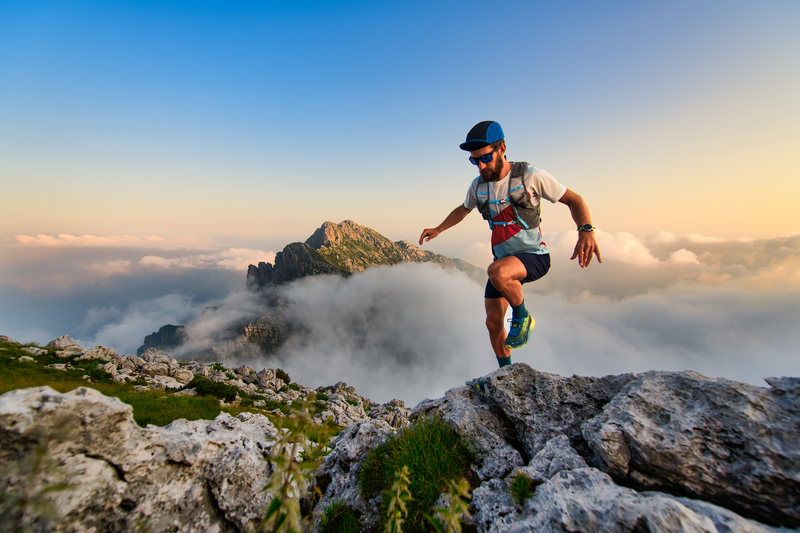
94% of researchers rate our articles as excellent or good
Learn more about the work of our research integrity team to safeguard the quality of each article we publish.
Find out more
ORIGINAL RESEARCH article
Front. Ecol. Evol. , 10 June 2020
Sec. Behavioral and Evolutionary Ecology
Volume 8 - 2020 | https://doi.org/10.3389/fevo.2020.00169
The use of miniature accelerometer (ACT) data-loggers for remote and continuous recording of animal movement behavior is becoming increasingly common. Until recently, size constraints limited most animal-borne ACT applications to large-bodied species. We capitalized on the ongoing miniaturization and advancement of these technologies and associated computational techniques to develop a framework for long-term, low-frequency ACT monitoring of activity in small and secretive terrestrial species. We achieved this by internally implanting coupled radio transmitters and tri-axial ACTs in rattlesnakes (Crotalus atrox). Periodic field-validation observations of behavior were used to train and test supervised learning models (Random Forest, RF; Generalized Linear Elastic Net, GLMNET) for activity classification. The best performing RF model classified periods of movement and immobility in rattlesnakes with high accuracy (movement = 96%, immobile = 99%), and was applied to extensive ACT field datasets (median = 35 days, range = 6–289 days; N = 12) to produce activity budgets at multiple temporal scales. In general, these cryptic ambush predators were found to be highly sedentary, with activity budgets characterized by long periods of immobility interrupted by punctuated bouts of movement. The same temporal daily activity pattern was conserved across all active seasons (spring, summer non-mating, summer mating, fall), as most movement always occurred during the evening or nocturnal diel periods. Contrary to movement timing, daily movement duration was seasonally variable, as movement increased during the summer-mating season—possibly reflecting a combination of more favorable weather conditions (onset of rainy season) and mate-searching efforts by male rattlesnakes. This radio telemetry-accelerometry (RT-ACT) framework provides an objective and flexible set of data collection and processing procedures for long-term ACT field datasets. Expanding our coarse-scale behavioral classification scheme could provide a foundation for future investigations using the RT-ACT framework to explore relationships between individual behavioral decisions and performance in snakes and other small and secretive terrestrial vertebrates.
When, how, and why an animal moves are fundamental aspects of its biology and ecology, as activity patterns reflect the complex interplay between intrinsic (e.g., sex, physiological state, motivational state) and extrinsic (e.g., environmental conditions, predation, competition) factors that shape behavioral decisions (Nathan et al., 2008; Shepard et al., 2008). Movement also has significant implications for biodiversity conservation, representing a critical mechanism in dispersal, inbreeding avoidance and gene flow, pathogen transmission, and responses to habitat disturbances and other global change phenomena (Holyoak et al., 2008; Nathan et al., 2008; Jeltsch et al., 2013; Chmura et al., 2018). Many modes of animal movement exist; such as migration, mate-searching, defending territories, and foraging (Clobert, 2012), and a key characteristic of these different motivational states is the variety of spatial and temporal scales at which they can occur. Migrations might be distinctly seasonal, occur over days or months, and span from hundreds of meters to over 1,000 kilometers depending on the taxon, whereas foraging movements can occur in multiple bouts over a single day and usually within an animal's home range or territory (Jeltsch et al., 2013). Consequently, to properly characterize the diversity of movement behaviors for a species or population as well as understand the roles of various internal and external factors in shaping various strategies, it is essential to evaluate activity patterns across multiple temporal scales (Yoda and Ropert-Coudert, 2007; Trierweiler et al., 2013; Bauder et al., 2016).
The recent emergence and advancement of sophisticated animal-borne monitoring technologies have circumvented many traditional limitations in field studies of animal movement behavior research (Nathan et al., 2008; Kays et al., 2015; Wassmer et al., 2020). Satellite collars can provide massive quantities of position data over multi-annual time periods, remote sensing technologies offer ever-improving data on environmental conditions, and animal-borne remote-sensing devices, or bio-loggers, have given researchers the ability to monitor behavior at resolutions and precisions historically restricted to laboratories (Ropert-Coudert and Wilson, 2005; Rutz and Hays, 2009; Kays et al., 2015; Allan et al., 2018). Miniaturized accelerometers, for example, are among the most widely used animal-borne data-logger technologies (Shepard et al., 2008; Brown et al., 2013), as they allow remote and continuous recording of animal activity free from limitations of observer presence, terrain, weather, time of day, and scale of space use (Yoda et al., 1999; Wilson et al., 2006; Shepard et al., 2008; Brown et al., 2013). However, to date, most ACT applications with wild-ranging animals have involved relatively large-bodied taxa (Brown et al., 2013), such as freshwater and marine fish (Murchie et al., 2011), marine mammals (Sato et al., 2003), large terrestrial mammals (Grünewälder et al., 2012; Williams et al., 2014; Graf et al., 2015; Wang et al., 2015; Pagano et al., 2017), and pelagic birds (Brown et al., 2013). Some case studies have demonstrated the utility of ACTs with smaller model organisms, such as small terrestrial mammals (Hammond et al., 2016), small tortoises (Lagarde et al., 2008), and large toads (Halsey and White, 2010), and ongoing miniaturization of ACT devices continues to diminish weight restrictions for smaller species. Advancement in sensor technology has now set the stage for researchers to further expand the diversity of organisms amenable to ACT applications.
In addition to morphological challenges with certain study species, significant computational hurdles and a general lack of standardization in data processing and modeling techniques also remain as prominent barriers to pilot ACT applications with novel study organisms (Collins et al., 2015). Although the idiosyncratic nature of individual study systems and species can reduce the transferability of specific protocols, a general methodological framework can still contribute to the standardization of broader procedural checkpoints, including field or lab-based observation approaches and best practices in classification modeling (Patterson et al., 2019). It is therefore essential that ACT studies provide detailed, step-wise descriptions of the full data collection, processing, and modeling procedures.
