- 1Wyoming Cooperative Fish and Wildlife Research Unit, Department of Zoology and Physiology, University of Wyoming, Laramie, WY, United States
- 2Haub School of Environment and Natural Resources, Wyoming Cooperative Fish and Wildlife Research Unit, Department of Zoology and Physiology, University of Wyoming, Laramie, WY, United States
- 3Department of Zoology and Physiology, University of Wyoming, Laramie, WY, United States
- 4Wyoming Game and Fish Department, Thayne, WY, United States
- 5U.S. Geological Survey, Wyoming Cooperative Fish and Wildlife Research Unit, Department of Zoology and Physiology, University of Wyoming, Laramie, WY, United States
Resource limitation at the population level is a function of forage quality and its abundance relative to its per capita availability, which in turn, determines nutritional condition of individuals. Effects of resource limitation on population dynamics in ungulates often occur through predictable and sequential changes in vital rates, which can enable assessments of how resource limitation influences population growth. We tested theoretical predictions of bottom-up (i.e., resource limitation) forcing on moose (Alces alces) through the lens of vital rates by quantifying the relative influence of intrinsic measures of nutritional condition and extrinsic measures of remotely sensed environmental data on demographic rates. We measured rates of pregnancy, parturition, juvenile, and adult survival for 82 adult females in a population where predators largely were absent. Life stage simulation analyses (LSAs) indicated that interannual fluctuations in adult survival contributed to most of the variability in λ. We then extended the LSA to estimate vital rates as a function of bottom-up covariates to evaluate their influence on λ. We detected weak signatures of effects from environmental covariates that were remotely sensed and spatially explicit to each seasonal range. Instead, nutritional condition strongly influenced rates of pregnancy, parturition, and overwinter survival of adults, clearly implicating resource limitation on λ. Our findings depart from the classic life-history paradigm of population dynamics in ungulates in that adult survival was highly variable and generated most of the variability in population growth rates. At the surface, lack of variation explained by environmental covariates may suggest weak evidence of resource limitation in the population, when nutritional condition actually underpinned most demographics. We suggest that variability in vital rates and effects of resource limitation may depend on context more than previously appreciated, and density dependence can obfuscate the relationships between remotely sensed data and demographic rates.
Introduction
Empirical evidence along with perceived declines in abundance of moose (Alces alces) across the southern distribution of their range has resulted in heightened interest in identifying factors limiting population growth. Recent studies indicate that nutritional limitation stemming from suboptimal habitat conditions, parasite abundance, and thermal stress associated with climatic warming and drying are contributing to poor population performance in the Intermountain West (Becker et al., 2010; Monteith et al., 2015), Minnesota (Murray et al., 2006; Lenarz et al., 2009; DelGiudice et al., 2011), Michigan (Peterson, 1999; Dodge et al., 2004), and in the northeastern United States (Musante et al., 2010; Bergeron et al., 2013). Confounding the evidence for nutritional limitation is the recolonization of large carnivores, namely, grizzly bears (Ursus arctos horribilis) and gray wolves (Canis lupus), which, in the Intermountain West, occurred concurrently with changes in temperature and precipitation patterns (Bangs and Fritts, 1996; Berger et al., 2001). The relative importance of predation and resource limitation (i.e., forage quality and abundance, its per capita availability) in structuring dynamics of animal populations has been debated for decades, which was catalyzed partially by the Green World Hypothesis (GWH; Hairston et al., 1960). For prey populations, the continuum of top-down and bottom-up forcing implies that trade-offs occur between acquisition of resources for survival and susceptibility to predation (McNamara and Houston, 1987), mediated by proximity to carrying capacity (K) (Sinclair and Krebs, 2002; Pierce et al., 2012; Bowyer et al., 2013). Nonetheless, declines in recruitment of young and abundance of moose are also apparent in regions where large carnivores are absent, further suggesting a predominant role of nutritional limitation in populations near the southern distribution of moose. The life-history paradigm (Eberhardt, 2002) exhibited by ungulates offers a useful predictive framework to assess the influence of resource limitation on animal populations (Gaillard et al., 2000; Eberhardt, 2002; Bonenfant et al., 2009; Monteith et al., 2014). Understanding how nutritional limitation is expressed in populations near K can enhance predictive models that aim to determine the relative influence of top-down and bottom-up forces on ungulate population dynamics.
The proximity of prey populations to K has considerable influence on the relative strength of top-down and bottom-up forces that structure ungulate demography (Kie et al., 2003; Bonenfant et al., 2009). For example, populations near K tend to be in poor nutritional condition with reduced fecundity and survival (McCullough, 1979; Simard et al., 2008). Nevertheless, predator populations can reduce density-dependent feedbacks by killing prey and reducing intraspecific competition (Boyce et al., 1999). Consequently, we expect vital rates and the factors that underpin them to differ between populations regulated by top-down and bottom-up forcing (Pierce et al., 2012). For example, in prey populations under strong top-down forcing, intraspecific competition should be reduced and nutritional condition should increase (Bowyer et al., 2014). In this instance, predation should dampen signals of resource limitation by pushing prey populations below K (Owen-Smith and Mills, 2006; Hopcraft et al., 2010). In contrast, most populations without predators should exhibit strong signals of resource limitation as density increases, and vital rates should respond accordingly (Coulson et al., 2001; Stewart et al., 2005; Monteith et al., 2014). Indeed, interactions between resource limitation and predation can obscure their relative influence at the population level. Thus, a hypothesis-driven approach to assessing variability in vital rates in populations exposed to few predators can help tease apart and detect the mechanisms of resource limitation, advancing our understanding of the influence of predators on ungulate prey (Gaillard et al., 2000; Bowyer et al., 2013).
Resource limitation on population dynamics in ungulates often occurs through predictable and sequential changes in vital rates: reduced survival of young followed by increased age at first reproduction, reduced rates of pregnancy, parturition, and reduced survival of prime-aged adults (i.e., the “Eberhardt Model”; Eberhardt, 2002). Typically, adult survival is high and largely invariant, and the greater variability in juvenile survival commonly drives population dynamics (Gaillard et al., 1998, 2000; Gaillard and Yoccoz, 2003; Raithel et al., 2007). With a reduced energetic buffer against the vagaries of weather, populations exposed to resource limitation may be more sensitive to weather (Portier et al., 1998; Kie et al., 2003; Hansen et al., 2019), and consequently, environmental variability can lead to dramatic changes in population growth rate (λ) for resource-limited populations (Coulson et al., 2001). In contrast, reduced intraspecific competition in response to top-down forcing can weaken environmental signatures on vital rates, because animals possess an energetic buffer against them (Bowyer et al., 2014).
Assessing nutritional status is critical for understanding the influence of habitat alterations on populations, because the nutritional condition of an individual is an integrated measure of energetic gains and losses relative to food quality and abundance (Cook et al., 2007; Monteith et al., 2014). Nutritional condition forms the foundation for life history of individuals and thus affects nearly every demographic component of a population leading to net effects on population growth (Monteith et al., 2014). Remotely sensed data [e.g., normalized difference vegetation index (NDVI) and standardized precipitation index] are increasingly being used as a proxy for assessing resource limitation in ungulate populations (Pettorelli et al., 2011; Monteith et al., 2015), and their accessibility, broad spatial and temporal availability makes them an attractive tool. Nevertheless, remotely sensed data do not discriminate between the species and quality of forage selected by the animal, and per capita availability is particularly difficult to quantify. Thus, linking relationships between resource limitation and remotely sensed data can be noisy or weak. Measures of nutritional condition inherently integrate density dependence because of its effect on per capita availability of food, which is realized in the nutritional status of an animal and not possible to measure through simple measures of habitat. Moreover, directly quantifying habitat quality is exceedingly difficult and often cost prohibitive (DeYoung et al., 2000; Stephenson et al., 2006). An integrated measure of habitat quality and food availability is possible by measuring nutritional status because animal condition is a direct product of its environment (Franzmann, 1977; Parker et al., 2009). Predictive equations for estimating ingesta-free body fat (IFBFat) of moose based on ultrasonography measurements of maximum depth of rump fat are quite accurate (r2 = 0.96; Stephenson et al., 1998), and thus represent a meaningful measurement of nutritional condition.
We sought to quantify how resource limitation influences population dynamics through the lens of variability in vital rates of moose in the Sublette Herd, located in a mountainous region of western Wyoming, United States. Further, we wanted to understand the relative context in which measures of nutritional condition would compare with remotely sensed environmental covariates in predicting vital rate responses. We estimated demographic rates of individuals to evaluate predictions of variability in vital rates and their relative influence on λ (Gaillard et al., 1998) in the context of Eberhardt’s model (Eberhardt, 2002). We did not incorporate population size into demographic models, because annual minimum counts and juvenile ratios were stable before, during, and after the study period (Wyoming Game and Fish Department, unpublished data). Predators were largely absent and female harvest was low; therefore, we expected negative effects of density dependence to be operating and moose to be sensitive to environmental signals of resource limitation (i.e., summer drought and NDVI). Specifically, we expected (1) reductions in survival of young, and fecundity of adults if resource limitation was occurring (Eberhardt, 2002) and (2) invariant survival of adults, relative to other vital rates (Gaillard et al., 1998). Further, we used individual measures of nutritional condition and remotely sensed data to quantify the ability to detect resource limitation, should it occur. We then used deterministic matrix models and life stage simulation analysis (LSA; Wisdom et al., 2000) to assess vital rate contributions to population-level responses to metrics of resource limitation. Additionally, our study provides insight into the interplay among bottom-up forces as they manifest through the life-history characteristics of moose in a variable environment.
Materials and Methods
Study Area
We monitored 82 adult female moose in the Sublette Herd from February 2011 until August 2014 in the Upper Green River basin and eastern foothills of the Wyoming Range (42.8653 degrees North, 110.0708 degrees West; Figure 1). Land ownership was 60% private and 40% public lands. During winter, moose occupied riparian areas at low to mid elevations (1866–2150 m), consisting mainly of willow (Salix spp.) interspersed with cottonwood (Populus spp.), subalpine fir (Abies lasiocarpa), Douglas fir (Pseudotsuga menziesii), and aspen (Populus tremuloides). Homogenous and mixed forests of subalpine fir, lodgepole pine (Pinus contorta), aspen, Engelmann spruce (Picea engelmannii), and Douglas fir also occurred throughout higher elevations along the foothills of the Wyoming Range. Winter ranges of moose typically spanned the relatively flat floodplains of the Green River, as well as Cottonwood, Horse, and Beaver Creeks, which were dominated by willow and intermixed with cottonwoods. Approximately half of the moose were resident with overlapping summer and winter seasonal ranges, while migratory individuals traveled short distances (5–20 miles) to higher elevations or other tributaries of the Green and Hoback Rivers containing stands of willow, subalpine fir, lodgepole pine, and Douglas fir interspersed with stands of aspen, limber pine (Pinus flexilis) and whitebark pine (Pinus albicaulis). Residents occupied willow communities, aspen forests, or mixed-conifer and aspen forest throughout the year. The climate is characterized by short, dry summers and long, cold winters.
