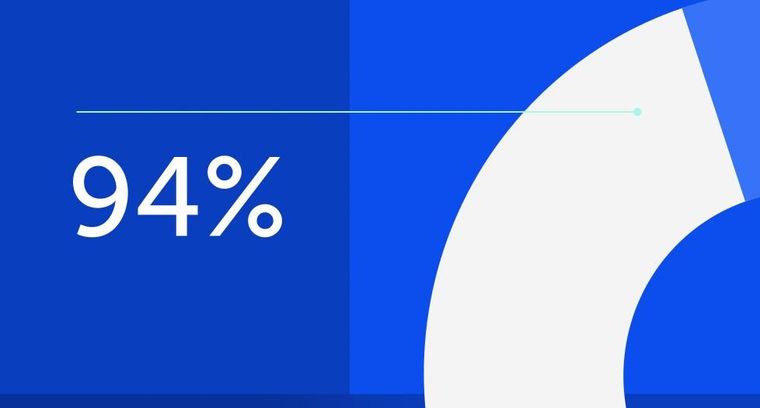
94% of researchers rate our articles as excellent or good
Learn more about the work of our research integrity team to safeguard the quality of each article we publish.
Find out more
ORIGINAL RESEARCH article
Front. Ecol. Evol., 26 January 2021
Sec. Evolutionary Developmental Biology
Volume 8 - 2020 | https://doi.org/10.3389/fevo.2020.618007
Evolution and diversification of cell types has contributed to animal evolution. However, gene regulatory mechanisms underlying cell fate acquisition during development remains largely uncharacterized in spiralians. Here we use a whole-organism, single-cell transcriptomic approach to map larval cell types in the annelid Capitella teleta at 24- and 48-h post gastrulation (stages 4 and 5). We identified eight unique cell clusters (undifferentiated precursors, ectoderm, muscle, ciliary-band, gut, neurons, neurosecretory cells, and protonephridia), thus helping to identify uncharacterized molecular signatures such as previously unknown neurosecretory cell markers in C. teleta. Analysis of coregulatory programs in individual clusters revealed gene interactions that can be used for comparisons of cell types across taxa. We examined the neural and neurosecretory clusters more deeply and characterized a differentiation trajectory starting from dividing precursors to neurons using Monocle3 and velocyto. Pseudotime analysis along this trajectory identified temporally-distinct cell states undergoing progressive gene expression changes over time. Our data revealed two potentially distinct neural differentiation trajectories including an early trajectory for brain neurosecretory cells. This work provides a valuable resource for future functional investigations to better understanding neurogenesis and the transitions from neural precursors to neurons in an annelid.
Proper development of multicellular organisms relies on precise regulation of the cell cycle relative to establishment of cell lineages and cell fate decisions, e.g., the maintenance of proliferating cells vs. the onset of differentiation. In general, many embryonic and postembryonic tissues are generated by stem cells that give rise to multipotent precursor cells whose daughters differentiate into tissue-specific, specialized cell types. Cell fate acquisition and differentiation are directly regulated by changes in transcriptional gene regulation. Therefore, understanding the underlying transcriptional dynamics is of utmost importance to understand developmental processes. Furthermore, alterations in gene regulatory networks (GRNs) may have driven diversification of cell types during animal evolution. According to Arendt et al. (2016), cell types are evolutionary units that can undergo evolutionary change. Therefore, to identify related cell types across taxa, it is necessary to compare genomic information, such as shared gene expression profiles or shared enhancers across individual cells from specific developmental regions and stages.
More recently, single-cell RNA sequencing (scRNAseq) has emerged as a powerful technique to understand the genome-wide transcriptomic landscapes of different cell types (Tang et al., 2010; Hashimshony et al., 2012; Saliba et al., 2014; Trapnell et al., 2014; Achim et al., 2015, 2018; Satija et al., 2015; Vergara et al., 2017; Svensson et al., 2018; Zhong et al., 2018). scRNAseq enables massively parallel sequencing of transcriptomic libraries prepared from thousands of individual cells and allows for in silico identification and characterization of distinct cell populations (Trapnell, 2015; Tanay and Regev, 2017). It can therefore provide information regarding the various cell types that emerge during developmental processes (e.g., neurogenesis) and elucidate how the transcriptomic landscape changes within stem cells and their progeny as development progresses. As scRNAseq analysis algorithms allow for a priori identification of individual cells within a population, one can process heterogenous cell populations and unravel the transcriptomic signatures underlying such heterogeneity. This allows for discovery of novel cell types and resolution of the transcriptional changes throughout a single cell type's developmental journey. Emergence of this technology has therefore made it possible to predict molecular trajectories that underlie cell fate specification by sampling across a large number of cells during development and connecting transcriptomes of cells that have similar gene expression profiles (Farrell et al., 2018). Such approaches have recently gained prominence in evolutionary developmental biology and are being used to understand evolutionary relationships between cell types across taxa. This has paved the way to systemic molecular characterization of cell types and developmental regulatory mechanisms in understudied metazoan lineages.
Although whole-organism scRNAseq approaches have been used to unravel cell type repertoires in animal clades outside and across Bilateria (Achim et al., 2018; Farrell et al., 2018; Plass et al., 2018; Sebe-Pedros et al., 2018a,b; Foster et al., 2020), there has been limited systemic information regarding cell type diversity and regulatory mechanisms underlying differentiation trajectories in the third major clade Spiralia (≈Lophotrochozoa) (Marletaz et al., 2019). Whole-body scRNAseq has been performed on a few spiralians such as the planarian Schmidtea mediterranea (Cao et al., 2017; Plass et al., 2018) and the annelid Platynereis dumerilii (Achim et al., 2015, 2018). In S. mediterranea, different classes of neoblasts and various differentiation trajectories emanating from a central neoblast population were detected using whole-body scRNAseq (Plass et al., 2018). In P. dumerilii larvae, whole-body scRNAseq yielded five differentiated states—anterior neural domain, gut, ciliary-bands, an unknown cell population and muscles (Achim et al., 2018). Similar transcriptomic information from other spiralian taxa can provide insight into conserved cell types and their evolution.
In this manuscript, we used scRNAseq to characterize larval cell types at 24- and 48-h post gastrulation in the annelid Capitella teleta (Blake et al., 2009) (Figure 1), highlighting potential genetic regulatory modules and differentiation trajectories underlying different cell types. We (i) classified the captured cells into several molecular domains, (ii) predicted lineage relationships between neural cells in an unbiased manner, and (iii) identified neurogenic gene regulatory modules comprising genes that are likely involved in programming neural lineages. We compared larval cell types identified in this study with those in P. dumerilii at roughly similar stages during development. This study provides a valuable resource of transcriptionally distinct cell types during C. teleta larval development and illuminates the use of scRNAseq approaches for understanding molecular mechanisms of larval development in other previously understudied invertebrates.
Figure 1. (A) Single-cell transcriptomics of stage 4 and 5 Capitella teleta larvae. Whole-body stage 4 and 5 (1) larvae were dissociated into single cells using a combination of mechanical and enzymatic dissociation. In the diagrams in (1), colors represent the anterior (red) and the ventral (blue) nervous system anlagen. Image showing cell suspension dissociated using 1% Trypsin (~96.5% viability) resuspended in cell-media post filtering and centrifugation, 45 min after dissociation. Red – Hoechst+ nuclei (2). Individual cells were randomly selected for droplet generation using the 10X Genomics Chromium Platform (3). Single-cell transcriptomes were pooled and sequenced using NextSeq500 High-output method (4) generating 573 million reads across the two samples. Sequences obtained were curated and aligned to the C. teleta genome v1.0 followed by application of downstream computational pipelines for clustering, trajectory analysis, pseudotime analysis and estimating RNA dynamics (5). (B) Table showing the different proteolytic enzymes tested across different concentrations for optimizing C. teleta cell dissociation protocols using stage 4 larvae. The first two columns indicate the enzymes and the respective concentrations used. The third column shows the number of biological replicates for each enzyme conducted, the fourth column indicates the total number of cells (± S.E.M) quantified post-dissociation from 300 stage 4 larvae, the fifth column indicates the percentage of cells recovered per stage 4 larvae (± S.E.M) and the sixth column shows the proportion of healthy cells (± S.D.) quantified following a Trypan Blue exclusion test 1 h post-dissociation using the respective enzymes. (C) Table showing the number of cells used for dissociation to sequencing and bioinformatic analysis. Scale bar – 10 μm.
For the data reported here, cell dissociation and scRNAseq using the 10X genomics platform was performed at the Single-cell Sequencing Core at Boston University, Boston, Massachusetts. We tried replicating the 10X experiment at the Bauer Sequencing Core, Harvard University; however, that run did not yield enough RNA for amplification and sequencing.
Total number of cells in C. teleta larvae at stages 4 and 5 (Figure 1A; 1) were estimated by counting Hoechst-labeled nuclei in the episphere at stages 4–5 (Supplementary Figures 1A,B) and from previously collected cells counts in the trunk at stages 4 and 5 (Sur et al., 2020). At both stages, the episphere was divided into 10 μm thick z-stacks and Hoechst+ nuclei were counted in each z-stack using the Cell Counter plugin in Fiji. At stage 4, Hoechst+ nuclei in the unsegmented trunk were counted within the presumptive neuroectoderm using a strategy previously described. Once the trunk neuroectoderm becomes segmented by stage 5, Hoechst+ nuclei were counted in segments 2–4 and 5–7 within specific region of interests (Sur et al., 2020). We also optimized cell-dissociation protocols in C. teleta (final protocol detailed below). Based on cell-dissociation trials using three different proteolytic enzymes (papain, trypsin and pronase), we found 1% papain yielded the highest number of dissociated cells but also led to a lower proportion of viable cells (Figure 1B). Cell counts were estimated after mechanical and proteolytic digestion, size-exclusion of >40 μm cells or cell-clumps, and three washes in artificial seawater or cell-media (see below). Papain does not readily dissolve in seawater and hence needs to be resuspended in dimethylformamide or dimethyl sulfoxide, which may have an adverse effect on the viability of the dissociated cells. Cell dissociation using 1% Trypsin yielded the greatest number of viable cells and was used to dissociate C. teleta cells for scRNAseq (Figure 1B).
Next, for collecting high-quality starting material for scRNAseq, healthy males, and females were mated under controlled conditions and their offspring collected at the gastrula stage (stage 3) (Seaver et al., 2005; Sur et al., 2017). Stage 4 and stage 5 larvae were collected from two different sets of parents, each from a single mother. Capitella teleta embryos and larvae were incubated in artificial seawater (ASW; 32–34 ppt) with 50 ug/mL penicillin and 60 ug/mL streptomycin (PenStrep) (SigmaAldrich) at 19°C for 1–2 days until they reached stage 4 prototroch or stage 5 (Figure 1A; 1). For single-cell dissociation, 300 larvae from a single brood (i.e., from one male and female) for each stage were then collected into 1.5 mL centrifuge tubes and equilibrated in Ca2+/Mg2+-free ASW (CMFSW). The recipe for the CMFSW used in this study is a modification from previously published recipes (Strathmann, 1987). To prepare CMFSW, 22.5 mL 2.0 M NaCl, 10 mL 0.33 M Na2SO4, 1.8 mL 0.5 M KCl, 0.5 mL 0.5 M NaHCO3 and 1.0 mL 1 M Tris + 0.25 M EGTA (pH = 8.0) were added to a plastic bottle as glass supposedly leaches calcium into the solution, and the volume was adjusted to 50 mL using RO water. Once the larvae were equilibrated in CMFSW, most of the liquid was removed from the tubes, and larvae were mechanically homogenized using separate, clean and sterile pestles as well as a hand-held homogenizer (Cole Parmer, LabGEN 7B) for 5–10 s. Homogenized larvae from each stage were then incubated in 1% Trypsin (SigmaAldrich, Cat# T4799-5G) in CMFSW for 30 min at room temperature with constant rocking. During incubation, dissociated tissues were periodically triturated using both wide-mouthed and narrow-mouthed Pasteur pipettes. After 30 min of incubation, the tissue lysate was passed through a 40 μm nylon cell-strainer (Fisherbrand, Cat# 22-363-547) to remove undissociated clusters of cells and large cells (i.e., yolky, endodermal midgut cells) since anything larger than 30 μm may not be captured efficiently during 10X encapsulation. The resultant cell-suspension was then centrifuged at 1,100 × g for 7 min with slow-braking and washed twice in cell media that was developed originally for marine hemichordate cell-cultures (3.3X Dulbecco's (Ca2+/Mg2+-free) PBS (SigmaAldrich, Cat# 59331C) and 20 mM HEPES, pH = 7.4; Paul Bump, Lowe lab, personal communication). The Ca2+/Mg2+-free cell-media was used as an alternative to ASW during 10X encapsulation as the salts (e.g., Mg2+) present in ASW inhibit the downstream reverse-transcription reaction in the 10X workflow, according to the 10X manufacturer's protocol. Furthermore, Ca2+/Mg2+-free seawater and buffers were used for the cell-dissociation steps as C. teleta cells often reaggregate in PBS or ASW. Dissociated cells were then resuspended in the cell media and checked under an inverted phase-contrast microscope to ensure a single-cell suspension was obtained (Figure 1A; 2).
