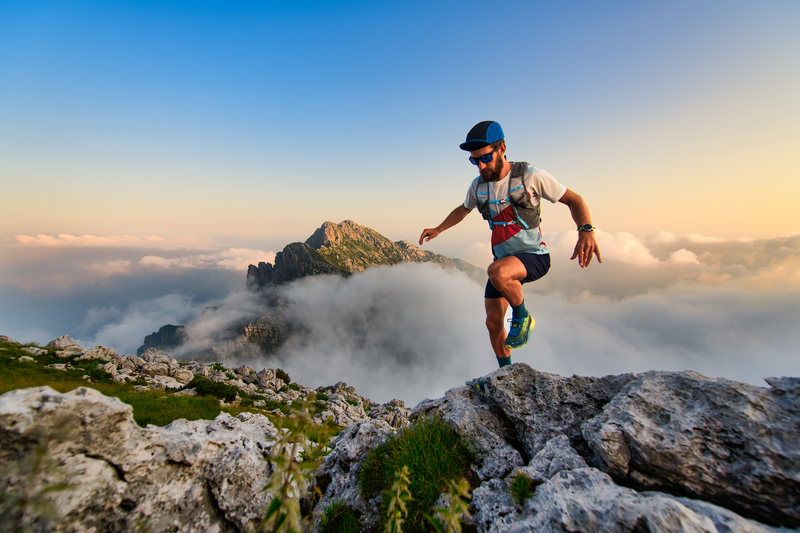
94% of researchers rate our articles as excellent or good
Learn more about the work of our research integrity team to safeguard the quality of each article we publish.
Find out more
ORIGINAL RESEARCH article
Front. Ecol. Evol. , 07 May 2020
Sec. Behavioral and Evolutionary Ecology
Volume 8 - 2020 | https://doi.org/10.3389/fevo.2020.00119
This article is part of the Research Topic Ecological, Behavioral and Genomic Consequences in the Rodent Family Sciuridae: Why Are Squirrels So Diverse? View all 14 articles
The color patterns of an animal’s pelage, feather, or skin serve a variety of adaptive functions; importantly, one function is concealment through background matching. In spatially and temporally heterogeneous environments, some species exhibit multiple distinct color patterns within a population (i.e., color polymorphism). The environmental drivers of color polymorphism are poorly understood. We used the polymorphic eastern fox squirrel (Sciurus niger ssp.; hereafter, fox squirrel) as a model species to investigate the role of environmental factors on pelage coloration. Building upon previous research that investigated the drivers of melanism, we measured fox squirrel pelage coloration across the visible light spectrum. Agouti-colored squirrels were positively associated with increased proportion of burned area in a fox squirrel dispersal buffer. Light-colored (less melanistic) squirrels were positively associated with increasing proportion of cropland in a fox squirrel dispersal buffer. We posit that agouti pelage is broadly adapted to a range of heterogeneous conditions created by fire. Conversely, croplands, once established, are relatively stable ecosystems which promote a consistently adaptive light-colored pelage morph. We suggest that in an increasingly human-dominated environment, spatially and temporally homogeneous processes, such as prescribed burning, may not sufficiently recreate environmental heterogeneity, which could result in lost pelage diversity.
One of the most distinct forms of animal diversity is the variety of color patterns animals exhibit. The color patterns of an animal’s pelage, feather, or skin serve a variety of adaptive functions such as concealment, communication, and thermoregulation (Caro, 2005; Ancillotto and Mori, 2017; Cuthill et al., 2017). Animal coloration patterns are an evolutionary response to selective forces that promote fitness-maximizing color patterns (Cloudsley-Thompson, 1999; Caro, 2005). In prey species, pelage coloration can reduce predation risk, often through concealment, thereby reducing an animal’s likelihood of being identified by a predator (Krupa and Geluso, 2000; Boratyñski et al., 2014). In heterogeneous or changing environments, a prey species with a consistent color pattern may be maladapted (Kettlewell, 1955; Mather, 1955). Accordingly, color polymorphism is often associated with spatial and temporal environmental heterogeneity that promotes multiple strong selective forces on coloration (Ford, 1945; Mather, 1955; Merilaita et al., 1999; Bond and Kamil, 2006; Gray and McKinnon, 2007). Understanding the specific environmental factors that influence pelage coloration provides insight into the adaptations of polymorphic species and the ecological factors that promote the persistence of polymorphism.
Eastern fox squirrels (Sciurus niger ssp.; hereafter, fox squirrel) found in the southeastern United States are a model species for polymorphism research in heterogeneous environments (i.e., fire-prone pine [Pinus spp.] forests). The fox squirrel is the most variably colored mammal in North America (Hall, 1981), such that individuals are identifiable using their unique pelage patterns (Tye et al., 2015). In the southeastern United States, fox squirrels typically have gray or agouti dorsal pelage, with varying amounts of melanism (Moore, 1956). Agouti pelage has multiple colors on individual hair follicles, and often appears as a mottled brown color. Fox squirrels show greater variation in ventral coloration, which can be cream, red, beige, or black (Moore, 1956). Broadly, fox squirrels in Florida can be classified into one of six color morphs: gray, buff/tan, agouti with partial dorsal melanism, black-bellied, dorsal melanism, and melanistic (Figure 1; Florida Fish and Wildlife Conservation Commission, 2013). Fox squirrels commonly exhibit countershading, a pelage pattern where an animal is dorsally dark and ventrally light (Steele and Koprowski, 2001). Countershading may conceal animals from above and below, especially in well-lit environments (Caro, 2005), though evidence for countershading as an adaptive coloration is mixed (Kiltie, 1988; Ruxton et al., 2004; Yahner, 2012). Countershading is thought to be a more effective antipredator adaptation in marine environments, where predators swim above and below prey (Kiltie, 1988). Similarly, arboreal animals, such as fox squirrels, face predation threats from above and below (Koprowski, 1994), and may therefore benefit from countershading.