Snakes are one vertebrate group that has been largely overlooked in animal-borne bio-logger applications (Beaupre, 2016), despite representing intriguing model organisms in this context. As highly secretive and often-nocturnal animals, significant gaps exist in our knowledge of snake biology, ecology, and behavior (Dorcas and Willson, 2009; Durso et al., 2011; Willson and Winne, 2015). Several challenges unique to snakes account for their absence from bio-logging studies, the most prominent being periodic ecdysis prohibiting long-term external attachment of loggers and morphological limitations on the implantation of bulky devices (i.e., small body sizes of most species, and linear body plan). The original use of radio transmitters for longitudinal monitoring of snakes in the 1980s was transformative to field studies of snake ecology (Reinert and Cundall, 1982; Reinert, 1992; Beaupre, 2016). However, coarse measures of movement based on Euclidean distances between relocation points often hinder examination of the mechanisms that shape activity patterns at different temporal scales (Whitaker and Shine, 2003; Lindström et al., 2015). Automated receiving units (ARUs) have been used to obtain continuous movement data for snakes (Davis et al., 2008; Sperry et al., 2013; Ward et al., 2013), but spatial limitations exist with this method (i.e., snake proximity to ARU), and study sites with high topographic relief (e.g., physical barriers to VHF radio signals) exacerbate this constraint. Herein, we aimed to validate long-term, low-frequency (1-Hz) ACT monitoring of activity in the field with Western Diamond-backed Rattlesnakes (Crotalus atrox). Our data collection, processing, and analysis pipeline is intended to provide a general framework for long-term activity monitoring for small and secretive terrestrial animals. We make use of extensive field validation observations of behavior to train supervised learning models for automated classification of coarse behavioral mode (movement vs. immobile). Using optimized models, we establish and evaluate fine-scale activity budgets for wild-ranging C. atrox.
We developed a streamlined data collection, processing, and analysis framework for long-term ACT monitoring in small and secretive terrestrial animals (Figure 1). Radio transmitters (Holohil Systems Ltd., Model SB-2T) and tri-axial ACTs (Technosmart Europe srl., AXY-3, AXY-4) were coupled using a biologically inert resin and internally implanted in study individuals (Reinert and Cundall, 1982). The coupled devices were securely sutured to a rib (Hardy and Greene, 1999, 2000) in the same position and orientation across individuals. Implants (SB-2T = 5 g; AXY = 2.5–7 g) comprised <3% of each individual's body mass at the time of implantation. Radio transmitters had a battery life of 10 months, and ACTs had data storage capacity (1 Gb) that enabled logging for approximately 9 months when recording at 1-Hz. Since our goal was to conduct long-term ACT recording in the field, we selected a low monitoring frequency for our ACTs (1-Hz; equivalent to one acceleration value/sec along each axis) to minimize the trade-off between recording frequency and logging duration. Recent work has demonstrated that high classification accuracy can be achieved with low-frequency monitoring, especially when paired with extensive validation data (i.e., time-matched observations of behavior; Tatler et al., 2018). Data were stored in each device's microprocessor until downloaded via USB connection following extraction from individual rattlesnakes.
Figure 1. Stepwise (Field Data Collection, Supervised Model Validation, Model Application) RT-ACT methodological framework for long-term, low-frequency accelerometer (ACT) data collection, processing, and analysis procedures validated herein. Details regarding each step in the framework can be found within methods sections.
All data collection procedures took place on Indio Mountains Research Station (IMRS) in Hudspeth County, Texas from June 2016 to August 2018. Detailed ecological and physiographic accounts of IMRS can be found in Mata-Silva et al. (2018), DeSantis et al. (2019), and Worthington et al. (2020). Because all study snakes were included in concurrent monitoring of spatial ecology via hand-held radio telemetry, a variety of spatial metrics were calculated to evaluate relationships between traditionally collected spatial data and ACT-derived activity data. These metrics included movement rate (meters per day, MPD), calculated as the straight-line distance between successive relocation points for an individual, distance per movement (DPM), calculated as the mean straight-line distance between relocation points that were ≥5 m apart, and minimum movement frequency (MMF), calculated as the number of movements (≥5 m) made by an individual out of N relocations in a specifically defined time period. Rattlesnakes were relocated at least twice weekly via radio telemetry, at which time individuals were observed for visual validation of ACT signals. These field-validation observations were typically 30 min. in length and occurred at various times of the day (i.e., morning, mid-day, evening, and night) to capture periods of movement and immobility for rattlesnakes. Hand-held video of surface behavior was recorded for later detailed inspection and manual behavioral annotation of ACT training data. All field observations were conducted independently by D. L. DeSantis.
We selected two broad behavioral categories for classification: “movement” and “immobile.” During field observations of ACT-equipped individuals, “movement” was classified as outstretched, full body movement. “Immobile” was an observation of a snake that was not in full-body motion (independent of body posture). To summarize acceleration data and make relationships between ACT signals and behavioral mode more discernable, we calculated a suite of 23 descriptive statistics across the x (forward and backward motion), y (side-to-side motion), and z (upward and downward motion) ACT axes. These summary stats were initially calculated to be “rolling” over varying temporal window sizes (1-s, 10-s, 20-s, and 1-min. period basis) to identify the optimal scale at which to classify activity with the machine learning models. We found a temporal window of 20 s. to be the optimal scale in our classification scheme. Rattlesnake movement behavior is often characterized by frequent and relatively brief pauses attributed to chemo-sensory probing, and, therefore, very fine (1-s.) and very coarse (1-min.) windows could potentially under and over-estimated periods of movement, respectively. Additional ACT studies have presented similar results (Ladds et al., 2017), whereas others have opted for longer windows up to 10 min. in length to improve overall accuracy of behavior classification (Diosdado et al., 2015). Appropriate window size is highly context-dependent, as study species, targeted behaviors for classification, ACT recording frequency, and the selected machine learning algorithms all combine to influence the optimal scale for classification (Jeantet et al., 2018). The “moving” or “rolling” statistics calculated here include the mean (average), standard deviation, minimum, maximum, and slope of a regression model. We also calculated Overall Dynamic Body Acceleration (ODBA) and Vectorial Dynamic Body Acceleration (VeDBA) to summarize signals using measures from the x, y, and z axes. The ODBA and VeDBA measures have been widely used for summarizing acceleration in previous studies (Wilson et al., 2006; Shepard et al., 2008; Gleiss et al., 2011; Bidder et al., 2012), but their usefulness with the unique morphology and movement mechanics of snakes is untested. ODBA is the absolute sum of the dynamic body acceleration (DBA) along the x, y, and z axes.
While VeDBA is the vector of DBA along the x, y, and z axes.
Dynamic body acceleration is the raw acceleration value remaining after the removal of static acceleration due to gravity. Static acceleration was a constant subtracted (−1) from the axis oriented in the “upward and downward” plain, which was the z axis, in the case of our ACT implantation orientation [C. Catoni (Technosmart Europe srl.), personal communication]. The relative importance of different summary statistics in the classification process was visualized by a variable importance plot for the best performing model. Variable importance was measured via mean decreases in the Gini Coefficient (Breiman, 2002; Cutler et al., 2007). Mean decrease in Gini measures how much each variable (summary stat) contributes to the homogeneity of the classification decisions by the model. The coefficient ranges from zero (homogeneous) to one (heterogeneous) and changes in Gini are summed for each variable and averaged at the end of the classification process. Variables that result in classifications with higher precision have a higher decrease in Gini coefficient.
