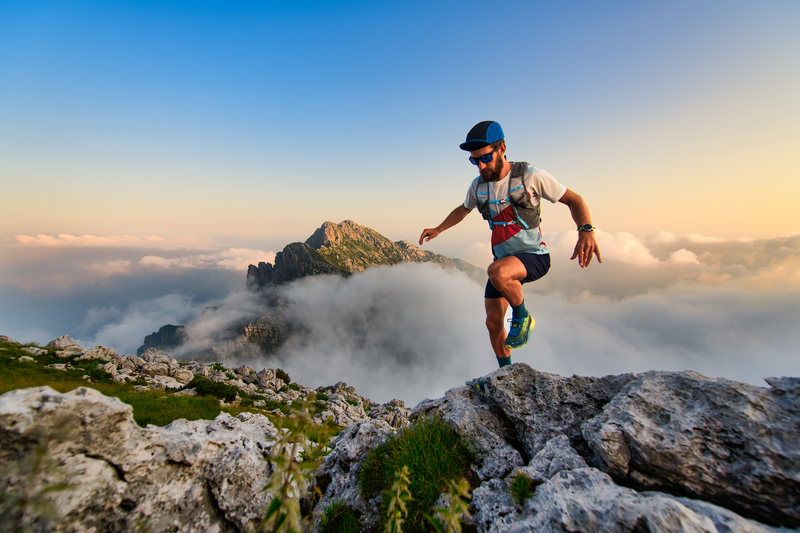
94% of researchers rate our articles as excellent or good
Learn more about the work of our research integrity team to safeguard the quality of each article we publish.
Find out more
PERSPECTIVE article
Front. Ecol. Evol. , 02 February 2021
Sec. Evolutionary Ecology of Social Behaviour
Volume 9 - 2021 | https://doi.org/10.3389/fevo.2021.581222
How individuals in a group lead to collective behavior is a fundamental question across biological systems, from cellular systems, to animal groups, to human organizations. Recent technological advancements have enabled an unprecedented increase in our ability to collect, quantify, and analyze how individual responses lead to group behavior. However, despite a wealth of data demonstrating that collective behavior exists across biological scales, it is difficult to make general statements that apply in different systems. In this perspective, we present a cohesive framework for comparing groups across different levels of biological organization, using an intermediate link of “collective mechanisms” that connects individual responses to group behavior. Using this approach we demonstrate that an effective way of comparing different groups is with an analysis hierarchy that asks complementary questions, including how individuals in a group implement various collective mechanisms, and how these various mechanisms are used to achieve group function. We apply this framework to compare two collective systems—cellular systems and honey bee colonies. Using a case study of a response to a disturbance, we compare and contrast collective mechanisms used in each system. We then discuss how inherent differences in group structure and physical constraints lead to different combinations of collective mechanisms to solve a particular problem. Together, we demonstrate how a hierarchical approach can be used to compare and contrast different systems, lead to new hypotheses in each system, and form a basis for common research questions in collective behavior.
Collective behavior spans across levels of biological organization, from cellular systems, to multi-cellular organisms, to animal and human societies. Even though such systems are wildly different, the fundamental challenges that they face can be strikingly similar: maintaining homeostasis, allocating resources, and coordinating group responses. Depending upon the available resources and the constraints on a system, the processes and solutions for a given challenge can be similar in some cases, but different in others. For example, similar effective forces of repulsion, alignment, and attraction describe basic motion characteristics in cell colonies (Bi et al., 2016; Camley and Rappel, 2017), schooling fish (Couzin et al., 2002), human pedestrians (Helbing and Molnár, 1995), and social insects (Janson et al., 2005; Diwold et al., 2011). Conversely, while the coordinated response of an insect colony is due to distributed processing, the coordinated response of the organ systems within a single insect comes from centralized control of the central nervous system. How, then, do different types of collectives deal with similar problems?
Although biological systems have many parameters governing behavior, all of the finer-scale details may not matter at a larger scale; because of this property, simplified models are a useful tool for understanding general system function (Machta et al., 2013; Transtrum et al., 2015). A hierarchical approach asks questions at different levels of organization, and then further asks how these levels connect. A common example of such an approach is Marr's three analysis levels of implementation, algorithm, and computation (Marr, 1982; Krakauer et al., 2017). Building on this, Carandini (2012) discusses the need for an analysis hierarchy by demonstrating the limitations of seeking connections between individual neurons and overall behavior without considering computational algorithms; using the analogy of brain as computer, this is likened to seeking correlations between individual transistors and computer function without considering the operating system. Recently, Hein et al. (2020) adopted Marr's approach to understand pursuit and evasion behavior in animal groups and demonstrated how separating analysis levels highlights the key details that enable a correspondence between experiment and theory. To fully understand a biological system, Tinbergen's four questions describe how both proximate and ultimate mechanisms need to be addressed (Tinbergen, 1963; Taborsky, 2014). By looking at different systems, we see that constraints and selection pressures may lead individuals of different systems to use different approaches to solve the same problem (Gordon, 2016), and that certain algorithms or mechanisms appear repeatedly even though systems may differ widely (Adams et al., 2012).
In this perspective we present a cohesive analysis framework with question levels that can be applied to collective behavior. This framework uses the term “collective mechanisms” to describe the intermediate link between individuals and overall group behavior (Figure 1). To analyze a collective system, we summarize this approach by asking the following questions:
1. Group description: Who is included in the group, and what is the group structure?
2. Implementation: How is individual behavior used to implement a certain collective mechanism?
3. Algorithm: How do various collective mechanisms contribute to overall group function?
4. Adaptation: How are behavioral algorithms and group function adapted to the surrounding environment?
Figure 1. Collective mechanisms link individual to group function. Different groups have different structures, and may use a different set of mechanisms to perform similar functions. The way mechanisms are implemented depends on the constraints and structure of the individuals in a particular group, and thus some groups may be more effective than others at implementing certain mechanisms. Differences between groups are illustrated by the connections and the transparency of the lines linking groups to each collective mechanism. To compare collective behavior in different systems, we use complementary questions: how groups are structured (Q1), how individual behavior is used to implement certain collective mechanisms (Q2), how multiple collective mechanisms contribute to group function (Q3), and how both individual and group behavioral algorithms are adapted to the particular environment (Q4).
To make these questions precise, we must first define what we mean by mechanism and function. We refer to a “collective mechanism” as any process that links individual behavior to group function (Table 1). Building on a previous framework (Craver, 2007; Glennan, 2017), we specify that a behavioral algorithm consists of individuals using various collective mechanisms to enact a particular group function. With “group function,” we refer to the mechanistic description of what the group does, not the evolutionary explanation of why a particular task is performed by the group (Craver, 2007; Keeling et al., 2019). Note that collective systems vary in how much the individual vs. the group may be adapted to a particular function in the environment (Figure 1); this depends on whether selection occurs at the level of individuals or at the level of groups.
