- 1Department of Aquatic Ecology, Netherlands Institute of Ecology (NIOO-KNAW), Wageningen, Netherlands
- 2Smithsonian Tropical Research Institute, Ancón, Panama
- 3Will Keith Kellogg Biological Station, Michigan State University, Hickory Corners, MI, United States
- 4Program in Ecology, Evolutionary Biology and Behavior, Department of Plant Biology, Michigan State University, East Lansing, MI, United States
- 5Department of Biology, University of Pennsylvania, Philadelphia, PA, United States
- 6Department of Ecology and Evolutionary Biology, University of California, Irvine, Irvine, CA, United States
- 7Department of Ecology and Evolutionary Biology, University of California, Santa Cruz, Santa Cruz, CA, United States
“Ecological stoichiometry,” a framework that focuses explicitly on the balances and flows of chemical elements within and between organisms and ecosystems, has provided crucial insights into many biological patterns and processes. Despite the proliferation of stoichiometrically-focused studies in recent decades and recognition of the potential for rapid evolution of stoichiometric traits, the prevalence of genetic variation in stoichiometric traits within species remains unclear. We compiled data from 30 published common garden studies of a broad range of taxa (including invertebrates, vertebrates, and autotrophs) to examine how genetic variation influences the acquisition, assimilation, allocation (AAA), composition, and excretion of elements. To quantify the extent of genetic variation for a given trait we calculated the absolute mean response ratio from pairwise comparisons of populations within the same common garden (820 population and 708 genotype comparisons). We observed substantial intraspecific variation of stoichiometric traits across populations and among genotypes; however, the magnitude of variation was greater in AAA traits (effect sizes of 20 and 164% for population and genotype contrasts, respectively) and excretion (effect sizes of 52 and 23%) than in content of carbon (2.1 and 3.1%) and nitrogen (4.5 and 24%). These results suggest that the content of some elements may be evolutionarily constrained relative to AAA traits that determine the processing of these elements, and that a sole focus on elemental content would underestimate the importance of intraspecific genetic variation, particularly within populations. Across many trait types the variation was greater among genotypes within a population than across populations. Finally, we compared pairs of populations from environments with different phosphorus (P) availability to pairs of populations with similar P availability. Genetic variation in the traits measured was similar regardless of the P environment from which genotypes were isolated, suggesting that differences in elemental availability across environments do not necessarily drive enhanced trait divergence. Overall, our results highlight the substantial amount of intraspecific variation in stoichiometric traits and underscore the potential importance of intraspecific variation in driving ecological and evolutionary processes.
Introduction
Ecological stoichiometry (ES) is a scientific framework that views living systems as composite chemical reactions which, like all physical processes, are governed by the law of mass balance (Sterner and Elser, 2002; Sterner et al., 2015). In doing so, ES focuses explicitly on the balance and flow of elements and energy within and between organisms and ecosystems. The ES framework has applicability across a wide range of disciplines, from astrobiology to cancer research (Elser, 2003; Elser et al., 2007), and can be applied across multiple levels of biological organization and across taxa. It has also been argued that explicit consideration of an organism's “stoichiometric traits” (i.e., elemental contents and traits that influence the flux of elements between an organism and its environment) is a natural and convenient way to investigate the complex interplay between ecology and evolution (Kay et al., 2005). This is because elements can be traced through space and time, such that genetic variation in stoichiometric traits can be linked mechanistically to variation in environmental elemental availability (Matthews et al., 2011; Leal et al., 2017b).
Despite the apparent potential for stoichiometric traits to link ecology and evolution, there remain some fundamental gaps in our understanding of the genetic basis of variation in stoichiometric traits. In particular, while the composition, acquisition (A), assimilation (A), allocation (A), and excretion (E) of elements (hereafter referred to as “elemental composition” and “AAA,” or “AAAE”) are regarded as the defining traits of the stoichiometric phenotype (Jeyasingh et al., 2014), it remains unclear how and to what extent genetic factors actually contribute to their variation within and between natural populations of conspecific organisms. Heritable trait variation is central to evolutionary processes, and high levels of intraspecific genetic trait variation within populations can be thought of as “fuel” for adaptation (Barrett and Schluter, 2008). At the same time, intraspecific genetic variation in stoichiometric traits has been identified as an important driver of variation in local ecological processes, such as leaf litter decomposition, that can scale up to affect overall ecosystem functioning (Whitham et al., 2006; Silfver et al., 2007). Quantification of the genetic component of intraspecific variation in stoichiometric traits can, therefore, help to identify which traits have the potential for contemporary evolution, and would represent an essential step toward the development of a stoichiometrically-explicit eco-evolutionary framework.