After cell dissociation and obtaining a single-cell suspension, we estimated cell viability around 1-h post dissociation. Cells were counted using a Neubauer hemocytometer, and survivability was assayed using a Trypan blue exclusion test. 0.4% Trypan blue (SigmaAldrich, Cat#T8154) was added to dissociated cells at a 1:1 ratio in a 0.5 mL centrifuge tube and the mixture was mixed well by gentle pipetting. After allowing the mixture to rest at r.t. for 3–5 min, 10 μL of the mixture was loaded onto a Neubauer hemocytometer and live cells that excluded the dye were counted. Cells were observed under the 20X objective of a Zeiss AxioObserver-5 inverted microscope following Trypan-blue staining available at the Boston University Single-Cell Sequencing Core to ensure cell viability prior to droplet generation. Previous practice dissociations of C. teleta larvae and visual inspection using a Zeiss M2 microscope at 40X resolution revealed dissociated cells ranging from 2 to 12 μm in diameter (Figure 1A; 2). Due to the small size of C. teleta cells and the unavailability of a high-resolution microscope, the total number of cells dissociated in the cell-suspension could not be quantified confidently at Boston University. Therefore, based on cell counts using the Zeiss AxioObserver-5 inverted microscope under the 20X objective, cells were diluted to a target of 400 cells/μL. However, due to our inability to accurately quantify cells and based on results from previous dissociation trials and total number of cells estimated per stage 4 and 5 larvae (Figure 1C and Supplementary Figures 1A,B), our final cell suspension may have contained in the range of ~4,000 cells/μL for stage 4 and ~2,000 cells/μL for stage 5. A total of 15 μL of the resuspended cell-suspension was used for droplet generation estimating a 67% efficiency in droplet capture as per 10X genomics standard guidelines.
Capitella teleta larval cells were captured in droplets and run on the 10X genomics scRNAseq platform at the Boston University Single Cell Sequencing Core following the manufacturer's instructions (Single Cell 3' v3 kit) (Figure 1A; 3). The cDNA library and final library after index preparation were checked with bioanalyzer (High Sensitivity DNA reagents, Agilent Technology #5067-4626; Agilent 2100 Bioanalyzer) for quality control (Supplementary Figure 1C). Following library preparation, sequencing was performed with paired-end sequencing of 150 bp each end on four lanes of NextSeq500 per sample using the Illumina NextSeq500 High-Output v2 kit generating ~573 million reads in total (Figure 1A; 4).
To rectify our inability to control the number of cells input in the first trial, we repeated the cell dissociation and cell-capture procedures at the Bauer Sequencing Core, Harvard University. In this trial, we carefully counted and diluted cells to 400 cells/μL under a Zeiss M2 microscope using a 40X objective and loaded 15 μL of the resuspended cell-suspension aiming to capture ~4,000 cells per stage estimating a 67% efficiency in droplet capture as per 10X genomics standard guidelines. However, in this second trial, ~4,000 captured cells did not generate sufficient cDNA yield following whole transcriptome amplification to enable library preparation for sequencing (Supplementary Figure 1D). This indicates that our first 10X genomics trial at Boston University probably represents the best quality output possible using the 10X genomics scRNAseq platform on Capitella teleta larval cells.
Transcriptome sequencing analysis and read mapping were performed using CellRanger 2.1.0 according to the manufacturer's guidelines. Reads were mapped onto the Capitella teleta genome v1.0 obtained from gene-models deposited at GenBank (GCA_000328365.1_Capca1_genomic.fasta; https://www.ncbi.nlm.nih.gov/assembly/GCA_000328365.1/) and Ensembl (Capitella_teleta.Capitella_teleta_v1.0.dna_sm.toplevel.fa; https://www.ebi.ac.uk/ena/browser/view/GCA_000328365.1) using standard CellRanger parameters. The gene annotation files (.gff) files were downloaded from the respective genome databases. However, as CellRanger cannot read .gff files, each .gff file was converted into .gtf files using the gffread command from the cufflinks package (http://cole-trapnell-lab.github.io/cufflinks/file_formats/). Read mapping to the Capitella teleta genome v1.0 was visualized using the IGV 2.8.0 viewer. Mapping of the sequence reads to both Ensembl and GenBank sequences yielded similar results. CellRanger generated a Digital Gene Expression (DGE) matrix with genes as rows and cells as columns where paired-end reads, one containing the cellular and molecular barcodes (Unique molecular identifiers, UMIs) and the other containing the captured RNA fragment, were joined together in a .bam file and sorted using samtools. Reads already tagged with the cell and molecular barcodes (UMIs) were further trimmed at the 5′ end to remove Illumina-specific sequencing adapter sequences and at the 3′ end to remove poly-A tails using CellRanger default parameters.
To annotate the genes from the two versions of the C. teleta genome (Simakov et al., 2013), reciprocal BLAST comparison of individual gene sequences against the Swiss-Prot database was performed. For each transcript, the BLAST hit with the highest E-value was selected for annotation. The translated reference transcriptome along with the C. teleta gene-models were scanned using the HMMER suite 3.3 program hmmscan using default settings. Using HMMER and Pfam v31.0 database, protein domains in the C. teleta transcriptome were identified (Finn et al., 2016).
DGE matrices were analyzed using the R package Seurat 3.1.4 (Satija et al., 2015). Because of our inability to control the number of cells that were used for droplet generation, and to understand cell-state specific gene and UMI metrics, we performed an initial cluster analysis using less stringent gene and UMI cutoffs. Initially, gene per cell cutoffs between >200 and <3,000 and UMI per cell cutoffs of >200 and <4,000 were set. Genes that were expressed in at least three cells were kept and cells that had more than 5% mitochondrial reads were excluded. High mitochondrial content may indicate that a cell was stressed or dying (Galluzzi et al., 2012). However, using such cutoffs, not enough unique cell clusters were detected. This may be because particular cell-doublet categories were not excluded in the cut-off selection. Therefore, this preliminary analysis led us to increase the gene per cell cutoffs to >300 and <2,500 in order to prevent the inclusion of cell-doublets in our analysis.
We refined our gene/UMI cutoffs, and only genes that were expressed in at least three cells with a minimum of 300 genes were included in the analysis. Moreover, we also discarded cells with more than 2,500 genes in sequences obtained from both samples in order to screen out cell-doublets. A total of 9,487 genes across 1,072 cells for stage 4 and 13,403 genes across 1,785 cells for stage 5 from one 10X genomics experiment were included in the final analysis. This accounts for around ~4 cells per stage 4 larva dissociated and ~6 cells per stage 5 larva dissociated that were bioinformatically recovered from initially loaded ~200 cells per larva for stage 4 and ~100 cells per larva loaded for stage 5. We only used 3.1% of Cell Ranger predicted captured cells for stage 4 and 10.86% of predicted captured cells at stage 5 for downstream analysis. UMI counts per gene in individual cells were normalized to the total UMI count of each cell using the “LogNormalize” function with a scale factor of 10,000 (Supplementary Figure 2). Clustering analysis of the cells was done using the top 2000 variable genes identified using the “FindVariableFeatures” function (selection.method = vst, nfeatures = 2,000) (Supplementary Figures 2A,B). Following variable gene selection, data were then centered and scaled using the “Scale Data” function with default parameters. These variable genes were then used to perform a principal component analysis (PCA) on the scaled data. The top 15 PCs obtained were then tested for significance using a JackStraw test that is part of the Seurat 3.1.4 parlance with 100 replicates. Principal components (PCs) with a p-value of <1e-5 were used to perform a Louvain-based clustering on the shared nearest neighbor (SNN) graph (Supplementary Figures 2E,F). For data visualization, we performed t-distributed stochastic neighbor embedding (t-SNE) and Uniform Manifold Approximation and Projection (UMAP) analysis. Specific cell-clusters were detected using the “FindClusters” function from Seurat using a resolution of 0.5. Dendrograms depicting relationships between cell-clusters were generated using the “PlotClusterTree” function.
Marker genes for individual clusters were identified using Seurat's “FindAllMarkers” function calculated using the Wilcoxon's rank sum test. Using this approach, cells from each population were compared against each of the other clusters in order to detect uniquely expressed genes. Only genes that were enriched and expressed in 25% of the cells in each population (min.pct = 2.5) and with a log fold difference larger than 0.25 (logfc.threshold = 0.25) were considered. These differentially expressed genes per cluster were plotted on the feature plot individually using the “FeaturePlot” function in Seurat for visualization in either UMAP or t-SNE space. The results also were visualized in a heatmap generated using the “DoHeatMap” function.
Apart from t-SNE and UMAP analysis, we also performed similarly weighted non-negative embedding (SWNE) analysis for visualizing high-dimensional single-cell gene expression datasets for each of our samples. SWNE captures both local and global structure in the data unlike t-SNE and UMAP embeddings, while enabling genes and biological factors that separate cell types to be embedded directly onto the visualization. To perform the SWNE analysis, a previously published R-based SWNE framework was used (Wu et al., 2018). The analysis was performed on log-normalized read count data for a set of variable genes from a previously generated Seurat object using the “RunSWNE” function. Because the number of factor embeddings representative of the dataset cannot be estimated a priori, this parameter (called K) needs to be determined empirically. As the SWNE algorithm has the non-negative matrix factorization (NMF) inherently built into it, we initially performed NMF analysis over a broad range of K values ranging from 2 to 60 with steps of 2. The outputs of these separate runs were compiled together in order to estimate the optimal K-value. To find the optimal number of factors to use, the “FindNumFactors” function was used. The function iterates over multiple values of k and provides the optimal number of factors that best represent the dataset. Following that, the NMF decomposition was run using the “RunNMF” function that generates an output of gene loadings (W) and NMF embeddings (H). Following the NMF analysis, the SWNE embedding was run using the parameters: alpha.exp = 1.25, snn.exp = 0.25 and n_pull = 3 that control how the factors and neighboring cells affect the cell coordinates. The SWNE output was analyzed using the gene loadings matrix. Since NMF creates a part-based representation of the data, the factors often correspond to key biological processes or gene modules that explain the data. The top factors for each gene were visualized as a heat map using the “ggHeat” function.
The neural and neurosecretory clusters obtained in the stage 5 t-SNE plot were isolated from the differential gene expression matrix, and the previously described Seurat analysis was repeated with the clustering resolution set at 0.5.
Pseudotime analysis of the neurogenic lineage was performed using the Bioconductor package Monocle3.0.2 (Trapnell et al., 2014). For pseudotime analysis, the previously used Seurat object generated from the neural cell subcluster was imported into Monocle3. Monocle3 was run on our normalized counts matrix for the subclustered neural dataset. The data was subject to UMAP dimensional reduction and cell clustering using the “cluster_cells” function (“cluster_cells”: resolution=0.001). A principal graph was plotted through the UMAP coordinates using the “learn_graph” function that represents the path through neurogenesis. This principal graph was further used to order cells in pseudotime using the “ordercells()” function in Monocle3. Following that, we identified the population of neural precursor cells (NPCs) based on expression of cell-cycle markers and re-ran “ordercells()” with NPCs as the root cell state. Genes changing as a function of pseudotime along the principal graph were determined using “graph_test” function. Cells and most differentially expressed genes were then plotted in pseudotime using default parameters in Monocle3. The most significantly expressed genes with the greatest q-values were plotted on a heatmap of expression over pseudotime using the “plot_pseudotime_heatmap” function in Monocle.
To calculate RNA velocity of single cells within the neural subcluster, we applied the velocyto R (v0.6) package (La Manno et al., 2018). Velocyto uses the mapped reads from CellRanger and counts the number of spliced and unspliced reads separately. As the CellRanger read-mapping algorithm is splice-sensitive, the RNA velocity analysis can very easily be applied on the .bam files generated by CellRanger. For our 10X output, counting was performed at the level of molecules, taking into consideration the annotation (spliced, unspliced etc.) of all reads associated with the molecule. A molecule was annotated as spliced, unspliced or ambiguous based on the following criteria: a molecule was considered spliced if all of the reads in the set mapped only to the exonic regions of the compatible transcripts whereas a molecule was called as unspliced if at least one of the supporting reads were found to span exon-intron boundaries or mapped to the intron of the transcript. Molecules for which some of the reads mapped exclusively to the exons and some exclusively to the introns were categorized as “ambiguous” and not used for downstream analysis (La Manno et al., 2018). The command-line interface (CLI) for velocyto R (v0.6) was run in permissive mode. In this setting, we only used the cells mapped to the transcriptome that were present in our final neural subclustering Seurat analysis. Using all cells from the stage 5 neural subcluster, we normalized the expression per cell and selected the top 2000 variable genes to perform a PCA. Using the first 15 principal components we performed a data imputation with a neighborhood of 200 cells (k = 200 nearest neighbors) and calculated RNA velocities. All steps were performed using in-built parameters for fitting gene-models, predicting velocity, extrapolating and plotting. To visualize the plots, we used the t-SNE embedding as produced by the Seurat analysis.