In this article we apply this framework with a focus on comparing two systems—groups of cells and honey bee colonies—which exist at different scales, but are similar in how individuals contribute to group function (Yang, 2007). In forming the comparison, we follow the four questions listed above and shown in Figure 1. We first describe the group and an associated division into functional subgroups. Then, using a case-study (response to a perturbation), we compare how various collective mechanisms contribute to the group's response, and how function is tied to environmental characteristics. While we focus on cellular systems and honey bees, in each part we also discuss similarities and differences with vertebrate animal groups and human organizations.
A group is made up of multiple individuals, with inclusions defined by genetic, reproductive, spatial, or functional factors. Both cellular systems and social insects have further levels of organization, where task- or function-specific subgroups coordinate activity for the maintenance and survival of the group as a whole (Figures 2A–C).
Figure 2. Cellular systems and honey bee colonies. (A) A group is made up of multiple individuals, with inclusions defined by genetic, reproductive, spatial, or functional factors. Within a group, divisions into subgroups can represent individuals that perform specific functions. (B) Scales of organization within an organ. Cells of a certain type form subgroups, each of which performs specific functions. These tissues combine to form an organ. (C) Nest structure illustrates functional subgroups in a honey bee colony. This nest has been organized into areas designated for brood care, honey storage, rearing male reproductives, and a dance floor. Workers organize themselves according to these nest areas, with young bees caring for brood and old bees advertising foraging sites on the dance floor. (D,E) Response to perturbation case study, where the group must restore homeostasis. (D) After being cut, epithelial cells quickly respond with directed motion to heal the wound. Leader cells are larger and more polarized than the other cells, and they guide the cells to move in the direction of the wound. (E) A honey bee colony responds to heat stress. Before the heat stress (left), few bees have evacuated the nest, but soon after, hundreds of bees will exit the nest (center). Some workers continue fanning at the entrance (right, top), whereas others simply evacuate (right, bottom).
Cellular systems have a multi-level organization: Cells of a certain type group together to form specific tissues, tissues performs distinct tasks relevant to the function of the organ or organ system, and together these systems make up the organism (Saxén and Lehtonen, 1986). For instance, connective tissue provides cohesion and internal support to the organs, muscle tissue enables movement, nervous tissue regulates and controls bodily functions, and epithelial tissue acts as a barrier to prevent invasions of pathogens as well as helping to absorb nutrients from food. Multiple tissue types can be found within a single organ (Figure 2B). Cell type differentiation is settled during morphogenesis, which is the beginning of shape and pattern formation during development. Because of the multi-level organization, the specification of who is included in a “group” depends on the group function that is being analyzed. For the purpose of understanding a particular function, we include individuals that contribute to that function. For a complex task such as reproduction, this includes multiple different organ systems or even the entire organism. Considering epithelial cells and the specific task of wound healing (Figure 2D) the group includes only the subset of cells involved in healing the wound, most of which belong to a single tissue type.
Insect societies are champions of individual specialization (Michener, 1969; Wilson, 1971). As “superorganisms” (Wheeler, 1928; Hölldobler and Wilson, 2009), colonies display physiological features that are typically attributed to organisms, such as gas exchange, nest homeostasis, and nutritional targets (Kleineidam et al., 2001; Dussutour and Simpson, 2009; Ostwald et al., 2016). A honey bee colony is made up of thousands of workers and a single queen (Smith et al., 2016). The workers can be divided into functional subgroups of those caring for brood, processing honey, or foraging (Figure 2C). Unlike other social insect systems, where a worker's tasks can be distinguished by their physical appearance (e.g., workers vs. soldiers in the army ant Eciton burchellii), workers in a honey bee colony organize tasks using a system of temporal polyethism. Generally, young bees care for brood, middle-aged bees work in the nest, and old bees forage outside (Seeley, 1982). A worker may also partition its time between several tasks, which together describe its “task repertoire” (Lindauer, 1952; Seeley, 1982). Changes between tasks are not purely age-driven; they are also mediated by interactions with other individuals, such that task allocation responds to the needs of the colony (Beshers et al., 2001; Johnson, 2010). In addition to age, definitions of subgroups may include interaction networks (Wild et al., 2020) or task-specific spatial localization (Mersch et al., 2013; Modlmeier et al., 2019).
Although in both cellular systems and honey bee colonies there is a clear division into functional subgroups (Figures 2A–C), there are differences in how individual function changes over time, how the “group” is defined for the purpose of analyzing a particular function, and in the overall structure of the organizational levels. In cellular systems, functional groupings are settled during morphogenesis, while the functional subgroup of a worker bee is flexible, depending on changes with age, glandular development, hormone titers such as juvenile hormone, colony requirements, and social interactions (Seeley, 1982; Huang and Robinson, 1992, 1996; Sullivan et al., 2000; Beshers et al., 2001; Grozinger et al., 2003; Wild et al., 2020). Note that here we refer to subgroups among workers, not the reproductive castes that distinguish workers, drones, and the queen (Winston, 1991). The definition of group depends on the function in cellular systems, which can range from the whole organism for complex tasks to only localized cells of a particular tissue for specific functions. For honey bees, the group includes the whole colony for most functions. In addition to these factors, within the multi-level organization of cellular systems the overall activity of multiple organ systems is often coordinated by a central nervous system (CNS). Thus, even at the lower level of individual cells, there may be some degree of centralized control from the CNS. In contrast, there are no centralized control structures in social insects.
In fission-fusion groups of fish, birds, or ungulates, the overall group function, such as migration, foraging, or avoiding predation, should be considered when defining and describing the group. In these cases there is no division into functional subgroups, but other structural elements may be used to describe the group. For example, faster fish tend to be near the front of the school and thus act as leaders (Jolles et al., 2017); “leader” vs. “follower” birds differ in their flapping ability and position in the group (Flack et al., 2018).
For other vertebrate groups that display a multi-level structure including up to hundreds of individuals (Schreier and Swedell, 2009; Papageorgiou et al., 2019), it can be useful to define the “group” in terms of specific functions. For example, baboons troops of up to approximately 50 individuals forage together, and thus can be considered a group with respect to the function of locating food resources (Strandburg-Peshkin et al., 2015). Many of these groups show consistent membership which is often defined by a dominance hierarchy (Noble, 1939; Schreier and Swedell, 2009; Maruska and Fernald, 2013).
Clear functional subgroups can also be observed in human organizations such as businesses, universities, or government entities. For example, a business may have functional divisions of marketing, research and development, manufacturing, finance, and human resources. While traditional organizational structures are hierarchical, some modern companies instead use a flat or “holocratic” structure that emphasizes distributed decision-making but retains functional distinctions among working groups (Robertson, 2015). While decision making in groups of honey bees and cellular societies follow entirely different processes, the structure seems to be similar to a holocracy, with functional distinctions yet distributed decision-making. In animal groups with hierarchical dominance structures, decision-making structures may not follow the same hierarchies, as demonstrated in the case of baboon movement decisions which can be initiated by any group member (Strandburg-Peshkin et al., 2015).
