- 1Pacific Northwest National Laboratory, Richland, WA, United States
- 2School of Biological Sciences, Washington State University, Pullman, WA, United States
- 3Department of Soil and Crop Sciences, Texas A&M University, College Station, TX, United States
Transparent, open, and reproducible research is still far from routine, and the full potential of open science has not yet been realized. Crowdsourcing–defined as the usage of a flexible open call to a heterogeneous group of individuals to recruit volunteers for a task –is an emerging scientific model that encourages larger and more outwardly transparent collaborations. While crowdsourcing, particularly through citizen- or community-based science, has been increasing over the last decade in ecological research, it remains infrequently used as a means of generating scientific knowledge in comparison to more traditional approaches. We explored a new implementation of crowdsourcing by using an open call on social media to assess its utility to address fundamental ecological questions. We specifically focused on pervasive challenges in predicting, mitigating, and understanding the consequences of disturbances. In this paper, we briefly review open science concepts and their benefits, and then focus on the new methods we used to generate a scientific publication. We share our approach, lessons learned, and potential pathways forward for expanding open science. Our model is based on the beliefs that social media can be a powerful tool for idea generation and that open collaborative writing processes can enhance scientific outcomes. We structured the project in five phases: (1) draft idea generation, (2) leadership team recruitment and project development, (3) open collaborator recruitment via social media, (4) iterative paper development, and (5) final editing, authorship assignment, and submission by the leadership team. We observed benefits including: facilitating connections between unusual networks of scientists, providing opportunities for early career and underrepresented groups of scientists, and rapid knowledge exchange that generated multidisciplinary ideas. We also identified areas for improvement, highlighting biases in the individuals that self-selected participation and acknowledging remaining barriers to contributing new or incompletely formed ideas into a public document. While shifting scientific paradigms to completely open science is a long-term process, our hope in publishing this work is to encourage others to build upon and improve our efforts in new and creative ways.
Introduction
Many areas of research have expressed the need for transparency and accessibility through all stages of the scientific process, collectively termed “open science” (Fecher and Friesike, 2014; Friesike et al., 2015; Hampton et al., 2015; Nosek et al., 2015; McKiernan et al., 2016; Vicente-Sáez and Martínez-Fuentes, 2018; Powers and Hampton, 2019). Open science has manifested via multiple avenues, most notably through collaborative networks and public access to data, code, and papers (Hampton et al., 2015; Vicente-Sáez and Martínez-Fuentes, 2018). Indeed, calls for transparency have been recognized by funding agencies which now largely require some extent of openness. The National Institutes of Health (NIH), National Science Foundation (NSF), Centers for Disease Control and Prevention (CDC), Departments of Defense (DoD) and Energy (DOE), and National Aeronautics and Space Administration (NASA) each have imposed data management and sharing requirements (McKiernan et al., 2016). However, although open science principles are recognized as vital by most scientists (Nosek et al., 2012; McNutt, 2014; Miguel et al., 2014), the implementation of these practices in research pipelines is still far from routine (Nosek et al., 2012; McKiernan et al., 2016; O’Boyle et al., 2017). Within open science, citizen science (Dickinson et al., 2010, 2012; Newman et al., 2012; Kobori et al., 2016) and crowdsourced science (Fink et al., 2014; Muller et al., 2015) have emerged as key contributors in the field of ecology. Crowdsourcing–defined as the usage of a flexible open call to a heterogeneous group of individuals to recruit volunteers for a task, a definition modified from Estellés-Arolas and González-Ladrón-de-Guevara (2012) who reviewed and synthesized 32 definitions of crowdsourcing in published literature–in particular is increasingly accessible with technological advances that facilitate connectivity among disparate individuals.
Changing scientific paradigms to completely open science necessitates significant cultural, perspective, and perhaps generational changes, but incremental progress is already evident. Within ecology, open science to some extent is mandated by most funding agencies, and practices that encourage data availability are pervasive. However, there are range of open science approaches and implementations within ecological research. Here, we review open science practices and describe a new experiment in using scientific crowdsourcing to facilitate synthesis of global perspectives in addressing one of the most pressing current ecological challenges – predicting, mitigating, and understanding the consequences of disturbances. In contrast to traditional publication models, we evaluated if a totally open and transparent publication model could be successful in today’s scientific landscape. Our model is based on the beliefs that social media can be a powerful facilitator of idea generation rather than a divider (Graham and Krause, 2020) and that collaborative and iterative writing processes done openly can enhance scientific outcomes. This process resulted in a published manuscript (Graham et al., 2021). Below, we review the benefits of open science and crowdsourcing approaches across scientific domains and within ecology. We share our approach for this project, lessons learned, and potential pathways forward. Our hope in publishing this work is to encourage others to build upon and improve our efforts in new and creative ways.
Open Science and Crowdsourcing in Ecology
Across all scientific domains including ecology, emerging models of research and publishing are shifting historical paradigms from small teams of researchers with limited scopes toward larger and more outwardly transparent collaborations that can yield many benefits. Termed “vertical” science by Uhlmann et al. (2019), traditional scientific models often consist of siloed research groups that work together to generate questions, hypotheses, data, and ultimately publications. After peer review by select colleagues under this model, research enters the scientific domain for discussion, criticism, and extension. While this traditional approach has produced many fruits, scientists are forced into many decisions in this framework due to the constraints of time, resources, and expertise. For example, within a given funding allocation, researchers often choose between small and detailed versus large and more cursory investigations; and cultural pressures and career incentives to publish can bias decisions toward more rapid studies versus longer and more replicated endeavors. Traditional vertical approaches have been shown to fail with respect to sample size and distribution (Henrich et al., 2010; Lemoine et al., 2016), independent experimental replication and variety in study design (Wells and Windschitl, 1999; Judd et al., 2012; Makel et al., 2012; Simons, 2014; Lemoine et al., 2016; Mueller-Langer et al., 2019; Fraser et al., 2020), and breadth of data collection and analysis perspectives (Simmons et al., 2011; Gelman and Loken, 2014; Silberzahn et al., 2018).
By contrast, newer open science approaches are comprised of widespread researchers that can collectively brainstorm, implement, and self-review work at every stage of the scientific pipeline [termed “horizontal science” by Uhlmann et al. (2019)]. Horizontal science can complement traditional approaches by increasing inclusivity and transparency, distributing resource burdens among many individuals, and increasing scientific rigor (Uhlmann et al., 2019). In ecology, horizontal science is exemplified by recent efforts in crowdsourced and citizen science (differentiated from crowdsourced science as the contribution of non-scientists specifically to data collection and/or analysis). These approaches been used to monitor insect, plant, coral, bird, and other wildfire populations (Marshall et al., 2012; Sullivan et al., 2014; Swanson et al., 2016; Hunt et al., 2017; Osawa et al., 2017; Hsing et al., 2018). Betini et al. (2017) recently highlighted the ability of horizontal science to evaluate multiple competing hypotheses, in contrast to the traditional scientific model of evaluating a limited set of hypotheses. Importantly, vertical and horizontal approaches need not be mutually exclusive, and there exists a continuum of implementations that span ranges of open science approaches and number of collaborators at every step (Uhlmann et al., 2019).
