- 1Department of Archaeology, University of Aberdeen, Aberdeen, United Kingdom
- 2Department of Human Evolution, Max-Planck-Institute for Evolutionary Anthropology, Leipzig, Germany
- 3Institute of Marine Sciences, University of California, Santa Cruz, Santa Cruz, CA, United States
- 4Southwest Fisheries Science Center, National Marine Fisheries Service, Santa Cruz, CA, United States
- 5Research School of Earth Sciences, The Australian National University, Canberra, ACT, Australia
- 6Archaeology, College of Humanities, Arts and Social Sciences, Flinders University, Bedford Park, SA, Australia
- 7Australian Research Centre for Human Evolution, Griffith University, Nathan, QLD, Australia
- 8Department of Archaeology, School of Culture and Society, Aarhus University, Højbjerg, Denmark
- 9School of Biological Sciences, University of Aberdeen, Aberdeen, United Kingdom
- 10Géosciences Environnement Toulouse, Observatoire Midi Pyrénées, Université de Toulouse III Paul Sabatier, Toulouse, France
Establishing strontium isotope (87Sr/86Sr) geographical variability is a key component of any study that seeks to utilize strontium isotopes as tracers of provenance or mobility. Although lithological maps can provide a guideline, estimations of bioavailable 87Sr/86Sr are often necessary, both in qualitative estimates of local strontium isotope “catchments” and for informing/refining isoscape models. Local soils, plants and/or animal remains are commonly included in bioavailability studies, although consensus on what (and how extensively) to sample is lacking. In this study, 96 biological samples (plants and snails) were collected at 17 locations spanning 6 lithological units, within a region of south-west France and an area with a high concentration of Paleolithic archaeological sites. Sampling sites aligned with those from a previous study on soil bioavailable strontium, and comparison with these values, and the influence of environmental and anthropogenic variables, was explored. Data confirm a broad correspondence of plant and snail 87Sr/86Sr values with lithological unit/soil values, although the correlation between expected 87Sr/86Sr values from lithology and bioavailable 87Sr/86Sr ratios from biological samples was higher for plants than for snails. Grass, shrub and tree 87Sr/86Sr values were similar but grasses had a stronger relationship with topsoil values than trees, reflecting differences in root architecture. Variability in 87Sr/86Sr ratios from all plant samples was lower for sites located on homogeneous geological substrates than for those on heterogeneous substrates, such as granite. Among environmental and anthropogenic variables, only an effect of proximity to water was detected, with increased 87Sr/86Sr values in plants from sites close to rivers originating from radiogenic bedrock. The results highlight the importance of analyzing biological samples to complement, inform and refine strontium isoscape models. The sampling of plants rather than snails is recommended, including plants of varying root depth, and (if sample size is a limitation) to collect a greater number of samples from areas with heterogeneous geological substrates to improve the characterizations of those regions. Finally, we call for new experimental studies on the mineralized tissues of grazers, browsers, frugivores and/or tree leaf feeders to explore the influence of 87Sr/86Sr variability with soil profile/root architecture on 87Sr/86Sr values of locally-feeding fauna.
Introduction
Although the first strontium isotope studies on ancient skeletal materials took place almost forty years ago (Ericson, 1985), it has only been in the past two decades that the technique has emerged as a leading means of exploring past human and animal movements in archaeology and paleoecology. In archaeological studies, strontium isotope analysis is used to study the geographical origins of humans, animals and artifacts, and can thus help provide insights into diverse demographic, economic and socio-cultural aspects of past lives. This includes the identification of individual and population patterns of immigration and emigration (e.g., Knudson et al., 2004; Leach et al., 2009); the inference of social conventions such as matri- or patri-locality (e.g., Bentley et al., 2005); the reconstruction of trade and exchange networks (e.g., Thornton, 2011; Laffoon et al., 2015); and the exploration of animal husbandry and herding practices (Balasse et al., 2002; Bentley and Knipper, 2005).
In Paleolithic archaeology, given the otherwise sparse record, insights into the past movement habits of humans and animals can be particularly valuable. The strontium isotope analysis of zooarchaeological materials from Paleolithic sites has been used to explore prey-species movements and seasonality (Julien et al., 2012; Price et al., 2017), giving insights into hominin landscapes use and hunting strategies (Pellegrini et al., 2008; Britton et al., 2011). These methods have also been applied to the fossilized hominin teeth, largely through the application of minimally destructive laser ablation sampling and analyses. These approaches have, for example, been used to infer landscape use and home range size amongst early hominins in South Africa (Copeland et al., 2011; Balter et al., 2012; Joannes-Boyau et al., 2019). Strontium isotope studies have also illuminated the lifetime movement habits of Neanderthals (Richards et al., 2008; Willmes et al., 2016) and Upper Paleolithic humans in Europe (Lugli et al., 2019). Strontium isotope studies have also focused on raw materials to infer the movement of crafted objects. For example, strontium isotopes were used to infer the provenance of Late Upper Paleolithic beads from a child burial in southwest France, and whether their source was contemporary coastlines or local fossil assemblages from Miocene marine deposits (Vanhaeren et al., 2004). Other types of material culture have been studied using similar techniques: for example, 87Sr/86Sr ratios, along with other rare earth elements, were also used to identify a single, French source of colorful fluorites found at Upper Paleolithic sites in Belgium (Goemaere et al., 2013).
While showing great potential, the number of applications in Paleolithic archaeology remains small and—as in all other research areas using strontium isotopes in archaeology—is at least in part limited by the extent to which local strontium isoscapes are understood. Assessing the range of biologically available strontium isotope values around sites of interest and across the wider landscape, and establishing an isoscape, are key components for any mobility and provenance study—from the Middle Paleolithic to the post-medieval period. However, the strategies for the construction of isoscapes remain contentious, and methods used to characterize and describe spatial variation in strontium isotope ratios vary—along with the methods for gauging local values. Approaches used to generate isoscapes include GIS-based techniques, including a priori approaches (Bataille and Bowen, 2012; Bataille et al., 2014), often incorporating mixing models, machine learning (Bataille et al., 2018), or spatial aggregation (often based on underlying the lithology) to demark discrete strontium isotope units or packages (Evans et al., 2010, 2018; Snoeck et al., 2020). All these approaches rely on source strontium data. While lithological maps, or the direct measurement of strontium local rock samples, can provide a guide for local bioavailable strontium, samples are normally collected to assess bioavailability directly. These may include modern plants, soils, micro-fauna, local waters, archaeological fauna, or a mixture of analytes. However, only a few studies have been published comparing these sample types (see Maurer et al., 2012 for an example), and there is a lack of consensus concerning what (and how extensively) to sample for bioavailability when generating or refining isoscapes (see discussion in Snoeck et al., 2020).
In southwest France, the Dordogne is an area famed for its prehistoric archaeology, particularly the late Middle and Upper Paleolithic caves and rockshelters of the Vézère valley and its tributaries. In addition to large assemblages of archaeological artifacts (including lithics from a number of type sites) and, of course, world-renowned cave art, this region boasts one of the densest and most significant early paleoanthropological records of late Middle/early Upper Paleolithic human remains, along with abundant faunal assemblages. Situated between the coastal lowlands to the west, the Loire Valley to the north, the Pyrenees to the south, and the Massif Central to the east, the region is at the intersection of different ecological, geological and topographic zones. Indeed throughout the history of study in the region, researchers have sought to forge connections across this significant eco-cultural landscape: for example, sites in the Périgord and the Pyrenees have been described as representing the different seasonal bases for the same groups of highly mobile Magdalenian “reindeer followers” (Bahn, 1977: 255; Gordon, 1988). The investigation of human movements in this region, and the landscape use of the prey-species they depended on, using strontium isotope analyses remains an important yet understudied research opportunity.
Here, we present a new strontium isotope bioavailability dataset for this region comprising almost 100 biological samples (plants, snails) collected at 17 different sampling locations and spanning 6 lithological units. Sampling locations were selected to align broadly with sampling locations of a previous study on soil strontium, including published and unpublished data (unpublished data from Moffat, 2013; published data from Willmes et al., 2014), allowing comparison of plant and snail measurements with soil values. The influence of a range of other variables was also explored including elevation, land-use/ forest cover, proximity to roads and rivers, and lithology. The aims of this research were three-fold: (1) to compare variability between substrate strontium values, and bioavailable values determined from the plants and malacofauna in this study; (2) to explore the influence of a range of landscape variables on those bioavailable strontium values in this region; and (3) to further refine the isoscape for this significant archaeological region.
Materials and Methods
Field Sampling and Sample Identification
Field sampling for plants and snails was conducted in August 2013. Sampling locations (Figure 1) were based on the distribution of major geological units in the region of study, and then—specifically within each unit—selected to align as closely as possible with 24 locations included in a previous nationwide study of soil strontium isotope variability (Willmes et al., 2014) and from the doctoral thesis from one of the authors (Moffat, 2013) (see Supplementary Table 1). A handheld GPS was used to record the location of each plant and snail sampling locality (Garmin 2465, which is accurate to ± 15 m). Typical sites included areas of open unmanaged ground, areas of forest, and unmanaged areas at the edges of agricultural fields or track ways. At each location, samples were taken in close proximity to each other and included multiple samples of grass (i.e., shallow rooted plants), leaves from shrubs (i.e., medium root-depth plants) and tree leaves (i.e., deep rooted plants), along with empty snail shells (where available). Plant samples were air dried in open polyethylene sample bags during the field season to avoid decomposition.
