- 1Organismal and Evolutionary Biology Research Programme, University of Helsinki, Helsinki, Finland
- 2Department of Biology, Centre for Biodiversity Dynamics, Norwegian University of Science and Technology, Trondheim, Norway
- 3Spatial Foodweb Ecology Group, Department of Agricultural Sciences, University of Helsinki, Helsinki, Finland
- 4Spatial Foodweb Ecology Group, Department of Ecology, Swedish University of Agricultural Sciences, Uppsala, Sweden
- 5Forest Health and Biodiversity, Natural Resources Institute Finland (LUKE), Helsinki, Finland
- 6Zoology, University of New England, Armidale, NSW, Australia
- 7Department of Evolutionary Biology and Environmental Studies, University of Zurich, Zurich, Switzerland
- 8Department of Bioscience, Aarhus University, Roskilde, Denmark
- 9Arctic Research Centre, Aarhus University, Aarhus, Denmark
- 10Terrestrial Ecology Research Group, Department of Ecology and Ecosystem Management, Technical University of Munich, Freising, Germany
- 11Field Station Fabrikschleichach, Department of Animal Ecology and Tropical Biology, Julius-Maximilians-University Würzburg, Rauhenebrach, Germany
- 12Centre for Biodiversity Genomics & Department of Integrative Biology, University of Guelph, Guelph, ON, Canada
The kingdom Fungi is a megadiverse group represented in all ecosystem types. The global diversity and distribution of fungal taxa are poorly known, in part due to the limitations related to traditional fruit-body survey methods. These previous hurdles are now being overcome by rapidly developing DNA-based surveys. Past fungal DNA surveys have predominantly examined soil samples, which capture high species diversity but represent only the local soil community. Recent work has shown that DNA samples collected from the air with cyclone samplers provide information on fungal diversity at the scale of some tens of kilometers around the sampling location. To test the feasibility of air sampling for investigating global patterns of fungal diversity, we established a new initiative called the Global Spore Sampling Project (GSSP). The GSSP currently involves 50 sampling locations distributed on all continents, with each location collecting two 24-h samples per week. Here we describe the GSSP methodology, including the sampling, DNA extraction and sequencing protocols, and the bioinformatics pipeline. We further report results based on 75 pilot samples from five locations, of which three in Europe, one in Australia, and one in Greenland. The results show highly consistent patterns, suggesting that GSSP holds much promise for systematic global fungal monitoring. The GSSP provides highly standardized sampling across space and time, enabling much-improved estimation of total fungal diversity, the global distribution of different fungal groups, fungal fruiting phenology, and the extent of long-distance dispersal in fungi.
Introduction
Species in the megadiverse fungal kingdom play fundamental roles in ecosystem functioning as mutualists, decomposers, and pathogens. Over 80% of land plants establish mutualistic associations with mycorrhizal fungi (Wang and Qiu, 2006), which facilitate mineral and water uptake (Smith and Read, 2008). Saprotrophic fungi are primary decomposers of the organic matter in terrestrial ecosystems (Baldrian and Valášková, 2008). Pathogenic fungi have important implications for human health and food production (Almeida et al., 2019). Despite their importance, our knowledge of global fungal diversity and biogeography is minimal. While ca. 100,000 species of fungi have been described, estimates of global species richness vary between 0.5 and 10 million (Hawksworth and Lücking, 2017). Furthermore, current knowledge on the diversity and ecology of fungi is biased toward those groups producing macroscopic structures (mostly those producing visible fruiting bodies), even if the diversity of microscopic fungi is vastly greater.
The recent proliferation of environmental DNA based studies have overcome many limitations of fruiting body-based surveys, advancing knowledge of large-scale patterns of fungal diversity (e.g., Sato et al., 2012; Tedersoo et al., 2014; Barberán et al., 2015; Davison et al., 2015). To date, most fungal biogeographical studies have focused on soil communities (but see Barberán et al., 2015) even if different substrates (e.g., wood, litter) support very different assemblages. Furthermore, large-scale fungal studies have mostly been based on samples acquired from distant sites, although it is known that fungal communities can exhibit high spatial variation at very small spatial scales (Hazard et al., 2012; Kubartová et al., 2012). Thus, there are major knowledge gaps regarding the large-scale distributions of fungi, in particular of fungi other than those inhabiting soil.
Since many fungi disperse by windborne spores, DNA surveys based on aerial samples provide an alternative for characterizing the regional fungal composition. As demonstrated by Abrego et al. (2018), aerial sampling can simultaneously sample fungi growing on diverse substrates, while providing a regional scale perspective of some tens of kilometers. To test the feasibility of air sampling for investigating the global patterns of fungal diversity, we established an initiative called the Global Spore Sampling Project (GSSP). We first made a preliminary survey to identify researchers who would be interested in joining the project as sampling teams, and then selected the sampling teams so that we achieved maximal global coverage. Additional partners were encouraged to join if they were able to purchase the sampling equipment themselves. The GSSP currently involves fifty sampling locations distributed across all continents, with each location collecting two 24-h samples per week.
The GSSP project is designed to address research questions of both fundamental and applied nature. To start with, by examining accumulation curves for operational taxonomical units (OTUs) within and among locations, it will improve estimates of fungal diversity. With the help of taxonomic placement of the unknown OTUs (Abarenkov et al., 2018), GSSP will further help to reveal those groups of fungi that are least represented in taxonomy and sequence reference databases. Most importantly, it will provide a much-improved view of global fungal biogeography, shedding light e.g. on how fungal diversity changes along latitude. Based on research on soil fungi, such patterns may deviate substantially from those in other organisms (Tedersoo et al., 2014). Furthermore, these global data may reveal the distributions and temporal dynamics of fungi affecting humans, such as pathogenic fungi causing diseases or infecting crop plants.
In this paper, we describe the GSSP methodology, including the sampling, DNA extraction, sequencing, and concentration estimation protocols, and the bioinformatics pipeline. We further report results based on 75 pilot samples from five locations, of which three in Europe, one in Australia, and one in Greenland.