While specific definitions of “open science” vary among fields and even among researchers within a given field, many derive from Nielsen (2011) that defines open science as “the idea that scientific knowledge of all kinds should be openly shared as early as is practical in the discovery process” (Friesike et al., 2015). With complete openness, this means communication with both the general public and scientists throughout the scientific process (from pre-concept to post-publication) that provides full transparency as well as sharing of data and code (Hampton et al., 2015; Powers and Hampton, 2019). For instance, ideas could be generated via social media, blog discussions, or other widely used global forums leading to emergent collaborations executed in open online platforms (e.g., JuPyter notebooks; Overleaf, Google Docs) (Powers and Hampton, 2019). Citizen science efforts that are organized via online platforms and/or provide updates on project development are a common effort toward transparency by ecologists engaging in open science (e.g., project via platforms like Pathfinder, CoralWatch, Marshall et al., 2012; eBird, Sullivan et al., 2014; PhragNet, Hunt et al., 2017). Nested within open science is the concept of open innovation that encourages transparency throughout a project’s life-cycle (Friesike et al., 2015). Open innovation can lead to iterative review and refinement that reduces redundancy between projects and accelerates research fields (Byrnes et al., 2014; Hampton et al., 2015). Yet, while there has been tremendous growth in open science, the implementation of open science strategies is heavily skewed toward the later stages of development in most fields (e.g., preprints; code, data, and postprint archiving) and largely ignore the initial stages of open innovation (Friesike et al., 2015). This is in part because researchers have varying levels of comfort with different aspects of open scientific pipelines, leading to a continuum of openness (McKiernan et al., 2016). Key reasons include a feeling of uncertainty surrounding how open science can impact careers, loss of control over idea development and implementation, and time investment in learning new standard practices (Hampton et al., 2015; McKiernan et al., 2016). Open science at its most basic level includes self-archiving postprints, while higher levels of openness may include sharing grant proposals, data, preprints, and research protocols (Berg et al., 2016; McKiernan et al., 2016).
Because of biases in open science toward later research stages, there’s an enormous amount of untapped potential to drive research even further toward complete openness. Among open science successes, software development and data analysis and archiving have led the way. They now have well-defined workflows implemented with online tools including widespread usage of GitHub and Python Notebooks, open codes and software packages (R and python), data standards and archiving (ICON-FAIR), and preprints (Woelfle et al., 2011). State-of-the-art data analysis packages are developed and used openly; a prime example is the “scikit-learn” machine learning Python package that yielded over 500 contributors and 2,500 citations within its first five years (Pedregosa et al., 2011; McKiernan et al., 2016). Though ecological fields have been slower to adopt open science approaches, an abundance of ecological networks have been established to provide open data and facilitate collaborations (e.g., long-term ecological research stations, critical zone observatories, Nutrient Network, International Soil Carbon Network), and preprinting submitted manuscripts and data archiving for accepted manuscripts have been broadly adopted (Powers and Hampton, 2019). Citizen science and crowdsourced data collection have also emerged as key open science approaches in the ecological sciences. For example, the Open Traits Network monitors a variety of species traits across the globe (Gallagher et al., 2019), PhragNet monitors invasive Phragmites populations (Hunt et al., 2017), eBird and the Neighborhood Nestwatch Program track bird populations (Evans et al., 2005; Sullivan et al., 2014), and CoralWatch monitors coral health (Marshall et al., 2012). Other disciplines are following similar trajectories – for example, half of cognitive science articles may include citizen contributed samples in the next few years (Stewart et al., 2017) and public and environmental health fields are increasingly reliant on open contributions and preprints to rapidly advance progress (English et al., 2018; Johansson et al., 2018).
Crowdsourcing distributes problem-solving among individuals through open calls and is a key contributor to open science advancement in many fields (Chatzimilioudis et al., 2012; Uhlmann et al., 2019). Crowdsourcing efforts vary in breadth from coordination of largely independent work to intense sharing of all activities. The benefits of crowdsourcing may include maximizing resources and diversifying contributions to facilitate large science questions and tasks and to increase reliability (Catlin-Groves, 2012; Pocock et al., 2017; Uhlmann et al., 2019), though less research has been done on the impacts of crowdsourcing approaches relative to other aspects of open science. As nicely stated by Uhlmann et al. (2019) crowdsourcing shifts the norms of scientific culture from asking “what is the best we can do with the resources we have to investigate our question?” to “what is the best way to investigate our question, so that we can decide what resources to recruit?.” A key feature of crowdsourcing is a reliance on raising project awareness to facilitate engagement (Woelfle et al., 2011). While a few platforms exist to help structure scientific crowdsourcing projects (e.g., Zooinverse, citizenscience.gov, pathfinderscience.net), the usage of crowdsourcing for commercial applications still outnumbers scientific crowdsourcing (e.g., InnoCentive, Jovoto, Waze, NoiseTube, City-Explorer, SignalGuru) (Chatzimilioudis et al., 2012; Friesike et al., 2015).
Benefits of Open Science
Our experience is an encouraging example of a new open science implementation applied to disturbance ecology in which both top-down leadership and open contributions are commingled to maximize benefits associated with different scientific models. Traditional vs. open approaches have been described with the analogy of a hierarchical “cathedral”-like model vs. a distributed “bazaar”-like model. In a cathedral-like model, one person is in charge of a small group of skilled workers with substantial barriers to entry, while bazaars encompass a more chaotic but fluid structure with little leadership that is reliant on community participation and has low barriers to entry (Raymond, 1999; Woelfle et al., 2011). However, there is a continuum of approaches between the two ends of this spectrum in which both organization and open contributions can exist. For example, in ecology, efforts have including both opportunistic cataloging of species distributions, water quality, and coral reef health (e.g., Marshall et al., 2012; Sullivan et al., 2014; Poisson et al., 2020; Ver Hoef et al., 2021) to targeted investigations of specific locations with more narrowly defined study objectives (e.g., McDuffie et al., 2019; Tang et al., 2020; Heres et al., 2021). We see the major benefits of intermediate approaches as: facilitating connections between networks of scientists that would not normally interact, providing opportunities for early career and underrepresented groups of scientists with perspectives that are muted by traditional approaches, faster knowledge dissemination that can spark creativity and new ideas in others, and generating multidisciplinary ideas that can only emerge when broad perspectives are synthesized.
The internet has enabled a “global college” of researchers and multi-institutional collaborations are now normal in high-impact research (Wuchty et al., 2007; Wagner, 2009; Hampton et al., 2015). Open science can facilitate these interactions and increase research visibility, while also leveling the playing field for early career researchers, underrepresented groups, and researchers with limited funding (McKiernan et al., 2016). Early career and underrepresented researchers, as well as those from lesser known institutions or poorly funded countries, are at a competitive disadvantage (Petersen et al., 2011; Wahls, 2018); however, these researchers possess a considerable amount of talent that can be suppressed by a lack of access to resources, for instance to specialized instrumentation or to student or postdoctoral researchers. Crowdsourcing can provide inclusiveness where these researchers can exchange ideas based on merit and contribute to high-impact projects without being as strongly inhibited by resource availability (Uhlmann et al., 2019). Additionally, open science projects do not need to stop with the termination of one individual’s funding, as others can continue the work, or a lack of funding entirely, as there are many ideas that can be facilitated by those with more access to funding or other available resources (Woelfle et al., 2011).
Other benefits include relatively rapid scientific progress and a large group to self-review projects that minimizes error. Hackett et al. (2008) describe “peer review on the fly” that results from collaboration and idea vetting during open science projects. Indeed, research from small teams is more error prone (García-Berthou and Alcaraz, 2004; Bakker and Wicherts, 2011; Salter et al., 2014), and work done by untrained citizen scientists yields comparable error to professional scientists (Kosmala et al., 2016). Brown and Williams (2019), for instance, completed a comprehensive evaluation of data from citizen science efforts in ecology. They concluded that well-designed projects with professional oversight generated comparable data to traditional scientific efforts. Open access to data and code also reduces error and increases reproducibility (Gorgolewski and Poldrack, 2016; Wicherts, 2016). These processes expedite scientific progress by making it easy for researchers to build on data and methods provided by previous research and/or repurpose existing data for new questions (Carpenter et al., 2009; Hampton et al., 2015; Powers and Hampton, 2019). Additionally, many journals require a formal submission to refute published findings, rather than a comments section that can promote more rapid discussion. Because of this, many errors go uncorrected or result in incremental progress from time lags in the publication process (Woelfle et al., 2011). When coupled to cultural pressures to publish quickly, traditional approaches can result in decreased scientific rigor (Bakker et al., 2012; Greenland and Fontanarosa, 2012; Nosek et al., 2012; Uhlmann et al., 2019).