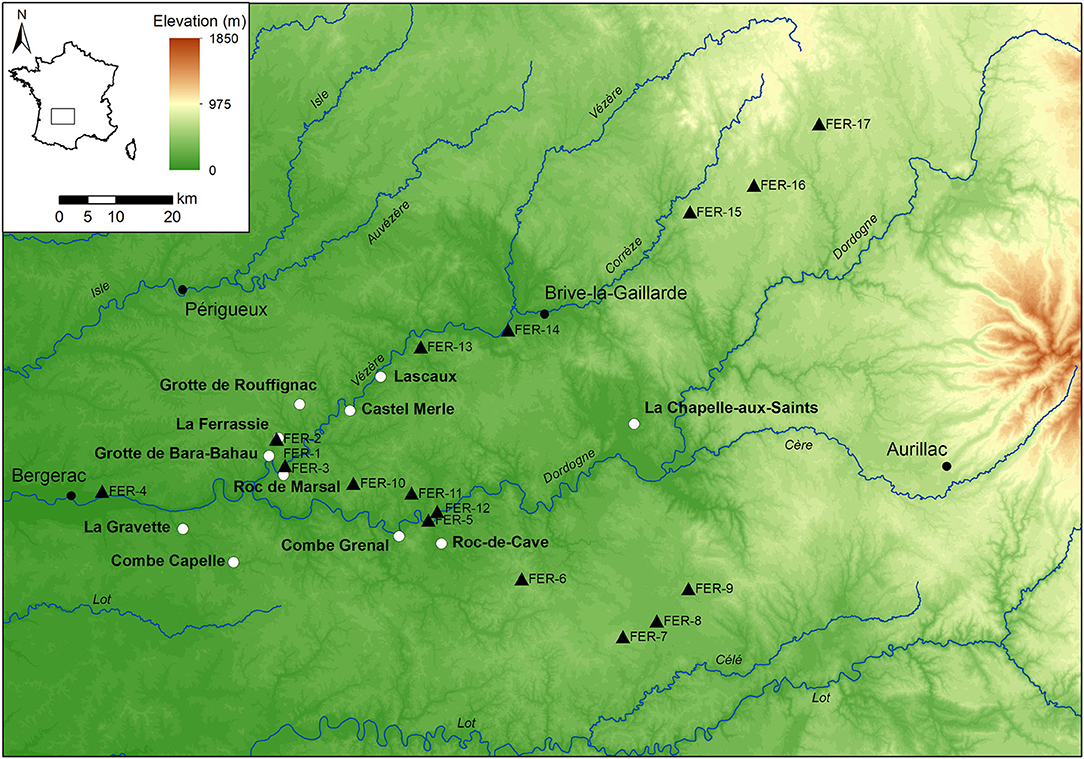
Figure 1. Elevational map of the study area with the sampling locations (black triangles), and the location of selected Middle and Upper Paleolithic archaeological sites (white circles).
After initial assessment in the field, plant and snail samples were given taxonomic assignments by two of the authors (SW and MAM, respectively). Identifications of plant samples followed Stace (2010) and Rose (1989), and malacofauna were identified using reference materials, as well as Cossignani and Cossignani (1995) and Kerney (1999). Species consisted of common northern and western European species and are listed in Supplementary Table 2 alongside all plant and snail strontium isotope data.
Laboratory Protocols and Data Generation
Plant and snail samples were prepared and analyzed at the Department of Human Evolution, Max Planck Institute for Evolutionary Anthropology, Leipzig, Germany. Air dried plant samples were sub-sampled and washed in ultra-pure MilliQ water to remove any adhering soils, dust or other materials, before being frozen and freeze dried. Snail shell samples were ultrasonicated for 15 min in ultra-pure MilliQ water for the same purpose, air dried overnight and crushed using a clean agate pestle and mortar. Dried plant samples were cut into smaller pieces and placed in porcelain crucibles with lids and then ashed in a muffle furnace overnight at 500°C.
Strontium was extracted from snails and plants using a modification of the method from Deniel and Pin (2001), described in detail in Copeland et al. (2008). Aliquots of ashed plant material and crushed snail shells (~3 to 50 mg) were weighed into 3 ml Savillex vials (Minnetonka, MN, USA) and digested in 1 ml of 14.3 M HNO3 (SupraPur grade, Sigma Aldrich) on a hotplate set at 80°C for 8 h. Samples were then dried down and re-dissolved in 1 ml 3 M HNO3 before loading into pre-conditioned microcolumns containing Sr-specific resin (EiChrom, Darian, Il, USA). Strontium was eluted from the resin in ultrapure deionized water (18.2 MΩ), dried and then re-dissolved in 3% HNO3 for analysis of 87Sr/86Sr ratios using a Thermo Fisher (Thermo Fisher Scientific, Bremen, Germany) Neptune multi-collector inductively coupled plasma mass spectrometer (MC-ICP-MS).
All 87Sr/86Sr measurements were corrected for interferences from krypton (Kr) and rubidium (Rb) and normalized for instrumental mass bias to 88Sr/86Sr = 8.375209 (exponential law). Analysis of the international strontium isotope standard NIST SRM987 (National Institute of Standards and Technology, Gaithersburg, USA) during each analytical session was used for external normalization of data [long-term 87Sr/86Sr value = 0.710273 ± 0.000033 (2 σ) (n = 97)]. All 87Sr/86Sr values reported here were adjusted so SRM987 = 0.710240 (Johnson and Fridrich, 1990), and this typically involved a data correction factor of −0.00002. Strontium concentrations of the enamel samples were determined using the method described in Copeland et al. (2008), which is accurate to within ±31 ppm. In addition, each batch of sample was prepared with the external standard SRM 1486 (bone meal). The nine samples analyzed gave an average value of 0.709297 ± 0.000030, to be compared to the long term in house value of 0.709298 ± 0.000026, n = 139 or the compiled value of GeoREM (0.70931, n = 8, http://georem.mpch-mainz.gwdg.de/).
Soil samples had been analyzed as part of a previous unrelated study and include published and unpublished data (Moffat, 2013; Willmes et al., 2014). Soil samples were prepared following the method described in Willmes et al. (2014). In brief, a ~30 g subsample of each topsoil sample was dried overnight at 60°C, sieved through a 2 mm sieve, and a 1 g aliquot was subsampled and leached by adding 2.5 ml 1M ammonium nitrate (NH4NO3) following the protocol DIN ISO 19730 and shaking for 8 h. Samples were then centrifuged at 3,000 rpm for 15 min, the supernatant extracted (~1–2 ml) and evaporated to dryness and then re-dissolved in 2 ml 2M nitric acid (HNO3). Strontium concentration was determined using ICP-AES before the samples were processed by ion exchange chromatography to isolate strontium from other interfering elements using two sets of columns filled with Eichrom Sr-specific resin (pre-filter and Sr-spec resin). Strontium isotope ratios of soils were measured in the Environmental Geochemistry and Geochronology Laboratory at the Research School of Earth Sciences, ANU, also using a Neptune MC-ICP-MS. Data reduction includes Kr and 87Rb isobar corrections, an exponential mass bias correction, and 3 sigma outlier rejection. Total procedural blanks varied between 50 and 250 pg strontium and measurements of the strontium carbonate standard SRM987 (National Institute of Standards and Technology) gave an average 87Sr/86Sr value of 0.71023 ± 0.00001 (n = 167, 2σ).
87Sr/86Sr values of plant, snail and soil samples used in this study are shown in Figure 2, and in Supplementary Table 1 (soils) and Supplementary Table 2 (plants and snails) in the Supplementary Material.
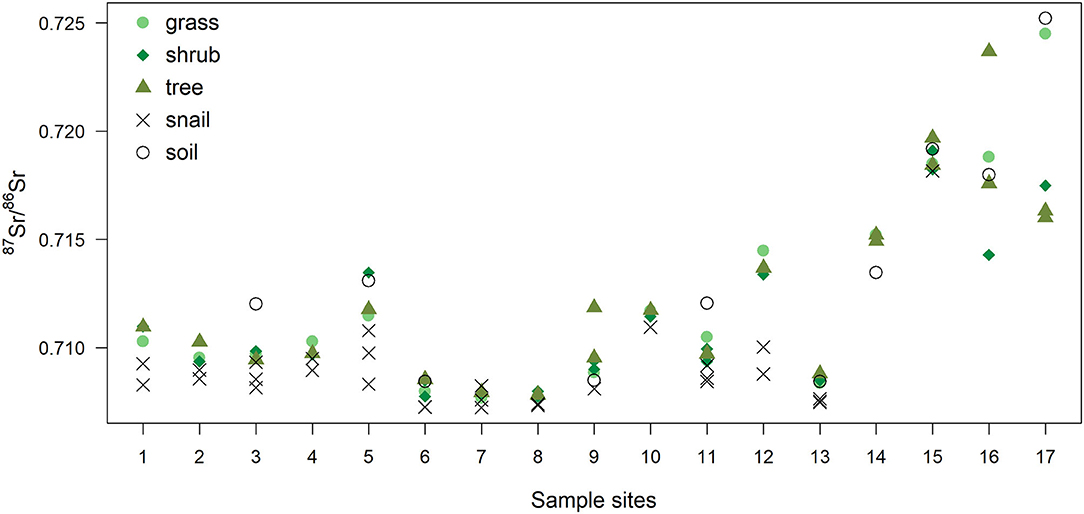
Figure 2. 87Sr/86Sr values of plants (grass, shrub, tree), snails and soil for each site. Sites for biological and soil samples were different. Soil 87Sr/86Sr values presented are from the closest site sampled within 1 km from each plant sampling site, and include data from Willmes et al. (2014), and unpublished data from Moffat (2013) (see Supplementary Table 1).
Data Analysis
Environmental Data
For each of the 17 plant and snail sampling sites, data pertaining to the elevation, minimal distance to a road, minimal distance to water, and the local habitat, were extracted using ArcGIS v10.5. Elevation data came from the Digital Elevation Map of Europe (DEM 25m, Copernicus Land Monitoring Service, 2018 European Union, https://land.copernicus.eu/). Distance to road and distance to water were calculated using road and hydrographic network data1. Habitat was assessed from Corine Land Cover data (https://land.copernicus.eu/). Deciduous, coniferous and mixed forest habitats were grouped into a “forest” category, and pasture and complex cultivation pattern habitats into a “crop” category. Each plant and snail sampling site in the current study was associated with the closest soil sampling sites in the area (Moffat, 2013; Willmes et al., 2014). Finally, the underlying lithology of each site, as well as associated 87Sr/86Sr isotopes values, were extracted from the strontium isotope group of France map available at the IRHUM (Isotopic Reconstruction of Human Migration, www.irhumdatabase.com) website (Willmes et al., 2014, 2018). Willmes et al. (2018) grouped the lithological units observed in France into 5 isotope groups (Table 1). The western part of the study area is dominated by limestone and carbonaceous sediments (isotope group 2), ranging from middle-early Jurassic close to the Massif Central, to Eocene/Oligocene on the west, with Quaternary clastic sediments basins along rivers (Figure 3). The Massif Central area included on the eastern part of the study area is dominated by Cambrian paragneiss (isotope group 3) and orthogneiss (isotope group 5), and Carboniferous granite (isotope group 4). Oligocene to Pleistocene volcanic geological units (isotope group 1) are also present on the eastern edge of the study area. The lithological units observed at each sampling site were grouped within three of the five isotope groups: isotope group 2, limestone and other carbonaceous sediments; isotope group 3, sand and clay; and isotope group 4 sandstone and granite (Table 1, Figure 3).