Methods
The Global Spore Sampling Project (GSSP) is a globally distributed network of sampling locations (Figure 1) equipped with a cyclone sampler (Burkard Cyclone Sampler for Field Operation, Burkard Manufacturing Co Ltd; http://burkard.co.uk/product/cyclone-sampler-for-field-operation; Emberlin and Baboonian, 1995). The current network includes fifty sampling locations that cover all continents, but is most dense in Europe (Figure 1). At each sampling location, two 24-h samples are taken each week. Although sampling was planned to start synchronously in October 2018, realized sampling was initiated earlier at a few localities and later in some other localities (Figure 1). The sampling locations represent varying latitudes and altitudes. Some samplers are located in urban environments, while others are positioned in natural environments (e.g., forests, tundra).
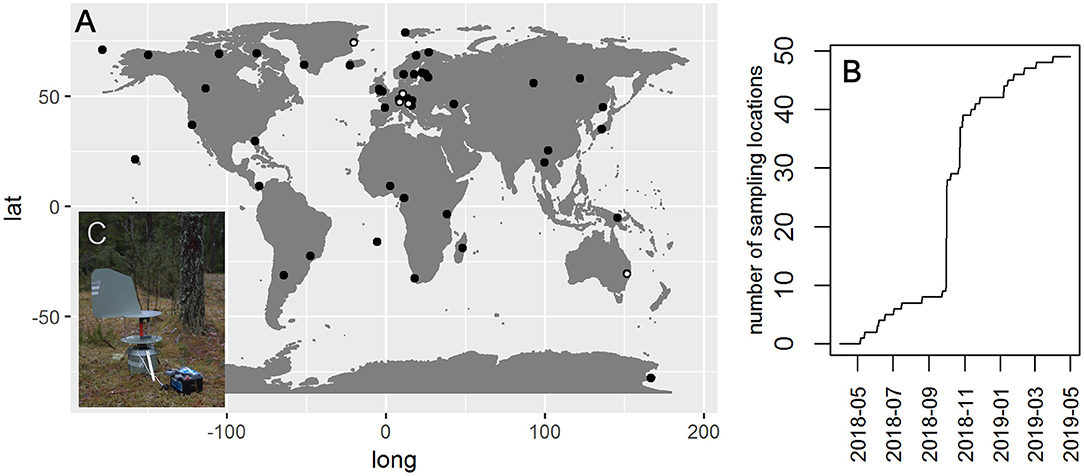
Figure 1. GSSP sampling locations (A) and the increase in the number of sampling locations over time (B). The five locations from which the pilot data analyzed in this paper originate are indicated by white dots. (C) Shows the cyclone sampler connected to a car battery.
In this paper, we utilize 75 samples collected during the pilot phase of GSSP from five sites (Figure 1). More details on these samples, such as the description of the sampling locations as well as the timing of the sampling, are given in Supplementary Information.
Sampling Protocol
Air DNA samples were acquired using cyclone samplers placed at ground level to ensure free airflow through the sampler. The cyclone sampler (shown in Figure 1) orientates itself in the direction of the wind and collects all particles from the air with a single reverse flow cyclone. The sampler collects particles with size >1 μm from the air directly into a sampling tube, including spores, pollen, bacteria, and small insects. The sampler's average air throughput is 16.5 liters per minute for a total of 23,800 liters during each 24-h sampling period. Sterile 1.5 ml Eppendorf vials were used as sampling tubes. After sampling, the vial was removed from the cyclone sampler, the lid was closed, and the vials were labeled with the site code and week number. Likewise, the time and duration of the sampling, as well as notes about the presence of rainwater or larger objects (e.g., arthropods), were recorded. Every week, two 24-h samples (henceforth called Sample A and Sample B) were collected from each site. To avoid contamination, gloves were used while handling the samples or the device. Sampling teams were instructed to clean the cyclone part of the device monthly with water and soap and to rinse it with ethanol, or to sterilize it with dry-heat, chlorine or UV when such equipment was available.
The samples were stored at −20°C until they were shipped to the University of Helsinki, Finland. Shipping was at room temperature as the shipping time was relatively short and refrigerated transport would be costly. In Helsinki, visible arthropods were removed from the samples. To avoid losing fungal spores attached to the arthropod bodies, their surface was rinsed by adding sterile water into the sample tube and vortexing. After washing, the arthropods were removed with sterile tweezers. Samples with rainwater were dried in a freeze drier (24 h, −80°C, 0.57 mbar) covered with a porous Parafilm to avoid cross-contamination between samples. After drying, all samples were sent to the University of Guelph, Canada, for DNA extraction and sequencing.
DNA Extraction, Primers, and Sequencing
Upon receipt, each sample tube was accessioned and assigned a unique Process ID. DNA extraction followed Ivanova et al. (2008) with minor modifications. Two hundred seventy microlitre of ILB (700 mM GuSCN, 30 mM EDTA pH 8.0, 30 mM Tris–HCl pH 8.0, 0.5% Triton® X-100, 5% Tween-20) with 30 μl Proteinase K (20 mg/ml) was added to each collection tube before it was gently rotated to wash spores off the tube walls and lid, and the tube was then centrifuged at 11,000 g for 5 s. The resultant pellet was re-suspended by gentle pipetting, and the entire volume was transferred to a Lysing Matrix A tube (MP-BIO). Tissue was ground in a TissueLyser (Qiagen) for 2 min at 28 Hz. Samples were then incubated for 1 h at 56°C, followed by 1 h at 65°C. Lysates were transferred to a MN block containing 600 μl of 5M GuSCN Binding Buffer (5 M GuSCN, 16.66 mM EDTA pH 8.0, 8.33 mM Tris–HCl pH 6.4, 3.33% Triton® X-100), and the entire volume was transferred in two equal aliquots of 350 μl (each followed by centrifugation at 5,000 xg) onto AcroPrep 96 Filter Plates with 3.0 μm glass fiber media/0.2 μm Bio-Inert membrane, followed by two washes with WB buffer (60% ethanol, 50 mM NaCl, 10 mM Tris–HCl pH 7.4, 0.5 mM EDTA pH 8.0). DNA was eluted in 45 μl of 10 mM Tris-HCl pH 8.0.
