter treatments throughout the second year of the study (Figure 7C) and contrasted the soil-only control, but two-way ANOVA with replication indicated no significant differences between the control and experimental communities (p = 0.14).
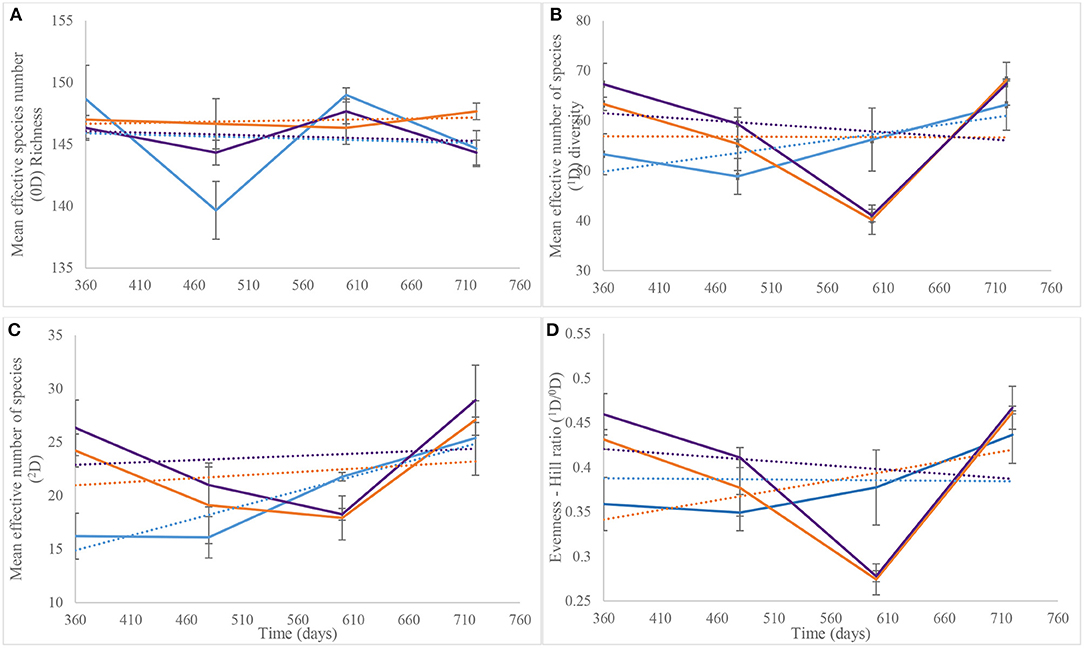
Figure 7. Average (n = 3) taxa richness (0D, A), common taxa diversity (1D, B), dominant taxa diversity (2D, C), and evenness (1D/0D, D) for bacterial communities of control (), leaf litter () and piglet () associated soils on days 360, 480, 600, and 720. The Hill numbers were calculated using species that were identified from next generation sequencing. Each datum point is the mean of triplicate samples. Error bars are SEM.
Similar to the 2D trends, two-way ANOVA with replication revealed a significant difference in bacterial species evenness (1D/0D) between the control and experimental treatments throughout Year 2 of study (p = 0.006) but no significant differentiation of the piglet from leaf litter soils (p = 0.78) (Table 1). Overall, the trendlines for the Hill ecological indices show: (i) increasing 0D divergence from day 360 to day 720 for the control and piglet vs. the leaf litter soils; (ii) convergence in 1D on day 600 with some divergence between control vs. both leaf litter and piglet treatments on day 720; (iii) divergence in 2D for experimental treatments vs. control on day 360 but convergence for all three around day 700; and (iv) similar trends for 1D/0D (Figure 7D).
Soil Mineral Composition
Calcium (Ca), iron (Fe), potassium (K), phosphorus (P), sulphur (S), and magnesium (Mg) were quantified in the different soil samples collected throughout the 2-year study to assess shifts in soil mineral composition that could be exploited for forensic applications (Melis et al., 2007; Aitkenhead-Peterson et al., 2012; Stokes et al., 2013; Macdonald et al., 2014; Szelecz et al., 2018). These were used subsequently to complement subsurface microbial community profiling for a more holistic toolkit. All the blank samples tested had elemental concentrations that were below the detection limit of the equipment. Piglet soil calcium content was marked by an overall increase during the first year of the study from 2.4 5 ± 0.26% on day 0 to 7.4 2 ± 0.35% on day 360, followed by a decrease until day 720 (5.02 ± 1.75%) (Figure 8). Leaf litter soil calcium content increased to its highest on day 180 (7.78 ± 0.66%) and then decreased until the end of the study, while control treatments had overall minor increases in calcium with a peak on day 300 (6.04 ± 1.56%). The Fe, P and K contents for the different treatments had comparable patterns throughout the 2-year study with constant concentrations overall, except on day 240 at which Fe and P decreased for the three treatments while K increased for the control and S. scrofa soils. Two-way ANOVA indicated a significant difference in calcium (Ca) composition between the control, piglet and leaf litter soils throughout the study (p = 0.00) while no significant differences were observed between the treatments for soil Fe, P, K, or S composition (p > 0.05). With the exception of magnesium (p > 0.05), significant differences were recorded for the different soil elements and between the various sampling intervals (p < 0.00).
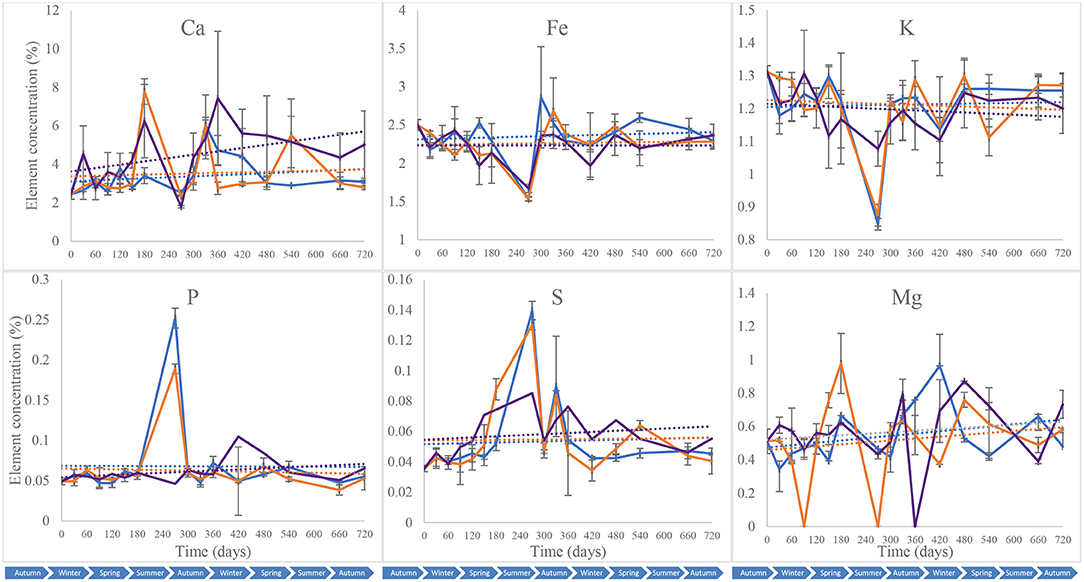
Figure 8. Average (n = 3) soil composition in calcium (Ca), iron (Fe), potassium (K), phosphorus (P), sulphur (S), and magnesium (Mg) for control (), leaf litter (), and S. Scrofa domesticus () treatments. Each data point is the mean of triplicate samples. Error bars are SEM.
Discussion
Previous studies have investigated decomposition in controlled environments (Metcalf et al., 2013) and often for relatively short periods of time. Although the approach is very useful to explore the different parameters that influence decomposition separately, it does not replicate or reflect the natural conditions that would be found in forensic investigations. In contrast, the current outdoor Sus scrofa-based decomposition study is, to our knowledge, the longest within the forensic ecology literature. It was set outdoor in a natural, non-controlled environment, taking into consideration the inherently different atmospheric, edaphic and biotic factors at the study site in order to mimic cadaver interment in case of homicide (clandestine grave).