Intraspecific genetic variation in stoichiometric traits might emerge as a direct response to nutritionally-based or other selection pressures (Leal et al., 2017b), or because the stoichiometric traits are mechanistically correlated with other physiological, life history, and morphological traits, as in the well-documented coupling between P content and individual growth rate (the Growth Rate Hypothesis or “GRH”; see Elser et al., 2000a, 2003, 2008). However, stoichiometric traits also exhibit considerable plasticity, i.e., variability in response to environmental parameters (i.e., Townsend et al., 2007; González et al., 2011). For example, plant foliar nutrient content and resorption are often strongly correlated with soil nutrient availability (Schade et al., 2003; Rejmánková, 2005), and the elemental composition of invertebrates can be highly sensitive to the elemental composition of their diets (e.g., Visanuvimol and Bertram, 2011; Zhou et al., 2018). Thus, in many prior studies that have considered intraspecific variation in stoichiometric traits, the respective genetic and environmental drivers of intraspecific variation have likely been confounded. However, as the field of ES has matured, an increasing number of studies have conducted “common garden experiments” that can be used to assess the magnitude of intraspecific genetic variation in stoichiometric traits. These experiments minimize the effects of the environmental factors by comparing genetically distinct individuals within a common “garden” environment, thus allowing for the quantification of genetic variation either within or between populations (Lynch and Walsh, 1998). Synthesis of such studies could illuminate whether genetic variation is present, the relative amount of genetic variation within and across populations, which stoichiometric traits show genetic variation, and whether elemental limitation could influence evolution in nature (Elser, 2006; Leal et al., 2017a; Rudman et al., 2019).
To determine the amount of intraspecific genetic variation in ES traits, we conducted a meta-analysis of studies that have measured ES traits on individuals reared in common gardens. These studies typically fall into two broad categories: those that compare ES traits between pairs of populations, and studies comparing ES traits of different genotypes from within a single population. From these studies we collected data on intraspecific genetic variation in ES traits, which we defined as measures of elemental content or movement, and life history data (specific growth rate, size/mass). We used these data to answer four questions about the extent of genetic variation in ES traits. (1) How much intraspecific genetic variation is present in ES traits relative to key life history traits? Here we used somatic growth rate and morphological characteristics to represent two key traits that are not explicitly stoichiometric, but have often been measured concurrently with ES traits. We predicted similar levels of genetic variation in life history traits and ES traits because all traits, at some level, have an elemental basis. (2) Do particular classes of ES traits show more genetic trait variation than others? We predicted that elemental composition would show reduced variation relative to AAAE traits, as there are well-documented constraints in elemental composition that are maintained by stoichiometric homeostasis (Sterner and Elser, 2002). (3) Is the magnitude of intraspecific variation among genotypes (i.e., within a population) similar to that observed across populations? Individuals from different populations are often reproductively isolated and likely experience a more divergent environment than genotypes from a single population (Schluter, 2000). As such, we predicted a greater magnitude of genetic trait variation between distinct populations than among genotypes from within a population. (4) How does the magnitude of intraspecific genetic variation differ when comparing populations from distinct and similar stoichiometric environments? As resource stoichiometry has been demonstrated to be an important selection pressure (Jeyasingh and Weider, 2007), we predict that populations from distinct stoichiometric environments will show greater genetic variation than populations from similar environments.
Materials and Methods
Data Collection
We collected studies for the meta-analyses by searching the ISI Web of Science (v. 5.32) and Google Scholar databases with “title” or “abstract” search terms “(‘ecological stoichiometry' OR ‘biological stoichiometry') AND (‘intraspecific variation' OR ‘evolution') AND ‘common garden”'. Searches were conducted between the 15–17th of May 2019. These search terms produced 4 results in Web of Science and 197 results in Google Scholar. We screened these 201 studies for suitability based on their titles, abstracts, methods, and results. We then examined the reference lists of the seemingly-appropriate articles for additional studies that did not appear in our search results. We excluded studies that did not include data on ES traits. Thirty studies were eventually selected via this process. Importantly, this was not an exhaustive collation of the studies that might have met our criteria. This is presumably a result of our selection of search terms, which were necessarily broad in order to capture the full range of taxa, study systems, and ES traits throughout the literature without biasing our search toward particular combinations of these study foci. Thus, our collection of studies is perhaps best thought of as a large, reproducible sample of the literature with a bias toward studies conducted within the ES framework. We also note here that we explicitly excluded studies of genetic variation that was created by artificial selection (i.e., the crop and livestock literature) because the amount of genetic variation across treatments in artificial selection experiments will largely be proportional to the amount of standing variation included in the study and the strength and duration of artificial selection.