Synthetic Positive Control
We applied a synthetic positive control approach (also called spiking approach), with the aim of translating the raw sequence counts into more quantitative estimates of DNA amount. The nine positive control plasmids were prepared from synthetic sequences that are generally consistent with fungal ITS sequences, yet different from all known natural sequences (Palmer et al., 2018). These contained ITS3 (GCATCGATGAAGAACGCAGC) and ITS4 (TCCTCCGCTTATTGATATGC) priming sites (White et al., 1990), and were synthetized as gBlocks at Integrated DNA Technologies (IDT). PCR products were amplified using Platinum Taq and cloned into TOPO4 vector using TOPO™ TA Cloning™ Kit for Sequencing, with One Shot™ TOP10 Chemically Competent Escherichia coli (Invitrogen) following manufacturer's instructions. Resulting clones were validated by Sanger sequencing, and each selected clone containing the desired insert was grown in 100 ml of liquid LB media with 150 μg/ml ampicillin. Plasmid DNA was extracted as described using standard protocols (Sambrook et al., 1989) with minor modifications. Plasmid DNA concentration was normalized using BR Qubit dsDNA kit and qPCR, resulting in a pool containing all nine plasmids at 0.01 ng/μl.
Estimating DNA Concentration With qPCR
As an alternative approach to the synthetic positive controls, we also estimated the DNA concentration on each sample using qPCR with a serial dilution of AMPtk plasmids. The target genetic marker ITS2 rDNA for fungi and plants was amplified using the Polymerase Chain Reaction (PCR) for 45 cycles on LightCycler96 (Roche). Each of the 12 μl reactions contained 6 μl of FastStart Essential DNA Green Master (Roche), 1.2 μl of each 10 μM primers (forward primer consisted of a cocktail of ITS_S2F and ITS3 mixed 1:1, ITS4 was used as single reverse primer), 1.6 μl of ddH2O and 2 μl of genomic DNA. Standard curve DNA dilutions for 0.01 ng/μl, 0.001 ng/μl, 0.0001 ng/μ were generated in three replicates using AMPtk plasmids DNA. The thermocycling profile consisted of denaturation at 95°C for 10 min; 55 cycles of 95°C for 10 s, 51°C for 10 s, 72°C−40 s; melting: 95°C for 10 s, 65°C for 60 s, 97°C−1 s. Absolute quantification analysis was performed in LightCycler96 software v1.1.
Next Generation Sequencing Workflow
CCDB PCR Master Mix with Platinum Taq was prepared as described in Hebert et al. (2013). The total reaction volume was 12.5 μl and contained 10.5 μl of MMix and 2 μl of DNA template. The positive synthetic control (0.01 ng/ μl) containing nine plasmids was spiked into the PCR mastermix at a 1:100 ratio. The target genetic marker ITS2 rDNA was amplified using the Polymerase Chain Reaction (PCR) for 20 cycles with fusion primers ITS_S2F (Chen et al., 2010), ITS3 and ITS4 (White et al., 1990) tailed with Illumina adapters:
ITS_S2F-mis
TCGTCGGCAGCGTCAGATGTGTATAAGAGACAGATGCGATACTTGGTGTGAAT
ITS3-mis
TCGTCGGCAGCGTCAGATGTGTATAAGAGACAGGCATCGATGAAGAACGCAGC
ITS4-mis
GTCTCGTGGGCTCGGAGATGTGTATAAGAGACAGTCCTCCGCTTATTGATATGC
PCR products were diluted 2x, and a volume of 2 μl of the diluted product was used in second round PCR amplification with Index 1 and Index 2 fusion primers containing i5 and i7 Nextera indices for dual-indexed PCR: https://support.illumina.com/content/dam/illumina-support/documents/documentation/chemistry_documentation/experiment-design/illumina-adapter-sequences-1000000002694-11.pdf.
The thermocycling for Platinum Taq PCR1 consisted of initial denaturation at 94°C for 2 min followed by 20 cycles of: denaturation at 94°C−1 min; annealing at 51°C– 1 min; extension at 72°C for 1 min; final extension at 72°C for 5 min. PCR2 with indexed Illumina primers consisted of 30 cycles of initial denaturation at 94°C for 2 min followed by 20 cycles of: denaturation at 94°C−1 min; annealing at 60°C– 1 min; extension at 72°C for 1 min; final extension at 72°C for 5 min. DNA template and indexed primer transfers were done on a Biomek FXP robot.
The library was pooled without normalization and purified using Ampure beads (Agencourt, Beckman Coulter) with 0.8:1 beads/PCR product ratio and sequenced on Illumina MiSeq following manufacturer's instructions with 25% spike of PhiX.
Bioinformatics Pipeline
Raw Illumina data were paired using Geneious Prime 2019.0.4, short sequences (<100 bp) were discarded and 5′-end and 3′-end were trimmed by quality (QV20) using BBDuk. The following bioinformatics workflow was used to process paired-end data: Cutadapt (v1.8.1) was used to trim primer sequences; Sickle (v1.33) was used for filtering (<200 bp) and Uclust (v1.2.22q) was used to cluster OTUs with 98% similarity threshold and minimum coverage 2.
We denote the total number of sequences obtained for sample i by ni and henceforth refer to it as the sequencing depth. Only those samples for which the sequencing depth was at least 10,000 were included. Clusters that corresponded to the spikes were identified using Ublast with 95% similarity threshold. We denote by sij the number of sequences that were assigned to the spike j (with j = 1, …, 11), by the total number of spike sequences in sample i, and by mi = ni−si the number of sequences not assigned to the spikes. The amount of fungal DNA in each sample was estimated by wi = mi/si, with the caveat that some mi sequences may not represent fungal DNA. With this definition, wi measures the amount of fungal DNA in units relative to the spike (presuming no inhibition or competition for PCR reagents). As the total input of spike DNA was ca. 0.001 ng (1/100 volume of 0.01 ng/μl (10.4 μl) spiked into 1,040 μl PCR mix before adding DNA), e.g., wi = 24 would theoretically correspond to a sample containing 0.024 ng of DNA in 2 μl of template DNA added to the reaction, indicating a DNA concentration of 0.012 ng/μl. During DNA extraction 78% of lysate was used for binding so total DNA yield can be calculated as 0.012 ng/μl *45 μl (elution volume), resulting in 0.54 ng in the 78% of the used lysate or 0.69 ng in the total initial sample. As the cyclone sampler processes 24 m3 of air in 24 h, the value of wi = 24 would theoretically correspond to 0.029 ng of fungal DNA per cubic meter of air. However, we note that this calculation assumes that all DNA amplifies equally across spikes and species, whereas many factors may cause variation in it (Polz and Cavanaugh, 1998; Sipos et al., 2007; Berry et al., 2011; Kennedy et al., 2014).