Atmospheric and Edaphic Conditions
Weather conditions have a significant impact on carrion decomposition and the associated soil microbial communities. For example, Carter et al. (2008) stated that temperature is one of the most important factors that affect decomposition rate as it governs biological processes. Various authors (Vass et al., 1992; Shean et al., 1993; Megyesi et al., 2005; Stokes et al., 2009) reported an inhibition of biochemical processes associated with biological activity, in general, and microbial activity, specifically, at temperatures lower than 4°C hence delayed putrefaction rates in cold and/or snowy weather. Also, UV radiation was reported to reduce litter biomass considerably (Gaxiola and Armesto, 2015) by increasing decomposition rate via photodegradation (Brandt et al., 2007). Wang et al. (2015) suggested that UV exposure time might increase litter biodegradability which would, consequently, enhance carbon supply to microorganisms and thus accelerate decomposition. The changes in fungal and bacterial richness, diversity and evenness observed during our study reflected seasonal temperature fluctuations with increases in the different ecological metrics at high temperatures, which decreased when temperatures dropped. This was reflected further by changes in the dominances of some taxa at specific intervals of the 2-year period, and highlighted the effect of temperature on microbial metabolism and biological reactions during subsurface decomposition at the current study site.
Multivariate analysis (PCA) of weather conditions revealed a positive correlation between temperature and UV index which clustered along component 1 (Figure 3). Similarly, rainfall, humidity and cloud coverage clustered together and correlated positively. Overall, the recorded trends aligned with the known and recognised relationships between parameters that clustered together and their related roles in decomposition. For example, rainfall which accounts for soil moisture content and partly impacts soil temperature, affects the structure and activity of microbial communities and, hence, influences decomposition (Hyde et al., 2017). Thus, seasonal changes in rainfall and temperature coincided with the successional shifts in soil fungal and bacterial communities. PCA of the 18S fungal ecological indices (Figure 4A) showed high similarities in trends for richness (0D) and, common (1D) and dominant (2D) taxa diversity of the control and experimental treatments while still permitting the distinction of leaf litter 0D, 1D, and 2D, and piglet 1D and 2D from control soils. Also, the positive clustering of temperature with the ecological indices for richness and diversity highlighted the impact of this key environmental parameter on soil fungal community composition and structure, while rainfall and cloud coverage had a lesser influence on this clade. In contrast to the fungal profiles, PCA of the 16S bacterial community diversity indices (Figure 4B) was marked by clustering of the control and piglet soils together, but separately from leaf litter treatments, and was characterised by a distinct bacterial community. In addition, the clustering and positive correlation of the environmental factors—temperature, rainfall, cloud cover, humidity—on component 1 reflected their impact on bacterial diversity throughout the study.
The control and experimental treatments had similar pH patterns throughout the 2-year period of study. Notwithstanding this, pH did not correlate with temperature, the other abiotic factors or diversity indices, except for the fungal and bacterial Hill ratio (1D/0D) with which it had moderate-negative and weak correlations, respectively. Analysis of clustering amongst the ecological indices showed a strong correlation in fungal community dynamics for the control and experimental soils for the study duration. Bacterial Hill indices allowed the distinction of leaf litter soils from control and piglet soils. The similarity between controls and piglet soils was potenitally due to the small-sized piglets (1.5 kg) which created confined cadaver decomposition islands (CDIs). According to Carter et al. (2007), nutrient leaching is limited both vertically and horizontally by the cadaver size and rate of decomposition and, hence, the soil samples collected outside the cage may not have been impacted sufficiently by decomposition to then register temporal differences in the fungal and bacterial communities. In addition, the land used for the experiment was located in a woodland ecosystem with clusters of oak trees and continuous input of organic material to the surrounding soil which potentially led to the recorded microbial community similarities (Olakanye et al., 2017). Snowfall and days of snow clustered negatively on component 1 and had a moderate negative correlation with the different bacterial ecological indices which was then reflected by the decrease in bacterial diversity as exemplified during spring 2015 (day 540).
Increases in bacterial diversity for the control and experimental soils recorded during the first month of the study were probably due to the disturbance of soil during interment (Kim et al., 2013). This increase coincided with an increase in piglet soil pH which remained stable until spring (day 120). Control and leaf litter soil pH increased on day 60 (winter) and coincided with increases in bacterial richness and common taxa diversity (1D) for the control, but decreases in richness, common taxa and dominant taxa diversity for the leaf litter. On day 240 (summer), bacterial community richness, diversity and evenness dropped minimally for control soils and considerably for piglet soils with similar decreases in community richness and diversity also recorded on days 360 (winter) and 660 (autumn). As also reported by Finley et al. (2015b), pH changes during decomposition lead, subsequently, to a change in the soil microbial community composition and structure. Therefore, the increase in Sus scrofa domesticus soil pH during the first months is attributable to the release and accumulation of ammonia-rich fluids in soil (Hopkins et al., 2000; Metcalf et al., 2016), while the subsequent decrease resulted from soil microbial communities utilisation of base cations such as K+, Na2+, or Mg2+(Carter et al., 2008).
Overall, these findings highlight the importance of profiling fungal and bacterial communities in conjunction with environmental factors, and applying multivariate analyses to understand fully their interconnectedness within decomposition. The correlation of temperature with soil fungal community ecological indices, and correlation of temperature and rainfall with the bacterial community indices, demonstrate the importance of understanding the effect of the environmental factors on both universal microbial diversity and specific taxonomic glades. Hence, combining the patterns of bacterial and fungal ecological indices to climatic records could indicate the presence of a carbon source. For example, low microbial community diversity during warm seasons, or increased diversity during winter, could be indicative of a recent disturbance to soil which might be due to carrion decomposition, especially decomposition processes that usually happen during the first few months of interment.
Community Diversity Analysis Based on DGGE and Hill Numbers
To date, most forensic microbiology studies have used the Shannon-Wiener and Simpson indices to establish soil microbial community diversity during decomposition. The current study is the first to use Hill numbers as ecological indices in a forensic context for further interpretation of DGGE and NGS data to measure temporal and spatial microbial community shifts during in situ sub-surface decomposition.
Fungal 18S rRNA Soil Community
The overall increases in fungal taxa richness recorded for all burials on days 60/ADD 258 and 90/ADD 396 were probably due to the introduced carbon sources, which constitutes a physical disturbance to the indigenous soil microbial communities (Kim et al., 2013). Also, Tukey HSD and Bonferroni post hoc tests revealed a significant change during this period. This trend suggests that although it was the middle to end of winter 2015, skin rupture and nutrient release happened before day 60/ADD258 and that the piglets were potentially at the decay/active decay stage. The overall increases in fungal 0D were largely sustained during the first year of the experiment and, therefore, reflected a protracted response of this clade to the presence of animal-based and plant-based sources of carbon and nitrogen in the experimental soils. Generally, a significant difference in fungal community richness that reflected seasonal variations resulted between the two experimental treatments of piglets and oak leaf litter, and for the experimental and control soils but only at specific time points during the study.
Decreases in piglet soil fungal diversity for common (1D) and dominant (2D) taxa on day 30/ADD 144 were possibly caused by the introduction of the mammalian surrogates which represent a high quality nutrient resource with high water content and a narrow C:N ratio (Carter et al., 2007), selecting for specialist degraders. This contrasted the control and leaf litter soils where the pronounced decrease in 1D and 2D on day 60/ADD 258 (4°C) were most likely because of the lower quality carbon source (plant litter), or absence thereof (control). Generally, two-way ANOVA showed a significant difference in fungal community 1D and 2D between samples from different times corresponding to seasonal variations. Hence decreases in the diversity metrics from end of autumn (2014) to end of winter (2015) were followed, for example, by increases from spring until autumn particularly in the presence of leaf litter. Next generation sequencing of the 18S rRNA gene would have identified the specific indicator fungal taxa and is therefore recommended for future studies.
Although total body scores were unachievable for the subsurface burials, the generally pronounced decreases in fungal community 1D and 2D during the second year of study were attributed to the reduction of soil nutrients where the added substrates were largely or completely decomposed and mineralized. This highlights the need for further studies into how long the microbial community signals remain traceable within decomposition-impacted soils to still be useful for location/identification of clandestine burials, including for cases where remains have been moved. Post hoc Tukey HSD and Bonferroni tests facilitated the differentiation between soils with animal carbon source (piglet) and those without (control and leaf litter) but not between control and leaf litter soils based on fungal community 1D and 2D. Also, analysis of fungal community Hill ratio (1D/0D) showed temporal changes in evenness for this microbial clade. This was potentially a consequence of seasonal weather variation despite there being no significant difference between control and experimental treatments.