Approach
While social media platforms are now widely used for sharing preprints and published papers, they remain underused at the beginning stages of innovation in which ideas are generated and developed collectively. Previous work has indicated two key features of successful crowdsourced efforts: a set starting point to drive activity and a low barrier to entry (Woelfle et al., 2011). Other key aspects of successful projects have included (1) thoughtful design, (2) a team of coordinators to guide content relevant to the research question, (3) the recruitment of individuals with specific expertise, and/or (4) an open for self-selection of participants with relevant interests (Brown and Williams, 2019; Uhlmann et al., 2019). With this in mind, this project was structured with 8-member leadership team to facilitate an open call for participants (via Twitter) and provide guidance to nearly fifty contributors with a variety of expertise. The entire team of contributors used our multidisciplinary expertise to derive a consensus statement on disturbance ecology that would be unfeasible with smaller disciplinary groups of participants. Details on our approach are below.
Project Structure and Implementation
We conceptualize the project’s structure in 5 phases: (1) starter idea and proposed project structure by a single person, (2) leadership team recruitment and refinement of project structure and goals, (3) open collaborator recruitment via social media, (4) iterative paper development, and (5) final editing, authorship assignment, and submission by the leadership team (Figure 1). The entire process encompassed ∼11 months from initial concept to first submission, with the first 2 months comprising individual or leadership team exchanges and the remaining 9 months being an open collaborative process. We have captured the entire process in a short video available with the doi: 10.6084/m9.figshare.12167952.
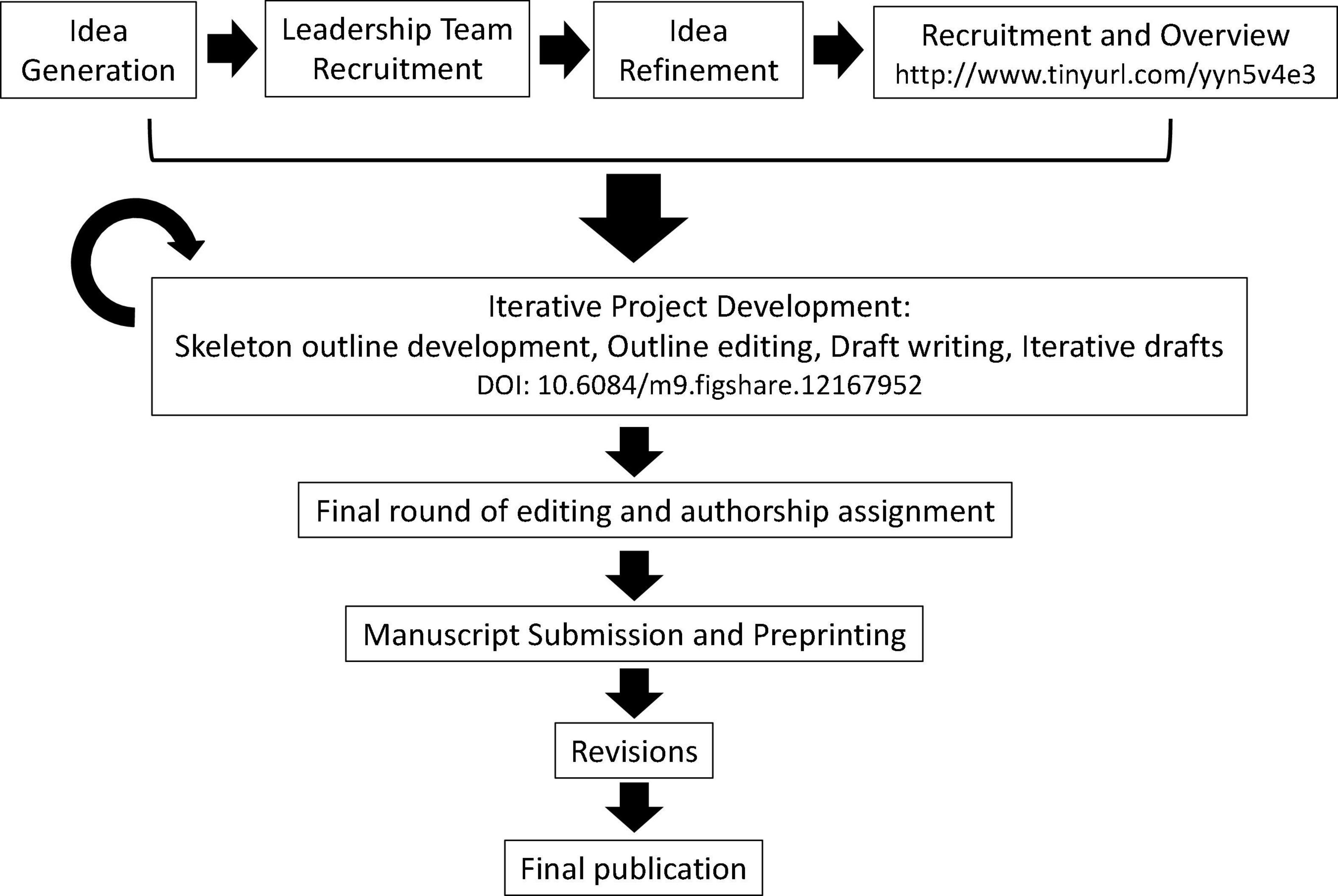
Figure 1. Project Workflow. The project featured an iterative writing process between contributors recruited with an open call on Twitter and eight leadership team members. It progressed from conception to first submission in under a year. The entire process, coordinated via Google Docs, is depicted in a workflow video available with the doi: 10.6084/m9.figshare.12167952.
The first phase began with an interest in large collaborative projects, open science approaches, and multidisciplinary questions. One member of what would become the leadership team began to brainstorm important and unanswered questions that could benefit from synthesizing perspectives across scientific disciplines and global cultures. This member selected a topic – disturbance ecology – and drafted a document describing the problem to be addressed in abstract-like form as well as guidelines for contributions and authorship and a concept of the process with a proposed timeline, with the overarching goal of generating a synthesis manuscript.
After initial idea generation, the initial member recruited other scientists to join the leadership team. This was done in a targeted fashion, whereby specific scientists spanning a variety of expertise relevant to disturbance ecology were contacted. While all members of the leadership team had some previous familiarity with the initial member, most had never worked together, and the team spanned ecological disciplines including soil science, forestry, empirical and computational modeling, ecohydrology and wetland science, and microbial ecology. This team worked together to further refine the project goals, produce an overview document to guide the process,1 and generate a skeleton outline to start the paper. A set of rules for contributions and authorship was included in the overview document (Box 1). These rules were based on existing guidelines from entities such as the International Committee of Medical Journal Editors, Nature Publishing Group, and Yale University Office of the Provost tailored to our project goals. The skeleton outline consisted of proposed sections with subtopics underneath that provided a tentative structure for paragraphs. The entire project was run through Google Docs.
BOX 1. Rules for contribution and authorship.
To inform our rules for authorship, we surveyed existing guidelines from entities such as the International Committee of Medical Journal Editors, Nature Publishing Group, and Yale University Office of the Provost. We synthesized this information into a list of five guidelines for authorship, and we set rules for contribution prior to our open call for contributors.
Rules for contribution.
