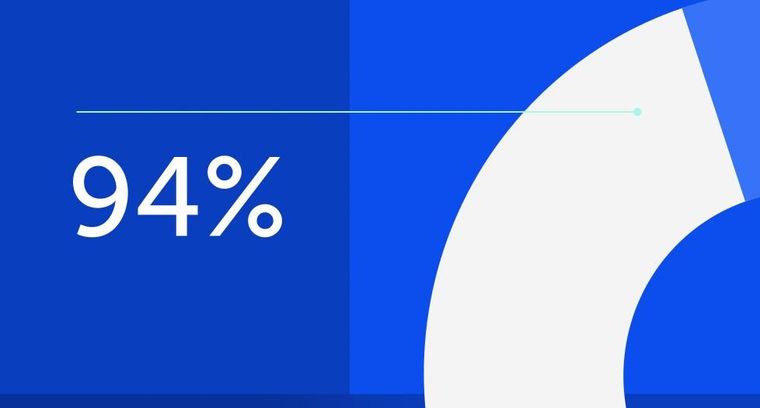
94% of researchers rate our articles as excellent or good
Learn more about the work of our research integrity team to safeguard the quality of each article we publish.
Find out more
ORIGINAL RESEARCH article
Front. Ecol. Evol., 09 February 2021
Sec. Biogeography and Macroecology
Volume 8 - 2020 | https://doi.org/10.3389/fevo.2020.540948
This article is part of the Research TopicTemporal Patterns and Mechanisms of Biodiversity Across Scales in East AsiaView all 12 articles
Large-scale patterns of species diversity are thought to be linked to contemporary climate variability and Quaternary glacial–interglacial climate change. For plants, growth forms integrate traits related to competition or migration capacity, which determine their abilities to deal with the climate variability they face. Evergreen broad-leaved woody plants (EBWPs) are major components of numerous biomes in the subtropical and tropical regions. Hence, incorporating phylogenetic (temporal) and biogeographic (spatial) approaches, we assessed the relative importance of short- and long-term climate variability for biodiversity patterns of different growth forms (i.e., tree, shrub, liana, and bamboo) in EBWPs. We used a dated phylogeny and the distribution records for 6,265 EBWP species which are naturally occurred in China, and computed the corrected weighted endemism, standardized phylogenetic diversity and net relatedness index for the four growth forms, respectively. Ordinary least squares linear regressions, spatial error simultaneous autoregressive models, partial regression and hierarchical variation partitioning were employed to estimate the explanatory power of contemporary climate variability and climate-change velocity from the Last Glacial Maximum to the present. Our results showed that short- and long-term climate variability play complementary role in the biogeographic patterns of Chinese EBWPs. The former had larger effects, but the legacy effects of past climate changes were also remarkable. There were also differences in the effects of historical and current climate among the four growth forms, which support growth forms as a critical plant trait in predicting vegetation response to climate change. Compared to the glacial-interglacial climate fluctuation, seasonality as a unique feature of mid-latitude monsoon climate played a dominant role in the diversification and distribution of EBWP species at the macroscale. The results indicated that the relative importance of climate variability at different temporal scales may relate to distinct mechanisms. To understand effects of future climate change on species distribution more thoroughly, climate conditions in different time scales should be incorporated.
The striking uneven distribution of global biodiversity across space and time has caused wide concerns of ecologists for two centuries (von Humboldt and Bonpland, 1807; Sandel et al., 2020). Despite a long history of studies, the underlying mechanisms of large−scale biodiversity patterns are not fully understood (Gaston, 2000; Lu et al., 2018). Specifically, a number of hypotheses relating to ecological, evolutionary and biogeographic processes have been proposed to explain the patterns along latitudinal gradient (Fine, 2015). While the overwhelming emphasis has been on diversity responses to average or extreme values of climate variables, increasing evidences indicate that climate variability may also play a deterministic or stochastic role in species persistence and biodiversity maintenance (Letten et al., 2013; Mohammadi et al., 2019; Zhang et al., 2019). It is widely detected that climate change causes major shifts in species distributions, reshuffling regional species composition (Parmesan and Yohe, 2003). Moreover, increasing extreme weather events arose from the altered climatic variability regimes, is urging ecologists to expand their purview beyond mean climate conditions (White et al., 2010). In this context, studying the influence of climate variability on biodiversity patterns is invaluable for insight into biodiversity conservation.
Biodiversity encompasses many aspects of biological complexity (Stevens and Tello, 2018). Comparison of taxonomic, ecological and phylogenetic diversity patterns clearly showed the multifaceted nature of biodiversity and incongruence across biodiversity dimensions (Smiley et al., 2020). Besides, climatic factors have different relationships with each dimension of biodiversity, and the variation provides opportunities to explore the mechanisms that underlie biodiversity patterns (Oliveira et al., 2016). Species endemism can reveal the limitation of a biological taxon to a specific geographical area, which is critical for understanding the distribution and evolution of regional flora (Myers et al., 2000; Feng et al., 2019). Phylogenetic diversity metrics reflect variation in species evolutionary history and may be better indicators of species vulnerability to climate change than is species richness (Cardinale et al., 2012; Srivastava et al., 2012). As a result, incorporating information of taxonomic, endemic and phylogenetic diversity distribution patterns may help to predict the change of biodiversity facing future climate scenarios, and to develop more effective approaches for long-term conservation (Burgio et al., 2019).
Different hypotheses have been proposed to relate climate variability with patterns of biodiversity. For example, climate stability hypothesis stipulates that areas subjected to more stable climate might accumulate more species over time (Fine, 2015). According to the Rapoport’s rule, organisms in high latitudes need to form a broader physiological tolerance to cope with the stronger climate seasonality, and species were able to widen their climatic niches and become more widespread (Stevens, 1989). It is also recognized that high environmental variability can be harmful to species persistence because of reduced population growth rates and increased vulnerability to stochastic extinction (Boyce et al., 2006). In contrast, tropical regions with less change in climate conditions could promote speciation and buffer extinction (Kozak and Wiens, 2010). Despite the quantification and application of climate variability in ecology and biogeography studies, the ecological implications of existing metrics and related temporal scales have not been fully understood so far (Garcia et al., 2014). For example, climatic seasonality is a frequent environmental variability compared to the life span of perennial plant species that physiological and phenological adaptation are generally required to ensure species survival (Preston and Sandve, 2013). In contrast, the glacial-interglacial climate fluctuations occur in such a long temporal scale that plant species respond mainly by population migration and species range shifting (Svenning et al., 2015; Ma et al., 2016). It was reported that instability of short-term variables such as temperature annual range and seasonality were the most important environmental variables associated with plant endemism in Borneo (Raes et al., 2009). Recent studies have also identified long−term climate variability as an important factor shaping plant species diversity and phylogenetic structure (Feng et al., 2016; Ma et al., 2016; Sandel et al., 2020). Although the multi-scale temporal variability of climate are presumed to convey complementary information, representing different threats and opportunities for biodiversity (Garcia et al., 2014), there is no consensus whether historical climate fluctuation or current climate seasonality will be more important for explaining modern biodiversity patterns.