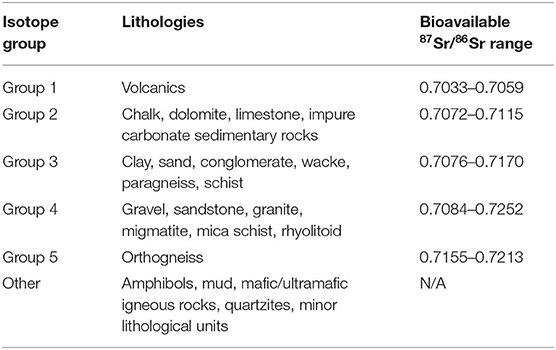
Table 1. Isotope groups with their corresponding lithologies and bioavailable 87Sr/86Sr range (adapted from Willmes et al., 2018).
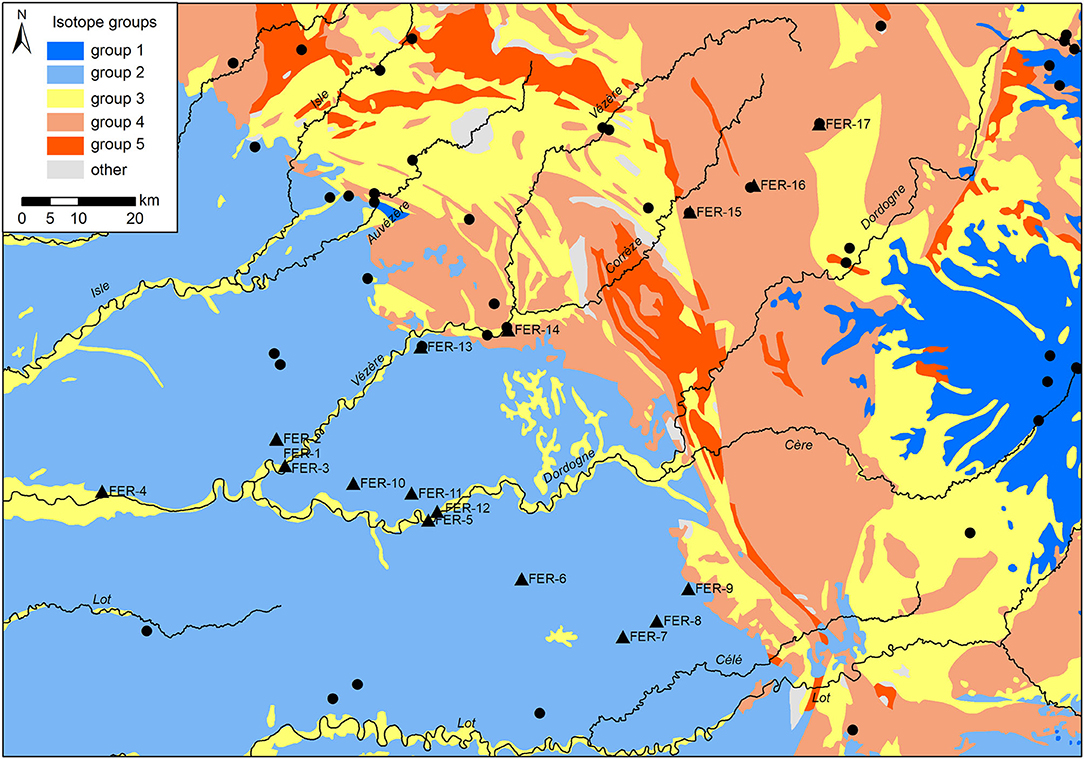
Figure 3. Lithological units of the study area classified by isotopes groups (adapted from Willmes et al., 2018). Sampling locations of the study are indicated (black triangles) as well as IRHUM sampling sites (black circles).
Statistical Analyses
A linear mixed-effects model was fitted to assess the effect of environmental variables on 87Sr/86Sr ratios within biological samples, with the sampling site as a random factor to control for inherent variability of each site (lme4 package in R software, Bates et al., 2015; R Core Team, 2019). In order to facilitate data analysis, strontium isotope ratios of biological and soil samples were presented in ε87Sr notation (Beard and Johnson, 2000), defined as:
where [87Sr/86Sr]sample is the 87Sr/86Sr values of the samples and [87Sr/86Sr]bulkearth is equal to 0.7045 (Beard and Johnson, 2000: 1050). To meet the assumptions of normality of the residuals and homogeneity of variance, the response variable was transformed from ε87Sr to -(ε87Sr)−1. Effects of the sample type (snail, grass, shrub, tree), the habitat (crop/forest), the distance to road, the distance to water and the elevation were tested separately. Variables were then regrouped into three categories: sample type, anthropogenic variables (habitat, distance to road), landscape structure (distance to water, elevation), and candidate models composed of various combinations of these categories were constructed. As the strontium isotope ratio of the underlying lithological units was expected to be the main driver of ε87Sr observed in both soils and biological samples (Bentley, 2006; Bataille et al., 2018), the ε87Sr lithological values of each site were included in all models in order to control for its effect. The list of all models tested in this study is provided in Supplementary Table 3. Variance inflation factor (VIF; Zuur et al., 2010) did not reveal multicollinearity among explanatory variables, as all variables had a VIF < 3. All models were ranked using AICc (Akaike's information criterion) and models with ΔAICc ≤ 2 were considered as equivalent (see Supplementary Table 3). From these models, parameter estimates, SE and 95% CI were calculated using model averaging (Burnham and Anderson, 2002). Effects of explanatory variables were considered significant when the 95% CI did not include 0.
To investigate the relationship between bioavailable ε87Sr in soil and the ε87Sr observed in plant according the type of plant (grass, shrub, tree), a linear mixed-effect model was fitted with -(ε87Sr)−1 of plant as the response variable, the ε87Sr of soil in interaction with the plant type as explanatory variables, and the plant sample sites as a random factor. As plant and soil sampling sites originated from two unrelated studies, and were paired post hoc, only plant sampling sites <1000 m away from soil sampling sites were kept for this analysis (sites: n = 10, plant samples: n = 39). Parameter estimates were provided with their SE and 95% CI.
Heterogeneous lithological units such as granite are expected to have a higher variability in their 87Sr/86Sr ratio than more homogeneous units, which may also be reflected in more variable bioavailable strontium values (Sillen et al., 1998; Willmes et al., 2018). Variability in sample 87Sr/86Sr ratio between lithological groups was compared using robust Levene test because 87Sr/86Sr ratio values did not follow a normal distribution. A robust Levene test is equivalent to an ANOVA performed on the absolute difference between the 87Sr/86Sr values of the samples and the median 87Sr/86Sr value of the group to which they belong. Therefore, a post-hoc Tukey's HSD (honestly significant difference) test on this difference was performed in order to compare all possible pairs of groups and identify how variability differed between groups. Differences in variability were also tested between sample type (snail, shrub, grass, trees) and between habitats.
Generating a Local 87Sr/86Sr Isoscape
In order to produce a new local 87Sr/86Sr isoscape of the study area, newly sampled sites were incorporated to an existing 87Sr/86Sr isoscape map of France from Willmes et al. (2018). This isoscape was generated by geostatistical interpolation of the 87Sr/86Sr values observed at sites recorded in the IRHUM database (Willmes et al., 2014, http://dx.doi.org/10.1594/PANGAEA.819142), using kriging with external drift (Willmes et al., 2018). The IRHUM database provides 87Sr/86Sr ratios for plant and soil samples from >800 locations in France (see Willmes et al., 2014 for details of samples and isotope analyses) as well as information about the underlying lithology (type, age). Kriging relies on the spatial autocorrelation between the sampled locations to interpolate value and this spatial autocorrelation is quantified by fitting a variogram model. Values at a given location are predicted by averaging known neighboring values weighted according to the variogram model. Kriging with external drift allows the accounting of an additional spatial trend defined by an auxiliary variable, such as elevation or other environmental variables.
Following Willmes et al. (2018), sites from the IRHUM database with both soil and plant samples were selected, excluding sites that were not representative of the lithology of their geographic area (minor geologic outcrop, riverbanks, sites with a strong anthropogenic influence). Strontium isotope groups defined by Willmes et al. (2018) were used as the auxiliary variable for the kriging with external drift. Lithological units of the surface geology map of France available in the IRHUM (Willmes et al., 2018) were regrouped according the isotope group to which they belonged (Table 1). The isotope group of each site in the IRHUM database were then extracted. Kriging with external drift was first performed on 498 IRHUM sample sites to obtain a plant isoscape and a soil isoscape of France, using their corresponding 87Sr/86Sr values, and the average isoscape was computed (Willmes et al., 2018). Then, the plant and soil data from the current study were added to the IRHUM database. A single mean 87Sr/86Sr value per sampling site was used for the plant samples (as kriging analysis requires a unique value per site). Eight of the 24 soil sites were already recorded in the IRHUM database but without plant data, and, consequently, were not included in the IRHUM-only isoscape. Snails 87Sr/86Sr values were not included in the isoscape model for comparability (as these are not included in the IRHUM database). Kriging was performed with the new plant and soil data and the average isoscape was computed, also at the scale of France. For each isoscape, the kriging was done using an exponential variogram model and a search neighborhood set to min = 5 and max = 50 (Willmes et al., 2018). Analysis was carried out with the gstat package in R (krige function, Gräler et al., 2016).
Results
Effects of Environmental Variables on ε87Sr of Biological Samples
The best models testing the effect of the environmental variables on ε87Sr within biological samples were the complete model that included ε87Sr lithological values, sample types, habitats, distance to road, distance to water and elevation, and the model with all variables but anthropogenic variables (Supplementary Table 3). Parameters estimates from the best mixed-effects models (random factor: sampling site) explaining variation in the 87Sr/86Sr ratio of biological samples, expressed in -(ε87Sr)−1, are shown in Table 2. As expected, ε87Sr observed in biological samples increased significantly with ε87Sr lithological values (Table 2).