Figure 1. Exemplars of the six fox squirrel color morphs found in Florida. From left to right, gray, agouti with partial dorsal melanism, buff/tan, black-bellied, dorsal melanism, melanistic.
Previous research on fox squirrel color polymorphism in the southeastern United States suggests that pale-agouti dorsal pelage, defined as agouti pelage with no non-agouti black pelage, is most cryptic when static against a background of bark from multiple species of tree (Kiltie, 1992b) and in unburned environments (Kiltie, 1992a). Dark and intermediate dorsal coloration is more cryptic against burned backgrounds, and moving squirrels with partial dark dorsal pelage may be more difficult for avian predators to identify (Kiltie, 1992b). Melanistic fox squirrels are most concealed against fire-blackened substrates, but trees, other vegetation, and bare soil only remain blackened after a fire for approximately 2 weeks (Kiltie, 1992b). Two weeks is likely too brief for concealment to be the adaptive benefit that allows melanistic fox squirrels to persist (Kiltie, 1992b).
Research on the ecological drivers of fox squirrel coloration show a positive correlation between dorsal melanism and historic lightning-caused wildfire frequency and temperature, and a negative correlation with elevation (Kiltie, 1989). Kiltie (1992b) suggested that dark coloration persists because dark squirrels are more difficult for aerial predators to identify, not because dark pelage is more cryptic in a fire-dominated environment. Historic fire patterns are only one possible ecological driver of squirrel polymorphism. Other ecological factors such as landcover (Kettlewell, 1955; Sumasgutner et al., 2018), precipitation/moisture (da Silva et al., 2016), and soil type (Krupa and Geluso, 2000) drive polymorphism in other species, and may therefore shape fox squirrel polymorphism. Furthermore, previous work on fox squirrels focused on the ecological drivers of dorsal melanism (Kiltie, 1989, 1992a), while explaining variation in fox squirrel pelage across the full color spectrum is likely to provide a more complete understanding of the evolutionary links between coloration and the environment. Color vision is important for hunting in some species of fox squirrel predators, including canids (Kasparson et al., 2013) and raptors (Håstad et al., 2005), suggesting that pelage darkness alone may not be enough to describe an animal’s cryptic pelage adaptation.
The aim of this study was to identify environmental variables that drive fox squirrel pelage polymorphism. We hypothesized that fox squirrel pelage variation would correlate with the background color of the environment as an antipredator adaptation. We predicted that squirrels with agouti-colored pelage would positively correlate with fire frequency and burned area (Kiltie, 1989). Dark-colored pelage in rodents, including sciurids, is often associated with low-light environments, including closed-canopy forests and increased tree density (Wauters et al., 2004; Ancillotto and Mori, 2017). Thus, we predicted that squirrels with more melanistic pelage would positively correlate with closed-canopy forests where decreased light penetration through the canopy makes dark pelage more cryptic (Wauters et al., 2004; Ancillotto and Mori, 2017). Lastly, we predicted that countershading would negatively correlate with canopy cover as countershading is an antipredator adaptation in well-lit environments (Caro, 2005).
Between 2012 and 2015, we collected roadkill fox squirrel specimens across Florida (Figure 2). To increase sample sizes and coverage throughout Florida, we enlisted the help of various wildlife agencies and citizen scientists to collect roadkill fox squirrel specimens. Although our sampling design was opportunistic, we managed to collect fox squirrels throughout most of their range within Florida. We recorded the location of each roadkill specimen using a handheld GPS. To prevent the pelage color from fading, we kept all specimens frozen until they were prepared as flat skins and dried. We stored all specimens in a dark cabinet at the Florida Museum of Natural History (FLMNH).
Figure 2. Maps showing the locations of 135 roadkill fox squirrels collected throughout Florida used to investigate the role of environmental drivers of polymorphism. The fill color of each circle corresponds with the color measured at each location on an individual squirrel. The size of the circle corresponds with the proportion of cropland (top) and burned area (bottom) within a fox squirrel dispersal buffer.
We used a digital color sensor (NixTM Pro Color Sensor, Nix Sensor Ltd., Hamilton, ON, Canada) to quantify squirrel pelage color composition (Hodgen, 2016; Stiglitz et al., 2016; Holman et al., 2018). In a separate study, we found that the sensor gives highly correlated measures of fox squirrel pelage compared to traditional photography methods (Potash et al., 2020). The sensor measures the average color within a 15 mm diameter round aperture. We described and recorded our color readings using the Commission Internationale de L’Eclairages (CIELab) color space which uses three axes, L∗, a∗, and b∗ to define colors (Schanda, 2007). The L∗ axis measures brightness and ranges from 0 to 100, with higher values indicating a lighter color (i.e., L∗ = 0 is black and L∗ = 100 is white). Values on the a∗ and b∗ axes can be positive or negative, where at a∗ = 0 and b∗ = 0 corresponds to the color gray. The a∗ axis describes a continuous gradient where negative values indicate more of the color green, while positive values indicate more of the color red. The b∗ axis describes a continuous gradient where negative values indicate more of the color blue, while positive values indicate more of the color yellow.