Overall, key differences in group structure in all of these different systems can be summarized by the degree of differentiation among group members (or lack thereof), whether individuals take on a consistent role or change over time, and the connectivity or communication structure among individuals in the group with respect to achieving a certain function.
Collective mechanisms act as building blocks that link individual behavior to group function. These building blocks are used and adapted by a given system in order to solve a variety of problems. Some collective mechanisms used in both cellular systems and honey bees include communication, individual specialization, distributed processing (or, conversely, “individual processing”), within-group competition, activation, inhibition, and feedback (Figure 1, Table 1). Note that this is not an exhaustive list; other mechanisms may be defined and used to describe collective behavior of other systems, or to form a comparison between systems. For example, Sumpter (2006) uses the terminology of “collective principles” to refer to the same concept, describing mechanisms of integrity/variability, positive feedback, negative feedback, response thresholds, leadership, inhibition, redundancy, synchronization, and selfishness; further mechanisms could include group-level selective attention, short-term memory/hysteresis, and stigmergy (Couzin, 2009). We note that to compare systems, it is important that collective mechanisms describe a process that links individual to group behavior in a way that does not depend on precise implementation details. While both cellular systems and honey bees systems can implement all of the collective mechanisms in Table 1, differences in the nature of the individuals means that the effectiveness of a particular collective mechanism may not be the same in each system. We use a case study to compare and contrast collective mechanisms that contribute to how a group responds to a particular perturbation.
As the outermost tissue layer, epithelial tissue needs to be able to respond quickly to changes in the surrounding environment. At homeostasis, cells of epithelial tissues are typically jammed and any activity within the epithelia stem mainly from cell maintenance, i.e., the continuous replenishment of aged/damaged cells with healthy individuals (Macara et al., 2014). When homeostasis is perturbed by a wound trauma, cells must move quickly to close the wound in order to protect the underlying organ. Studies have demonstrated that wound healing relies on coordination between cells in order to migrate efficiently toward the exposed area (Poujade et al., 2007; Park et al., 2017). The collective sensing and response during wound healing includes a complex interplay of chemical and physical signals between individuals and with the extra-cellular environment (Ladoux and Mège, 2017). Cells at the wound edge sense a chemical change in their environment due the mitogens released by wounded cells and due to the cell-free region created by the wound (Ganapathy et al., 2012). In addition, some edge cells specialize into “leader cells,” which have a strong polarization in the direction of the wound (Omelchenko et al., 2003). Leader cells mediate the coordinated motion of the group by using cell-cell contacts to transfer mechanical forces to follower cells (Figure 2D; Vishwakarma et al., 2018).
Honey bee colonies respond to environmental conditions to maintain nest homeostasis. The brood nest, for example, is carefully regulated between 33 and 35°C; temperatures outside this range are potentially lethal for the developing brood (Lindauer, 1954; Becher et al., 2009). When ambient temperatures rise, honey bee colonies have a series of graded responses to thermoregulate. Workers begin by fanning their wings to increase air circulation within the nest, and foragers switch from collecting nectar to water. These water deliveries are passed to younger receiver bees, who spread the water throughout the nest for evaporative cooling. If the nest temperature continues to rise, hundreds to thousands of workers will evacuate the nest, thereby reducing the number of heat-generating individuals in the nest, and providing additional space for air to circulate (Figure 2E; Lindauer, 1954; Robinson et al., 1984; Kühnholz and Seeley, 1997; Cook and Breed, 2013; Ostwald et al., 2016). As long as honey bees have access to water, workers can maintain broodnest homeostasis, even when faced with ambient temperatures as high as 60°C (Lindauer, 1954). When colonies are subjected to repeated days of heat stress, workers will even begin to store water in their stomachs, and in the honeycomb, to use overnight when foragers cannot fly to collect water (Ostwald et al., 2016).
In both cases, the group must respond to a perturbation—a wound or a temperature change—in order to maintain and restore overall function. How do these different systems solve a similar problem? Both rely on specialists taking on specific roles as part of the group-level response: Leader cells specialize by coordinating the movement to close the wound, and water collector bees specialize on water to initiate evaporative cooling in the nest. The systems, however, differ in their use of individual processing (behavior determined by intrinsic properties) vs. distributed processing (behavior determined by interactions with other individuals, see Table 1). Distributed processing is dominant in the case of cellular wound healing; interactions mediate the switch to leader cells (Vishwakarma et al., 2018). A bee colony's response to heat stress is a mix of individual and distributed processing. A distributed algorithm determines the number of workers collecting vs. spreading water throughout the nest, while individual processing describes how individual workers have different heat thresholds to initiate fanning. In the case of wound healing, we do not know if such individual processing plays a role, e.g., if differences between individual cells at the wound site could be a factor in determining the selection of leader cells. While honey bees adapt their behavior in the case of repeated days of heat stress, there is no evidence that epithelial cells adapt their behavior to facilitate healing after multiple wounds.
How does this compare to the perturbation response of vertebrate animal groups? One example is the response of a school of fish to a predator. Fish release a chemical called “Schreckstoff” that conveys danger to others. When exposed to this chemical, group members will come closer to one another and make the overall size of the group smaller (Sosna et al., 2019). In this tightened configuration, the group is able to spread information more quickly and respond to future threats (Rosenthal et al., 2015). Interestingly, experiments demonstrate that exposure to Schreckstoff does not change individual response thresholds; rather, structural reorganization is the major determinant of the observed changes in the group response (Sosna et al., 2019), making this an example of distributed processing. In contrast, honey bee defense provides a clear example where individual response thresholds do change with exposure: after one bee stings, the tendency for subsequent individuals to sting increases (Millor et al., 1999).
A natural disaster is a example of a perturbation that a human organization must respond to. During a fire, the goal of the fire department is to create a quick and targeted response in order to facilitate a return to homeostasis. Since natural disasters are occasional events but require coordination between people for an effective response, fire departments or other humanitarian organizations rely on a mix of volunteer and paid employees to meet demand. Similar to honey bees and the cellular system described above, optimizing the problem of task allocation requires components of both individual and distributed processing. Task allocation that considers both organizational needs and individual preferences (Falasca and Zobel, 2012) can be considered a form of individual processing, since allocation is linked to intrinsic preferences, not an emergent outcome of interactions. The alternative, fully distributed processing, would represent an ad hoc approach where individuals determine their role based on their interactions with other individuals, and modify according to the need of the collective.