Related studies have rarely examined the different responses of biodiversity to climate variability among growth forms of plants. Plant growth-form is characterized by morphological and physiological attributes of plant species that enable them to respond to environmental (e.g., climatic) stresses (Shmida and Burgess, 1988). Empirical studies indicate that there is no consistent pattern in the diversity distribution for different life-form categories (Sánchez-González and López-Mata, 2005; Desalegn and Beierkuhnlein, 2010). Plant species of different growth forms generally differ in life span, dispersal capacities, nutrient cycling, and physiological regulation, evolution rate, and environmental tolerance (Smith and Donoghue, 2008; Way and Oren, 2010; Chen et al., 2013; Kubota et al., 2017; Beckman et al., 2018). These differences of ecological strategies among growth forms may have in turn distinctly constrained plant species in their capacities of responding to climate changes (Valladares et al., 2000). For example, climate variability has been found to exert stronger effects on groups with poorer dispersal abilities (Feng et al., 2016). Thus, growth-form provides critical information for exploring plant diversity patterns and understanding their relationship with climate variability.
Evergreen broad-leaved woody plants (EBWPs) widely distribute all over the world, supporting the persistence of other biodiversity components in forest, shrubland and savanna ecosystems (DeFries et al., 2000; Xu et al., 2017). EBWPs are of great importance for biodiversity conservation. Most of the 35 global hotspots of biodiversity are located in tropical and subtropical regions dominated by EBWPs (Mittermeier et al., 2011). As one of the most important vegetation types, subtropical evergreen broadleaved forests represent the natural vegetation in approximately 25% of terrestrial area in China (Shen et al., 2012). Subjected to severe human disturbance, most of the primary subtropical evergreen broadleaved forests have degraded to secondary forests and shrubs (Song et al., 2013). Understanding the factors shaping species distributions of EBWPs in China could shed light on predicting possible future changes in plant species distributions and assist in generating more efficient conservation and management strategies.
In the present study, we addressed the question of how short- and long-term climate variabilities shape biogeographic patterns of Chinese EBWPs. In particular, we assessed how the present distribution of species endemism and phylogenetic structure are affected by (a) contemporary climate seasonality and, (b) climate fluctuation between the Last Glacial Maximum and present. According to their morphological features, we classified 6,265 EBWP species into four growth forms: trees, shrubs, lianas and bamboos, regarding their differences in regeneration, competition or migration capacity. Specifically, we tested the following three hypotheses: (1) The relative importance of climate variability on EBWPs’ biodiversity patterns may vary between time scales. (2) Endemism as an integrative metrics of species range should be more responsive to historical climate change, while the environmental filtering effect of climate seasonality would dominate the phylogenetic diversity and phylogenetic structure of plant assemblages. (3) The extent that biodiversity patterns of different growth forms influenced by climate variability metrics should be affected by their life-history strategies.
The county-level species distribution data of EBWPs were collected from the “Atlas of woody plants in China: Distribution and climate” (Fang et al., 2011), which contains 11,405 woody species and is the most complete atlas of native woody plants in China until present. To eliminate the potential bias of unequal area on subsequent analyses, we converted the county distribution of each species into a grid map at a resolution of 50 km × 50 km. Grid cells with more than 50% of land area located within China’s border were included in this study. We further supplemented the species distribution data using field records of 1,494 forest plots on 63 mountains across China (Shen et al., 2012). The Plant List1 was used to standardize the botanical nomenclature of the species in this database. Growth form information (tree, shrub, liana or bamboo) of each species were gathered from Flora of China,2 and resulted in a total of 1,925 trees, 3,320 shrubs, 550 lianas, and 470 bamboos (the species list and growth form of each species is available in Supplementary Materials of this manuscript).
Comparative studies showed that values of phylogenetic diversity calculated based on phylogenies derived from common synthesis trees are correlated significantly with those of purpose-built phylogenies (Li et al., 2019). Therefore, we did not build phylogenetic trees specifically based on gene sequence data. Instead, we used a recently published, the currently largest dated mega phylogeny for seed plants, constructed using 79,881 taxa in GenBank with a backbone tree provided by Open Tree of Life version 9.1 (GBOTB), as a backbone to generate a phylogeny for the Chinese EBWPs. We used the R package V. PhyloMaker (Jin and Qian, 2019) to generate species-level phylogenetic trees of different growth forms in EBWPs.
Here we adopted three common biodiversity metrics—corrected weighted endemism (CWE), standardized phylogenetic diversity (SPD) and net relatedness index (NRI) — to quantify the multi-facetted biodiversity patterns.
In order to avoid high correlations between species richness and endemism due to the sampling effect caused by species numbers, we used CWE to measure species endemism. CWE emphasizes areas incorporating a high proportion of range-restricted species, where are not necessarily species-rich. CWE is calculated with the following formula (Crisp et al., 2001):
where Si is the number of taxa in the ith grid cell, Rj is the total grid cell number of the jth species.
To quantify the relationship between phylogenetic diversity (PD) and species richness, we used a randomization-adjusted method to calculate SPD. Specifically, we calculated PD for each grid cell using a randomly selected sample of EBWPs of identical species number, from the species pool of China, and this step was repeated for 1,000 times to estimate a mean of the randomized PD values, and then calculated SPD with the following formula (Kembel et al., 2010):
where, PDobserved refers to the observed PD, mean PDrandomized refers to the expected PD of the randomized sample assemblages of EWBPs (n = 1000), and sdPDrandomized refers to the standard deviation of the PD for the randomized assemblages.
NRI estimates the mean pairwise phylogenetic relatedness in an assemblage. We calculated NRI based on the mean phylogenetic distance (MPD) for each grid cell using the following formula (Webb et al., 2002):
where, MPDobserved refers to the observed MPD, MPDrandomized refers to the expected MPD of randomly generated assemblages, and sdMPDrandomized refers to the standard deviation of 1000 iterations of MPDrandomized. A positive NRI value indicates that species are more closely related than expected in a random sample, and thus corresponds to phylogenetic clustering. By contrary, a negative NRI corresponds to phylogenetic evenness or overdispersion.
The value frequency distributions were calculated to detect the normality of the three biogeographic indices (Figure 1). Since CWE for EWBPs in four growth-forms were prominently left-skewed, it was log-transformed in the following statistics. SPD and NRI were calculated using the R package “picante.” The calculation of SPD and NRI for distinct growth forms (tree, shrub, liana and bamboo) followed the above procedure.
Figure 1. Frequency distribution histogram of corrected weighted endemism (CWE), standardized phylogenetic diversity (SPD), and net relatedness index (NRI) for trees, shrubs, lianas and bamboos of evergreen broad-leaved woody plant species (EBWPs) within the grids of 50 km × 50 km resolution in China.
Variability in climate conditions was initially considered at two time-scales: short-term (contemporary seasonality) and long-term (glacial-interglacial fluctuation). To estimate the effects of contemporary climate variability on the biogeographic pattern of EBWP species, we chose two variables to represent the short-term climate variability, i.e., annual temperature range (ATR); and precipitation seasonality (PSN).
To test the effects of long-term climate variability, we applied the climate-change velocity for mean annual temperature (MATV) and mean annual precipitation (MAPV) from the Last Glacial Maximum (LGM, c. 21 ka) to the present (Sandel et al., 2011). Climate-change velocity represents the local rate of displacement of climate conditions along Earth’s surface, and is defined as the ratio of temporal vs spatial gradients of climate (Loarie et al., 2009). Implementation of the four climate variability metrics nationwide revealed that each characterized a distinct pattern (Figure 2).