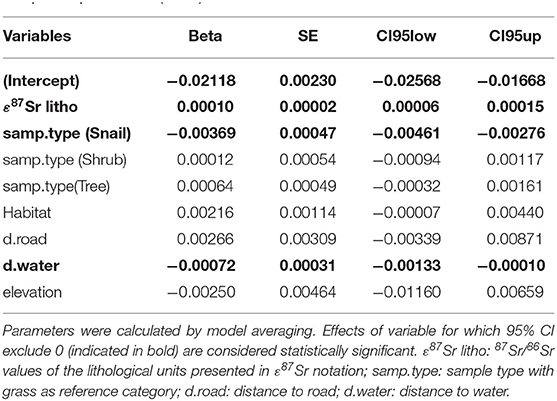
Table 2. Parameters estimates from the best mixed-effects models (random factor: sampling site) explaining variation in the 87Sr/86Sr ratio of biological samples expressed in -(ε87Sr)−1.
As also shown in Figure 4, using 87Sr/86Sr ratios, ε87Sr values were lower in snails than in plants, but did not differ systematically between grasses, shrubs and trees (Table 2). Sample ε87Sr values decreased as the distance of sampling site from water increased (Table 2), with a mean 87Sr/86Sr value of 0.7126 ± 0.0043 (SD) for sites within 0.5 km of water, and 0.7088 ± 0.0014 for sites distant by more than 1 km. Anthropogenic variables, habitats and distance to road, did not have a significant effect on ε87Sr of biological samples (Table 2). Both ε87Sr observed in snails and plants correlated significantly with ε87Sr expected from lithological units but we observed the highest correlation with plants (plants: n = 64, p < 0.001, R2 = 0.79, snails: n = 32, p < 0.001, R2 = 0.51). Based on soil sampling sites within 5 km of plant sampling sites, ε87Sr in soil was not correlated with distance to water (n = 19, p = 0.24, R2 = 0.08).
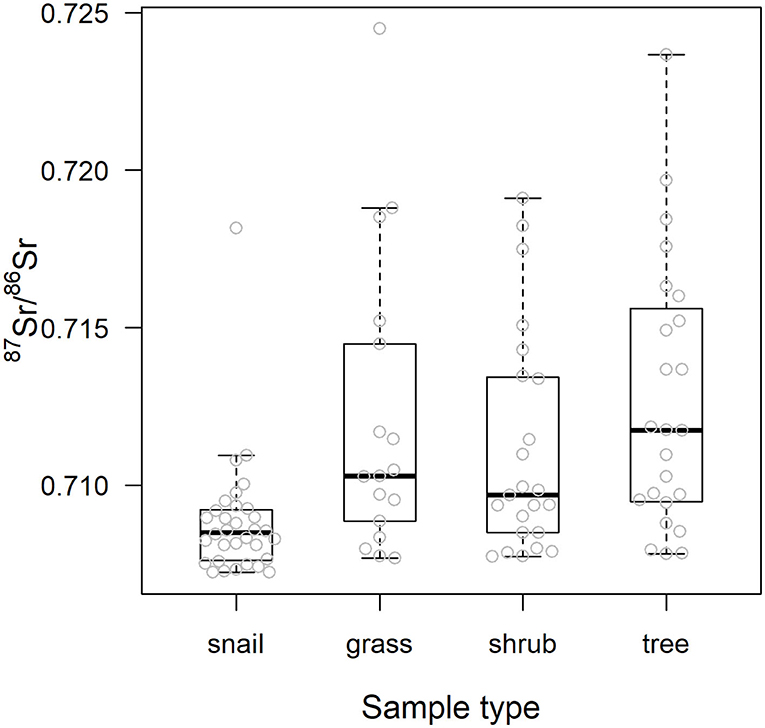
Figure 4. 87Sr/86Sr values of the biological samples by sample type. Center line and box edges are, respectively the median and the 1st and 3rd quartiles, and whiskers represent data points within the range quartile ± 1.5*(interquartile range). Raw values are displayed with gray dots.
Variability of Biological Sample 87Sr/86Sr Values Between Lithological Groups
Table 3 details the results of the post-hoc Tukey's HSD test for variables for which robust Levene tests identified a significant difference in the variability of the sample 87Sr/86Sr values between the different lithological groups. Variability in sample 87Sr/86Sr differed between lithological isotope groups (F = 4.86, p = 0.010, Figure 5) with a higher variability observed in samples from isotope group 4 (granites, sandstone) than in samples from isotope group 2 (limestone, sediment, p = 0.01, Table 3). The samples from isotope group 3 (sand, clay) presented an intermediate variability in their 87Sr/86Sr values but not significantly different from group 2 (p = 0.38) and group 4 (p = 0.25). Variability in 87Sr/86Sr did not differ whether we compared samples from the forest and agricultural habitats (F = 0.01, p = 0.907) or, in more detail, from the five different class of habitats (F = 1.28, p = 0.283). Variability in 87Sr/86Sr plant and snail samples was significantly different (F = 12.25, p < 0.001, Figure 2), with a lower variability in snails (p < 0.001, Table 3). Specifically, the variability in snail 87Sr/86Sr was lower than the variability observed in that of grasses and tree leaves but did not differ from variability observed in shrubs (Table 3). Grass, shrubs and trees presented the same variability in 87Sr/86Sr (Table 3). However, the relationship between soil and plant 87Sr/86Sr, expressed in ε87Sr, varied according the plant type, with the increase of 87Sr/86Sr of grasses as 87Sr/86Sr in soils increased being significantly stronger than in trees in particular (Table 4, Figure 6). According to the predictions of the model, an increase from 0.7100 to 0.7200 in soil 87Sr/86Sr would result in an increase from 0.7090 to 0.7206 and from 0.7094 to 0.7172, respectively for grass and tree 87Sr/86Sr. Shrubs presented an intermediate increase (Table 4, Figure 6).
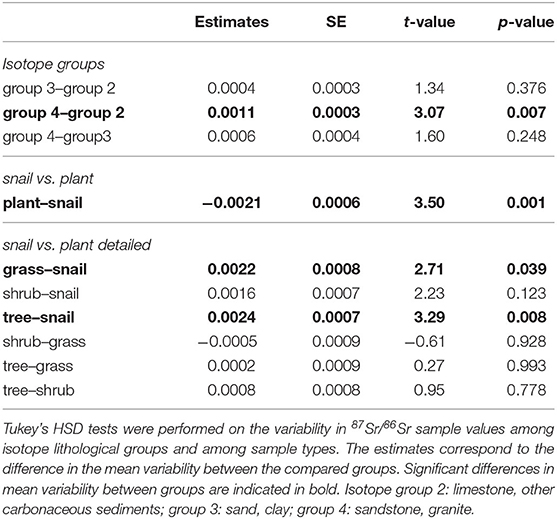
Table 3. Post-hoc Tukey's HSD test for variables for which robust Levene tests identified a significant difference in the variability of the 87Sr/86Sr sample values between groups.
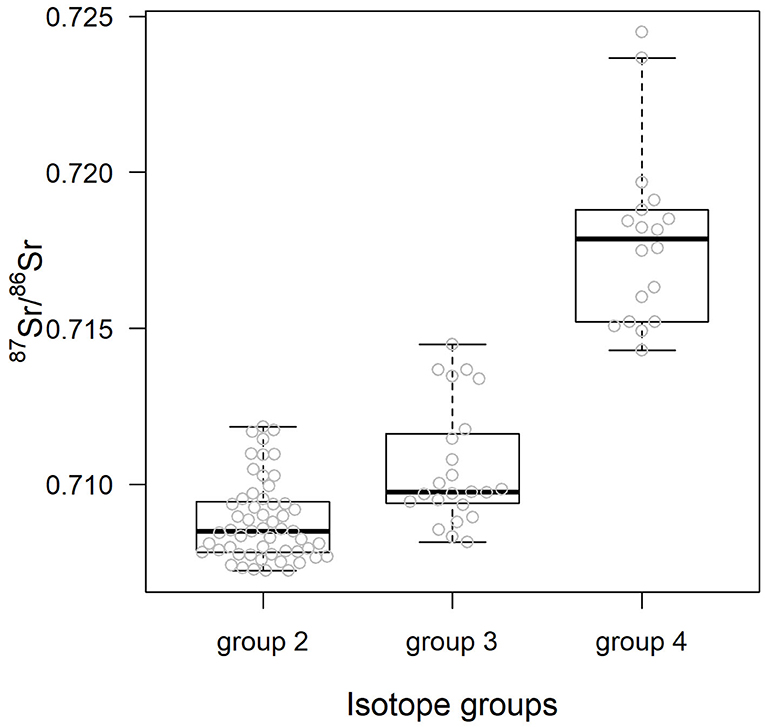
Figure 5. 87Sr/86Sr values of the biological samples according the isotope group of the lithological unit underlying the sample sites. Center line and box edges are, respectively the median and the 1st and 3rd quartiles, and whiskers represent data points within the range quartile ± 1.5*(interquartile range). Raw values are displayed with gray dots.
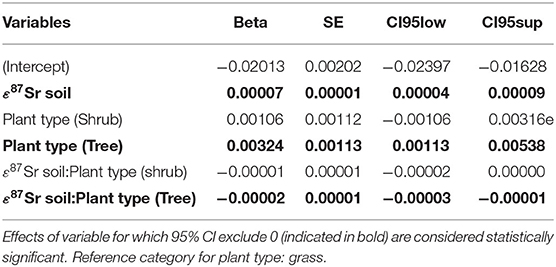
Table 4. Parameters estimates from the mixed-effects model (random factor: sampling site) explaining variation in the 87Sr/86Sr ratio of biological samples, expressed in -(ε87Sr)−1, according 87Sr/86Sr ratio of soil samples, expressed in ε87Sr, in interaction with the type of plant.
