- Department of Ecology and Evolutionary Biology, Applied Wildlife Ecology Lab, University of Michigan, Ann Arbor, MI, United States
Rapid urbanization coupled with increased human activity induces pressures that affect predator-prey relations through a suite of behavioral mechanisms, including alteration of avoidance and coexistence dynamics. Synergisms of natural and anthropogenic threats existing within urban environments exacerbate the necessity for species to differentially modify behavior to each risk. Here, we explore the behavioral response of a key prey species, cottontail rabbits (Sylvilagus floridanus), to pressures from humans, domestic dogs, and a natural predator, coyotes (Canis latrans) in a human-dominated landscape. We conducted the first camera survey in urban parks throughout Detroit, Michigan in 2017–2020 to assess vigilance response corresponding to a heterogeneous landscape created from variation in the occupancy of threats. We predicted a scaled response where cottontail rabbits would be most vigilant in areas with high coyote activity, moderately vigilant in areas with high domestic dog activity, and the least vigilant in areas of high human activity. From 8,165 independent cottontail rabbit detections in Detroit across 11,616 trap nights, one-third were classified as vigilant. We found vigilance behavior increased with coyote occupancy and in locations with significantly high domestic dog activity, but found no significant impact of human occupancy or their spatial hotspots. We also found little spatial overlap between rabbits and threats, suggesting rabbits invest more in spatial avoidance; thus, less effort is required for vigilance. Our results elucidate strategies of a prey species coping with various risks to advance our understanding of the adaptability of wildlife in urban environments. In order to promote coexistence between people and wildlife in urban greenspaces, we must understand and anticipate the ecological implications of human-induced behavioral modifications.
Introduction
The 20th and 21st centuries have seen unprecedented population growth and expansion of cities, with 60% of the global population expected to live in urban centers by the year 2030 (United Nations, 2018). Urbanization coupled with other increased anthropogenic pressures has fundamentally changed ecosystems worldwide (Foley et al., 2005; Grimm et al., 2008; Pickard et al., 2017; Chen et al., 2020). Cities fragment natural habitat and restrict gene flow, change species assemblages, and alter the behavior of animals and people alike (Romano, 2002; Tigas et al., 2002; Crooks et al., 2004; Lowry et al., 2013; Johnson and Munshi-South, 2017). These environmental perturbations have implications for wildlife and a myriad of ecological interactions including predator-prey relationships.
Non-consumptive fear effects induced by humans are pervasive in urban environments and drive behavioral changes in wildlife (Ciuti et al., 2012; Gaynor et al., 2018). For example, eastern gray squirrels (Sciurus carolinensis) in New York City have become sensitive to human movements and show behavioral plasticity in their ability to adjust flight initiation distance based on human activity (Bateman and Fleming, 2014). Exposure to human audio cues reduced foraging time and increased the amount of time spent being vigilant in badgers (Meles meles) in Great Britain as compared to exposure to non-human predator audio cues (Clinchy et al., 2016). Behavioral plasticity in predator and prey species alike directly influence their ability to avoid and coexist with intense human pressures in urban centers (Muhly et al., 2011; Lowry et al., 2013). While prey modify their behavior to avoid attempted predation, predators modify their behavior to account for prey behavior and to increase the likelihood of success of their predation attempts. Specifically, prey are forced to modify their behavior spatially or temporally to avoid threats from humans as well as associated domestic animals or natural predators (Fenn and Macdonald, 1995; Gliwicz and Dąbrowski, 2008; Reilly et al., 2017). Modification of behaviors have therefore become necessary for the survival of both predators and prey in urban environments, as risks govern behavior (Lima, 1998). However, despite the recent burgeoning of urban ecology studies, how humans and domestic animals alter mammalian vigilance behavior remains understudied.
Highly adaptable species and those with relatively smaller body sizes are more successful at coexisting with humans in urban areas (Bateman and Fleming, 2012). Carnivores, particularly large bodied carnivores, have historically faced intense persecution from humans (Bruskotter et al., 2017). Large predators depredate livestock and compete with humans for resources including space and prey, often resulting in humans employing lethal interventions (Mech, 1995; Witmer and Whittaker, 2001; Treves and Karanth, 2003; Muhly and Musiani, 2009). However, many mid to small-sized predators are able to thrive in areas of high anthropogenic influence (Wilkinson and Smith, 2001; Ikeda et al., 2004). In particular, coyotes (Canis latrans) have adapted to living with humans in part, by exploiting anthropogenic food subsidies and shifting diurnal movement in response to human disturbance (Kitchen et al., 2000; Gese and Beckoff, 2004). This, in conjunction with wide extirpations of the gray wolf (Canis lupus), has allowed coyotes to expand their range to the entirety of the United States beyond previous restrictions to the central and western portions of the country (Crooks and Soulé, 1999; Hody and Kays, 2018). Domestic dogs (Canis familiaris) have similarly become abundant within urban areas and thus, can exert top-down pressures as a member of the carnivore community (Ordeñana et al., 2010). These ecological and behavioral changes in carnivores can have cascading effects on their prey species, subsequently altering their behavior.
Concurrent with predators employing strategies for coexistence, their prey must also mitigate risks in human dominated landscapes. Threats for prey species in urban environments are often exacerbated by multiple sources including direct mortality from natural and anthropogenic sources. Prey may employ similar strategies to mitigate risks from humans as they do to mitigate risks from natural predators (Parsons et al., 2016). As such, fear effects in urban environments can result in prey modifying temporal activity or habitat selection to reduce predation risks (Chambers and Dickman, 2002; Dowding et al., 2010). Discernment between immediate and distal threats requires delegating time to vigilance in order to assess and respond to risks across the landscape. However, there are tradeoffs because more time spent being vigilant means less time foraging, mating, and performing other behaviors like grooming (Quenette, 1990). Environmental conditions including vegetation height, tree cover, and the distribution of water sources can interact to produce varying levels of predation risk and thus influence the amount of time prey spend being vigilant (Scheel, 1993; Tchabovsky et al., 2001).
Cottontail rabbits (Sylvilagus floridanus) are a key prey source for many mammalian carnivores as well as avian predators and occasionally snakes in urban environments throughout the United States (Beasom and Moore, 1977; Litvaitis and Shaw, 1980; Wittenberg, 2012). Because rabbits are an important part of coyotes’ diet, along with small rodents, coyotes exert top-down pressures to control their populations (Poessel et al., 2017). Cottontail rabbits have high reproductive rates that result in rapidly growing populations that interact, directly or indirectly, with humans in gardens, yards, parks and other green spaces throughout city limits (Hunt et al., 2014; Baker et al., 2015). We conducted a non-invasive camera survey to investigate the vigilance behavior of rabbits in response to anthropogenic and natural threats. Our work occurred throughout Detroit, the largest city in Michigan, located in the Great Lakes region of the United States from 2017 to 2020. Here, we delineated human, coyote, and domestic dog risk zones to detect differences in cottontail vigilance response and investigated the potential factors influencing vigilance.
Species exploiting urban environments may exhibit higher plasticity to cope and acclimate with anthropogenic threats (Samia et al., 2015). The gray squirrel (Sciurus carolinensis), another common urban prey species, is less wary of humans in areas more densely populated by humans (Parker and Nilon, 2008). This suggests a level of acclimation to human presence, which we reasonably anticipate occurring in cottontail rabbits who are exposed to similar pressures of human activity in an urban environment. Therefore, we expect a similar level of acclimation in cottontail rabbits where they are less vigilant in areas heavily populated by humans. Because of the similarities in body size and behavior between domestic dogs and coyotes, we anticipate rabbits will show more vigilance in areas with high domestic dog presence than areas with high human presence. However, domestic dog populations are generally larger in urban areas because of association with humans. In Detroit, we anticipate some level of acclimation to their presence from cottontail rabbits and therefore, we expect the response to dogs to be less dramatic than the response to coyotes. However, unaccompanied dogs could illicit pronounced fear responses. Overall, we expect a scaled response where rabbits will be least vigilant in areas with high human activity, with vigilance response increasing slightly in the areas with high domestic dog activity, and the most vigilance being displayed in areas of high coyote activity, as coyotes are an actual formidable predator of rabbits (Figure 1). Results will further our understanding of how a key prey species behaves in dynamic urban landscapes, information necessary to foster safe and positive interactions between people and wildlife coexisting in the city.