By comparing collective behavior across different systems we can begin to answer question four, which asks how functional mechanisms and behavioral algorithms are adapted to their environment. The characteristics of the environment can specify the types of problems organisms typically encounter, as well as the effectiveness of different solutions. Given a similar problem, the use of different collective mechanisms may reflect adaptations to specific environmental characteristics, different biological constraints, or a combination of both (Gordon, 2016). Within our analysis framework, biological constraints determine the implementation details of different collective mechanisms, as well as the suite of available collective mechanisms. For honey bees and epithelial cells, the constraints on movement, sensory input/output, and information processing abilities are very different, and thus the implementation details and possible application extent of certain collective mechanisms are different. For example, individual epithelial cells have less individual processing ability than individual bees (and also less than other cell types such as neurons). By using a distributed algorithm, the group has more processing ability than individuals (Levin, 2019). While this is true in both systems, the lesser processing abilities of cells may be one reason that they rely more on a distributed algorithm compared to honey bees in our example of the perturbation response. Nonetheless, as the cases of heat stress and wound healing illustrate, the environmental characteristics of both honey bees and epithelial cells have similarities: both systems need to able to respond rapidly to changes in the surrounding environment.
Other social insects interact with different environmental conditions. For example, desert harvester ants forage for seeds that are randomly scattered, but the overall supply is relatively constant over time. Gordon (2016) discusses how this difference can affect behavioral algorithms, noting that because of the scattered distribution of resources in their environment, harvester ants do not exchange spatial information when they recruit additional ants to forage. Conversely, for honey bees, the patchy and ephemeral nature of nectar resources makes it essential to share spatial information among foragers. Nonetheless, because they are both social insects, we may still expect similarities between harvester ants and honey bees in their implementation and use of collective mechanisms, such as the ability of individuals to change task specialization over time. A hierarchical approach provides a natural way to compare collective systems with similar implementation details or available mechanisms but different environmental characteristics (e.g., harvester ants and honey bees), or with different implementations but similar environmental characteristics (e.g., honey bees and epithelial cells).
Human organizations also differ in the types of problems they need to solve. Many human organizations, including businesses, exist in a relatively stable environment; indeed, a stable political and economic environment may be considered a requirement for the advanced specialization and optimization that exemplify modern economies (Acemoglu and Robinson, 2012). Analogous to the environmental differences faced by honey bees and harvester ants, humanitarian logistics for disaster response differs from business logistics because of the unpredictability of the response, and thus needs to be organized differently (Kovacs and Spens, 2007). To this end, human organizations can learn from biological systems in employing mechanisms that are appropriate to address a certain problem (Levin and Lo, 2015).
A key difference between social insects and vertebrate groups or human organizations is the consideration of individual vs. group interests. For honey bees, since reproduction is at the level of the colony, individual, and group interests align. Thus, while a distributed algorithm can contribute to a flexible yet robust group response for honey bees, it could lead to discontent and ineffective group function among human volunteers who each have distinct individual preferences. A distributed process describes the overall decision-making structure of social insect societies, and human organizations with a flat or holocratic organizational structure have similarities in terms of distributed decision processes. However, there are again key distinctions due to individual vs. group interests. While individuals in social insect societies work for the overall function of the group in a structure without dominance hierarchies, a common complaint of human organizations with distributed decision-making structures is that even though it may not be explicitly acknowledged, individual interests cause hierarchy to persist (Freeman, 2013; Bernstein et al., 2016).
A comparative approach allows one to ask if a particular mechanism is used in another system, as well as how it is used (Adams et al., 2012). An example of this is the “individual processing” mechanism of having a distribution of individual response thresholds among group members. For honey bees, this contributes to a colony's ability to maintain stable temperatures inside the nest, as well as enabling a colony's foragers to produce a graded response to resources of different quality. Using a similar mechanism, recent work has used a distribution of individual response thresholds to model how a population of neurons can represent the probability distribution of future rewards by implementing a form of distributional reinforcement learning (Dabney et al., 2020). Collective decision-making processes in animal groups have many similarities to neural systems, including for example the speed-accuracy trade-off during decision-making (Couzin, 2009). A further example of comparing mechanisms across systems is the observed use of cross-inhibition in cellular systems, which led to the hypothesis that similar inhibitory signals should be present between groups during house hunting in honey bees (Seeley et al., 2012).
There are multiple areas for interesting future research, including for example how the use of different behavioral algorithms reflect both individual constraints and environmental conditions. Parallel or convergent evolution refers to when similar phenotypes evolve in similar environments (Langerhans and DeWitt, 2004; Losos, 2011). Examining parallel evolution of collective behavior, e.g., in cichlid fish (Turner, 2007), can reveal the role of the environment in shaping the success of certain collective mechanisms. One could also ask what individual capabilities, collective mechanisms, and/or environmental characteristics are associated with successful adaptations of animal groups. For example, Barrett et al. (2019) describe instances of human-induced environmental change where the use of social learning in a group led to either an adaptive (e.g., Teitelbaum et al., 2016) or maladaptive (e.g., Sigaud et al., 2017) group response.
A comparative approach could furthermore be applied to tumorigenesis, and how a group deals with oncogenic cells. For a tumor to metastasize, tumor cells must pass through the constraints and ecology of the tissue and circulatory system, while avoiding detection and death. To do so, tumor cells change their phenotype by undergoing an epithelial to mesenchymal transition, (EMT), break off from the group, and migrate one cell at a time (Mittal, 2018). In honey bees, worker ovary activation provides an analogy for how an individual can change its phenotype in an attempt to reproduce. Workers typically do not lay reproductive eggs as long as a reproductive queen is present (but see Goudie and Oldroyd, 2018), and workers with fully activated ovaries risk aggression from nest mates (Visscher and Dukas, 1995; Smith et al., 2009). Still, partial ovary activation is widespread in colonies (Smith et al., 2013), even though partial activation incurs costs to the colony (Mattila et al., 2012). Is partial ovary activation permitted because workers lack the ability to detect low-level ovarian development (Q2), because they lack an effective mechanism to form a collective response (Q3), or because there is a functional advantage to having partially-developed workers (Q4)? These questions parallel current cancer research, which asks why some oncogenic cells turn into super-competitors that manifest into tumors, while other mutants are effectively contained and stopped by the epithelial defense against cancer (Vishwakarma and Piddini, 2020). We hope this article inspires further comparisons of collective systems that build on the framework and examples described here.
The raw videos associated with Figures 2D,E are available upon request.
JD, MV, and MS conceived of the study, performed research, and wrote the manuscript. All authors contributed to the article and approved the submitted version.
The authors declare that the research was conducted in the absence of any commercial or financial relationships that could be construed as a potential conflict of interest.