During study selection, it became clear that studies generally fell into two broad categories. Specifically, some studies reported stoichiometric trait variation within populations (i.e., variation among distinct genotypes), while others reported variation among distinct populations (i.e., variation in population trait means). We assigned each study to one of these categories (Table 1), and the categories were used as fixed effects variables as part of the meta-analysis.
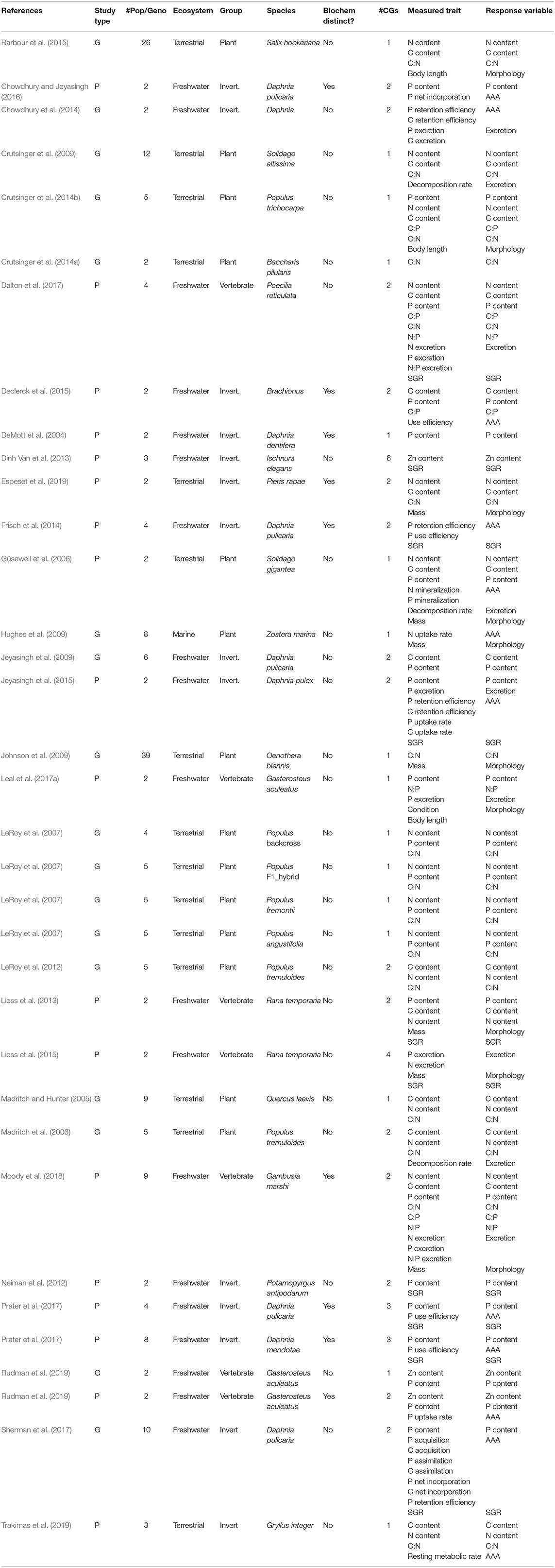
Table 1. A complete list of the studies included in the meta-analysis, with information on study type—either populations (“P”) or genotypes (“G”); the number of populations/genotypes (“# Pop/Geno”), the type of ecosystem considered (Terrestrial, Freshwater or Marine); the coarse taxonomic group of the study organism (Plant, Invertebrate, or Vertebrate); the Latin name of the study organism; for populations, if they were from biogeochemically distinct environments (always “no” for genotypes); the number of common gardens in the experimental setup (“# CGs”); the list of traits that were measured in the study (“Measured Trait”), and; the trait category under which they were grouped in the meta-analysis (“Response Variable,” with “SGR” standing for Somatic Growth Rate).