For the mi sequences that were not assigned to the spikes and thus represented DNA, a probabilistic taxonomic placement was performed with PROTAX (Somervuo et al., 2017). The specific implementation of PROTAX to fungal identification (Abarenkov et al., 2018) is based on a taxonomy database that includes ca. 130,000 species, and a reference database with about 420,000 reference sequences that represent 22,300 species. We recorded for each query sequence the most likely taxonomic identity at the levels of phylum, class, order, family, genus, and species, and the uncertainty in these assignments as measured by probabilistic placement. We note that the uncertainty estimates of PROTAX account for the possibility that the species might be unknown to science (i.e., not included in the taxonomy database), or known to science but lacking reference sequences (Somervuo et al., 2017; Abarenkov et al., 2018). We followed Somervuo et al. (2017) in treating an identification as plausible if the probability of taxonomic placement was >50%, and reliable if the probability of taxonomic placement was >90%.
Statistical Analyses of the Pilot Data
The statistical analyses were aimed at testing the feasibility of the method in surveying local species communities. Given the lack of controlled mock-community data, the assessment on the reliability of the GSSP pipeline is based on the consistency of observed patterns, especially on the comparison of samples from the five sampling locations.
First, to examine how consistently different spikes were captured among the samples, we defined the relative spike proportion as ŝij = sij/si. We performed an analysis of variance to examine how much of the variation among the relative spike proportions was explained by spike identity j.
Second, to validate the accuracy of the sequence-based estimate of fungal DNA amount (wi), we compared it to the qPCR-based estimate of fungal DNA amount (see above) with the help of linear regression. To identify variation in DNA amount over orders of magnitude, we log10 transformed both the sequence- and the qPCR-based estimates.
Third, we used generalized linear models to examine variation in the estimated amount of DNA (wi) and OTU richness among the samples. For amount of DNA, we fitted a linear model where the response variable was log10 wi. For OTU richness, we fitted a negative binomial model. In both models, the explanatory variables were the sampling location, the presence of insects in the sample, the presence of water in the sample, and the log10 transformed number of sequences, i.e., the sequencing depth. While the samples were collected over several months and thus contain seasonal variation, we expected that most of the variation would be explained by the sampling location and that the three European sampling locations would yield similar amounts of DNA and OTU richness compared to the Greenland and Australian samples. We further expected that the treatment of the samples due to insects or water might influence the amount of fungal DNA and consequently OTU richness. As we normalized the samples by the spiking approach, we did not expect sequencing depth to have an influence on the estimated amount of DNA, but we expected that OTU richness may increase with sequencing depth.
Fourth, to examine how reliably the sequences could be identified, we computed the proportion of sequences that could be either plausibly or reliably identified for each taxonomic level and each sampling location. To examine how much diversity the samples contained, we computed for each taxonomic level and each sampling location the numbers of distinct OTUs that could be plausibly or reliably identified.
Fifth, to examine how community composition varied among the five locations, we employed a non-metric multidimensional scaling. For the community data we used gij, defined as the number of sequences in sample i that were plausibly (with probability >50%) identified to the genus j. To account for sequencing depth and the variation that covered many orders of magnitude, we transformed the data as yij = log((gij+1)/(ni+1)). The non-metric multidimensional scaling was performed using Euclidian distance by the sammon function of the MASS R-package with the default settings as sammon(dist(y)). We further used the anosim function to test whether the five locations separated in the ordination space. We note that the NMDS analyses are aimed primarily for illustrating the data, and that we plan to apply more rigorous model-based analyses (see e.g., Gloor et al., 2017; Ovaskainen et al., 2017) after we have obtained data from all localities.
Results
Among the 75 samples, 73 yielded >10,000 sequences with a mean of 52,000 sequences (standard deviation = 10,000; range = 25,000–67,000). The results below are based on the 3.8 million sequences represented by the 73 samples, whereas the two samples with <10,000 sequences are excluded.
Does the Use of Spikes Allow Accurate Estimation of DNA Amount?
Different spikes generated very different sequence proportions, ranging from ca. 0.05 to 0.20 (Figure 2A). These proportions were found to be stable since in the linear model spike identity explained R2 = 0.88 (p < 0.001, df = 8, df-residual = 648, F = 646) of the variance among the proportions (reflected by the clear separation of the boxes in Figure 2A). The use of the spikes allowed very accurate estimation of the amount of fungal DNA, shown by the fact that the sequence-based estimate correlated very closely with the qPCR-based estimate (Figure 2B). The proportion of fungal DNA sequences (i.e., non-spike sequences) varied greatly among the samples: the median proportion was 24.9%, the minimum proportion 0.1%, and the maximum proportion 99.7%. Consequently, the estimated amount of DNA varied by five orders of magnitude among the samples, ranging from 0.001 to 264 units of spike DNA, corresponding to from 1.2e-6 to 0.3 ng of fungal DNA per cubic meter of air.