Figure 2. The spatial patterns of current (1970–2000) annual temperature range, precipitation seasonality, and historical (LGM to present) temperature-change velocity and precipitation-change velocity in China.
Current (1970–2000) and LGM climate data were downloaded on October 25, 2019 from the WorldClim v2.0 database3 at a spatial resolution of 1 km × 1 km (Fick and Hijmans, 2017). Because of the uncertainty of past climatic models, we used the average value of three available LGM simulations based on the CCSM4 (Community Climate System Model version 4) models, MPI-ESM−P (Max-Planck-Institute Earth System Model) and MIROC-ESM (The Earth system model, Model for Interdisciplinary Research on Climate-Earth system model) (Xu et al., 2019).
NRI cannot be calculated with less than two species in each grid cell. Therefore, after deleting grids with a land area smaller than half of the grid cell (i.e., 1250 km2), grid cells containing fewer than two EBWP species were also excluded from the statistical analyses. Based on the value frequency distribution, MATV and MAPV were log-transformed to improve the normality of model residuals. Afterward, we standardized all variables to make the regression coefficients comparable.
Firstly, we performed ordinary least squares (OLS) linear regressions to explore the bivariate relationships between CWE, SPD, NRI and each explanatory variable. To account for spatial autocorrelation in the residuals of OLS models which may bias parameter estimation, we also used spatial error simultaneous autoregression (SAR) models for single variable analyses. We used a partial regression method (Legendre and Legendre, 1998) to partition the contributions of contemporary climate variability and Quaternary glacial–interglacial climate change to the spatial variation of CWE, SPD, and NRI for each growth form, respectively. Moreover, we used a Hierarchical Partitioning (HP) method to compare the independent contributions of each environmental variable to the variation in the spatial distributions of CWE, SPD, and NRI of the four growth forms, respectively. The method resolves the collinearity problems among variables through comparing all possible models fitted with all subsets of explanatory variables to estimate the independent effect of each variable (Olea et al., 2010). SAR modeling and related OLS modeling were implemented using the open macroecological software “SAM 4.0” (4, Rangel et al., 2010). Partial regressions were conducted in R 3.6.2 with the “vegan” package (Oksanen et al., 2010), and HP was performed with the “hier.part” package (Walsh and Mac Nally, 2008).
The evergreen broadleaved tree, shrub, liana and bamboo species distributed in 33.7, 86.6, 40.5, and 36.7% of all grid cells, respectively. Shrub species in all have a much broader distribution range, covering most area across China, while trees, lianas and bamboos showed comparable distribution ranges, mostly restricted to the tropic and subtropic regions south of 30°N. Within the subtropic-tropic region, the latitudinal gradient of species richness is prominent for trees, shrubs and liana, but not for bamboos (Figure 3).
Figure 3. The spatial patterns of species richness of the four growth forms (i.e., tree, shrub, liana, and bamboo) of evergreen broad-leaved woody plants (EBWPs) in China. Empty areas indicate the absence of EBWPs.
There was no clear latitudinal gradient for the CWE of EBWPs (Figure 4, up). Generally, the regions with high endemism value distributed more to the southwestern than the eastern part of China for three growth-forms other than bamboo. Several endemism centers of EBWPs for trees, shrubs and lianas emerged, most in the Eastern Himalaya and the Hengduan Mountain Ranges (EHHMRs), southwest border regions, Hainan Island and Taiwan Island. Bamboos extended to the mountains of North China with low CWE values. While in the south of 30°N, CWE of bamboos was generally high except for the plain regions along the Yangtze River in the northern part.
Figure 4. The spatial patterns of CWE (up), SPD (middle), and NRI (low) of the four growth forms of EBWPs in China. Empty areas are those with less than two species of EBWP recorded in one grid cell.
For each growth form, the high SPD values generally concentrated in the tropical area of South and Southwest China (Figure 4, middle). For trees, SPD was lower in the eastern part than that in the western part of subtropical region. For shrubs, there were two regions with obvious low SPD values, i.e., the Greater Hing’an Mountains (GHMs) in Northeast China, and the EHHMRs in Southwest China. Lianas generally had much lower SPD value in subtropical than in tropical areas. Meanwhile, the SPD value of bamboos was more homogeneous across the range of bamboos, although higher values located more to the southern subtropical region and the eastern Himalaya.
With respect to NRI (Figure 4, bottom), evergreen broad-leaved trees exhibited strong phylogenetic overdispersion in the tropics, while tended to be clustering in the extratropical region and reached the highest clustering in the northern boundary of their distribution. Shrubs revealed distinct NRI pattern, with overdispersion in the broad tropic-subtropic area while strongly clustered in the EHHMRs and GHMs. Lianas and bamboos were mostly phylogenetic clustering across their distribution ranges, with phylogenetic random or overdispersion patches scattered mainly in the southern tropic regions.
Corrected weighted endemism of all EBWPs had a negative power associated with ATR, MATV, and MATP, but the relationship with PSN appeared much weaker and showed a rough triangle shape, with highest CWE corresponding to middle values of PSN. These relationships similarly applied to all four growth forms of EWBPs (Supplementary Figure S1a), indicating that a lower endemism of EWBPs can resulted from either larger current temperature seasonality or faster climate velocities.
Non-linear relationships were generally shown between climate variability indices and SPD of EBWPs (Supplementary Figure S1b). Specifically, along the gradient of ATR, a negative trend of SPD (when ATR < 30) was followed by a random pattern generally applicable for four growth-forms. The relationships between SPD and PSN were all complex and unclear. Whereas, both MATV and MAPV had a generally triangle relationship with SPD, i.e., largest and lest SPD values occurred at the lowest climate velocity, and high climate velocities corresponded to SPD values around zero.
Likewise, the effect of climate variability on NRI varied substantially across climate indices and growth forms (Supplementary Figure S1c). A triangle pattern generally applied to the relationship between NRI and climate velocity (MATV and MAPV) for four growth forms of EBWPs. NRI increased with ATR (when ATR < 30) and then roughly fluctuated around zero, while the variability of NRI on the gradient of PSN were less clear for different growth forms.
The climate variability factors considered here accounted for 0∼52.2, and 5.4∼68.3% of variance in geographical patterns of CWE by OLS and SAR models, respectively (Table 1). The R2 values were mostly lower for moisture than temperature index. ATR generally explained the most variations in CWE, while PSN accounted for the least variance in CWE of shrubs, lianas and bamboos.
Table 1. R-squares of single climate variable of the ordinary least squares linear regressions model (OLS) and simultaneously autoregressive model (SAR) for predicting spatial patterns of corrected weighted endemism (CWE), standardized phylogenetic diversity (SPD) and net relatedness index (NRI) of tree, shrub, liana and bamboo EBWPs.
The variability of SPD and NRI were accounted for by single climate variables at much lower percentages in general, i.e., 0∼14.2 and 2.3∼27.5% by OLS and SAR models for SPD, and 0∼23.5 and 4.7∼71.0% by OLS and SAR models for NRI (Table 1). ATR was negatively associated with SPD and positively associated with NRI across growth forms. PSN had inconsistent relationship with SPD and NRI across growth forms. For MATV and MAPV, OLS models were mostly insignificant, while SAR models provided some explanatory powers.