Because of the high-resolution logging of ACTs, the resulting datasets from field deployments are typically very large (herein, routinely exceeding one million data points per individual). As a result, manually labeling periods of behavior within full datasets is practically impossible, making automated labeling via machine learning techniques a critical step in ACT applications. The process of selecting the appropriate model for specific classification tasks can often be complicated and time consuming (Ladds et al., 2017), as a wide variety of algorithms are available for use in classification of behavior from ACT signals. These models range from relatively simple approaches like simple linear discriminant analyses (Nathan et al., 2012) and decision trees (Nishizawa et al., 2013), to more advanced techniques such as random forests (Lush et al., 2016; Ladds et al., 2017; Tatler et al., 2018), support vector machines (Hokkanen et al., 2011), gradient booster machines (Ladds et al., 2016, 2017), artificial neural networks (Banerjee et al., 2012), and Gaussian mixture models (Chimienti et al., 2016). Here, we initially opted to use the k-nearest neighbor clustering algorithm, the random forest (RF), and the generalized linear elastic net (GLMNET). Supervised models make use of algorithms to “learn” (via model training) the relationship between a set of input variables (i.e., summary statistics) and output variables (i.e., assigned classifications). After initial exploratory analyses, we ruled out the k-nearest neighbor clustering approach, as significant variation within our two broad behavioral categories reduced its usefulness. We selected the RF and GLMNET algorithms as they are both reasonable approaches, but assume different solutions to this classification problem. Random forest models have been used extensively in the ACT literature (Lush et al., 2016; Ladds et al., 2017), and often perform well in behavioral classification with a diversity of focal taxa (Tatler et al., 2018). Random forests make use of multiple learning algorithms simultaneously through bootstrapping to find a unified classification tree that corrects for overfitting and noise (Efron and Hastie, 2016). We make use of the default split of for each bootstrapped tree as it decreases bias, but also results in relatively independent trees. This is a powerful methodology since it is relatively efficient and, because of the random selection of data features, should be more accurate (and less biased) than other learning algorithms. We also make use of a generalized linear model (GLM) with an elastic net penalty applied, given that we have a binary predictor (movement, immobile) and data that are highly correlated. In the GLM elastic net model (GLMNET), the regularization path has an elastic net penalty applied on a grid of values for the regularization parameter, lambda. This is intuitively appealing and efficient because it maximizes the estimating equation with respect to the regression parameters over a grid of reasonable values for λ.
The tuning parameter λ controls the strength of the penalty and α controls the type of penalty applied (ranging from a lasso penalty to a ridge penalty). In the model training stage, we specified the cross-validation folds and validated λ across a grid of values for α. We called the elastic net function multiple times and averaged over the results while using the same fold vector for each call. This approach reduces noise and produces an optimum value for α. After the optimal penalty was chosen using inner cross-validation loops, it was applied for modeling and prediction. This is preferred over simultaneously validating α and λ because the tuning parameter is informatively selected in this approach and, hence, an optimal value for both parameters is more likely to be selected.
The RF and GLMNET algorithms provided supervised learning models that were validated using holdout data (test data) prior to making predictions on the full training datasets. Once we illustrated model efficacy, we then used the full set of ACT data (field-collected datasets) for activity classification. The training data was substantial, with 42,224 observations at a 1-Hz resolution (1-Hz = one value recorded per-second along each axis). Using a 20% holdout sample, this allotted 33,713 observations for training and 8,511 for model testing/validation. These data were derived entirely from the time-matched observations of behavior in the field. We assess the correspondence between observed and predicted behavioral state for both models in a confusion matrix reporting classification accuracy, precision, and recall. We also report the training errors and test errors for the elastic net and random forest models. Accuracy is the overall proportion of positive classifications (i.e., predicted matches observed), precision is defined as the proportion of predictions that matched the observed behavior within each category (i.e., movement and immobile), and recall is the proportion of observations within a behavior category that were correctly predicted as that behavior (Campbell et al., 2013). All random forest and GLMNET estimations were performed in R (R Core Team, 2020) using the randomForest (Liaw and Wiener, 2002) and glmnet packages (Friedman et al., 2010), respectively.
Following model validation procedures, we selected the best performing model to classify periods of movement and immobility in the full dataset with 95,599,407 data points (1-Hz resolution). Using these behavioral predictions, we visualized activity budgets (time classified as movement vs. immobile) using radial histograms to explore daily patterns and evaluate intra- and inter-individual variation in movement timing, duration, and frequency. We also explored relationships between daily activity, season, and diel period by fitting a Generalized Linear Mixed (GLMM) effects model with snake ID and day of the year modeled as random effects to account for the non-independence of ACT data across time. Least Squared (LS) mean comparisons with multiplicity adjustments were employed to test for statistical differences in the timing and duration of movement within diel periods across seasons. Linear regressions were performed to explore relationships between ACT-derived movement and concurrently recorded radio telemetry-derived spatial metrics.
In total, 19 rattlesnakes (17 males, two females) were implanted with RT-ACTs and released for field monitoring and recording between June 2016 and August 2018. Because of battery malfunctions in seven units (five ACT failures, two RT failures), only 12 datasets from all male C. atrox with sufficient data-recording durations were included in processing and analysis procedures. Recording durations for these 12 datasets ranged from 6 to 289 days (median = 35 days, mean ± s.e. = 94 ± 29 days) for a cumulative total of 1,131 ACT recording days (Table 1). There were 152 independent field-validation observations conducted on these individuals, culminating in just under 32 h of time-matched observations of behavior that were used in model training procedures. The number of observations vary considerably among individuals (Table 1), in part because of variable logging durations, but also the inability to confirm behavior during relocations when individuals were not visible. Manual annotation of ACT datasets was based on field video (when available) and detailed notes of validation observations. Time-matched behavioral labeling was initially conducted independently by D. L. DeSantis (field observer) and a second time by an individual who was not present for the field observation (to minimize bias in interpretation of video or field notes). In rare cases where annotations were considerably different between labelers for a specific observation, those periods were re-evaluated and discarded if a clear behavioral classification could not be distinguished (often because of poor video quality or inconsistent subject visibility).
Table 1. Body size [Snout-Vent Length (SVL), Body Mass], ACT logging periods, and number of field validation observations for 12 male C. atrox included in processing and analyses.
The RF and GLMNET models were developed using the full set of training data. The GLMNET utilized a two-class logistic link function for cross-validation and incorporates 5-folds and uses the optimum λ and α. This is a strong approach considering the scale of the data and provided we are attempting binary classification. For our datasets, the RF models are arguably a less relevant approach than an elastic net GLM, but one used successfully for behavioral classification in other ACT applications (Lush et al., 2016; Ladds et al., 2017; Tatler et al., 2018). In the RF models, 1,000 random starts for bootstrap-based tree generations were utilized and proximity measures were saved for predictions. Both models (GLMNET and RF) were highly accurate at classifying known periods of movement and immobility in the training data (Tables 2, 3), with the RF model slightly outperforming the GLMNET model. In general, models more accurately classified immobility (RF = 99.3%, GLMNET = 97.7%) than movement (RF = 96.2%, GLMNET = 91.8%), which is not surprising considering the tremendous variation in raw ACT signals during movement (see sample ACT waveform data in Figure 1). A variable importance plot was produced to illustrate the relative rank of each summary statistic used in the classification process for the optimized RF model (Figure 2). Measures of data dispersion (standard deviation, maximum value) were the top five ranking variables, with the standard deviation of values on the x, y, and z ACT axes being the most informative for distinguishing between periods of movement and immobility identified in the training dataset.
Table 3. General Linear Mixed-elastic net (GLMNET) and Random Forest (RF) model specifications with the optimum λ and α values as well as the number of folds for validation.
Figure 2. Variable importance plot for optimized Random Forest model used for classification in full ACT datasets. Y-axis depicts top 15 ranked variables (based on mean decrease in Gini, x-axis) out of 23 summary statistics calculated and used in model training. Note that measures of data dispersion (s.d., maximum) were the most informative variables for classification.
Overall, rattlesnakes were highly sedentary during logging periods (Figure 3), although with considerable inter-individual variation, as overall proportions of time classified as movement per-individual (over entire logging period) ranged from 1.6 to 37% (mean ± s.d.; 13.5 ± 13.9%, Table 4). There was some variation in the proportion of time spent moving within diel periods across seasons, particularly in regard to the increase in movement duration during the summer mating season (Table 4; Figure 4). However, LS mean comparisons with multiplicity adjustments revealed no significant differences in movement timing and duration (relative to diel period) within and between active seasons [spring, summer non-mating (NM), summer mating (M), fall]. The only significant differences detected in diel period movement proportions were between the inactive (winter) and active seasons (Table 4; Figure 4).