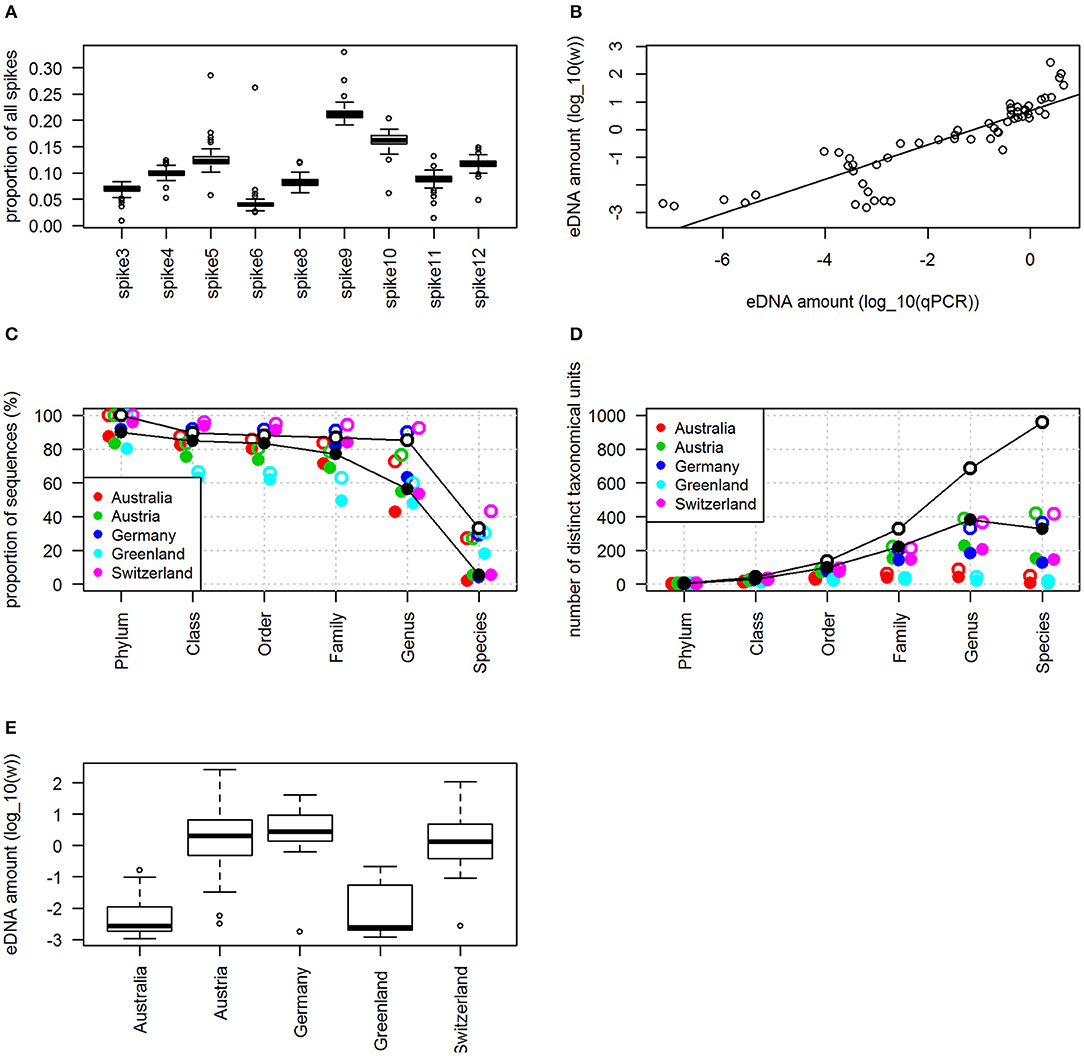
Figure 2. Exploration of the pilot data. (A) Shows the relative spike proportions ŝij. (B) Compares the amount of DNA as measured by qPCR (x-axis) to the sequencing-based measure w (y-axis), both log10-transformed (R2 of linear regression 0.79). (C) Shows the proportion of samples and (D) the number of distinct taxa that could be identified at each taxonomic level. In (C,D) open circles refer to plausibly identified taxa (probability of correct taxonomic placement >50%), whereas closed dots refer to reliably identified taxa (probability of correct taxonomic placement >90%). In (C,D) black circles represent pooled samples, and other colors to locations-specific samples. (E) Shows the amount of DNA, measured by log10 wi, with samples sorted by location.
How Much Diversity Was Detected in the Samples?
The taxonomic placement was generally successful up to the family level, as 75% of the sequences were reliably, and 85% were plausibly, identified (Figure 2C). As expected, due to the incompleteness of the reference databases (Abarenkov et al., 2018), a large proportion of the sequences remained unidentified at the genus and especially species levels. The samples yielded considerable taxonomic diversity, totalling about 1,000 species that could be plausibly identified (Figure 2D). The true diversity is likely much higher as many sequences remained unidentified at the species level and thus were not included in this estimate.
4088 OTU sequences representing 130,309 sequences (3.5% of all sequences) were assigned to unknown phyla in fungal classification. The best BLAST hits of these sequences against Genbank suggest that 66% of them belong to Viridiplantae, 18% to Fungi, 11% to Metazoa, 3% to Oomycetes, and 1% to Alveolata.
How Did Fungal Communities Vary Among the Sampling Locations?
The samples from the three European locations had consistently high levels of fungal DNA, whereas those from Australia and Greenland did not (Figure 2E). Variation in DNA amount was not explained by the presence of water (p = 0.50), insects (p = 0.49), or sequencing depth (p = 0.19), but location had a major effect (p < 0.001, R2 = 0.56, df = 4, df-residual = 68, F = 22). Similarly, variation in OTU richness was not explained by the presence of water (p = 0.08), insects (p = 0.69), or sequencing depth (p = 0.99), but location had a major effect (p < 0.001, df = 4, df-residual = 68, null deviance = 161, residual deviance = 87). The NMDS suggested consistent variation in fungal community composition, as the five locations separated in the ordination space (anosim, p < 0.001), including the three European samples from neighboring countries (Figure 3). The Australian and Greenland samples were close in the NMDS plot because we chose to use a Euclidean distance metric and samples from both of these locations contained little DNA and low species diversity. The NMDS further suggested that the removal of insects did not influence the fungal communities e.g., due to introducing contamination, as the samples that contained insects did not deviate systematically from those that lacked them (Figure 3).