In summary, the 18S rRNA gene DGGE-derived richness (0D) and common taxa diversity (1D) allowed clear differentiation between the mammalian decomposition and that of oak leaf litter while dominant taxa diversity (2D) permitted the distinction between piglet and control soils, with the resolutions more pronounced at specific intervals. Therefore, the different Hill numbers derived from fungal community profiles can be analysed for soils and conditions similar to our study specifically to establish temporal subsurface decay of mammalian or plant matter (0D, 1D) or no mammalian decomposition (2D).
Bacterial 16S rRNA Soil Community
The initial increase in 16S rRNA bacterial community richness (0D), common taxa diversity (1D) and dominant taxa diversity (2D) observed on day 30, concomitant with ADD 144, was due to the physical disturbance caused by digging, carbon source interment and high soil temperature (12°C) at the beginning of the study. On the other hand, the subsequent decrease in piglet soil bacterial community 0D, 1D, and 2D at day 60/ADD 258 might be due to the influx of nutrients after the introduction of a high quality protein resource (Carter et al., 2007), which would decompose at a faster rate compared to leaf litter selecting, momentarily, for fast-growing members (r-strategists) of this clade. Lower temperatures during winter, without added nutrients, led to the decline in taxa richness and diversity for the control soils. Two-way ANOVA with replication highlighted seasonal variations in the different treatments. Furthermore, Tukey HSD and Bonferonni post hoc tests detected statistically significant differences between the leaf litter soils when compared to the control and S. scrofa based on 1D, 2D, and 1D/0D but no significant differences between control and piglet treatments. The trends were highlighted further by principal component analysis where the piglet and control ecological metrics (0D, 1D, 2D, and 1D/0D) clustered along component 1, separately from the leaf litter indices. As a result, bacterial ecological analysis using Hill metrics would not afford a distinction between mammalian gravesoils from their non-burial equivalents for this and similar soils/sites.
Bacterial Community Profiling
The aim of this study was to compare soil microbial communities associated with the decomposition of stillborn piglets as human proxies and oak leaf litter in a natural setting, analyze their successional shifts in composition and structure, and determine their potential as tools for forensic investigation.
Soil Metabarcoding
Soil necrobiome metabarcoding that focused on the second year of our study identified the most predominant bacterial phyla as Acidobateria, Proteobacteria, Actinobacteria, Plancomycetes, Verrumicrobiota, Chloroflexi, Bacteroidetes, Nitrospira, and Firmicutes. This is partly in accordance with Finley et al. (2016) who reported Proteobacteria, Actinobacteria and Acidobacteria as the most abundant phyla during terrestrial decomposition and Damann et al. (2015) who reported predominances of Firmicutes, Proteobacteria, Acidobacteria, Actinobacteria, Bacteroidetes, and Chloroflexi in decomposing human bone. Also, Acidobacteria, Proteobacteria, and Bacteroidetes correlated positively with temperature. Increased abundance of Acidobacteria during the second year of study was in contrast to the findings for the first 9 months after interment where Olakanye et al. (2017) reported Proteobacteria as the most abundant phylum for these burials. Therefore, phylum-level soil metabarcoding may afford preliminary temporal resolution of a 2-year subsurface post-burial interval in sites with similar soil, environmental and climatic conditions as our study area.
Similar patterns in Acidobacteria, Proteobacteria, Firmicutes and Chloroflexi abundances were observed in piglet and leaf litter soils as compared to controls (Figure 5A). Thus, two-way ANOVA detected significant differences in phyla abundances between control and experimental treatments throughout the second year of study, with no significant difference between piglet and leaf litter soils. Similarly, no significant temporal changes in piglet or leaf litter soils were detected while there were significant seasonal phylum-based shifts in control soils. Singh et al. (2018) reported a lower abundance of Chloroflexi in soil in the presence of human cadaver. This was not apparent in the current study, both in Year 1 (Olakanye et al., 2017) and Year 2, as there were no significant differences in Chloroflexi abundance between the different treatments (p = 0.69). In particular, two-way ANOVA with replication on the different taxa belonging to Proteobacteria and Chloroflexi showed no significant seasonal or treatment variations during the second year of study for the experimental treatments (p > 0.05), although control soils showed a seasonal variation in phyla abundance (p = 0.0001). Overall, the four phyla that presented the same patterns in piglet and leaf litter soils could help distinguish decomposition soils from soil-only controls, however, they would not detect or separate animal from plant gravesoils. Notably, the difference between controls and experimental soils during the second year of the study suggested some persistence of a microbial decomposition signature for a post-burial interval of up to 24 months. Although similarities in phylum-based succession and clock indicators between the current Sus scrofa domesticus-based study and those using human cadavers justify further the utility of pigs, research initiatives that directly compare these surrogates with human cadavers are essential. Only then can knowledge developed from swine decomposition studies be considered for transfer and application in criminal cases involving human remains.
Orders such as Acidobacteria iii1-15, Rhizobiales, Actinomycetales, and Saprospirales correlated positively with temperature and became more abundant in summer (day 600/ADD 6136). These were likely contributors to the recorded increases in richness as reflected by both DGGE and NGS Hill 0D results at the same sampling sampling interval. In contrast, CCU21, JG30-KF-CM45, and Ellin 6529 did not correlate with temperature. Two-way ANOVA with replication showed a significant difference in taxa abundance for the control and leaf litter treatments at the different sampling times while piglet soils recorded no significant variation with time. Methylococcales, which were reported during months 0–9, were associated with piglet treatments during the second year of the experiment and had distinct successional trends from those associated with control and leaf litter soils. This order could, therefore, be used as an indicator of gravesoil in this experimental setting and conditions. In a 10-month study, Finley et al. (2016) reported a consistent abundance of Rhizobiales and Chtoniobacterales during subsurface decomposition. This was reflected in our study as these taxonomic orders correlated positively with temperature and showed seasonal variation. However, they were detected in the different treatments and, therefore, non-specific to piglet decomposition as a human analogue.
When developing their prediction model for PMI, Belk et al. (2018) found that phylum-level analysis permitted the most accurate prediction than other taxonomic levels. This contrasted the current study where phylum-level resolution, except for dominances for Proteobacteria (Year 1) and Acidobacteria (Year 2), provided no consistent differentiation between the experimental treatments. Nevertheless, most studies have focused on the use of phylum or family-level resolution (Metcalf et al., 2013; Pechal et al., 2013, 2014), which makes their findings potentially scale specific. According to Zhou and Bian (2018), microbial communities profiling at higher taxonomic resolution, of genus- or species-level, would ensure more comprehensive data analysis and probably facilitate the discovery of exploitable trends. Therefore, this approach was adopted in the current study.
At family level, the most abundant taxa recorded during the second year of the study, and hence the most likely microbial clock indicators for long PBI, were: RB40_family, mn2424_family, Chloroflexi, Chtoniobacteraceae, Hyphomacrobiaceae, Pirellulaceae, Chitinophagaceae, Gemmataceae, Sinobacteraceae, Gaiellaceae, and Cytophagaceae. The structure and dynamics of the community at family level were similar to ordinal and phyletic levels, with seasonal variations throughout the second year of the study. Pirellulaceae, Chitinophagaceae, Gemmataceae, Hyphomicrobiaceae, Sinobacteraceae, Cytophagaceae, and Gaiellaceae correlated differently according to season and/or type of treatment (Figure 5B). Furthermore, Sphingomonadaceae correlated negatively with temperature in the piglet and leaf litter treatments and positively in control samples. Methylophilaceae and Anaerolineaceae were reported by Olakanye et al. (2017) as potential microbial clocks for piglet and leaf litter, respectively, during the first year of the study. The results of the second year showed that Methylophilaceae correlated positively with temperature in piglet soils, however, it also correlated positively for control, which makes it non-specific to piglet soils for the late stages of temperature-related decomposition for the current scenario. On the other hand, Anaerolineaceae was not detected in any of the control or experimental treatments. Based on two-way ANOVA, there were no significant differences between the different treatment groups, however, there was a significant difference in samples collected at different times, which corresponded to seasonal variation.