Previous studies of neural development in the marine annelid Capitella teleta found that the first neurons arise in the brain at stage 4 and in the VNC at stage 5 hence representing early stages of neurogenesis (Meyer et al., 2015). Furthermore, different types of cells (neural precursors, neural progenitors, and differentiating neurons) were molecularly identified at stage 4 and 5 in C. teleta using a candidate gene approach (Sur et al., 2017, 2020). In order to further explore developmental trajectories and how transcriptomic landscapes across cells change during early neurogenesis and larval development in C. teleta, we dissociated 300 whole larvae from a single brood at both 24- and 48-h post gastrulation, which corresponds to stage 4 just after appearance of the prototroch ciliary band, and stage 5, respectively (Figure 1A; 1). Based on Hoechst-labeled nuclei counts in the episphere and the trunk, we estimated that a stage 4 larva has ~2,000 cells and a stage 5 larva has ~4,000 cells (Supplementary Figures 1A,B). To enable random sampling of cells, we used 300 animals per stage to maximize the initial pool of cells for cell-capture. We also tested multiple methods of cell dissociation and examined cell survival rate (Figure 1B). For scRNAseq, we dissociated cells in 1% trypsin for 30 min since this yielded the best survival rate (97%). We also passed the dissociated cells through a 40 μm nylon mesh filter to remove clusters of undissociated cells and any large cells that would not likely be efficiently captured by 10x genomics (i.e., large, yolky endodermal midgut cells). This step likely biased our sample toward ectodermal and mesodermal cell populations, which tend to be much smaller in size in C. teleta. Furthermore, due to the unavailability of a high-resolution microscope at the genomics facility and the small size of C. teleta dissociated cells (2–12 μm), the total number of cells could not be counted accurately (See Materials and Methods). Therefore, based on previous pilot cell-dissociation trials (Figure 1B), the number of cells were roughly estimated in the dissociated cell-suspension. We intended to sequence ~4,000 cells per stage but due to technical limitations, we estimate that a much higher concentration of cells (see Materials and Methods) was loaded into the droplet-based scRNAseq platform 10X Genomics Chromium (Figure 1A; 3).
After sequencing and read-mapping, CellRanger predicted to have recovered 34,592 cells with 7,251 mean reads/cells from stage 4 and 16,434 cells with 17,837 mean reads/cell from stage 5 (Figure 1C and Supplementary Table 1). Based on our rough estimations, we recovered around 55% of the total number of cells input into the 10X Genomics system (~60,000 for stage 4 and ~30,000 for stage 5; Figure 1C). However, there appeared to be a lot of noise due to the presence of cell-doublets and free-flowing RNA following cell-capture and sequencing. Hence, to identify distinct cell types from the stage 4 and 5 single-cell datasets, the assembled reads were passed through stringent Seurat quality control and UMI filtering algorithms (Supplementary Figure 2). A second trial conducted with careful estimation of cell counts and capturing ~4,000 cells/stage did not yield enough cDNA to make high quality sequencing libraries unlike the first trial (Supplementary Figures 1C,D). Following computational filtering of our dataset to remove low-complexity transcriptomes, lowly-expressed genes and transcriptome doublets, we bioinformatically recovered 1,072 cells from 300 stage 4 larvae and 1,785 cells from 300 stage 5 larvae that were used for downstream analysis (Figure 1C and Supplementary Table 1). Although we only captured a small fraction of cells after computational filtering, this is the first ever scRNAseq experiment on C. teleta larvae using the 10X genomics platform, and we were able to resolve some discrete transcriptional profiles and their underlying developmental trajectories.
To classify cell population identities in the global dataset across the two C. teleta larval stages, Seurat unsupervised clustering (Butler et al., 2018) of the aggregated data from stages 4 and 5 was conducted. UMAP analysis revealed six computationally identified clusters with a tight group of cells (C0, C1, C3, C4, and C5) and one cell-population situated farther away (C2; Figure 2A). As at stages 4 and 5, majority of the cells in the C. teleta body are undifferentiated, and these individual clusters likely represent distinct developmental trajectories through which cells are progressing. At these stages, there may be cells that are committed toward a specific lineage, but as these cells are still undifferentiated and dividing, they did not separate out as distinct clusters because of a large overlap of highly expressed cell-cycle regulatory genes. Undifferentiated cells expressing receptors of growth factors (e.g., fgfrl1, egf-like receptors) and cell-cycle regulatory genes (e.g. cdc6, mcmbp, cks1; Figures 2B,C) were found to be scattered across all cell clusters. In order to assign cluster identity, we used previously characterized genes in C. teleta and uncharacterized C. teleta genes homologous to known tissue markers in other taxa. Each cluster was identified based on the analysis of the top 30 significantly enriched genes per cluster. Gene annotations are reported in Supplementary Table 2.
Figure 2. Mapping of C. teleta larval tissues from aggregated stage 4 and 5 datasets. (A) UMAP representation of the aggregated data (stages 4 and 5), where the clustering of cells depicts their transcriptional similarity. (B) Heatmap of the top 10 genes significantly enriched in each cluster. Representative gene names obtained from closest reciprocal BLAST hits are shown close to each row. The full gene-list is in Supplementary Table 2. (C) UMAP plots showing log-normalized counts of select representative genes from each cluster. Color intensity is proportional to the expression level (purple: high; gray: low). (D) Dotplot of representative genes involved in C. teleta neurogenesis. (E) Dotplot showing novel markers implicated in C. teleta myogenesis. (F) Dotplot showing orthologs of neurotransmitter and neuropeptide/neurohormone receptors across clusters.
Cluster C0 was enriched in genes predicted to be involved in extracellular matrix remodeling such as protogenin-A, protocadherin fat-4, tyrosine protein kinase csk1, chaoptin and hepatocyte growth factor (hgf), indicating that these cells may be epidermal precursor cells (Figures 2B,C). Similarly, differentially expressed genes in the C1 cluster included UDP-D-xylose:L-fucose alpha-1,3-D-xylosyltransferase 3 (rgxt3), D-threonine aldolase (dta), a chitin-binding peritrophin-A domain containing protein, and vacuolar protein sorting-associated protein 51 homolog (vps51) (Figures 2B,C), all of which represent chitin-binding proteins and proteoglycans (Shen and Jacobs-Lorena, 1999); however, the exact identity of cells in this cluster remained unclear. The other clusters also had distinct expression profiles suggestive of specific identities, C2: ciliary bands + neural cells (tekt4a, rsph1, Ct-elav1, Ct-syt1) among others, C3: gut secretory cells (colq, Ct-blimp, glna2, enteric neuropeptides), C4: myoblasts (Ct-wnt2, tetratricopeptide domain containing unc45b, F-box protein homolog fbx22, vegfb, rer1, myosin heavy-chain), and C5: protonephridia (S-formylglutathione hydrolase, hercynylcysteine sulfoxide lyase and carbohydrate sulfotransferase 1) (Figures 2B–E). C3 also expressed some myogenic markers, albeit at a lower level (Figure 2E), which could indicate that a subset of developing muscle precursors clustered here.
Interestingly, apart from C2, all other clusters expressed receptors for neurotransmitters and neurohormones (Figure 2F). C5 (protonephridia) was found to express dopaminergic neuroreceptors (drd5l) and atrial-natriuretic peptide receptors (anpra) (Figure 2F), while C3 was particularly enriched in receptors for neurotransmitters and neuropeptides/hormones like acetylcholine (acm2 and acha6), GABA (plcl2), FMRF-amide (fmar), gonadotropin (gnrr2), and somatostatin (ssr5). Even though the C3 cluster contained cells that expressed neurotransmitter and neurohormone receptors, we think this cluster could largely contain gut and muscle cells based on expression of these types of receptors in these cell types in other taxa (Florey and Rathmayer, 1978; Walker et al., 1993; Terra et al., 2006; Crisp et al., 2010; Mirabeau and Joly, 2013; Hung et al., 2020; Wu K. et al., 2020). Receptors for acetylcholine, FMRF-amide and GABA have been reported to be localized to the body wall muscle in earthworms and leeches (Walker et al., 1993). Spiralian FMRF-amide G-protein coupled receptors (GPCRs) were first reported in P. dumerilii and were found to be homologous to insect neuropeptide receptors responsive to neuropeptide-F (Elphick et al., 2018). In C. teleta, FMRF-amide+ neurons have been shown to be associated with the midgut (Meyer et al., 2015). Both glutamate and GABA signaling have been reported in midgut epithelial cells in insects (Terra et al., 2006; Hung et al., 2020). Somatostatin/allatostatin-C encodes for a neuropeptide family of hormones that are expressed in D. melanogaster midgut endocrine cells (Wu K. et al., 2020), while octopamine GPCRs have been reported in the annelid P. dumerilii and the priapulid Priapulus caudatus where they were shown to be activated in presence of dopamine, tyramine and octopamine ligands (Bauknecht and Jekely, 2017). Such neuropeptide- and neurotransmitter-signaling repertoires may regulate diverse behavioral changes associated with life-phase transitions in C. teleta based on previous evidence from P. dumerilli (Conzelmann et al., 2013).
The neurotransmitter and neuropeptide receptors characterized in our dataset can also serve as a valuable resource for better understanding neurotransmitter and neuropeptide signaling in C. teleta.
An unsupervised graph-based clustering approach was used to separately analyze transcriptomic data at stages 4 and 5. Datasets were visualized with t-SNE dimensionality reduction (Figures 2, 3A, 4A). In our stage 4 dataset, we detected ~174 median genes per cell and around ~740 median UMIs per cell, while in our stage 5 dataset, we detected ~241 median genes per cell and ~1,145 median UMIs per cell (Supplementary Figure 2 and Supplementary Table 1). At both stages 4 and 5, t-SNE analysis revealed a large population of cells (C0; gray) that were enriched in ribosomal genes (RL10, RS9, RS4), cell proliferation markers (e.g., pcna), S-phase and M-phase cell-cycle markers (e.g., cks1, mcm3, rfa3, wee1) and chromatin remodeling genes (e.g., acinu, bptf), indicating that these cells are undifferentiated, developmental precursors (Figures 3A,B,D,G, 4A,B and Supplementary Tables 3, 4). Such a finding is expected as the C. teleta larval body at this stage largely comprises proliferative cells (Seaver et al., 2005; Sur et al., 2020). The C0 cluster may contain different subsets of precursors or stem cells that give rise to different tissues throughout C. teleta development. Expression of Ct-soxB1 in many of these cells indicates that at least a subset is ectodermal in origin (Figures 3B,D, 4B,C). At stage 5 but not stage 4, some cells within C0 were also found to express muscle-associated markers such as hand2, troponinC and twitchin (data not shown). Therefore, we generically named this cluster “precursors.” In both datasets, we detected a few C0 cells that expressed Ct-piwi1 and Ct-hes2 (Supplementary Figures 3A, 5A). Ct-piwi1 has been characterized as a marker of both somatic and germline stem-cells in C. teleta (Giani et al., 2011), while Ct-hes2 is a homolog of the vertebrate hes1a gene, which is a Notch target and regulates stem-cell maintenance and cell-cycle progression. Ct-hes2 is broadly expressed in larvae, including in the lateral ectoderm and the posterior growth zone where new segments are generated from stage 7 onward in C. teleta (Thamm and Seaver, 2008). C0 in both datasets comprises a complex set of cells that express markers representative of various tissues and may represent uncommitted cells with different developmental trajectories.
Figure 3. Single-cell molecular landscape of stage 4 C. teleta larvae. (A) t-SNE representation of stage 4 larval single cells with clusters labeled by molecular identities. (B) Cell type specific marker genes reflect cellular identities and functions. Heatmap showing log-normalized differentially expressed genes per molecular domain identified. Each row represents a single gene whereas each column represents a cell. (C) Analysis with PlotClusterTree in Seurat to reveal transcriptomic similarities between clusters. (D) t-SNE plots of cells colored by expression of selected marker genes that were used for identifying each molecular domain. The color key indicates expression levels (purple: high; gray: low). (E) Violin plots summarizing the expression levels of select representative genes per cluster. Data points depicted in each cluster represent single cells expressing each gene shown. (F) Dotplot of representative genes involved in C. teleta neurogenesis at stage 4. (G) Dotplot showing cell proliferation (S-phase and G2/M-phase) markers in the stage 4 clusters. cb, ciliary-band.