The total climate variability could account for considerable percentages of variance in the spatial patterns of CWE, i.e., 51.5, 48.0, 62.0, and 37.2% for trees, shrubs, lianas and bamboos, respectively (Figure 5). However, the explanatory power of climate variability for SPD (6.6∼32.9%) and NRI (9.3∼33.8%) were much lower. The effect of climate variability on biogeographic patterns of EBWPs varied substantially across growth forms. Climate variability had the highest explanatory power on CWE for lianas, and the highest explanatory power on SPD and NRI for trees.
Figure 5. The partitioning of the variance (R2,%) in CWE, SPD, and NRI of trees (A–C), shrubs (D–F), lianas (G–I), bamboos (J–L) of EBWPs, accounted for by contemporary climate seasonality (ART + PSN) and Quaternary glacial–interglacial climate change (MATV + MAPV) using partial regression model of OLS.
Contemporary climate seasonality overwhelmed historical climate velocities in affecting biogeographic variables for trees of EBWPs. For shrubs and bamboos, the two aspects showed comparable effects on CWE and SPD, but stronger effects of current climate variability revealed for NRI. For lianas, climate seasonality dominated CWE, while climate variability at two temporal scales were comparable for SPD and NRI. Moreover, the current climate seasonality and historical climate velocity had consistently strong joint effects on the geographic pattern of CWE for all growth types. Whereas, the effect of contemporary climate seasonality and that of long-term glacial-interglacial cycle were almost independent of each other for SPD and NRI.
On the other hand, the effects of temperature variability at different temporal scales (i.e., ATR and MATV) were generally stronger than those of moisture variability (i.e., PSN and MAPV) for CWE of four growth forms (Table 1 and Supplementary Figure S2). In contrast, the contemporary precipitation seasonality was more important than temperature range for SPD and NRI, while temperature-change velocity was more important than precipitation-change velocity.
Temporal climatic variability is increasingly recognized as a critical determinant of biogeographic patterns (Guo and Werger, 2010; Letten et al., 2013; Irl et al., 2015; Ma et al., 2016). Seasonal and interannual climate variability were frequently selected among the predictors in explaining variation of relative dominance of a particular biological assemblage (Gray et al., 2006; Engemann et al., 2016). Species richness or endemism at different scales were also found to be strongly affected by paleoclimate changes (Jansson, 2003; Feng et al., 2016; Xu et al., 2019). In the meantime, species in unstable environments tend to be more phylogenetically clustered, as Patrick and Stevens (2016) argued, because species with narrow ranges were generally limited by dispersal capacity and more easily whipped out by interannual climate variability, indicating an interdependent relationship among different mechanisms. However, which of the biogeographic patterns, i.e., diversity, endemism and phylogenetic structure, were more suspect to climate variability, and how do different mechanisms contribute to the patterns, remain unresolved.
This study simultaneously assessed the importance of climate variability on patterns of three biogeographic diversity indices for EBWPs. The results revealed that the influence of temporal climate variability is more important for species endemism than for phylogenetic diversity and structure of EBWPs in China. And the current climate seasonality had a greater or at least equivalent impact than the Quaternary glacial-interglacial climate changes. Meanwhile, energy dominated the effect of climate variability on endemism while moisture variability was generally more important for SPD and NRI patterns.
Although this study focused on climate variability for biogeographic patterns, additional abiotic factors such as mean resource availability and biotic interactions such as competition most probably play important roles as well. As shown by our results, there is no reason to claim that either short- or long-term climate variability or a combination of them adequately account for geographic variations of EBWPs biodiversity. The geographical variance explained is relatively low especially for the phylogenetic structure, in spite of the statistically significant relationships. In other words, those models do not explain biodiversity patterns well enough, which most likely caused by omission of key variables driving biodiversity.
A higher percentage of variations in CWE than those in SPD and NRI patterns of EBWPs were accounted for by climate variability of different temporal scales (Figure 5). In other words, climate variability had much stronger effects on species distribution process than on species assembling for EBWPs.
The constraint effects of climate variability on species ranges is straightforward and has been commonly reported (Jansson, 2003; Feng et al., 2019), and this effect also linked the latitudinal patterns of species richness with the Rapoport’s role (Stevens, 1989). On the other hand, the phylogenetic diversity and structure patterns of biological assemblage are outcomes of deterministic and stochastic assembly processes acting at multi-scales across space and time (Kozak and Wiens, 2010; Feng et al., 2014; Eiserhardt et al., 2015). With regard to SPD and NRI patterns of EBWPs in China, the very complex patterns and a generally lack of latitudinal trend (Supplementary Figures S1b,c) indicated that the patterns were dominated by regional rather than global or hemispheric scale process. Indeed, the difference between tropic and out-of-tropic were prominent in the NRI patterns, supportive to the tropic niche conservatism theory (Kubota et al., 2017). Moreover, the longitudinal variations and topography related anomaly highlighted the role of mechanisms such as dispersal barriers, disturbance and vegetation degradation, other than climate variability.
Overall, the current climate seasonality showed a greater or equivalent influence on the biogeographic patterns of EBWPs in China compared with the glacial-interglacial climate velocities, supporting our first hypothesis. Contemporary climate seasonality showed stronger or comparable effects than historical climate velocities on all three biogeographic variables for EBWPs, which is inconsistent with our second hypothesis. And the effects of climate variability at two temporal scales were more interdependent on endemism, but less (or even offset) on phylogenetic structure, indicating potentially distinct underlying mechanisms.
The existence of ecliptic obliquity determines climate seasonality as a fundamental feature of climate out of tropics. Precipitation seasonality in China is also intensified by Asian monsoons (Murakami and Matsumoto, 1994; Ji et al., 2005). The existence of climate seasonality is much longer (i.e., ∼140 million year) than the glacial-interglacial climate fluctuations in Quaternary (GICFQ) (Preston and Sandve, 2013). Therefore, it should be imprinted deeper within the evolutionary and biogeographic processes of plant species. This may help to explain the more prominent effect of seasonality than GICFQ on the biogeographic patterns. Plants respond to seasonality mainly through physiological and phenological adaptations, such as the time of flowering, fruiting and seed dispersal, resulting in life history traits evolution (Peng et al., 2008; Post, 2013) and deterministic community assembly (Che et al., 2019). Meanwhile, climate seasonality influences the location of species range margins by driving episodes of population colonization and local extinction (Guo and Werger, 2010; Bennie et al., 2013).
It is showed that areas with higher climate velocities tend to show neutral NRI and SPD value, while very low (phylogenetic overdispersaion) and high (clustering) NRI values both corresponded to low climate velocities (Figure 4 and Supplementary Figures S1b,c). Regions with low climate velocities corresponded to high mountains, where environmental filtering is intensive and lead to a clustered phylogenetic structure; while regions with low climate velocities and very low NRI (and high SPD) mainly located in or near tropics, where biological competition dominates and phylogenetic structure is overdispersed. Long−term climate variability may contribute to current endemism and phylogenetic diversity gradients in three ways. Firstly, areas with high climatic variability may favor species with broader range sizes and high dispersal abilities that enable them to cope with rapid climate displacement (Sandel et al., 2011). Secondly, climatic variability may reduce speciation rates by lowering the likelihood of clade specialization on a narrow array of climate conditions (Jansson and Dynesius, 2002). Thirdly, small-ranged species face greater extinction risks in areas with unstable climates because of the challenges of persistence and diversification under strong climate variability (Zuloaga et al., 2019). Extinction risk studies on phylogenetic trees suggest that extinction is often selective and concentrated in certain lineages (Eiserhardt et al., 2015), which was in line with the decrease of SPD and increase of NRI with increasing temperature-change velocity in this study.