Cobaugh et al. (2015) reported the persistence of microbial signatures of decomposition for more than a year after interment of a human cadaver, which would help detect clandestine graves. Similarly, Olakanye et al. (2017) used piglets instead of human cadaver and reported distinct signatures at order and family-levels during Year 1 of the current study. In contrast, the findings from Year 2 showed no detectable signatures of bacterial orders or families that could be used to establish the extent of animal decomposition in soil. This might be attributable to differences in the mass of the decomposing materials where an adult human cadaver would have a bigger and more protracted impact on the gravesoil microbial community structure and composition compared to piglets. Therefore, future experiments should compare the soil necrobiome during simultaneous subsurface decompositions of human cadavers and adult pigs where the size/weight of the interred materials are as similar as possible to establish comparison baselines and/or proof of concept.
At genus level, similar trends in abundance between piglet and leaf litter soils were observed in genera from iii1-15_family, mb2424_family, Chitinophagaceae_family, JG30-KF-CM45_order, CCU21_order, Candidatus, and Flavobacterium, which may help discriminate decomposition soils from soil-only controls. However, they did not permit the distinction between animal-based and plant-based decomposition. Notwithstanding this, the significant temporal shifts in predominant genera abundance of control and leaf litter showed the seasonality in soil bacterial composition. No species-level markers were detected specifically for piglet decompositions during the second year of study. However, species from Sinobacteraceae and Cytophagaceae family, JG30-KF-CM45 and CCU21 order, Rhodoplanes spp, and Flavobacterium spp were present in experimental soils only, while species from Pirellulaceae, Gaiellaceae, Gemmataceae, and Ellin6075 families showed distinct shifting patterns when comparing control and leaf litter treatments. These species might, therefore, be targeted as potential markers to detect gravesoil in an outdoors crime scene with conditions similar to those of the current research setting. For method validation, the findings need to be confirmed in a controlled experimental setting to rule out the effects of the variables associated with outdoor studies where several environmental parameters are uncontrolled.
The study of alpha diversity in the different treatments was performed using Faith phylogenetic metric and complemented subsequently by Hill ecological indices analysis for a comprehensive comparison with the DGGE-based results. Supplementary Figure 7 shows the similarity in alpha-diversity between the different treatments, especially piglet and leaf litter soils during the second year of decomposition. Also, one-way ANOVA on ranks (Kruskal-Wallis) did not show any significant differences between the control and experimental treatments for this period. This similarity might be indicative of a convergence in soil bacterial community composition due to decreased decomposition and/or amounts of mineralizable carbon sources more than a year after interment, the effect of weather conditions on soil bacterial community, and continuous input of new carbon sources in the open-air study site.
During the second year of the experiment, the Beta-diversity metric of Bray-Curtis distance showed the clustering of most of the samples at the end of autumn (day 360) and during summer (day 600), which were more distanced by the end of winter (days 480). Similarly, weighted UNIFRAC distance reflected the clustering of samples from spring (day 480) and summer (day 600) together, and separately from those of winter (day 360). These findings suggest a seasonal variation in soil bacterial community based on beta-diversity. However, there were no significant differences in beta-diversity between the control and experimental treatments.
In a study on human decomposition, Cobaugh et al. (2015) reported that cadaver-related microorganisms, that are very distinct and scarce in nature, could persist in soil and still be detected more than a year after placement, and could be used as biomarkers for human decomposition. Although the results of the current in situ study did not track the piglet-derived necrobiome or identify soil bacterial species associated solely with the piglet treatments, some taxa reported distinct shifts that were specific to piglet treatments and not leaf litter or control soils. Therefore, their successional shifts, and not their presence or absence, can be used as temporal indicators for post-burial interval determinations for similar study sites.
Ecological Indices of the Bacterial Community Metabarcode
Ecological indices are used typically to facilitate spatial and temporal comparisons between treatments, and across different studies. These data are often analysed parallel to those derived from next generation sequencing. In this study, we report the first time use of Hill ecological indices analysis of NGS-derived data specifically to complement, and directly compare with, the metrics calculated from DGGE community profiling. The aim was to address the limitations of each approach and test the reliability for data extrapolation between the techniques, to then inform a proposal that DGGE can be used instead of NGS by forensic microbiology laboratories with limited (financial) resources. In a similar way to Hill numbers calculated from DGGE results, Hill numbers calculated using NGS data showed seasonal shifts in diversity (1D and 2D) and evenness (1D/0D) for the different treatments. Although there was no significant difference in species richness (0D) between samples collected at different seasons for Year 2 of the study, increases in the abundance of some orders on day 600 suggested an alignment between DGGE and NGS-based Hill analysis.
The current findings suggest that each taxonomic level can give additional information such as whether it is related to discriminating soil, finding decomposition markers, confirming seasonality, or checking the extent/longevity of the bacterial signature in time. NGS-based analysis of the different taxonomic levels afforded a better insight into the dynamics of the bacterial community, implying that NGS should not necessarily be replaced with DGGE. Also, analysis of bacterial community structure using the different DGGE-based Hill ecological indices calculated for all 24 months of the study did not permit a consistent distinction between control and experimental treatments. Nevertheless, the similarity in bacterial trends between piglet and leaf litter soils, and marked difference with the control community at different taxonomic levels, suggested some persistence of decomposition signature of both animal and plant-based carbon sources up to 24 months. The taxonomic resolutions from an in situ study, and their interpretations via Hill numbers, need to be investigated under controlled experimental settings for proof-of-concept. Furthermore, future studies should track the necrobiome of the interred mammalian surrogates parallel to that of the gravesoil to identify key markers and then determine the persistence of signature microorganisms/community after carrion decomposition.
Soil Mineral Composition
Calcium, phosphorous, potassium and magnesium are major minerals in the human body with blood concentrations dropping from 8.45 to 3.70 mg dL−1 for calcium, and increasing from 100.72 to 188.49 μg dL−1 for iron after 264 h of putrefaction (Costa et al., 2015), while sulphur and iron are trace elements necessary for biochemical functions. As a result, soil mineral composition is often included in the suite of physicochemical analyses that are used in criminal investigations, with considerable potential for preliminary location of clandestine graves. In contrast to separate analyses, this study aimed to complement soil necrobiome metabarcoding with analysis of key elements for an enhanced toolkit for decomposition studies. Phosphorus, magnesium, calcium, potassium, iron and sulphur were selected for their importance in biological functions, nutrient recycling and microbial activity. Sulphur represents about 0.15% of pig tissue mass. It is found in protein-forming amino acids such as methionine and cysteine, which are degraded by microbial-driven decomposition to form volatile sulphur compounds that attract necrofauna insects (Statheropoulos et al., 2011; Kasper et al., 2012; Agapiou et al., 2015). Potassium is important for muscles and the nervous system functioning and has been used for the estimation of time since death based on its concentration in the vitreous humour (Ortmann et al., 2016; Cordeiro et al., 2019) and synovial fluid (Siddhamsetty et al., 2014). In addition, it is present in soil and plays an important role in plant growth and metabolism (Wang et al., 2013). Likewise, phosphorous, magnesium and calcium are important elements for biological functions and soil nutrients. Phosphorous concentration was reported to change during decomposition (Benninger et al., 2008; Stokes et al., 2009, 2013; Szelecz et al., 2018) while calcium changes underneath bison carcasses were detectable up to 7 years after interment (Melis et al., 2007).
In this study, the patterns for calcium content between piglet soils, and the control and leaf litter soils, were distinct as indicated by the trendlines (Figure 8). In addition, two-way ANOVA identified significant differences between the different treatments throughout the study. Calcium content increased markedly in the piglet treatments compared with control and leaf litter. This might have been due to the release of calcium from the piglets as they decomposed while the minor increase in control and leaf litter treatments calcium content was potentially due to the continuous input of oak leaves in the woodland-classified study site. Although still higher than at the beginning of the study, the decrease in calcium content during the second year might have been a result of the slower release of calcium from the piglet remains that were at the late stages of decomposition, and utilisation of the calcium already available in soil. Also, unlike potassium and phosphorous, which are mainly available in labile tissues and released during the early stages of decomposition (Szelecz et al., 2018), calcium is released continuously during the different stages of decomposition until total degradation of bones (Melis et al., 2007), probably leading to the observed statistically significant differences between the treatments throughout the study. Piglet soil calcium content had a moderate positive correlation with rainfall but did not correlate with temperature.