Figure 4. Single-cell molecular landscape of stage 5 C. teleta larvae. (A) t-SNE representation of stage 5 larval single cells with clusters labeled by transcriptional identities. (B) Cell type specific marker genes reflect cellular identities and functions. Heatmap showing log normalized differentially expressed genes per molecular domain identified. Each row represents a single gene whereas each column represents a cell. (C) t-SNE plots of cells colored by expression of selected marker genes that were used for identifying each cluster. The color key indicates expression levels (purple: high; gray: low). ns, neurosecretory; pn, protonephridia.
C1 in the stage 4 dataset and C2 in the stage 5 dataset primarily expressed markers associated with cellular tight junctions and extracellular matrix (e.g., claudin, lamin a/c, annexin7, and p4ha2) as well as genes shared with C0 and the neural cluster (Figures 3B, 4B and Supplementary Figures 3B, 5B). Lamin A/C and p4ha2 have been identified in the epidermis of P. dumerilii and other spiralians (Kim et al., 2012; Achim et al., 2018). Claudin is a tetraspanning transmembrane protein that is an integral component of tight junctions (Krause et al., 2008; Piontek et al., 2008) while annexin-7 has calcium-dependent membrane-binding activity in most animals. Finding shared expression of putative epidermal and neural markers could corroborate previous lineage tracing data suggesting that individual precursor cells in the neuroectoderm generate anywhere from one to 50 neural cells as well as one or two epidermal cells (Meyer and Seaver, 2009). Therefore, based on the expression of extracellular matrix remodeling genes and neural markers, C1 in stage 4 and C2 in stage 5 was identified as “ectodermal precursor cells.” Cells in the C1 cluster in the stage 5 dataset (Figure 4A) expressed similar genes as in C1 of the aggregated dataset (Figures 2A,B) such as D-threonine aldolase (dta) and vacuolar protein sorting-associated protein 51 homolog (vps51) as a result of which its identity remained unclear.
In the stage 4 dataset, C2 represents ciliary-band cells that express homologs in the dynein family (dyhc, dyhc2, dyh7, dyh5, dy13, hydin etc.), radial spoke-head genes (rsph1 and rsph3) and intraflagellar transport-proteins (ift80) associated with the axonemal apparatus of cilia (Figures 3B,E and Supplementary Figure 3C), similar to P. dumerilii (Achim et al., 2018). Moreover, these ciliary-band cells at stage 4 do not express any of the S-phase or M-phase markers indicating that these cells are not proliferating (Figures 3B,G). Hydin encodes for a protein that constitutes the axonemal central-pair apparatus that regulates cilia motility while IFT80 constitute part of the molecular machinery underlying cilia motility. However, at stage 5, cells expressing these same ciliary markers were found to be scattered across all clusters and did not resolve as a distinct cluster (Figure 4B and Supplementary Figure 5C).
C3 in the stage 4 dataset (Figures 3A,B) and C5 in the stage 5 dataset (Figures 4A,B) were identified as “gut” based on some of the highly expressed markers in that cell-cluster including peptidases (e.g., antistatin, tyrosinase), secretory proteins (e.g., lipophilin, profilin) and glycotransferases (e.g., lrg2b and alg13) (Figures 3B, 4B). These cells were also found to express hepatocyte nuclear factor 4a (hnf4a), tetraspanin-11 and collagen alpha (Figures 3B,D,E, 4B,C and Supplementary Figures 3D, 4D), which have been shown to be expressed in midgut cells in P. dumerilii (Achim et al., 2018) and in digestive cells of the cnidarian Nematostella vectensis, the ctenophore Mnemiopsis lyeidi and the sponge Amphimedon queenslandica (Sebe-Pedros et al., 2018a,b). However, collagen alpha expression was not detected in the stage 5 “gut” cluster while tetraspanin-11 was one of the most enriched genes in the C5 cluster of the stage 5 dataset (Figure 4C). Interestingly, Ct-gataB1, which is expressed in endodermal cells at stage 4 in C. teleta and in the large, yolky midgut cells at later larval stages, was found to be excluded from the “gut” cluster at stage 4 but not at stage 5 (Supplementary Figure 3D). However, at both stages, hnf4a and Ct-gataB1 were expressed in a subset of cells in the C0 “precursors” clusters (Supplementary Figures 3D, 5D). Previous lineage tracing experiments identified a population of small, interstitial cells in the midgut of C. teleta larvae (Meyer et al., 2010), but the genes expressed in these interstitial midgut cells have not been characterized. Since the C3 cluster at stage 4 expresses digestive enzymes but not Ct-gataB1, these could represent interstitial midgut cells. At stage 5, the C5 cluster may include both Ct-gataB1+ large, yolky midgut cells as well as interstitial midgut cells. One possible reason for the clustering of Ct-gataB1+ cells among precursor cells at stage 4 may be because of the proliferative nature of early endodermal cells. As a result, our bioinformatic pipeline detected these Ct-gataB1+ cells to be more similar to the dividing precursors than the C3 gut cells. At stage 5, the Ct-gataB1+ cells may have a decreased proliferative potential and hence are clustered with the other “gut” cells. However, this awaits further verification using cell proliferation assays and in-situ hybridization.
Lastly, the C4 cluster in the stage 4 dataset and C3 in the stage 5 dataset likely have a neural identity based on expression of neural differentiation markers such as Ct-elav1, Ct-syt1, Ct-msi, Ct-neuroD, and Ct-syt1 (Meyer and Seaver, 2009; Meyer et al., 2015; Sur et al., 2017) (Figures 3B,D–F, 4B,C and Supplementary Figures 3F,G, 5F,G). S-phase markers were also expressed in the “neural” cluster at stages 4 and 5 indicating that these may be dividing neural progenitors given their spatial proximity to Ct-elav1+ and Ct-syt1+ cells in the tSNE plot (Figures 3B,D, 4B,C and Supplementary Figures 3E, 5E). In the stage 4 dataset, a few cells in C1 were also found to express some neural markers (e.g., Ct-ngn, Ct-ash1, Ct-msi and Ct-elav1) (Figure 3D). At stages 4 and 5, previous work using whole-mount in situ hybridization found that Ct-ngn and Ct-ash1 are expressed in neural precursor cells (NPCs) and dividing foregut precursor cells. Ct-ash1 is also expressed weakly in dividing mesodermal precursor cells and in some ectodermal cells outside the neuroectoderm (Meyer and Seaver, 2009; Sur et al., 2017, 2020). Therefore, in the C1 cluster at stage 4, the Ct-ngn+/Ct-ash1+ cells may be ectodermal precursors, NPCs, and/or foregut precursor cells, while Ct-ngn−/Ct-ash1+ cells may be mesodermal precursor cells. Previous work has also shown that Ct-msi, Ct-elav1 and Ct-syt1 are exclusively expressed in differentiating and differentiated neurons (Meyer and Seaver, 2009; Meyer et al., 2015; Sur et al., 2017, 2020). Therefore, Ct-elav1+/Ct-syt1+ cells in the C4 cluster at stage 4 are likely neurons, which first form in the developing brain and around the mouth (Meyer et al., 2015). At stage 5, the neural cells form a more coherent cluster C3, comprising NPCs expressing cell-cycle markers, intermediate differentiation states expressing Ct-ngn, Ct-neuroD and Ct-ash1, and mature neurons expressing Ct-elav1, Ct-msi and Ct-syt1 (Figures 4B,C and Supplementary Figures 5F,G). Ct-elav1 and Ct-syt1 were found to be more enriched and restricted to C3 in the stage 5 dataset unlike our observations at stage 4. At both stages we also observed the expression of Ct-hunchback in the neural cluster as previously reported in Capitella (Werbrock et al., 2001) and P. dumerilii (Kerner et al., 2006), and a homolog of MAP-kinase interacting serine/threonine kinase (MKNK1) in neural cells possibly indicating the involvement of the MAP-kinase signaling pathway during C. teleta neural development.
At stage 5, we also identified two additional discrete clusters from stage 4. C4 in the stage 5 dataset was classified as “neurosecretory” based on the expression of markers genes such as the sodium- and chloride-dependent glycine transporter (sc6a5) and synaptotagmin-4 (syt4) and secretogranin-V (scg5) (Figures 4B,C and Supplementary Figures 5G,H). Neurosecretory cells are a category of neurons that secrete hormones (peptidergic and non-peptidergic neuromodulators) into the surrounding tissues in response to neural input, instead of synaptic transmission (Lovejoy, 2005; Hartenstein, 2006). A few neurosecretory cells were also detected as early as stage 4, and these cells clustered within the neural cluster (C4) (Figure 3B and Supplementary Figures 3G,H). These cells could represent neurosecretory brain centers, which have been previously reported in other annelids (Tessmar-Raible et al., 2007; Williams et al., 2017). The function of the sc6a5 gene is to impart neurosecretory fate by inhibiting glycinergic neurotransmission. In addition, non-calcium binding members of the Synaptotagmin family (i.e., Syt4) and Syt-alpha are implicated in the generation of large, dense-core vesicles for neurosecretion and have been found to be highly expressed in neurosecretory cells (Moghadam and Jackson, 2013; Park et al., 2014). Secretogranin-V is a neuroendocrine precursor protein that regulates pituitary hormone secretion in mammals. Marker genes that characterize the neurosecretory cell-cluster (C4) are largely expressed within the neural cluster (C3) as well in the stage 5 dataset. A few unique genes expressed by the neurosecretory cells were neuroendocrine convertase 2, prohormone convertase (Supplementary Figure 5H), and conopressin/neurophysin (data not shown). Neurophysin has been characterized in the developing neurosecretory brain centers in the annelid P. dumerilii and zebrafish D. rerio (Tessmar-Raible et al., 2007). In addition to neurosecretory cells, we also identified a protonephridia cluster, C6, at stage 5 based on the expression of sulfotransferases involved in the urea-cycle, e.g., uronyl sulfotransferase, UDP glucouronic acid decarboxylase and carbohydrate sulfotransferases (Figures 4B,C).
We further examined relationships between all cell-clusters using PlotClusterTree in Seurat as this better represents transcriptional similarities between clusters than t-SNE distance. We found that the neural cluster branches out first followed by the other non-neural cell-clusters (Figure 3C). This further confirms that the neural tissue is the first to be specified during C. teleta development and exhibits more transcriptional similarity to ectodermal precursor cells (C1) than any other cluster.
To decipher differentially-expressed, coregulatory gene modules within each cluster, we also projected both datasets using SWNE on a high-dimensional space correlated with non-negative matrix factorization (NMF) factor embeddings (Wu et al., 2018). In the stage 4 dataset, our SWNE visualization (Supplementary Figure 4) showed a central precursor population that branches into four differentiation trajectories: ectoderm, ciliary-band, foregut and neural (Supplementary Figures 4A,B). At stage 5, the protonephridia cluster emanated from the central ectodermal cluster while the neural cluster split into two, giving rise to the neurosecretory cluster (Supplementary Figures 6A,B).
Based on our SWNE embeddings plot, we deciphered differentially expressed genes for each cluster. The highest number of differentially expressed genes were found in the neural cell-cluster in both stages. For example, at stage 4, a glutamate receptor gene grik4 and a tyrosine-protein phosphatase non-receptor type 4 gene (ptn4) were found to be coregulated together in a subset of neural cells (Supplementary Figure 4B). The gene ptn4 encodes for a non-receptor tyrosine kinase (nRTK), and members of this family have been found to be abundantly present in excitatory synapses in the mammalian brain where they interact directly with glutamate receptors and phosphorylate tyrosine sites (Mao and Wang, 2016). Hence, cells expressing “factor 3” within the neural cluster may represent neurons that are excited by glutamate (Supplementary Figure 4A). These may also represent one of the first neuronal sub-types to differentiate during early C. teleta development. At stage 5, some uniquely expressed genes in the neurosecretory cells revealed by our SWNE analysis include myom1 (myomodulin neuropeptides 1) and orckB (orcokinin neuropeptides class B) (Supplementary Figure 6B). Myomodulin is a bioactive neuropeptide that was found to be secreted by a cholinergic motor neuron in the mollusk Aplysia californica and regulates contraction of the buccal muscles during feeding (Cropper et al., 1987). Putative neurosecretory cells expressing myom1 in C. teleta were coregulated with other G-protein coupled receptor messengers such as plpr1 and y1760 that provide insight into the myom1 mediated neuropeptide signaling pathway (Supplementary Figure 6B). An orcokinin-like neuropeptide was previously identified in the C. teleta genome (Veenstra, 2011). Orcokinins have been detected in multiple other taxa such as insects, crustaceans, tardigrades, mollusks, and sea-stars. In crustaceans, orcokinin neuropeptides have been shown to act as neuromodulators in the CNS and regulate peripheral neuromuscular junctions (Li et al., 2002). Using our unsupervised graph clustering and SWNE analysis, we show developmental trajectories of multiple cell types simultaneously, which was previously not possible using other techniques in C. teleta.