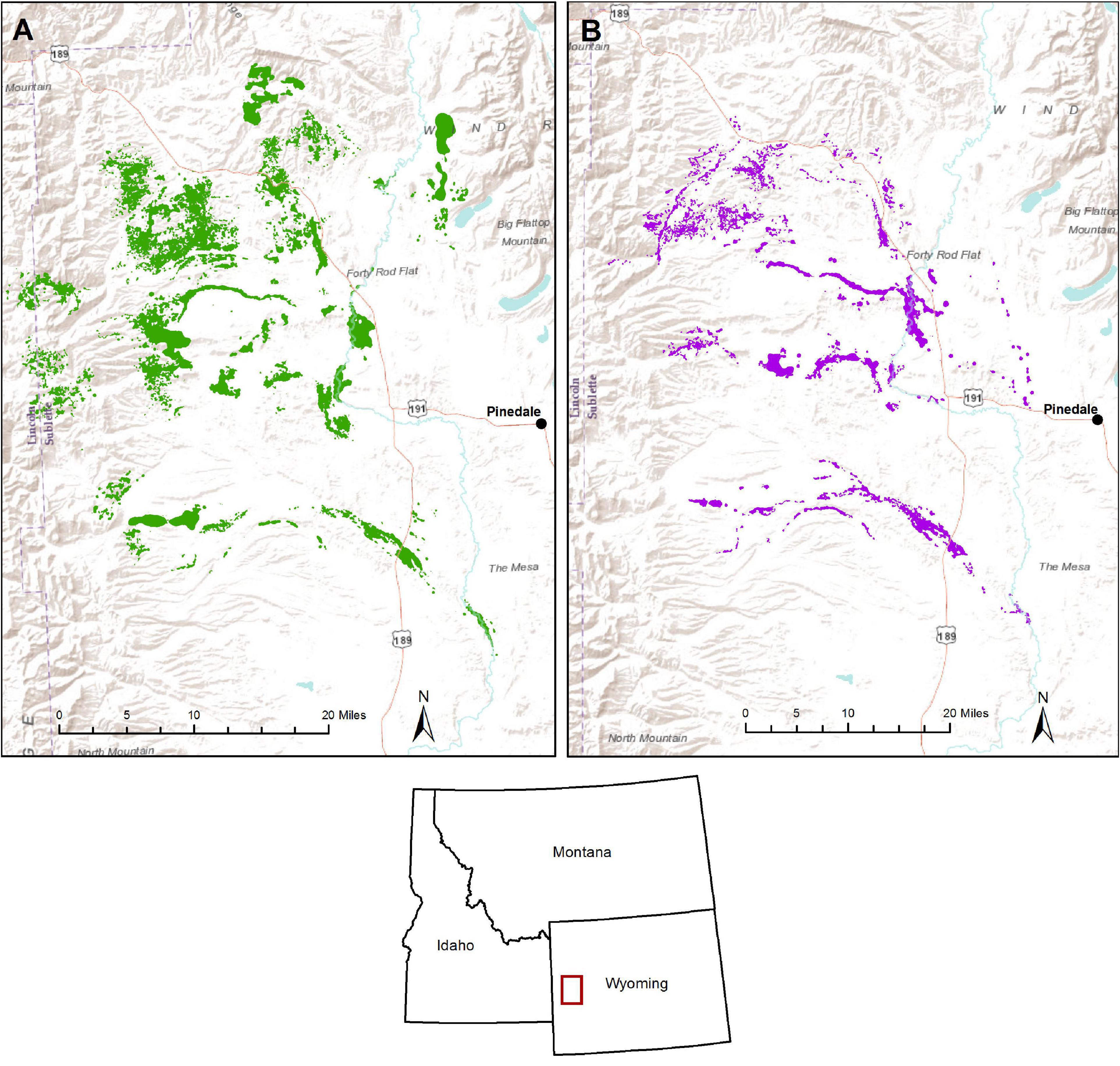
Figure 1. Map of study area showing a sample (n = 63) of seasonal home ranges of adult (>2.5 years), GPS-collared moose from 2012 and 2013, derived from dynamic Brownian bridge movement models for summer (A) and winter (B) in Sublette County of western Wyoming, United States.
The study area was host to four wild ungulates, cougars (Puma concolor), and American black bears (Ursus americanus) (Buskirk, 2016). Elk (Cervus canadensis) were the most numerous ungulate. Mule deer (Odocoileus hemionus) and pronghorn (Antilocapra americana) occurred throughout. Gray wolves (C. lupus) and grizzly bears (U. arctos) were rarely documented in the study area.
Captures
We captured moose via helicopter net-gunning (Native Range Capture Services, Inc.) without immobilization agents, and blindfolded, hobbled, and restrained in a sternal-recumbent position. We fit 72 moose with GPS store-on-board collars (TGW-3700 and -4700, Telonics, Inc., Mesa, AZ, United States), and fit 12 with GPS satellite-uplink collars (various D-cell models, North Star Science and Technology, LLC, King George, VA, United States). Telonics collars recorded hourly locations, and North Star collars recorded locations every 3–5 h, depending on the model. Both Telonics and North Star collars were programmed to release from the animal approximately 2 years after deployment. For 13 moose in 2013 with expiring GPS collars, we deployed VHF-collars (M2230B, Advanced Telemetry Systems, Isanti, MN, United States) to continue demographic monitoring. One experienced investigator (K. L. Monteith) collected all data on nutritional condition from 2012 to 2014 (n = 70 individuals; nutritional condition estimates were not available for 2011). We first assessed condition of animals by palpation and acquired a modified rBCS, which was based on the extent (cm) to which the index finger could be inserted on the caudal side of the sacro-sciatic ligament. This rBCS was analogous to that validated for elk (Cook et al., 2001) and mule deer (Cook et al., 2007), which were highly correlated with percent IFBFat (r2 > 0.88). We measured depth of rump fat and thickness of the bicep femoris using the electronic calipers (±0.1 cm) of a Bantam II portable ultrasound device (E.I. Medical Imaging, Loveland, CO, United States) with a 5-MHz linear-array transducer. We measured maximum depth of subcutaneous rump fat (MAXFat) along a line parallel to the spine and cranial to the ischial tuber (pin bone), which occurred immediately adjacent to the cranial process of the ischial tuber (Stephenson et al., 1998). We used subcutaneous rump fat to estimate percent IFBFat for moose with measurable fat. For animals without subcutaneous fat, we used rBCS to estimate percent IFBFat using the linear relationship between IFBFat and rBCS of moose with measurable rump fat (Cook et al., 2010; Jesmer et al., 2017). During captures for years 2013–2014, we removed the right-incisiform canine to estimate age of each moose (Boertje et al., 2015). For study animals that died before 2013, we collected the I-1 incisor from the mortality site. All age estimates were conducted via cementum annuli by Matson’s Laboratory, Milltown, MT, United States. We omitted yearlings from all analyses (<2 and ≥1 years), because their vital rates can be lower or more variable compared with reproductive adults (Gaillard et al., 2000; Bonenfant et al., 2009). We collected 20 ml of blood from each female by jugular venipuncture. For analyses, we omitted all mortalities that occurred within 2 weeks of capture (n = 4). The University of Wyoming Institutional Animal Care and Use Committee approved procedures for captures and handling (protocol number 20140124JG00057).
Seasonal Ranges of Moose
We delineated summer and winter ranges of each moose using characteristics of net squared displacement. First, we identified moose as migratory if they exhibited directional movement between distinct, non-overlapping winter and summer ranges. Second, we visually inspected plots of net squared displacement of migratory animals to identify start and end dates of migration. Migratory moose typically left winter ranges between late April and late May, and arrived on summer ranges between early and late June, returning to winter ranges around mid to late December. If seasonal ranges overlapped between winter and summer, we classified moose as residents and used the median start and end migration dates from migratory moose relative to year to define the temporal extent of winter and summer ranges. Net squared displacement calculations were derived from GPS-collar locations by calculating the squared distance between the first location identified from capture and every subsequent location along the annual travel path (Bunnefeld et al., 2011). After identifying migration dates, we delineated seasonal range polygons for moose with GPS collars using 95% contours derived from dynamic Brownian bridge movement models (dBBMMs; Kranstauber et al., 2012; see Figure 1 for examples). We specified all dBBMMs with a location error of 20 m, raster cell size of 20 m, window size of 31 locations, and a margin of 11 locations. Fix rates of the GPS collars varied, depending on the year and season. For each of the 13 moose in our study that wore a VHF collar after wearing a GPS collar for two or more years, we used the extent of the seasonal range from the most recent year of the GPS collar to represent seasonal ranges during years the individual was fitted with a VHF collar.
Monitoring of Vital Rates
Pregnancy and Parturition
We separated red blood cells from serum collected at capture via centrifugation and submitted the latter to BioTracking LLC to determine pregnancy status using the presence of Pregnancy-Specific Protein B (PSPB; Huang et al., 2000). We used a PSPB cut-off value of ≥2.5 ng/ml to identify pregnant females with viable fetuses for each sample (Josh Branen, BioTracking LLC, personal communication). To identify parturition events, we evaluated females deemed pregnant during capture, observing collared moose aerially with a Bell-47 helicopter between 9 and 13 June, shortly after peak parturition (Schwartz, 1998, pp. 141–171; Poole et al., 2007). We calculated parturition rates as the proportion of pregnant individuals that were parturient. We calculated twinning rates as the proportion of parturient females observed with twins during the June survey.
Survival of Young
Ungulates are most vulnerable to mortality during the first 6 weeks of life (i.e., the neonatal phase), after which mortality typically decreases until winter (Ballard et al., 1991; Barber-Meyer et al., 2008). Thus, we distinguished between survival of neonates (parturition to mid-July) and survival of juveniles (mid-July to February). Approximately 4–8 weeks after parturition surveys, we relocated adult females from a helicopter to record presence and estimate survival of neonates at heel. The following February we estimated survival of juveniles by relocating females that had at least one neonate at heel in July from the air. We used Kaplan–Meier estimators (Pollock et al., 1989) to calculate annual survival rates of neonates and juveniles.
Adult Survival
We monitored the survival of collared moose with monthly fixed-wing flights and recovered collars from the field when logistically feasible. For GPS-collared moose, we verified date of death by visually inspecting fix locations relative to mortality site or dropped collar. We calculated annual survival rates at monthly intervals using a Kaplan–Meier estimator, with the biological year starting 1 June and ending 31 May.
Covariates
To evaluate the influence of metrics of resource limitation on each vital rate, we used IFBFat measurements and extracted a suite of covariates annually from seasonal ranges of each moose (Table 1). For newly captured moose (i.e., individuals for which the previous summer and winter ranges were not known), we used the seasonal range from the current year to represent the seasonal range for the previous year, because moose in the Greater Yellowstone Ecosystem are faithful to their seasonal ranges (Vartanian, 2011). For all environmental covariates, we used the spatially weighted mean of the raster value extracted from each seasonal range (i.e., polygon derived from the 95% isopleth of dBBMM from GPS-collared moose). Some moose died during the same winter they were first captured, and therefore, had no measurable summer range. To avoid bias associated with censoring these individuals because we lacked data for their summer range, we applied the annual mean value of each environmental covariate extracted from all other summer home ranges. We conducted all data extraction and modeling with R version 4.0.2 (R Core Team, 2020).