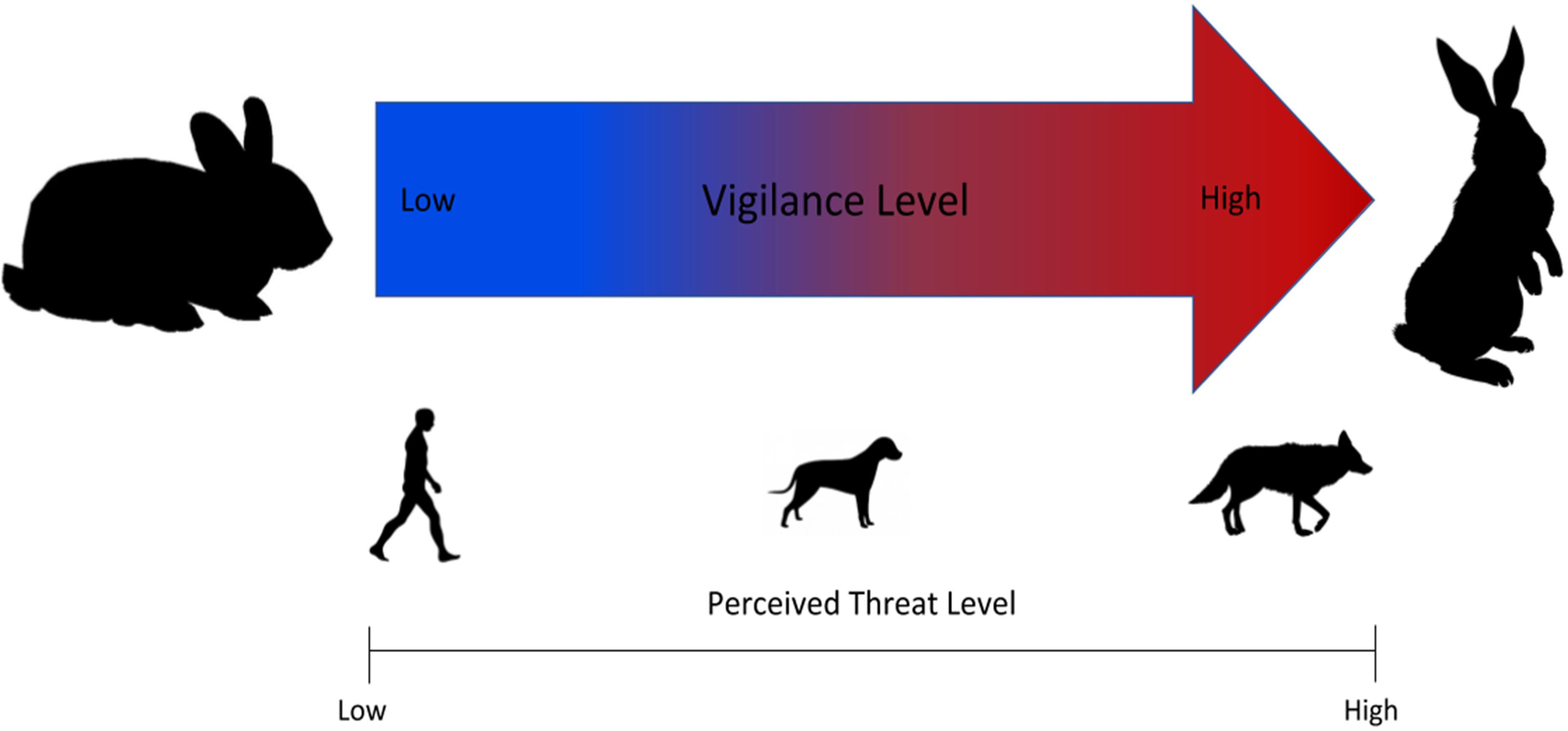
Figure 1. Expected vigilance response of cottontail rabbits with scaled response across natural and anthropogenic threats.
Materials and Methods
Study Site
We implemented a systematic camera survey throughout metro parks in Detroit, the largest city in Michigan covering 359.2 km2 of land (Figure 2). The declining city holds a human population of 672,000 people with an average density of ∼5,144 people per square mile (U.S. Census Bureau, 2016). The Detroit metro park system contributes to the green space and available habitat for wildlife within the city. All 28 total parks sampled within the city are impacted directly or indirectly by humans and are embedded within an urban matrix including roads, neighborhoods, and buildings. The parks range in size from ∼0.016 to 4.79 km2 with varying levels of vegetation and human influence. In Detroit, the largest native carnivore present is the coyote. However, domestic dogs are also present and may exert pressures on the coyote’s natural prey species such as rabbits.
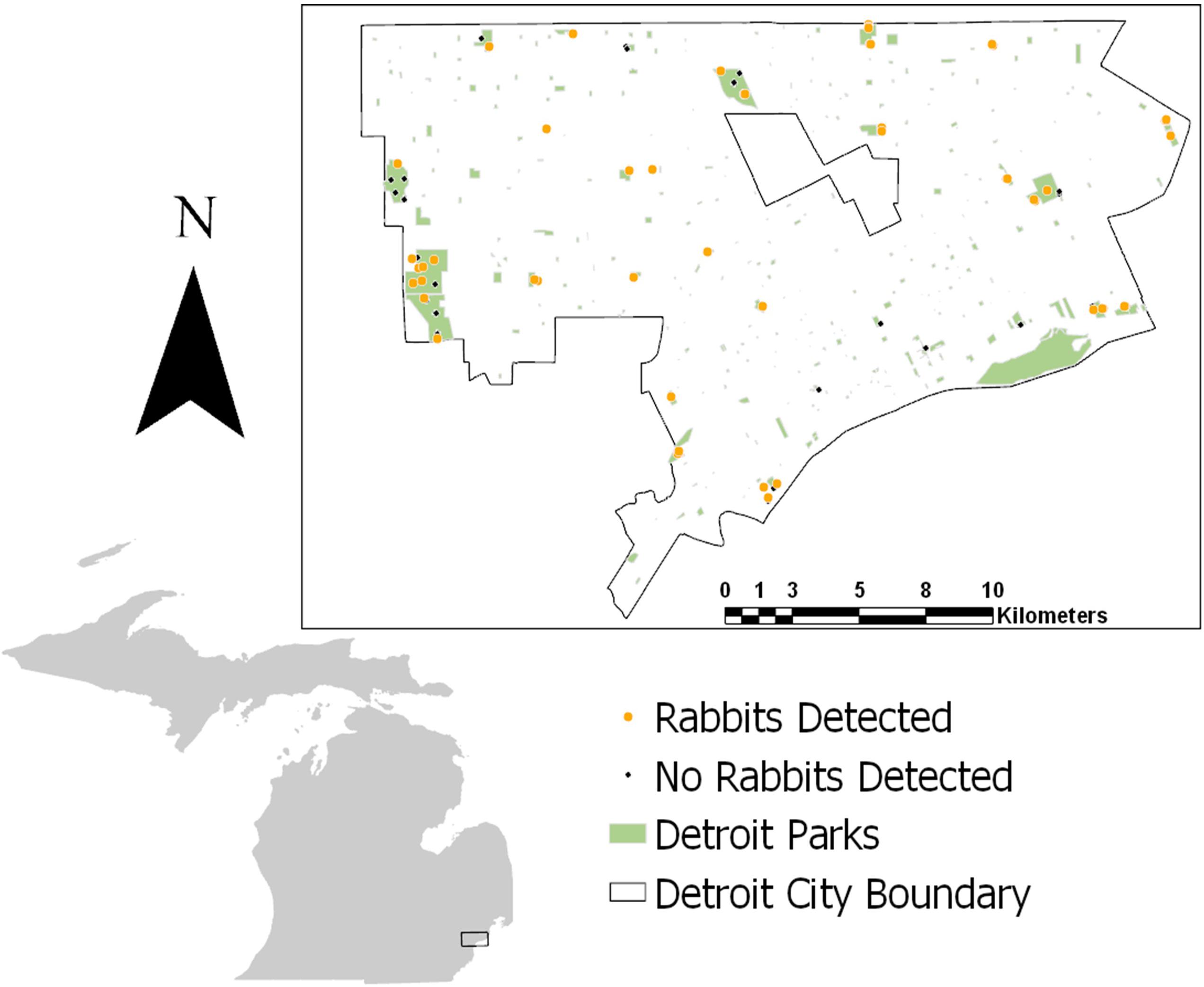
Figure 2. Study site in Detroit with dots indicating camera placement from 2017 to 2020. Orange dots indicate camera stations where rabbits were detected in at least one of the years of study. Black dots indicate camera stations where no rabbits were detected in any years.