We measured L∗, a∗, and b∗ values at five locations on each squirrel specimen. These locations were between the ears (head), centered between the front legs (shoulder), at the base of the tail (rump), midway between the shoulder and rump (dorsum), and laterally perpendicular to the dorsum along the edge of the specimen (venter; Figure 3). To increase the accuracy of our measurements, we averaged 7 repeated measurements at each location, removing and replacing the sensor at each location between each recording (Holman et al., 2018).
Figure 3. An example of an eastern fox squirrel specimen used to investigate the environmental drivers of color polymorphism. Each circle, scaled to 15 mm diameter, shows the location where we measured coloration using a handheld digital color sensor ((A) = Head, (B) = Shoulder, (C) = Rump, (D) = Dorsum, (E) = Venter).
We measured environmental characteristics that previous studies show influence pelage variation within a 3.7 km radius around each specimen (Greene and McCleery, 2017). The 3.7 km radius (hereafter, buffer) is the average dispersal distance of southeastern fox squirrels (Wooding, 1997). We measured seven environmental variables, the proportion of burned area between 1992–2015 (Prop_Burned), the number of fires that occurred between 1992–2015 (Count_Fire), the mean percent canopy cover (Canopy), the mean elevation in meters (Mean_Elevation), the mean annual precipitation from 1981–2014 in cm (Precip), the dominant soil order (Soil), and proportion of landcover type.
We chose proportion of landcover as a metric to describe the available habitat to each squirrel, and to test how much variation in pelage could be ascribed to habitat. We calculated the proportion of landcover type in each squirrel buffer using the 2011 National Land Cover Database (NLCD; Homer et al., 2015). To reduce the number of landcover types, we binned the original classifications into 19 classes based on the Florida Natural Areas Inventory (Florida Natural Areas Inventory, 2010; Tye et al., 2017). To further reduce the number of landcover types in our analysis, we only included landcover classes where fox squirrels have been observed in Florida, which were Hardwood and Hammock, Pinelands, Crops, Coniferous Wetlands, Parks Cemeteries and Golf Courses, and Tree Plantations (Greene and McCleery, 2017).
We selected Prop_Burned and Count_Fire as explanatory variables because previous studies have shown correlations between fox squirrel dorsal melanism and fire-blackened substrates (Kiltie, 1992b) as well as the number of historic wildfires (Kiltie, 1989). We calculated Prop_Burned and Count_Fire in each buffer using the spatial wildfire occurrence dataset from 1992–2015 (Short, 2017). The spatial wildfire occurrence dataset consists of ignition points for wildfires, each of which has an associated attribute for the final fire size. We created circular polygons around each ignition point such that the area of the circle equaled the fire size of the associated point and measured the amount of burned overlap in each squirrel buffer. In each buffer, we measured a burned area only once even if the same area burned on multiple occasions.
We included Canopy as an explanatory variable to describe the openness of the habitat, which influences dorsal pelage in rodents (Ancillotto and Mori, 2017). We calculated mean percent canopy in each squirrel buffer from the 2011 NLCD Tree Canopy Cartographic dataset (Homer et al., 2015). Each 30 m2 pixel in this raster layer contains a single value for percent canopy cover, which we averaged for each squirrel buffer. We included Precip and Elevation as explanatory variables in our model because both variables have been correlated with fox squirrel pelage variation in previous studies (Kiltie, 1989). We used a digital elevation model (DEM; Gesch et al., 2018) with 30 m2 resolution to calculate Mean_Elevation within a squirrel buffer. To calculate Precip, we averaged the annual precipitation from 1981–2010 (PRISM Climate Group, 2020) within a squirrel buffer. We retrieved soil data from the Web Soil Survey (USDA Natural Resources Conservation Service, 2020), and calculated the proportion of all soil orders in a fox squirrel dispersal buffer. To reduce the number of parameters in our model, we included only the most common (greatest proportion in a buffer) soil order for each squirrel in our analysis.
To better understand the environmental variables that influence fox squirrel polymorphism, we subset the pelage color data into four non-exclusive groups. The first subset (Specimen, Full Color [SFC]) contained the L∗, a∗, and b∗ color data from all the locations we measured on each specimen. The second subset (Specimen, Brightness [SB]) included only the L∗ axis from each of the measured body components. This allowed us to explicitly test our prediction that darker pelage would positively correlate with increasing canopy cover in a fox squirrel’s dispersal buffer. The third (Dorsum, Full Color [DFC]) and fourth (Dorsum, Brightness [DB]) data subsets also included the L∗, a∗, and b∗, and the L∗ measurements only, respectively, but only used the three measurements from dorsal locations (shoulder, dorsum, rump). These subsets allowed us to compare our results to past research that focused only on fox squirrel dorsal pelage variation (Kiltie, 1989, 1992a,b).