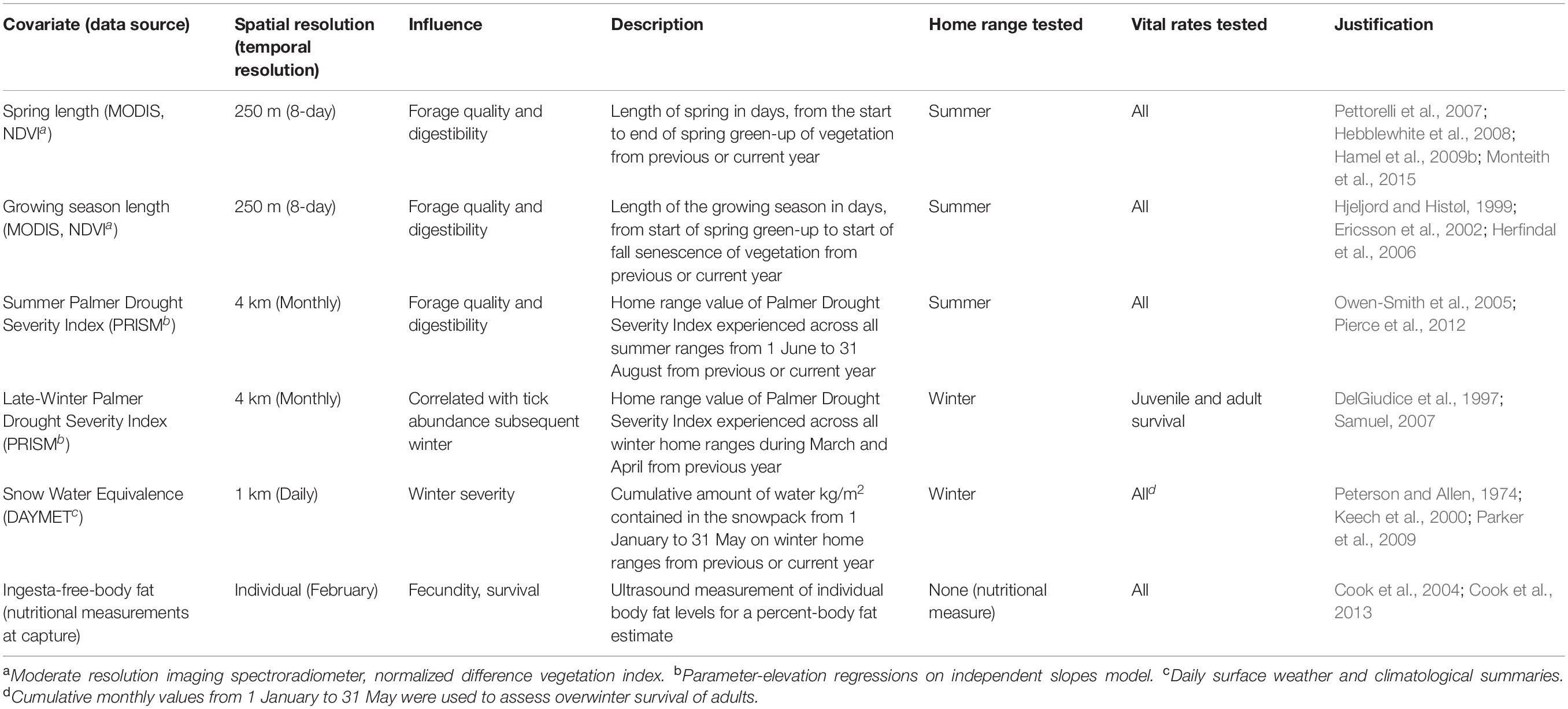
Table 1. Descriptions of covariates evaluated for their relative influence on probability of pregnancy, parturition, survival of juveniles (July–February), and overwinter adults (January–May) of Sublette moose from 2012 to 2014 moose in western Wyoming, United States.
Resource Limitation
Length of spring and growing season can affect fecundity and survival in ungulates (Herfindal et al., 2006; Hamel et al., 2010; Table 1). As proxies for environmental effects and characteristics of summer ranges for moose, we used NDVI from the MODIS terra satellite (8-day temporal resolution) to calculate the annual length of spring (start to end of spring-green up of vegetation) and growing season (start of spring-green up until senescence of vegetation) in days (Table 1). We calculated NDVI annually from 2011 to 2014 using the MOD09Q1 data product from the MODIS terra satellite and followed the same protocol for processing NDVI as outlined in Merkle et al. (2016). We extracted length of spring and growing season in days from each summer range using the spatially-weighted mean of all cells overlapping the 95% dBBMM isopleth of the home range. We defined length of spring as the number of days from the start to end of vegetation green-up and length of the growing season from the start of vegetation green-up until the start of fall senescence of vegetation. Length of spring usually included the months of May and June, whereas the length of the growing season usually included months May to September. To evaluate responses of vital rates to NDVI metrics, we used either the value from current year, in addition to or in place of the previous year’s value, depending on the vital rate. For example, pregnancy status was measured in February, so we used the value from the previous year to account for carry-over effects (Monteith et al., 2014, 2015). For survival of neonates (June–July), we evaluated length of spring from the current year and evaluated length of the growing season (calculated at the end of summer) from the previous year. We used the value from the previous year for length of spring and length of growing season to evaluate survival of juveniles (July–February). Although fires have occurred in Sublette, we did not assess their influence on vital rates because proportional overlap with home ranges was low.
We estimated drought using PRISM raster data of the Palmer Drought Severity Index (PDSI; Palmer, 1965; Table 1) extracted from the seasonal home range of each individual. Warm temperatures during late winter associated with reduced snow cover have been correlated with winter tick (Dermacentor albipictus) abundance the subsequent year (DelGiudice et al., 1997), which can decrease body condition of moose, especially juveniles, through loss of blood and hair (Samuel, 2007).
We estimated seasonal drought annually from the PDSI (Palmer, 1965) using rasters of 4-km2 resolution from PRISM (Daly et al., 1994). The annual PDSI per summer home range of moose was calculated at the end of summer; therefore, we applied the PDSI value from the previous year to evaluate the influence of drought on pregnancy (February), parturition (June), and overwinter (January–May) survival of adults. We used the PDSI value from the current year to evaluate survival of (July–February) juveniles. We evaluated the influence of late winter (March and April) drought on the survival of juveniles and adults. We did not evaluate the influence of late winter drought on pregnancy, because mating season for moose typically occurs during the early stages of tick loading, and it would be unlikely that an effect would be detected.
To relate the energetic costs of snow on moose vital rates, we used raster data of Snow Water Equivalent (SWE) from Daymet (Thornton et al., 1997). Although moose are well adapted to deep snow (Coady, 1974), harsh winters reduce mobility and increase energetic demands, negatively influencing survival of juveniles (Peterson, 1977; Keech et al., 2011) and adults (Peterson and Allen, 1974). Additionally, nutritional costs of moving through snow on maternal condition while calves are in utero could produce less viable neonates (Schwartz, 1998, pp. 141–171). We calculated cumulative values of daily SWE from moose winter ranges annually from 1 January to 31 May to evaluate the influence of snow on parturition, survival of neonates (SWE experienced while in utero), and survival of juveniles (in utero, and first winter as a juvenile). We calculated cumulative values of monthly SWE from January to May to evaluate the influence of winter severity on survival of adults.
Endogenous fat reserves are critical for survival and fecundity of ungulates (Cook et al., 2004, 2010; Monteith et al., 2014). We measured and evaluated the influence of IFBFat in addition to the aforementioned environmental covariates on pregnancy, parturition, and survival of juveniles and adults.
Ungulate populations that have senesced in age can confound estimates of survival and fecundity (Festa-Bianchet et al., 2003; Bonenfant et al., 2009). We included age as a covariate for all vital rate analyses, and determined whether a quadratic transformation was appropriate using residual diagnostics.
Statistical Analyses
Vital Rate Models
For probability of pregnancy, parturition, and survival of neonates and juveniles, we fit binomial GLMMs with the logit link function and individual animal as a random intercept. To assess monthly survival of adults, we used the Andersen–Gill formulation (Andersen and Gill, 1982) of Cox proportional hazards CPHs models (Cox, 1972). We used an information-theoretic approach to model selection by assessing all possible combinations of covariates (Doherty et al., 2012) selected a priori based on Akaike information criteria adjusted for small sample size (AICc), ΔAICc, and Akaike weights (wi) (Burnham and Anderson, 2002). If Pearson correlation coefficients between each pair of covariates were >| 0.5|, we assessed univariate models between each pair and selected the covariate with the minimum AIC to use for model selection. Consequently, all variables that entered AICc model selection had Pearson correlation coefficients < | 0.5|. To improve convergence of the GLMMs and CPHs, we scaled all covariate values so that their mean was zero and their standard deviation was one. For all analyses, we omitted mortalities attributed to anthropogenic causes (e.g., harvest, poaching; n = 4). During model selection, we followed Babyak (2004), using approximately 10 events (i.e., failures or mortalities) per covariate (hereafter, “EPC”) that entered a model. Model fit was assessed using area under the receiver operating characteristic curve (AUC; Hanley and McNeil, 1982).
We deemed covariates important if their 95% confidence intervals did not overlap zero within ΔAICc four of the top model.
In modeling monthly survival of adults, we assessed winter (January–May) and summer (June–December) separately, because we expected environmental covariates to affect moose survival differently with regard to season (Gaillard et al., 2000). We used the Andersen–Gill formulation of CPHs with each month representing a time interval (i.e., the counting process; Therneau and Grambsch, 2000), allowing for left-staggered entry (i.e., newly captured moose added to sample size) and right-censoring of adults (e.g., if the VHF transmitter failed, collar dropped from the individual, or the moose emigrated from study area). Coefficient estimates from CPHs specify the mortality hazard, where positive values increase mortality hazard (i.e., negatively relate to survival). Adults were allowed to contribute to the risk sample every year they were monitored; therefore, we used a robust “sandwich” estimator to account for correlated observations within individuals (Therneau and Grambsch, 2000). We chose a recurrent time-scale for seasonal CPHs based off of the biological year (i.e., 1 June–31 May), because adult mortality occurred largely during late winter (i.e., a strong seasonal hazard; Fieberg and DelGiudice, 2009). We allowed monthly SWE values to cumulatively increase over winter (e.g., a time-varying covariate; Therneau and Grambsch, 2000). Model fit of CPHs was assessed using concordance (Harrell, 2015), which is analogous to AUC. Diagnostic tests were performed on all CPH models to evaluate the proportional hazards assumption using Schoenfeld residuals (Therneau and Grambsch, 2000), but were not reported unless significant violations were detected (i.e., P < 0.05).
Life Stage Simulation Analysis
To assess the influence of interannual variation in vital rates on λ, we conducted LSA. We followed Morris and Doak (2002), and estimated vital rates separately by biological year and then derived a beta distribution of 10,000 estimates for each vital rate by randomly sampling the mean and variance estimated from each year. To derive the 10,000 estimates of λ from beta distributions, we used a 3 × 3 stage-structured, post-birth, female-based matrix model (Caswell, 2001) consisting of the following form:
The first stage calculates reproduction rates by multiplying adult survival (Sa) by fecundity (F = pregnancy rate × parturition rate), and probability of twinning [T = (1−t) + (2t)].