To gain better insight into the different neural cell types present in our stage 5 dataset, we further subclustered and curated cells from the neural (C3) and neurosecretory clusters (C4) using Seurat to obtain neural-specific t-SNE and UMAP plots (Figures 5, 6 and Supplementary Figure 7). Some proliferative cells expressing Ct-soxB1 and bHLH factors like Ct-ash1 and Ct-ngn were found to cluster within the C0 and C2 cells, however, their exact identity was not clear (see previous section) and hence these cells were not included in this analysis. As t-SNE plots do not preserve global data structure, i.e., only within cluster distances are meaningful and between cluster similarities are not guaranteed, we also plotted UMAP plots to better project the relationships of the individual neural subclusters (Figure 6A and Supplementary Figure 7). SWNE analysis was also performed on the neural sub-cluster dataset to identify co-expressed genes (Supplementary Figure 8).
Figure 5. Neural cell type diversity in stage 5 larvae. (A) t-SNE representation of single cells obtained from the neural + neurosecretory cell clusters generated from unsupervised clustering of the stage 5 dataset, labeled and colored based on cluster identity (B) Dotplot showing differentially expressed genes per neural cell type identified. Each row represents a single gene regulating individual aspects of neurogenesis whereas each column represents one of the four neural cell types. The expression is log normalized per gene. (C) t-SNE plots colored by expression of selected marker genes that were used for identifying each cell type. The color key indicates expression levels (purple: high; gray: low). Blue arrow highlights expression of Ct-ash1 in the NPC cluster. db, differentiation bridge; ns, neurosecretory.
Figure 6. Lineage relationships between neural cell types and pseudotime analysis. (A) UMAP representation of single-cells from the neural + neurosecretory cell-cluster generated in the stage 5 unsupervised clustering. (B) Trajectory analysis using Monocle3 predicts a neural differentiation trajectory that begins with NPCs and ends with the mature neuronal and neuroendocrine cell cluster. The root of the trajectory lies within the NPC cell-cluster. (C) Monocle3 pseudo-temporal ordering of neural cells superimposed on the Seurat UMAP plot. Cells are colored based on their progression along pseudo-temporal space from pseudotime 0 in violet to the end of differentiation in yellow. (D) Monocle analysis predicts progressive expression dynamics of mcm7 homolog, Ct-ngn, Ct-soxB1, Ct-neuroD, secretogranin-V, and Ct-syt1. (E) Heatmaps showing most significant TFs and effector genes clustered by pseudotemporal expression pattern (q < 0.01). Pseudo-temporal ordering is from left (NPCs) to right (differentiated neurons). Selected transcription factors are shown for each cellular state along the differentiation trajectory.
Based on our t-SNE plot, we identified four clusters within the combined neural and neurosecretory group: (a) undifferentiated neural progenitors, (b) intermediate differentiation bridge, (c) differentiating neurosecretory cells and (d) mature neurons/neurosecretory cells containing a mixture of neurons with both neurotransmitter and neurohormonal output (Figure 5A). The undifferentiated progenitors were identified based in the expression of S-phase markers such as cdc6, cks1, rfa2, dpolA, wee1 and replication licensing factors such as mcm3 and mcm7 (Figures 5B,C) as well as M-phase markers such as ccnb, mpip and cdk1. These cells were also found to exclusively express Ct-notch (Figures 5B,C). Ct-notch is expressed in both surface and subsurface cells in the anterior neuroectoderm at stage 5 (Meyer and Seaver, 2009). We have previously shown in the C. teleta anterior neuroectoderm that surface cells primarily comprise rapidly dividing neural precursor cells (NPCs) while subsurface cells are largely post-mitotic neural cells (Meyer and Seaver, 2009; Sur et al., 2017, 2020). Hence this cluster may represent a combination of rapidly dividing NPCs and a few progenitors with limited proliferative potential. We named this cluster “NPCs.” Similar to our previous observations using EdU and fluorescent in-situ hybridization (FISH) (Sur et al., 2020), we observed Ct-ash1 and Ct-ngn expression in this cluster (Figures 5B,C, blue arrow). These undifferentiated cells were also found to express Ct-msi and Ct-elav2 albeit at a much lower level (Figure 5B) indicating that neural progenitors possibly express Ct-msi at lower expression levels.
We detected two transitional differentiation states (blue and green populations), one uniquely expressing pan-neural markers like Ct-msi and Ct-elav2 that we named “differentiation bridge” and the other uniquely expressing glutamine synthetase (glna2), androglobin (adgb), endophilin-1 (shlb1), neuroendocrine convertase (nec2), and synaptotagmin-4 (syt4) (Figures 5A,B). Glna2 is an enzyme involved in glutamine synthesis in excitatory glutaminergic neurons and has been shown to regulate the secretion of various adenohypophyseal hormones (Hrabovszky and Liposits, 2008). Syt4 was found to be expressed in the neuroendocrine center of the vertebrate hypothalamus regulating oxytocin secretion (Zhang et al., 2011) as well as in the neuroendocrine center of the P. dumerlii head (Achim et al., 2018). Based on the expression of these genes, which are involved in the neuroendocrine pathway, we named this cell cluster (green population) “neurosecretory.” Neurosecretory cells are a type of neural cell that secrete hormones in response to neural input (Lovejoy, 2005; Hartenstein, 2006). Both the intermediate differentiation bridge and differentiating neurosecretory cells (blue and green), expressed Ct-elav1, Ct-neuroD and Ct-pou6 (Figures 5B,C, 6A and Supplementary Figure 7A). A subset of cells in the “differentiation bridge” also expressed Ct-ngn and Ct-ash1 indicating a later role in C. teleta neurogenesis (Figures 5B,C).
The fourth cell population within the neural cluster expressed mature neuronal markers such as synaptotagmin-1 (Ct-syt1), alpha-tubulin, Ct-synapsin as well as neurosecretory markers such as Ct-syt4 (Figures 5B,C). Within the mature neuronal cell type, we detected a variety of neuronal subtypes: (i) glutaminergic neurons expressing glutamine synthetase (glna2) and vesicular glutamate transporter (vgl2b), (ii) cholinergic neurons expressing acetylcholinesterase (aces) and vesicular acetylcholine transporter (vacht), (iii) GABAergic neurons expressing sodium- and chloride-dependent GABA transporter 1 (sc6a1), and (iv) neuroendocrine subtypes expressing Ct-syt4, secretogranin-V and prohormone-4 among others (Figures 5A–C). Neuroendocrine genes were expressed at a much higher level among cells in the “mature neuron” cluster (red) than in the “neurosecretory” cluster (green) indicating that the “mature neuron” cluster comprises both mature neuronal and neurosecretory cell populations (Figure 5B). Overall, our t-SNE and UMAP analyses highlighted different neural cell types that were previously reported (Meyer and Seaver, 2009; Sur et al., 2017, 2020) as well as previously unknown neuronal subtypes within each cell-cluster that await further characterization.
Next, we applied SWNE analysis to identify coregulated gene modules within each neural cell type (Supplementary Figure 8). We observed different sets of co-expressed genes in the undifferentiated cluster. One such coregulated subset of genes included cks1 (cyclin-dependent kinase regulatory subunit 1), bafB (Barrier to autointegration factor B) and hgv2 nucleosomal assembly factor. All three genes in this module play important roles in cell-cycle progression (Furukawa et al., 2003) (Supplementary Figures 8A,B). The differentiation transition states were also found to co-express genes such as neuroD, dpys and rdh11 and talin-1 indicating that cells in this cluster are already on distinct neural differentiation trajectories. Talin-1 was expressed in another gene module present in the differentiating neurosecretory cells along with an EF-hand domain containing protein and a gene encoding a potassium voltage-gated channel subfamily H8 (kcnh8). Among the cells that clustered within the mature neuronal cluster, we detected subsets of cells expressing genes encoding V-type proton ATPase (vatl) as well as peptidergic markers such as myom1 and orckB (Supplementary Figures 8A,B).
To understand pseudotemporal relationships between the different neural cell types at stage 5, we used Monocle3.0.2, which orders cells based on similarities of their global transcriptional profiles. Due to the poor resolution of NPCs and presence of only a few cells in the neural cluster in our stage 4 dataset (Figure 3A), stage 4 cells were not included in our pseudotemporal analysis. Starting from the neighborhood graph generated in t-SNE or UMAP space (Figure 6A), Monocle uses reversed graph embedding to reconstruct single-cell trajectories in a fully unsupervised manner (Trapnell et al., 2014; Qiu et al., 2017). Using Monocle, we also identified variable gene sets or modules in different cell states (Supplementary Figure 9). While running the Monocle3 algorithm without any assumptions about the trajectory, we obtained an abstracted graph that allowed us to derive a single differentiation tree that included all the neural cell types and linked them to one root, the NPC cluster (Figure 6B). Along the trajectory, cells were ordered based on their developmental origin and state of differentiation (Figures 6A,B). This generated a pseudotime trajectory with six distinct cell states (Figures 6C–E). These were defined by the expression of Ct-notch and S-phase markers (mcm7, rfa2, dpolA, cks1) for the NPC state; Ct-ngn and wee1 for a progenitor state; kif1a, band7, mprg, hemicentin-1, and Ct-syt4 for an intermediate neuronal differentiation state; pal2, glna2, plp, and rdh11 for an intermediate neurosecretory differentiation state; Ct-syt1, Ct-synapsin, synaptobrevin, neurensin, neuroendocrine convertase-2 (nec2) among others in the final state comprising both mature neuronal and neuroendocrine cell types (Figure 6E and Supplementary Figures 7A–G). Interestingly, the proximity of these cell types in the UMAP plot (Figure 6A) indicated that their transcriptomes are closely related in a continuous fashion.
To identify temporal progression of genes that may be involved in neurogenic cell fate decisions, we mapped some previously characterized genes that significantly varied in their pseudotemporal expression and looked more closely at their expression dynamics (Figure 6C). This analysis showed several discrete shifts in gene expression patterns during C. teleta neurogenesis. For example, S-phase markers (mcm7, rfa2, and dpolA) and Ct-notch were only expressed in proliferating NPCs and were rapidly downregulated at the onset of differentiation (Figures 6D,E). In another subset of NPCs (Figures 6A–C), genes like wee1, Ct-ngn and other bHLH transcription factors such as Ct-ash1 and Ct-atonal were upregulated later in pseudotime than the S-phase markers and were not downregulated until the latter stages of neural differentiation (Figures 6D,E and Supplementary Figure 9). These Ct-ngn+ NPCs possibly represent a transient population of NPCs or a more committed progenitor state as discussed previously (Sur et al., 2020). Expression of Ct-neuroD peaked as Ct-soxB1 and Ct-ngn began to become downregulated (Figure 6D). Such an observation closely follows patterns obtained using double-FISH and FISH+EdU experiments reported previously (Sur et al., 2020). Genes involved in imparting a neurosecretory identity such as secretogranin-V, pal2 and glutamine synthestase (glna2) were found in the next step of the cascade of differentiating cells (Figures 6A–E). These genes turned on as Ct-neuroD expression began to decline (Figure 6D) but were downregulated prior to the expression of the next subset of markers such as Ct-syt1, Ct-syt4, Ct-synapsin, neurensin, and synaptobrevin (snaa) among others, which initiated their expression and increased later in pseudotime (Figure 6E and Supplementary Figure 7G). These late-expressing genes like Ct-syt1, Ct-syt4, Ct-synapsin and snaa likely modulate neurotransmitter and neurohormonal release and hence are expressed in mature neuronal subtypes that exhibit synaptic transmission as well as neurosecretion. Therefore, our pseudotemporal analysis revealed an early commitment of NPCs to the neurosecretory program prior to the neuronal program during C. teleta neurogenesis. Neural subtype specific neurotransmitter synthesis markers such as acetylcholinesterase (aces), vesicular acetylcholine transporter (vacht), glna2 and sc6a1 were expressed even later in pseudotime and represent different neuronal subtypes such as cholinergic and GABAergic neurons (Figure 6E and Supplementary Figure 7D). Interestingly, we also observed expression of neuroendocrine genes such as neuroendocrine convertase (nec2) in this pseudotemporal cluster indicating some mature neurosecretory cells as well. Overall, our trajectory analysis along with tSNE/UMAP plots elucidate that the C. teleta CNS at stage 5 comprises neurons that likely communicate via presynaptic transmission as well as neurosecretion (i.e., secreting hormones into nearby extracellular space). Based on our analysis, it seems that both types of neurons may arise from a common pool of NPCs. These NPCs commit to a neurosecretory gene expression program earlier in pseudotime than their commitment to a presynaptic gene expression program, and they employ different gene expression programs between the two differentiation trajectories. Interestingly, we also identified a transient cell-state of NPCs expressing bHLH transcription factors such as Ct-ngn and Ct-ash1 later in pseudotime than proliferative NPCs expressing cell-cycle genes. Most of these proliferative NPCs expressed Ct-soxB1 but only a subset of these Ct-soxB1+ NPCs were found to express Ct-ngn. In previous work, we identified at least two population of actively-dividing neural precursors in the brain and VNC, one Ct-soxB1+/Ct-ngn+ population and another Ct-soxB1+/Ct-ngn+/Ct-ash1+ population. We hypothesized that Ct-ash1 may act as a molecular switch that regulates the transition from cell proliferation to neural differentiation. Therefore, we speculated that these Ct-soxB1+/Ct-ngn+/Ct–ash1+ cells may represent a transient population of NPCs or may represent a more committed progenitor state (Sur et al., 2020). This needs functional validation and further characterization, but is consistent with our scRNAseq analyses, which recovered two temporal phases of NPCs including a later Ct-ash1+ transition state.