(1) Anyone is welcome to contribute regardless of degree status, skillset, gender, race, etc. Contributions are self-reported using the link below and can include but are not limited to: literature review, outline development, conceptual input, data collection, data analysis, code development, and drafting and revising the manuscript.
(2) Please provide references for ideas as appropriate. Short-form references can be used in text with long-form references pasted at the end of the document.
(3) Be kind to each other. Not everyone will agree, and not everyone’s ideas will make it into the final paper. Edits will be made toward crafting a coherent story, and extraneous ideas may be shelved for side discussions.
Rules for full authorship.
(1) Must contribute to outline, writing, data collection, data analysis, and/or revisions in a manner that is critically important for intellectual content
(2) Open communication and reasonable responsiveness to leadership team
(3) Willingness to make data publicly available
(4) Agreement to be accountable for the accuracy and integrity of all aspects of the work
(5) Discretion by the leadership team on the above criteria and any other contributions
Contributors who do not meet criteria for full authorship and wish to be a co-author will be listed as part of group author on the publication.
Once an overview document and skeleton outline were solidified, paper development started in earnest via an open call for collaborators on Twitter (Figure 2A). Leadership team members tweeted a link to the overview document and a call for contributors. A stream of re-tweeting ensued leading to widespread distribution of the project. The project proceeded with an iterative contribution process in which periods of time for open contributions were followed by periods in which the leadership team edited documents during which open contributions were not accepted. This iterative process took substantial time but generated good content with editing and opportunities for re-assessment by the broader team. During time periods of open contributions, the working document was set to “comment only” to lock all contributors into suggesting mode and enable tracking of contributions. Contributions were also self-reported on a separate (linked) Google Doc that was later used for authorship assignments and notifying contributions of new stages of the project open for contribution. Throughout the process, the overview document remained posted with a note describing the status and a link to the current stage of the document at the top. The document was locked during leadership team edited and re-posted as an updated version when contributions became open again. The overview document also contained a proposed timeline that was updated as needed (with the current stage highlighted). Editing by the leadership team was a crucial part of the process as some stages of contribution generated an enormous amount of content (e.g., 25 + pages of outline), and executive decisions were necessary to craft the manuscript into a cohesive document.
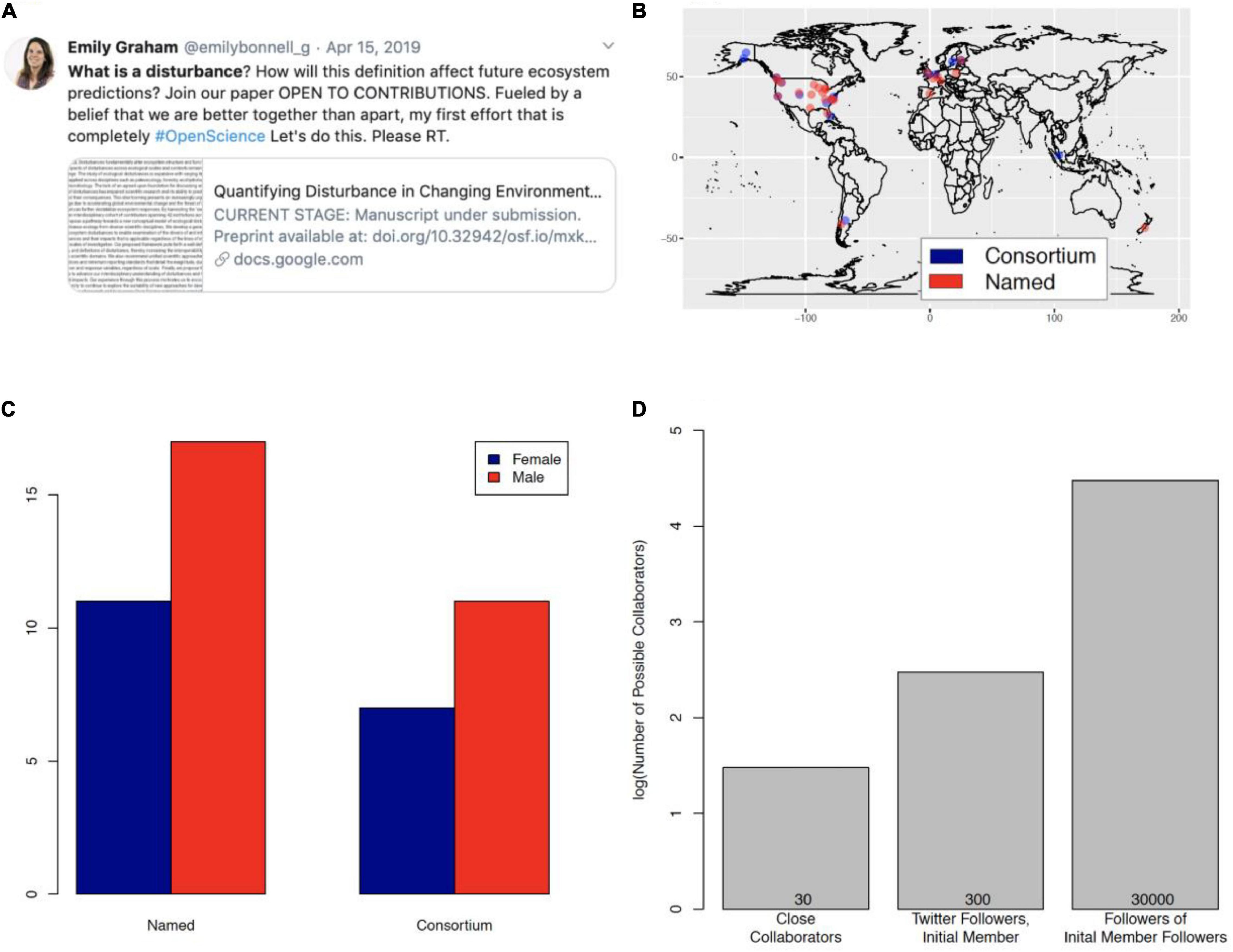
Figure 2. Contributors Recruited through Open Call. We used of Twitter to recruit a diverse cohort of contributors. (A) shows an example of our recruitment process, (B) shows the global distribution of contributors, (C) shows contributor gender distribution, and (D) shows the power of social media for extending collaborator networks.
Finally, once the manuscript took shape, a final round of editing was performed by the leadership team, and the document was released one final time for comments. At this stage (approximately one week prior to submission), authorship was assigned as “named author” meaning that the contributor’s name would be listed on the published article or “consortium authorship” meaning that a consortium author would be listed as an author on the publication with details on consortium contributors listed at the end of the manuscript (Graham et al., 2021). Contributors were notified of proposed assignments via e-mail. Decisions were based upon transparent guidelines described in the overview document at the beginning of the project according to the judgment of the leadership team. Because there were many contributors, and therefore a chance for the leadership team to overlook contributions despite good faith efforts, authors were given a chance to dispute their assignment prior to submission. In the end, we had three tiers of authorship: leadership team, named authors, and consortium authors. The leadership team handled the logistics of journal selection, submission, and pre-printing.
Contributors
A total of 46 researchers contributed to the project, 38 through our open call plus 8 leadership team members. Thirty-eight institutions were represented across the globe (Figure 2B). Among contributors, 18 were female and 28 were male, including 24 (63%) male and 14 (37%) female participants contributing through the open call (Figure 2C).
Notably, few contributors had a prior relationship to the initial member. Only 3 co-authors (of 46, 6.5%) had a prior publication with the initial member, 2 of which were part of the leadership team. This speaks to the power of open calls via social media in establishing previously unrelated groups of collaborators. For instance, a typical scientist may have somewhere on the order of 30 close collaborators in comparison to a modest 300 Twitter followers (according to a 2016 blog, the average number of Twitter follower is 7072). Because networks of twitter followers allow for exponential reach, if each of those followers has only 100 unique new followers, the scientist’s reach with one degree of separation is 30,000 potential collaborators (Figure 2D). Extrapolating outward, a single scientist’s potential collaborative network is nearly endless when generated through social media vs. traditional models.