The second stage is juvenile survival (Sj), calculated as the neonatal (June to July) survival rate × juvenile survival (July to Feb) rate. Our surveys of juvenile survival were conducted during February; therefore, to correct for missed late-winter mortality of calves (Gaillard et al., 1998), we assumed that an additional 10% of mortality would occur between February and May 31. Effectively, this additional 10% did not change the variability of the vital rate in the LSA and only influenced estimates of λ. The third stage consists of yearling survival (Sy) and adult survival (Sa). Rates of yearling survival in the Sublette herd were unavailable, so we calculated a mean estimate from a range of yearling survival rates for moose reported in Kunkel and Pletscher (1999) and McLaren et al. (2000). On average, yearling survival was 6.43% lower than adult survival, thus our estimate was calculated as Sy = Sa – 6.43%. We assumed no reproduction of yearlings in our model. The resulting beta distributions of each vital rate were randomly sampled for vital rate estimates to produce 10,000 matrix replicates, estimates of λ, elasticity and sensitivity. Elasticity analysis estimates the effect of a proportional change in the vital rates on population growth rate, whereas sensitivity analysis estimates the impact of an absolute change in vital rates on lambda. We then regressed λ on each vital rate, providing an estimate of the proportion of variation in λ explained by each vital rate (r2). We were unable to account for sampling variance due to the limited time-frame of the study.
Covariate LSA
After assessing vital rate variability and its influence on λ, we evaluated the influence of the covariates on λ through their relative effect on vital rates. To estimate the strength and variability of covariates on vital rates, we extended the LSAs to incorporate the effects of covariates on the vital rates. We estimated rates of pregnancy, parturition, neonatal, juvenile, and overwinter adult survival as a function of the coefficient estimates of the covariates for 10,000 iterations, and populated the same 3 × 3 stage-structured, female-based, post-birth matrix models used in LSAs with these predicted values (hereafter, “covariate LSA”). For the covariate LSA, we used estimates of adult survival from the overwinter (January–May) model only, because summer models failed to converge due to small sample size of mortalities. We accounted for total annual mortality by subtracting the average rate of summer (June–December) mortality from each predicted estimate of overwinter survival. For vital rate responses that were not predicted by any covariate, we applied the beta distribution from the LSA to account for unexplained variation. Yearling survival was also set as a constant, estimated at 6.43% lower than adult survival (Sy = Sa – 6.43%). Similar to the LSA, we then regressed λ on each covariate, providing an estimate of the amount of variation (r2) in λ explained by the covariate.
Results
Probabilities of pregnancy, parturition, and overwinter survival of adults were positively and strongly related to IFBFat (Figures 2A–C and Table 3). Drought conditions on summer home ranges from the previous year reduced probability of parturition (Figure 2D and Table 3). We did not detect important predictors of juvenile survival. Variation in survival of adults was a strong driver of interannual changes in λ (r2 = 0.70; Figures 3A,F). Variation in neonate and juvenile survival, as well as rates of parturition, and pregnancy contributed comparatively little to population growth (Figures 3B–F). Elasticity values for fecundity and survival of juveniles were 0.12, compared with 0.64 for survival of adults. Sensitivity values for fecundity, survival of juveniles, and survival of adults were 0.30, 0.25, and 0.75, respectively. From the time period of the demographic rates measured, the Sublette moose population was increasing (λ = 1.027). Twinning rates were low, neonatal survival remained stable and high, and overwinter survival of juveniles was stable, but slightly reduced (Table 2). Although age competed with other predictors in top models for probability of parturition and pregnancy, all confidence intervals overlapped zero (Table 3). Age as a sole predictor of adult survival was important (CPH β = 0.73; 95% CI: 0.41–1.05), but was clearly outweighed by IFBFat (Table 3). Prime-aged (2–8 years) moose accounted for 63% (SE ± 0.10; n = 15) of total adult mortalities (n = 24), and 84% of all individuals were ≤ 8 years in age ( = 6; range: 2.5–15 years).
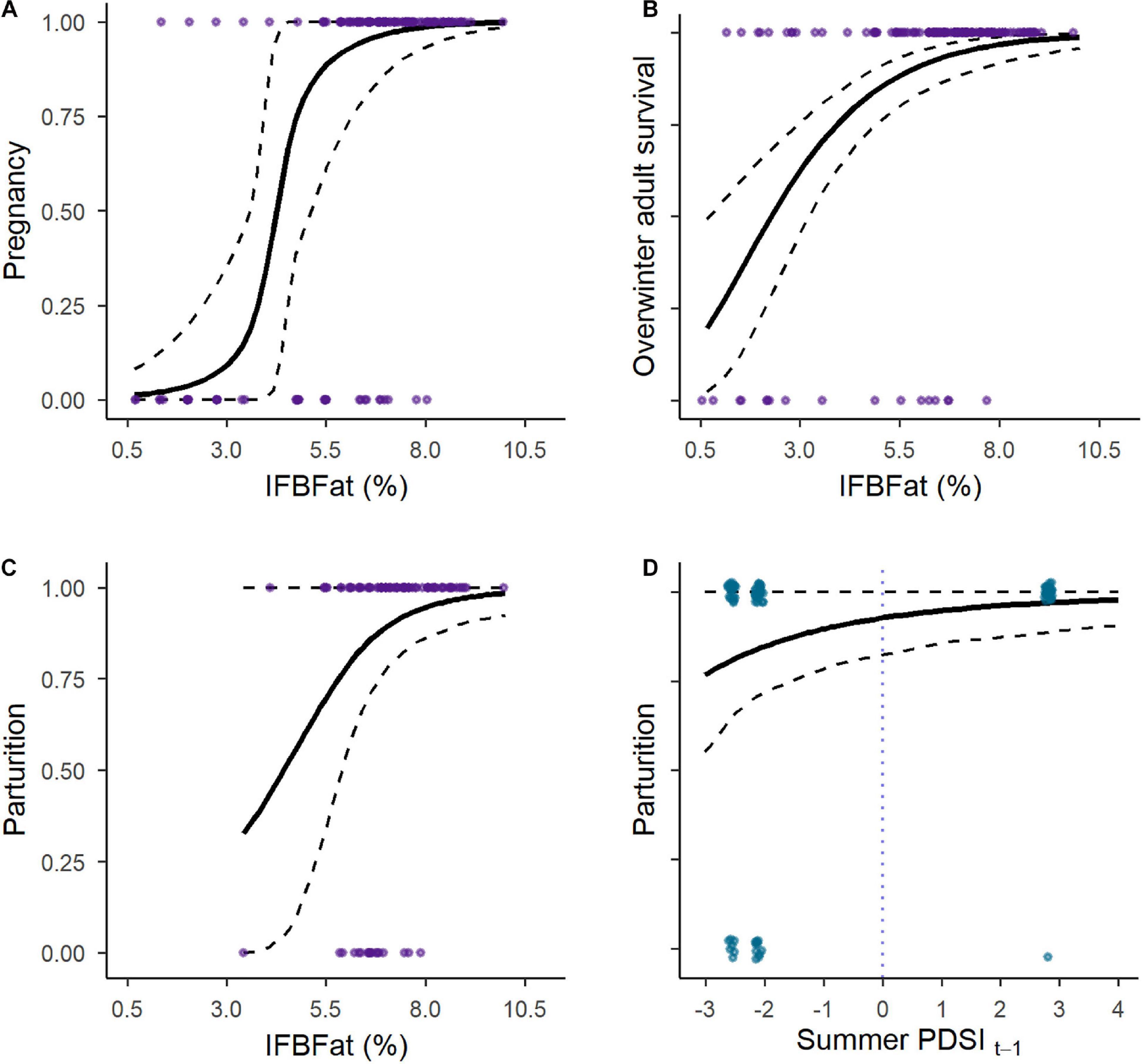
Figure 2. Predicted probabilities (±95% CI) and observed values of (A) pregnancy, (C,D) parturition, and (B) overwinter adult (>2.5 years) survival as a function of percent IFBFat for moose in Sublette 2012–2014 in western Wyoming, United States.
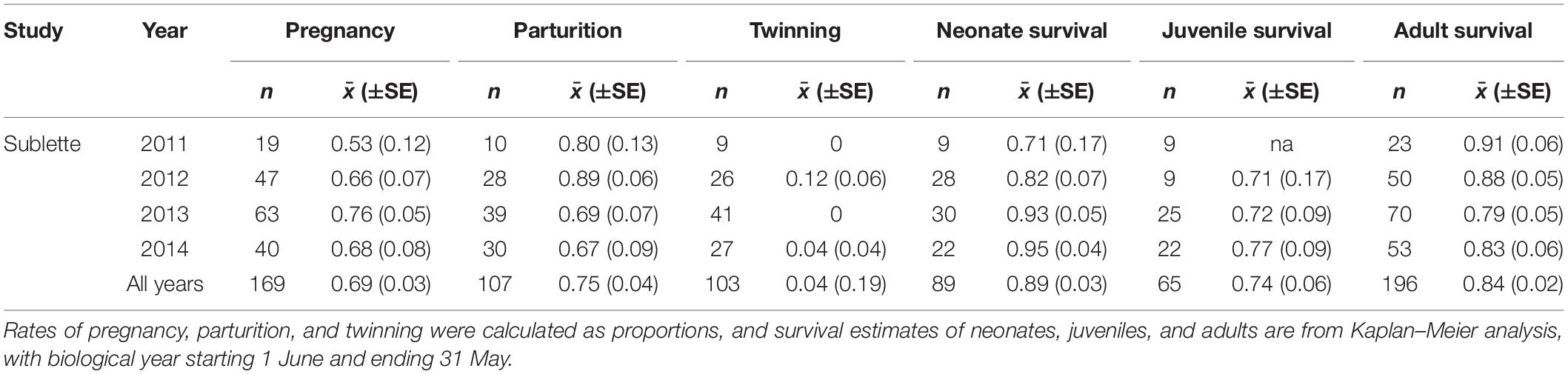
Table 2. Mean estimates (SE) and sample sizes of demographic rates from 2011 to 2014 for Sublette moose in western Wyoming, United States.