Further correlation analysis indicated a moderate-positive correlation in iron and potassium and a strong correlation in sulphur trends between the control and experimental treatments, which had overall similar trends throughout the study. In addition, there were significant temporal changes in soil iron content, however, there were no significant differences between the different treatments. Phosphorus content correlated very strongly between control and leaf litter soils while none of these treatments correlated with piglet soil samples. Overall, no statistically significant differences were observed when performing two-way ANOVA, which, therefore, did not allow the discernment of gravesoil from control and leaf litter soils.
The correlation of calcium and iron with decomposition observed during the study is in accordance with Costa et al. (2015) who reported a significant linear correlation of these elements with the time of blood putrefaction. Also, Marín-Roldan et al. (2013) reported a positive correlation of elements such as Mg, Na and K in swine skeletal muscles with PMI due to a variation in their concentration caused by chemical reactions in tissues. While it was not possible to determine the decomposition stages during the subsurface S. scrofa domesticus (piglet) study, increases in calcium content might have coincided with early and mid-stages of decomposition as these are expected to happen during the first few months of interment in the north of England (Irish et al., 2019; Procopio et al., 2019). Nevertheless, further case studies on decomposition are needed, especially on specific geo-climatic regions. On the other hand, the decrease in soil calcium content during the second year of the experiment might be attributed to the reduction in calcium released by the cadaver and, therefore, the late/advanced decomposition of the animal model. This was further confirmed when exhuming the piglets after 2 years of interment, at which point only small fragments of disarticulated bones were left while the skin, flesh, cartilage, and most of the bone tissue were completely decomposed. These findings highlight the potential of using elemental analysis, particularly for calcium, to consolidate the findings from microbial community profiling to confirm PMI/PBI and clandestine grave location.
Throughout this study, the calculated diversity metrics of 0D, 1D and 2D correlated negatively with time while the 1D/0D evenness correlated positively with iron and potassium concentrations, and negatively with phosphorous, sulphur and magnesium (Data not shown). The patterns in piglet soil potassium, phosphorous and iron concentrations were comparable to the changes in ecological indices 0D, 1D, and 2D during the first 5 months of the study, until day 150. Also, two-way ANOVA indicated a significant difference between soils samples collected at different times. These findings suggest that the changes in soil elemental and bacterial community composition were most probably a response to carrion decomposition.
Conclusions
The advent of culture-independent high-throughput molecular identification technologies has revolutionised the study of the microbial community allowing the characterisation of microbial species up to the strain or sub-strain level. Microorganisms are ubiquitous; they adapt to different biotopes and have predictable successions. Also, they are transferred from the cadaver to soil during decomposition and might be considered as physical evidence to be used in forensic investigation (Bishop, 2019) for gravesoil detection, PMI assessment or confirmation/exclusion of links between persons, items and locations (Oliveira and Amorim, 2018). Using the northeast of England for context, this experiment was designed to enrich the knowledge based on carrion decomposition and test the potential of using a combined molecular microbial ecological and elemental analysis approach in decomposition studies to enhance gravesoil detection and/or PMI estimation. Overall, the study's four hypotheses were proven and accepted.
Similar to work by other researchers who investigated tissues that are typical of late decomposition stages, the second year of the current in situ piglet study revealed predominances of phyla such as Acidobacteria, Proteobacteria, Planctomycetes, Verrumicrobia, and Chloroflexi. Generally, phylum level analysis did not provide sufficient differentiation between treatments during the second year of the experiment but were partly indicative of shifts in temperature during subsurface decomposition. These changes in correlation could be used to distinguish soils where carrion decomposition occurred as opposed to soil only. Other key findings were of taxa at higher levels of resolution such as Methylococcales, Sinobacteraceae, Candidatus, and Flavobacterium, which, although not distinguishing mammalian from plant decomposition, differentiated experimental soils (piglet and oak leaf litter) from control-only soils. These are, therefore, potential microbial clock and clandestine grave indicators including for burials where plant material is used to cover criminal activity.
Statistical analysis of 18S rRNA gene DGGE-derived Hill ecological metrics allowed clear differentiation between mammalian decomposition and that of oak leaf litter. Also, it suggested the time at which piglet skin rupture happened (start of active decay). On the other hand, and although bacterial 16S rRNA community diversity analysis did not permit the distinction between piglet and leaf litter soils, it did delineate gravesoils from non-burial controls. For the first time in the literature, NGS-based Hill ecological metrics were calculated and compared to their DGGE equivalents while bacterial signature persistence in soil was tested over a 24-month period. Analysis of the sequencing data confirmed some similarity of the results obtained from the NGS-based and DGGE-derived diversity metrics when considering specific Hill numbers or decomposition/sampling intervals. Similarly, the elemental analysis of soil samples showed that calcium profiles of the piglet-associated soils were distinct from those without carrion.
Overall, this study achieved its aims with several key outcomes highlighted (Supplementary Table 1). (1) The importance of environmental and weather conditions in decomposition is evidenced by revealing the impact of seasonal temperature variation on substrate decomposition and soil microbial community composition. This includes parallel interpretation of temperature as ADD—a measure of time and heat necessary for biological functions instead of time only. (2) Likely bacterial markers that could help discriminate animal-based from plant-based decomposition are identified by NGS and provide further emphasis of the high potential of using soil microbial community characterisation in forensic investigation for the detection of gravesoil and estimation of time-since-death. (3) For the first time in forensic microbial research, we test the usefulness of Hill numbers as ecological indices to discriminate decomposition-impacted soils from controls. (4) Another novel contribution is testing the applicability and relevance for a combined approach re molecular and physicochemical soil analysis for enhanced precision. (5) As proposed previously (Schaldach et al., 2005; Ralebitso-Senior, 2018), we show that holistic multi-parameter approaches such as combining bacterial and fungal ecological indices, soil metabarcoding of the successional microbial community, and climatic records using multivariate analysis, have the potential to help detect gravesoil. For example, an increased microbial diversity during winter could indicate a disturbance to soil due to a recent carrion interment while a low diversity during a warm season might be the consequence of nutrient influx into soil during carrion decomposition. The application of this complementary approach in forensic cases, however, necessitates further case studies, preferably using donated human remains alone and in parallel to mammalian surrogates, and replicates/repeats for increased statistical power in order to confirm and, subsequently, validate the findings in different conditions.
Data Availability Statement
The data generated in this article can be found in the European Nucleotide archive using the accession number PRJEB43763.
Author Contributions
TR-S conceived and designed the study. CB did all the laboratory work and analysed all the study data. TR-S, GT, and HC supervised the laboratory work. CB and TR-S drafted the manuscript. All authors contributed to manuscript revision and read and approved the submitted version.
Funding
CB acknowledges gratefully funding from the Algerian NICC for his Ph.D. research program. TR-S acknowledges gratefully the QR-Global Challenges Research Fund (2019/2020) support from Liverpool John Moores University.
Conflict of Interest
The authors declare that the research was conducted in the absence of any commercial or financial relationships that could be construed as a potential conflict of interest.
Acknowledgments
Grateful acknowledgements are made to Dr. Ayodeji O. Olakanye and Professor Tim Thompson who conceived and designed the initial study with TR-S.