Figure 3. Radial histograms depicting activity budgets (time classified as movement vs. immobile) at monthly (A,B) and daily scales (C) for male Crotalus atrox monitored during October 2016 and 2017. Proportions are derived from classifications made by the optimized RF model. Individual bars correspond to a single hour within a 24 h period. The aggregated plot (A) depicts the mean activity budget for all rattlesnakes monitored during October (N = 6). The individual monthly plots (B) depict the mean daily activity budgets for each of the five C. atrox that contributed data across every day of October (N = 5). The daily plot mosaic (C) depicts the proportion of time spent moving (blue) or immobile (pink) within each hour of individual days during the week of October 11–October 17 (2016 or 2017). October was selected for visualization because it had the largest concurrent ACT monitoring sample size among the active season months (Apr–Oct) during the study. Note that although periods of movement are highly infrequent and often temporally dispersed between extensive periods of immobility, there is considerable snake-to-snake variation in patterns.
Table 4. Mean proportions of time spent moving (Movement) within diel periods and seasons over entire ACT logging period for C. atrox (N = 12).
Figure 4. Movement durations (bars denote mean proportion of time classified as “movement” by RF model) within diel periods and seasons over entire ACT logging period for C. atrox (N = 12). Seasons consisted of: Spring [Apr–May], Summer Non-Mating (NM) [Jun–Jul], Summer-Mating (M) [Aug–Sep], Fall [Oct–Nov]), and Winter [Dec–Mar]. Diel periods were: Morning [0601–1000], Diurnal [1001–1800], Evening [1801–2100], Nocturnal [2101–0600]. During the active seasons (spring, summer, fall), activity patterns follow the same general pattern with higher proportions of time spent moving during the evening and nocturnal periods relative to the morning and diurnal periods. Although there is a noticeable increase in movement duration during the summer-mating season (summer-M), those proportions were not significantly different than those during other active season diel periods [spring, summer non-mating (summer NM), fall].
By internally implanting coupled radio transmitters and tri-axial accelerometers in rattlesnakes, we achieved two important goals: (1) we validated broad and easily transferrable procedures for the long-term accelerometer recording of snakes in the field, and (2) we developed an automated system for accurate classification of coarse behavioral states (movement, immobile) in extensive field datasets. With these field data we constructed long-term activity budgets (Figure 3) and evaluated patterns in the timing and duration of movement at multiple temporal scales. The high classification accuracy achieved for both the GLMNET (97.0%) and RF models (99.0%), despite the very low monitoring frequency (1-Hz), illustrates the efficacy of our framework (Figure 1). The periodic field-validation observations of behavior proved to be critical to the success of these procedures by providing time-matched behavioral data for model training and testing, thereby making our models robust to inter-individual variability in ACT signals (Nathan et al., 2012; Bom et al., 2014; Hammond et al., 2016; Sur et al., 2017). This latter detail was particularly important for this study because RT-ACTs were internally implanted, which prevented visual confirmation of consistent logger positioning during field deployments. During extraction procedures, we did not document any instances of significant logger repositioning within the body cavities of rattlesnakes, indicating that suturing RT-ACTs to a rib had successfully affixed implants over the entire recording durations. Nevertheless, field validation observations are an important additional safeguard against subtle variation in ACT orientation, which can have significant effects on ACT signals within and between individuals and is a potential source of error in relating signals to behavior [(Brown et al., 2013); C. Catoni (Technosmart Europe srl.), personal communication].
Although both algorithms performed well within our classification scheme, the RF model slightly outperformed the GLMNET (Tables 2, 3). This was somewhat surprising given the GLMNET was selected as an ideal approach for this study because of its proficiency in binary classification. Both models were more accurate at classifying the “immobile” behavioral state in rattlesnakes (Table 2), likely as a result of the relative scarcity of “movement” observations in the training dataset (1.2 of 32 h) and the substantially greater variation in ACT signals seen during periods of movement. While RFs remain computationally intensive to train (Cutler et al., 2007), they have been used to successfully classify behavior from ACT signals in a wide variety of terrestrial and aquatic taxa (reviewed in Shuert et al., 2018). However, the vast majority of such studies, similar to ours, found it difficult to achieve high accuracy with a large number of behavioral states and often opted for classifying fewer categories (i.e., two to four; Shuert et al., 2018). For this project, we were restricted to classifying two coarse behaviors (movement and immobility) largely because of limited observations of other cryptic behaviors during field observations. Within this scheme, the most important variables (summary statistics) for classification were consistently identified as the standard deviation of DBA along the x, y, and z axes (Figure 2). Further, the x and y axes were generally more informative than the z for activity classification, likely because of the general lack of upward and downward (z) movement involved in rattlesnake locomotion (particularly in the posterior third of the body where ACTs were implanted). Some infrequently observed behaviors, such as ritualized male combat or predatory behaviors, might involve movements that would be best distinguished using signals on the z axis. More anterior placement of ACTs could also enable classification of several additional behaviors, but this would either involve external attachment, which is impractical because of periodic ecdysis and a high potential for behavioral obstruction, or subcutaneous implantation (requiring a very small, low-profile battery). The ODBA and VeDBA measures were not as informative as standard measures of data dispersion (s.d., range, etc.) for distinguishing between movement and immobile (Figure 2). These two metrics are widely used in ACT studies as a proxy for energy expenditure in free-ranging animals (most often in terrestrial quadrupeds; Wilmers et al., 2015) and have been shown to correlate well with the overall “intensity” of different movement behaviors (Tatler et al., 2018). In our case, both measures could prove to be more informative for a classification scheme involving additional behavior states that vary in intensity.
Long-term activity budgets allowed for detailed visualization (Figure 3) and examination of the timing and duration of movement. In general, C. atrox movement was found to be highly punctuated and infrequent, characterized by extensive bouts of immobility between brief periods of movement (Figure 3). Considerable inter-individual variation was also observed (Figure 3), which is characteristic of most high-resolution bio-logging data. Overall (entire logging period) proportions of time spent moving per-individual range from 1.6 to 37% (Table 4). As ectothermic, ambush foraging, low-energy specialists, pitvipers are known to spend extensive periods of time waiting motionless for potential prey to pass within striking distance (Clark, 2016). Numerous radio telemetry studies on rattlesnakes corroborate these general findings (Reinert and Cundall, 1982; Reinert, 1992; Glaudas and Rodríguez-Robles, 2011), as pitvipers can often exhibit impressive displays of immobility but can also make substantial long-range movements within large home ranges (in isolated cases we observed movements by C. atrox that exceeded 1 km in under 24 h). Evaluation of concurrent spatial data from RT-ACT C. atrox showed positive relationships between movement rate (meters-per-day, MPD) and movement frequency (MMF) and the proportion of time spent moving over entire ACT logging durations for individual rattlesnakes (MPD: adjusted R2 = 0.56, P < 0.01; MMF: adjusted R2 = 0.63, P < 0.01; Table 5). Conversely, there was no significant relationship between distance-per-movement and movement (adjusted R2 = −0.05, P = 0.49). Although we are cautious of drawing conclusions from these results considering the limited sample size, the general correspondence between spatial metrics and ACT-derived movement further validates the classifications provided by the RF model. Additionally, by simultaneously informing on the temporal and spatial aspects of movement behavior, there is potential for these distinct data types to be complementary, much like animal-attached GPS data-loggers and ACTs in other bio-logging studies (Wilson et al., 2008; Berlincourt et al., 2015; Walker et al., 2015). Combining spatial data with high-resolution activity data has provided important insights with larger study organisms, such as identifying different behavioral states along movement paths by foraging animals (Nams, 2014). RT-ACTs could allow detection of temporal or spatial shifts in behavior by small, secretive species that would be overlooked without an integrated approach.
Table 5. Concurrent spatial metrics and accelerometer-derived proportions of time spent moving (Movement) for the entire ACT-logging period.