JD, MV, and MS acknowledge support from the Heidelberger Akademie der Wissenschaften under the WIN (wissenschaftlichen Nachwuchs) program. MS acknowledges support from the Simons Foundation as a Postdoctoral Fellow of the Life Sciences Research Foundation. JD and MS acknowledge support from the Deutsche Forschungsgemeinschaft (DFG, German Research Foundation) under Germany's Excellence Strategy—EXC 2117—422037984. MV acknowledges support from the Max Planck Institute for Medical Research, Department of Cellular Biophysics, as well as from the School of Cellular and Molecular Medicine, University of Bristol.
Acemoglu, D., and Robinson, J. A. (2012). Why Nations Fail: The Origins of Power, Prosperity, and Poverty. New York, NY: Crown. doi: 10.1355/ae29-2j
Adams, G. K., Watson, K. K., Pearson, J., and Platt, M. L. (2012). Neuroethology of decision-making. Curr. Opin. Neurobiol. 22, 982–989. doi: 10.1016/j.conb.2012.07.009
Barrett, B., Zepeda, E., Pollack, L., Munson, A., and Sih, A. (2019). Counter-culture: does social learning help or hinder adaptive response to human-induced rapid environmental change? Front. Ecol. Evol. 7:183. doi: 10.3389/fevo.2019.00183
Barton, L. J., LeBlanc, M. G., and Lehmann, R. (2016). Finding their way: themes in germ cell migration. Curr. Opin. Cell Biol. 42, 128–137. doi: 10.1016/j.ceb.2016.07.007
Becher, M. A., Scharpenberg, H., and Moritz, R. F. (2009). Pupal developmental temperature and behavioral specialization of honeybee workers (Apis mellifera L.). J. Compar. Physiol. 195, 673–679. doi: 10.1007/s00359-009-0442-7
Bernstein, E., Bunch, J., Canner, N., and Lee, M. (2016). Beyond the Holacracy Hype. Harvard Business Review. Available online at: https://hbr.org/2016/07/beyond-the-holacracy-hype (accessed July 1, 2016).
Beshers, S. N., Huang, Z. Y., Oono, Y., and Robinson, G. E. (2001). Social inhibition and the regulation of temporal polyethism in honey bees. J. Theor. Biol. 213, 461–479. doi: 10.1006/jtbi.2001.2427
Bi, D., Yang, X., Marchetti, M. C., and Manning, M. L. (2016). Motility-driven glass and jamming transitions in biological tissues. Phys. Rev. X 6:021011. doi: 10.1103/PhysRevX.6.021011
Camley, B. A., and Rappel, W. J. (2017). Physical models of collective cell motility: from cell to tissue. J. Phys. D Appl. Phys. 50:113002. doi: 10.1088/1361-6463/aa56fe
Carandini, M. (2012). From circuits to behavior: a bridge too far? Nat. Neurosci. 15, 507–509. doi: 10.1038/nn.3043
Cook, C. N., and Breed, M. D. (2013). Social context influences the initiation and threshold of thermoregulatory behaviour in honeybees. Anim. Behav. 86, 323–329. doi: 10.1016/j.anbehav.2013.05.021
Couzin, I. D. (2009). Collective cognition in animal groups. Trends Cogn. Sci. 13, 36–43. doi: 10.1016/j.tics.2008.10.002
Couzin, I. D., Krause, J., James, R., Ruxton, G. M., and Franks, N. R. (2002). Collective memory and spatial sorting in animal groups. J. Theor. Biol. 218, 1–11. doi: 10.1006/jtbi.2002.3065
Craver, C. F. (2007). Explaining the Brain: Mechanisms and the Mosaic Unity of Neuroscience. Oxford: Clarendon Press. doi: 10.1093/acprof:oso/9780199299317.003.0007
Dabney, W., Kurth-Nelson, Z., Uchida, N., Starkweather, C. K., Hassabis, D., Munos, R., et al. (2020). A distributional code for value in dopamine-based reinforcement learning. Nature 577, 671–675. doi: 10.1038/s41586-019-1924-6
Das, T., Safferling, K., Rausch, S., Grabe, N., Boehm, H., and Spatz, J. P. (2015). A molecular mechanotransduction pathway regulates collective migration of epithelial cells. Nat. Cell Biol. 17, 276–287. doi: 10.1038/ncb3115
De, S. (2011). Somatic mosaicism in healthy human tissues. Trends Genet. 27, 217–223. doi: 10.1016/j.tig.2011.03.002
Di Gregorio, A., Bowling, S., and Rodriguez, T. (2016). Cell competition and its role in the regulation of cell fitness from development to cancer. Dev. Cell 38, 621–634. doi: 10.1016/j.devcel.2016.08.012
Diwold, K., Schaerf, T. M., Myerscough, M. R., Middendorf, M., and Beekman, M. (2011). Deciding on the wing: in-flight decision making and search space sampling in the red DWARF honeybee Apis florea. Swarm Intell. 5, 121–141. doi: 10.1007/s11721-011-0054-z
Duncan, E. J., Hyink, O., and Dearden, P. K. (2016). Notch signalling mediates reproductive constraint in the adult worker honeybee. Nat. Commun. 7:12427. doi: 10.1038/ncomms12427
Dussutour, A., and Simpson, S. J. (2009). Communal nutrition in ants. Curr. Biol. 19, 740–744. doi: 10.1016/j.cub.2009.03.015
Falasca, M., and Zobel, C. (2012). An optimization model for volunteer assignments in humanitarian organizations. Socio-Econ. Plann. Sci. 46, 250–260. doi: 10.1016/j.seps.2012.07.003
Flack, A., Nagy, M., Fiedler, W., Couzin, I. D., and Wikelski, M. (2018). From local collective behavior to global migratory patterns in white storks. Science 360, 911–914. doi: 10.1126/science.aap7781
Freeman, J. (2013). The tyranny of structurelessness. Women's Stud. Q. 41, 231–246. doi: 10.1353/wsq.2013.0072
Ganapathy, N., Venkataraman, S. S., Daniel, R., Aravind, R. J., and Kumarakrishnan, V. B. (2012). Molecular biology of wound healing. J. Pharm. Bioall. Sci. 4(Suppl. 2), S334–S337. doi: 10.4103/0975-7406.100294
Georgopoulos, N. T., Kirkwood, L. A., and Southgate, J. (2014). A novel bidirectional positive-feedback loop between Wnt-beta-catenin and EGFR-ERK plays a role in context-specific modulation of epithelial tissue regeneration. J. Cell Sci. 127, 2967–2982. doi: 10.1242/jcs.150888
Glennan, S. (2017). The New Mechanical Philosophy. Oxford: Oxford University Press. doi: 10.1093/oso/9780198779711.001.0001
Gordon, D. M. (2016). The evolution of the algorithms for collective behavior. Cell Syst. 3, 514–520. doi: 10.1016/j.cels.2016.10.013
Goudie, F., and Oldroyd, B. P. (2018). The distribution of thelytoky, arrhenotoky and androgenesis among castes in the eusocial Hymenoptera. Insect. Soc. 65, 5–16. doi: 10.1007/s00040-017-0597-0
Grozinger, C. M., Sharabash, N. M., Whitfield, C. W., and Robinson, G. E. (2003). Pheromone-mediated gene expression in the honey bee brain. Proc. Natl. Acad. Sci. U.S.A. 100(Suppl. 2), 14519–14525. doi: 10.1073/pnas.2335884100
Hein, A. M., Altshuler, D. L., Cade, D. E., Liao, J. C., Martin, B. T., and Taylor, G. K. (2020). An algorithmic approach to natural behavior. Curr. Biol. 30, R663–R675. doi: 10.1016/j.cub.2020.04.018
Helbing, D., and Molnár, P. (1995). Social force model for pedestrian dynamics. Phys. Rev. E 51, 4282–4286. doi: 10.1103/PhysRevE.51.4282
Hölldobler, B., and Wilson, E. O. (2009). The Superorganism: the Beauty, Elegance, and Strangeness of Insect Societies. New York, NY: WW Norton.