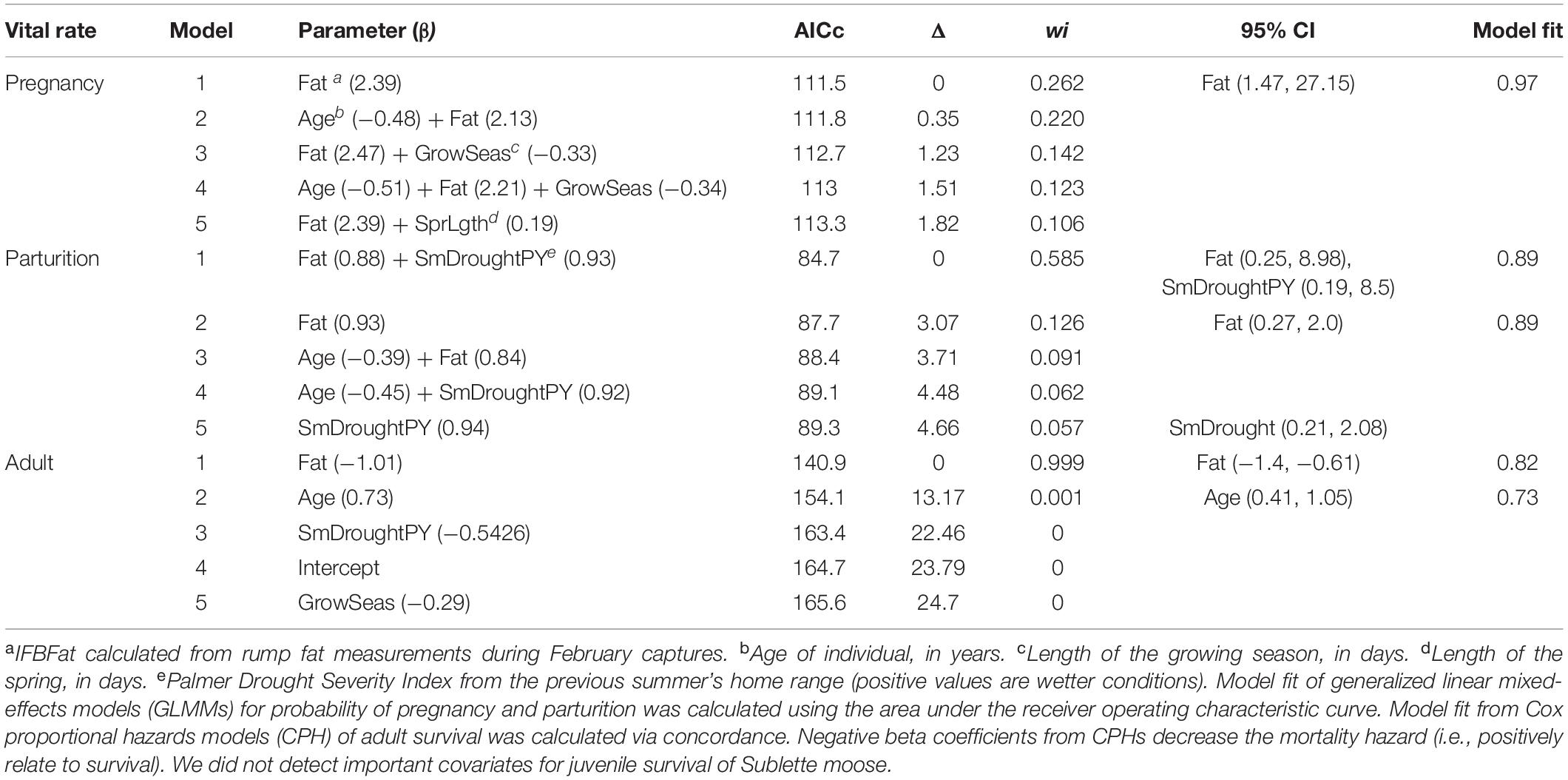
Table 3. Top models derived from AICc model selection with coefficient estimates and 95% confidence intervals (of all terms in model) that did not overlap zero for Sublette moose (n = 70 individuals) from 2012 to 2014.
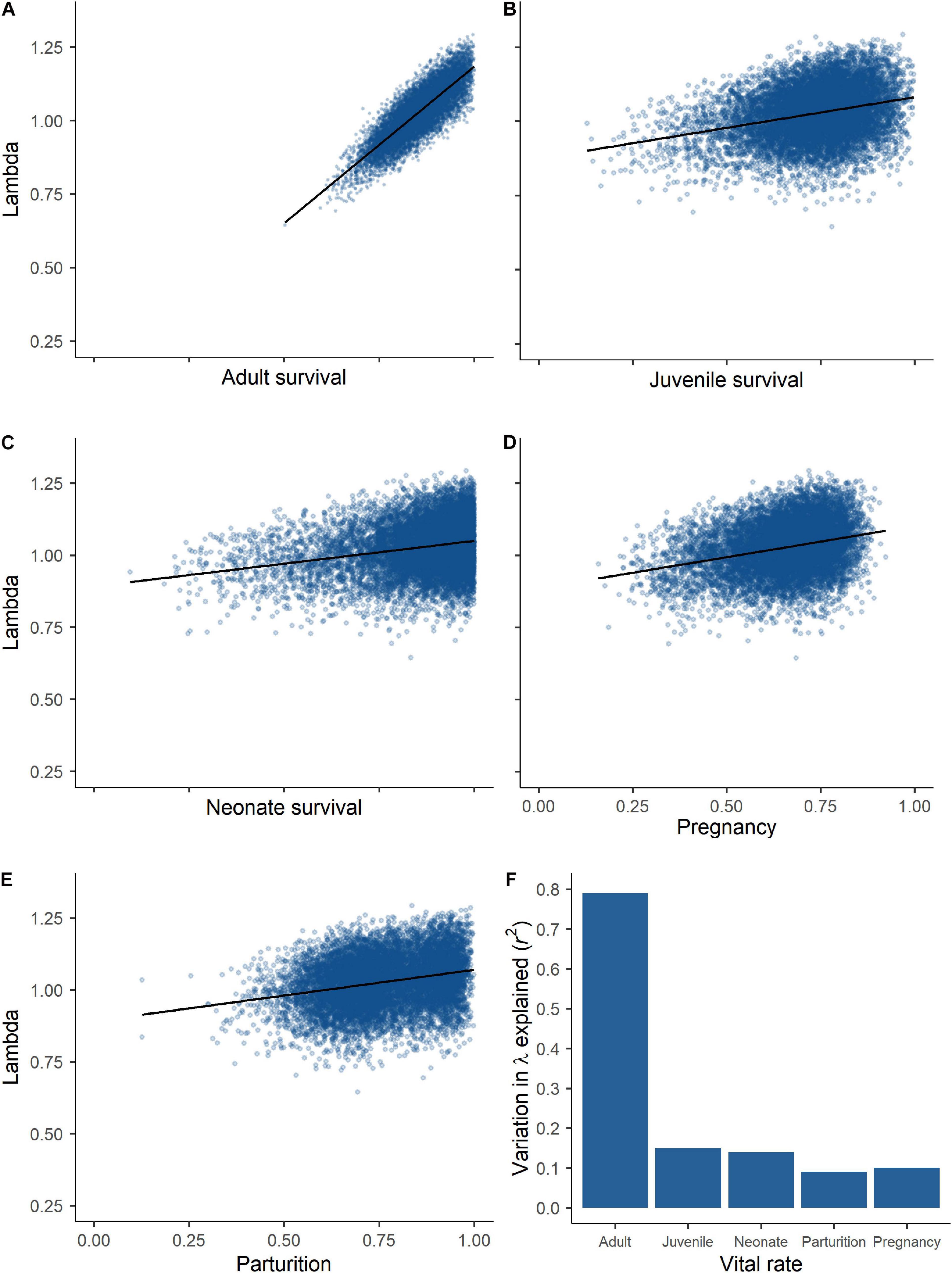
Figure 3. Results from life stage simulation analysis for moose in Sublette from 2011 to 2014, showing the variation in lambda explained by (A) adult (>2.5 years) survival (r2 = 0.70), (B) juvenile survival (r2 = 0.08), (C) neonate survival (r2 = 0.06), (D) pregnancy status (r2 = 0.08), (E) parturition status (r2 = 0.07), and (F) proportion of variation in λ explained by each vital rate (see Table 2 for annual estimates) in western Wyoming, United States.
The covariate LSA revealed a strong influence of IFBFat on population growth rate, explaining 82% of variation in λ through its combined influence on overwinter survival of adults, parturition, and pregnancy (Figure 4). Only 0.7% of the variation in λ was explained by drought conditions from the previous summer.
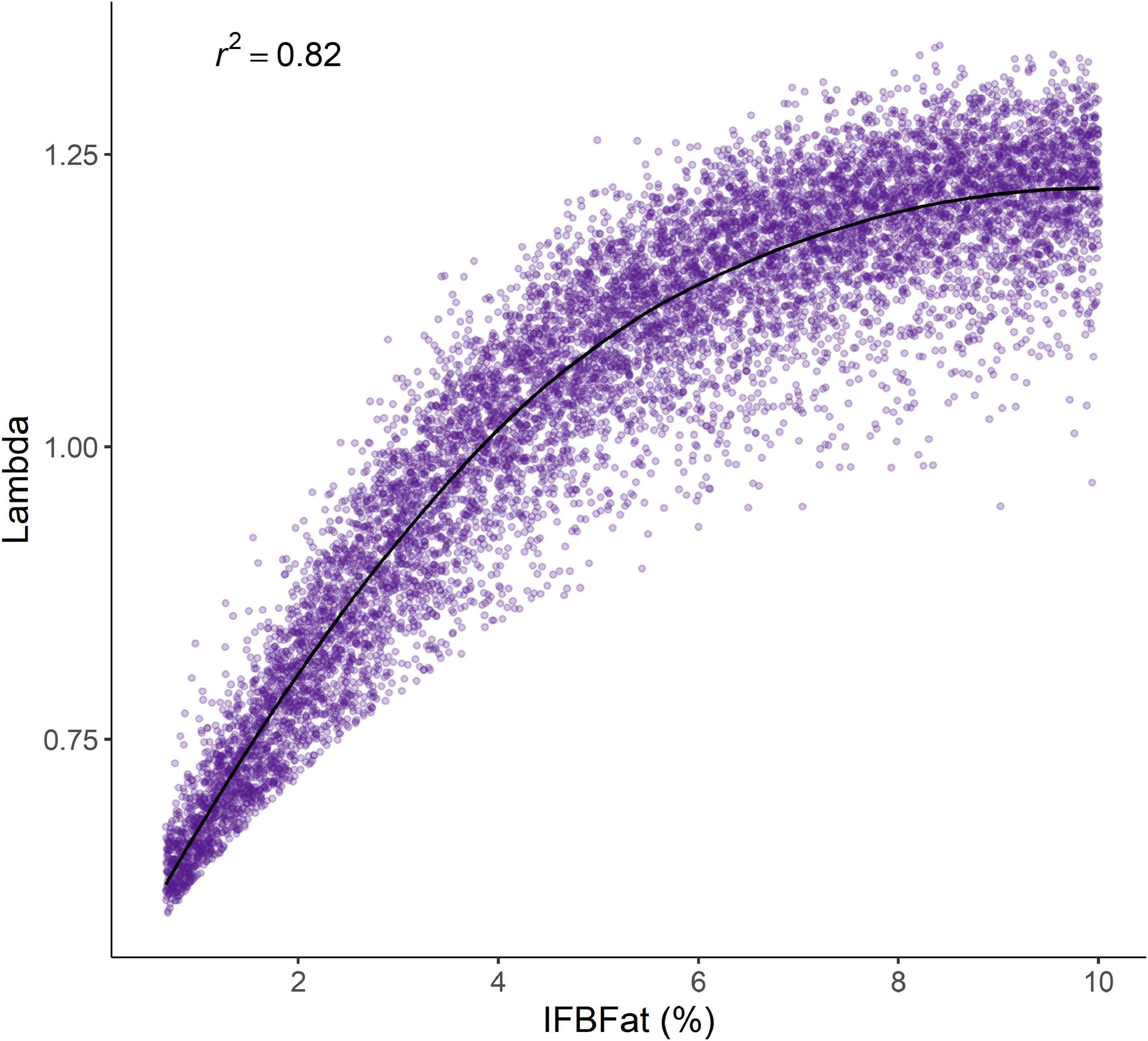
Figure 4. The variation in λ (fitted with smoothing loess) explained by the cumulative effects of percent IFBFat on pregnancy, parturition, and overwinter survival of adult (>2.5 years) female moose in Sublette 2012–2014, western Wyoming, United States.