Outcome
By assembling an interdisciplinary cohort of contributors, we addressed the lack of cross-disciplinary foundation for discussing and quantifying the complexity of disturbances. This resulted in a publication that identified an essential limitation in disturbance ecology–that the word “disturbance” is used interchangeably to refer to both the events that cause and the consequences of ecological change–and proposed a new conceptual model of ecological disturbances. We also recommended minimum reporting standards, and we proposed four future directions to advance the interdisciplinary understanding of disturbances and their social-ecological impacts. Such broad and multidisciplinary outcomes would not have been possible without the contributions of researchers from vastly different ecological perspectives.
Lessons Learned
Effective Strategies
As first noted by Woelfle et al. (2011), when faced with a scientific problem we cannot solve, most scientists would engage close colleagues in our limited professional network. The crowdsourcing approach here allowed us to navigate around this limitation by engaging an almost unlimited network of collaborators through Twitter. While we chose to use Twitter due to its concise format and widespread usage for sharing scientific works, the same approach could be used on any social media platform that has a significant number of users.
The project had many promising successes that resulted in achieving the project’s ultimate goal of a completed synthesis manuscript. Primary among these was the successful use of social media for idea generation and synthesis from an otherwise largely unconnected group of scientists. The reach of social media far extended that which we would have been able to garner by reaching out to individual colleagues or potential collaborators (Figure 2A). This enabled us to capture a broader background of literature than would otherwise be possible and yielded substantial contributions from many disciplines. At later stages, we supplemented Twitter announcements of new project stages with e-mails to contributors, as people differ in the frequency that they check social media accounts. Many emergent and exciting ideas were generated throughout the process. Importantly, having a leadership team to provide some top-down structure was crucial to this process. The multidisciplinary nature of the leadership team itself extended our reach via social media, as significant portions of our Twitter followers did not overlap. The leadership team was also critical in organizing contributions and resolving competing ideas, both of which were smoother processes than expected a priori. For example, with over 25 pages of contributed outline, top-down decisions needed to be made about content to keep for a cohesive paper, and each member of the leadership team was able to spearhead a section of the manuscript to lighten the burden on any one specific member. All residual outline content was archived and remains publicly available.
There were many specific aspects of the project we felt worked as or better than intended. Among these was version control implemented via Google Docs. Version control is an important aspect of open science that allows researchers to prevent losses in generated content, easily recall older versions, and enable contribution tracking (Ram, 2013; Hampton et al., 2015). Google Docs automatically tracks every change to a document and allows for versions to be named for easy recall. Additionally, the ease of document creation and organization via Google Docs allowed us to create new files for each stage of the manuscript (e.g., as documents were edited and re-released by the leadership team) to enable easier archiving and retrieval of information from defined steps in the project. Documents were easy to close for contributions and/or archive by simply changing the shared link between “view only” and “comment only.” Additionally, a set timeline and rules for authorship at the onset of the project were critical in providing potential contributors information to consider when deciding to participate. We attempted to keep authorship as inclusive as possible by guaranteeing all contributors at least authorship as part of a consortium author, and we did not change authorship rules after the start of the project. However, we allowed flexibility in other parts of the project to adapt to new contributions and other responsibilities of all team members. For example, while we attempted to keep to our timeline as much as possible, some deadlines were extended, either to give the leadership team more time to go through extensive contributions or to allow for more contributions through longer open time periods. We also adjusted our scope from a more data-driven synthesis paper to conceptual model based on contributions received.
Obstacles Faced and Remaining Challenges
Despite overall success of the project, we encountered several challenges that future work can build upon. While our call for contributors was completely open, we noticed a number of biases in the individuals that self-selected participation. For example, women and early career (graduate student/postdoc) contributors were notably underrepresented. While we did not track ethnicities, the distribution of contributors was heavily weighted toward the Americas and Europe. However, post-manuscript submission, we sent out an optional demographic survey to all contributors and received a 70% response rate (32 individuals). Of the 32 individuals who responded to the survey, 26 self-identified their ethnicity as Caucasian, 4 as Asian, and only 2 as Latin. Scientifically, we also had a wide distribution of specialties (e.g., community-, ecosystem-, evolutionary-, disturbance-, fire-, forest-, landscape-, microbial-, paleo-, population-, etc. ecological fields), but commonalities between leadership team members may have led to specific fields being overrepresented. For example, 34% (11 individuals) of respondents identified microbial ecology as their area of expertise. Additionally, while we had many interactive opportunities for contributors, the leadership team made editorial decisions. Though this was a necessity as not all ideas can be incorporated into a cohesive manuscript, a challenge remains: “how do we craft a synthesized story with minimal bias?” Finally, while we received a surplus of contributions for outline development, most contributors were hesitant to start actively writing during the second phase. To jumpstart the process, the leadership team decided to write starter material, often just putting outline material in full sentences broadly grouped into paragraphs. The starter material allowed for contributors to heavily edit and/or contribute small additions instead of needing to generate written material themselves and garnered much more engagement.
We also highlight a number of more specific issues for improvement. First, although Google Docs worked very well for project management, potential collaborators in certain countries were unable to participate due to embargoes against Google (e.g., China). Alternative platforms with widespread international usage should be explored in the future, ideally ones that would allow more permanent archiving of project materials than in an individual’s Google Drive. In retrospect, it also would have been useful to assign a strong hashtag to the project before initiation and to collect more metadata on contributors. A set hashtag would have allowed better tracing of the project through tweets and retweets. With an eye toward authorship assignment, we only asked contributors for their name, institution, e-mail, and summary of their contributions, all listed in a Google Doc. Providing a spreadsheet to collect optional information on home country, scientific specialty, gender/pronoun, and ethnicity would provide valuable information for evaluating the reach of our crowdsourcing efforts. Similarly, many colleagues anecdotally commented that they were following the project but not contributing, and we had no way of tracking this sort of project impact. Finally, while many journals now have flexible formats, a significant number have limitations on the numbers of authors and/or citations or other formatting requirements that are limiting.
Additional Comments
Through this process, we also garnered many pieces of anecdotal advice that may be beneficial in future efforts. Others have noted tension in open science that derives from an expectation that public facing scientific ideas be “correct” or “right,” despite failure being recognized as a necessary part of the scientific process (Merton, 1957; Hampton et al., 2015). We noticed a similar effect, particularly at the initial stages of true manuscript text development. While it certainly takes courage to put new and incompletely formed ideas into a public document, there is almost tremendous benefit in doing so, both to one’s individual career and to a group project. In a few instances, individuals e-mailed contributions instead of participating openly. In these cases, we encouraged them to contribute publicly in the spirit of open science, and only those who contributed openly were considered for authorship (other contributions were noted in the acknowledgments section). We encourage contributors to be fearless in their contributions, and not to be afraid to contribute “beta” ideas, as these can be the seed for emergent concepts. Similarly, in some cases, the existing paradigm of co-authorship persisted, whereby some participants contributed heavily while others did so more editorially, in contrast to a newer paradigm of co-creation, whereby all participants feel equally responsible for the generation of a group project. All the ideas discussed above are relatively new aspects of the scientific process and will inevitably take time to fully embrace. We encourage continued participation in open science to advance the cultural shift and diminish feels of doubt.