For each study we recorded details of location, ecosystem type, focal taxa, the purported reasons for differentiation between populations and all measured stoichiometric traits. For a given trait to be included in our analyses, it was necessary that at least two studies reported common garden data about that trait. We considered traits to be explicitly “stoichiometric” if they were part of the elemental phenotype defined by Jeyasingh et al. (2014) as elemental composition, acquisition, assimilation, allocation, and excretion (CAAAE). Many organismal traits, including growth rate and body size, are often closely coupled with CAAAE traits in such ways that could justify their categorization as a “stoichiometric trait” if defined broadly. By adopting a strict definition of stoichiometric trait, we are able to qualitatively compare the intraspecific genetic variation of unambiguously stoichiometric traits with life history traits that do not explicitly involve the movement of elements.
Due to differences in how often CAAAE traits have been measured we found it necessary to subdivide or group certain traits. We considered the content of carbon (C), nitrogen (N) and phosphorus (P) and their ratios as separate traits due to the abundant reporting of elemental composition. Few studies measured acquisition, assimilation, or allocation, therefore we grouped these aspects of the elemental phenotype into a single trait category (AAA) for all elements. We also recorded somatic growth rate and measures of mass and size as “morphological traits” because they were the most commonly measured life history traits for comparison. Studies on invertebrates and vertebrates measured and reported elemental composition of whole organisms, while studies on plants used leaf tissues. In studies on plants, we included data from studies that measured either green or senesced vegetation as elemental content and data on the decomposition rate of plant material, which has modest heritability (Rodriguez-Cabal et al., 2017), was included as excretion (Table 1).
Means, variances, and sample sizes for traits were extracted from repositories, tables, figures, or text. Standard deviation was estimated from SE, 95% confidence intervals or quantiles using standard methods (Higgins and Green, 2008; Bland, 2015). We used DataThief III (Tummers, 2006) to extract data from figures. Where data were not provided in a format suitable for our analyses, we contacted study authors via email to request the relevant data. In cases where study designs featured more than one type of common garden (e.g., Dinh Van et al., 2013), population contrasts within different common gardens were treated as unique observations. For example, if there were two common gardens, and population A and population B were both raised in both common gardens, then there were two population contrasts included in our meta-analysis dataset, one for each common garden.
Effect Size Calculation
For each trait within a study, we compared the difference in genotype/population's trait values by calculating the log response ratios (LRR) for all pairwise combinations of genotypes/populations grown in the same common garden.
Where i is the study, is the genotype/population mean, SDj the standard deviation and nj is the sample size (number of replicates), with j = 1, 2 corresponding to the two genotypes/populations being compared. We choose to use LRR because (i) it is dimensionless, allowing for comparison between studies with different methodologies and units, (ii) it is easily interpretable as it quantifies the proportionate change (Hedges et al., 1999), and (iii) the value of the LRR is not affected by non-independent samples (Noble et al., 2017). We defined nj differently for studies comparing genotypes and those comparing populations. For genotype studies, nj was the number of individuals measured for a given genotype. For population studies, nj was the number of genetically distinct individuals measured for a given population. For example, in a sexually reproducing species nj is the number of individuals measured, but in studies of cyclical parthenogens (e.g., Daphnia) nj is the number of distinct clone lines measured. If it was not clearly indicated that genetically different individuals were included in the study, then nj was recorded as 1.
In the set of studies that compared traits of different genotypes from the same population, we were not always able to use all pairwise comparisons to calculate LRRs. This group of studies often had a larger number of genotypes to be compared than studies comparing distinct populations (maximum number of genotypes compared was 39, and populations was 9), and the use of all pairwise comparisons was too computationally demanding. Instead, if a common garden measured <5 genotypes we calculated the LRR for all pairwise combinations. However, if six or more genotypes were measured (six studies), pairwise comparisons were performed for only five genotypes (min, first quartile, median, third quartile, max). With this method we took a subset of the data to be computationally reasonable and to represent the full range of trait values. We also used a conservative approach and calculated all LRRs within a common garden using the median trait value as the denominator in Equation 1 (see Supplementary Material). Although the magnitude and 95% credibility interval of some mean effect estimates of these two methods differed, it did not change the interpretation of the data. In the studies that compared mean traits between populations, the maximal number of distinct populations was small enough to enable us to compute all possible pairwise comparison, even for the two studies that had more than five distinct populations.