Huang, Z. Y., and Robinson, G. E. (1992). Honeybee colony integration: worker-worker interactions mediate hormonally regulated plasticity in division of labor. Proc. Natl. Acad. Sci. U.S.A. 89, 11726–11729. doi: 10.1073/pnas.89.24.11726
Huang, Z. Y., and Robinson, G. E. (1996). Regulation of honey bee division of labor by colony age demography. Behav. Ecol. Sociobiol. 39, 147–158. doi: 10.1007/s002650050276
Janson, S., Middendorf, M., and Beekman, M. (2005). Honeybee swarms: how do scouts guide a swarm of uninformed bees? Anim. Behav. 70, 349–358. doi: 10.1016/j.anbehav.2004.10.018
Johnson, B. R. (2010). Division of labor in honeybees: form, function, and proximate mechanisms. Behav. Ecol. Sociobiol. 64, 305–316. doi: 10.1007/s00265-009-0874-7
Jolles, J. W., Boogert, N. J., Sridhar, V. H., Couzin, I. D., and Manica, A. (2017). Consistent individual differences drive collective behavior and group functioning of schooling fish. Curr. Biol. 27, 2862–2868.e7. doi: 10.1016/j.cub.2017.08.004
Jones, J. C., Myerscough, M. R., Graham, S., and Oldroyd, B. P. (2004). Honey bee nest thermoregulation: diversity promotes stability. Science 305, 402–404. doi: 10.1126/science.1096340
Keeling, D. M., Garza, P., Nartey, C. M., and Carvunis, A.-R. (2019). The meanings of 'function' in biology and the problematic case of de novo gene emergence. eLife 8:e47014. doi: 10.7554/eLife.47014
Keller, L., and Nonacs, P. (1993). The role of queen pheromones in social insects: queen control or queen signal? Anim. Behav. 45, 787–794. doi: 10.1006/anbe.1993.1092
Kietzman, P. M., and Visscher, P. K. (2015). The anti-waggle dance: use of the stop signal as negative feedback. Front. Ecol. Evol. 3:14. doi: 10.3389/fevo.2015.00014
Kleineidam, C., Ernst, R., and Roces, F. (2001). Wind-induced ventilation of the giant nests of the leaf-cutting ant Atta vollenweideri. Naturwissenschaften 88, 301–305. doi: 10.1007/s001140100235
Koenig, P. A., Smith, M. L., Horowitz, L. H., Palmer, D. M., and Petersen, K. H. (2020). Artificial shaking signals in honey bee colonies elicit natural responses. Sci. Rep. 10, 4–11. doi: 10.1038/s41598-020-60421-8
Kovacs, G., and Spens, K. M. (2007). Humanitarian logistics in disaster relief operations. Int. J. Phys. Distrib. Logist. Manage. 37, 99–114. doi: 10.1108/09600030710734820
Krakauer, J. W., Ghazanfar, A. A., Gomez-Marin, A., MacIver, M. A., and Poeppel, D. (2017). Neuroscience needs behavior: correcting a reductionist bias. Neuron 93, 480–490. doi: 10.1016/j.neuron.2016.12.041
Kühnholz, S., and Seeley, T. D. (1997). The control of water collection in honey bee colonies. Behav. Ecol. Sociobiol. 41, 407–422. doi: 10.1007/s002650050402
Ladoux, B., and Mège, R.-M. (2017). Mechanobiology of collective cell behaviours. Nat. Rev. Mol. Cell Biol. 18, 743–757. doi: 10.1038/nrm.2017.98
Langerhans, R., and DeWitt, T. (2004). Shared and unique features of evolutionary diversification. Am. Nat. 164, 335–349. doi: 10.1086/422857
Lau, S., Feitzinger, A., Venkiteswaran, G., Wang, J., Lewellis, S. W., Koplinski, C. A., et al. (2020). A negative-feedback loop maintains optimal chemokine concentrations for directional cell migration. Nat. Cell Biol. 22, 266–273. doi: 10.1038/s41556-020-0465-4
Levin, M. (2019). The computational boundary of a “self”: developmental bioelectricity drives multicellularity and scale-free cognition. Front. Psychol. 10:2688. doi: 10.3389/fpsyg.2019.02688
Levin, S. A., and Lo, A. W. (2015). Opinion: a new approach to financial regulation. Proc. Natl. Acad. Sci. U.S.A. 112, 12543–12544. doi: 10.1073/pnas.1518385112
Lindauer, M. (1952). Ein beitrag zur frage der arbeitsteilung im bienenstaat. Zeitsch. Vergleich. Physiol. 34, 299–345. doi: 10.1007/BF00298048
Lindauer, M. (1954). Temperaturregulierung und wasserhaushalt im bienenstaat. Zeitsch. Vergleich. Physiol. 36, 391–432. doi: 10.1007/BF00345028
Losos, J. B. (2011). Convergence, adaptation, and constraint. Evolution 65, 1827–1840. doi: 10.1111/j.1558-5646.2011.01289.x
Macara, I., Guyer, R., Richardson, G., Huo, Y., and Ahmed, S. (2014). Epithelial homeostasis. Curr. Biol. 24, R815–R825. doi: 10.1016/j.cub.2014.06.068
Machta, B. B., Chachra, R., Transtrum, M. K., and Sethna, J. P. (2013). Parameter space compression underlies emergent theories and predictive models. Science 342, 604–607. doi: 10.1126/science.1238723
Marr, D. (1982). Vision: A Computational Investigation into the Human Representation and Processing of Visual Information. New York, NY: Henry Holt and Co., Inc.