Data Collection/Camera Survey
We deployed unbaited, remotely triggered cameras (Reconyx© PC 850, 850C, 900, 900C) throughout city parks to monitor the wildlife community from October – March in 2017–2020. Placement within the parks was determined based on evidence of wildlife presence such as scat, and vegetation type. Park size determined the number of cameras deployed, ranging from 1 to 7 cameras. For parks with multiple cameras, we deployed cameras with a minimum distance of 500 m between individual cameras. Cameras were affixed to medium sized trees approximately 0.5–1 m off the ground. We programmed cameras to take three images when triggered at high sensitivity with 1-s between each image and a 15-s quiet period. Every image was independently sorted and confirmed by at least two members of the Applied Wildlife Ecology Lab at the University of Michigan. We only used images confirmed as rabbit as well as their associated threat species of interests: humans, domestic dogs, and coyotes. Both gray (Urocyon cinereoargenteus) and red foxes (Vulpes vulpes) are also potential predators of cottontails, but sample sizes were too low to include in our analysis. Team members were excluded from human images.
Hotspot Analysis and Occupancy Modeling
To determine the level of risk from each of our three potential predator focal species, we used two methods to capture their spatial variation in parks across Detroit. First, we used kernel density analysis to construct utilization distributions from rabbit, human, coyote, and domestic dog camera triggers in ArcMap (v. 10.6.1). To test for significant spatial clustering (i.e., hotspots), we applied the Getis-Ord GI∗ statistic to species triggers, which summarizes spatial autocorrelation with resultant high positive z-scores indicating clustering and low negative z-scores indicating dispersion (Getis and Ord, 1992). Specifically, significant trigger hotspots and coldspots are derived from z-scores greater than 1.96 and less than 1.96 (α < 0.05), respectively. Finally, we overlaid significant trigger hotspots for rabbits with associated threats to determine if rabbits avoided hotspots for humans, dogs, or coyotes across the city. In other words, we assessed whether trigger hotspots for rabbits were congruent with any of the threats. Evidence of spatial avoidance may represent a sufficient evasion strategy that necessitates less vigilance behavior.
Second, we constructed single-species, single-season occupancy models for humans, domestic dog, and coyotes, which correct for imperfect detections from repeated surveys (MacKenzie et al., 2003; MacKenzie and Royle, 2005). In our case, we used 1-week sampling intervals to generate detection histories. By holding occupancy constant, we first built detection models with camera model (CAM), understory vegetation at camera (UAC), number of trap nights (TN), and park size (AREA) as covariates. We then used the top detection model to build occupancy models with housing density within 500 meters (HOUSE), prey trap success (PREYTS), UAC, and AREA. PREYTS was calculated at the camera level as the ratio of cottontail rabbit, squirrel, chipmunk (Tamias striatus), and small mammal total triggers by number of trap nights. We identified top models using Akaike’s Information Criterion corrected for small sample sizes (AICc) based on the lowest Δ AIC and greatest weight (w). We also assessed goodness-of-fit for each model using the chi-squared discrepancy method in the “ResourceSelection” package. We constructed detection histories using “camtrapR,” and completed occupancy modeling in “unmarked” packages. All analysis was completed in Program R.
Vigilance Scoring
We extracted behavioral information from images in order to quantify vigilance response in cottontail rabbits. For each image containing a rabbit, we scored vigilance based upon the position of the body and head (Figure 3). For images with two individuals, each individual was given its own classification and counted as independent from other individuals in the image. Rabbits were considered “vigilant” if their head was in an upright position; while “non-vigilant” was assigned when their head was down in a foraging position. For images where the rabbit did not display an obvious head up or head down stance, we used six other classifications: moving, active, eating, sniffing, out of frame, and unknown. “Moving” included any rabbit in motion, which was often indicated by motion blur in the images. We considered moving to be a potential indicator of vigilance as it could denote rabbits leaving an area due potentially to a detected threat. “Sniffing” included rabbit attention turned to monitoring an aspect of its environment with its head up such as sniffing twigs. Because we are investigating the impact of canid species on rabbit behavior and canids often mark their territory (Bowen and Cowan, 1980), we considered sniffing to potentially indicate vigilance as it is a show of risk assessment. Both sniffing and moving were left out of our initial vigilant vs. non-vigilant analysis but were included in the vigilant category in our extended analysis. “Active” was used for activity where the animal’s attention was pointed inward at themselves. This included any rabbits scratching, licking, or otherwise attending to their fur, this also included stretching. “Eating” was used in the event that a rabbit had its head up, but clearly had vegetation in its mouth or the image series showed it chewing. Although both active and eating involve attention being pointed inward at the rabbit, we did not include them as non-vigilant in our analysis as we could not confirm non-vigilance. “Out of frame” included any images where the rabbit exited the frame of the picture and nothing was in the image. Images that were sorted as out of frame were removed from the data set and not counted in the final total. Finally, “unknown” was used for rabbits where only parts of the whole body were in the picture, the head was too blurry to determine, or if the body position could not be determined for any other reason. Unknown photos were also removed from the final total. Each individual was only designated one category per each image in which it appeared. All images with rabbits present were used to best estimate the amount of time actually spent in front of the camera at the particular station. We only used photos where rabbits were in the frame, meaning our photos are estimates of time spent in frame. Each image was scored independently for vigilance by at least two members of the Applied Wildlife Ecology Lab at the University of Michigan. Any discrepancies that were not resolved resulted in classifying the image as unknown.