To identify the environmental drivers of pelage variation, we conducted a redundancy analysis (RDA) for each subset of squirrel pelage color values. An RDA extends multiple regression to test relationships between a multivariate response matrix and multiple predictor variables. For analysis the response matrix was a squirrel-by-color measurement matrix for the particular group (i.e., SFC SB, DFC, or DB) and the response variables were the seven environmental variables we detailed above (van den Wollenberg, 1977). We standardized all continuous response and predictor variables to have a mean of 0 and standard deviation (SD) of 1. For each RDA, we used a forward selection approach to identify the model terms that create the most parsimonious model of predictor variables (Blanchet et al., 2008). Forward selection iteratively models individual predictor variables to the response variables, and additively combines significant predictor variables until additional predictors do not significantly improve model fit (R2). We used α ≤ 0.05 for determining significant variables. Using both α and R2 as stopping criteria in forward selection reduces type I error, a common problem with forward selection approaches (Blanchet et al., 2008). If forward selection identified more than one significant predictor variable, we used variance partitioning to quantify the unique amount of variation explained by each term (Peres-Neto et al., 2006).
For all RDAs we used a permutation test with 999 permutations to test for significance of the model terms and canonical axes (Legendre et al., 2011). We calculated an adjusted R2 (R2adj) using Ezekiel’s formula (Legendre et al., 2011) to determine the overall amount of variation explained by each model. To ensure model results were not influenced by spatial autocorrelation (Tobler, 1970) we plotted residuals from each model as a function of spatial distance between specimens (Wagner, 2004). We found that model residuals showed no dependency on distance and model results were not affected by spatial autocorrelation (Wagner, 2004). We therefore did not include any spatial covariates in our model. We performed all multivariate statistical analysis in R Version 3.5.0 (R Core Team, 2018) using the package Vegan (Oksanen et al., 2017).
We measured pelage coloration from 135 fox squirrel specimens. Fox squirrels showed the greatest variation along the L∗ axis (brightness), followed by the b∗ axis (blue to yellow coloration), and the least amount of variation along the a∗ axis (green to red coloration). Fox squirrels exhibited the least amount of variation overall in head coloration as indicated by the narrowest range and lowest SD along the L∗ (mean = 18.8 ± 3.10 SD), a∗ (mean = 0.50 ± 0.47 SD), and b∗ (mean = 1.00 ± 1.00 SD) axes (Figure 4). Fox squirrel venters had the widest range and largest SD for all color axes (L∗: mean = 62.90 ± 11.40 SD; a∗: mean = 5.40 ± 2.69 SD; b∗: mean = 16.5 ± 5.57 SD). The three dorsal metrics, shoulder, dorsum, and rump were similar along the a∗ and b∗ axis (a∗Shoulder: mean = 2.17 ± 1.09 SD; b∗Shoulder: mean = 7.72 ± 3.20 SD; a∗Dorsum: mean = 1.94 ± 1.06 SD; b∗Dorsum: mean = 7.55 ± 3.41 SD; a∗Rump: mean = 2.13 ± 0.98 SD; b∗Rump: mean = 8.66 ± 2.84 SD). The L∗ value was similar for shoulder and dorsum (L∗Shoulder: mean = 35.30 ± 8.34 SD; L∗Dorsum: mean = 36.20 ± 9.58 SD), but was greater and had a lower SD for rump (L∗Rump: mean = 40.40 ± 7.59 SD). Greater L∗ values for the rump compared to the dorsum and shoulder indicate that fox squirrel dorsal coloration lightens (decreased melanism) between the central portion of the dorsum and the base of the tail. Qualitatively, increased a∗ and b∗ dorsal values represent agouti-colored pelage, while lower L∗ values represent melanistic pelage (Figure 4).
Figure 4. Distribution of color measurements along the L* (dark to light), a* (green to red) and b* (blue to yellow) axes from eastern fox squirrels (A) head, (B) shoulder, (C) rump, (D) dorsum, and (E) venter. Each circle represents an individual squirrel and the fill color of each circle matches the color recorded.
Using the SFC data subset, the forward selection identified the proportion of burned area (Prop_Burned) as the only significant model term (p < 0.001) in the RDA. Since there was only one predictor variable, there was only one RDA axis that explained pelage variation (p = 0.003). The model adjusted R2 = 0.03. In general, a greater proportioned of burned area positively correlated with greater values of a∗ (increased red) and b∗ (increased yellow), and negatively correlated with increasing L∗ (lighter colors) for the venter (Figure 5A).
Figure 5. Results of forward selection redundancy analysis (RDA) showing associations between ecological factors and eastern fox squirrel pelage color measurements at multiple locations on each specimen. Each plot shows a different, non-exclusive data subset ((A) = full specimen [head, shoulder, dorsum, rump, venter]), full color [L*, a*, b*]; (B) = Full specimen, brightness only [L*]; (C) = dorsal [shoulder, dorsum, rump], full color; (D) = dorsal, brightness only). The length of each environmental variable (blue arrow) along each axis correlates to the amount of variation that variable accounts for. Pelage measurements (red arrows) positively correlate with an environmental variable if both arrows stretch in the same direction. For plots (A), (C), and (D), the x-axis shows the only significant RDA axis from forward selection, while the y-axis shows the first principal components (PCA). The PCA axis represents the residuals of the constrained ordination.