Finally, one established benefit of open science that cannot yet be evaluated for our project is the propensity to gain more visibility (Hitchcock, 2004). Numerous studies have demonstrated such an effect. For example, Hajjem et al. (2006) found that open access articles had at least a 36% increase in citations in a comprehensive analysis of 1.3 million articles across 10 disciplines, and Adie (2014) showed that open access articles in Nature Communications received over twice as many unique tweeters as traditional publications, work later supported by Wang et al. (2015). Similarly, when considering 7,000 NSF and NIH awards, projects that archived data produced 10 publications (median) vs. 5 for those that did not (Pienta and Lyle, 2009). Such works show a clear trend that various ways of conducting open science generally result in higher research visibility.
Conclusion and Pathways Forward
Our scientific landscape has been significantly changed by technology over the past several decades, allowing new forms of publication and collaboration that have brought with them a change in thinking toward open and interdisciplinary science. New ways of conducting science are continually emerging. Among these, the average size of authorship teams doubled from 1960 to 2005, which has been associated with greater individual successes (Valderas, 2007; Wuchty et al., 2007; Kniffin and Hanks, 2018). Other general trends include: a shift toward open access publications, increases in more open and multidisciplinary research institutes, the ability to outsource aspects of research, projects funded by multiple sources, cultural changes toward interdisciplinary thinking, and increases in patent donations (Friesike et al., 2015).
Here, we present a workflow for crowdsourced science using social media in ecology, and we encourage others to build upon and improve our efforts. We believe, as suggested by Uhlmann et al. (2019), that groups of individuals from different cultures, demographics, and research areas have the potential to improve scientific research by balancing biases toward certain perspectives (Galton, 1907; Surowiecki, 2005; Mannes et al., 2012). As such, similar crowdsourcing endeavors in ecology have the potential to create new and unique opportunity spaces for large-scale contributions. For example, many large datasets are being generated that could be used to address a variety of questions, and actively using crowdsourcing for their analysis could yield both creative research investigations and greater equality among preeminent researchers and talented scientists with less access to resources. Another application may be the distribution of proposal ideas to assemble appropriate collaborators, particularly in the case where the research is highly multidisciplinary and there is a gap in a specific expertise. Our work demonstrates that crowdsourcing via social media in the ecological sciences is a viable avenue for producing peer-reviewed scientific literature, and we are excited to see others build upon this and similar approaches in the future.
Author Contributions
EG and PS conceived of this manuscript and conducted all writing, data analysis, and editing. Both authors contributed to the article and approved the submitted version.
Funding
This research was supported by the U.S. Department of Energy (DOE), Office of Biological and Environmental Research (BER), as part of Subsurface Biogeochemical Research Program’s Scientific Focus Area (SFA) at the Pacific Northwest National Laboratory (PNNL). PNNL is operated for DOE by Battelle under contract DE-AC06-76RLO 1830. PS: This work was supported Texas A&M Agrilife and by the USDA National Institute of Food and Agriculture, Hatch project 1018999.
Conflict of Interest
The authors declare that the research was conducted in the absence of any commercial or financial relationships that could be construed as a potential conflict of interest.
Publisher’s Note
All claims expressed in this article are solely those of the authors and do not necessarily represent those of their affiliated organizations, or those of the publisher, the editors and the reviewers. Any product that may be evaluated in this article, or claim that may be made by its manufacturer, is not guaranteed or endorsed by the publisher.
Acknowledgments
We thank our fellow leadership team members C. Averill, B. Bond-Lamberty, S. Krause, J. Knelman, A. Peralta, and A. Shade for all their hard work during this project. We also thank all contributors without whom this process would not be possible.
Footnotes
- ^ http://www.tinyurl.com/yyn5v4e3
- ^ https://kickfactory.com/blog/average-twitter-followers-updated-2016/
References
Adie, E. (2014). Attention! a study of open access vs non-open access articles. Figshare. J. Contrib. doi: 10.6084/m9.figshare.1213690.v1
Bakker, M., Van Dijk, A., and Wicherts, J. M. (2012). The rules of the game called psychological science. Perspect. Psychol. Sci. 7, 543–554. doi: 10.1177/1745691612459060
Bakker, M., and Wicherts, J. M. (2011). The (mis) reporting of statistical results in psychology journals. Behav. Res. Methods 43, 666–678. doi: 10.3758/s13428-011-0089-5
Berg, J. M., Bhalla, N., Bourne, P. E., Chalfie, M., Drubin, D. G., Fraser, J. S., et al. (2016). Preprints for the life sciences. Science 352, 899–901.
Betini, G. S., Avgar, T., and Fryxell, J. M. (2017). Why are we not evaluating multiple competing hypotheses in ecology and evolution? R. Soc. Open Sci. 4:160756. doi: 10.1098/rsos.160756
Brown, E. D., and Williams, B. K. (2019). The potential for citizen science to produce reliable and useful information in ecology. Conserv. Biol. 33, 561–569. doi: 10.1111/cobi.13223
Byrnes, J. E., Baskerville, E. B., Caron, B., Neylon, C., Tenopir, C., Schildhauer, M., et al. (2014). The four pillars of scholarly publishing: the future and a foundation. Ideas Ecol. Evol. 7, 27–33.
Carpenter, S. R., Armbrust, E. V., Arzberger, P. W., Chapin Iii, F. S., Elser, J. J., Hackett, E. J., et al. (2009). Accelerate synthesis in ecology and environmental sciences. BioScience 59, 699–701.
Catlin-Groves, C. L. (2012). The citizen science landscape: from volunteers to citizen sensors and beyond. Int. J. Zool. 2012, 1–14. doi: 10.1155/2012/349630
Chatzimilioudis, G., Konstantinidis, A., Laoudias, C., and Zeinalipour-Yazti, D. (2012). Crowdsourcing with smartphones. IEEE Int. Comp. 16, 36–44.
Dickinson, J. L., Shirk, J., Bonter, D., Bonney, R., Crain, R. L., Martin, J., et al. (2012). The current state of citizen science as a tool for ecological research and public engagement. Front. Ecol. Environ. 10:291–297. doi: 10.1890/110236
Dickinson, J. L., Zuckerberg, B., and Bonter, D. N. (2010). Citizen science as an ecological research tool: challenges and benefits. Ann. Rev. Ecol. Evol. Systemat. 41, 149–172. doi: 10.1146/annurev-ecolsys-102209-144636
English, P., Richardson, M., and Garzón-Galvis, C. (2018). From crowdsourcing to extreme citizen science: participatory research for environmental health. Annu. Rev. Public Health 39, 335–350. doi: 10.1146/annurev-publhealth-040617-013702
Estellés-Arolas, E., and González-Ladrón-de-Guevara, F. (2012). Towards an integrated crowdsourcing definition. J. Inform. Sci. 38, 189–200. doi: 10.1016/j.earlhumdev.2020.105191
Evans, C., Abrams, E., Reitsma, R., Roux, K., Salmonsen, L., and Marra, P. P. (2005). The neighborhood nestwatch program: participant outcomes of a citizen-science ecological research project. Conserv. Biol. 19, 589–594. doi: 10.1111/j.1523-1739.2005.00s01.x
Fecher, B., and Friesike, S. (2014). “Open science: one term, five schools of thought,” in Opening Science, eds S. Bartling and S. Friesike (Cham: Springer).
Fink, D., Damoulas, T., Bruns, N. E., La Sorte, F. A., Hochachka, W. M., Gomes, C. P., et al. (2014). Crowdsourcing meets ecology: hemisphere-wide spatiotemporal species distribution models. AI Magazine 35, 19–30. doi: 10.1609/aimag.v35i2.2533
Fraser, H., Barnett, A., Parker, T. H., and Fidler, F. (2020). The role of replication studies in ecology. Ecol. Evol. 10, 5197–5207.