It is noteworthy that, climatic variability indicators act not in an isolation way, but interact constantly. Long-term changes that drive shifts in species ranges can cover up the influences of short-term climatic variability (Gray et al., 2006). In this study, the effect of GICFQ on CWE patterns was mostly nested within the effect of the current climate variability. Moreover, the historical climate velocities had much weaker and even negative joint effects with climate seasonality on the SPD and NRI patterns. In other words, the legacy of GICFQ may be offset by seasonal climate variability, or vice versa. Therefore, examining multiple metrics simultaneously can show how different dimensions of climate change interact to intensify or lighten species’ exposure to climate change (Garcia et al., 2014).
The effects of temperature variability, in short or long-term, was consistently stronger on EBWPs endemism than that of precipitation. The results indicated that temperature variability has a generally more crucial role in affecting biodiversity than precipitation, that’s why latitudinal pattern is a primary biodiversity pattern (Fine, 2015). In fact, the fluctuations of temperature in both short- and long-term presented a more predictable latitudinal gradient, making migration a worthy and successful strategy. While precipitation and its seasonal variation are both influenced by ground features such as topographic heterogeneity, and thus less predictable and more difficult for plants to track (White et al., 2010).
On the other hand, precipitation seasonality was more important than temperature range for phylogenetic diversity and structure, indicating that the Asia monsoons are critical for the generation and assemblage of EBWPs. It is also noteworthy that while the CWE of EBWPs in China consistently decreased with increasing ATR, it reached the maximum value at medium PSN (Supplementary Figure S1a). It may suggest that, while temperature seasonal range primarily limits the distribution of EBWPs endemic to China, this floristic component has more or less adapted to precipitation seasonality, which is a fundamental feature of summer monsoon of East Asia (Yao et al., 2010).
Linking multiple temporal scales of climate variability to threats for species is just the first step. To ultimately understand the influences of climate variability on biodiversity, the abilities of plants to deal with, or adapt to challenges they face must be taken in consideration (Chevin et al., 2010). Previous studies on the response of plants to climate variability across growth forms have pointed to mixed results. For the vascular plants in Europe, there are no significant differences between the importance of postglacial migration limitations between shrubs and trees in determining species ranges (Normand et al., 2011). However, the relationship between range size of vascular plants in China and velocity was more significant for shrubs than for trees and lianas (Xu et al., 2018). Consistent with our third hypothesis, the effect of climate variability metrics differs considerably among the four growth forms of EBWPs (Table 1 and Figure 5). In general, the constraints of climate variability in short- or long-term, and in temperature or precipitation were relatively consistent and more significant for trees, while the responses of other growth patterns to climate variability were weaker and less consistent.
Shrubs exhibited larger responses than other growth forms to paleoclimate change (Supplementary Figure S2). The climate-change velocity in temperature and precipitation was high in temperate regions in China, which is in accordance with global patterns (Garcia et al., 2014). The evergreen broadleaved trees, lianas and bamboos were mostly limited to the tropical and subtropical regions, whereas evergreen shrubs were distributed throughout the whole country. The stronger effects of climate-change velocity for distribution patterns of shrubs may be attributed to their extension into the high-latitude regions. As for species with specialized climatic conditions in the tropical and subtropical regions like evergreen trees and lianas, they are likely threatened more by short-term variability, because of reduced phenotypic plasticity and physiological adaptation in such circumstances (Araújo et al., 2013).
With nearly 1,500 species, bamboos are grouped into three tribes: Arundinarieae (temperate woody), Bambuseae (tropical woody), and Olyreae (herbaceous) (Bamboo Phylogeny Group, 2012). All bamboos distributed in China are woody species, which have a tree-like habit and highly lignified culms. The influences of both short-term and long-term climate variability in bamboos were less important than that of other growth forms (Table 1 and Figure 5). This should mainly relate to their unique life history. As a group of typical clonal plants, bamboo ordinarily rely on asexual reproduction from below ground, spread horizontally within their habitat by plagiotropic stems, and establish localized and patched ramets with differences in resource supply (Evans and Turkington, 1988). This life history strategy can thus effectively buffer against small scale environmental variability in both spatial and temporal dimensions (Stuefer et al., 1994; Zhuang et al., 2011).
The differential links between biodiversity patterns of four growth forms and climate variability indicate that vulnerability to climate change of EBWPs is non-randomly distributed across growth forms. Growth form is associated with functional traits, which determine the ability to survive in the changing climate of a species (Engemann et al., 2016). It has been reported that particular functional groups were removed by glaciation from a given area depending on their traits with respect to cold and drought tolerance (Svenning, 2003). Furthermore, due to the significant phylogenetic signal in growth forms across phylogeny (Qian and Zhang, 2014), differential responses of growth forms to climate variability may also result in phylogenetically selective extinction and cause significantly greater losses of phylogenetic diversity (Feng et al., 2014; Eiserhardt et al., 2015). Therefore, this study supports the prediction that future climate change could result in shifted trait compositions, altered patterns of plant functional groups and changed ecosystem functioning (Engemann et al., 2016).
We used EBWP species in China to quantify biogeographic patterns for four growth forms separately. We found that areas with high species endemism and phylogenetic diversity of EBWPs corresponded well with the tropical and subtropical mountains. Our results showed that both current and historical environmental variability is influential to the maintenance of biodiversity, and the relative importance of climate variability on diversity varied along with the change of ecological and evolutionary time scales. Our study suggested differential effects of short- and long-term climate variability on growth forms, highlighting the need for scientists to integrate the different responses of growth forms to future climate change. To understand effects of future climate change on biodiversity more thoroughly, climate conditions in different time scales should be incorporated.
The datasets generated for this study are available on request to the corresponding author.
ZS and YX designed the study and compiled the data. YX and JZ conducted the phylogenetic analyses. YX conducted the statistical analyses and wrote the first version of the manuscript. ZS, JZ, RZ, and YJ contributed to manuscript revisions. All authors contributed to the article and approved the submitted version.
This work was supported by the projects of the National Natural Science Foundation of China [grant numbers 41701055 and 41971228] and the National Key Research and Development Program of China [grant number 2017YFC0505200].
The authors declare that the research was conducted in the absence of any commercial or financial relationships that could be construed as a potential conflict of interest.
The Supplementary Material for this article can be found online at: https://www.frontiersin.org/articles/10.3389/fevo.2020.540948/full#supplementary-material
FIGURE S1 | The point patterns of biogeographical metrics [(a) CWE, (b) SPD, and (c) NRI] and four climate change variables for four growth-forms of EWBPs. Four columns from left to right were trees, shrubs, lianas, and bamboos. ATR, annual temperature range; PSN, precipitation seasonality; MATV, temperature-change velocity since Last Glacial Maximum; MAPV, precipitation-change velocity since Last Glacial Maximum.
FIGURE S2 | Independent contributions of environmental variables accounting for the variation in the spatial patterns of CWE, SPD, and NRI of tree, shrub, liana and bamboo EBWPs. ATR, annual temperature range; PSN, precipitation seasonality; MATV, temperature-change velocity since Last Glacial Maximum; MAPV, precipitation-change velocity since Last Glacial Maximum.