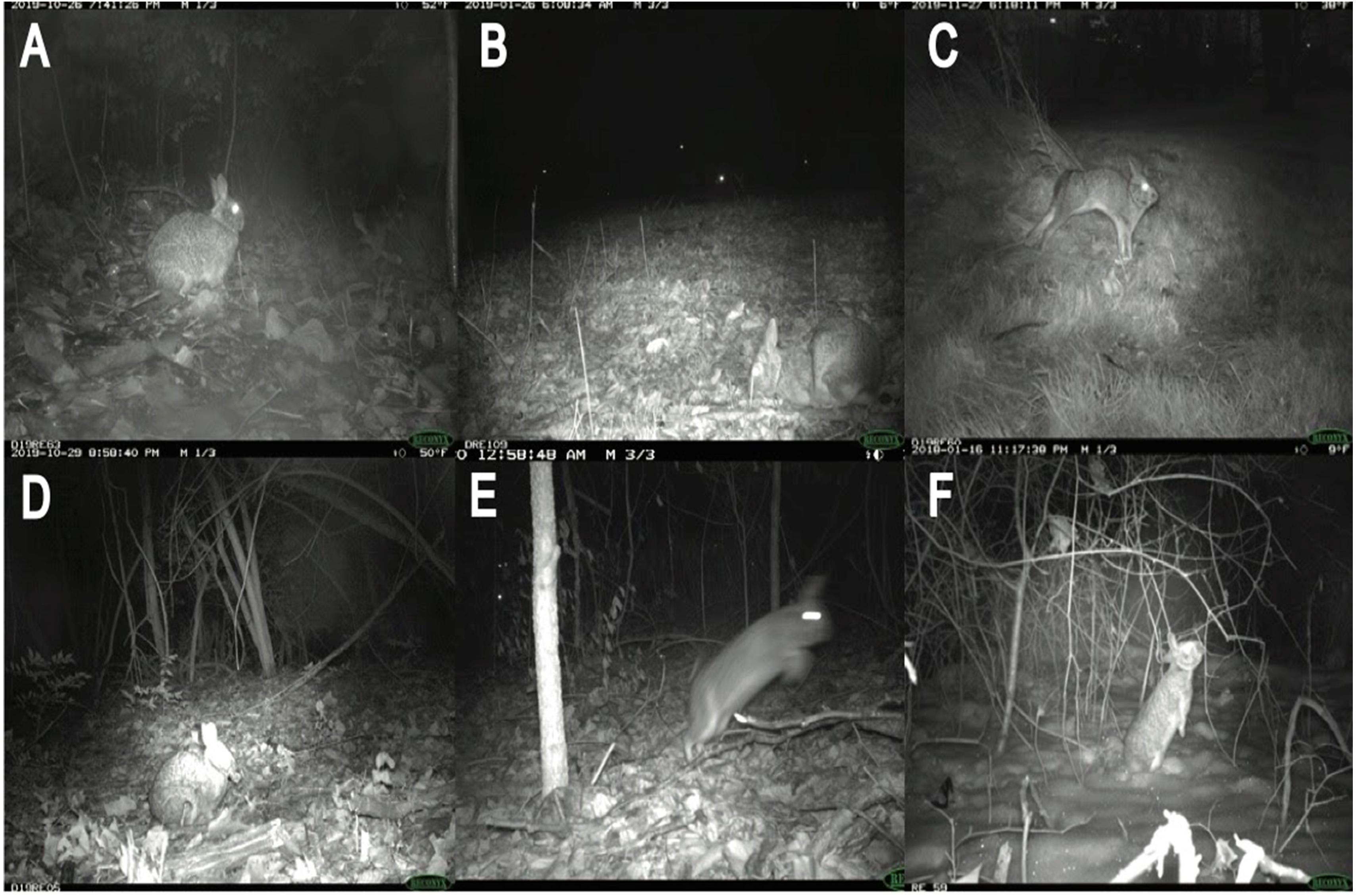
Figure 3. Vigilance classifications based on body position of cottontail rabbits: (A) vigilant, head-up; (B) non-vigilant, head down; (C) active; (D) eating; (E) moving; and (F) sniffing.
We calculated multiple metrics of vigilance as a response variable to each risk factor. Initially, we used the raw number of images classified as vigilant per camera. Our second measure of vigilance was the ratio of vigilant photos to the total number of photos. This was used as a proxy for the relative amount of time spent being vigilant at each camera. For both these metrics, we started with just vigilant and non-vigilant and then expanded the classification of vigilant beyond head up versus head down and included moving and sniffing as vigilant. We used the total raw counts for these combined categories as well as the ratio of those categories out of the total number of detections as our “vigilant” response variable.
Statistical Analysis
We used negative binomial generalized linear models (GLM.nb) to determine which factors best-explained cottontail rabbit vigilance across cameras. We used results from the hotspot analysis to identify locations of significant high use based on kernel density estimates from detection data to categorize threat levels for humans, domestic dogs, and coyotes. This resulted in a binary explanatory variable indicating whether a hotspot was presence (1) or absence (0) for each threat. We also used occupancy estimates from top models for coyotes (COYO), humans (HUMO), and domestic dogs (DOGO) as threat covariates. We also included environmental and abiotic factors in our analysis. We calculated distance from each camera station to water sources (WATER), to roads (ROADS), and the area of each park (AREA, in acres) using ArcMap. We quantified understory cover (VEG) as a binary variable of whether trees, tall shrubs, bushes, or grasses were present or not in the field of view at the camera level.
Support for models was evaluated using Akaike’s Information Criterion (AICc) to select top-performing model (Δ AIC < 2) with highest weight (w). We also assessed goodness-of-fit for each model using the chi-squared discrepancy method the “ResourceSelection” package. We completed modeling in the “lme4” package and model selection in the “MuMIn” package. All analysis was completed in Program R.
Results
We obtained 8,165 cottontail rabbit detections from 58 camera locations in Detroit across 11,616 trap nights from our 2017–2020 surveys (Table 1). The average trap night per camera for the survey period was 99.8 (Range: 18–121) including two cameras which malfunctioned after 18 days, excluding the outliers the average was 101.2 (Range: 74–121). For parks with >1 camera station, cameras were spaced on average 1.4 km apart within parks spaced an average distance of 3.2 km apart. We recorded 1,345 human detections at 27 camera stations, 484 domestic dog detections at 33 stations, and 271 coyote detections at 29 stations. Three stations (one in 2017 and two in 2019) had no coyote, domestic dog, or human detections. No cameras had significant trigger densities for all three threat species at the same station for the entire duration of study based on Getis-Ord Gi∗ statistics (Figure 4). Instead, coyotes had significantly high trigger densities to form a hotspot at only one station in 2019. Domestic dogs had hotspots at the same station across two different years. Humans had hotspots at three stations across the 3 years of study, with two of those stations recurring across years. Rabbits had significant trigger densities at the same two stations across 2 years. We found spatial aggregation of rabbits with dog at one hotspot location in 2 years. However, we saw no significant overlap in hotspots between rabbits and humans or coyotes (Figure 4).
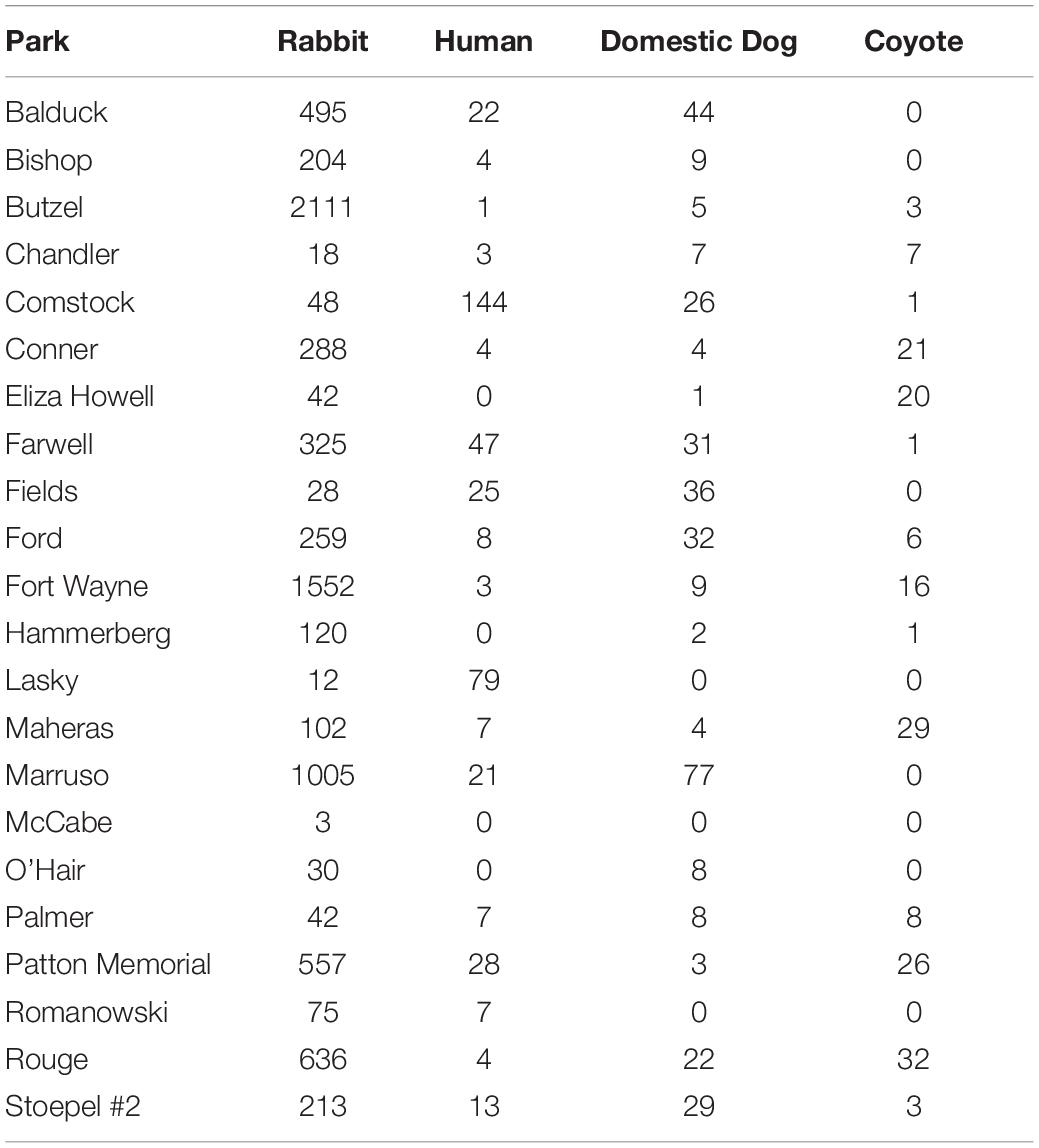
Table 1. Number of detections for cottontail rabbits and associated threats tested that may influence their vigilance behavior in urban parks, Detroit Michigan 2017–2020.