Discussion
Our study illustrates how measurements of nutritional condition can better characterize degree of resource limitation in populations of a large herbivore than remotely sensed metrics of environmental variation. Although we did not measure density dependence directly, we suspect that the influence of nutritional condition at least partially reflects a key role of density dependence operating within a population of moose largely free of large predators with low female harvest—a conclusion that would have been impossible to draw based on inference from remotely sensed environmental measures as proxies of resource limitation. Lambda was explained almost entirely by nutritional condition of females, which clearly illustrated that this population was resource limited and likely experiencing density dependence at a level that obscured the effects of environmental variation detected from remotely sensed data. Moreover, response and variability of vital rates to resource limitation for a population of moose at the southern extent of its distribution conflicted with the Eberhardt paradigm of life history for long-lived ungulates. Despite the typical vulnerability of juveniles and robustness of adults to resource limitation (Gaillard and Yoccoz, 2003), nutritional condition of adults strongly influenced survival, yet survival of juveniles had little predictive power. Further, our LSA results depart from typical expectations for the life-history characteristics of ungulates, where variation in juvenile survival typically fluctuates widely and underpins variation in population growth (Gaillard et al., 1998, 2000). In our study, annual changes in rates of population growth were driven mostly by variation in survival of adults, whereas survival of juveniles explained substantially less variation. Our findings serve as a reminder that the influences of top-down and bottom-up forces are context-dependent relative to a population’s proximity to K, and that the relative influence of each factor is obscured or magnified depending on the strength of density dependence.
The detection of resource limitation in Sublette moose through the lens of vital rates more clearly revealed the potential for interacting relationships from top-down and bottom-up forces, and the importance of context dependence in ungulate demography. In our study, signals of drought influencing population growth rates were barely detected, yet we can logically infer that drought negatively influenced levels of body fat in moose. Warm temperatures during spring and summer reduce forage quality for ungulates (Hamel et al., 2009b), thereby limiting fat gains during the growing season that are necessary for reproduction and overwinter survival (Monteith et al., 2014). Indeed, in response to drought, Sublette moose experienced reduced rates of parturition, as well as low rates of twinning (Table 2), a symptom of resource limitation in moose (Boertje et al., 2007). A lack of predators combined with strong resource limitation revealed strong relationships between nutritional condition and vital rates. Measurements of nutritional condition thus simultaneously integrate disparate influences on resource limitation, and as a result, they are more informative than remotely sensed covariates in linking bottom-up effects to ungulate demography.
As prey populations approach K and competition for food increases, nutritional condition declines, and animals are consequently more sensitive to environmental variation (Aanes et al., 2000; Kie et al., 2003; Monteith et al., 2015), parasites, and disease. Effects of remotely sensed data on vital rate variability of moose in Sublette were subtle, and could have led to a conclusion of weak resource limitation in an area that was almost devoid of large predators. Nevertheless, measures of nutritional condition explained most of the variation in survival of adults, pregnancy, and parturition, leading to a cumulative explanation for 82% of the variation in λ (Figure 4). The striking connections between nutritional condition, and both individual and population performance were unequivocally indicative of a population regulated strongly by resource limitation and, presumably, density dependence which apparently obscured signals of environmental influence from remotely sensed data. In other words, measures of nutritional condition were a more precise representation of resource limitation when compared with remotely sensed proxies such NDVI and drought, even when those proxies were explicitly measured in a spatial and temporal context. There is increasing support for using remotely sensed data to detect resource limitation in ungulates (Tveraa et al., 2007; Pettorelli et al., 2011; Bastille-Rousseau et al., 2015; Monteith et al., 2015); however, our work provides evidence that these relationships can go undetected if integrative measures of resource limitation (e.g., nutritional condition) are not considered. Short-term studies such as ours are common for implementing management actions, and remotely sensed data might not be sufficient for detecting strong resource limitation. Similarly, Stewart et al. (2005) noted that signatures of resource limitation can be dampened in populations experiencing heightened density dependence. In Sublette moose, relationships between vital rates and environmental covariates were relatively weak compared with IFBFat, highlighting the potential for Type II errors in relating only remotely sensed covariates to ungulate demography. We recommend that future studies examining the effects of bottom-up forcing on ungulates carefully consider multiple pathways before declaring the absence of either pressure, and we also agree with others (Cook et al., 2004; Monteith et al., 2014; Stephenson et al., 2020) that nutritional condition is an effective indicator of resource limitation because it integrates both environmental variation and density dependence.
Our results depart from the life-history paradigm proposed by Eberhardt (2002), and deviate from life-history characteristics typical of ungulates (Gaillard et al., 2000). Juveniles are typically more sensitive to environmental variation than adults and are commonly the first stage class to experience declines as populations approach K (Eberhardt, 2002), ultimately driving interannual variability in λ (Gaillard et al., 2000). Nevertheless, adult survival was highly variable and explained the majority of variation in λ for our study population. Variable survival of adults is considered rare for ungulates, although exceptions have been noted in declining populations exposed to predators, including bighorn sheep (Johnson et al., 2010), mountain caribou (Rangifer tarandus) (Hervieux et al., 2013), and tropical ungulates (Owen-Smith and Mason, 2005). Notably, variable survival of adults in Sublette in response to suppressed nutritional condition suggests that the population surpassed K, despite an estimated stable-to-increasing population growth rate. Moreover, survival of neonates and juveniles was not sensitive to nutritional condition. As intraspecific competition for resources increases, the detection of reproductive tradeoffs (lower juvenile survival) relative to environmental variation may be confounded by factors difficult to measure, such as experience in rearing young (Hamel et al., 2009a), heterogeneity of genotypic quality (van Noordwijk and de Jong, 1986), and immune function (Downs et al., 2015; Cheynel et al., 2017). There are multiple factors that can influence vital rates, and as such, their expected patterns (Gaillard et al., 2000) may be more context dependent than previously appreciated.
Our findings provide three important contributions that can help advance our understanding of resource limitation in ungulate populations. First, in our LSA, variation in adult survival strongly affected lambda, which is notable since adult survival is typically robust to environmental variation. Second, measurements of nutritional condition proved to be considerably more informative for detecting resource limitation compared with multiple proxies of environmental variation via remote sensing, indicating that seemingly intuitive relationships between proxies of resource limitation and vital rates can be obscured in ungulate populations experiencing strong density dependence. Finally, we documented low variability in survival of young that had little influence on λ, in contrast to expectations (Gaillard et al., 2000). Our results indicate that the life-history paradigm for long-lived ungulates may be more variable across taxa than originally proposed, and moose at the southern extent of their range may uniquely deviate from theoretical expectations.
Data Availability Statement
The datasets generated for this study are available on request to the corresponding author.
Ethics Statement
The study was reviewed and approved by the University of Wyoming Institutional Animal Care and Use Committee.
Author Contributions
BO, MK, KM, JG, and JM conceived the ideas and designed methodology. BO analyzed the data and led the writing of the manuscript. All authors collected the data, critically contributed to the drafts, and gave final approval for publication.
Funding
This study was funded by the US Forest Service (Bridger-Teton), Safari Club International Foundation, Sublette County Outfitters and Guides Association, Wyoming Outfitters and Guides Association, Wyoming Governor’s Big Game License Coalition, the Wyoming Governor’s Office, Plains Exploration and Production, Tim Delaney of Rolling Thunder Ranch LLC, Wyoming Game and Fish Department Commissioner Price (Commissioner license), Jim Finley of Finley Resources, Inc., Teton Conservation District, and Ricketts Conservation Foundation. Any use of trade, firm, or product names is for descriptive purposes only and does not imply endorsement by the U.S. Government.
Conflict of Interest
The authors declare that the research was conducted in the absence of any commercial or financial relationships that could be construed as a potential conflict of interest.
Acknowledgments
We thank Native Range Capture Services, Inc., Savage Air Services, Gallatin Flying Service, and Sky Aviation for safe piloting. We are indebted to landowners of Sublette County, Gary Hanvey (retired) of the Bridger-Teton National Forest, and personnel from Grand Teton National Park, and the Wyoming Game and Fish Department for logistical support. We thank T. McDonald of WEST, Inc. for statistical advice. This manuscript contains content that can also be referenced in Oates (2016).
References
Aanes, R., Sæther, B.-E., and Øritsland, N. A. (2000). Fluctuations of an introduced population of Svalbard reindeer: the effects of density dependence and climatic variation. Ecography 23, 437–443. doi: 10.1111/j.1600-0587.2000.tb00300.x
Andersen, P. K., and Gill, R. D. (1982). Cox’s regression model for counting processes: a large sample study. Ann. Statist. 10, 1100–1120. doi: 10.1214/aos/1176345976
Babyak, M. A. (2004). What you see may not be what you get: a brief, nontechnical introduction to overfitting in regression-type models. Psychosomatic Med. 66, 411–421. doi: 10.1097/01.psy.0000127692.23278.a9
Ballard, W. B., Whitman, J. S., and Reed, D. J. (1991). Population Dynamics of Moose in South-Central Alaska. Wildlife Monographs 55, 3–49.
Bangs, E. E., and Fritts, S. H. (1996). Reintroducing the gray wolf to central Idaho and Yellowstone National Park. Wildlife Soc. Bull. 24, 402–413.
Barber-Meyer, S. M., Mech, L. D., and White, P. J. (2008). Elk calf survival and mortality following wolf restoration to Yellowstone National Park. Wildlife Monographs 2008, 1–30. doi: 10.2193/2008-2004
Bastille-Rousseau, G., Schaefer, J. A., Lewis, K. P., Mumma, M., Ellington, E. H., Rayl, N. D., et al. (2015). Phase-dependent climate-predator interactions explain three decades of variation in neonatal caribou survival. J. Anim. Ecol. 85, 445–456. doi: 10.1111/1365-2656.12466
Becker, S. A., Kauffman, M. J., and Anderson, S. H. (2010). Nutritional condition of adult female Shiras moose in northwest Wyoming. Alces 46, 151–166.
Berger, J., Swenson, J. E., and Persson, I.-L. (2001). Recolonizing carnivores and naïve prey: conservation lessons from pleistocene extinctions. Science 291, 1036–1039. doi: 10.1126/science.1056466
Bergeron, D. H., Pekins, P. J., and Rines, K. (2013). Temporal assessment of physical characteristics and reproductive status of moose in New Hampshire. Alces 49, 39–48.
Boertje, R. D., Ellis, M. M., and Kellie, K. A. (2015). Accuracy of moose age determinations from canine and incisor cementum annuli. Wildlife Soc. Bull. 39, 383–389. doi: 10.1002/wsb.537
Boertje, R. D., Kellie, K. A., Seaton, C. T., Keech, M. A., Young, D. D., Dale, B. W., et al. (2007). Ranking alaska moose nutrition: signals to begin liberal antlerless harvests. J. Wildlife Manag. 71, 1494–1506. doi: 10.2193/2006-2159
Bonenfant, C., Gaillard, J. M., Coulson, T., Festa-Bianchet, M., Loison, A., Garel, M., et al. (2009). Empirical evidence of density-dependence in populations of large herbivores. Adv. Ecol. Res. 41, 313–357. doi: 10.1016/s0065-2504(09)00405-x
Bowyer, R. T., Bleich, V. C., Stewart, K. M., Whiting, J. C., and Monteith, K. L. (2014). Density dependence in ungulates: a review of causes, and concepts with some clarifications. California Fish Game 100, 550–572.