To independently validate the differentiation trajectories predicted by Monocle3 and to gain insight into dynamics of stem-cell activation and differentiation, we used velocyto (La Manno et al., 2018), a computational method that tracks recent changes in transcriptional rate of a gene to predict future mRNA levels of that gene (Figures 7A,B). These transcriptional rate changes are estimated for each gene by calculating the ratio of spliced vs. unspliced reads in the sequencing data (Supplementary Figures 10A,B, 11) and extrapolating over all genes across all cells in the dataset. The timescale of future cell-state prediction is on the scale of a few hours (La Manno et al., 2018).
Figure 7. RNA velocity plotted in t-SNE space for neural cell types. (A) Velocyto force field showing the average differentiation trajectories (velocity) for cells located in different parts of the tSNE plot. For each cell, arrows indicate the location of the future cell state. RNA dynamics vary between NPCs, the two differentiation trajectories and mature neurons and also within each cell type. Velocity estimates based on nearest cell pooling (k = 5) were used. Red circle shows two cells with velocity fields pointing along the two differentiation trajectories possibly representing a progenitor population for neural and neurosecretory cells, respectively. (B) Same velocity field as A, visualized using Gaussian smoothing on a regular grid.
We estimated RNA velocity for each cell within the combined neural and neurosecretory group at stage 5 (C3 + C4) to assess the relationship between NPCs, differentiating neurons and mature neurons. We projected the estimated cell states onto the t-SNE plot, which describes the path predicted by the RNA velocity algorithm and visualized the results by plotting an arrow for each cell spanning its actual and predicted future cell state. Hence cells that are transcriptionally active have long arrows, whereas cells that are undergoing very low transcriptional turnover have either short or no arrows. For example, in the mature neuronal cell-cluster we observed little and uncoordinated RNA velocity indicating that these cells are transcriptionally stable and are undergoing less changes at the RNA level, reinforcing that these cells represent terminally differentiated cell types (Figures 7A,B). Projecting the RNA velocity of individual cells states on a PCA plot separated each cell cluster and captured the main neural differentiation axis (Supplementary Figures 10C–F). Similarly, within the NPC cluster (purple), a subset of cells exhibited very short arrows indicating very low RNA metabolism whereas another subset was found to have relatively longer arrows pointing along the differentiation axis (Figures 7A,B). Finding differential transcriptional activity in this cluster highlights that this is a heterogenous population, similar to what we detected using Monocle3 pseudotemporal analysis (Figure 6E).
We observed differences in RNA velocity between the two differentiation trajectories as well. In the neuronal differentiation trajectory, RNA velocity was higher than that in the neurosecretory trajectory. However, arrows within both trajectories pointed away from the NPCs indicating the direction of differentiation. Decreasing RNA velocity toward the far end of the neurosecretory trajectory led us to speculate that these cells have a stable transcriptome and may represent terminally differentiated neurosecretory cells. We identified the root of the differentiation process in the NPC cluster similar to our observations from pseudotime analysis and trajectory prediction using Monocle3. We also identified two cells that lie close to each other in t-SNE space and point along the direction of each differentiation trajectory, possibly representing neural and neurosecretory progenitors, respectively (Figure 7A, red circle). The paths predicted by velocyto largely agree with the trajectories predicted by Monocle, which supports two independent differentiation trajectories for neurons and neurosecretory cells. The continuity of cells in t-SNE space obtained using our RNA velocity analysis (Figures 7A,B) coupled with pseudotime analysis (Figures 6C–E) and gene expression across cell types (Figures 5B,C) highlights the continuous nature of C. teleta neurogenesis, making rigid classification of neural cell types along the differentiation trajectory difficult.
We present here one of the first scRNAseq studies on a spiralian annelid using the 10X genomics platform. Our experimental design and analysis revealed that 10X genomics may not be the optimal platform for sequencing dissociated cells from spiralian larvae due to their smaller cell size and low RNA content as compared to most well-studied vertebrate taxa. Because of the small size of C. teleta cells and our inability to limit the dilution in which cells were loaded, that may have led to a high-doublet rate post encapsulation causing some background noise in our 10X output. As a result, we used stringent quality control metrics to screen out such doublets. Similar background noise and a considerable amount of cell-free RNA can be detected in another 10X dataset obtained from the blastema of the head-regenerating adult earthworm Eisenia andrei although clustering analysis was able to generate 12 discrete clusters, the majority of which appeared to be undifferentiated stem cells (Shao et al., 2020). Our second attempt with more careful quantification of cells failed to yield enough cDNA to create high-quality libraries for sequencing probably because of low RNA content in C. teleta cells. We excluded the possibility that cells were dying during encapsulation as ambient (cell-free) RNA would still have undergone reverse-transcription and contributed to the total cDNA. Although scRNAseq approaches are an exciting step forward for spiralian evo-devo studies, there are still technical challenges that needs to be overcome to obtain better resolution spiralian scRNAseq atlases. Whole-body scRNAseq analysis on 373 cells dissociated from P. dumerilii 48 hpf larvae using the Fluidigm C1 Single-cell AutoPrep system also yielded limited resolution into the various cell-types present at that stage (Achim et al., 2018). Therefore, future scRNAseq approaches in spiralian taxa need to be designed using manual capture of cells more along the lines of SmartSeq approaches that have the potential to generate high-quality libraries from low RNA input samples (Satija et al., 2015; Farrell et al., 2018). However, SmartSeq approaches are less cost-effective than bulk droplet-capture procedures like 10X genomics and DropSeq (Ziegenhain et al., 2017), making future efforts at optimizing these techniques for spiralians important.
The technical challenges we encountered could partly explain the limited resolution that we obtained in the individual clusters in the tSNE plot. In both stage 4 and 5 datasets, we recovered 2–3 large clusters that represented many undifferentiated cells. We think there may have been multiple types of precursors and/or progenitors contained within, but that these did not resolve into fate-specific groups because the mostly highly expressed set of genes in each group of cells were shared cell-cycle regulatory genes. Any unique fate-specific genes may not have been expressed highly enough to be detected or may have been excluded based on the expression fold change cutoff we set. Another possibility is that because the Capitella teleta genome is not well-curated and we did not have a reference transcriptome, the read-mapping was not comprehensive enough to detect more fate-specific genes, some of which could have served as key differentially expressed genes in our dataset.
Despite the technical challenges of using 10X genomics with cells of small sizes, our study allowed us to investigate gene expression, developmental trajectories and identify molecular domains in larvae at two different stages for the annelid Capitella teleta. Using this approach, we demonstrate that (i) previously characterized marker genes in C. teleta can be used to annotate bioinformatically derived cell-clusters, (ii) Louvain clustering analysis can resolve the entire C. teleta body plan into distinct molecular domains based on differential gene expression, and (iii) trajectory analysis can track continuous changes in pseudotemporal gene expression patterns during differentiation of certain cell types. Recent work has only examined gene expression dynamics in C. teleta using WMISH and immunolabeling experiments, and we view our study as a first step toward understanding annelid and eventually spiralian development at a systems level. Furthermore, we present the differential gene expression analyses and cell types in this study as hypotheses that require validation via functional and in situ expression studies to test the accuracy of the in-silico predictions made here.
Our data allow comparison of cell types at the single-cell level across the entire animal. During cell dissociation, we excluded the yolky endodermal cells present in the C. teleta larvae at these stages, that were larger than 40 μm in size as 10X genomics can only capture cells up to 30 μm in diameter. Therefore, our data is biased toward ectodermal and mesodermal cell populations, which are smaller in size. Our unsupervised clustering approaches classified the C. teleta larval whole-body into eight distinct molecular domains—(i) generic precursor cells, (ii) ectodermal precursors, (iii) myoblasts, (iv) ciliary-bands, (v) gut secretory cells, (vi) neural cells, (vii) neurosecretory cells, and (viii) protonephridia. By comparing our findings with previous studies, we created an annotated list of markers for each of the cell clusters that were previously characterized as well as novel markers as highlighted in the Results section. Our multifaceted analyses revealed the progressive origin of tissues and how the genes underlying the development of these tissues change across the two larval stages. The first few discrete clusters to originate soon after gastrulation included gut, ciliary-band and nervous system. After 24 h of development, more discrete clusters were identified including neurosecretory cells and protonephridia. Interestingly, neurosecretory properties were detected as early as stage 4 (~24 h post gastrulation), but these cells clustered together with neurons. Based on previous work, neurons in C. teleta are first detected in the developing brain at stage 4 (Meyer and Seaver, 2009; Meyer et al., 2015; Sur et al., 2017). The presence of neurosecretory cells together with neurons in our stage 4 t-SNE plot indicates a considerable neuronal diversity at the initial stages of C. teleta development. Such early appearance of diverse neurons may enable C. teleta larvae to respond to environmental stimuli. Neurons identified at stage 5 are also representative of the CNS (brain and VNC) as other aspects of the nervous system such as the peripheral nervous system (PNS) does not develop until stages 6 or 7 (24–48 h from stage 5). Moreover, we also did not detect any presence of glial cells at these stages. To our knowledge, no studies so far have shown the presence of glial cells in C. teleta. Furthermore, from our pseudotemporal analysis on the neural and neurosecretory subclusters at stage 5, we inferred progressive changes in gene expression during the progression of NPCs to neurons. Our data suggest a cascade in which early cell-cycle markers are rapidly downregulated followed by an upregulation of neural differentiation markers such as Ct-neuroD and subsequently by orthologs of genes involved in neuronal migration and terminal differentiation genes that function in mature neurons. Using a marker-independent approach, velocyto, we also computed RNA velocity within the neural cell clusters and recovered similar differentiation trajectories and transcriptional dynamics that we deduced using Monocle, showing the robustness of our approaches.
Our C. teleta single-cell analysis presented here enables a systematic comparison of cell types across species. We found that cells at stages 4 and 5 in C. teleta expressed many genes shared with homologs in P. dumerilii larvae around a similar developmental stage (Achim et al., 2018). For example, gut primordial cells of endodermal origin expressing hnf4a and collagen alpha 3, ciliary-bands expressing rsph3, tekt4a, tekt1a, and ift80, and myoblasts expressing myosin were found to be similar to that in P. dumerlii (Achim et al., 2018). We observed consistent expression of tektin homologs in the ciliary band cluster across the two stages in C. teleta, and these homologs were previously reported in ciliary bands of P. dumerlii and another annelid Hydroides elegans (Arenas-Mena et al., 2007; Achim et al., 2018). Recent reports show the expression of tektin homologs in the P. dumerilii prototroch, ciliated apical organ, telotroch and two pairs of paratrochs, and axonemal dyenin homologs in all ciliary structures of the mollusk Tritia obsoleta (Wu L. et al., 2020), similar to our findings in C. teleta. This suggests that a role of tektin homologs in the ciliary bands of annelid larval trocophores may have been a conserved feature. A recent report by Wu L. et al. (2020) uncovered two spiralian-specific genes expressed in the ciliary bands of most spiralians called lophotrochin and trochin. These genes along with the markers identified in our study provide a valuable resource in further characterization of the origin of ciliary bands within Spiralia.