As with other desert-dwelling ectotherms, surface activity in C. atrox is largely dictated by ambient temperature. At our study site in the arid northern Chihuahuan desert, we expected daily and seasonal activity patterns to largely reflect this constraint, with the majority of movement occurring during nocturnal periods in the warmer summer months (Jun–Sep) and a more cathemeral (i.e., morning, evening) activity pattern during the comparatively cooler spring (Apr–May) and fall (Oct–Nov) months. We also expected greatly reduced movement by rattlesnakes during the winter inactive season (Dec–Mar). Counter to our prediction, rattlesnakes displayed the same general daily activity pattern across all active seasons [spring, summer (NM, M), fall] with the majority of movement always occurring during the evening or nocturnal diel periods (Table 4). Conversely, the mean proportion of time spent moving within diel periods did vary seasonally, as movement duration increases during the summer-mating season (Figure 4). The mating season for C. atrox on IMRS is unimodal, with a single annual period from August through October where all reproductive behavior is observed (DeSantis et al., 2019). Unsurprisingly, activity is greatly reduced during all diel periods in winter. The apparent increase in movement by male C. atrox during the summer-mating season corresponds with numerous observations of male pitvipers elevating movement during mating seasons in mate-searching efforts (Duvall et al., 1993; Madsen et al., 1993; Duvall and Schuett, 1997; Glaudas and Rodríguez-Robles, 2011; Clark et al., 2014), including male C. atrox monitored on IMRS (DeSantis et al., 2019). In the context of these analyses, it is important to again acknowledge the relatively small sample of rattlesnakes obtained for this study (N =12). Additionally, note that the margins between movement durations discussed here are relatively thin (i.e., mean proportion of time spent moving within diel periods ranges from <0.01 to 6.4%). The highest recorded mean activity duration was within the evening diel period during the summer-mating season, where, on average, individuals were moving for 11.52 out of 180 min. For these reasons, we are cautious of drawing conclusions from these results beyond using them as further validation of our procedures.
Validation studies, such as this, are prerequisite to using novel techniques to improve upon hypothesis testing in field studies with secretive or difficult-to-monitor focal taxa. This pilot study involved validation of internal implantation and field deployment procedures for ACTs with an entirely new focal group (snakes) for field bio-logging studies. To the best of our knowledge, protocols for internal implantation of ACTs for long-term field monitoring have only been developed for free-living teleost fish (Wright et al., 2014). Implantation was essential for our study to enable long-term field deployment and to minimize the effects of the device on individual behavior. The ability to securely suture ACTs to a rib (in the same position and orientation across individuals) also further minimized inter-individual variation in ACT signals. The well-established protocols for the internal implantation of similarly sized radio transmitters in snakes combined with the relatively small size of our coupled RT-ACT implants (see image in Figure 1) makes this technique widely applicable for a diversity of similarly sized snakes and other small species amenable to implantation procedures. In fact, the primary contributor to the overall size of RT-ACTs was the ACT battery, and ongoing improvements to miniaturized battery technology should continue to diminish this constraint.
While our results demonstrate that snakes, and pitvipers (Viperidae: Crotalinae), specifically, are intriguing model organisms for ACT applications, they also carry unique challenges. This study made use of a relatively simple classification scheme targeting only two coarse behavioral modes (movement, immobile). Unsurprisingly, one of the primary motivations for developing ACT monitoring protocols for snakes (i.e., secretive life histories) proved to be a significant hurdle for validation, given that instances where snakes were observed actively moving on the surface were infrequent (1.2 of 32 h), and even then, it was difficult to conduct extensive observations without disturbing the individual (often because of the use of flashlights during nocturnal observations). Fortunately, the long-term deployment of data-loggers yielded sufficient opportunities, but model training likely would have been less effective with short-duration deployments seen in many other ACT field studies of animal behavior that select higher monitoring frequencies.
The highly secretive life histories of C. atrox ultimately precluded the inclusion of additional cryptic behaviors that were infrequently or never observed in the field (i.e., feeding, mating, and male-male combat). Future studies with independent captive-validation observations would likely be required to achieve finer-scale behavioral segmentation. Some of these fine-scale behaviors could require a higher ACT monitoring frequency for accurate classification, which amplifies the trade-offs between recording frequency, battery capacity, and field deployment duration. The unique biomechanics of pitvipers also largely lack the frequent and repetitive movements that aid in the segmentation of many behaviors for animals with limbs, wings, or aquatic habits, and the optimal site of internal implantation is in the posterior third of the body where anterior movements are less detectable (such as striking, male-male combat, and chemosensory probing; Barbour and Clark, 2012). Any additional behaviors added to a classification scheme must be consistently distinct from other modes, and this is challenging for animals in which similar body movements are seen across behaviors (such as snakes). All of these factors combine to complicate classifying multiple discrete behaviors in C. atrox and other pitvipers. However, as demonstrated herein, high model accuracy and precision are attainable given extensive “ground-truthing” data for model training, and recent work has also shown that fine-scale behavioral segmentation is achievable at low monitoring frequencies (i.e., 1-Hz; Tatler et al., 2018). Further, increasingly complex classification algorithms are available for segmenting increasingly subtle animal behavior states (Ladds et al., 2017; Wilson et al., 2018a). It remains to be seen if a combination of independent captive observation experiments, extensive field-validation observations, and advanced machine learning techniques can allow fine-scale behavioral segmentation under long-term, low-frequency monitoring in snakes. Even using the coarse-scale classification scheme validated herein, there are exciting future applications well suited for the RT-ACT framework. For example, exploring relationships between activity budgets and foraging mode (active vs. ambush foraging) across snake taxa could contribute to efforts to provide a quantitative method for assigning foraging mode to snakes, as has been accomplished with lizards (Glaudas et al., 2019). Additionally, ACT-derived movement might also improve evaluation of temporal scale-dependent variation in the factors influencing movement decisions (DeSantis et al., unpublished data).
Moving forward, larger samples with improved data overlap will be necessary to realize the full potential of this technology in hypothesis-driven field studies with pitvipers and other focal species. Nevertheless, our validated framework illustrates the ability to remotely collect data on the timing and duration of movement at resolutions, scales, and precisions not previously possible with snakes. These data, especially if paired with additional animal-borne data-logging technologies (Allan et al., 2018; Wilson et al., 2018b), can dramatically improve the ability to evaluate drivers of behavioral decisions in small-bodied and secretive terrestrial species. This project can also be added to a growing list of studies that demonstrate the importance of time-matched behavioral observations and cross-validation model training procedures in ACT applications (Bom et al., 2014; Sur et al., 2017; Tatler et al., 2018).
The raw data supporting the conclusions of this article will be made available by the authors, without undue reservation.
The animal study was reviewed and approved by The University of Texas at El Paso Animal Care and Use Committee (Protocol A-201405-1) and Texas Parks and Wildlife (Scientific Permit Number: SPR-0290-019). Written informed consent was obtained from the individual(s) for the publication of any potentially identifiable images or data included in this article.
DD conceived the project, oversaw and conducted data collection, and wrote the manuscript. DD and VM-S designed the data collection methodology. AW and DD analyzed the data. AW, JJ, and VM-S provided editorial advice. JJ provided access to the study site and logistical support.
This project was funded by a National Science Foundation Graduate Research Fellowship (NSF-GRFP) awarded to DD and grants from the UTEP Graduate School (Dodson Research Grant) awarded to DD. This work was also supported by Grant 5U54MD007592 from the National Institute on Minority Health and Health Disparities (NIMHD), a component of the National Institutes of Health (NIH).
The authors declare that the research was conducted in the absence of any commercial or financial relationships that could be construed as a potential conflict of interest.
We gratefully acknowledge J. D. Emerson, M. J. Gaupp, S. Ebert, H. Smith, R. Gamez, Z. Ramirez, and D. Sanchez for assistance in field data collection. We thank C. Catoni and Technosmart Europe srl. for logistical advice and development of accelerometers.