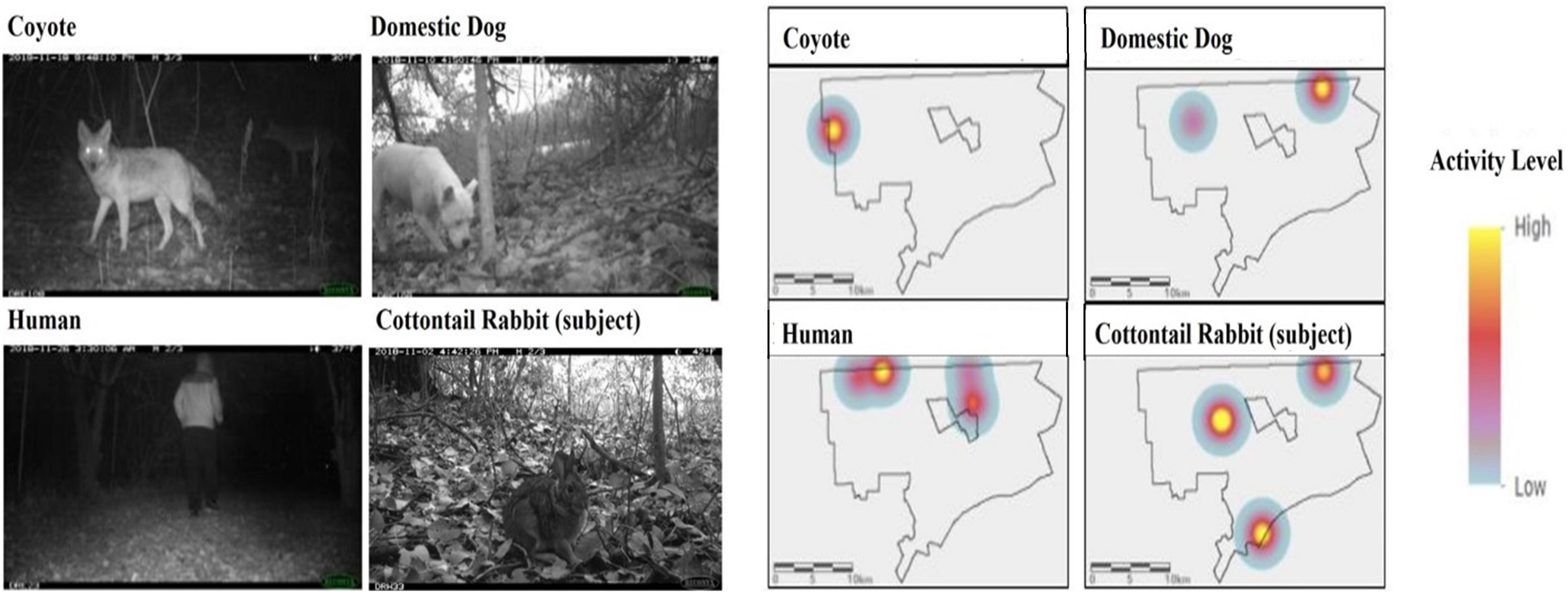
Figure 4. Spatial use within Detroit for rabbits and their three threat species as shown by significant hotspots based on kernel density activity patterns from camera images in the city of Detroit parks from 2017 to 2020.
Top occupancy models for all threats included HOUSE with PREYTS being important for both canid species (Supplementary Table 1). Detection models highlighted SIZE for all threats as important as well as TN, UAC, and CAM for humans and domestic dogs. Although comparable, estimates from top models indicated occupancy was highest for humans and lowest for coyotes throughout Detroit city parks (HUMAN = 0.684 SE = 0.057; DOG = 0.662 SE = 0.058; COYOTE = 0.598 SE = 0.061).
Of the rabbit detections, with vigilance being determined by head position, we categorized 2,774 images as vigilant (i.e., head-up, 34%) and 1,327 images as non-vigilant (i.e., head down, 16.3%). We classified the remaining 4,064 photos into the following categories: 17.4% moving, 1% active, 1.8% sniffing, and 1% eating. Over a quarter of the total images were either unknown or out of frame, with these categories both being removed from analysis.
Models further support differential effects of threats on rabbit vigilance (Table 2). The top model (highest w with Δ AIC < 2) indicated that the presence of domestic dog hotspots (β = 2.63, p = 0.002), coyote occupancy (β = 0.869, p = 0.013), vegetation cover (β = 0.735, p = 0.031) and distance to water (β = 0.0001, p = 0.078) all positively influenced vigilance, when the response variable represented was number of images with rabbits exhibited vigilance behavior. Though park size, roads, and human occupancy are in other top models, none of these variables had significant beta coefficients in explaining rabbit vigilance. Results of top models were consistent when using the extended categories of vigilance to include counts of moving and sniffing. The intercept-only model was included in top models when using ratio of vigilance photos. Therefore, we did not have sufficient power to investigate whether other variables explained the variation in the proportion of vigilant photos.