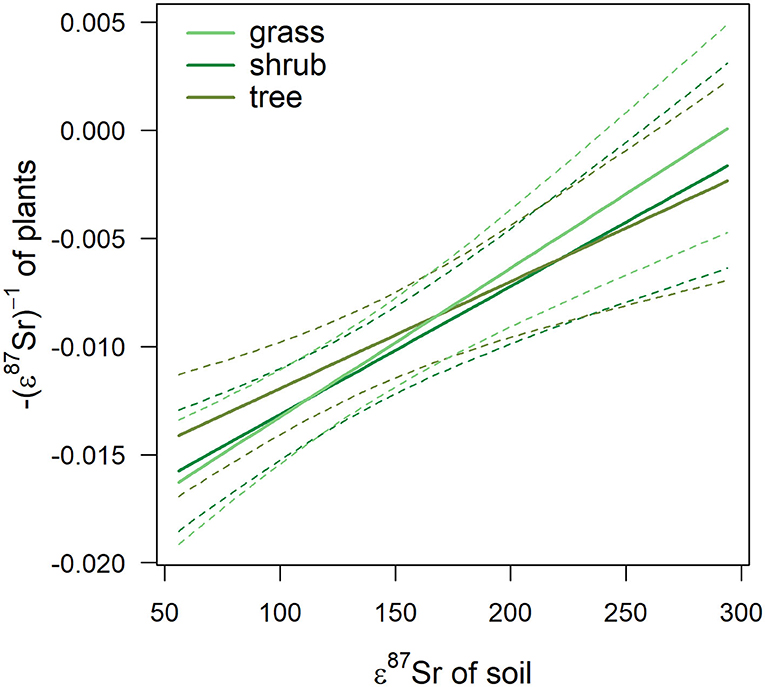
Figure 6. Effect of 87Sr/86Sr values of soil, expressed in ε87Sr, on the 87Sr/86Sr values of plant, expressed in -(ε87Sr)−1, according to plant type. Fitted trends are presented with 95% CI (dashed lines).
Modified Isoscape
Adding 17 plant sampling sites and 24 soil sampling sites to the 498 IRHUM sites did not improved the root-mean-square error (RMSE) of the kriging with external drift using the IRHUM sites for the whole of France (RMSE: PlantIRHUM = 0.0033; PlantIRHUM+studysites = 0.0034; SoilIRHUM = 0.0034; SoilIRHUM+studysites = 0.0033). However, at the local scale, the kriging prediction error over the study area decreased with the new sites (Figures 7c,d). Moreover, including the new sites to the isoscape led to lower 87Sr/86Sr values in the lowland along the Vézère and the Dordogne rivers and slightly higher 87Sr/86Sr values at higher elevation (Figures 7a,b) than observed in the IRHUM database (Willmes et al., 2018). Plant and soil 87Sr/86Sr isoscapes used to compute the average isoscape were relatively similar, both presenting the decrease in kriging prediction error rate, as well as the changes in lowland and high elevation 87Sr/86Sr values (Supplementary Figure 4).
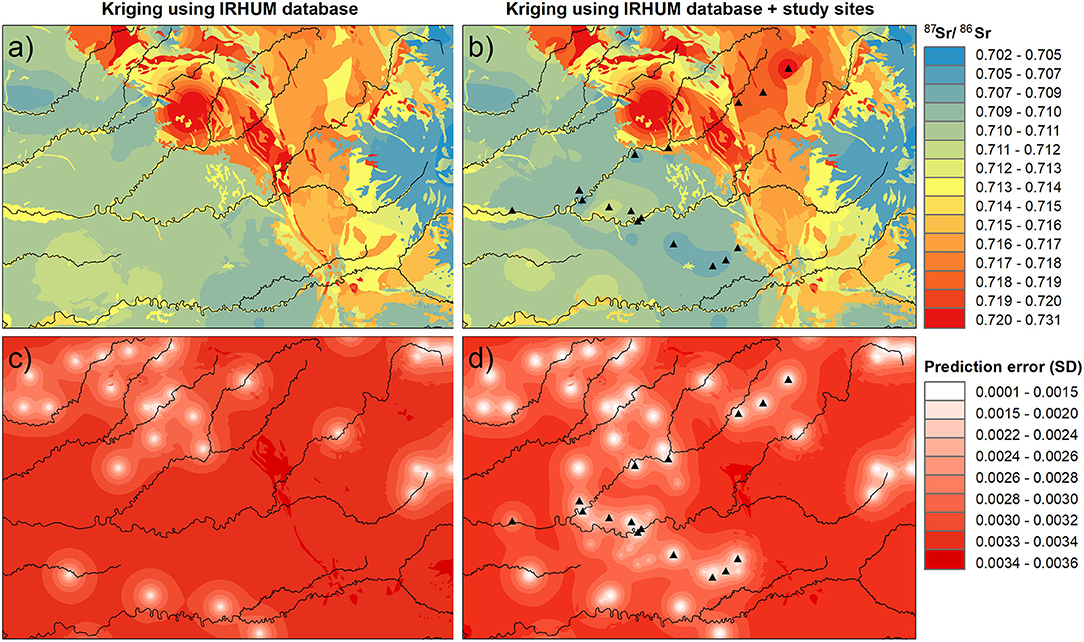
Figure 7. Averaged 87Sr/86Sr isoscapes of plant and soil 87Sr/86Sr isoscapes generated by kriging with external drift using IRHUM sites (a) and IRHUM and sites in this study (b), and their respective kriging prediction error maps (c,d). Kriging prediction error is given as the standard deviation. Plant sampling sites are indicated by triangles.
Discussion
The results explored above highlight several trends in the 87Sr/86Sr values of plants and malacofauna analyzed in this study, both inter- and intra- site, and in comparison to each other and to values expected based on underlying lithology. As expected, a positive relationship between the 87Sr/86Sr values of the lithological units and that of the biological samples analyzed (bioavailable 87Sr/86Sr) was observed. This is in agreement with the findings of other bioavailability studies (e.g., Maurer et al., 2012; Willmes et al., 2018; Snoeck et al., 2020), which have shown that—despite there being multiple environmental factors known to influence bioavailable strontium—the type and age of the lithology are the main driver of the spatial distribution of bioavailable strontium (Bentley, 2006; Bataille et al., 2018).
Animals and plants acquire 87Sr/86Sr from different sources, and consequently some sample types may be more relevant to the landscape mapping of bioavailable 87Sr/86Sr than others (Evans et al., 2010; Maurer et al., 2012). Here, 87Sr/86Sr in snail shells was lower than 87Sr/86Sr in plants across all sampling locations, and plants of all types generally had a better correlation with the 87Sr/86Sr values expected from the lithology and soils. The snail taxa included in this study feed primarily on living and dead plant material (e.g., leaf litter, see Williamson and Cameron, 1976). While the strontium isotope ratio of leaf litter should reflect the composition of exchangeable pools within soil, recent studies have shown 87Sr/86Sr (and thus Ca) sources in land snails can differ, and be obtained through sources other than major food sources (i.e., precipitation or stemflow) (Ohta and Saeki, 2020). Previous strontium bioavailability studies have also reported low 87Sr/86Sr values for snail shells, with a bias toward rainwater (Evans et al., 2010) and the 87Sr/86Sr values of soil carbonates (Maurer et al., 2012). The results of the current study agree with those of others and suggest malacofauna are a less suitable analyte than plants when mapping bioavailable strontium.
In the current study, differences in 87Sr/86Sr were also expected between plant types due to differences in root depth (Poszwa et al., 2004; Maurer et al., 2012) as water and nutrient uptake will occur in different soil horizons and soil 87Sr/86Sr can vary with depth. Mineral weathering is the main source of 87Sr/86Sr in deep soil horizons, but topsoil can be influenced by external sources such as atmospheric deposition or the application of fertilizers (Prohaska et al., 2005; Maurer et al., 2012). While in this study 87Sr/86Sr values in grasses, shrubs and trees were broadly similar, different rates of increase in plant 87Sr/86Sr as soil 87Sr/86Sr increased were observed between grasses and trees across different sites, with intermediate values for shrubs. Grasses thus appear to be more representative of bioavailable 87Sr/86Sr values of soil (Figure 8), which is in agreement with the findings of other studies (e.g., Maurer et al., 2012). Due to their deep and wide root systems, trees at the same location can display variable 87Sr/86Sr values in response to local variation in soil composition and mineral weathering rates as they can draw 87Sr/86Sr in different pools (Aguzzoni et al., 2019). On the other hand, water and nutrient uptake of grasses is mostly limited to topsoil horizons. External sources of 87Sr/86Sr, such as rainfall (Evans et al., 2010), occurs at a large scale and—although rainwater values inland deviate from sea salts and can be similar to terrestrial underlying values due to the incorporation of atmospheric dust (e.g., Négrel et al., 2007; Raiber et al., 2009)—this could still serve to decrease the 87Sr/86Sr variability of the topsoil horizon between sites. Soil samples were taken from the topsoil horizon (Willmes et al., 2014) and the lower rate of increase in trees may be linked to their use of multiple and deeper 87Sr/86Sr sources. Therefore, targeting a specific plant category in a sampling protocol would reflect the bioavailable 87Sr/86Sr values from a particular soil horizon. Sampling multiple species with different root systems/root depths at a same location should be more representative of the bulk soil bioavailable 87Sr/86Sr. Beyond isoscape mapping, variations in plant 87Sr/86Sr with root depth could have implications for the reconstruction of human and animal mobility patterns in archaeology. Humans make choices about what they consume and when and where they source those foods, and are thus not passive recipients of environmental strontium (Johnson et al., 2019). Furthermore, while it has been long known that strontium inputs are normalized to dietary calcium budgets (e.g., Comar, 1963), the influence of dietary composition on 87Sr/86Sr in humans has received little attention. While it is currently accepted that the dominant contributor of metabolized strontium in both herbivores and omnivores originates from plant foods (as opposed to being derived from animal sources or water) (see review in Montgomery, 2010), little consideration has been given to the potential influence of different types of plant foods in the diet. Grazing animals, for example, would be expected to represent grass and therefore topsoil values, and perhaps be more directly relatable to isoscapes generated using such proxy data. The same may be true of human groups eating grain-based agricultural diets, and human tissues could reasonably be expected to show lower intra-group variability were those individuals subsisting on locally-grown grains. However, hunter-gatherer groups reliant on a wider range of plant food sources including berries, fruits or tubers, as well as greens (as well as animal foods, in varying quantities) could be incorporating strontium from a variety of different sources and catchments, even locally. Furthermore, these different plant foods may have different concentrations of strontium (Millour et al., 2012). Although any influence may not substantial enough to affect the inference of provenance, further studies are required to determine if a “soil profile” influence could be apparent in mammalian skeletal bioapatite. Experimental studies on browser and grazers, or even frugivorous and tree-leaf feeding species, with similar home range sizes from modern ecosystems would be an important first step in determining the extent of possible effects of different types of local plant foods on the 87Sr/86Sr of hard tissues. To date, however, experimental or controlled feeding studies incorporating 87Sr/86Sr have been rare (e.g., Lewis et al., 2017). Other experiments, for example concerning the extent to which the 87Sr/86Sr ratios of skeletal tissues are affected by marine resource consumption or by diets very low in plant foods would also be welcome in archaeology.