Using the SB data subset, the forward selection identified an additive model with the predictor variables Crops (p = 0.01), Precip (p = 0.03), and Canopy (p = 0.014) as the most parsimonious model. Using variance partitioning we found that Crops, Precip, and Canopy accounted for 3.8, 1.9, and 1.9% of the variation in pelage brightness, respectively. Only the first RDA axis explained a significant amount of variation in the pelage data (RDA1, p = 0.002). Crops loaded most on RDA1 (0.67) followed by Precip (−0.46) and Canopy (0.15). Precip loaded most heavily (0.81) on RDA2, but RDA2 did not explain a significant amount of variation in the data (p = 0.31). The model adjusted R2 = 0.05. Along RDA1, increasing proportion of row crops was positively associated with increasing L∗ values for all body components except head (Figure 5B).
The forward selection for the DFC subset identified only Prop_Burned as a significant model term (p < 0.001). Since there was only one predictor variable, there was only one RDA axis that explained pelage variation (p < 0.001) with model adjusted R2 = 0.05. Increasing Prop_Burned was most associated with increased rump a∗ coloration, as well as increased rump b∗ and dorsum a∗ (Figure 5C).
The forward selection for the DB subset identified only Crops as a significant model term (p = 0.016). Since there was only one predictor variable, there was only one RDA axis that explained pelage variation (p = 0.009) with model adjusted R2 = 0.03. Increasing proportion of row crops was associated with increasing L∗ in shoulder, dorsum, and rump coloration (Figure 5D).
We found that variation in fox squirrel pelage coloration was associated with differences in spatial and temporal environmental heterogeneity. Fires can increase heterogeneity in the environment at a variety of scales (McKenzie et al., 1995), while cropland remains comparatively stable. Fire may therefore promote pelage variation while crops promote a more uniform light-colored pelage morph. Our results support previous findings that environmental heterogeneity shapes pelage polymorphism by manifesting a variety of different colored backgrounds for prey to match (Kettlewell, 1955; Merilaita et al., 1999; Bond and Kamil, 2006).
We found support for our prediction that agouti pelage was positively associated with fire (Figures 3, 5). Previous research found that dorsally pale-agouti and intermediate-agouti (partial melanism) colored fox squirrels were superiorly camouflaged against a variety of backgrounds in fire-prone ecosystems (Kiltie, 1992a, b). Compared to other fox squirrel pelage morphs, agouti pelage is the best overall at background matching against the ground and common trees in the southeast (Kiltie, 1992b). The large amount of variation within the agouti morph may make it well-adapted for concealment in the spatially and temporally heterogeneous habitats found in fire-driven ecosystems (McKenzie et al., 1995). For example, in the open pine forests of the southeastern United States, frequent fire can create a bare understory (Darracq et al., 2016), in which pale agouti rodents are most cryptic (Ancillotto and Mori, 2017). Fox squirrels in open pine forests rely on interspersed hardwoods for food and nest sites (Perkins et al., 2008), and hardwoods can create fire shadows which prevent complete burning (Jack and Pecot, 2017), increasing heterogeneity at a fine spatial scale. In contrast with burned areas, croplands in the Southeast are temporally and spatially stable, likely favoring a more consistent, uniformly colored population. We found that cropland was associated with decreased melanism in fox squirrels, possibly indicating that fox squirrels in human-engineered landscapes converge toward a uniform light-colored morph.
Dark-colored rodents are more cryptic in darker environments (Wauters et al., 2004; Ancillotto and Mori, 2017). However, we found no support for our prediction, that dark-colored pelage would be positively associated with closed canopy ecosystems. Fox squirrels are primarily found in open canopy forests (Moore, 1957; Weigl et al., 1989). Although fox squirrels use closed canopy hammocks occasionally (Weigl et al., 1989; Conner et al., 1999), periodic usage of closed canopy areas may not be enough to influence pelage coloration. Thus, fox squirrels may not use poorly lit closed canopy forests enough for dark pelage to have a cryptic advantage in these areas.
We did not find that countershading was associated with open canopy forest. Although forward selection identified canopy cover as a significant term in the SB model, canopy cover explained relatively little variation along the only significant RDA axis (RDA1). Furthermore, increased canopy cover was associated with lighter colored pelage in fox squirrel dorsal and ventral coloration (Figure 4). Countershading may not be an effective antipredator adaptation in terrestrial environments (Kiltie, 1988; Ruxton et al., 2004) and thus, countershading is unlikely to convey an antipredator advantage to fox squirrels.
Our analysis was limited by measuring pelage coloration in CIELab color space, which is based on human vision. Most animals have different visual acuity and color perception than humans (Endler, 1978; Cuthill et al., 2017), and therefore may not respond to the colors as we measured them; however, it was beyond the scope of this study to incorporate predator vision and behavior into our analysis. Our results explained only a small amount of pelage variation, suggesting that additional environmental factors not included in our analysis are likely driving pelage variation. Animal coloration is driven by additional factors other than predation risk (Smith et al., 1972), such as communication and thermoregulation (Stoner et al., 2003; Caro, 2005), hair strength (Bonser, 1995), or a combination of multiple factors (Cloudsley-Thompson, 1999). Fox squirrels are capable of frequent long-distance movements (Potash et al., 2018) and may therefore encounter a variety of ecological conditions that influence pelage coloration. Thus, there are likely many factors driving pelage coloration, preventing any individual variable we included in our analysis from explaining large amounts of pelage variation.