Maruska, K. P., and Fernald, R. D. (2013). Social regulation of male reproductive plasticity in an African cichlid fish. Integr. Compar. Biol. 53, 938–950. doi: 10.1093/icb/ict017
Mattila, H. R., Reeve, H. K., and Smith, M. L. (2012). Promiscuous honey bee queens increase colony productivity by suppressing worker selfishness. Curr. Biol. 22, 2027–2031. doi: 10.1016/j.cub.2012.08.021
Mayor, R., and Carmona-Fontaine, C. (2010). Keeping in touch with contact inhibition of locomotion. Trends Cell Biol. 20, 319–328. doi: 10.1016/j.tcb.2010.03.005
Mersch, D. P., Crespi, A., and Keller, L. (2013). Tracking individuals shows spatial fidelity is a key regulator of ant social organization. Science 340, 1090–1093. doi: 10.1126/science.1234316
Michener, C. D. (1969). Comparative social behavior of bees. Annu. Rev. Entomol. 14, 299–342. doi: 10.1146/annurev.en.14.010169.001503
Millor, J., Pham-Delegue, M., Deneubourg, J. L., and Camazine, S. (1999). Self-organized defensive behavior in honeybees. Proc. Natl. Acad. Sci. U.S.A. 96, 12611–12615. doi: 10.1073/pnas.96.22.12611
Mittal, V. (2018). Epithelial mesenchymal transition in tumor metastasis. Annu. Rev. Pathol. 13, 395–412. doi: 10.1146/annurev-pathol-020117-043854
Modlmeier, A. P., Colman, E., Hanks, E. M., Bringenberg, R., Bansal, S., and Hughes, D. P. (2019). Ant colonies maintain social homeostasis in the face of decreased density. eLife 8:e38473. doi: 10.7554/eLife.38473
Nieh, J. C. (1993). The stop signal of honey bees: reconsidering its message. Behav. Ecol. Sociobiol. 33, 51–56. doi: 10.1007/BF00164346
Nieh, J. C. (1998). The honey bee shaking signal: function and design of a modulatory communication signal. Behav. Ecol. Sociobiol. 42, 23–36. doi: 10.1007/s002650050408
Noble, G. K. (1939). The role of dominance in the social life of birds. Auk 56, 263–273. doi: 10.2307/4079047
Nowell, P. C. (1976). The clonal evolution of tumor cell populations. Science 194, 23–28. doi: 10.1126/science.959840
Omelchenko, T., Vasiliev, J. M., Gelfand, I. M., Feder, H. H., and Bonder, E. M. (2003). Rho-dependent formation of epithelial “leader” cells during wound healing. Proc. Natl. Acad. Sci. U.S.A. 100, 10788–10793. doi: 10.1073/pnas.1834401100
Ostwald, M. M., Smith, M. L., and Seeley, T. D. (2016). The behavioral regulation of thirst, water collection and water storage in honey bee colonies. J. Exp. Biol. 219, 2156–2165. doi: 10.1242/jeb.139824
Papageorgiou, D., Christensen, C., Gall, G. E. C., Klarevas-Irby, J. A., Nyaguthii, B., Couzin, I. D., et al. (2019). The multilevel society of a small-brained bird. Curr. Biol. 29, R1120–R1121. doi: 10.1016/j.cub.2019.09.072
Park, S., Gonzalez, D. G., Guirao, B., Boucher, J. D., Cockburn, K., Marsh, E. D., et al. (2017). Tissue-scale coordination of cellular behaviour promotes epidermal wound repair in live mice. Nat. Cell Biol. 19, 155–163. doi: 10.1038/ncb3472
Passino, K. M., and Seeley, T. D. (2006). Modeling and analysis of nest-site selection by honeybee swarms: the speed and accuracy trade-off. Behav. Ecol. Sociobiol. 59, 427–442. doi: 10.1007/s00265-005-0067-y
Poujade, M., Grasland-Mongrain, E., Hertzog, A., Jouanneau, J., Chavrier, P., Ladoux, B., et al. (2007). Collective migration of an epithelial monolayer in response to a model wound. Proc. Natl. Acad. Sci. U.S.A. 104, 15988–15993. doi: 10.1073/pnas.0705062104
Ratnieks, F. L. W., and Visscher, P. K. (1989). Worker policing in the honeybee. Nature 342, 796–797. doi: 10.1038/342796a0
Robertson, B. J. (2015). Holacracy: The New Management System for a Rapidly Changing World. New York, NY: Henry Holt and Company. doi: 10.15358/9783800650880
Robinson, G. E., Underwood, B. A., and Henderson, C. E. (1984). A highly specialized water-collecting honey bee. Apidologie 15, 355–358. doi: 10.1051/apido:19840307
Rosenthal, S. B., Twomey, C. R., Hartnett, A. T., Wu, H. S., and Couzin, I. D. (2015). Revealing the hidden networks of interaction in mobile animal groups allows prediction of complex behavioral contagion. Proc. Natl. Acad. Sci. U.S.A. 112, 4690–4695. doi: 10.1073/pnas.1420068112
Saxén, L., and Lehtonen, E. (1986). “Cells into organs,” in Coordinated Regulation of Gene Expression, eds R. M. Clayton and D. E. S. Truman (Boston, MA: Springer US), 269–278. doi: 10.1007/978-1-4613-2245-0_24
Schreier, A. L., and Swedell, L. (2009). The fourth level of social structure in a multi-level society: ecological and social functions of clans in hamadryas baboons. Am. J. Primatol. 71, 948–955. doi: 10.1002/ajp.20736
Seeley, T. D. (1982). Adaptive significance of the age polyethism schedule in honeybee colonies. Behav. Ecol. Sociobiol. 11, 287–293. doi: 10.1007/BF00299306
Seeley, T. D. (1992). The tremble dance of the honey bee: message and meanings. Behav. Ecol. Sociobiol. 31, 375–383. doi: 10.1007/BF00170604
Seeley, T. D. (1994). Honey bee foragers as sensory units of their colonies. Behav. Ecol. Sociobiol. 34, 51–62. doi: 10.1007/BF00175458
Seeley, T. D., Kühnholz, S., and Weidenmüller, A. (1996). The honey bee's tremble dance stimulates additional bees to function as nectar receivers. Behav. Ecol. Sociobiol. 39, 419–427. doi: 10.1007/s002650050309
Seeley, T. D., Visscher, P. K., Schlegel, T., Hogan, P. M., Franks, N. R., and Marshall, J. A. R. (2012). Stop signals provide cross inhibition in collective decision-making by honeybee swarms. Science 335, 108–111. doi: 10.1126/science.1210361
Seeley, T. D., Weidenmuller, A., and Kuhnholz, S. (1998). The shaking signal of the honey bee informs workers to prepare for greater activity. Ethology 104, 10–26. doi: 10.1111/j.1439-0310.1998.tb00026.x
Sigaud, M., Merkle, J. A., Cherry, S. G., Fryxell, J. M., Berdahl, A., and Fortin, D. (2017). Collective decision-making promotes fitness loss in a fusion-fission society. Ecol. Lett. 20, 33–40. doi: 10.1111/ele.12698