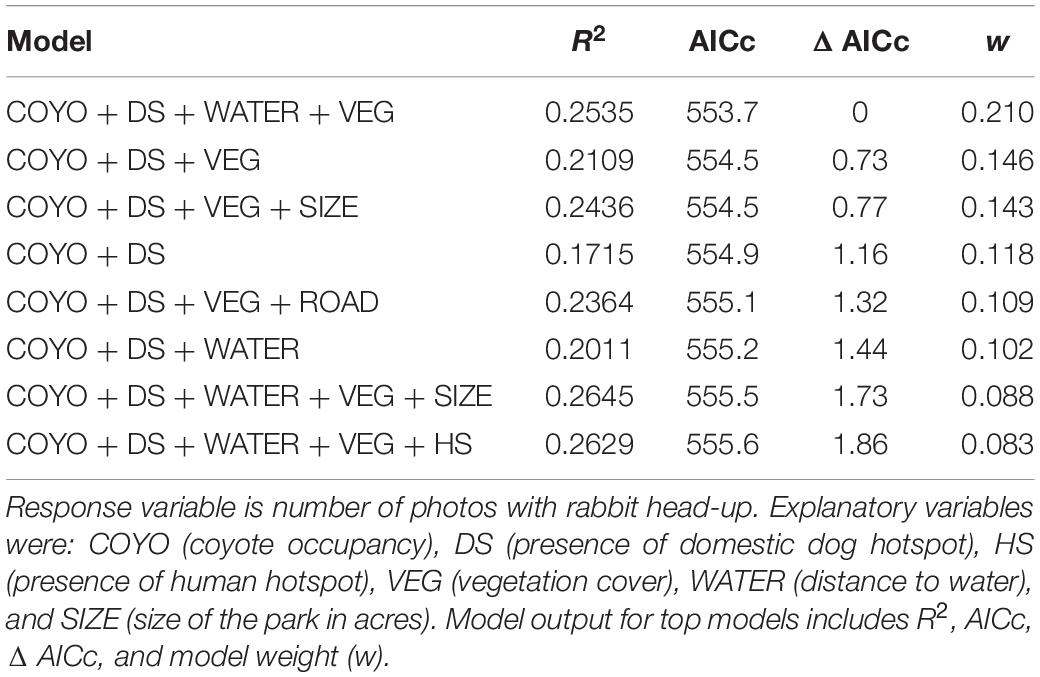
Table 2. Top models (<2 Δ AICc) that explained rabbit vigilance behavior using detection data from camera survey in Detroit city parks, 2017–2020.
Discussion
Urban wildlife must employ various behavioral strategies to cope with risks in their environment from naturogenic and anthropogenic sources (Stillfried et al., 2017; Blecha et al., 2018). Like other urban prey species, cottontail rabbits are facing predation threats that are dynamic in an increasingly urbanized world (Mccleery, 2009; Duarte and Young, 2011). We anticipated a scaled response where rabbits showed the lowest vigilance in areas of high human density, then progressively increased with in areas of high domestic dog density and even more in areas of high coyote density. Our analysis showed that occupancy of coyote positively influenced vigilance, consistent with expectations. We did find that rabbit vigilance behavior was heightened in hotspots of domestic dogs across the city. Further, consistent with our expectation, rabbit vigilance was not significantly affected by human occupancy suggesting more acclimation in a human-dominated landscape. Similarly, Gámez and Harris (in press) found no response of human occupancy on carnivore occupancy throughout Detroit in the same parks we surveyed here to access rabbit vigilance behavior. We also found that rabbit vigilance was significantly higher with more vegetation cover, which could be a response to lower visibility to detect predators.
While it is possible rabbits have acclimated to human presence (Samia et al., 2015; Dunagan et al., 2019), their response to domestic dogs indicates that they continue to perceive them as a threat. Domestic dogs are morphologically similar to coyotes, but occupy much higher densities in urban areas and may represent a novel threat similar enough to a natural predator to induce a stronger vigilance response. Coyotes may not occur above the density threshold required to induce behavioral modifications in rabbits in Detroit. Dogs may have functionally replaced coyotes in this capacity posing greater predation risk to cottontail rabbits. Similarly, vigilance behavior increased in association with domestic dogs, but not coyotes in white-tailed deer (Odocoileus virginianus) in the mid-Atlantic region of the United States (Schuttler et al., 2017). Parsons et al. (2016) found that white-tailed deer and gray squirrel avoided humans with and without dogs more strongly than coyotes throughout the southeastern United States. Their findings were notably in contrast with other studies such as Parker and Nilon (2008) that suggested squirrels habituated to human activity in urban areas.
Ziege et al. (2016) found European rabbits (Oryctolagus cuniculus) were less vigilant in urban areas as compared to their counterparts in rural areas. This suggests that perhaps the important difference in vigilance lies in the urban-rural gradient, rather than entirely within the urban matrix. Similar to rural areas where there is more vegetation cover than urban areas, we found vigilance increased within areas with more vegetation cover. Rabbits occurring in areas with more vegetative cover increased their vigilance, which could indicate fear that the covered environment may obscure predators. In Missouri, Jones et al. (2016) reported that forest cover did not influence rabbit or squirrel occupancy across an urban-rural gradient study. We also found that as rabbits moved further away from water their vigilance level increased in the urban parks we sampled, which could reflect increased exposure to more developed areas in the urban matrix. Urban systems represent a novel landscape for rabbits that requires dynamic changes in vigilance based on the environment and threats of specific locations within the landscape.
Our hotspot analysis indicated very little spatial overlap between species, with domestic dogs and rabbits being the only two species to have significant densities at the same camera location in the same year. As a result, we conclude that generally, rabbits are investing more in spatial avoidance, requiring less effort for vigilance. By mostly avoiding their predators, rabbits may be better able to maintain constant levels of vigilance across the landscape rather than heightening vigilance in areas their predators occupy at significant densities. These hotspots of activity might also be confounded by other factors affecting vigilance that were not incorporated in our models. For example, rabbits might be selecting environments based on proximity to housing, overall vegetation density, or grass cover that might be less desirable for their predators, allowing the rabbits to spend less time being vigilant.
Notably, our analysis was limited in scope by only examining behavior in areas where these species co-occur. It is entirely possible that spatial or temporal partitioning plays a larger role in mediating predator-prey interactions than vigilance solely in prey. We examined interactions within patches in the city, but neglected to examine the amount of interaction occurring between these spaces. Quantifying the level of risks between patches in the city could be the next step in examining threat impacts on prey behavior. Furthermore, seasonality may influence vigilance behavior and interact with food availability (Périquet et al., 2017; Favreau et al., 2018). Our survey did not sample during warmer months. However, one could argue risk assessment in cottontail rabbits may be more extreme in the winter months when predators are more food-limited.
A growing number of studies on prey behavior have shown increasing evidence for multiple factors affecting predator prey dynamics including human influence and urbanization (Magle et al., 2014; Gallo et al., 2019). Our work contributes to the growing number of studies on urban wildlife and particularly predator-prey dynamics within urban systems. Further, we underscore that studying behavioral ecology across city topologies including cities where human populations are declining such as Detroit is necessary for understanding how humans, not just their built environment, affect wildlife to better promote coexistence between humans and wildlife (Guerrieri et al., 2012; Herrmann et al., 2016). Understanding the dynamics of predators and their prey in urban systems will be key to the continued coexistence of wildlife and humans in urban spaces. Our results elucidate how a common prey species changes, or fails to change, their vigilance behavior across anthropogenic and naturogenic risk factors in an urban ecosystem. Ultimately, these findings advance our understanding of the adaptability of wildlife in human-dominated environments.
Data Availability Statement
The raw data supporting the conclusions of this article will be made available by the authors, without undue reservation.
Author Contributions
SL and NH conceived the study. SL wrote the manuscript and conducted analysis with support from SG and NH. SL and NA designed the graphics. NH secured funding and supervised work. All authors contributed to field efforts, data curation, and editing the manuscript.
Funding
This work was supported by Detroit Zoological Society.
Conflict of Interest
The authors declare that the research was conducted in the absence of any commercial or financial relationships that could be construed as a potential conflict of interest.
Acknowledgments
First, we recognize implementing our field research with camera traps was conducted on lands originally belonging to the People of the Three Fires indigenous tribal community. We sincerely thank members past and present of the Applied Wildlife Ecology (AWE) Lab at the University of Michigan, specifically R. Malhotra, S. Bower, and G. Gadsden who contributed to the data collection, image sorting, and logistical support of this project. We also thank the University of Michigan Undergraduate Research Opportunity Program and the honors committee (P. Tucker and A. Ostling) for support of S. Lima during her B.S. degree. Finally, we extend our appreciation to our partners at the Detroit Zoological Society for their financial support and the City of Detroit for collaboration, permits, and access to the parks in our study.