In this study we showed clear links exist between environmental heterogeneity and color polymorphism. To preserve diverse animal color patterns, management and conservation efforts should consider the spatial and temporal scale of all management actions. Pelage adaptations to heterogeneity may be ineffective and lost in regularly managed ecosystems. Managing ecological processes, such as prescribed fire to promote heterogeneity rather than uniformity (Darracq et al., 2016) may be key for maintaining animal color diversity.
The datasets generated for this study are available on request to the corresponding author.
Ethical review and approval was not required for the animal study because the animals used in this study were previously collected opportunistically as roadkill and were part of the Florida Museum of Natural History’s mammal collections.
AP, DG, LC, and RM conceived the original idea. AP, DG, and VM collected the data and developed data collection protocols. AP, BB, and RM developed the statistical analyses. All authors contributed to the final manuscript.
Funding for this study was provided by Florida’s State Wildlife Grant through Florida’s Wildlife Legacy Initiative. The University of Florida Open Access Publishing Fund provided funding for open access publishing fees.
DG was employed by the company Weyerhaeuser Company.
The remaining authors declare that the research was conducted in the absence of any commercial or financial relationships that could be construed as a potential conflict of interest.
We thank the numerous citizens and agency staff, especially from the Florida Fish and Wildlife Conservation Commission (FWC) for their help with collecting specimens for this research. Several people made substantial contributions to this project: P. Moler, C. Tye, and A. Mitchell of FWC, J. Kellam and D. Jansen from the National Park Service’s Big Cypress National Preserve, L. Wagner and S. Harris of the University of Florida, and S. Zoellner, contributed to collecting the specimens. We thank E. Webb, J. Newkirk, M. Herfurth, F. Hayes, B. Clemons, R. Bäck, S. Ryburn, M. Duque, J. Brown, and G. Foursa for their assistance preparing specimens and measuring coloration. We thank the University of Florida, the Jones Center at Ichauway, and FWC for support.
Ancillotto, L., and Mori, E. (2017). Adaptive significance of coat colouration and patterns of Sciuromorpha (Rodentia). Ethol. Ecol. Evol. 29, 241–254. doi: 10.1080/03949370.2016.1145146
Blanchet, F. G., Legendre, P., and Borcard, D. (2008). Forward selection of explanatory variables. Ecology 89, 2623–2632.
Bond, A. B., and Kamil, A. C. (2006). Spatial heterogeneity, predator cognition, and the evolution of color polymorphism in virtual prey. Proc. Natl. Acad. Sci. U.S.A. 103, 3124–3219. doi: 10.1073/pnas.0509963103
Bonser, R. H. C. (1995). Melanin and the abrasion resistance of feathers. Condor 97, 590–591. doi: 10.2307/1369048
Boratyñski, Z., Brito, J. C., Campos, J. C., Karala, M., and Mappes, T. (2014). Large spatial scale of the phenotype-environment color matching in two cryptic species of African desert jerboas (Dipodidae: Jaculus). PLoS One 9:e94342. doi: 10.1371/journal.pone.0094342
Cloudsley-Thompson, J. L. (1999). Multiple factors in the evolution of animal coloration. Naturwissenschaften 86, 123–132. doi: 10.1007/s001140050584
Conner, L. M., Landers, J. L., and Michener, W. K. (1999). Fox squirrel and gray squirrel associations within minimally disturbed longleaf pine forests. Proc. Annu. Conf. Southeast. Assoc. Fish Wildl. Agencies 53, 364–374.
Cuthill, I. C., Allen, W. L., Arbuckle, K., Caspers, B., Chaplin, G., Hauber, M. E., et al. (2017). The biology of color. Science 357:eaan0221. doi: 10.1126/science.aan0221
da Silva, L. G., de Oliveira, T. G., Kasper, C. B., Cherem, J. J., Moraes, E. A., Paviolo, A., et al. (2016). Biogeography of polymorphic phenotypes: mapping and ecological modelling of coat colour variants in an elusive Neotropical cat, the jaguarundi (Puma yagouaroundi). J. Zool. 299, 295–303. doi: 10.1111/jzo.12358
Darracq, A. K., Boone, W. W., and McCleery, R. A. (2016). Burn regime matters: a review of the effects of prescribed fire on vertebrates in the longleaf pine ecosystem. For. Ecol. Manage. 378, 214–221. doi: 10.1016/j.foreco.2016.07.039
Endler, J. A. (1978). “A predator’s view of animal color patterns,” in Evolutionary Biology, eds M. K. Hacht, W. C. Steere, and B. Wallace (New York, NY: Plenum Press), 319–364.
Florida Fish and Wildlife Conservation Commission (2013). A Species Action Plan for the Sherman’s Fox Squirrel Sciurus niger shermani. Tallahassee, FL: Florida Natural Areas Inventory.
Florida Natural Areas Inventory (2010). Guide to the Natural Communities of Florida, 2010 edition. Tallahassee, FL: Florida Natural Areas Inventory.
Gesch, D. B., Evans, G. A., Oimoen, M. J., and Arundel, S. (2018). “The national elevation dataset,” in DEM Users Manual, ed. D. F. Maune (Bethesda, MD: American Society for Photogrammetry and Remote Sensing), 83–110.