Friesike, S., Widenmayer, B., Gassmann, O., and Schildhauer, T. (2015). Opening science: towards an agenda of open science in academia and industry. J. Technol. Transfer 40, 581–601. doi: 10.1007/s10961-014-9375-6
Gallagher, R., Falster, D., Maitner, B., Salguero-Gomez, R., Vandvik, V., Pearse, W., et al. (2019). The open traits network: using open science principles to accelerate trait-based science across the tree of life. Nat. Ecol. Evol. 4, 294–303. doi: 10.1038/s41559-020-1109-6
García-Berthou, E., and Alcaraz, C. (2004). Incongruence between test statistics and P values in medical papers. BMC Med. Res. Methodol. 4:13. doi: 10.1186/1471-2288-4-13
Gelman, A., and Loken, E. (2014). The statistical crisis in science: data-dependent analysis–a” garden of forking paths”–explains why many statistically significant comparisons don’t hold up. Am. Sci. 102, 460–466.
Gorgolewski, K. J., and Poldrack, R. A. (2016). A practical guide for improving transparency and reproducibility in neuroimaging research. PLoS Biol. 14:e1002506. doi: 10.1371/journal.pbio.1002506
Graham, E., and Krause, S. (2020). Social media sows consensus in disturbance ecology. Nature 577:170. doi: 10.1038/d41586-020-00006-7
Graham, E. B., Averill, C., Bond-Lamberty, B., Knelman, J. E., Krause, S., Peralta, A. L., et al. (2021). Toward a generalizable framework of disturbance ecology through crowdsourced science. Front. Ecol. Evol. 9:588940. doi: 10.3389/fevo.2021.588940
Greenland, P., and Fontanarosa, P. B. (2012). Ending honorary authorship. Washington, DC: American Association for the Advancement of Science.
Hackett, E. J., Parker, J. N., Conz, D., Rhoten, D., and Parker, A. (2008). “Ecology transformed: NCEAS and changing patterns of ecological research,” in Scientific Collaboration on the Internet, eds G. M. Olson, A. Zimmerman, and N. Bos (Cambridge, MA: MIT Press).
Hajjem, C., Harnad, S., and Gingras, Y. (2006). Ten-year cross-disciplinary comparison of the growth of open access and how it increases research citation impact. arXiv [Preprint]. Available online at: https://arxiv.org/abs/cs/0606079 (accessed April 28, 2021).
Hampton, S. E., Anderson, S. S., Bagby, S. C., Gries, C., Han, X., Hart, E. M., et al. (2015). The Tao of open science for ecology. Ecosphere 6, 1–13.
Henrich, J., Heine, S. J., and Norenzayan, A. (2010). The weirdest people in the world? Behav. Brain Sci. 33, 61–83.
Heres, B., Crowley, C., Barry, S., and Brockmann, H. (2021). Using citizen science to track population trends in the american horseshoe crab (Limulus polyphemus) in Florida. Citizen Sci. Theory Practice 6:19. doi: 10.5334/cstp.385
Hitchcock, S. (2004). The Effect of Open Access and Downloads (‘hits’) on Citation Impact: a Bibliography of Studies. Southampton, GB: University of Southampton.
Hsing, P. Y., Bradley, S., Kent, V. T., Hill, R. A., Smith, G. C., Whittingham, M. J., et al. (2018). Economical crowdsourcing for camera trap image classification. Remote Sens. Ecol. Conserv. 4, 361–374. doi: 10.1002/rse2.84
Hunt, V. M., Fant, J. B., Steger, L., Hartzog, P. E., Lonsdorf, E. V., Jacobi, S. K., et al. (2017). PhragNet: crowdsourcing to investigate ecology and management of invasive Phragmites australis (common reed) in North America. Wetlands Ecol. Manag. 25, 607–618. doi: 10.1007/s11273-017-9539-x
Johansson, M. A., Reich, N. G., Meyers, L. A., and Lipsitch, M. (2018). Preprints: an underutilized mechanism to accelerate outbreak science. PLoS Med. 15:e1002549. doi: 10.1371/journal.pmed.1002549
Judd, C. M., Westfall, J., and Kenny, D. A. (2012). Treating stimuli as a random factor in social psychology: a new and comprehensive solution to a pervasive but largely ignored problem. J. Pers. Soc. Psychol. 103:54. doi: 10.1037/a0028347
Kniffin, K. M., and Hanks, A. S. (2018). The trade-offs of teamwork among STEM doctoral graduates. Am. Psychol. 73:420. doi: 10.1037/amp0000288
Kobori, H., Dickinson, J. L., Washitani, I., Sakurai, R., Amano, T., Komatsu, N., et al. (2016). Citizen science: a new approach to advance ecology, education, and conservation. Ecol. Res. 31, 1–19. doi: 10.1007/s11284-015-1314-y
Kosmala, M., Wiggins, A., Swanson, A., and Simmons, B. (2016). Assessing data quality in citizen science. Front. Ecol. Environ. 14:551–560. doi: 10.1002/fee.1436
Lemoine, N. P., Hoffman, A., Felton, A. J., Baur, L., Chaves, F., Gray, J., et al. (2016). Underappreciated problems of low replication in ecological field studies. Ecology 97, 2554–2561. doi: 10.1002/ecy.1506
Makel, M. C., Plucker, J. A., and Hegarty, B. (2012). Replications in psychology research: how often do they really occur? Perspect. Psychol. Sci. 7, 537–542. doi: 10.1177/1745691612460688
Mannes, A. E., Larrick, R. P., and Soll, J. B. (2012). “The social psychology of the wisdom of crowds,” in Social Judgment and Decision Making, ed. J. I. Krueger (Hove: Psychology Press), 227–242.
Marshall, N. J., Kleine, D. A., and Dean, A. J. (2012). CoralWatch: education, monitoring, and sustainability through citizen science. Front. Ecol. Environ. 10:332–334. doi: 10.1890/110266
McDuffie, L. A., Hagelin, J. C., Snively, M. L., Pendleton, G. W., and Taylor, A. R. (2019). Citizen science observations reveal long-term population trends of common and Pacific Loon in urbanized Alaska. J. Fish Wildlife Manag. 10, 148–162. doi: 10.3996/082018-naf-002
McKiernan, E. C., Bourne, P. E., Brown, C. T., Buck, S., Kenall, A., Lin, J., et al. (2016). Point of view: how open science helps researchers succeed. eLife 5:e16800. doi: 10.7554/eLife.16800
Merton, R. K. (1957). Priorities in scientific discovery: a chapter in the sociology of science. Am. Soc. Rev. 22, 635–659.
Miguel, E., Camerer, C., Casey, K., Cohen, J., Esterling, K. M., Gerber, A., et al. (2014). Promoting transparency in social science research. Science 343, 30–31.
Mueller-Langer, F., Fecher, B., Harhoff, D., and Wagner, G. G. (2019). Replication studies in economics—how many and which papers are chosen for replication, and why? Res. Pol. 48, 62–83. doi: 10.1016/j.respol.2018.07.019
Muller, C., Chapman, L., Johnston, S., Kidd, C., Illingworth, S., Foody, G., et al. (2015). Crowdsourcing for climate and atmospheric sciences: current status and future potential. Int. J. Climatol. 35, 3185–3203. doi: 10.1002/joc.4210
Newman, G., Wiggins, A., Crall, A., Graham, E., Newman, S., and Crowston, K. (2012). The future of citizen science: emerging technologies and shifting paradigms. Front. Ecol. Environ. 10:298–304. doi: 10.2307/41811393
Nielsen, M. (2011). An informal definition of OpenScience. OpenScience Project 28. Available online at: https://openscience.org/an-informal-definitionof-openscience/ (accessed April 28, 2020).
Nosek, B. A., Alter, G., Banks, G. C., Borsboom, D., Bowman, S. D., Breckler, S. J., et al. (2015). Promoting an open research culture. Science 348, 1422–1425.