Supplementary Material
The Supplementary Material for this article can be found online at: https://www.frontiersin.org/articles/10.3389/fevo.2021.570734/full#supplementary-material
References
Baker, M. A. A., Emerson, S. E., and Brown, J. S. (2015). Foraging and habitat use of eastern cottontails (Sylvilagus floridanus) in an urban landscape. Urban Ecosystems 18, 977–987. doi: 10.1007/s11252-015-0463-7
Bateman, P. W., and Fleming, P. A. (2012). Big city life: carnivores in urban environments. J. Zool. 287, 1–23. doi: 10.1111/j.1469-7998.2011.00887.x
Bateman, P. W., and Fleming, P. A. (2014). Does human pedestrian behaviour influence risk assessment in a successful mammal urban adapter? J. Zool. 294, 93–98. doi: 10.1111/jzo.12156
Beasom, S. L., and Moore, R. A. (1977). Bobcat food habit response to a change in prey abundance. Southwestern Nat. 21, 451–457.
Blecha, K. A., Boone, R. B., and Alldredge, M. W. (2018). Hunger mediates apex predator’s risk avoidance response in wildland–urban interface. J. Anim. Ecol. 87, 609–622. doi: 10.1111/1365-2656.12801
Bowen, W. D., and Cowan, I. M. (1980). Scent marking in coyotes. Can. J. Zool. 58, 473–480. doi: 10.1139/z80-065
Bruskotter, J. T., Vucetich, J. A., Manfredo, M. J., Karns, G. R., Wolf, C., Ard, K., et al. (2017). Modernization, risk, and conservation of the world’s largest carnivores. BioScience 67, 646–655. doi: 10.1093/biosci/bix049
Chambers, L. K., and Dickman, C. R. (2002). Habitat selection of the long-nosed bandicoot, Perameles nasuta (Mammalia, Peramelidae), in a patchy urban environment. Austral Ecol. 27, 334–342. doi: 10.1046/j.1442-9993.2002.t01-1-01185.x
Chen, G., Li, X., Liu, X., Chen, Y., Liang, X., Leng, J., et al. (2020). Global projections of future urban land expansion under shared socioeconomic pathways. Nat. Commun. 11:537.
Ciuti, S., Northrup, J. M., Muhly, T. B., Simi, S., Musiani, M., Pitt, J. A., et al. (2012). Effects of humans on behaviour of wildlife exceed those of natural predators in a landscape of fear. PLoS One 7:e50611. doi: 10.1371/journal.pone.0050611
Clinchy, M., Zanette, L. Y., Roberts, D., Suraci, J. P., Buesching, C. D., Newman, C., et al. (2016). Fear of the human “super predator” far exceeds the fear of large carnivores in a model mesocarnivore. Behav. Ecol. 27, 1826–1832.
Crooks, K. R., and Soulé, M. E. (1999). Mesopredator release and avifaunal extinctions in a fragmented system. Nature 400, 563–566. doi: 10.1038/23028
Crooks, K. R., Suarez, A. V., and Bolger, D. T. (2004). Avian assemblages along a gradient of urbanization in a highly fragmented landscape. Biol. Conserv. 115, 451–462. doi: 10.1016/s0006-3207(03)00162-9
Dowding, C. V., Harris, S., Poulton, S., and Baker, P. J. (2010). Nocturnal ranging behaviour of urban hedgehogs, Erinaceus europaeus, in relation to risk and reward. Anim. Behav. 80, 13–21. doi: 10.1016/j.anbehav.2010.04.007
Duarte, M. H., and Young, R. J. (2011). Sleeping site selection by urban marmosets (Callithrix penicillata) under conditions of exceptionally high predator density. Int. J. Primatol. 32, 329–334. doi: 10.1007/s10764-010-9468-5
Dunagan, S. P., Karels, T. J., Moriarty, J. G., Brown, J. L., and Riley, S. P. D. (2019). Bobcat and rabbit habitat use in an urban landscape. J. Mammol. 100, 401–409. doi: 10.1093/jmammal/gyz062
Favreau, F., Goldizen, A. W., Fritz, H., and Pays, O. (2018). Food supply fluctuations constrain group sizes of kangaroos and in turn shape their vigilance and feeding strategies. Anim. Behav. 135, 165–176. doi: 10.1016/j.anbehav.2017.11.020
Fenn, M. G., and Macdonald, D. W. (1995). Use of middens by red foxes: risk reverses rhythms of rats. J. Mammal. 76, 130–136. doi: 10.2307/1382321
Foley, J. A., DeFries, R., Asner, G. P., Barford, C., Bonan, G., et al. (2005). Global consequences of land use. Science 309, 570–574. doi: 10.1126/science.1111772
Gallo, T., Fidino, M., Lehrer, E. W., and Magle, S. (2019). Urbanization alters predator-avoidance behaviours. J. Anim. Ecol. 88, 793–803. doi: 10.1111/1365-2656.12967
Gámez, S., and Harris, N. C. (in press). Living in the concrete jungle:(carnivore)spatial ecology in urban parks. Ecol. Appl.
Gaynor, K. M., Hojnowski, C. E., Carter, N. H., and Brashares, J. S. (2018). The influence of human disturbance on wildlife nocturnality. Science 360, 1232–1235. doi: 10.1126/science.aar7121
Gese, E. M., and Beckoff, M. (2004). “Coyote Canis Latrans,” in Canids: Foxes, Wolves, Jackals, and Dogs: Status Survey and Conservation Action Plan, eds C. Sillero-Zubiri, M. Hoffmann, and D. W. Macdonald (Gland: IUCN).
Gliwicz, J., and Dąbrowski, M. J. (2008). Ecological factors affecting the diel activity of voles in a multi-species community. Annal. Zool. Fennici 45, 242–247. doi: 10.5735/086.045.0401
Grimm, N. B., Foster, D., Groffman, P., Grove, J. M., Hopkinson, C. S., et al. (2008). The changing landscape: ecosystem responses to urbanization and pollution across climatic and societal gradients. Front. Ecol. Environ. 6:264–272. doi: 10.1890/070147
Guerrieri, V., Hartley, D., and Hurst, E. (2012). Within-City variation in urban decline: the case of detroit. Am. Econom. Rev. 102, 120–126. doi: 10.1257/aer.102.3.120
Herrmann, D. L., Schwarz, K., Shuster, W. D., Berland, A., Chaffin, B. C., Garmestani, A. S., et al. (2016). Ecology for the Shrinking City. Bioscience 66, 965–973.
Hody, J. W., and Kays, R. (2018). Mapping the expansion of coyotes (Canis latrans) across North and Central America. ZooKeys 759, 81–97. doi: 10.3897/zookeys.759.15149
Hunt, V. M., Magle, S. B., Vargas, C., Brown, A. W., Lonsdorf, E. V., et al. (2014). Survival, abundance, and capture rate of eastern cottontail rabbits in an urban park. Urban Ecosystems 17, 547–560. doi: 10.1007/s11252-013-0334-z
Ikeda, T., Asano, M., Matoba, Y., and Abe, G. (2004). Present status of invasive alien raccoon and its impact in Japan. Global Environ. Res. 8, 125–131.