Slessor, K. N., Winston, M. L., and Le Conte, Y. (2005). Pheromone communication in the honeybee (Apis mellifera l.). J. Chem. Ecol. 31, 2731–2745. doi: 10.1007/s10886-005-7623-9
Smith, A. A., Hölldobler, B., and Liebig, J. (2009). Cuticular hydrocarbons reliably identify cheaters and allow enforcement of altruism in a social insect. Curr. Biol. 19, 78–81. doi: 10.1016/j.cub.2008.11.059
Smith, M. L. (2018). Queenless honey bees build infrastructure for direct reproduction until their new queen proves her worth. Evolution 72, 2810–2817. doi: 10.1111/evo.13628
Smith, M. L., Mattila, H. R., and Reeve, H. K. (2013). Partial ovary development is widespread in honey bees and comparable to other eusocial bees and wasps. Commun. Integr. Biol. 6:e25004. doi: 10.4161/cib.25004
Smith, M. L., Ostwald, M. M., and Seeley, T. D. (2016). Honey bee sociometry: tracking honey bee colonies and their nest contents from colony founding until death. Insect. Soc. 63, 553–563. doi: 10.1007/s00040-016-0499-6
Sosna, M. M. G., Twomey, C. R., Bak-Coleman, J., Poel, W., Daniels, B. C., Romanczuk, P., et al. (2019). Individual and collective encoding of risk in animal groups. Proc. Natl. Acad. Sci. U.S.A. 116, 20556–20561. doi: 10.1073/pnas.1905585116
Strandburg-Peshkin, A., Farine, D. R., Couzin, I. D., and Crofoot, M. C. (2015). Shared decision-making drives collective movement in wild baboons. Science 348, 1358–1361. doi: 10.1126/science.aaa5099
Sullivan, J. P., Jassim, O., Fahrbach, S. E., and Robinson, G. E. (2000). Juvenile hormone paces behavioral development in the adult worker honey bee. Hormones Behav. 37, 1–14. doi: 10.1006/hbeh.1999.1552
Sumpter, D. (2006). The principles of collective animal behaviour. Philos. Trans. R. Soc. B Biol. Sci. 361, 5–22. doi: 10.1098/rstb.2005.1733
Taborsky, M. (2014). Tribute to tinbergen: the four problems of biology. A critical appraisal. Ethology 120, 224–227. doi: 10.1111/eth.12209
Teitelbaum, C. S., Converse, S. J., Fagan, W. F., Böhning-Gaese, K., O'Hara, R. B., Lacy, A. E., et al. (2016). Experience drives innovation of new migration patterns of whooping cranes in response to global change. Nat. Commun. 7:12793. doi: 10.1038/ncomms12793
Tinbergen, N. (1963). On aims and methods of Ethology. Zeigsch. Tierpsychol. 20, 410–433. doi: 10.1111/j.1439-0310.1963.tb01161.x
Transtrum, M. K., Machta, B. B., Brown, K. S., Daniels, B. C., Myers, C. R., and Sethna, J. P. (2015). Perspective: Sloppiness and emergent theories in physics, biology, and beyond. J. Chem. Phys. 143:010901. doi: 10.1063/1.4923066
Turner, G. F. (2007). Adaptive radiation of cichlid fish. Curr. Biol. 17, R827–R831. doi: 10.1016/j.cub.2007.07.026
Vishwakarma, M., and Piddini, E. (2020). Outcompeting cancer. Nat. Rev. Cancer 20, 187–198. doi: 10.1038/s41568-019-0231-8
Vishwakarma, M., Russo, J. D., Probst, D., Schwarz, U. S., Das, T., and Spatz, J. P. (2018). Mechanical interactions among followers determine the emergence of leaders in migrating epithelial cell collectives. Nat. Commun. 9:3469. doi: 10.1038/s41467-018-05927-6
Visscher, P. K., and Dukas, R. (1995). Honey bees recognize development of nestmates' ovaries. Anim. Behav. 49, 542–544. doi: 10.1006/anbe.1995.0074
von Frisch, K. (1967). The Dance Language and Orientation of Bees. Transl. by Leigh E. Chadwick. Cambridge, MA: Belknap Press of Harvard University Press.
Weijer, C. J. (2009). Collective cell migration in development. J. Cell Sci. 122, 3215–3223. doi: 10.1242/jcs.036517
Wheeler, W. M. (1928). The Social Insects: Their Origin and Evolution. London: K. Paul, Trench, Trubner & Company, Limited. doi: 10.5962/bhl.title.140774
Wild, B., Dormagen, D. M., Zachariae, A., Smith, M. L., Traynor, K. S., Brockmann, D., et al. (2020). Social networks predict the life and death of honey bees. bioRxiv [Preprint]. doi: 10.1101/2020.05.06.076943
Wilson, E. O. (1971). The Insect Societies. Cambridge, MA: Belknap Press of Harvard University Press.
Wu, J. (2014). Novel factors for cell differentiation and reprogramming. J. Mol. Cell Biol. 6:355. doi: 10.1093/jmcb/mju035
Keywords: multi-scale, systems theory, honey bees, cellular systems, group behavior, mechanisms
Citation: Davidson JD, Vishwakarma M and Smith ML (2021) Hierarchical Approach for Comparing Collective Behavior Across Scales: Cellular Systems to Honey Bee Colonies. Front. Ecol. Evol. 9:581222. doi: 10.3389/fevo.2021.581222
Received: 09 July 2020; Accepted: 04 January 2021;
Published: 02 February 2021.
Edited by:
Mark A. Elgar, The University of Melbourne, AustraliaReviewed by:
Sharoni Shafir, Hebrew University of Jerusalem, IsraelCopyright © 2021 Davidson, Vishwakarma and Smith. This is an open-access article distributed under the terms of the Creative Commons Attribution License (CC BY). The use, distribution or reproduction in other forums is permitted, provided the original author(s) and the copyright owner(s) are credited and that the original publication in this journal is cited, in accordance with accepted academic practice. No use, distribution or reproduction is permitted which does not comply with these terms.
*Correspondence: Jacob D. Davidson, amRhdmlkc29uQGFiLm1wZy5kZQ==; Medhavi Vishwakarma, bWVkaGF2aS52aXNod2FrYXJtYUBtci5tcGcuZGU=; Michael L. Smith, bXNtaXRoQGFiLm1wZy5kZQ==
Disclaimer: All claims expressed in this article are solely those of the authors and do not necessarily represent those of their affiliated organizations, or those of the publisher, the editors and the reviewers. Any product that may be evaluated in this article or claim that may be made by its manufacturer is not guaranteed or endorsed by the publisher.
Research integrity at Frontiers
Learn more about the work of our research integrity team to safeguard the quality of each article we publish.