Some of gut cells described here express Ct-blimp1 and represent endodermal midgut precursors (Boyle et al., 2014). In both C. teleta and P. dumerilii, the large, yolky midgut cells originate from the vegetal macromeres (Ackermann et al., 2005; Meyer et al., 2010). We only noticed shared expression of hnf4a between both annelids but not expression of any of the other P.dumerlii “gut” markers in our dataset (Achim et al., 2018). This may be due to the fact that we have size-excluded a majority of large, yolky midgut cells at stages 4 and 5 that were larger than 40 μm in size prior to cell-capture (see Materials and methods). Whether the genetic developmental program of the gut is conserved between the two annelids needs more investigation. Recently, hnf4 has been shown to be expressed in specialized gut stem cells of the blood feeding parasitic flatworm Schistosoma mansoni that regulates gut maintenance and blood feeding (Wendt et al., 2020). Therefore, hnf4 seems to be a key regulator of digestive gut cells in annelids and planarians; however, no data on hnf4 exists from mollusks to deduce it's role in the spiralian ancestor.
Within the neural and neurosecretory cell types, we also identified markers that were previously detected in the scRNAseq dataset for P. dumerlii larvae (Achim et al., 2018). Subclustering these cell types allowed us to detect coherent sets of effector genes and transcription factors expressed at different pseudotimes, representing distinct cellular modules, e.g., NPCs, intermediate differentiation bridge, differentiating neurosecretory cells, and mature neurons and neuroendocrine cells. In addition, we also observed considerable differences in gene expression even within individual neural subgroups highlighting distinct but related cell types. For example, within the NPC cluster, we observed two different gene modules that were expressed at different pseudotimes and had differential transcriptional activity, indicating a heterogenous population of NPCs. From our subclustering analysis of neural cells, we identified putative phc2+ neurosecretory cells in C. teleta, which may be homologous to phc2+ neurosecretory centers in P. dumerlii and other spiralians (Tessmar-Raible et al., 2007; Achim et al., 2018). Phc2+ neuroendocrine centers were also detected apically in the developing larval brain of P. dumerlii are were found to express other vertebrate-type neuropeptides such as the Vasotocin/neurophysin preprohormone (Achim et al., 2018). Vasotocin/neurophysin homologs have been found in many spiralians including annelids (Oumi et al., 1994; Tessmar-Raible et al., 2007), gastropods (van Kesteren et al., 1992), and cephalopods (Takuwa-Kuroda et al., 2003). However, we could not detect a vasotocin/neurophysin homolog in C. teleta from our scRNAseq analysis although we only detected one or two conopressin/neurophysin-expressing cells at stages 4 and 5. Therefore, presence of larval neuroendocrine centers regulating neurohypophyseal hormonal activity seems to be a conserved feature among spiralians that has been lost in D. melanogaster and C. elegans (Tessmar-Raible et al., 2007).
Altogether, our C. teleta scRNAseq study suggest that comparative studies of cell types across animal evolution using high-throughput scRNAseq is a promising direction for evo-devo research and needs to be expanded to more taxa. As exemplified here, whole-organism scRNAseq across many taxa can provide comprehensive insights into metazoan cell type evolution and tissue-specific genome-wide regulatory networks.
The datasets presented in this study can be found in online repositories. The names of the repository/repositories and accession number(s) can be found below: https://www.ncbi.nlm.nih.gov/geo/query/acc.cgi?acc=GSE159564, GSE159564.
AS and NM: conceptualization, methodology, writing—original draft, writing—review & editing, and funding acquisition. AS: software, formal analysis, and investigation. NM: supervision. All authors: contributed to the article and approved the submitted version.
This work was funded partly by the Sigma Xi Grants-in-Aid-of- Research (GIAR) acquired by AS (Grant #G2018031596148406) and partly by two grants from Clark University, a research grant supported by the Lise Ann and Leo E. Beavers' fund and a faculty development grant supported by the Fechter-Stansbury fund to NM.
The authors declare that the research was conducted in the absence of any commercial or financial relationships that could be construed as a potential conflict of interest.
The authors are grateful to the Boston University Single-Cell Sequencing Core for their services and their excellent support. The authors also thank the two reviewers for their insightful comments.
The Supplementary Material for this article can be found online at: https://www.frontiersin.org/articles/10.3389/fevo.2020.618007/full#supplementary-material
Achim, K., Eling, N., Vergara, H. M., Bertucci, P. Y., Musser, J., Vopalensky, P., et al. (2018). Whole-body single-cell sequencing reveals transcriptional domains in the annelid larval body. Mol. Biol. Evol. 35, 1047–1062. doi: 10.1093/molbev/msx336
Achim, K., Pettit, J. B., Saraiva, L. R., Gavriouchkina, D., Larsson, T., Arendt, D., et al. (2015). High-throughput spatial mapping of single-cell RNA-seq data to tissue of origin. Nat. Biotechnol. 33, 503–509. doi: 10.1038/nbt.3209
Ackermann, C., Dorresteijn, A., and Fischer, A. (2005). Clonal domains in postlarval Platynereis dumerilii (Annelida: Polychaeta). J. Morphol. 266, 258–280. doi: 10.1002/jmor.10375
Arenas-Mena, C., Wong, K. S., and Arandi-Forosani, N. (2007). Ciliary band gene expression patterns in the embryo and trochophore larva of an indirectly developing polychaete. Gene Expr. Patterns 7, 544–549. doi: 10.1016/j.modgep.2007.01.007
Arendt, D., Musser, J. M., Baker, C. V. H., Bergman, A., Cepko, C., Erwin, D.H., et al. (2016). The origin and evolution of cell types. Nat. Rev. Genet. 17, 744–757. doi: 10.1038/nrg.2016.127
Bauknecht, P., and Jekely, G. (2017). Ancient coexistence of norepinephrine, tyramine, and octopamine signaling in bilaterians. BMC Biol. 15:6. doi: 10.1186/s12915-016-0341-7
Blake, A. J., and Grassle, J. P., Eckelbarger, K., and Eckelbarger, J. (2009). Capitella teleta, a new sp. designation for the opportunistic and experimental Capitella sp. I. with a review of the literature for confirmed records. Zoosymposia 2, 25–53. doi: 10.11646/zoosymposia.2.1.6
Boyle, M. J., Yamaguchi, E., and Seaver, E. C. (2014). Molecular conservation of metazoan gut formation: evidence from expression of endomesoderm genes in Capitella teleta (Annelida). Evodevo 5:39. doi: 10.1186/2041-9139-5-39
Butler, A., Hoffman, P., Smibert, P., Papalexi, E., and Satija, R. (2018). Integrating single-cell transcriptomic data across different conditions, technologies, and species. Nat. Biotechnol. 36, 411–420. doi: 10.1038/nbt.4096
Cao, J., Packer, J. S., Ramani, V., Cusanovich, D. A., Huynh, C., Daza, R., et al. (2017). Comprehensive single-cell transcriptional profiling of a multicellular organism. Science 357, 661–667. doi: 10.1126/science.aam8940
Conzelmann, M., Williams, E. A., Tunaru, S., Randel, N., Shahidi, R., Asadulina, A., et al. (2013). Conserved MIP receptor-ligand pair regulates Platynereis larval settlement. Proc. Natl. Acad. Sci. U.S.A. 110, 8224–8229. doi: 10.1073/pnas.1220285110
Crisp, K. M., Grupe, R. E., Lobsang, T. T., and Yang, X. (2010). Biogenic amines modulate pulse rate in the dorsal blood vessel of Lumbriculus variegatus. Comp. Biochem. Physiol. C Toxicol. Pharmacol. 151, 467–472. doi: 10.1016/j.cbpc.2010.02.003
Cropper, E. C., Tenenbaum, R., Kolks, M. A., Kupfermann, I., and Weiss, K. R. (1987). Myomodulin: a bioactive neuropeptide present in an identified cholinergic buccal motor neuron of Aplysia. Proc. Natl. Acad. Sci. U.S.A. 84, 5483–5486. doi: 10.1073/pnas.84.15.5483
Elphick, M. R., Mirabeau, O., and Larhammar, D. (2018). Correction: evolution of neuropeptide signalling systems. J. Exp. Biol. 221(Pt 19):jeb193342. doi: 10.1242/jeb.193342
Farrell, J. A., Wang, Y., Riesenfeld, S. J., Shekhar, K., Regev, A., and Schier, A. F. (2018). Single-cell reconstruction of developmental trajectories during zebrafish embryogenesis. Science 360:eaar3131. doi: 10.1126/science.aar3131
Finn, R. D., Coggill, P., Eberhardt, R. Y., Eddy, S. R., Mistry, J., Mitchell, A. L., et al. (2016). The Pfam protein families database: towards a more sustainable future. Nucleic Acids Res. 44, D279–D285. doi: 10.1093/nar/gkv1344
Florey, E., and Rathmayer, M. (1978). The effects of octopamine and other amines on the heart and on neuromuscular transmission in decapod crustaceans: further evidence for a role as neurohormone. Comp. Biochem. Physiol. C 61C, 229–237. doi: 10.1016/0306-4492(78)90136-3
Foster, S., Oulhen, N., and Wessel, G. (2020). A single cell RNA sequencing resource for early sea urchin development. Development 147:dev191528. doi: 10.1242/dev.191528
Furukawa, K., Sugiyama, S., Osouda, S., Goto, H., Inagaki, M., Horigome, T., et al. (2003). Barrier-to-autointegration factor plays crucial roles in cell cycle progression and nuclear organization in Drosophila. J. Cell Sci. 116(Pt 18), 3811–3823. doi: 10.1242/jcs.00682
Galluzzi, L., Kepp, O., Trojel-Hansen, C., and Kroemer, G. (2012). Mitochondrial control of cellular life, stress, and death. Circ. Res. 111, 1198–1207. doi: 10.1161/CIRCRESAHA.112.268946
Giani, V. C. Jr., Yamaguchi, E., Boyle, M. J., and Seaver, E. C. (2011). Somatic and germline expression of piwi during development and regeneration in the marine polychaete annelid Capitella teleta. Evodevo 2:10. doi: 10.1186/2041-9139-2-10
Hartenstein, V. (2006). The neuroendocrine system of invertebrates: a developmental and evolutionary perspective. J. Endocrinol. 190, 555–570. doi: 10.1677/joe.1.06964
Hashimshony, T., Wagner, F., Sher, N., and Yanai, I. (2012). CEL-Seq: single-cell RNA-Seq by multiplexed linear amplification. Cell Rep. 2, 666–673. doi: 10.1016/j.celrep.2012.08.003
Hrabovszky, E., and Liposits, Z. (2008). Novel aspects of glutamatergic signalling in the neuroendocrine system. J. Neuroendocrinol. 20, 743–751. doi: 10.1111/j.1365-2826.2008.01719.x
Hung, R. J., Hu, Y., Kirchner, R., Liu, Y., Xu, C., Comjean, A., et al. (2020). A cell atlas of the adult Drosophila midgut. Proc. Natl. Acad. Sci. U.S.A. 117, 1514–1523. doi: 10.1073/pnas.1916820117
Kerner, P., Zelada Gonzalez, F., Le Gouar, M., Ledent, V., Arendt, D., and Vervoort, M. (2006). The expression of a hunchback ortholog in the polychaete annelid Platynereis dumerilii suggests an ancestral role in mesoderm development and neurogenesis. Dev. Genes Evol. 216, 821–828. doi: 10.1007/s00427-006-0100-9
Kim, Y., McDole, K., and Zheng, Y. (2012). The function of lamins in the context of tissue building and maintenance. Nucleus 3, 256–262. doi: 10.4161/nucl.20392
Krause, G., Winkler, L., Mueller, S. L., Haseloff, R. F., Piontek, J., and Blasig, I. E. (2008). Structure and function of claudins. Biochim. Biophys. Acta 1778, 631–645. doi: 10.1016/j.bbamem.2007.10.018
La Manno, G., Soldatov, R., Zeisel, A., Braun, E., Hochgerner, H., Petukhov, V., et al. (2018). RNA velocity of single cells. Nature 560, 494–498. doi: 10.1038/s41586-018-0414-6
Li, L., Pulver, S. R., Kelley, W. P., Thirumalai, V., Sweedler, J. V., and Marder, E. (2002). Orcokinin peptides in developing and adult crustacean stomatogastric nervous systems and pericardial organs. J. Comp. Neurol. 444, 227–244. doi: 10.1002/cne.10139
Lovejoy, D. A. (2005). Neuroendocrinology: An Integrated Approach. West Sussex: John Wiley and Sons, Ltd (2005). doi: 10.1002/0470027878
Mao, L. M., and Wang, J. Q. (2016). Tyrosine phosphorylation of glutamate receptors by non-receptor tyrosine kinases: roles in depression-like behavior. Neurotransmitter 3:e1118.