Araújo, M. B., Ferri-Yáñez, F., Bozinovic, F., Marquet, P. A., Valladares, F., and Chown, S. L. (2013). Heat freezes niche evolution. Ecol. Lett. 16, 1206–1219. doi: 10.1111/ele.12155
Bamboo Phylogeny Group (2012). An updated tribal and subtribal classification of the bamboos (Poaceae: Bambusoideae). J. Am. Bamboo Soc. 1, 3–27.
Beckman, N. G., Bullock, J. M., and Salguero-Gómez, R. (2018). High dispersal ability is related to fast life-history strategies. J. Ecol. 106, 1349–1362. doi: 10.1111/1365-2745.12989
Bennie, J., Hodgson, J. A., Lawson, C. R., Holloway, C. T., Roy, D. B., Brereton, T., et al. (2013). Range expansion through fragmented landscapes under a variable climate. Ecol. Lett. 16, 921–929. doi: 10.1111/ele.12129
Boyce, M. S., Haridas, C. V., Lee, C. T., and Group, N. S. D. W. (2006). Demography in an increasingly variable world. Trends Ecol. Evol. 21, 141–148. doi: 10.1016/j.tree.2005.11.018
Burgio, K. R., Presley, S. J., Cisneros, L. M., Davis, K. E., Dreiss, L. M., Klingbeil, B. T., et al. (2019). Dimensions of passerine biodiversity along an elevational gradient: a nexus for historical biogeography and contemporary ecology. bioRxiv doi: 10.1101/842138 [Preprint].
Cardinale, B. J., Duffy, J. E., Gonzalez, A., Hooper, D. U., Perrings, C., Venail, P., et al. (2012). Biodiversity loss and its impact on humanity. Nature 486, 59–67. doi: 10.1038/nature11148
Che, X., Chen, D., Zhang, M., Quan, Q., Møller, A. P., and Zou, F. (2019). Seasonal dynamics of waterbird assembly mechanisms revealed by patterns in phylogenetic and functional diversity in a subtropical wetland. Biotropica 51, 421–431. doi: 10.1111/btp.12648
Chen, Y., Han, W., Tang, L., Tang, Z., and Fang, J. (2013). Leaf nitrogen and phosphorus concentrations of woody plants differ in responses to climate, soil and plant growth form. Ecography 36, 178–184. doi: 10.1111/j.1600-0587.2011.06833.x
Chevin, L. M., Lande, R., and Mace, G. M. (2010). Adaptation, plasticity, and extinction in a changing environment: towards a predictive theory. PLoS Biol. 8:e1000357. doi: 10.1371/journal.pbio.1000357
Crisp, M. D., Laffan, S., Linder, H. P., and Monro, A. N. N. A. (2001). Endemism in the Australian flora. J. Biogeogr. 28, 183–198. doi: 10.1046/j.1365-2699.2001.00524.x
DeFries, R. S., Hansen, M. C., Townshend, J. R., Janetos, A., and Loveland, T. R. (2000). A new global 1-km dataset of percentage tree cover derived from remote sensing. Global Change Biol. 6, 247–254. doi: 10.1046/j.1365-2486.2000.00296.x
Desalegn, W., and Beierkuhnlein, C. (2010). Plant species and growth form richness along altitudinal gradients in the southwest Ethiopian highlands. J. Veg. Sci. 21, 617–626.
Eiserhardt, W. L., Borchsenius, F., Plum, C. M., Ordonez, A., and Svenning, J. C. (2015). Climate-driven extinctions shape the phylogenetic structure of temperate tree floras. Ecol. Lett. 18, 263–272. doi: 10.1111/ele.12409
Engemann, K., Sandel, B., Enquist, B. J., Jørgensen, P. M., Kraft, N., Marcuse-Kubitza, A., et al. (2016). Patterns and drivers of plant functional group dominance across the Western Hemisphere: a macroecological re-assessment based on a massive botanical dataset. Bot. J. Linn. Soc. 180, 141–160. doi: 10.1111/boj.12362
Evans, R. C., and Turkington, R. (1988). Maintenance of morphological variation in a biotically patchy environment. New Phytol. 109, 369–376. doi: 10.1111/j.1469-8137.1988.tb04207.x
Fang, J., Wang, Z., and Tang, Z. (2011). Atlas of Woody Plants in China: Distribution and Climate. Beijing: Springer Science and Business Media Press.
Feng, G., Ma, Z., Sandel, B., Mao, L., Normand, S., Ordonez, A., et al. (2019). Species and phylogenetic endemism in angiosperm trees across the Northern Hemisphere are jointly shaped by modern climate and glacial–interglacial climate change. Global Ecol. Biogeogr. 28, 1393–1402. doi: 10.1111/geb.12961
Feng, G., Mao, L., Sandel, B., Swenson, N. G., and Svenning, J. C. (2016). High plant endemism in China is partially linked to reduced glacial-interglacial climate change. J. Biogeogr. 43, 145–154. doi: 10.1111/jbi.12613
Feng, G., Mi, X., Bøcher, P. K., Mao, L., Sandel, B., Cao, M., et al. (2014). Relative roles of local disturbance, current climate and paleoclimate in determining phylogenetic and functional diversity in Chinese forests. Biogeosciences 11, 1361–1370. doi: 10.5194/bg-11-1361-2014
Fick, S. E., and Hijmans, R. J. (2017). WorldClim 2: new 1-km spatial resolution climate surfaces for global land areas. Int. J. Climatol. 37, 4302–4315. doi: 10.1002/joc.5086
Fine, P. V. (2015). Ecological and evolutionary drivers of geographic variation in species diversity. Annu. Rev. Ecol. Evol. S. 46, 369–392. doi: 10.1146/annurev-ecolsys-112414-054102
Garcia, R. A., Cabeza, M., Rahbek, C., and Araújo, M. B. (2014). Multiple dimensions of climate change and their implications for biodiversity. Science 344:1247579. doi: 10.1126/science.1247579
Gray, S. T., Betancourt, J. L., Jackson, S. T., and Eddy, R. G. (2006). Role of multidecadal climate variability in a range extension of pinyon pine. Ecology 87, 1124–1130. doi: 10.1890/0012-9658(2006)87[1124:romcvi]2.0.co;2
Guo, K., and Werger, M. J. (2010). Effect of prevailing monsoons on the distribution of beeches in continental East Asia. For. Ecol. Manage. 259, 2197–2203. doi: 10.1016/j.foreco.2009.11.034
Irl, S. D. H., Harter, D. E. V., Steinbauer, M. J., Puyol, D. G., Fernández-Palacios, J. M., Jentsch, A., et al. (2015). Climate vs. topography – spatial patterns of plant species diversity and endemism on a high-elevation island. J. Ecol. 103, 1621–1633. doi: 10.1111/1365-2745.12463
Jansson, R. (2003). Global patterns in endemism explained by past climatic change. P. Roy. Soc. B-Biol. Sci. 270, 583–590. doi: 10.1098/rspb.2002.2283
Jansson, R., and Dynesius, M. (2002). The fate of clades in a world of recurrent climatic change: milankovitch oscillations and evolution. Annu. Rev. Ecol. S. 33, 741–777. doi: 10.1146/annurev.ecolsys.33.010802.150520
Ji, J., Shen, J., Balsam, W., Chen, J., Liu, L., and Liu, X. (2005). Asian monsoon oscillations in the northeastern Qinghai–Tibet Plateau since the late glacial as interpreted from visible reflectance of Qinghai Lake sediments. Earth Planet. Sci. Lett. 233, 61–70. doi: 10.1016/j.epsl.2005.02.025
Jin, Y., and Qian, H. (2019). V. PhyloMaker: an R package that can generate very large phylogenies for vascular plants. Ecography 42, 1353–1359. doi: 10.1111/ecog.04434
Kembel, S. W., Cowan, P. D., Helmus, M. R., Cornwell, W. K., Morlon, H., Ackerly, D. D., et al. (2010). Picante: r tools for integrating phylogenies and ecology. Bioinformatics 26, 1463–1464. doi: 10.1093/bioinformatics/btq166
Kozak, K. H., and Wiens, J. J. (2010). Accelerated rates of climatic-niche evolution underlie rapid species diversification. Ecol. Lett. 13, 1378–1389. doi: 10.1111/j.1461-0248.2010.01530.x
Kubota, Y., Kusumoto, B., Shiono, T., and Tanaka, T. (2017). Phylogenetic properties of Tertiary relict flora in the East Asian continental islands: imprint of climatic niche conservatism and in situ diversification. Ecography 40, 436–447. doi: 10.1111/ecog.02033
Letten, A. D., Ashcroft, M. B., Keith, D. A., Gollan, J. R., and Ramp, D. (2013). The importance of temporal climate variability for spatial patterns in plant diversity. Ecography 36, 1341–1349. doi: 10.1111/j.1600-0587.2013.00346.x
Li, D., Trotta, L., Marx, H. E., Allen, J. M., Sun, M., Soltis, D. E., et al. (2019). For common community phylogenetic analyses, go ahead and use synthesis phylogenies. Ecology 100:e02788. doi: 10.1002/ecy.2788
Loarie, S. R., Duffy, P. B., Hamilton, H., Asner, G. P., Field, C. B., and Ackerly, D. D. (2009). The velocity of climate change. Nature 462, 1052–1055.