Johnson, M. T., and Munshi-South, J. (2017). Evolution of life in urban environments. Science 358:eaam8327. doi: 10.1126/science.aam8327
Jones, B. M., Cove, M. V., Lashley, M. A., and Jackson, V. L. (2016). Do coyotes Canis latrans influence occupancy of prey in suburban forest fragments? Curr. Zool. 62, 1–6. doi: 10.1093/cz/zov004
Kitchen, A. M., Gese, E. M., and Schauster, E. R. (2000). Changes in coyote activity patterns due to reduced exposure to human persecution. Can. J. Zool. 78, 853–857. doi: 10.1139/z00-003
Lima, S. L. (1998). Nonlethal effects in the ecology of predator-prey interactions. Bioscience 48, 25–34. doi: 10.2307/1313225
Litvaitis, J. A., and Shaw, J. H. (1980). Coyote movements, habitat use, and food habits in southwestern Oklahoma. J. Wildlife Manag. 44, 62–68. doi: 10.2307/3808351
Lowry, H., Lill, A., and Wong, B. B. (2013). Behavioural responses of wildlife to urban environments. Biol. Rev. 88, 537–549. doi: 10.1111/brv.12012
MacKenzie, D. I., Nichols, J. D., Hines, J. E., Knutson, M. G., and Franklin, A. B. (2003). Estimating site occupancy, colonization, and local extinction when a species is detected imperfectly. Ecology 84, 2200–2207. doi: 10.1890/02-3090
MacKenzie, D. I., and Royle, J. A. (2005). Designing occupancy studies: general advice and allocating survey effort. J. Appl. Ecol. 42, 1105–1114. doi: 10.1111/j.1365-2664.2005.01098.x
Magle, S. B., Simoni, L. S., Lehrer, E. W., and Brown, J. S. (2014). Urban predator–prey association: coyote and deer distributions in the Chicago metropolitan area. Urban Ecosystems 17, 875–891. doi: 10.1007/s11252-014-0389-5
Mccleery, R. A. (2009). Changes in fox squirrel anti-predator behaviors across the urban–rural gradient. Landscape Ecol. 24, 483–493. doi: 10.1007/s10980-009-9323-2
Mech, L. D. (1995). The challenge and opportunity of recovering wolf populations. Conserv. Biol. 9, 270–278. doi: 10.1046/j.1523-1739.1995.9020270.x
Muhly, T. B., and Musiani, M. (2009). Livestock depredation by wolves and the ranching economy in the Northwestern US. Ecol. Econom. 68, 2439–2450. doi: 10.1016/j.ecolecon.2009.04.008
Muhly, T. B., Semeniuk, C., Massolo, A., Hickman, L., and Musiani, M. (2011). Human activity helps prey win the predator-prey space race. PLoS One 6:e17050. doi: 10.1371/journal.pone.0017050
Ordeñana, M. A., Crooks, K. R., Boydston, E. E., Fisher, R. N., Lyren, L. M., et al. (2010). Effects of urbanization on carnivore species distribution and richness. J. Mammol. 91, 1322–1331. doi: 10.1644/09-mamm-a-312.1
Parker, T. S., and Nilon, C. H. (2008). Gray squirrel density, habitat suitability, and behavior in urban parks. Urban Ecosystems 11, 243–255. doi: 10.1007/s11252-008-0060-0
Parsons, A. W., Bland, C., Forrester, T., Baker-Whatton, M. C., Schuttler, S. G., McShea, W. J., et al. (2016). The ecological impact of humans and dogs on wildlife in protected areas in eastern North America. Biol. Conserv. 203, 75–88. doi: 10.1016/j.biocon.2016.09.001
Périquet, S., Richardson, P., Cameron, E. Z., Ganswindt, A., Belton, L., Loubser, E., et al. (2017). Effects of lions on behaviour and endocrine stress in plains zebras. Ethology 123, 667–674. doi: 10.1111/eth.12638
Pickard, B. R., Van Berkel, D., Petrasova, A., and Meentemeyer, R. K. (2017). Forecasts of urbanization scenarios reveal trade-offs between landscape change and ecosystem services. Landscape Ecol. 32, 617–634. doi: 10.1007/s10980-016-0465-8
Poessel, S. A., Mock, E. C., and Breck, S. W. (2017). Coyote (Canis latrans) diet in an urban environment: variation relative to pet conflicts, housing density, and season. Can. J. Zool. 95, 287–297. doi: 10.1139/cjz-2016-0029
Quenette, P. Y. (1990). Functions of vigilance behaviour in mammals: a review. Acta Oecol. 11, 801–818.
Reilly, M. L., Tobler, M. W., Sonderegger, D. L., and Beier, P. (2017). Spatial and temporal response of wildlife to recreational activities in the San Francisco Bay ecoregion. Biol. Conserv. 207, 117–126. doi: 10.1016/j.biocon.2016.11.003
Romano, B. (2002). “Evaluation of urban fragmentation in the ecosystems,” in Proceedings of International Conference on Mountain Environment and Development (ICMED), (Chengdu), 15–19.
Samia, D. S. M., Nakagawa, S., Nomura, F., Rangel, T. F., and Blumstein, D. T. (2015). Increased tolerance to humans among disturbed wildlife. Nat. Commun. 6:8877.
Scheel, D. (1993). Watching for lions in the grass: the usefulness of scanning and its effects during hunts. Anim. Behav. 46, 695–704. doi: 10.1006/anbe.1993.1246
Schuttler, S. G., Parsons, A. W., Forrester, T. D., Baker, M. C., McShea, W. J., Costello, R., et al. (2017). Deer on the lookout: how hunting, hiking and coyotes affect white-tailed deer vigilance. J. Zool. 301, 320–327. doi: 10.1111/jzo.12416
Stillfried, M., Gras, P., Börner, K., Göritz, F., Painer, J., et al. (2017). Secrets of success in a landscape of fear: urban wild boar adjust risk perception and tolerate disturbance. Front. Ecol. Evol. 5:157. doi: 10.3389/fevo.2017.00157
Tchabovsky, A. V., Krasnov, B., Khokhlova, I. S., and Shenbrot, G. I. (2001). The effect of vegetation cover on vigilance and foraging tactics in the fat sand rat Psammomys obesus. J. Ethol. 19, 105–113. doi: 10.1007/s101640170006
Tigas, L. A., Van Vuren, D. H., and Sauvajot, R. M. (2002). Behavioral responses of bobcats and coyotes to habitat fragmentation and corridors in an urban environment. Biol. Conserv. 108, 299–306. doi: 10.1016/s0006-3207(02)00120-9
Treves, A., and Karanth, K. U. (2003). Human-carnivore conflict and perspectives on carnivore management worldwide. Conserv. Biol. 17, 1491–1499. doi: 10.1111/j.1523-1739.2003.00059.x
United Nations (2018). World Urbanization Prospects - Population Division. New York, NY: United Nations.
U.S. Census Bureau (2016). Decennial Census of Population and Housing. Suitland, MD: U.S. Census Bureau.
Wilkinson, D., and Smith, G. C. (2001). A preliminary survey for changes in urban fox (Vulpes vulpes) densities in England and Wales, and implications for rabies control. Mammal Rev. 31, 107–110. doi: 10.1046/j.1365-2907.2001.00076.x
Witmer, G. W., and Whittaker, D. G. (2001). Dealing with Nuisance and Depredating Black Bears. Londonderry: USDA National Wildlife Research Center-Staff Publications, 581.
Wittenberg, R. D. (2012). Foraging ecology of the timber rattlesnake (Crotalus horridus) in a fragmented agricultural landscape. Herpetol. Conserv. Biol. 7, 449–461.
Keywords: camera survey, domestic dog, anti-predator, cottontail rabbit, coyote, urban, occupancy
Citation: Lima SL, Gámez S, Arringdale N and Harris NC (2021) Vigilance Response of a Key Prey Species to Anthropogenic and Natural Threats in Detroit. Front. Ecol. Evol. 9:570734. doi: 10.3389/fevo.2021.570734
Received: 08 June 2020; Accepted: 04 January 2021;
Published: 25 January 2021.
Edited by:
David Andrew Luther, George Mason University, United StatesReviewed by:
Seth Magle, Lincoln Park Zoo, United StatesMicaela Gunther, Humboldt State University, United States
Copyright © 2021 Lima, Gámez, Arringdale and Harris. This is an open-access article distributed under the terms of the Creative Commons Attribution License (CC BY). The use, distribution or reproduction in other forums is permitted, provided the original author(s) and the copyright owner(s) are credited and that the original publication in this journal is cited, in accordance with accepted academic practice. No use, distribution or reproduction is permitted which does not comply with these terms.
*Correspondence: Nyeema C. Harris, nyeema@umich.edu; orcid.org/0000-0001-5174-2205