Bowyer, R. T., Kie, J. G., Person, D. K., and Monteith, K. L. (2013). Metrics of predation: perils of predator-prey ratios. Acta Theriol. 58, 329–340. doi: 10.1007/s13364-013-0133-131
Boyce, M. S., Sinclair, A. R. E., and White, G. C. (1999). Seasonal compensation of predation and harvesting. Oikos 87, 419–426. doi: 10.2307/3546808
Bunnefeld, N., Borger, L., van Moorter, B., Rolandsen, C. M., Dettki, H., Solberg, E. J., et al. (2011). A model-driven approach to quantify migration patterns: individual, regional and yearly differences. J. Anim. Ecol. 80, 466–476. doi: 10.1111/j.1365-2656.2010.01776.x
Burnham, K., and Anderson, D. (2002). Information and Likelihood Theory: a Basis for Model Selection and Inference. New York, NY: Springer-Verlag.
Buskirk, S. W. (2016). Wild Mammals of Wyoming and Yellowstone National Park. Berkeley, CA: Univ of California Press.
Cheynel, L., Lemaître, J. F., Gaillard, J. M., Rey, B., Bourgoin, G., Ferté, H., et al. (2017). Immunosenescence patterns differ between populations but not between sexes in a long-lived mammal. Sci. Rep. 7:13700. doi: 10.1038/s41598-017-13686-13685
Cook, J. G., Johnson, B. K., Cook, R. C., Riggs, R. A., Delcurto, T., Bryant, L. D., et al. (2004). Effects of summer-autumn nutrition and parturition date on reproduction and survival of elk. Wildlife Monographs 155, 1–61. doi: 10.1002/wmon.1020
Cook, R. C., Cook, J. G., Murray, D. L., Zager, P., Johnson, B. K., and Gratson, M. W. (2001). Development of predictive models of nutritional condition for Rocky Mountain elk. J. Wildlife Manag. 65, 973–987. doi: 10.2307/3803046
Cook, R. C., Cook, J. G., Stephenson, T. R., Myers, W. L., McCorquodale, S. M., Vales, D. J., et al. (2010). Revisions of rump fat and body scoring indices for deer, elk, and moose. J. Wildlife Manag. 74, 880–896. doi: 10.2193/2009-2031
Cook, R. C., Cook, J. G., Vales, D. J., Johnson, B. K., McCorquodale, S. M., Shipley, L. A., et al. (2013). Regional and seasonal patterns of nutritional condition and reproduction in elk. Wildlife Monographs 184, 1–45. doi: 10.1002/wmon.1008
Cook, R. C., Stephenson, T. R., Myers, W. L., Cook, J. G., and Shipley, L. A. (2007). Validating predictive models of nutritional condition for mule deer. J. Wildlife Manag. 71, 1934–1943. doi: 10.2193/2006-2262
Coulson, T., Catchpole, E. A., Albon, S. D., Morgan, B. J., Pemberton, J., Clutton-Brock, T. H., et al. (2001). Age, sex, density, winter weather, and population crashes in Soay sheep. Science 292, 1528–1531. doi: 10.1126/science.292.5521.1528
Cox, D. R. (1972). Regression models and life-tables. J. R. Statist. Soc. Ser. B (Methodological) 34, 187–220.
Daly, C., Neilson, R. P., and Phillips, D. L. (1994). A statistical-topographic model for mapping climatological precipitation over mountainous terrain. J. Appl. Meteorol. 33, 140–158. doi: 10.1175/1520-0450(1994)033<0140:astmfm>2.0.co;2
DelGiudice, G. D., Rolf, O., Peterson, William, M., and Samuel. (1997). Trends of winter nutritional restriction, ticks and numbers of moose on Isle Royale. J. Wildlife Manag. 61, 895–903. doi: 10.2307/3802198
DelGiudice, G. D., Sampson, B. A., Lenarz, M. S., Schrage, M. W., and Edwards, A. J. (2011). Winter body condition of moose (alces Alces) in a declining population. J. Wildlife Dis. 47, 30–40. doi: 10.7589/0090-3558-47.1.30
DeYoung, R. W., Hellgren, E. C., Fulbright, T. E., Robbins, W. F., and Humphreys, I. D. (2000). Modeling nutritional carrying capacity for translocated desert bighorn sheep in Western Texas. Restorat. Ecol. 8, 57–65. doi: 10.1046/j.1526-100x.2000.80066.x
Dodge, W. B. Jr., Winterstein, S. R., Beyer, D. E. Jr., and Campa, H. III (2004). Survival, reproduction, and movements of moose in the western Upper Peninsula of Michigan. Alces 40, 71–85.
Doherty, P. F., White, G. C., and Burnham, K. P. (2012). Comparison of model building and selection strategies. J. Ornithol. 152, 317–323. doi: 10.1007/s10336-010-0598-595
Downs, C. J., Stewart, K. M., and Dick, B. L. (2015). Investment in constitutive immune function by North American Elk experimentally maintained at two different population densities. PLoS One 10:e0125586. doi: 10.1371/journal.pone.0125586
Eberhardt, L. (2002). A paradigm for population analysis of long-lived vertebrates. Ecology 83, 2841–2854. doi: 10.1890/0012-9658(2002)083[2841:apfpao]2.0.co;2
Ericsson, G., Ball, J. P., and Danell, K. (2002). Body mass of moose calves along an altitudinal gradient. J. Wildlife Manag. 66, 91–97. doi: 10.2307/3802875
Festa-Bianchet, M., Gaillard, J. M., and Côté, S. D. (2003). Variable age structure and apparent density dependence in survival of adult ungulates. J. Anim. Ecol. 72, 640–649. doi: 10.1046/j.1365-2656.2003.00735.x
Fieberg, J., and DelGiudice, G. D. (2009). What time is it? choice of time origin and scale in extended proportional hazards models. Ecology 90, 1687–1697. doi: 10.1890/08-0724.1
Franzmann, A. W. (1977). Condition assessment of Alaskan moose. Proc. North Am. Moose Conf. Workshop 13, 119–127.
Gaillard, J.-M., Festa-Bianchet, M., Yoccoz, N., Loison, A., and Toigo, C. (2000). Temporal variation in fitness components and population dynamics of large herbivores. Ann. Rev. Ecol. Systemat. 31, 367–393. doi: 10.1146/annurev.ecolsys.31.1.367
Gaillard, J.-M., Festa-Bianchet, M., and Yoccoz, N. G. (1998). Population dynamics of large herbivores: variable recruitment with constant adult survival. Trends Ecol. Evol. 13, 58–63. doi: 10.1016/s0169-5347(97)01237-8
Gaillard, J.-M., and Yoccoz, N. G. (2003). Temporal variation in survival of mammals: a case of environmental canalization? Ecology 84, 3294–3306. doi: 10.1890/02-0409
Hairston, N. G., Smith, F. E., and Slobodkin, L. B. (1960). Community structure, population control, and competition. Am. Nat. 94, 421–425. doi: 10.1086/282146
Hamel, S., Côté, S. D., and Festa-Bianchet, M. (2010). Maternal characteristics and environment affect the costs of reproduction in female mountain goats. Ecology 91, 2034–2043. doi: 10.1890/09-1311.1
Hamel, S., Côté, S. D., Gaillard, J.-M., and Festa-Bianchet, M. (2009a). Individual variation in reproductive costs of reproduction: high-quality females always do better. J. Anim. Ecol. 78, 143–151. doi: 10.1111/j.1365-2656.2008.01459.x
Hamel, S., Garel, M., Festa-Bianchet, M., Gaillard, J.-M., and Côté, S. D. (2009b). Spring Normalized Difference Vegetation Index (NDVI) predicts annual variation in timing of peak faecal crude protein in mountain ungulates. J. Appl. Ecol. 46, 582–589. doi: 10.1111/j.1365-2664.2009.01643.x
Hanley, J. A., and McNeil, B. J. (1982). The meaning and use of the area under a receiver operating characteristic (ROC) curve. Radiology 143, 29–36. doi: 10.1148/radiology.143.1.7063747
Hansen, B. B., Gamelon, M., Albon, S. D., Lee, A. M., Stien, A., Irvine, R. J., et al. (2019). More frequent extreme climate events stabilize reindeer population dynamics. Nat Commun. 10:1616. doi: 10.1038/s41467-019-09332-9335
Harrell, F. (2015). Regression Modeling Strategies: with Applications to Linear Models, Logistic and Ordinal Regression, and Survival Analysis. Berlin: Springer.
Hebblewhite, M., Merrill, E., and McDermid, G. (2008). A multi-scale test of the forage maturation hypothesis in a partially migratory ungulate population. Ecol. Monographs 78, 141–166. doi: 10.1890/06-1708.1
Hebblewhite, M., Whittington, J., Bradley, M., Skinner, G., Dibb, A., and White, C. A. (2007). Conditions for caribou persistence in the wolf-elk-caribou systems of the Canadian Rockies. Rangifer 27, 79–91. doi: 10.7557/2.27.4.322
Herfindal, I., Sæther, B. E., Solberg, E. J., Andersen, R., and Høgda, K. A. (2006). Population characteristics predict responses in moose body mass to temporal variation in the environment. J. Anim. Ecol. 75, 1110–1118. doi: 10.1111/j.1365-2656.2006.01138.x
Hervieux, D., Hebblewhite, M., DeCesare, N. J., Russell, M., Smith, K., Robertson, S., et al. (2013). Widespread declines in woodland caribou (Rangifer tarandus caribou) continue in Alberta. Can. J. Zool. 91, 872–882. doi: 10.1139/cjz-2013-0123
Hjeljord, O., and Histøl, T. (1999). Range-body mass interactions of a northern ungulate–a test of hypothesis. Oecologia 119, 326–339. doi: 10.1007/s004420050793
Hopcraft, J. G., Olff, H., and Sinclair, A. R. (2010). Herbivores, resources and risks: alternating regulation along primary environmental gradients in savannas. Trends Ecol. Evol. 25, 119–128. doi: 10.1016/j.tree.2009.08.001
Huang, F., Cockrell, D. C., Stephenson, T. R., Noyes, J. H., and Sasser, R. G. (2000). A serum pregnancy test with a specific radioimmunoassay for moose and elk pregnancy-specific protein B. J. Wildlife Manag. 64, 492–499. doi: 10.2307/3803246
Jesmer, B. R., Goheen, J. R., Monteith, K. L., and Kauffman, M. J. (2017). State-dependent behavior alters endocrine–energy relationship: implications for conservation and management. Ecol. Appl. 27, 2303–2312. doi: 10.1002/eap.1608
Johnson, H. E., Mills, L. S., Stephenson, T. R., and Wehausen, J. D. (2010). Population-specific vital rate contributions influence management of an endangered ungulate. Ecol. Appl. 20, 1753–1765. doi: 10.1890/09-1107.1
Keech, M. A., Bowyer, R. T., Jay, M., Hoef, V., Boertje, R. D., Dale, B. W., et al. (2000). Life-history consequences of maternal condition in Alaskan moose. J. Wildlife Manag. 64, 450–462. doi: 10.2307/3803243
Keech, M. A., Lindberg, M. S., Boertje, R. D., Valkenburg, P., Taras, B. D., Boudreau, T. A., et al. (2011). Effects of predator treatments, individual traits, and environment on moose survival in Alaska. J. Wildlife Manag. 75, 1361–1380. doi: 10.1002/jwmg.188
Kie, J. G., Bowyer, R. T., and Stewart, K. M. (2003). “Ungulates in western coniferous forests: habitat relationships, population dynamics, and ecosystem processes,” in Mammal Community Dynamics: Management and Conservation in the Coniferous forests of Western North America, eds C. Zabel and R. G. Anthony (New York, NY: Cambridge University Press).