Supplementary Material
The Supplementary Material for this article can be found online at: https://www.frontiersin.org/articles/10.3389/fevo.2021.605817/full#supplementary-material
References
Agapiou, A., Zorba, E., Mikedi, K., McGregor, L., Spiliopoulou, C., and Statheropoulos, M. (2015). Analysis of volatile organic compounds released from the decay of surrogate human models simulating victims of collapsed buildings by thermal desorption-comprehensive two-dimensional gas chromatography-time of flight mass spectrometry. Anal. Chim. Acta 883, 99–108. doi: 10.1016/j.aca.2015.04.024
Aitkenhead-Peterson, J. A., Owings, C. G., Alexander, M. B., Larison, N., and Bytheway, J. A. (2012). Mapping the lateral extent of human cadaver decomposition with soil chemistry. Forensic Sci. Int. 216, 127–134. doi: 10.1016/j.forsciint.2011.09.007
Behrensmeyer, A. K. (1978). Taphonomic and ecologic information from bone weathering. Paleobiology 4, 150–162. doi: 10.1017/S0094837300005820
Belk, A., Xu, Z. Z., Carter, D. O., Lynne, A., Bucheli, S., Knight, R., et al. (2018). microbiome data accurately predicts the postmortem interval using random forest regression models. Genes 9:104. doi: 10.3390/genes9020104
Benninger, L. A., Carter, D. O., and Forbes, S. L. (2008). The biochemical alteration of soil beneath a decomposing carcass. Forensic Sci. Int. 180, 70–75. doi: 10.1016/j.forsciint.2008.07.001
Bishop, A. H. (2019). The signatures of microorganisms and of human and environmental biomes can now be used to provide evidence in legal cases. FEMS Microbiol. Lett. 366:fnz021. doi: 10.1093/femsle/fnz021
Bisker, C., and Ralebitso-Senior, T. K. (2018). “The method debate: a state-of-the-art analysis of PMI estimation techniques,” in Forensic Ecogenomics: The Application of Microbial Ecology Analyses in Forensic Contexts, ed T. K. Ralebitso-Senior (London: Academic Press), 61–86. doi: 10.1016/B978-0-12-809360-3.00003-5
Bolyen, E., Rideout, J. R., Dillon, M. R., Bokulich, N. A., Abnet, C. C., Gabriel, A., et al. (2018). QIIME 2 : reproducible, interactive, scalable, and extensible microbiome data science. PeerJ. 37, 852–857. doi: 10.1038/s41587-019-0209-9
Brandt, L. A., King, J. Y., and Milchunas, D. G. (2007). Effects of ultraviolet radiation on litter decomposition depend on precipitation and litter chemistry in a shortgrass steppe ecosystem. Glob. Chang. Biol. 13, 2193–2205. doi: 10.1111/j.1365-2486.2007.01428.x
Campobasso, C., Pietro Di Vella, G., and Introna, F. (2001). Factors affecting decomposition and Diptera colonization. Forensic Sci. Int. 120, 18–27. doi: 10.1016/S0379-0738(01)00411-X
Can, I., Javan, G. T., Pozhitkov, A. E., and Noble, P. A. (2014). Distinctive thanatomicrobiome signatures found in the blood and internal organs of humans. J. Microbiol. Methods 106, 1–7. doi: 10.1016/j.mimet.2014.07.026
Carter, D. O., and Tibbett, M. (2006). Microbial decomposition of skeletal muscle tissue (Ovis aries) in a sandy loam soil at different temperatures. Soil Biol. Biochem. 38, 1139–1145. doi: 10.1016/j.soilbio.2005.09.014
Carter, D. O., Yellowlees, D., and Tibbett, M. (2007). Cadaver decomposition in terrestrial ecosystems. Naturwissenschaften 94, 12–24. doi: 10.1007/s00114-006-0159-1
Carter, D. O., Yellowlees, D., and Tibbett, M. (2008). Temperature affects microbial decomposition of cadavers (Rattus rattus) in contrasting soils. Appl. Soil Ecol. 40, 129–137. doi: 10.1016/j.apsoil.2008.03.010
Chao, A., Chiu, C. -H., and Jost, L. (2010). Phylogenetic diversity measures based on Hill numbers. Philos. Transac. R. Soc. B Biol. Sci. 365, 3599–3609. doi: 10.1098/rstb.2010.0272
Chao, A., Chiu, C. -H., and Jost, L. (2014). Unifying species diversity, phylogenetic diversity, functional diversity, and related similarity and differentiation measures through Hill numbers. Ann. Rev. 45, 297–324. doi: 10.1146/annurev-ecolsys-120213-091540
Chimutsa, M., Olakanye, A. O., Thompson, T. J. U., and Ralebitso-Senior, T. K. (2015). Soil fungal community shift evaluation as a potential cadaver decomposition indicator. Forensic Sci. Int. 257, 155–159. doi: 10.1016/j.forsciint.2015.08.005
Cobaugh, K. L., Schaeffer, S. M., and DeBruyn, J. M. (2015). Functional and structural succession of soil microbial communities below decomposing human cadavers. PLoS ONE 10:e0130201. doi: 10.1371/journal.pone.0130201
Cockle, D. L., and Bell, L. S. (2017). The environmental variables that impact human decomposition in terrestrially exposed contexts within Canada. Sci. Just. 57, 107–117. doi: 10.1016/j.scijus.2016.11.001
Connor, M., Baigent, C., and Hansen, E. S. (2018). Testing the use of pigs as human proxies in decomposition studies. J. Forensic Sci. 63, 1350–1355. doi: 10.1111/1556-4029.13727
Cordeiro, C., Ordóñez-Mayán, L., Lendoiro, E., Febrero-Bande, M., Vieira, D. N., and Muñoz-Barús, J. I. (2019). A reliable method for estimating the postmortem interval from the biochemistry of the vitreous humor, temperature and body weight. Forensic Sci. Int. 295, 157–168. doi: 10.1016/j.forsciint.2018.12.007
Costa, I., Carvalho, F., Magalhães, T., Guedes de Pinho, P., Silvestre, R., and Dinis-Oliveira, R. J. (2015). Promising blood-derived biomarkers for estimation of the postmortem interval. Toxicol. Res. 4, 1443–1452. doi: 10.1039/C5TX00209E
Damann, F. E., Williams, D. E., and Layton, A. C. (2015). Potential use of bacterial community succession in decaying human bone for estimating postmortem interval. J. Forensic Sci. 60, 844–850. doi: 10.1111/1556-4029.12744
Elfstrand, S., Båth, B., and Mårtensson, A. (2007). Influence of various forms of green manure amendment on soil microbial community composition, enzyme activity and nutrient levels in leek. Appl. Soil Ecol. 36, 70–82. doi: 10.1016/j.apsoil.2006.11.001
Finley, S. J., Benbow, M. E., and Javan, G. T. (2015a). Microbial communities associated with human decomposition and their potential use as postmortem clocks. Int. J. Legal Med. 129, 623–632. doi: 10.1007/s00414-014-1059-0
Finley, S. J., Benbow, M. E., and Javan, G. T. (2015b). Potential applications of soil microbial ecology and next-generation sequencing in criminal investigations. Appl. Soil Ecol. 88, 69–78. doi: 10.1016/j.apsoil.2015.01.001
Finley, S. J., Pechal, J. L., Benbow, M. E., Robertson, B. K., and Javan, G. T. (2016). Microbial signatures of cadaver gravesoil during decomposition. Microb. Ecol. 71, 524–529. doi: 10.1007/s00248-015-0725-1
Gaxiola, A., and Armesto, J. J. (2015). Understanding litter decomposition in semiarid ecosystems: linking leaf traits, UV exposure and rainfall variability. Front. Plant Sci. 6:140. doi: 10.3389/fpls.2015.00140
Goff, M. L. (2009). “Early Postmortem Changes and Stages of Decomposition”, in Current Concepts in Forensic Entomology, eds J. Amendt, M. Goff, C. Campobasso, M. Grassberger (Dordrecht: Springer), 1–24. doi: 10.1007/978-1-4020-9684-6_1
Guo, J., Fu, X., Liao, H., Hu, Z., Long, L., and Yan, W. (2016). Potential use of bacterial community succession for estimating post-mortem interval as revealed by high-throughput sequencing. Nat. Publish. Group 2015, 1–11. doi: 10.1038/srep24197
Hall, M., and Beiko, R. G. (2018). “16S rRNA gene analysis with QIIME2,” in Microbiome Analysis, eds R. Beiko, W. Hsiao, and J. Parkinson (New York, NY: Humana Press), 113–129. doi: 10.1007/978-1-4939-8728-3_8