Allan, B. M., Nimmo, D. G., Ierodiaconou, D., VanDerWal, J., Koh, L. P., Ritchie, E. G., et al. (2018). Future casting ecological research: the rise of technoecology. Ecosphere 9:e02163. doi: 10.1002/ecs2.2163
Banerjee, D., Daigle, C., Biswas, S., and Siegford, J. (2012). “Remote activity classification of hens using wireless body mounted sensors,” in Ninth International Conference on Wearable and Implantable Body Sensor Networks, 107–112. doi: 10.1109/BSN.2012.5
Barbour, M. A., and Clark, R. W. (2012). Diel cycles in chemosensory behaviors of free-ranging rattlesnakes lying in wait for prey. Ethology 118, 480–488. doi: 10.1111/j.1439-0310.2012.02035.x
Bauder, J. M., Breininger, D. R., Rebecca Bolt, M., Legare, M. L., Jenkins, C. L., Rothermel, B. B., et al. (2016). Seasonal variation in Eastern Indigo Snake (Drymarchon couperi) movement patterns and space use in peninsular Florida at multiple temporal scales. Herpetologica 72, 214–226. doi: 10.1655/Herpetologica-D-15-00039.1
Beaupre, S. J. (2016). “Novel tracking and remote monitoring technologies: Applications to studying wild rattlesnakes,” in Rattlesnakes of Arizona, Vol. 2, eds G. W. Schuett, M. J. Feldner, C. F. Smith, and R. S. Reiserer (New Mexico: Eco Publishing, Rodeo), 58–91.
Berlincourt, M., Angel, L. P., and Arnould, J. P. (2015). Combined use of GPS and accelerometry reveals fine scale three-dimensional foraging behavior in the Short-Tailed Shearwater. PLoS ONE 10:e0139351. doi: 10.1371/journal.pone.0139351
Bidder, O. R., Qasem, L. A., and Wilson, R. P. (2012). On higher ground: how well can dynamic body acceleration determine speed in variable terrain? PLoS ONE 7:e50556. doi: 10.1371/journal.pone.0050556
Bom, R. A., Bouten, W., Piersma, T., Oosterbeek, K., and van Gils, J. A. (2014). Optimizing acceleration-based ethograms: the use of variable-time versus fixed-time segmentation. Mov. Ecol. 2:6. doi: 10.1186/2051-3933-2-6
Breiman, L. (2002). Manual on Setting Up, Using, and Understanding Random Forests v3. 1. Berkeley, CA: Statistics Department University of California Berkeley.
Brown, D. D., Kays, R., Wikelski, M., Wilson, R. P., and Klimley, A. P. (2013). Observing the unwatchable through acceleration logging of animal behavior. Anim. Biotelem. 1, 1–20. doi: 10.1186/2050-3385-1-20
Campbell, H. A., Gao, L., Bidder, O. R., Hunter, J., and Franklin, C. E. (2013). Creating a behavioural classification module for acceleration data: using a captive surrogate for difficult to observe species. J. Experim. Biol. 216, 4501–4506. doi: 10.1242/jeb.089805
Chimienti, M., Cornulier, T., Owen, E., Bolton, M., Davies, I. M., Travis, J. M. J., et al. (2016). The use of an unsupervised learning approach for characterizing latent behaviors in accelerometer data. Ecol. Evolut. 6, 727–741. doi: 10.1002/ece3.1914
Chmura, H., Glass, T., and Williams, C. (2018). Bio-logging physiological and ecological responses to climatic variation: new tools for the climate change era. Front. Ecol. Evol. 6:92. doi: 10.3389/fevo.2018.00092
Clark, R. W. (2016). “The hunting and feeding behavior of wild rattlesnakes,” in Rattlesnakes of Arizona, Vol. 2, eds. Schuett, G. W., Feldner, M. J., Smith, C. F., and Reiserer, R. S. (Eco Publishing, Rodeo, NM), 58–91.
Clark, R. W., Schuett, G. W., Repp, R. A., Amarello, M., Smith, C. F., and Herrmann, H.-W. (2014). Mating systems, reproductive success, and sexual selection in secretive species: a case study of the western diamond-backed rattlesnake, Crotalus atrox. PLoS ONE 9:e90616. doi: 10.1371/journal.pone.0090616
Collins, P. M., Green, J. A., Warwick-Evans, V., Dodd, S., Shaw, P. J. A., Arnould, J. P. Y., et al. (2015). Interpreting behaviors from accelerometry: a method combining simplicity and objectivity. Ecol. Evol. 5, 4642–4654. doi: 10.1002/ece3.1660
Cutler, D. R., Edwards, T. C., Beard, K. H., Cutler, A., Hess, K. T., Gibson, J., et al. (2007). Random forests for classification in ecology. Ecology 88, 2783–2792. doi: 10.1890/07-0539.1
Davis, J. R., Taylor, E. N., and DeNardo, D. F. (2008). An automated temperature-based option for estimating surface activity and refuge use patterns in free-ranging animals. J. Arid Environ. 72, 1414–1422. doi: 10.1016/j.jaridenv.2008.02.018
DeSantis, D. L., Mata-Silva, V., Wagler, A. E., and Johnson, J. D. (2019). Effects of human-made resource hotspots on seasonal spatial strategies by a desert pitviper. Sci. Rep. 9, 1–13. doi: 10.1038/s41598-019-52957-1
Diosdado, J. A. V., Vázquez Diosdado, J. A., Barker, Z. E., Hodges, H. R., Amory, J. R., Croft, D. P., et al. (2015). Classification of behaviour in housed dairy cows using an accelerometer-based activity monitoring system. Anim. Biotelem. 3:15. doi: 10.1186/s40317-015-0045-8
Dorcas, M. E., and Willson, J. D. (2009). “Innovative methods for studies of snake ecology and conservation,” in Snakes: Ecology and Conservation, eds S. J. Mullins and R. A. Seigel (Ithaca, NY: Cornell University Press), 5–37.
Durso, A. M., Willson, J. D., and Winne, C. T. (2011). Needles in haystacks: estimating detection probability and occupancy of rare and cryptic snakes. Biol. Conserv. 144, 1508–1515. doi: 10.1016/j.biocon.2011.01.020
Duvall, D., and Schuett, G. W. (1997). Straight-line movement and competitive mate searching in Prairie Rattlesnakes, Crotalus viridis viridis. Anim. Behav. 54, 329–334. doi: 10.1006/anbe.1996.0418
Duvall, D., Schuett, G. W., and Arnold, S. J. (1993). “Ecology and evolution of snake mating systems,” in Snakes: Ecology and Behavior, eds R. A. Seigel and J. T. Collins (New York: McGraw-Hill), 165–200.
Efron, B., and Hastie, T. (2016). Computer Age Statistical Inference, Vol. 5. Cambridge: Cambridge University Press.
Friedman, J., Hastie, T., and Tibshirani, R. (2010). Regularization paths for generalized linear models via coordinate descent. J. Statist. Softw. 33, 1–22. doi: 10.18637/jss.v033.i01
Glaudas, X., Glennon, K. L., Martins, M., Luiselli, L., Fearn, S., Trembath, D. F., et al. (2019). Foraging mode, relative prey size and diet breadth: A phylogenetically explicit analysis of snake feeding ecology. J. Anim. Ecol. 88, 757–767. doi: 10.1111/1365-2656.12972
Glaudas, X., and Rodríguez-Robles, J. A. (2011). Vagabond males and sedentary females: Spatial ecology and mating system of the Speckled Rattlesnake (Crotalus mitchellii). Biol. J. Linn. Soc. 103, 681–695. doi: 10.1111/j.1095-8312.2011.01677.x
Gleiss, A. C., Wilson, R. P., and Shepard, E. L. (2011). Making overall dynamic body acceleration work: on the theory of acceleration as a proxy for energy expenditure. Methods Ecol. Evol. 2, 23–33. doi: 10.1111/j.2041-210X.2010.00057.x
Graf, P. M., Wilson, R. P., Qasem, L., Hackländer, K., Rosell, F., Petit, O., . (eds.). (2015). The use of acceleration to code for animal behaviours; A case study in free-ranging Eurasian Beavers (Castor fiber). PLoS ONE 10:e0136751. doi: 10.1371/journal.pone.0136751
Grünewälder, S. F., Grünewälder, S., Broekhuis, F., Macdonald, D. W., Wilson, A. M., et al. (2012). Movement activity based classification of animal behaviour with an application to data from cheetah (Acinonyx jubatus). PLoS ONE 7:e49120. doi: 10.1371/journal.pone.0049120
Halsey, L. G., and White, C. R. (2010). Measuring energetics and behaviour using accelerometry in Cane Toads (Bufo marinus). PLoS ONE 5:e10170. doi: 10.1371/journal.pone.0010170
Hammond, T. T., Springthorpe, D., Walsh, R. E., and Berg-Kirkpatrick, T. (2016). Using accelerometers to remotely and automatically characterize behavior in small animals. J. Experim. Biol. 219, 1618–1624. doi: 10.1242/jeb.136135
Hardy, D. L. Sr., and Greene, H. W. (1999). Surgery on rattlesnakes in the field for implantation of transmitters. Son. Herpetol. 12, 25–27.