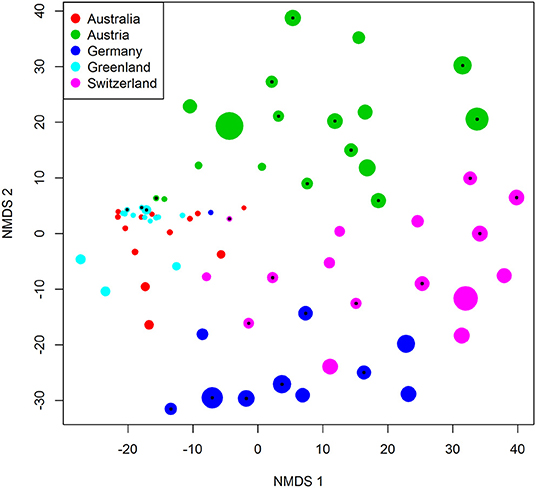
Figure 3. NMDS ordination of the pilot data. Each dot corresponds to one sample. The color indicates sampling location, the size of the sample corresponds to the amount of DNA (measured by wi), while the black dots mark samples that contained an insect. The final stress achieved in the NMDS was 0.061.
In terms of taxonomic composition, all samples were dominated by Ascomycota, but they also contained a substantial proportion of Basidiomycota, and the Greenland samples also Zygomygota (Figure 4). We note that samples from all five locations consistently contained a high proportion of the genus Cladosporium.
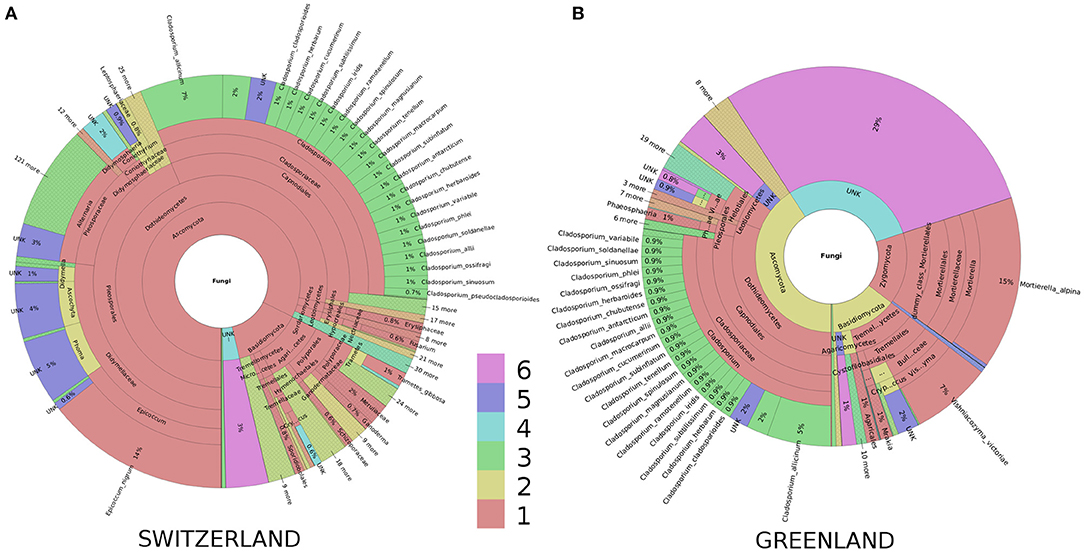
Figure 4. Krona-wheels illustrating taxonomic distributions in the samples. Shown are the results for pooled samples from Switzerland (A) and pooled samples from Greenland (B). Similar Krona-wheels for all five sampling locations (that can be interactively explored by the user e.g., to zoom to certain taxa) are given in Supplementary Information. The colors show the type and confidence level of each taxonomical placement. Colors 1–3 correspond to well-identified taxonomic units for which the proportion of reliable identifications is in the range [50%…100%] (Color 1), (0%…50%) (Color 2), or 0% (Color 3). Colors 4–6 correspond to unknown taxonomic units for which the proportion of reliable identifications is in the range [50%…100%] (Color 4), (0%…50%) (Color 5), or 0% (Color 6).
Discussion
The results of the present pilot study are encouraging in three ways. First, DNA was obtained from nearly all samples, and sequence characterization recovered a substantial taxonomic diversity despite the small number of samples. Second, we could quantify DNA concentrations consistently by two methods, even if DNA amount was generally low and varied over five orders of magnitude. Third, the results showed a strong biological signal as samples were consistent at each site, suggesting low levels of contamination or other problems related to the workflow. Community composition was well separated over space among the three neighboring countries of Europe. This result is consistent with an earlier study (Abrego et al., 2018), which showed that air samples represent the regional diversity of fungi procuding airborne spores at some tens of kilometers around the sampling site. However, the more precise spatial scale represented by the samples needs to be determined by further studies, such as sampling at different distances from known point sources.
The success of the pilot study suggests that the GSSP project has great promise for improving knowledge of fungal diversity and distribution at a global scale, with the caveat that GSSP is limited to those fungi only that can sampled from the air. Importantly, the results are quantitative in the sense that one can ask how many times more common (in terms of the number of ITS sequences) a species is in a sample from a particular location and time than in samples from another location and time. This will allow incorporating abundance information in many kinds of analyses. Since spores may disperse over very long distances, the presence of the species in a sample does not necessarily mean that it is part of the local fungal community. Thus, if using the data to construct “species lists,” it will be important to separate local spore sources from long-distance dispersal. This can be done by accounting for sequence abundance, and in particular repeated occurrence of species at the same location. Conversely, examining the amount and origin of DNA that does not originate from the local community (e.g., samples acquired during winter in the arctic regions) will allow quantitative estimation of long-distance dispersal in fungi under current air circulation patterns.
The abundance of Cladosporium species at all sampling locations raises the possibility of contamination (Czurda et al., 2016). What speaks against this is that the total amount of DNA varied by five orders of magnitude among the samples, while the proportion of Cladosporium remained relatively stable. If its presence is due to contamination, one would expect the Greenland and Australian samples (with little DNA) to be dominated by it, whereas the other samples with much DNA would be less influenced. However, no such pattern was observed. Cladosporium spores are wind-dispersed and they are known to be very abundant in outdoor air (Harvey, 1967; Kurkela, 1997), and thus we consider their consistent presence in our samples biologically plausible.