Hart, M. L., Meyer, A., Johnson, P. J., and Ericsson, A. C. (2015). Comparative evaluation of DNA extraction methods from feces of multiple host species for downstream next-generation sequencing. PLoS ONE 10:e014333. doi: 10.1371/journal.pone.0143334
Hau, T. C., Hamzah, N. H., Lian, H. H., and Amir Hamzah, S. P. A. (2014). Decomposition process and post mortem changes: review. Sains Malays. 43, 1873–1882. doi: 10.17576/jsm-2014-4312-08
Hopkins, D. W., Wiltshire, P. E. J., and Turner, B. D. (2000). Microbial characteristics of soils from graves: an investigation at the interface of soil microbiology and forensic science. Appl. Soil Ecol. 14, 283–288. doi: 10.1016/S0929-1393(00)00063-9
Hyde, E. R., Metcalf, J. L., Bucheli, S. R., Lynne, A. M., and Knight, R. (2017). “Microbial communities associated with decomposing corpses”, in Forensic Microbiology, eds D. O. Carter, J. K. Tomberlin, M. E. Benbow, and J. L. Metcalf (Chichester: John Wiley & Sons Ltd.), 245–273. doi: 10.1002/9781119062585.ch10
Irish, L., Rennie, S. R., Parkes, G. M. B., and Williams, A. (2019). Identification of decomposition volatile organic compounds from surface- deposited and submerged porcine remains. Sci. Just. 59, 503–515. doi: 10.1016/j.scijus.2019.03.007
Iyengar, A., and Hadi, S. (2014). Use of non-human DNA analysis in forensic science: a mini review.' Med. Sci. Law 54, 41–50. doi: 10.1177/0025802413487522
Kasper, J., Mumm, R., and Ruther, J. (2012). The composition of carcass volatile profiles in relation to storage time and climate conditions. Forensic Sci. Int. 223, 64–71. doi: 10.1016/j.forsciint.2012.08.001
Kim, M., Heo, E., Kang, H., and Adams, J. (2013). Changes in oil bacterial community structure with increasing disturbance frequency. Microb. Ecol. 66, 171–181. doi: 10.1007/s00248-013-0237-9
Knobel, Z., Ueland, M., Nizio, K. D., Patel, D., and Forbes, S. L. (2018). A comparison of human and pig decomposition rates and odour profiles in an Australian environment. Aust. J. Forensic Sci. 51, 557–572. doi: 10.1080/00450618.2018.1439100
Kozich, J. J., Westcott, S. L., Baxter, N. T., Highlander, S. K., and Schloss, P. D. (2013). Development of a dual-index sequencing strategy and curation pipeline for analyzing amplicon sequence data on the miseq illumina sequencing platform. Appl. Environ. Microbiol. 79, 5112–5120. doi: 10.1128/AEM.01043-13
Lauber, C. L., Metcalf, J. L., Keepers, K., Ackermann, G., Carter, D. O., and Knight, R. (2014). Vertebrate decomposition is accelerated by soil microbes. Appl. Environ. Microbiol. 80, 4920–4929. doi: 10.1128/AEM.00957-14
Litten-Brown, J. C., Corson, A. M., and Clarke, L. (2010). Porcine models for the metabolic syndrome, digestive and bone disorders: a general overview. Animal 4, 899–920. doi: 10.1017/S1751731110000200
Macdonald, B. C. T., Farrell, M., Tuomi, S., Barton, P. S., Cunningham, S. A., and Manning, A. D. (2014). Carrion decomposition causes large and lasting effects on soil amino acid and peptide flux. Soil Biol. Biochem. 69, 132–140. doi: 10.1016/j.soilbio.2013.10.042
Marín-Roldan, A., Manzoor, S., Moncayo, S., Navarro-Villoslada, F., Izquierdo-Hornillos, R. C., and Caceres, J. O. (2013). Determination of the postmortem interval by Laser Induced Breakdown Spectroscopy using swine skeletal muscles. Spectrochim. ActaPart B Atomic Spectrosc. 88, 186–191. doi: 10.1016/j.sab.2013.07.008
Megyesi, M. S., Nawrocki, S. P., and Haskell, N. H. (2005). Using accumulated degree-days to estimate the postmortem interval from decomposed human remains. J. Forensic Sci. 50, 618–626. doi: 10.1520/JFS2004017
Melis, C., Selva, N., Teurlings, I., Skarpe, C., Linnell, J. D. C., and Andersen, R. (2007). Soil and vegetation nutrient response to bison carcasses in Białowieza Primeval Forest, Poland. Ecol. Res. 22, 807–813. doi: 10.1007/s11284-006-0321-4
Metcalf, J. L., Wegener Parfrey, L., Gonzalez, A., Lauber, C. L., Knights, D., Ackermann, G., et al. (2013). A microbial clock provides an accurate estimate of the postmortem interval in a mouse model system. Elife 2:e01104. doi: 10.7554/eLife.01104
Metcalf, J. L., Xu, Z. Z., Weiss, S., Lax, S., Van Treuren, W., Hyde, E. R., et al. (2016). Microbial community assembly and metabolic function during mammalian corpse decomposition. Science 351, 158–162. doi: 10.1126/science.aad2646
Muyzer, G., De Waal, E. C., and Uitterlinden, A. G. (1993). Profiling of complex microbial populations by denaturing gradient gel electrophoresis analysis of polymerase chain reaction-amplified genes coding for 16S rRNA. Appl. Environ. Microbiol. 59, 695–700. doi: 10.1128/AEM.59.3.695-700.1993
Nearing, J. T., Douglas, G. M., Comeau, A. M., and Langille, M. G. I. (2018). Denoising the Denoisers : an independent evaluation of microbiome sequence error-correction approaches. PeerJ. 6:e5364. doi: 10.7717/peerj.5364
Nolan, A.-N., Mead, R. J., Maker, G., Bringans, S., Chapman, B., and Speers, S. J. (2019). Examination of the temporal variation of peptide content in decomposition fluid under controlled conditions using pigs as human substitutes. Forensic Sci. Int. 298, 161–168. doi: 10.1016/j.forsciint.2019.02.048
Olakanye, A. O., Nelson, A., and Ralebitso-Senior, T. K. (2017). A comparative in situ decomposition study using still born piglets and leaf litter from a deciduous forest. Forensic Sci. Int. 276, 85–92. doi: 10.1016/j.forsciint.2017.04.024
Olakanye, A. O., and Ralebitso-Senior, T. K. (2018). Soil metabarcoding identifies season indicators and differentiators of pig and Agrostis/Festuca spp. decomposition. Forensic Sci. Int. 288, 53–58. doi: 10.1016/j.forsciint.2018.04.015
Olakanye, A. O., Thompson, T., and Ralebitso-Senior, T. K. (2015). Shifts in soil biodiversity - a forensic comparison between Sus scrofa domesticus and vegetation decomposition. Sci. Just. 55, 402–407. doi: 10.1016/j.scijus.2015.07.004
Oliveira, M., and Amorim, A. (2018). Microbial forensics: new breakthroughs and future prospects. Appl. Microbiol. Biotechnol. 102, 10377–10391. doi: 10.1007/s00253-018-9414-6
Ortmann, J., Markwerth, P., and Madea, B. (2016). Precision of estimating the time since death by vitreous potassium - comparison of 5 different equations. Forensic Sci. Int. 269, 1–7. doi: 10.1016/j.forsciint.2016.10.005
Pechal, J. L., Crippen, T. L., Benbow, M. E., Tarone, A. M., Dowd, S., and Tomberlin, J. K. (2014). The potential use of bacterial community succession in forensics as described by high throughput metagenomic sequencing. Int. J. Legal Med. 128, 193–205. doi: 10.1007/s00414-013-0872-1
Pechal, J. L., Crippen, T. L., Tarone, A. M., Lewis, A. J., Tomberlin, J. K., and Benbow, M. E. (2013). Microbial community functional change during vertebrate carrion decomposition. PLoS ONE 8:e79035. doi: 10.1371/journal.pone.0079035
Pechal, J. L., Schmidt, C. J., Jordan, H. R., and Benbow, M. E. (2018). A large-scale survey of the postmortem human microbiome, and its potential to provide insight into the living health condition. Sci. Rep. 8, 1–15. doi: 10.1038/s41598-018-23989-w
Pinheiro, J. (2006). “Decay process of a cadaver,” in A. Schmitt, E. Cunha, and J. Pinheiro, eds. Forensic Anthropology and Medicine, Complementary Sciences From Recovery to Cause of Death (New Jersey, NJ: Humana Press Inc.), 85–116.