Marletaz, F., Peijnenburg, K., Goto, T., Satoh, N., and Rokhsar, D. S. (2019). A new spiralian phylogeny places the enigmatic arrow worms among gnathiferans. Curr. Biol. 29, 312–318 e313. doi: 10.1016/j.cub.2018.11.042
Meyer, N. P., Boyle, M. J., Martindale, M. Q., and Seaver, E. C. (2010). A comprehensive fate map by intracellular injection of identified blastomeres in the marine polychaete Capitella teleta. Evodevo 1:8. doi: 10.1186/2041-9139-1-8
Meyer, N. P., Carrillo-Baltodano, A., Moore, R. E., and Seaver, E. C. (2015). Nervous system development in lecithotrophic larval and juvenile stages of the annelid Capitella teleta. Front. Zool. 12:15. doi: 10.1186/s12983-015-0108-y
Meyer, N. P., and Seaver, E. C. (2009). Neurogenesis in an annelid: characterization of brain neural precursors in the polychaete Capitella sp. I. Dev. Biol. 335, 237–252. doi: 10.1016/j.ydbio.2009.06.017
Mirabeau, O., and Joly, J. S. (2013). Molecular evolution of peptidergic signaling systems in bilaterians. Proc. Natl. Acad. Sci. U.S.A. 110, E2028–E2037. doi: 10.1073/pnas.1219956110
Moghadam, P. K., and Jackson, M. B. (2013). The functional significance of synaptotagmin diversity in neuroendocrine secretion. Front. Endocrinol. 4:124. doi: 10.3389/fendo.2013.00124
Oumi, T., Ukena, K., Matsushima, O., Ikeda, T., Fujita, T., Minakata, H., et al. (1994). Annetocin: an oxytocin-related peptide isolated from the earthworm, Eisenia foetida. Biochem. Biophys. Res. Commun. 198, 393–399. doi: 10.1006/bbrc.1994.1055
Park, D., Li, P., Dani, A., and Taghert, P. H. (2014). Peptidergic cell-specific synaptotagmins in Drosophila: localization to dense-core granules and regulation by the bHLH protein DIMMED. J. Neurosci. 34, 13195–13207. doi: 10.1523/JNEUROSCI.2075-14.2014
Piontek, J., Winkler, L., Wolburg, H., Muller, S. L., Zuleger, N., Piehl, C., et al. (2008). Formation of tight junction: determinants of homophilic interaction between classic claudins. FASEB J. 22, 146–158. doi: 10.1096/fj.07-8319com
Plass, M., Solana, J., Wolf, F. A., Ayoub, S., Misios, A., Glazar, P., et al. (2018). Cell type atlas and lineage tree of a whole complex animal by single-cell transcriptomics. Science 360:eaaq1723. doi: 10.1126/science.aaq1723
Qiu, X., Mao, Q., Tang, Y., Wang, L., Chawla, R., Pliner, H. A., et al. (2017). Reversed graph embedding resolves complex single-cell trajectories. Nat. Methods 14, 979–982. doi: 10.1038/nmeth.4402
Saliba, A. E., Westermann, A. J., Gorski, S. A., and Vogel, J. (2014). Single-cell RNA-seq: advances and future challenges. Nucleic Acids Res. 42, 8845–8860. doi: 10.1093/nar/gku555
Satija, R., Farrell, J. A., Gennert, D., Schier, A. F., and Regev, A. (2015). Spatial reconstruction of single-cell gene expression data. Nat. Biotechnol. 33, 495–502. doi: 10.1038/nbt.3192
Seaver, E. C., Thamm, K., and Hill, S. D. (2005). Growth patterns during segmentation in the two polychaete annelids, Capitella sp. I and Hydroides elegans: comparisons at distinct life history stages. Evol. Dev. 7, 312–326. doi: 10.1111/j.1525-142X.2005.05037.x
Sebe-Pedros, A., Chomsky, E., Pang, K., Lara-Astiaso, D., Gaiti, F., Mukamel, Z., et al. (2018a). Early metazoan cell type diversity and the evolution of multicellular gene regulation. Nat. Ecol. Evol. 2, 1176–1188. doi: 10.1038/s41559-018-0575-6
Sebe-Pedros, A., Saudemont, B., Chomsky, E., Plessier, F., Mailhe, M. P., Renno, J., et al. (2018b). Cnidarian cell type diversity and regulation revealed by whole-organism single-cell RNA-Seq. Cell 173, 1520-1534.e1520. doi: 10.1016/j.cell.2018.05.019
Shao, Y., Wang, X. B., Zhang, J. J., Li, M. L., Wu, S. S., Ma, X. Y., et al. (2020). Genome and single-cell RNA-sequencing of the earthworm Eisenia andrei identifies cellular mechanisms underlying regeneration. Nat. Commun. 11:2656. doi: 10.1038/s41467-020-16454-8
Shen, Z., and Jacobs-Lorena, M. (1999). Evolution of chitin-binding proteins in invertebrates. J. Mol. Evol. 48, 341–347. doi: 10.1007/PL00006478
Simakov, O., Marletaz, F., Cho, S. J., Edsinger-Gonzales, E., Havlak, P., Hellsten, U., et al. (2013). Insights into bilaterian evolution from three spiralian genomes. Nature 493, 526–531. doi: 10.1038/nature11696
Strathmann, M. F. (1987). Reproduction and Development of Marine Invertebrates of the Northern Pacific Coast. Data and Methods for the Study of Eggs, Embryos, and </Collab>Larvae: University of Washington Press.
Sur, A., Magie, C. R., Seaver, E. C., and Meyer, N. P. (2017). Spatiotemporal regulation of nervous system development in the annelid Capitella teleta. Evodevo. 8:13. doi: 10.1186/s13227-017-0076-8
Sur, A., Renfro, A., Bergmann, P. J., and Meyer, N. P. (2020). Investigating cellular and molecular mechanisms of neurogenesis in Capitella teleta sheds light on the ancestor of Annelida. BMC Evol. Biol. 20:84. doi: 10.1186/s12862-020-01636-1
Svensson, V., Vento-Tormo, R., and Teichmann, S. A. (2018). Exponential scaling of single-cell RNA-seq in the past decade. Nat. Protoc. 13, 599–604. doi: 10.1038/nprot.2017.149
Takuwa-Kuroda, K., Iwakoshi-Ukena, E., Kanda, A., and Minakata, H. (2003). Octopus, which owns the most advanced brain in invertebrates, has two members of vasopressin/oxytocin superfamily as in vertebrates. Regul. Pept. 115, 139–149. doi: 10.1016/S0167-0115(03)00151-4
Tanay, A., and Regev, A. (2017). Scaling single-cell genomics from phenomenology to mechanism. Nature 541, 331–338. doi: 10.1038/nature21350
Tang, F., Barbacioru, C., Bao, S., Lee, C., Nordman, E., Wang, X., et al. (2010). Tracing the derivation of embryonic stem cells from the inner cell mass by single-cell RNA-Seq analysis. Cell Stem Cell 6, 468–478. doi: 10.1016/j.stem.2010.03.015
Terra, W. R., Costa, R. H., and Ferreira, C. (2006). Plasma membranes from insect midgut cells. An Acad Bras Cienc 78, 255–269. doi: 10.1590/S0001-37652006000200007
Tessmar-Raible, K., Raible, F., Christodoulou, F., Guy, K., Rembold, M., Hausen, H., et al. (2007). Conserved sensory-neurosecretory cell types in annelid and fish forebrain: insights into hypothalamus evolution. Cell 129, 1389–1400. doi: 10.1016/j.cell.2007.04.041
Thamm, K., and Seaver, E. C. (2008). Notch signaling during larval and juvenile development in the polychaete annelid Capitella sp. I. Dev. Biol. 320, 304–318. doi: 10.1016/j.ydbio.2008.04.015
Trapnell, C. (2015). Defining cell types and states with single-cell genomics. Genome Res 25, 1491–1498. doi: 10.1101/gr.190595.115
Trapnell, C., Cacchiarelli, D., Grimsby, J., Pokharel, P., Li, S., Morse, M., et al. (2014). The dynamics and regulators of cell fate decisions are revealed by pseudotemporal ordering of single cells. Nat. Biotechnol. 32, 381–386. doi: 10.1038/nbt.2859
van Kesteren, R. E., Smit, A. B., Dirks, R. W., de With, N. D., Geraerts, W. P., and Joosse, J. (1992). Evolution of the vasopressin/oxytocin superfamily: characterization of a cDNA encoding a vasopressin-related precursor, preproconopressin, from the mollusc Lymnaea stagnalis. Proc. Natl. Acad. Sci. U.S.A. 89, 4593–4597. doi: 10.1073/pnas.89.10.4593
Veenstra, J. A. (2011). Neuropeptide evolution: neurohormones and neuropeptides predicted from the genomes of Capitella teleta and Helobdella robusta. Gen Comp. Endocrinol. 171, 160–175. doi: 10.1016/j.ygcen.2011.01.005
Vergara, H. M., Bertucci, P. Y., Hantz, P., Tosches, M. A., Achim, K., Vopalensky, P., et al. (2017). Whole-organism cellular gene-expression atlas reveals conserved cell types in the ventral nerve cord of Platynereis dumerilii. Proc. Natl. Acad. Sci. U.S.A. 114, 5878–5885. doi: 10.1073/pnas.1610602114
Walker, R. J., Holden-Dye, L., and Franks, C. J. (1993). Physiological and pharmacological studies on annelid and nematode body wall muscle. Comp. Biochem. Physiol. C 106, 49–58. doi: 10.1016/0742-8413(93)90253-H
Wendt, G., Zhao, L., Chen, R., Liu, C., O'Donoghue, A. J., Caffrey, C. R., et al. (2020). A single-cell RNA-seq atlas of Schistosoma mansoni identifies a key regulator of blood feeding. Science 369, 1644–1649. doi: 10.1101/2020.02.03.932004
Werbrock, A. H., Meiklejohn, D. A., Sainz, A., Iwasa, J. H., and Savage, R. M. (2001). A polychaete hunchback ortholog. Dev. Biol. 235, 476–488. doi: 10.1006/dbio.2001.0272
Williams, E. A., Veraszto, C., Jasek, S., Conzelmann, M., Shahidi, R., Bauknecht, P., et al. (2017). Synaptic and peptidergic connectome of a neurosecretory center in the annelid brain. Elife 6:42. doi: 10.7554/eLife.26349.042
Wu, K., Li, S., Wang, J., Ni, Y., Huang, W., Liu, Q., et al. (2020). Peptide hormones in the insect midgut. Front. Physiol. 11:191. doi: 10.3389/fphys.2020.00191
Wu, L., Hiebert, L. S., Klann, M., Passamaneck, Y., Bastin, B. R., Schneider, S. Q., et al. (2020). Genes with spiralian-specific protein motifs are expressed in spiralian ciliary bands. Nat. Commun. 11:4171. doi: 10.1038/s41467-020-17780-7
Wu, Y., Tamayo, P., and Zhang, K. (2018). Visualizing and interpreting single-cell gene expression datasets with similarity weighted nonnegative embedding. Cell Syst. 7:e654. doi: 10.1016/j.cels.2018.10.015
Zhang, G., Bai, H., Zhang, H., Dean, C., Wu, Q., Li, J., et al. (2011). Neuropeptide exocytosis involving synaptotagmin-4 and oxytocin in hypothalamic programming of body weight and energy balance. Neuron 69, 523–535. doi: 10.1016/j.neuron.2010.12.036
Zhong, S., Zhang, S., Fan, X., Wu, Q., Yan, L., Dong, J., et al. (2018). A single-cell RNA-seq survey of the developmental landscape of the human prefrontal cortex. Nature 555, 524–528. doi: 10.1038/nature25980
Keywords: neurogenesis, single-cell RNAseq, annelid, cell type, differentiation trajectory, pseudotime, RNA velocity, gene regulatory network
Citation: Sur A and Meyer NP (2021) Resolving Transcriptional States and Predicting Lineages in the Annelid Capitella teleta Using Single-Cell RNAseq. Front. Ecol. Evol. 8:618007. doi: 10.3389/fevo.2020.618007
Received: 16 October 2020; Accepted: 23 December 2020;
Published: 26 January 2021.
Edited by:
Maria Ina Arnone, Zoological Station Anton Dohrn, ItalyReviewed by:
Deirdre Lyons, University of California, San Diego, United StatesCopyright © 2021 Sur and Meyer. This is an open-access article distributed under the terms of the Creative Commons Attribution License (CC BY). The use, distribution or reproduction in other forums is permitted, provided the original author(s) and the copyright owner(s) are credited and that the original publication in this journal is cited, in accordance with accepted academic practice. No use, distribution or reproduction is permitted which does not comply with these terms.
*Correspondence: Néva P. Meyer, bm1leWVyQGNsYXJrdS5lZHU=
Disclaimer: All claims expressed in this article are solely those of the authors and do not necessarily represent those of their affiliated organizations, or those of the publisher, the editors and the reviewers. Any product that may be evaluated in this article or claim that may be made by its manufacturer is not guaranteed or endorsed by the publisher.
Research integrity at Frontiers
Learn more about the work of our research integrity team to safeguard the quality of each article we publish.