To conclude, our pilot study suggests that the GSSP will contribute a fungal dimension to the increasing number of global trapping efforts combined with DNA methods, such as the Global Malaise Trap Program (https://biodiversitygenomics.net/projects/gmp/) or the African Soil Microbiology Project (Wild, 2016). Within the fungal realm, the program will add an important airborne perspective to ongoing perspectives focused on soil (Tedersoo et al., 2014; Bahram et al., 2018) and water (http://pk.emu.ee/en/structure/hydrobiologyandfishery/research/projects/international-projects/funaqua/). While we have focused here on fungal diversity, samples collected by the GSSP network can potentially also be utilized for other taxonomic groups such as plants where the same sampling method has been succesfully applied (Brennan et al., 2019). The GSSP network is open to new sampling teams, and thus we encourage new members to join (https://www.helsinki.fi/en/projects/lifeplan), especially from locations that are currently poorly covered.
Data Availability Statement
Raw sequence data were deposited into ENA, accession PRJEB33255 (https://www.ebi.ac.uk/ena/browser/view/PRJEB33255).
Author Contributions
OO, NA, PS, BH, and TR initiated the GSSP network. NRA, PN, NS, SS, and JV collected the data. IP, BH, and J-MP acted as project coordinators, and IP processed the samples in Helsinki. EZ and NI conducted DNA extraction, sequencing, and bioinformatics analyses. PS and OO conducted the statistical analyses. OO, NA, IP, and NI wrote the first draft of the manuscript. All authors contributed substantially to the revisions.
Funding
The research was funded by the Academy of Finland (Grant 309581 to OO, and postdoctoral Grant 308651 to NA), the Jane and Aatos Erkko Foundation, and the Research Council of Norway (SFF-III Grant 223257), the Australian Discovery Grant DP160101561 to NRA.
Conflict of Interest
The authors declare that the research was conducted in the absence of any commercial or financial relationships that could be construed as a potential conflict of interest.
Acknowledgments
We thank Victoria Eisenbach for acquiring the samples from Hainich, and Arusyak Abrahamyan for preparing plasmids for the synthetic positive control.
Supplementary Material
The Supplementary Material for this article can be found online at: https://www.frontiersin.org/articles/10.3389/fevo.2019.00511/full#supplementary-material
References
Abarenkov, K., Somervuo, P., Nilsson, H., Kirk, P., Huotari, T., Abrego, N., et al. (2018). PROTAX-fungi: a web-based tool for probabilistic taxonomic placement of fungal ITS sequences. N. Phytol. 220, 517–525. doi: 10.1111/nph.15301
Abrego, N., Norros, V., Halme, P., Somervuo, P., Ali-Kovero, A., and Ovaskainen, O. (2018). Give me a sample of air and I will tell which species are found from your region: molecular identification of fungi from airborne spore samples. Mol. Ecol. Resour. 18, 511–524. doi: 10.1111/1755-0998.12755
Almeida, F., Rodrigues, M. L., and Coelho, C. (2019). The still underestimated problem of fungal diseases worldwide. Front. Microbiol. 10:214. doi: 10.3389/fmicb.2019.00214
Bahram, M., Hildebrand, F., Forslund, S. K., Anderson, J. L., Soudzilovskaia, N. A., Bodegom, P. M., et al. (2018). Structure and function of the global topsoil microbiome. Nature 560, 233–237. doi: 10.1038/s41586-018-0386-6
Baldrian, P., and Valášková, V. (2008). Degradation of cellulose by basidiomycetous fungi. FEMS Microbiol. Rev. 32, 501–521. doi: 10.1111/j.1574-6976.2008.00106.x
Barberán, A., Ladau, J., Leff, J. W., Pollard, K. S., Menninger, H. L., Dunn, R. R., et al. (2015). Continental-scale distributions of dust-associated bacteria and fungi. Proc. Natl. Acad. Sci. U.S.A. 112, 5756–5761. doi: 10.1073/pnas.1420815112
Berry, D., Ben Mahfoudh, K., Wagner, M., and Loy, A. (2011). Barcoded primers used in multiplex amplicon pyrosequencing bias amplification. Appl. Environ. Microbiol. 77, 7846–7849. doi: 10.1128/AEM.05220-11
Brennan, G. L., Potter, C., de Vere, N., Griffith, G. W., Skjøth, C. A., Osborne, N. J., et al. (2019). Temperate airborne grass pollen defined by spatio-temporal shifts in community composition. Nat. Ecol. Evol. 3, 750–754. doi: 10.1038/s41559-019-0849-7
Chen, S., Yao, H., Han, J., Liu, C., Song, J., Shi, L., et al. (2010). Validation of the ITS2 region as a novel DNA barcode for identifying medicinal plant species. PLoS ONE 5:e8613. doi: 10.1371/journal.pone.0008613
Czurda, S., Smelik, S., Preuner-Stix, S., Nogueira, F., and Lion, T. (2016). Occurrence of fungal DNA contamination in PCR reagents: approaches to control and decontamination. J. Clin. Microbiol. 54, 148–152. doi: 10.1128/JCM.02112-15
Davison, J., Moora, M., Öpik, M., Adholeya, A., Ainsaar, L., Bâ, A., et al. (2015). Global assessment of arbuscular mycorrhizal fungus diversity reveals very low endemism. Science 349, 970–973. doi: 10.1126/science.aab1161
Emberlin, J. C., and Baboonian, C. (1995). “The development of a new method of sampling airborne particles for immunological analysis,” in: 16th European Congress of Allergology and Clinical Immunology (Bologna: Monduzzi Editore S.p.A, 39–43.
Gloor, G. B., Macklaim, J. M., Pawlowsky-Glahn, V., and Egozcue, J. J. (2017). Microbiome datasets are compositional: and this is not optional. Front. Microbiol. 15:2224. doi: 10.3389/fmicb.2017.02224
Harvey, R. (1967). Air-spora studies at Cardiff: I. Cladosporium. Transact. Br. Mycol. Soc. 50, 479–495. doi: 10.1016/S0007-1536(67)80017-2
Hawksworth, D., and Lücking, R. (2017). “Fungal Diversity Revisited: 2.2 to 3.8 Million Species,” in The Fungal Kingdom, eds J. Heitman, B. J. Howlett, P. W. Crous, E. H. Stukenbrock, T. Y. James, and N. A. R. Gow (Washington, DC: ASM Press, 79–95.