Gray, S. M., and McKinnon, J. S. (2007). Linking color polymorphism maintenance and speciation. Trends Ecol. Evol. 22, 71–79. doi: 10.1016/j.tree.2006.10.005
Greene, D. U., and McCleery, R. A. (2017). Multi-scale responses of fox squirrels to land-use changes in Florida: utilization mimics historic pine savannas. For. Ecol. Manage. 391, 42–51. doi: 10.1016/j.foreco.2017.02.001
Håstad, O., Victorsson, J., and Ödeen, A. (2005). Differences in color vision make passerines less conspicuous in the eyes of their predators. Proc. Natl. Acad. Sci. U.S.A. 102, 6391–6394. doi: 10.1073/pnas.0409228102
Hodgen, J. (2016). Comparison of nix color sensor and nix color sensor pro to standard meat science research colorimeters. Meat Sci. 112:159. doi: 10.1016/j.meatsci.2015.08.129
Holman, B. W. B., Collins, D., Kilgannon, A. K., and Hopkins, D. L. (2018). The effect of technical replicate (repeats) on Nix Pro Color SensorTM measurement precision for meat: a case-study on aged beef colour stability. Meat Sci. 135, 42–45. doi: 10.1016/j.meatsci.2017.09.001
Homer, C. C. G., Dewitz, J. J. A., Yang, L., Jin, S., Danielson, P., Xian, G., et al. (2015). Completion of the 2011 national land cover database for the conterminous United States - representing a decade of land cover change information. Photogramm. Eng. Remote Sens. 81, 345–354. doi: 10.14358/PERS.81.5.345
Jack, S. B., and Pecot, S. D. (2017). “Regeneration dynamics, competition, and seedling response,” in Ecological Restoration and Management of Longleaf Pine Forests, eds L. K. Kirkman and S. B. Jack (Boca Raton, FL: CRC Press), 71–88.
Kasparson, A. A., Badridze, J., and Maximov, V. V. (2013). Colour cues proved to be more informative for dogs than brightness. Proc. R. Soc. B Biol. Sci. 280:20131356. doi: 10.1098/rspb.2013.1356
Kettlewell, H. B. D. (1955). Selection experiments on industrial melanism in the Lepidoptera. Heredity 9, 323–342. doi: 10.1038/hdy.1955.36
Kiltie, R. A. (1988). Countershading: universally deceptive or deceptively universal? Trends Ecol. Evol. 3, 21–23. doi: 10.1016/0169-5347(88)90079-90071
Kiltie, R. A. (1989). Wildfire and the evolution of dorsal melanism in fox squirrels, Sciurus niger. J. Mammal. 70, 726–739.
Kiltie, R. A. (1992a). Camouflage comparisons among fox squirrels from the Mississippi river delta. J. Mammal. 73, 906–913.
Kiltie, R. A. (1992b). Tests of hypotheses on predation as a factor maintaining polymorphic melanism in coastal plain fox squirrels (Sciurius niger L.). Biol. J. Linn. Soc. 45, 17–37. doi: 10.1017/CBO9781107415324.004
Krupa, J. J., and Geluso, K. N. (2000). Matching the color of excavated soil: cryptic coloration in the plains pocket gopher (Geomys bursarius). J. Mammal. 81, 86–96. doi: 10.1644/1545-15422000081<0086:MTCOES>2.0.CO;2
Legendre, P., Oksanen, J., and ter Braak, C. J. F. (2011). Testing the significance of canonical axes in redundancy analysis. Methods Ecol. Evol. 2, 269–277. doi: 10.1111/j.2041-210X.2010.00078.x
McKenzie, D., Miller, C., and Falk, D. A. (1995). The landscape ecology of Fire - Chapter 1 toward a theory of landscape fire. Landsc. Ecol. Fire 213:346. doi: 10.1007/978-94-007-0301-308
Merilaita, S., Tuomi, J., and Jormalainen, V. (1999). Optimization of cryptic coloration in heterogenous habitats. Biol. J. Linn. Soc. 67, 151–161. doi: 10.1006/bijl.1998.0298
Moore, J. C. (1957). The natural history of the fox squirrel, Sciurus niger shermani. Bull. Am. Museum Nat. Hist. 113, 1–71.
Oksanen, J., Guillaume Blanchet, F., Friendly, M., Kindt, R., Legendre, P., McGlinn, D., et al. (2017). Vegan: Community Ecology Package. R Package Version 2.4-4. Available online at: https://cran.r-project.org/package=vegan (accessed August 24, 2017).
Peres-Neto, P. R., Legendre, P., Dray, S., and Borcard, D. (2006). Variation partitioning of species data metrices: estimation and comparison of fractions. Ecology 87, 2614–2625. doi: 10.2307/20069271
Perkins, M. W., Conner, L. M., and Brent Howze, M. (2008). The importance of hardwood trees in the longleaf pine forest ecosystem for Sherman’s fox squirrels. For. Ecol. Manage. 255, 1618–1625. doi: 10.1016/j.foreco.2007.11.020
Potash, A. D., Conner, L. M., and McCleery, R. A. (2018). Extreme movements of an individual male fox squirrel. Southeast. Nat. 17, N40–N43. doi: 10.1656/058.017.0304
Potash, A. D., Greene, D. U., Foursa, G. A., Mathis, V. L., Conner, L. M., and McCleery, R. A. (2020). A comparison of animal color measurements using a commercially available digital color sensor and photograph analysis. Curr. Zool. zoaa016. doi: 10.1093/cz/zoaa016
PRISM Climate Group (2020). PRISM Climate Data. Available online at: prism.oregonstate.edu (accessed July 8, 2019).