Hardy, D. L. Sr., and Greene, H. W. (2000). Inhalation anesthesia of rattlesnakes in the field for processing and transmitter implantation. Son. Herpetol. 13, 109–113.
Hokkanen, A. H., Hänninen, L., Tiusanen, J., and Pastell, M. (2011). Predicting sleep and lying time of calves with a support vector machine classifier using accelerometer data. Appl. Anim. Behav. Sci. 134, 10–15. doi: 10.1016/j.applanim.2011.06.016
Holyoak, M., Holyoak, M., Casagrandi, R., Nathan, R., Revilla, E., Spiegel, O. S., et al. (2008). Trends and missing parts in the study of movement ecology. Proc. Nat. Acad. Sci. U. S. A. 105, 19060–19065. doi: 10.1073/pnas.0800483105
Jeantet, L., Dell'Amico, F., Forin-Wiart, M.-A., Coutant, M., Bonola, M., Etienne, D., et al. (2018). Combined use of two supervised learning algorithms to model sea turtle behaviours from tri-axial acceleration data. J. Experim. Biol. 221:177378. doi: 10.1242/jeb.177378
Jeltsch, F. D., Bonte, D., Pe'er, G., Reineking, B., Leimgruber, P., et al. (2013). Integrating movement ecology with biodiversity research-exploring new avenues to address spatiotemporal biodiversity dynamics. Movem. Ecol. 1:6. doi: 10.1186/2051-3933-1-6
Kays, R., Crofoot, M. C., Jetz, W., and Wikelski, M. (2015). Terrestrial animal tracking as an eye on life and planet. Science 348:6240. doi: 10.1126/science.aaa2478
Ladds, M. A., Ladds, M. A., Thompson, A. P., Kadar, J. P., Slip, D. J., Hocking, D. P., et al. (2017). Super machine learning: improving accuracy and reducing variance of behaviour classification from accelerometry. Anim. Biotel. 5:8. doi: 10.1186/s40317-017-0123-1
Ladds, M. A., Thompson, A. P., Slip, D. J., Hocking, D. P., and Harcourt, R. G. (2016). Seeing it all: evaluating supervised machine learning methods for the classification of diverse otariid behaviours. PLoS ONE 11:e0166898. doi: 10.1371/journal.pone.0166898
Lagarde, F., Guillon, N., Dubroca, L., Bonnet, X., Kaddour, K. B., Slimani, T., et al. (2008). Slowness and acceleration: A new method to quantify the activity budget of chelonians. Anim. Behav. 75, 319–329. doi: 10.1016/j.anbehav.2007.01.010
Lindström, T., Phillips, B. L., Brown, G. P., and Shine, R. (2015). Identifying the time scale of synchronous movement: a study on tropical snakes. Mov. Ecol. 3:12. doi: 10.1186/s40462-015-0038-5
Lush, L., Ellwood, S., Markham, A., Ward, A. I., and Wheeler, P. (2016). Use of tri-axial accelerometers to assess terrestrial mammal behaviour in the wild. J. Zool. 298, 257–265. doi: 10.1111/jzo.12308
Madsen, T., Shine, R., Loman, J., and Håkansson, T. (1993). Determinants of mating success in male adders, Vipera berus. Anim. Behav. 45, 491–499. doi: 10.1006/anbe.1993.1060
Mata-Silva, V., DeSantis, D. L., Wagler, A. E., and Johnson, J. D. (2018). Spatial ecology of Rock Rattlesnakes (Crotalus lepidus) in far west Texas. Herpetologica 74, 245–254. doi: 10.1655/Herpetologica-D-16-00030.1
Murchie, K. J., Cooke, S. J., Danylchuk, A. J., and Suski, C. D. (2011). Estimates of field activity and metabolic rates of bonefish (Albula vulpes) in coastal marine habitats using acoustic tri-axial accelerometer transmitters and intermittent-flow respirometry. J. Exp. Mar. Biol. Ecol. 396, 147–155. doi: 10.1016/j.jembe.2010.10.019
Nams, V. O. (2014). Combining animal movements and behavioural data to detect behavioural states. Ecol. Lett. 17, 1228–1237. doi: 10.1111/ele.12328
Nathan, R., Getz, W. M., Revilla, E., Holyoak, M., Kadmon, R., Saltz, D., et al. (2008). A movement ecology paradigm for unifying organismal movement research. Proc. Nat. Acad. Sci. U. S. A. 105, 19052–19059. doi: 10.1073/pnas.0800375105
Nathan, R., Spiegel, O., Fortmann-Roe, S., Harel, R., Wikelski, M., Getz, W. M., et al. (2012). Using tri-axial acceleration data to identify behavioral modes of free-ranging animals: general concepts and tools illustrated for griffon vultures. J. Exp. Biol. 215, 986–996. doi: 10.1242/jeb.058602
Nishizawa, H., Noda, T., Yasuda, T., Okuyama, J., Arai, N., and Kobayashi, M. (2013). Decision tree classification of behaviors in the nesting process of green turtles (Chelonia mydas) from tri-axial acceleration data. J. Ethol. 31, 315–322. doi: 10.1007/s10164-013-0381-1
Pagano, A. M., Rode, K. D., Cutting, A., Owen, M. A., Jensen, S., Ware, J. V., et al. (2017). Using tri-axial accelerometers to identify wild polar bear behaviors. Endang. Spec. Res. 32, 19–33. doi: 10.3354/esr00779
Patterson, A., Gilchrist, H. G., Chivers, L., Hatch, S., and Elliott, K. (2019). A comparison of techniques for classifying behavior from accelerometers for two species of seabird. Ecol. Evol. 9, 3030–3045. doi: 10.1002/ece3.4740
R Core Team. (2020). R: A Language and Environment for Statistical Computing. Vienna: R Foundation for Statistical Computing. Available online at: https://www.R-project.org/ (accessed March 6, 2020).
Reinert, H. E. (1992). “Radiotelemetric field studies of pitvipers: data acquisition and analysis,” in The Biology of the Pitvipers, eds J. A. Campbell and E. D. Brodie (Tyler, TX: Selva Publishing), 185–197.