Lu, L. M., Mao, L. F., Yang, T., Ye, J. F., Liu, B., Li, H. L., et al. (2018). Evolutionary history of the angiosperm flora of China. Nature 554, 234–238.
Ma, Z., Sandel, B., and Svenning, J. C. (2016). Phylogenetic assemblage structure of North American trees is more strongly shaped by glacial–interglacial climate variability in gymnosperms than in angiosperms. Ecol. Evol. 6, 3092–3106. doi: 10.1002/ece3.2100
Mittermeier, R. A., Turner, W. R., Larsen, F. W., Brooks, T. M., and Gascon, C. (2011). “Global biodiversity conservation: the critical role of hotspots,” in Biodiversity Hotspots, ed. J. C. Habel (Springer), 3–22. doi: 10.1007/978-3-642-20992-5_1
Mohammadi, S., Ebrahimi, E., Moghadam, M. S., and Bosso, L. (2019). Modelling current and future potential distributions of two desert jerboas under climate change in Iran. Ecol. Inform. 52, 7–13. doi: 10.1016/j.ecoinf.2019.04.003
Murakami, T., and Matsumoto, J. (1994). Summer Monsoon over the Asian Continent and Western Northern Pacific. J. Meteoro. Soc. Jap. 72, 719–745. doi: 10.2151/jmsj1965.72.5_719
Myers, N., Mittermeier, R. A., Mittermeier, C. G., Da Fonseca, G. A., and Kent, J. (2000). Biodiversity hotspots for conservation priorities. Nature 403, 853–858. doi: 10.1038/35002501
Normand, S., Ricklefs, R. E., Skov, F., Bladt, J., Tackenberg, O., and Svenning, J.-C. (2011). Postglacial migration supplements climate in determining plant species ranges in Europe. P. Roy. Soc. B. 278, 3644–3653. doi: 10.1098/rspb.2010.2769
Oksanen, J., Blanchet, F. G., Kindt, R., Legendre, P., O’hara, R., Simpson, G. L., et al. (2010). Vegan: Community Ecology Package. R Package Version 1.17-4.
Olea, P. P., Mateo-Tomas, P., and De Frutos, Á (2010). Estimating and modelling bias of the hierarchical partitioning public-domain software: implications in environmental management and conservation. PLoS One 5:e11698. doi: 10.1371/journal.pone.0011698
Oliveira, B. F., Machac, A., Costa, G. C., Brooks, T. M., Davidson, A. D., Rondinini, C., et al. (2016). Species and functional diversity accumulate differently in mammals. Global Ecol. Biogeogr. 25, 1119–1130. doi: 10.1111/geb.12471
Parmesan, C., and Yohe, G. (2003). A globally coherent fingerprint of climate change impacts across natural systems. Nature 421, 37–42. doi: 10.1038/nature01286
Patrick, L. E., and Stevens, R. D. (2016). Phylogenetic community structure of North American desert bats: influence of environment at multiple spatial and taxonomic scales. J. Anim. Ecol. 85, 1118–1130. doi: 10.1111/1365-2656.12529
Peng, Y., Lin, W., Wei, H., Krebs, S. L., and Arora, R. (2008). Phylogenetic analysis and seasonal cold acclimation associated expression of early light-induced protein genes of Rhododendron catawbiense. Physiol. Plant. 132, 44–52.
Post, E. (2013). Ecology of Climate Change: the Importance of Biotic Interactions. Princeton, NJ: Princeton University Press.
Preston, J. C., and Sandve, S. R. (2013). Adaptation to seasonality and the winter freeze. Front. Plant Sci. 4:167. doi: 10.3389/fpls.2013.00167
Qian, H., and Zhang, J. (2014). Using an updated time-calibrated family-level phylogeny of seed plants to test for non-random patterns of life forms across the phylogeny. J. Syst. Evol. 52, 423–430. doi: 10.1111/jse.12086
Raes, N., Roos, M. C., Slik, J. F., Van Loon, E. E., and Steege, H. T. (2009) Botanical richness and endemicity patterns of Borneo derived from species distribution models. Ecography 32, 180–192. doi: 10.1111/j.1600-0587.2009.05800.x
Rangel, T. F., Diniz-Filho, J. A. F., and Bini, L. M. (2010). SAM: a comprehensive application for spatial analysis in macroecology. Ecography 33, 46–50. doi: 10.1111/j.1600-0587.2009.06299.x
Sánchez-González, A., and López-Mata, L. (2005). Plant species richness and diversity along an altitudinal gradient in the Sierra Nevada, Mexico. Divers. Distrib. 11, 567–575. doi: 10.1111/j.1366-9516.2005.00186.x
Sandel, B., Arge, L., Dalsgaard, B., Davies, R. G., Gaston, K. J., Sutherland, W. J., et al. (2011). The influence of Late Quaternary climate-change velocity on species endemism. Science 334, 660–664. doi: 10.1126/science.1210173
Sandel, B., Weigelt, P., Kreft, H., Keppel, G., van der Sande, M. T., Levin, S., et al. (2020). Current climate, isolation and history drive global patterns of tree phylogenetic endemism. Global Ecol. Biogeogr. 29, 4–15. doi: 10.1111/geb.13001
Shen, Z., Fei, S., Feng, J., Liu, Y., Liu, Z., Tang, Z., et al. (2012). Geographical patterns of community-based tree species richness in Chinese mountain forests: the effects of contemporary climate and regional history. Ecography 35, 1134–1146. doi: 10.1111/j.1600-0587.2012.00049.x
Shmida, A., and Burgess, T. L. (1988). “Plant growth form strategies and vegetation types in arid environments,” in Plant Form and Vegetation Structure, eds M. J. A. Werger, P. J. M. van der. Aart, H. J. During, and J. T. A. Verhoeven (Hague: SPB Academic Publishing), 211–241.