Kranstauber, B., Kays, R., LaPoint, S., Wikelski, M., and Safi, K. (2012). A dynamic Brownian bridge movement model to estimate utilization distributions for heterogeneous animal movement. J. Anim. Ecol. 81, 738–746. doi: 10.1111/j.1365-2656.2012.01955.x
Kunkel, K., and Pletscher, D. H. (1999). Species-specific population dynamics of cervids in a multipredator ecosystem. J. Wildlife Manag. 63, 1082–1093. doi: 10.2307/3802827
Lenarz, M. S., Nelson, M. E., Schrage, M. W., and Edwards, A. J. (2009). Temperature mediated moose survival in Northeastern Minnesota. J. Wildlife Manag. 73, 503–510. doi: 10.2193/2008-2265
McCullough, D. R. (1979). The George Reserve Deer Herd: Population Ecology of a K-selected Species. Ann Arbor, MI: University of Michigan Press.
McLaren, B., McCarthy, C., and Mahoney, S. (2000). Extreme moose demographics in Gros Morne National Park. Newfoundland. Alces 36, 217–233.
McNamara, J. M., and Houston, A. I. (1987). Starvation and predation as factors limiting population size. Ecology 68, 1515–1519. doi: 10.2307/1939235
Merkle, J. A., Monteith, K. L., Aikens, E. O., Hayes, M. M., Hersey, K. R., Middleton, A. D., et al. (2016). Large herbivores surf waves of green-up during spring. Proc. R. Soc. London B: Biol. Sci. 283:20160456. doi: 10.1098/rspb.2016.0456
Monteith, K. L., Bleich, V. C., Stephenson, T. R., Pierce, B. M., Conner, M. M., Kie, J. G., et al. (2014). Life-history characteristics of mule deer: effects of nutrition in a variable environment. Wildlife Monographs 186, 1–62. doi: 10.1002/wmon.1011
Monteith, K. L., Klaver, R. W., Hersey, K. R., Holland, A. A., Thomas, T. P., and Kauffman, M. J. (2015). Effects of climate and plant phenology on recruitment of moose at the southern extent of their range. Oecologia 178, 1137–1148. doi: 10.1007/s00442-015-3296-3294
Murray, D. L., Cox, E. W., Ballard, W. B., Whitlaw, H. A., Lenarz, M. S., Custer, T. W., et al. (2006). Pathogens, nutritional deficiency, and climate influences on a declining moose population. Wildlife Monographs. 166, 1–30. doi: 10.2193/0084-0173(2006)166[1:pndaci]2.0.co;2
Musante, A. R., Pekins, P. J., and Scarpitti, D. L. (2010). Characteristics and dynamics of a regional moose Alces alces population in the northeastern United States. Wildlife Biol. 16, 185–204. doi: 10.2981/09-014
Oates, B. A. (2016). Effects of Predators and Resource Limitation on Demography and Behavior of Moose in the Greater Yellowstone Ecosystem. MSc thesis, University of Wyoming: Laramie, WY.thesis
Owen-Smith, N., and Mason, D. R. (2005). Comparative changes in adult vs. juvenile survival affecting population trends of African Ungulates. J. Anim. Ecol. 74, 762–773. doi: 10.1111/j.1365-2656.2005.00973.x
Owen-Smith, N., Mason, D. R., and Ogutu, J. O. (2005). Correlates of survival rates for 10 African ungulate populations: density, rainfall and predation. J. Anim. Ecol. 74, 774–788. doi: 10.1111/j.1365-2656.2005.00974.x
Owen-Smith, N., and Mills, M. G. L. (2006). Manifold interactive influences on the population dynamics of a multispecies ungulate assemblage. Ecol. Monographs 76, 73–92. doi: 10.1890/04-1101
Parker, K. L., Barboza, P. S., and Gillingham, M. P. (2009). Nutrition integrates environmental responses of ungulates. Funct. Ecol. 23, 57–69. doi: 10.1111/j.1365-2435.2009.01528.x
Peterson, R. (1977). Wolf Ecology and Prey Relationships on Isle Royale US National Park Service Scientific Monograph Series 11. Washington, DC: US Government Printing Office.
Peterson, R. O. (1999). Wolf–moose interaction on isle royale: the end of natural regulation? Ecol. Appl. 9, 10–16. doi: 10.2307/2641163
Peterson, R. O., and Allen, D. (1974). Snow conditions as a parameter in moose-wolf relationships. Nat. Can. 101, 481–492.
Pettorelli, N., Pelletier, F., Hardenberg, A. V., Festa-Bianchet, M., and Côté, S. D. (2007). Early onset of vegetation growth vs. rapid green-up: impacts on juvenile mountain ungulates. Ecology 88, 381–390. doi: 10.1890/06-0875
Pettorelli, N., Ryan, S., Mueller, T., Bunnefeld, N., Jedrzejewska, B., Lima, M., et al. (2011). The Normalized Difference Vegetation Index (NDVI): unforeseen successes in animal ecology. Climate Res. 46, 15–27. doi: 10.3354/cr00936
Pierce, B. M., Bleich, V. C., Monteith, K. L., and Bowyer, R. T. (2012). Top-down versus bottom-up forcing: evidence from mountain lions and mule deer. J. Mammal. 93, 977–988. doi: 10.1644/12-mamm-a-014.1
Pollock, K. H., Winterstein, S. R., Bunck, C. M., and Curtis, P. D. (1989). Survival analysis in telemetry studies: the staggered entry design. J. Wildlife Manag. 53, 7–15. doi: 10.2307/3801296
Poole, K. G., Serrouya, R., and Stuart-Smith, K. (2007). Moose calving strategies in interior montane ecosystems. J. Mammal. 88, 139–150. doi: 10.1644/06-mamm-a-127r1.1
Portier, C., Festa-Bianchet, M., Gaillard, J. M., Jorgenson, J., and Yoccoz, N. (1998). Effects of density and weather on survival of bighorn sheep lambs (Ovis canadensis). J. Zool. 245, 271–278. doi: 10.1111/j.1469-7998.1998.tb00101.x
R Core Team (2020). R: A Language and Environment for Statistical Computing. Vienna: R Foundation for Statistical Computing.
Raithel, J. D., Kauffman, M. J., and Pletscher, D. H. (2007). Impact of spatial and temporal variation in calf survival on the growth of Elk populations. J. Wildlife Manag. 71, 795–803. doi: 10.2193/2005-2608
Samuel, W. (2007). Factors affecting epizootics of winter ticks and mortality of moose. Alces 43, 39–48.
Schwartz, C. C. (1998). “Reproduction, natality and growth,” in Ecology and Management of the North American Moose, 2nd Edn, eds A. W. Franzmann and C. C. Schwartz (Washington, DC: Smithsonian Institution Press), 141–171.
Simard, A. M., Côté, S. D., Weladji, R. B., and Huot, J. (2008). Feedback effects of chronic browsing on life-history traits of a large herbivore. J. Anim. Ecol. 77, 678–686. doi: 10.1111/j.1365-2656.2008.01374.x
Sinclair, A. R., and Krebs, C. J. (2002). Complex numerical responses to top-down and bottom-up processes in vertebrate populations. Philos. Trans. R. Soc. Lond. B Biol. Sci. 357, 1221–1231. doi: 10.1098/rstb.2002.1123
Stephenson, T. R., German, D. W., Cassirer, E. F., Walsh, D. P., Blum, M. E., Cox, M., et al. (2020). Linking population performance to nutritional condition in an alpine ungulate. J. Mammal. 101, 1244–1256. doi: 10.1093/jmammal/gyaa091
Stephenson, T. R., Hundertmark, K. J., Schwartz, C. C., and Van Ballenberghe, V. (1998). Predicting body fat and body mass in moose with ultrasonography. Can. J. Zool. 76, 717–722. doi: 10.1139/cjz-76-4-717
Stephenson, T. R., Van Ballenberghe, V., Peek, J. M., and MacCracken, J. G. (2006). Spatio-Temporal constraints on moose habitat and carrying capacity in coastal alaska: vegetation succession and climate. Rangeland Ecol. Manag. 59, 359–372. doi: 10.2111/04-063.1
Stewart, K. M., Bowyer, R. T., Dick, B. L., Johnson, B. K., and Kie, J. G. (2005). Density-dependent effects on physical condition and reproduction in North American elk: an experimental test. Oecologia 143, 85–93. doi: 10.1007/s00442-004-1785-y
Therneau, T. M., and Grambsch, P. M. (2000). Modeling Survival Data: Extending the Cox Model. Berlin: Springer Science & Business Media.
Thornton, P. E., Running, S. W., and White, M. A. (1997). Generating surfaces of daily meteorological variables over large regions of complex terrain. J. Hydrol. 190, 214–251. doi: 10.1016/s0022-1694(96)03128-9
Tveraa, T., Fauchald, P., Gilles Yoccoz, N., Anker Ims, R., Aanes, R., and Arild Høgda, K. (2007). What regulate and limit reindeer populations in Norway? Oikos 116, 706–715. doi: 10.1111/j.0030-1299.2007.15257.x
van Noordwijk, A. J., and de Jong, G. (1986). Acquisition and allocation of resources: their influence on variation in life history tactics. Am. Nat. 128, 137–142. doi: 10.1086/284547
Vartanian, J. M. (2011). Habitat Condition and the Nutritional Quality of Seasonal Forage and Diets: Demographic Implications for a Declining Moose Population in Northwest Wyoming, USA. MSc thesis, University of Wyoming, Laramie: Wyoming, WYthesis
Keywords: bottom-up, life stage simulation analysis, survival, ungulate, density dependence, vital rate, moose, population
Citation: Oates BA, Monteith KL, Goheen JR, Merkle JA, Fralick GL and Kauffman MJ (2021) Detecting Resource Limitation in a Large Herbivore Population Is Enhanced With Measures of Nutritional Condition. Front. Ecol. Evol. 8:522174. doi: 10.3389/fevo.2020.522174
Received: 21 December 2019; Accepted: 31 December 2020;
Published: 28 January 2021.
Edited by:
Paul Richard Krausman, University of Arizona, United StatesReviewed by:
Inger Suzanne Prange, Appalachian Wildlife Research Institute (United States), United StatesJohn Alfred Bissonette, Utah State University, United States
Copyright © 2021 Oates, Monteith, Goheen, Merkle, Fralick and Kauffman. This is an open-access article distributed under the terms of the Creative Commons Attribution License (CC BY). The use, distribution or reproduction in other forums is permitted, provided the original author(s) and the copyright owner(s) are credited and that the original publication in this journal is cited, in accordance with accepted academic practice. No use, distribution or reproduction is permitted which does not comply with these terms.
*Correspondence: Brendan A. Oates, YmFvYXRlc0BnbWFpbC5jb20=
†Present address: Brendan A. Oates, Washington Department of Fish and Wildlife, Ellensburg, WA, United States