Reinert, H. E., and Cundall, D. (1982). An improved surgical implantation method for radio-tracking snakes. Copeia 1982, 702–705. doi: 10.2307/1444674
Ropert-Coudert, Y., and Wilson, R. P. (2005). Trends and perspectives in animal-attached remote sensing. Front. Ecol. Environm. 3, 437–444. doi: 10.1890/1540-9295(2005)003[0437:TAPIAR]2.0.CO;2
Rutz, C., and Hays, G. C. (2009). New frontiers in bio-logging science. Biol. Lett. 5, 289–292. doi: 10.1098/rsbl.2009.0089
Sato, K., Mitani, Y., Cameron, M. F., Siniff, D. B., and Naito, Y. (2003). Factors affecting stroking patterns and body angle in diving Weddell Seals under natural conditions. J. Experim. Biol. 206, 1461–1470. doi: 10.1242/jeb.00265
Shepard, E. L. C., Wilson, R. P., Quintana, F., Gómez Laich, A., Liebsch, N., Albareda, D. A., et al. (2008). Identification of animal movement patterns using triaxial accelerometry. Endang. Spec. Res. 10, 47–60. doi: 10.3354/esr00084
Shuert, C. R., Pomeroy, P. P., and Twiss, S. D. (2018). Assessing the utility and limitations of accelerometers and machine learning approaches in classifying behaviour during lactation in a phocid seal. Anim. Biotelem. 6:14. doi: 10.1186/s40317-018-0158-y
Sperry, J. H., Ward, M. P., and Weatherhead, P. J. (2013). Effects of temperature, moon phase, and prey on nocturnal activity in ratsnakes: an automated telemetry study. J. Herpetol. 47, 105–111. doi: 10.1670/11-325
Sur, M., Suffredini, T., Wessells, S. M., Bloom, P. H., Lanzone, M., Blackshire, S., et al. (2017). Improved supervised classification of accelerometry data to distinguish behaviors of soaring birds. PLoS ONE 12:e0174785. doi: 10.1371/journal.pone.0174785
Tatler, J., Cassey, P., and Prowse, T. A. (2018). High accuracy at low frequency: detailed behavioural classification from accelerometer data. J. Experim. Biol. 23:jeb184085. doi: 10.1242/jeb.184085
Trierweiler, C., Mullié, W. C., Drent, R. H., Exo, K.-M., Komdeur, J., Bairlein, F., et al. (2013). A Palaearctic migratory raptor species tracks shifting prey availability within its wintering range in the Sahel. J. Anim. Ecol. 82, 107–120. doi: 10.1111/j.1365-2656.2012.02036.x
Walker, J. S., Jones, M. W., Laramee, R. S., Holton, M. D., Shepard, E. L. C., Williams, H. J., et al. (2015). Prying into the intimate secrets of animal lives; software beyond hardware for comprehensive annotation in ‘Daily Diary’ tags. Movem. Ecol. 3:29. doi: 10.1186/s40462-015-0056-3
Wang, Y., Nickel, B., Rutishauser, M., Bryce, C. M., Williams, T. M., Elkaim, G., et al. (2015). Movement, resting, and attack behaviors of wild pumas are revealed by tri-axial accelerometer measurements. Mov. Ecol. 3:2. doi: 10.1186/s40462-015-0030-0
Ward, M. P., Sperry, J. H., and Weatherhead, P. J. (2013). Evaluation of automated radio telemetry for quantifying movements and home ranges of snakes. J. Herpetol. 47:337. doi: 10.1670/12-018
Wassmer, T., Jensen, H., Fahlman, A., and Murray, D. (2020). Editorial: ecology and behavior of free-ranging animals studied by advanced data-logging and tracking techniques. Front. Ecol. Evol. Provision 8:113. doi: 10.3389/fevo.2020.00113
Whitaker, P. B., and Shine, R. (2003). A radiotelemetric study of movements and shelter-site selection by free-ranging brownsnakes (Pseudonaja textilis, Elapidae). Herpetol. Monograp. 17, 130–144. doi: 10.1655/0733-1347(2003)017[0130:ARSOMA]2.0.CO;2
Williams, T. M., Wolfe, L., Davis, T., Kendall, T., Richter, B., Wang, Y., et al. (2014). Mammalian energetics. Instantaneous energetics of puma kills reveal advantage of felid sneak attacks. Science 346, 81–85. doi: 10.1126/science.1254885
Willson, J. D., and Winne, C. T. (2015). Evaluating the functional importance of secretive species: a case study of aquatic snake predators in isolated wetlands. J. Zool. 298, 266–273. doi: 10.1111/jzo.12311
Wilmers, C. C., Nickel, B., Bryce, C. M., Smith, J. A., Wheat, R. E., Yovovich, V., et al. (2015). The golden age of bio-logging: how animal-borne sensors are advancing the frontiers of ecology. Ecology 96, 1741–1753. doi: 10.1890/14-1401.1
Wilson, R. P., Holton, M. D., di Virgilio, A., Williams, H., Shepard, E. L. C., Lambertucci, S., et al. (2018a). Give the machine a hand: a Boolean time-based decision-tree template for rapidly finding animal behaviours in multisensor data. Methods Ecol. Evol. 9, 2206–2215. doi: 10.1111/2041-210X.13069
Wilson, R. P., Neate, A., Holton, M. D., Shepard, E. L. C., MichaelScantlebury, D., Lambertucci, S. A., et al. (2018b). Luck in food finding affects individual performance and population trajectories. Curr. Biol. 28, 3871–3877. doi: 10.1016/j.cub.2018.10.034
Wilson, R. P., Shepard, E. L. C., and Liebsch, N. (2008). Prying into the intimate details of animal lives: use of a daily diary on animals. Endang. Spec. Res. 4, 123–137. doi: 10.3354/esr00064
Wilson, R. P., White, C. R., Quintana, F., Halsey, L. G., Liebsch, N., Martin, G. R., et al. (2006). Moving towards acceleration for estimates of activity-specific metabolic rate in free-living animals: the case of the cormorant. J. Anim. Ecol. 75, 1081–1090. doi: 10.1111/j.1365-2656.2006.01127.x
Worthington, R. D., Johnson, J. D., Lieb, C. S., and Anderson, W. (2020). Biotic Resources of Indio Mountains Research Station (IMRS) Southeastern Hudspeth County, Texas: A Handbook for Students and Researchers. El Paso, TX: The University of Texas at El Paso, USA, 1, 90.
Wright, S., Metcalfe, J. D., Hetherington, S., and Wilson, R. (2014). Estimating activity-specific energy expenditure in a teleost fish, using accelerometer loggers. Marine Ecology Progress Series 496, 19–32. doi: 10.3354/meps10528
Yoda, K., and Ropert-Coudert, Y. (2007). Temporal changes in activity budgets of chick-rearing Adélie penguins. Mar. Biol. 151, 1951–1957. doi: 10.1007/s00227-007-0631-2
Keywords: accelerometer, activity patterns, movement behavior, machine learning, radio telemetry, snakes
Citation: DeSantis DL, Mata-Silva V, Johnson JD and Wagler AE (2020) Integrative Framework for Long-Term Activity Monitoring of Small and Secretive Animals: Validation With a Cryptic Pitviper. Front. Ecol. Evol. 8:169. doi: 10.3389/fevo.2020.00169
Received: 09 April 2020; Accepted: 13 May 2020;
Published: 10 June 2020.
Edited by:
Piotr Jablonski, Seoul National University, South KoreaReviewed by:
Gordon Wayne Schuett, Georgia State University, United StatesCopyright © 2020 DeSantis, Mata-Silva, Johnson and Wagler. This is an open-access article distributed under the terms of the Creative Commons Attribution License (CC BY). The use, distribution or reproduction in other forums is permitted, provided the original author(s) and the copyright owner(s) are credited and that the original publication in this journal is cited, in accordance with accepted academic practice. No use, distribution or reproduction is permitted which does not comply with these terms.
*Correspondence: Dominic L. DeSantis, ZG9taW5pYy5kZXNhbnRpc0BnY3N1LmVkdQ==
†Present address: Dominic L. DeSantis, Department of Biological and Environmental Sciences, Georgia College and State University, Milledgeville, GA, United States
Disclaimer: All claims expressed in this article are solely those of the authors and do not necessarily represent those of their affiliated organizations, or those of the publisher, the editors and the reviewers. Any product that may be evaluated in this article or claim that may be made by its manufacturer is not guaranteed or endorsed by the publisher.
Research integrity at Frontiers
Learn more about the work of our research integrity team to safeguard the quality of each article we publish.