Hazard, C., Gosling, P., van der Gast, C. J., Mitchell, D. T., Doohan, F. M., and Bending, G. D. (2012). The role of local environment and geographical distance in determining community composition of arbuscular mycorrhizal fungi at the landscape scale. ISME J. 7, 498–508. doi: 10.1038/ismej.2012.127
Hebert, P. D. N., deWaard, J. R., Zakharov, E. V., Prosser, S. W. J., Sones, J. E., McKeown, J. T. A., et al. (2013). A DNA “barcode blitz”: rapid digitization and sequencing of a natural history collection. PLoS ONE 8:e68535. doi: 10.1371/journal.pone.0068535
Ivanova, N. V., Fazekas, A. J., and Hebert, P. D. N. (2008). Semi-automated, membrane-based protocol for DNA isolation from plants. Plant Mol. Biol. Report. 26:186. doi: 10.1007/s11105-008-0029-4
Kennedy, K., Hall, M. W., Lynch, M. D. J., Moreno-Hagelsieb, G., and Neufeld, J. D. (2014). Evaluating bias of illumina-based bacterial 16S rRNA gene profiles. Appl. Environ. Microbiol. 80, 5717–5722. doi: 10.1128/AEM.01451-14
Kubartová, A., Ottosson, E., Dahlberg, A., and Stenlid, J. (2012). Patterns of fungal communities among and within decaying logs, revealed by 454 sequencing. Mol. Ecol. 21, 4514–4532. doi: 10.1111/j.1365-294X.2012.05723.x
Kurkela, T. (1997). The number of Cladosporium conidia in the air in different weather conditions. Grana 36, 54–61. doi: 10.1080/00173139709362591
Ovaskainen, O., Tikhonov, G., Norberg, A., Blanchet, F. G., Duan, L., Dunson, D., et al. (2017). How to make more out of community data? A conceptual framework and its implementation as models and software. Ecol. Lett. 20, 561–576. doi: 10.1111/ele.12757
Palmer, J. M., Jusino, M. A., Banik, M. T., and Lindner, D. L. (2018). Non-biological synthetic spike-in controls and the AMPtk software pipeline improve mycobiome data. PeerJ. 6:e4925. doi: 10.7717/peerj.4925
Polz, M. F., and Cavanaugh, C. M. (1998). Bias in template-to-product ratios in multitemplate PCR. Appl. Environ. Microbiol. 64, 3724–3730. doi: 10.1128/AEM.64.10.3724-3730.1998
Sambrook, J., Fritsch, E. F., and Maniatis, T. (1989). Molecular Cloning: A Laboratory Manual. 2nd ed. New York, NY: Cold Spring Harbor Laboratory Press.
Sato, H., Tsujino, R., Kurita, K., Yokoyama, K., and Agata, K. (2012). Modelling the global distribution of fungal species: new insights into microbial cosmopolitanism. Mol. Ecol. 21, 5599–5612. doi: 10.1111/mec.12053
Sipos, R., Székely, A. J., Palatinszky, M., Révész, S., Márialigeti, K., and Nikolausz, M. (2007). Effect of primer mismatch, annealing temperature and PCR cycle number on 16S rRNA gene-targetting bacterial community analysis. FEMS Microbiol. Ecol. 60, 341–350. doi: 10.1111/j.1574-6941.2007.00283.x
Somervuo, P., Yu, D., Xu, C., Ji, Y., Hultman, J., Wirta, H., et al. (2017). Quantifying uncertainty of taxonomic placement in DNA barcoding and metabarcoding. Methods Ecol. Evol. 8, 398–407 doi: 10.1111/2041-210X.12721
Tedersoo, L., Bahram, M., Põlme, S., Kõljalg, U., Yorou, N. S., Wijesundera, R., et al. (2014). Global diversity and geography of soil fungi. Science 346:1256688. doi: 10.1126/science.1256688
Wang, B., and Qiu, Y. L. (2006). Phylogenetic distribution and evolution of mycorrhizas in land plants. Mycorrhiza 16, 299–363. doi: 10.1007/s00572-005-0033-6
White, T. J., Bruns, T. D., Lee, S., and Taylor, J. (1990). “Amplification and direct sequencing of fungal ribosomal RNA genes for phylogenetics,” in PCR Protocols: A Guide to Methods and Applications, eds M. A. Innis, D. H. Gelfaud, J. J. Sninsky, and T. J. White (London: Academic Press), 315–322. doi: 10.1016/B978-0-12-372180-8.50042-1
Keywords: biomonitoring, cyclone sampler, environmental DNA, fungi, global diversity
Citation: Ovaskainen O, Abrego N, Somervuo P, Palorinne I, Hardwick B, Pitkänen J-M, Andrew NR, Niklaus PA, Schmidt NM, Seibold S, Vogt J, Zakharov EV, Hebert PDN, Roslin T and Ivanova NV (2020) Monitoring Fungal Communities With the Global Spore Sampling Project. Front. Ecol. Evol. 7:511. doi: 10.3389/fevo.2019.00511
Received: 10 July 2019; Accepted: 17 December 2019;
Published: 14 January 2020.
Edited by:
Dominique Gravel, Université de Sherbrooke, CanadaReviewed by:
Primrose Boynton, Max Planck Institute for Evolutionary Biology, GermanyBrian John Pickles, University of Reading, United Kingdom
Copyright © 2020 Ovaskainen, Abrego, Somervuo, Palorinne, Hardwick, Pitkänen, Andrew, Niklaus, Schmidt, Seibold, Vogt, Zakharov, Hebert, Roslin and Ivanova. This is an open-access article distributed under the terms of the Creative Commons Attribution License (CC BY). The use, distribution or reproduction in other forums is permitted, provided the original author(s) and the copyright owner(s) are credited and that the original publication in this journal is cited, in accordance with accepted academic practice. No use, distribution or reproduction is permitted which does not comply with these terms.
*Correspondence: Otso Ovaskainen, b3Rzby5vdmFza2FpbmVuQGhlbHNpbmtpLmZp
†ORCID: Nigel R. Andrew orcid.org/0000-0002-2850-2307