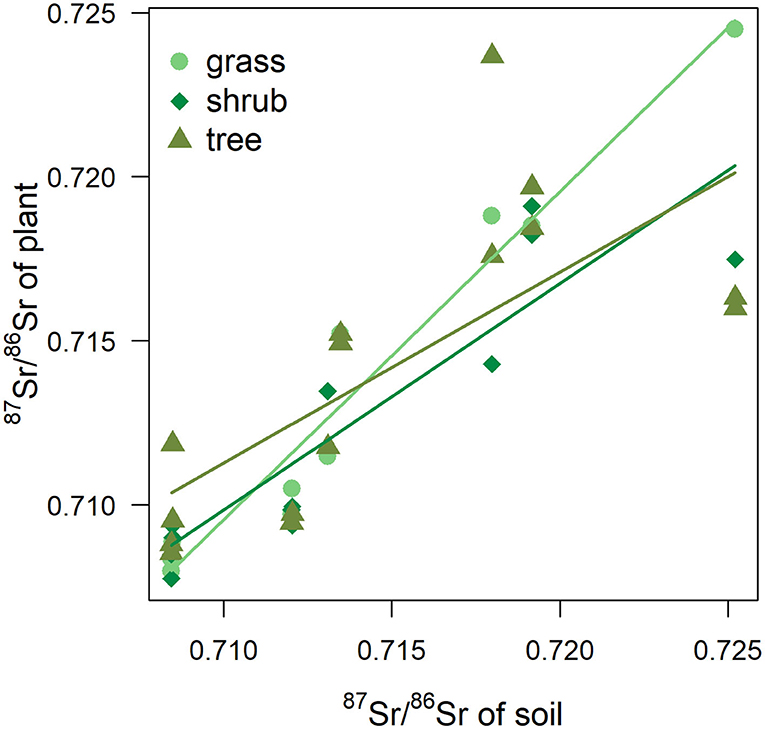
Figure 8. 87Sr/86Sr values of plant according 87Sr/86Sr values of soil. Fitted trends correspond to simple linear regressions for each plant type (grass, shrub, tree).
Beside differences in intra-site 87Sr/86Sr variability between sample type, samples from sites with different underlying lithology did not show the same 87Sr/86Sr variability. As a product of soil mineral weathering, variability in soil 87Sr/86Sr increases with the heterogeneity of the geological substrates (Bataille et al., 2018; Willmes et al., 2018). It follows that such variability in soil 87Sr/86Sr should then be reflected in plants (e.g., Hartman and Richards, 2014; Aguzzoni et al., 2019). Indeed, in the current study, a positive correlation between sample variability and substrate variability (based on underlying lithology) was observed. Variability in bioavailable strontium values from different plants and snails at the same site was lower for sites located on homogeneous geologic substrates, such as limestones, than for sites located on heterogeneous geologic substrates, such as granites. These findings suggest that—when undertaking bioavailability studies—sampling efforts should focus on areas of expected greater heterogeneity to capture the whole range of bioavailable 87Sr/86Sr. This heterogeneity can be broadly predicted from lithological maps, helping to guide sampling. The argument for more intensive and multi-proxy sampling (i.e., soils, water, plant leaves) in geologically complex areas has been made by others (e.g., Ladegaard-Pedersen et al., 2020); here we add that diverse sampling even within the same broad category of sample type as explained above (e.g., tree leaves, shrub leaves, grasses) may help to capture such variability.
Beyond lithology, the influence of other aspects of landscape structure, such as elevation and distance to water, was also explored in this study. While bioavailable 87Sr/86Sr values were generally increased in areas of higher elevation, when controlling for the 87Sr/86Sr values of the lithological units themselves, no effect of elevation on bioavailable 87Sr/86Sr values was observed. This is because, in this study area, the lithological units presenting high 87Sr/86Sr values, such as granite, also presented a higher elevation than lithological units with low 87Sr/86Sr values. On the other hand, distance to water seemed to affect the 87Sr/86Sr values of samples. A decrease in 87Sr/86Sr values was observed as distance to rivers increased, suggesting river water 87Sr/86Sr values in the region are higher than surrounding bedrock and/or that rivers are depositing sediments or other materials of higher 87Sr/86Sr values on areas close to rivers. Higher values in the rivers—of either water or suspended material—is consistent with the fact that the Vézère and the Dordogne rivers originate in the Massif Central, which is dominated by granite and metamorphic bedrocks that tend to have elevated 87Sr/86Sr ratios. Indeed, the radiogenic influence of the Massif Central bedrock was also observed in the Loire river in a previous study (Négrel et al., 2003). Mineral weathering is a major source of 87Sr/86Sr upstream (Pierson-Wickmann et al., 2009; Maurer et al., 2012). At lower elevations, the relationship between river content and local bedrock is blurred further, due to the carrying of upstream rocks and solids (Bentley, 2006, 144). The absence of a relationship between distance to river and the 87Sr/86Sr ratio of soils suggests that the influence of rivers on the 87Sr/86Sr of plants was, however, not due to changes in soil/sediment composition, but due to the addition of strontium from river water itself. Indeed, other studies have observed variation in riverine 87Sr/86Sr values based on the size and lithological diversity of the watershed (Hegg et al., 2013).
Anthropogenic input of 87Sr/86Sr in the ecosystem is a major concern in bioavailability studies, and therefore for archaeological applications of strontium isotope analysis. For example, dust derived from road surfaces, such as that produced as a consequence of use by heavy vehicles, can modify topsoil composition (Prohaska et al., 2005, 246), and the use of mineral fertilizers or carbonate amendment (liming) has been demonstrated to lower soil 87Sr/86Sr (Pierson-Wickmann et al., 2009; Maurer et al., 2012). Lime has typically low 87Sr/86Sr values (~0.7087), and analysis of deionized water-soluble strontium from three fertilizers in a French study determined strontium isotope ratios ranging from 0.70794 to 0.70830 (Négrel, 1999, 155). However, unlike some other bioavailability studies (e.g., Maurer et al., 2012; Thomsen and Andreasen, 2019), no differences were detected between plant samples collected in locations proximal to agricultural fields compared to those collected in forests in the current study. The landscape of the study area was a complex mosaic with small fields and forest patches, which may suggest the influence of fertilizers was either minimal due to a lack of significant local use or may have been homogenized across the landscape through runoff.
The addition of the new data to that in the IRHUM database did not improve the overall performance of the already existing France isoscape (Willmes et al., 2018), as south western France was already well covered by IRHUM with a low prediction error compared to areas with lower sampling density (Willmes et al., 2018). However, it has served to reduce the predicted error and refine the isoscape for the study area. Notably this addition has resulted in an increased difference in 87Sr/86Sr values between the areas of low and high elevation in this important archaeological region. Increased contrast between areas with lower uncertainty can help to achieve better isotopic discrimination between regions and thus in archaeological provenance studies using strontium isotopes (Bataille et al., 2018). This demonstrates that more extensive bioavailability mapping is essential, can increase resolution at the local scale in regional studies, and highlights the benefit of combining large scale mapping and modeling with focused sampling of specific areas of interest. More broadly, the current study in particular highlights the importance of focusing efforts on areas of heterogeneous geological substrate when undertaking bioavailability studies—in this case study, the granitic upland areas. Potential environmental and anthropogenic effects must also be considered. Despite no visible anthropogenic effects on the 87Sr/86Sr sample values in this study, modern and historic human activity should always be born in mind when seeking to apply modern datasets to the study of past landscape use (Maurer et al., 2012; Thomsen and Andreasen, 2019).
Conclusions
The results of this study confirm the consistency between plant and snail 87Sr/86Sr values with lithological unit and soil 87Sr/86Sr values observed in other studies. While no obvious impact of fertilizers or other modern contaminants on the samples included within this study could be discerned, we note these remain an ongoing concern for bioavailability studies. In the current study, plants appeared to be a more reliable analyte to describe 87Sr/86Sr bioavailability than snails. Results also highlight that sampling should ideally not only focus on one plant category but should integrate plants with different root systems/root depths in order to capture the bulk soil variability of any particular area. These data also serve to emphasize that dietary composition, even of type of plant consumed, could, theoretically, contribute to strontium isotope variability in the tissues of local humans or animals, and highlights the need for experimental studies.
In light of the data explored here, we also argue that sampling strategies in bioavailability studies should account for spatial heterogeneity in predicted bioavailable 87Sr/86Sr distribution, and sampling should ideally be more intensive in areas where high variability in bioavailable 87Sr/86Sr values is expected. This may, in many circumstances, be predictable based on lithological maps (e.g., areas with lithologies of more heterogeneous composition could be sampled more intensively than homogeneous deposits). Our results also demonstrate that the integration of new regional datasets into already existing datasets can improve overall isoscape accuracy and refine prediction at the local scale. Such datasets can in turn then be included in broader scale studies (e.g., Bataille et al., 2018), although we note that the integration of multiple data sources from different locations face disparity in sampling methods and analyses. While far from being a proposal for a unified framework of sampling, we suggest the sampling of plants of differing root depth at each sampling location (incorporating grasses, shrub and tree leaves, for example) can be a practical step in better representing variability in bioavailable 87Sr/86Sr on a local level.
Data Availability Statement
The original contributions presented in the study are included in the article/Supplementary Material, further inquiries can be directed to the corresponding author/s.
Author Contributions
KB designed the study, undertook field sampling, sample processing and laboratory analyses (plants), and wrote the initial draft of manuscript with ML. ML designed data treatment and undertook data analyses, including all statistics and produced all images. MW, IM, and RG undertook field sampling and laboratory analyses and isotope measurements (soils). MM and SW undertook identifications of malacofauna and plants respectively. KJ undertook isotope measurements (snails and plants). All the authors provided critical review of and contributions to subsequent versions of the manuscript.