Procopio, N., Ghignone, S., Williams, A., Chamberlain, A., Mello, A., and Buckley, M. (2019). Metabarcoding to investigate changes in soil microbial communities within forensic burial contexts. Forensic Sci. Int. Genet. 39, 73–85. doi: 10.1016/j.fsigen.2018.12.002
Ralebitso-Senior, T. K., (ed.). (2018). “Summary: an assessment of achievements, limitations, and potential of forensic ecogenomics,” in Forensic Ecogenomics: The Application of Microbial Ecology Analyses in Forensic Contexts, (London: Academic Press), 211–234. doi: 10.1016/B978-0-12-809360-3.00009-6
Ralebitso-Senior, T. K., and Olakanye, A. O. (2018). “General Introduction: Method applications at the interface of microbial ecology and forensic investigation,” in Forensic Ecogenomics: The Application of Microbial Ecology Analyses in Forensic Contexts, ed T. K. Ralebitso-Senior (London: Academic Press), 1–35. doi: 10.1016/B978-0-12-809360-3.00001-1
Ralebitso-Senior, T. K., and Pyle, M. K. P. (2018). “Implications of the investigative animal model,” in Forensic Ecogenomics: The Application of Microbial Ecology Analyses in Forensic Contexts, ed T. K. Ralebitso-Senior (London: Academic Press), 87–111. doi: 10.1016/B978-0-12-809360-3.00004-7
Ravansari, R., and Lemke, L. D. (2018). Portable X-ray Fluorescence trace metal measurement in organic rich soils: pXRF response as a function of organic matter fraction. Geoderma 319, 175–184. doi: 10.1016/j.geoderma.2018.01.011
Ritz, K., McNicol, J. W., Nunan, N., Grayston, S., Millard, P., Atkinson, D., et al. (2004). Spatial structure in soil chemical and microbiological properties in an upland grassland. FEMS Microbiol. Ecol. 49, 191–205. doi: 10.1016/j.femsec.2004.03.005
Schaldach, C. M., Bench, G., DeYoreo, J. J., Esposito, T., Fergenson, D. P., Ferreira, J., et al. (2005). “Non-DNA methods for biological signatures,” in Microbial Forensics, B. Budowle, S. E. Schutzer, and R. G. Breeze (Cambridge, MA: Elsevier), 251–294. doi: 10.1016/B978-012088483-4/50016-0
Shand, C. A., and Wendler, R. (2014). Portable X-ray Fluorescence analysis of mineral and organic soils and the influence of organic matter. J. Geochem. Explorat. 143, 31–42. doi: 10.1016/j.gexplo.2014.03.005
Shean, B. S., Messinger, L., and Papworth, M. (1993). Observations of differential decomposition on sun exposed v. shaded pig carrion in coastal Washington State. J. Forensic Sci. 38, 938–949.
Shirley, N. R., Wilson, R. J., and Jantz, L. M. (2011). Cadaver use at the University of Tennessee's Anthropological Research Facility. Clin. Anat. 24, 372–380. doi: 10.1002/ca.21154
Siddhamsetty, A. K., Verma, S. K., Kohli, A., Verma, A., Puri, D., and Singh, A. (2014). Exploring time of death from potassium, sodium, chloride, glucose and calcium analysis of postmortem synovial fluid in semi arid climate. J. Forensic Leg. Med. 28, 11–14. doi: 10.1016/j.jflm.2014.09.004
Singh, B., Minick, K. J., Strickland, M. S., Wickings, K. G., Crippen, T. L., Tarone, A. M., et al. (2018). Temporal and spatial impact of human cadaver decomposition on soil bacterial and arthropod community structure and function. Front. Microbiol. 8:2616. doi: 10.3389/fmicb.2017.02616
Statheropoulos, M., Agapiou, A., Zorba, E., Mikedi, K., Karma, S., Pallis, G. C., et al. (2011). Combined chemical and optical methods for monitoring the early decay stages of surrogate human models. Forensic Sci. Int. 210, 154–163. doi: 10.1016/j.forsciint.2011.02.023
Stokes, K. L., Forbes, S. L., and Tibbett, M. (2009). Freezing skeletal muscle tissue does not affect its decomposition in soil: evidence from temporal changes in tissue mass, microbial activity and soil chemistry based on excised samples. Forensic Sci. Int. 183, 6–13. doi: 10.1016/j.forsciint.2008.08.013
Stokes, K. L., Forbes, S. L., and Tibbett, M. (2013). Human versus animal: contrasting decomposition dynamics of mammalian analogues in experimental taphonomy. J. Forensic Sci. 58, 583–591. doi: 10.1111/1556-4029.12115
Sutherland, A., Myburgh, J., Steyn, M., and Becker, P. J. J. (2013). The effect of body size on the rate of decomposition in a temperate region of South Africa'. Forensic Sci. Int. 231, 257–262. doi: 10.1016/j.forsciint.2013.05.035
Szelecz, I., Koenig, I., Seppey, C. V. W., Le Bayon, R. C., and Mitchell, E. A. D. (2018). Soil chemistry changes beneath decomposing cadavers over a one-year period. Forensic Sci. Int. 286, 155–165. doi: 10.1016/j.forsciint.2018.02.031
Torsvik, V. L. (1980). Isolation of bacterial DNA from soil. Soil Biol. Biochem. 12, 15–21. doi: 10.1016/0038-0717(80)90097-8
Tumer, A. R., Karacaoglu, E., Namli, A., Keten, A., Farasat, S., Akcan, R., et al. (2013). Effects of different types of soil on decomposition: an experimental study. Leg. Med. 15, 149–156. doi: 10.1016/j.legalmed.2012.11.003
Vass, A. A., Bass, W. M., Wolt, J. D., Foss, J. E., and Ammons, J. T. (1992). Time since death determinations of human cadavers using soil solution. J. Forensic Sci. 37, 1236–1253. doi: 10.1520/JFS13311J
Voss, S. C., Cook, D. F., and Dadour, I. R. (2011). Decomposition and insect succession of clothed and unclothed carcasses in Western Australia. Forensic Sci. Int. 211, 67–75. doi: 10.1016/j.forsciint.2011.04.018
Wang, J., Liu, L., Wang, X., and Chen, Y. (2015). The interaction between abiotic photodegradation and microbial decomposition under ultraviolet radiation. Glob. Chang. Biol. 21, 2095–2104. doi: 10.1111/gcb.12812
Wang, M., Zheng, Q., Shen, Q., and Guo, S. (2013). The critical role of potassium in plant stress response. Int. J. Mol. Sci. 14, 7370–7390. doi: 10.3390/ijms14047370
White, T., Bruns, T., and Lee, S. (1990). “Amplification and direct sequencing of fungal ribosomal RNA genes for phylogenetics,” in PCR Protocols: A Guide to Methods and Applications, eds M. A. Innis, D. H. Gelfand, J. J. Sninsky, and T. J. White (Cambridge, MA: Academic Press) Vol. 18, 315–322.
Williams, A., Rogers, C. J., and Cassella, J. P. (2019). Why does the UK need a Human Taphonomy Facility? Forensic Sci. Int. 296, 74–79. doi: 10.1016/j.forsciint.2019.01.010
Williams, R., Taylor, G., and Orr, C. (2020). pXRF method development for elemental analysis of archaeological soil. Archaeometry 62, 1–19. doi: 10.1111/arcm.12583
Keywords: decomposition, Sus scrofa domesticus, microbial community succession, hill diversity indices, clandestine grave
Citation: Bisker C, Taylor G, Carney H and Ralebitso-Senior TK (2021) A Combined Application of Molecular Microbial Ecology and Elemental Analyses Can Advance the Understanding of Decomposition Dynamics. Front. Ecol. Evol. 9:605817. doi: 10.3389/fevo.2021.605817
Received: 02 December 2020; Accepted: 06 April 2021;
Published: 13 May 2021.
Edited by:
Manuela Oliveira, University of Porto, PortugalReviewed by:
Sheree J. Finley, Alabama State University, United StatesDanijela P. Miljkovic, University of Belgrade, Serbia
Jie Liu, Nankai University, China
Copyright © 2021 Bisker, Taylor, Carney and Ralebitso-Senior. This is an open-access article distributed under the terms of the Creative Commons Attribution License (CC BY). The use, distribution or reproduction in other forums is permitted, provided the original author(s) and the copyright owner(s) are credited and that the original publication in this journal is cited, in accordance with accepted academic practice. No use, distribution or reproduction is permitted which does not comply with these terms.
*Correspondence: Theresia Komang Ralebitso-Senior, dC5rLnJhbGViaXRzb3NlbmlvciYjeDAwMDQwO2xqbXUuYWMudWs=