Smiley, T. M., Title, P. O., Zelditch, M. L., and Terry, R. C. (2020). Multi-dimensional biodiversity hotspots and the future of taxonomic, ecological and phylogenetic diversity: a case study of North American rodents. Global Ecol. Biogeogr. 29, 516–533. doi: 10.1111/geb.13050
Smith, S. A., and Donoghue, M. J. (2008). Rates of molecular evolution are linked to life history in flowering plants. Science 322, 86–89. doi: 10.1126/science.1163197
Song, Y., Wang, X., and Yan, E. (2013). Evergreen Broad-leaved Forests in China: Classification, Ecology, Conservation. Beijing: Science Press.
Srivastava, D. S., Cadotte, M. W., MacDonald, A. A. M., Marushia, R. G., and Mirotchnick, N. (2012). Phylogenetic diversity and the functioning of ecosystems. Ecol. Lett. 15, 637–648. doi: 10.1111/j.1461-0248.2012.01795.x
Stevens, G. C. (1989). The latitudinal gradient in geographical range: how so many species coexist in the tropics. Am. Nat. 133, 240–256. doi: 10.1086/284913
Stevens, R. D., and Tello, J. S. (2018). A latitudinal gradient in dimensionality of biodiversity. Ecography 41, 2016–2026. doi: 10.1111/ecog.03654
Stuefer, J. F., During, H. J., and de Kroon, H. (1994). High benefits of clonal integration in two stoloniferous species, in response to heterogeneous light environments. J. Ecol. 82, 511–518. doi: 10.2307/2261260
Svenning, J. C. (2003). Deterministic Plio-Pleistocene extinctions in the European cool-temperate tree flora. Ecol. Lett. 6, 646–653. doi: 10.1046/j.1461-0248.2003.00477.x
Svenning, J. C., Eiserhardt, W. L., Normand, S., Ordonez, A., and Sandel, B. (2015). The influence of paleoclimate on present-day patterns in biodiversity and ecosystems. Annu. Rev. Ecol. Syst. 46, 551–572. doi: 10.1146/annurev-ecolsys-112414-054314
Valladares, F., Martinez-Ferri, E., Balaguer, L., Perez-Corona, E., and Manrique, E. (2000). Low leaf-level response to light and nutrients in Mediterranean evergreen oaks: a conservative resource-use strategy? New Phytol. 148, 79–91. doi: 10.1046/j.1469-8137.2000.00737.x
von Humboldt, A., and Bonpland, A. (1807). Essai sur la Géographie des Plantes. Paris: Levrault, Schell and Co Press.
Walsh, C., and Mac Nally, R. (2008). Package Hier. Part Version 1.0–3. R Statistics Project, University of Bristol.
Way, D. A., and Oren, R. (2010). Differential responses to changes in growth temperature between trees from different functional groups and biomes: a review and synthesis of data. Tree physiol. 30, 669–688. doi: 10.1093/treephys/tpq015
Webb, C. O., Ackerly, D. D., McPeek, M. A., and Donoghue, M. J. (2002). Phylogenies and community ecology. Annu. Rev. Ecol. Syst. 33, 475–505. doi: 10.1146/annurev.ecolsys.33.010802.150448
White, E. P., Ernest, S. M., Adler, P. B., Hurlbert, A. H., and Lyons, S. K. (2010). Integrating spatial and temporal approaches to understanding species richness. Phil. T. Roy. Soc. B 365, 3633–3643. doi: 10.1098/rstb.2010.0280
Xu, W., Svenning, J. C., Chen, G., Chen, B., Huang, J., and Ma, K. (2018). Plant geographical range size and climate stability in China: growth form matters. Global Ecol. Biogeogr. 27, 506–517. doi: 10.1111/geb.12710
Xu, Y., Shen, Z., Ying, L., Wang, Z., Huang, J., Zang, R., et al. (2017). Hotspot analyses indicate significant conservation gaps for evergreen broadleaved woody plants in China. Sci. Rep. 7, 1–10.
Xu, Y., Shen, Z., Ying, L., Zang, R., and Jiang, Y. (2019). Effects of current climate, paleo-climate, and habitat heterogeneity in determining biogeographical patterns of evergreen broad-leaved woody plants in China. J. Geogr. Sci. 29, 1142–1158. doi: 10.1007/s11442-019-1650-x
Yao, C., Qian, W., Yang, S., and Lin, Z. (2010). Regional features of precipitation over Asia and summer extreme precipitation over Southeast Asia and their associations with atmospheric–oceanic conditions. Meteorol. Atmos. Phys. 106, 57–73. doi: 10.1007/s00703-009-0052-5
Zhang, Y., Clauzel, C., Li, J., Xue, Y., Zhang, Y., Wu, G., et al. (2019). Identifying refugia and corridors under climate change conditions for the Sichuan snub-nosed monkey (Rhinopithecus roxellana) in Hubei Province. China. Ecol. Evol. 9, 1680–1690. doi: 10.1002/ece3.4815
Zhuang, M., Li, Y., and Chen, S. (2011). Advances in the researches of bamboo physiological integration and its ecological significance. J. Bamb. Res. 30, 5–9.
Keywords: biodiversity patterns, climate variability, evergreen broadleaved woody plants, multi-scale, temporal
Citation: Xu Y, Shen Z, Zhang J, Zang R and Jiang Y (2021) The Effects of Multi-Scale Climate Variability on Biodiversity Patterns of Chinese Evergreen Broad-Leaved Woody Plants: Growth Form Matters. Front. Ecol. Evol. 8:540948. doi: 10.3389/fevo.2020.540948
Received: 06 March 2020; Accepted: 20 August 2020;
Published: 09 February 2021.
Edited by:
Luciano Bosso, Università degli Studi di Napoli Federico II, ItalyReviewed by:
Liy Mingyang, Nanjing Forestry University, ChinaCopyright © 2021 Xu, Shen, Zhang, Zang and Jiang. This is an open-access article distributed under the terms of the Creative Commons Attribution License (CC BY). The use, distribution or reproduction in other forums is permitted, provided the original author(s) and the copyright owner(s) are credited and that the original publication in this journal is cited, in accordance with accepted academic practice. No use, distribution or reproduction is permitted which does not comply with these terms.
*Correspondence: Zehao Shen, c2h6aEB1cmJhbi5wa3UuZWR1LmNu
†These authors have contributed equally to this work
Disclaimer: All claims expressed in this article are solely those of the authors and do not necessarily represent those of their affiliated organizations, or those of the publisher, the editors and the reviewers. Any product that may be evaluated in this article or claim that may be made by its manufacturer is not guaranteed or endorsed by the publisher.
Research integrity at Frontiers
Learn more about the work of our research integrity team to safeguard the quality of each article we publish.