R Core Team (2018). R: A Language and Environment for Statistical Computing. Available online at: https://www.r-project.org/ (accessed April 24, 2018).
Ruxton, G. D., Speed, M. P., and Kelly, D. J. (2004). What, if anything, is the adaptive function of countershading? Anim. Behav. 68, 445–451. doi: 10.1016/j.anbehav.2003.12.009
Short, K. C. (2017). Spatial Wildfire Occurrence Data for the United States, 1992-2015 [FPA_FOD_20170508]. Fort Collins, CO: Forest Service Research Data Archive.
Smith, M. H., Carmon, J. L., and Gentry, J. B. (1972). Pelage color polymorphism in Peromyscus polionotus. J. Mammal. 53, 824–833.
Steele, M. A., and Koprowski, J. L. (2001). North American Tree Squirrels. Washington, DC: Smithsonian Books.
Stiglitz, R., Mikhailova, E., Post, C., Schlautman, M., and Sharp, J. (2016). Evaluation of an inexpensive sensor to measure soil color. Comput. Electron. Agric. 121, 141–148. doi: 10.1016/j.compag.2015.11.014
Stoner, C. J., Caro, T. M., and Graham, C. M. (2003). Ecological and behavioral correlates of coloration in artiodactyls: systematic analyses of conventional hypotheses. Behav. Ecol. 14, 823–840. doi: 10.1093/beheco/arg072
Sumasgutner, P., Rose, S., Koeslag, A., and Amar, A. (2018). Exploring the influence of urbanization on morph distribution and morph-specific breeding performance in a polymorphic African raptor. J. Raptor Res. 52, 19–30. doi: 10.3356/JRR-17-37.1
Tobler, W. R. (1970). A computer movie simulating urban growth in the Detroit region. Econ. Geogr. 46, 234–240.
Tye, C. A., Greene, D. U., Giuliano, W. M., and McCleery, R. A. (2015). Using camera-trap photographs to identify individual fox squirrels (Sciurus niger) in the Southeastern United States. Wildl. Soc. Bull. 39, 645–650. doi: 10.1002/wsb.573
Tye, C. A., McCleery, R. A., Fletcher, R. J., Greene, D. U., and Butryn, R. S. (2017). Evaluating citizen vs. professional data for modelling distributions of a rare squirrel. J. Appl. Ecol. 54, 628–637. doi: 10.1111/1365-2664.12682
USDA Natural Resources Conservation Service (2020). Web Soil Survey. Available online at: https://websoilsurvey.sc.egov.usda.gov/(accessed July 11, 2019).
van den Wollenberg, A. L. (1977). Redundancy analysis an alternative for canonical correlation analysis. Psychometrika 42, 207–219. doi: 10.1007/BF02294050
Wagner, H. H. (2004). Direct multi-scale ordination with canonical correspondence analysis. Ecology 85, 342–351. doi: 10.1890/02-0738
Wauters, L. A., Zaninetti, M., Tosi, G., and Bertolino, S. (2004). Is coat-colour polymorphism in Eurasian red squirrels (Sciurus vulgaris L.) adaptive? Mammalia 68, 37–48. doi: 10.1515/mamm.2004.005
Weigl, P. D., Steele, M. A., Sherman, L. J., and Ha, J. C. (1989). The Ecology of the Fox Squirrel (Sciurus niger) in North Carolina: Implications for Survival in the Southeast. Tallahassee, FL: Tall Timbers Research Station.
Wooding, J. B. (1997). Distribution and Population Ecology of the Fox Squirrel in Florida. Ph.D. dissertation. University of Florida, Florida.
Keywords: adaptation, color, fire, fox squirrel, pelage, polymorphism
Citation: Potash AD, Greene DU, Mathis VL, Baiser B, Conner LM and McCleery RA (2020) Ecological Drivers of Eastern Fox Squirrel Pelage Polymorphism. Front. Ecol. Evol. 8:119. doi: 10.3389/fevo.2020.00119
Received: 16 March 2020; Accepted: 14 April 2020;
Published: 07 May 2020.
Edited by:
Toni Gossmann, Bielefeld University, GermanyReviewed by:
Emiliano Mori, University of Siena, ItalyCopyright © 2020 Potash, Greene, Mathis, Baiser, Conner and McCleery. This is an open-access article distributed under the terms of the Creative Commons Attribution License (CC BY). The use, distribution or reproduction in other forums is permitted, provided the original author(s) and the copyright owner(s) are credited and that the original publication in this journal is cited, in accordance with accepted academic practice. No use, distribution or reproduction is permitted which does not comply with these terms.
*Correspondence: Alex D. Potash, YXBvdGFzaEB1ZmwuZWR1
†Present address: Daniel U. Greene, Weyerhaeuser Company, Environmental Research South, Columbus, MS, United States
‡ORCID: Benjamin Baiser orcid.org/0000-0002-3573-1183
Disclaimer: All claims expressed in this article are solely those of the authors and do not necessarily represent those of their affiliated organizations, or those of the publisher, the editors and the reviewers. Any product that may be evaluated in this article or claim that may be made by its manufacturer is not guaranteed or endorsed by the publisher.
Research integrity at Frontiers
Learn more about the work of our research integrity team to safeguard the quality of each article we publish.