Nosek, B. A., Spies, J. R., and Motyl, M. (2012). Scientific utopia: II. restructuring incentives and practices to promote truth over publishability. Perspect. Psychol. Sci. 7, 615–631. doi: 10.1177/1745691612459058
O’Boyle, E. H. Jr., Banks, G. C., and Gonzalez-Mulé, E. (2017). The chrysalis effect: how ugly initial results metamorphosize into beautiful articles. J. Manag. 43, 376–399. doi: 10.1177/0149206314527133
Osawa, T., Yamanaka, T., Nakatani, Y., Nishihiro, J., Takahashi, S., Mahoro, S., et al. (2017). A crowdsourcing approach to collecting photo-based insect and plant observation records. Biodiversity Data J. 6:e21271. doi: 10.3897/BDJ.5.e21271
Pedregosa, F., Varoquaux, G., Gramfort, A., Michel, V., Thirion, B., Grisel, O., et al. (2011). Scikit-learn: machine learning in Python. J. Machine Learn. Res. 12, 2825–2830.
Petersen, A. M., Jung, W.-S., Yang, J.-S., and Stanley, H. E. (2011). Quantitative and empirical demonstration of the Matthew effect in a study of career longevity. Proc. Natl. Acad. Sci. U S A. 108, 18–23. doi: 10.1073/pnas.1016733108
Pienta, A. M., and Lyle, J. (2009). Data Sharing in the Social Sciences, 2009 [United States] Public Use Data. ICPSR29941-v1. Ann Arbor, MI: Inter-university Consortium for Political and Social Research [distributor]. doi: 10.3886/ICPSR29941.v1
Pocock, M. J., Tweddle, J. C., Savage, J., Robinson, L. D., and Roy, H. E. (2017). The diversity and evolution of ecological and environmental citizen science. PLoS One 12:e0172579. doi: 10.1371/journal.pone.0172579
Poisson, A. C., Mccullough, I. M., Cheruvelil, K. S., Elliott, K. C., Latimore, J. A., and Soranno, P. A. (2020). Quantifying the contribution of citizen science to broad-scale ecological databases. Front. Ecol. Environ. 18:19–26. doi: 10.1002/fee.2128
Powers, S. M., and Hampton, S. E. (2019). Open science, reproducibility, and transparency in ecology. Ecol. Appl. 29:e01822.
Ram, K. (2013). Git can facilitate greater reproducibility and increased transparency in science. Source Code Biol. Med. 8:7. doi: 10.1186/1751-0473-8-7
Raymond, E. (1999). The cathedral and the bazaar. Know Techn. Pol. 12, 23–49. doi: 10.1007/s12130-999-1026-0
Salter, S. J., Cox, M. J., Turek, E. M., Calus, S. T., Cookson, W. O., Moffatt, M. F., et al. (2014). Reagent and laboratory contamination can critically impact sequence-based microbiome analyses. BMC Biol. 12:87. doi: 10.1186/s12915-014-0087-z
Silberzahn, R., Uhlmann, E. L., Martin, D. P., Anselmi, P., Aust, F., Awtrey, E., et al. (2018). Many analysts, one data set: making transparent how variations in analytic choices affect results. Adv. Methods Pract. Psychol. Sci. 1, 337–356.
Simmons, J. P., Nelson, L. D., and Simonsohn, U. (2011). False-positive psychology: undisclosed flexibility in data collection and analysis allows presenting anything as significant. Psychol. Sci. 22, 1359–1366. doi: 10.1177/0956797611417632
Simons, D. J. (2014). The value of direct replication. Perspect. Psychol. Sci. 9, 76–80. doi: 10.1177/1745691613514755
Stewart, N., Chandler, J., and Paolacci, G. (2017). Crowdsourcing samples in cognitive science. Trends Cogn. Sci. 21, 736–748. doi: 10.1016/j.tics.2017.06.007
Sullivan, B. L., Aycrigg, J. L., Barry, J. H., Bonney, R. E., Bruns, N., Cooper, C. B., et al. (2014). The eBird enterprise: an integrated approach to development and application of citizen science. Biol. Conserv. 169, 31–40. doi: 10.1016/j.biocon.2013.11.003
Swanson, A., Kosmala, M., Lintott, C., and Packer, C. (2016). A generalized approach for producing, quantifying, and validating citizen science data from wildlife images. Conserv. Biol. 30, 520–531. doi: 10.1111/cobi.12695
Tang, V., Rösler, B., Nelson, J., Thompson, J., Van Der Lee, S., Chao, K., et al. (2020). Citizen scientists help detect and classify dynamically triggered seismic activity in alaska. Front. Earth Sci. 8:321. doi: 10.3389/feart.2020.00321
Uhlmann, E. L., Ebersole, C. R., Chartier, C. R., Errington, T. M., Kidwell, M. C., Lai, C. K., et al. (2019). Scientific utopia III: crowdsourcing science. Perspect. Psychol. Sci. 14, 711–733. doi: 10.1177/1745691619850561
Valderas, J. M. (2007). Why do team-authored papers get cited more? Science 317, 1496–1498. doi: 10.1126/science.317.5844.1496b
Ver Hoef, J. M., Johnson, D., Angliss, R., and Higham, M. (2021). Species density models from opportunistic citizen science data. Methods Ecol. Evol. 121, 1911–1925.
Vicente-Sáez, R., and Martínez-Fuentes, C. (2018). Open Science now: a systematic literature review for an integrated definition. J. Bus. Res. 88, 428–436. doi: 10.1016/j.jebdp.2018.05.001
Wagner, C. S. (2009). The New Invisible College: Science for Development. Washington, D.C: Brookings Institution Press.
Wahls, W. P. (2018). High cost of bias: diminishing marginal returns on NIH grant funding to institutions. BioRxiv [preprint] doi: 10.1101/367847
Wang, X., Liu, C., Mao, W., and Fang, Z. (2015). The open access advantage considering citation, article usage and social media attention. Scientometrics 103, 555–564. doi: 10.1007/s11192-015-1547-0
Wells, G. L., and Windschitl, P. D. (1999). Stimulus sampling and social psychological experimentation. Personal. Soc. Psychol. Bull. 25, 1115–1125. doi: 10.1177/01461672992512005
Wicherts, J. M. (2016). Peer review quality and transparency of the peer-review process in open access and subscription journals. PLoS One 11:e0147913. doi: 10.1371/journal.pone.0147913
Woelfle, M., Olliaro, P., and Todd, M. H. (2011). Open science is a research accelerator. Nat. Chem. 3, 745–748. doi: 10.1038/nchem.1149
Keywords: FAIR, ICON, disturbance, open science, Twitter, open innovation (OI)
Citation: Graham EB and Smith AP (2021) Crowdsourcing Global Perspectives in Ecology Using Social Media. Front. Ecol. Evol. 9:588894. doi: 10.3389/fevo.2021.588894
Received: 29 July 2020; Accepted: 13 October 2021;
Published: 11 November 2021.
Edited by:
David Jack Coates, Department of Biodiversity, Conservation and Attractions (DBCA), AustraliaReviewed by:
Gregor Kalinkat, Leibniz-Institute of Freshwater Ecology and Inland Fisheries (IGB), GermanyJen Martin, The University of Melbourne, Australia
Chantelle Doyle, University of New South Wales, Australia, in collaboration with reviewer JM
Copyright © 2021 Graham and Smith. This is an open-access article distributed under the terms of the Creative Commons Attribution License (CC BY). The use, distribution or reproduction in other forums is permitted, provided the original author(s) and the copyright owner(s) are credited and that the original publication in this journal is cited, in accordance with accepted academic practice. No use, distribution or reproduction is permitted which does not comply with these terms.
*Correspondence: Emily B. Graham, ZW1pbHkuZ3JhaGFtQHBubmwuZ292