Funding
This research was funded by the Max Planck Society and a Leverhulme Trust grant to KB (RPG-2017-410), with additional support from Australian Research Council Discovery grants DP0664144 and DP110101417 to RG. KJ thanks the ERC ARCHEIS 803676, and IM thanks Australian Research Council Discovery Early Career Award (DE160100703), for salary support during production of this manuscript.
Conflict of Interest
The authors declare that the research was conducted in the absence of any commercial or financial relationships that could be construed as a potential conflict of interest.
Acknowledgments
We thank Annabell Reiner and Sven Steinbrenner (MPI-EVA) for technical and practical support with preparation and analysis of samples. We thank the La Ferrassie Team for support during field sampling and project development, especially Harold Dibble, Shannon McPherron (MPI-EVA), Teresa Steele (UC Davies), Vera Aldeias (MPI-EVA, University of Algarve), Paul Goldberg (University of Wollongong, University Tübingen), Dennis Sandgathe (Simon Fraser University, University of Pennsylvania), Alain Turq (Musée national de Préhistoire, CNRS), and Jean-Jacques Hublin (MPI-EVA), as well as Mike Richards (SFU). Special thanks to Daphne Katranides and Aaron Katranides. We also thank the editor (TP) and two reviewers whose constructive comments greatly improved this manuscript.
Supplementary Material
The Supplementary Material for this article can be found online at: https://www.frontiersin.org/articles/10.3389/fevo.2020.579473/full#supplementary-material
Footnotes
1. ^Institut National de l'Information Géographique et Forestière road: ROUTE 500 edition 191, Hydrography: DB TOPO v3. Available online at: https://geoservices.ign.fr/.
References
Aguzzoni, A., Bassi, M., Robatscher, P., Scandellari, F., Tirler, W., and Tagliavini, M. (2019). Intra- and intertree variability of the 87Sr/86Sr ratio in apple orchards and its correlation with the soil 87Sr/86Sr ratio. J. Agricult. Food Chem. 67, 5728–5735. doi: 10.1021/acs.jafc.9b01082
Bahn, P. (1977). Seasonal migration in South-west France during the late glacial period. J. Arch. Sci. 4, 245–257. doi: 10.1016/0305-4403(77)90092-9
Balasse, M., Ambrose, S. H., Smith, A. B., and Price, T. D. (2002). The seasonal mobility model for prehistoric herders in the South-western Cape of South Africa assessed by isotopic analysis of sheep tooth enamel. J. Arch. Sci. 29, 917–932. doi: 10.1006/jasc.2001.0787
Balter, V., Braga, J., Télouk, P., and Thackeray, J. F. (2012). Evidence for dietary change but not landscape use in South African early hominins. Nature 489, 558–560. doi: 10.1038/nature11349
Bataille, C. P., and Bowen, G. J. (2012). Mapping 87Sr/86Sr variations in bedrock and water for large scale provenance studies. Chem. Geol. 304–305, 39–52. doi: 10.1016/j.chemgeo.2012.01.028
Bataille, C. P., Brennan, S. R., Hartmann, J., Moosdorf, N., Wooller, M. J., and Bowen, G. J. (2014). A geostatistical framework for predicting variations in strontium concentrations and isotope ratios in Alaskan rivers. Chem. Geol. 389, 1–15. doi: 10.1016/j.chemgeo.2014.08.030
Bataille, C. P., von Holstein, I. C. C., Laffoon, J. E., Willmes, M., Liu, X.-M., and Davies, G. R. (2018). A bioavailable strontium isoscape for Western Europe: A machine learning approach. PLoS ONE 13:e0197386. doi: 10.1371/journal.pone.0197386
Bates, D., Mächler, M., Bolker, B., and Walker, S. (2015). Fitting linear mixed-effects models using lme4. 2015 67, 48. doi: 10.18637/jss.v067.i01
Beard, B. L., and Johnson, C. M. (2000). Strontium isotope composition of skeletal material can determine the birth place and geographic mobility of humans and animals. J. Foren. Sci. 45, 1049–1061. doi: 10.1520/JFS14829J
Bentley, R. A. (2006). Strontium isotopes from the earth to the archaeological skeleton: a review. J. Arch. Method Theor. 13, 135–187. doi: 10.1007/s10816-006-9009-x
Bentley, R. A., and Knipper, C. (2005). Transhumance at the Early Neolithic Settlement at Vaihingen (Germany). Antiquity 79, Online Project Gallery.
Bentley, R. A., Pietrusewsky, M., Douglas, M. T., and Atkinson, T. C. (2005). Matrilocality during the prehistoric transition to agriculture in Thailand? Antiquity 79, 865–881. doi: 10.1017/S0003598X00115005
Britton, K., Grimes, V., Niven, L., Steele, T., McPherron, S., Soressi, M., et al. (2011). Strontium isotope evidence for migration in late Pleistocene Rangifer: implications for Neanderthal hunting strategies at the Middle Palaeolithic site of Jonzac, France. J. Hum. Evol. 61, 176–185. doi: 10.1016/j.jhevol.2011.03.004
Burnham, K. P., and Anderson, D. R. (2002). A Practical Information-Theoretic Approach. Model Selection and Multimodel Inference. 2nd ed. New York, NY: Springer.
Comar, C. L. (1963). “Some over-all aspects of strontium-calcium discrimination,” in The Transfer of Calcium and Strontium Across Biological Membranes, ed R. H. Wasserman (London: New York, NY: Academic Press), 405–417.
Copeland, S. R., Sponheimer, M., de Ruiter, D. J., Lee-Thorp, J. A., Codron, D., le Roux, P. J., et al. (2011). Strontium isotope evidence for landscape use by early hominins. Nature 474, 76–78. doi: 10.1038/nature10149
Copeland, S. R., Sponheimer, M., le Roux, P. J., Grimes, V., Lee-Thorp, J. A., de Ruiter, D. J., et al. (2008). Strontium isotope ratios (87Sr/86Sr) of tooth enamel: comparison of solution and laser ablation multicollector inductively coupled plasma mass spectrometry methods. Rap. Commun. Mass Spectr. 22, 3187–3194. doi: 10.1002/rcm.3717
Copernicus Land Monitoring Service. (2018). Copernicus Land Monitoring Service. European Environment Agency (EEA). Available online at: https://land.copernicus.eu/
Cossignani, T., and Cossignani, V. (1995). Atlante delle Conchiglie Terrestri e Dulciacquicole Italiane. Ancona: L'Informatore Piceno.
Deniel, C., and Pin, C. (2001). Single-stage method for the simultaneous isolation of lead and strontium from silicate samples for isotopic measurements. Anal. Chim. Acta 426, 95–103. doi: 10.1016/S0003-2670(00)01185-5
Ericson, J. E. (1985). Strontium isotope characterization in the study of prehistoric human ecology. J. Hum. Evol. 14, 503–514. doi: 10.1016/S0047-2484(85)80029-4
Evans, J. A., Mee, K., Chenery, C. A., Cartwright, C. E., Lee, K. A., and Marchant, A. P. (2018). User Guide for the Biosphere Isotope Domains GB (Version 1) Dataset and Web Portal. Nottingham: British Geological Survey, 21.
Evans, J. A., Montgomery, J., Wildman, G., and Boulton, N. (2010). Spatial variations in biosphere 87Sr/86Sr in Britain. J. Geol. Soc. 167, 1–4. doi: 10.1144/0016-76492009-090
Goemaere, E., Honings, J., Jungels, C., Golitko, M., Degryse, P., and Schneider, J. (2013). Discerning geological and geographical sources of Belgian Upper Paleolithic fluorites by rare earth elements and Sr-isotopic geochemistry. J. Arch. Sci. 40, 2892–2901. doi: 10.1016/j.jas.2013.02.029
Gordon, B. C. (1988). Of Men and Reindeer Herds in French Magdalenian Prehistory. Oxford: British Archaeological Reports (International Series 390). doi: 10.30861/9780860545040
Gräler, B., Pebesma, E., and Heuvelink, G. (2016). Spatio-Temporal Interpolation using gstat. R J. 8:204–18. doi: 10.32614/RJ-2016-014
Hartman, G., and Richards, M. (2014). Mapping and defining sources of variability in bioavailable strontium isotope ratios in the Eastern Mediterranean. Geochim. Cosmochim. Acta 126, 250–264. doi: 10.1016/j.gca.2013.11.015
Hegg, J. C., Kennedy, B. P., and Fremier, A. K. (2013). Predicting strontium isotope variation and fish location with bedrock geology: understanding the effects of geologic heterogeneity. Chem. Geol. 360–361, 89–98. doi: 10.1016/j.chemgeo.2013.10.010
Joannes-Boyau, R., Adams, J. W., Austin, C., Arora, M., Moffat, I., Herries, A. I. R., et al. (2019). Elemental signatures of Australopithecus africanus teeth reveal seasonal dietary stress. Nature 572, 112–115. doi: 10.1038/s41586-019-1370-5
Johnson, C. M., and Fridrich, C. J. (1990). Nonmonotonic Chemical and O, Sr, Nd, and Pb Isotope Zonations and Heterogeneity in the Mafic to Silicic-Composition Magma Chamber of the Grizzly Peak Tuff, Colorado. Contrib. Mineral. Petrol. 105, 677–690. doi: 10.1007/BF00306533
Johnson, L., Montgomery, J., Evans, J., and Hamilton, E. (2019). Contribution of strontium to the human diet from querns and millstones: an experiment in digestive strontium isotope uptake. Archaeometry 61, 1366–1381. doi: 10.1111/arcm.12485
Julien, M.-A., Bocherens, H., Burke, A., Drucker, D., Patou-Mathis, M., Krotova, O., et al. (2012). Were European steppe bison migratory? 18O, 13C and Sr intra-tooth isotopic variations applied to a palaeoethological reconstruction. Q. Int. 271:106–119. doi: 10.1016/j.quaint.2012.06.011
Kerney, M. P. (1999). Atlas of Land and Freshwater Molluscs of Britain and Ireland. Colchester: Harley Books.
Knudson, K. J., Price, T. D., Buikstra, J. E., and Blom, D. E. (2004). The use of strontium isotope analysis to investigate tiwanaku migration and mortuary ritual in Bolivia and Peru. Archaeometry 46, 5–18. doi: 10.1111/j.1475-4754.2004.00140.x
Ladegaard-Pedersen, P., Achilleos, M., Dörflinger, G., Frei, R., Kristiansen, K., and Frei, K. M. (2020). A strontium isotope baseline of Cyprus. Assessing the use of soil leachates, plants, groundwater and surface water as proxies for the local range of bioavailable strontium isotope composition. Sci Total Environ. 708, 134714. doi: 10.1016/j.scitotenv.2019.134714
Laffoon, J. E., Plomp, E., Davies, G. R., Hoogland, M. L. P., and Hofman, C. L. (2015). The movement and exchange of dogs in the prehistoric caribbean: an isotopic investigation. Int. J. Osteoarchaeol. 25, 454–465. doi: 10.1002/oa.2313
Leach, S., Lewis, M., Chenery, C., Müldner, G., and Eckardt, H. (2009). Migration and diversity in Roman Britain: a multidisciplinary approach to the identification of immigrants in Roman York, England. Am. J. Phys. Anthropol. 140, 546–561. doi: 10.1002/ajpa.21104
Lewis, J., Pike, A. W. G., Coath, C. D., and Evershed, R. P. (2017). Strontium concentration, radiogenic (87Sr/86Sr) and stable (δ88Sr) strontium isotope systematics in a controlled feeding study. Sci. Technol. Archaeol. Res. 3, 45–57. doi: 10.1080/20548923.2017.1303124
Lugli, F., Cipriani, A., Capecchi, G., Ricci, S., Boschin, F., Boscato, P., et al. (2019). Strontium and stable isotope evidence of human mobility strategies across the Last Glacial Maximum in southern Italy. Nat. Ecol. Evol. 3, 905–911. doi: 10.1038/s41559-019-0900-8
Maurer, A.-F., Galer, S. J. G., Knipper, C., Beierlein, L., Nunn, E. V., Peters, D., et al. (2012). Bioavailable 87Sr/86Sr in different environmental samples - effects of anthropogenic contamination and implications for isoscapes in past migration studies. Sci. Total Environ. 433, 216–229. doi: 10.1016/j.scitotenv.2012.06.046
Millour, S., Noël, L., Chekri, R., Vastel, C., Kadar, A., Sirot, V., et al. (2012). Strontium, silver, tin, iron, tellurium, gallium, germanium, barium and vanadium levels in foodstuffs from the Second French Total Diet Study. J. Food Comp. Anal. 25, 108–129. doi: 10.1016/j.jfca.2011.10.004
Moffat, I. (2013). Spatially Resolved Strontium Isotope Micro-Analysis of Lower and Middle Palaeolithic Fauna from Archaeological Sites in Israel and Southern France. Unpublished Ph.D. Thesis: The Australian National University.
Montgomery, J. (2010). Passports from the past: Investigating human dispersals using strontium isotope analysis of tooth enamel. Ann. Hum. Biol. 37, 325–346. doi: 10.3109/03014461003649297
Négrel, P. (1999). Geochemical study of a granitic area - the margeride mountains, france: chemical element behavior and 87Sr/86Sr constraints. Aquat. Geochem. 5, 125–165. doi: 10.1023/A:1009625412015
Négrel, P., Guerrot, C., and Millot, R. (2007). Chemical and strontium isotope characterization of rainwater in France: influence of sources and hydrogeochemical implications. Isot. Environ. Health Stud. 43, 179–196. doi: 10.1080/10256010701550773
Négrel, P., Petelet-Giraud, E., Barbier, J., and Gautier, E. (2003). Surface water-groundwater interactions in an alluvial plain: chemical and isotopic systematics. J. Hydrol. 277, 248–267. doi: 10.1016/S0022-1694(03)00125-2
Ohta, T., and Saeki, I. (2020). Comparisons of calcium sources between arboreal and ground-dwelling land snails: implication from strontium isotope analyses. J. Zool. 311, 137–144. doi: 10.1111/jzo.12767
Pellegrini, M., Donahue, R. E., Chenery, C., Evans, J., Lee-Thorp, J., Montgomery, J., et al. (2008). Faunal migration in late-glacial central Italy: implications for human resource exploitation. Rapid Commun. Mass Spectr. 22, 1714–1726. doi: 10.1002/rcm.3521
Pierson-Wickmann, A.-C., Aquilina, L., Weyer, C., Molénat, J., and Lischeid, G. (2009). Acidification processes and soil leaching influenced by agricultural practices revealed by strontium isotopic ratios. Geochim. Cosmochim. Acta 73, 4688–4704. doi: 10.1016/j.gca.2009.05.051
Poszwa, A., Ferry, B., Dambrine, E., Pollier, B., Wickman, T., Loubet, M., et al. (2004). Variations in bioavailable Sr concentration and 87Sr/86Sr ratio in boreal forest ecosystems: Role of biocycling, mineral weathering and depth of rooth uptake. Biogeochemistry 67, 1–20. doi: 10.1023/B:BIOG.0000015162.12857.3e
Price, T. D., Meiggs, D., Weber, M.-J., and Pike-Tay, A. (2017). The migration of Late Pleistocene reindeer: isotopic evidence from northern Europe. Arch. Anthropol. Sci. 9, 371–394. doi: 10.1007/s12520-015-0290-z
Prohaska, T., Wenzel, W. W., and Stingeder, G. (2005). ICP-MS-based tracing of metal sources and mobility in a soil depth profile via the isotopic variation of Sr and Pb. Int. J. Mass Spectr. 242, 243–250. doi: 10.1016/j.ijms.2004.11.028
R Core Team. (2019). R: A Language and Environment for Statistical Computing. Vienna: R Foundation for Statistical Computing. Available online at: http://www.R-project.org/.
Raiber, M., Webb, J. A., and Bennetts, D. A. (2009). Strontium isotopes as tracers to delineate aquifer interactions and the influence of rainfall in the basalt plains of southeastern Australia. J. Hydrol. 367, 188–199. doi: 10.1016/j.jhydrol.2008.12.020
Richards, M. P., Harvati, K., Grimes, V., Smith, C., Smith, T., Hublin, J. J., et al. (2008). Strontium isotope evidence of Neanderthal mobility at the site of Lakonis, Greece using laser-ablation PIMMS. J. Arch. Sci. 35, 1251–1256. doi: 10.1016/j.jas.2007.08.018
Rose, F. (1989). Colour Identification Guide to the Grasses, Sedges, Rushes and Ferns of the British Isles and North Western Europe. London: Viking (Penguin Group).
Sillen, A., Hall, G., Richardson, S., and Armstrong, R. (1998). 87Sr/86Sr ratios in modern and fossil food-webs of the Sterkfontein Valley: Implications for early hominid habitat preference. Geochim. Cosmochim. Acta 62, 2463–2478. doi: 10.1016/S0016-7037(98)00182-3
Snoeck, C., Ryan, S., Pouncett, J., Pellegrini, M., Claeys, P., Wainwright, A. N., et al. (2020). Towards a biologically available strontium isotope baseline for Ireland. Sci. Total Environ. 712:136248. doi: 10.1016/j.scitotenv.2019.136248
Stace, C. A. (2010). New Flora of the British Isles (Third Edition). Cambridge: Cambridge University Press.
Thomsen, E., and Andreasen, R. (2019). Agricultural lime disturbs natural strontium isotope variations: implications for provenance and migration studies. Sci. Adv. 5:eaav8083. doi: 10.1126/sciadv.aav8083
Thornton, E. K. (2011). Reconstructing ancient Maya animal trade through strontium isotope (87Sr/86Sr) analysis. J. Arch. Sci. 38, 3254–3263. doi: 10.1016/j.jas.2011.06.035
Vanhaeren, M., d'Errico, F., Billy, I., and Grousset, F. (2004). Tracing the source of Upper Palaeolithic shell beads by strontium isotope dating. J. Arch. Sci. 31, 1481–1488. doi: 10.1016/j.jas.2004.03.011
Williamson, P., and Cameron, R. A. D. (1976). Natural diet of the landsnail cepaea nemoralis. Oikos 27, 493–500. doi: 10.2307/3543468
Willmes, M., Bataille, C. P., James, H. F., Moffat, I., McMorrow, L., Kinsley, L., et al. (2018). Mapping of bioavailable strontium isotope ratios in France for archaeological provenance studies. Appl. Geochem. 90, 75–86. doi: 10.1016/j.apgeochem.2017.12.025
Willmes, M., Kinsley, L., Moncel, M. H., Armstrong, R. A., Aubert, M., Eggins, S., et al. (2016). Improvement of laser ablation in situ micro-analysis to identify diagenetic alteration and measure strontium isotope ratios in fossil human teeth. J. Arch. Sci. 70, 102–116. doi: 10.1016/j.jas.2016.04.017
Willmes, M., McMorrow, L., Kinsley, L., Armstrong, R., Aubert, M., Eggins, S., et al. (2014). The IRHUM (Isotopic Reconstruction of Human Migration) database - bioavailable strontium isotope ratios for geochemical fingerprinting in France. Earth Syst. Sci. Data 6, 117–122. doi: 10.5194/essd-6-117-2014
Keywords: strontium, bioavailability, isoscape, provenance, mobility, snails, plants, archaeology
Citation: Britton K, Le Corre M, Willmes M, Moffat I, Grün R, Mannino MA, Woodward S and Jaouen K (2020) Sampling Plants and Malacofauna in 87Sr/86Sr Bioavailability Studies: Implications for Isoscape Mapping and Reconstructing of Past Mobility Patterns. Front. Ecol. Evol. 8:579473. doi: 10.3389/fevo.2020.579473
Received: 02 July 2020; Accepted: 19 November 2020;
Published: 15 December 2020.
Edited by:
T. Douglas Price, Retired, Madison, WI, United StatesReviewed by:
Kelly Knudson, Arizona State University, United StatesDavid Meiggs, Rochester Institute of Technology, United States
Copyright © 2020 Britton, Le Corre, Willmes, Moffat, Grün, Mannino, Woodward and Jaouen. This is an open-access article distributed under the terms of the Creative Commons Attribution License (CC BY). The use, distribution or reproduction in other forums is permitted, provided the original author(s) and the copyright owner(s) are credited and that the original publication in this journal is cited, in accordance with accepted academic practice. No use, distribution or reproduction is permitted which does not comply with these terms.
*Correspondence: Kate Britton, ay5icml0dG9uQGFiZG4uYWMudWs=
†These authors have contributed equally to this work