Global Meta-Analysis
We were interested in the magnitude, rather than the direction of, trait differences across populations and among genotypes; therefore, we analyzed the absolute values of LRRs. We used an “analyze and transform” approach derived from Morrissey (2016) to account for the folded-normal distribution of absolute value effect sizes (Hereford et al., 2004; Kingsolver et al., 2012). First, we implemented Bayesian meta-analytic random-effects models in R (R Core Team, 2016) with the MCMCglmm package (Hadfield, 2010). We ran a separate model for each trait with study type as a fixed effect to obtain separate effect sizes for distinct population and genotype studies. Each trait may have a different level of sample error due to measurement techniques and technology. We account for measurement error in our analyses in two ways. First, we weighted the effect sizes by the inverse of the sample variance. Second, we have modeled each trait separately, allowing each trait to have a different error structure. The model structure was:
where yij is the untransformed LRR for the trait from the j-th common garden (cg) which is nested in the i-th study; β is the estimate of the fixed effect; X is a dummy-coded variable representing whether the LRRs were comparing distinct populations or genotypes; studyi represents the random intercept in the i-th study, cgj|i represents the random intercept for the j-th common garden in the i-th study, and eij represents residual error. Effect size estimates were weighted using mij, which accounts for the known sample variance associated with trait yij and the covariance structure of effect sizes through the use of matrices Mij. For each common garden j, the Mij matrix was constructed following Noble et al. (2017) to account for within-common-garden covariance of effect sizes due to pairwise comparisons (i.e., covariation because each population was represented in multiple contrasts). The matrix was created using the function make_VCV_matrix() (Noble, 2019). Every effect is represented by both a row and column in the matrix which has sample error variance on the diagonal and estimates of covariance between effect sizes on the off-diagonals. Covariance was estimated as , where k and l are the row and column ID of the within-common-garden matrix, V is the sampling variance calculated for each effect (“variance LRR” in Equation 2) and r is the correlation between effect size k and l which was assumed to be 0.5 for all off-diagonal elements.
For each model, we used non-informative priors for fixed effects and inverse-Wishart priors for random effects (Hadfield, 2010). We ran the model for 150,000 iterations, discarded the first 30,000 as burn-in and used a thinning interval of 15. We examined plots of Markov chain Monte Carlo to ensure good mixing. We generated posterior predictive distributions for each type of contrast (distinct populations or genotype comparisons) for each trait. These distributions were then transformed using a folded-normal distribution. This transformation allowed us to present the mode and 95% highest posterior density intervals (95% credible intervals) that relate to absolute effect sizes (Morrissey, 2016). When using absolute effect sizes, confidence intervals that do not cross zero are not necessarily indicative of effects that are significantly different than zero (Morrissey, 2016). Thus, we use the absolute effect size magnitude to compare each contrast of our study on the same scale.
Effect of Phosphorus Environment on Population Traits
We performed an analysis on a subset of data to investigate if populations from stoichiometrically distinct environments displayed a greater amount of trait variation than populations that were not identified as stoichiometrically distinct. For our stoichiometrically distinct populations we specifically looked at studies in which it was stated that resource P availability differed between the environments from which the populations originated. We focused on environmental P availability in this subanalysis because P is an essential, non-substitutable elemental resource for all life forms, and because it was the only element for which there were sufficient studies and observations to enable the analysis. Populations were considered to be from environments with similar P availability if there was no mention of nutrient resource stoichiometry in the methods or results. After filtering studies, we had limited data for this analysis, therefore we focused on the three traits which had at least 10 pairwise comparisons: P content, AAA and somatic growth rate. We used the same statistical procedure as in the global meta-analysis to determine mean effect size, now using differences in P availability (binary Yes/No) as a modifier.
Results
From the 30 studies we found, 17 compared populations, 12 compared genotypes and one had both population and genotype comparisons (Table 1). The 18 studies comparing distinct populations had a total of 44 common gardens, with a range of 1–6 common gardens per study. Most of these studies were on invertebrates (n = 11) while comparatively fewer focused on vertebrates (n = 6) and plants (n = 1). The studies were overwhelmingly conducted on freshwater organisms (n = 15), with fewer terrestrial examples (n = 3). Eleven different genera were represented, with Daphnia being the most commonly studied (n = 5). Elemental composition was the ES trait measured most frequently (study n = 15) while excretion (n = 5) and AAA traits (n = 8) were less commonly measured.
The 13 studies that assessed variation among genotypes within a population had a total of 23 common gardens, with a range of 1–4 common gardens per study. Most of the genotype studies were focused on plants (terrestrial n = 8, marine n = 1), and a few focused on freshwater invertebrates (n = 3) and vertebrates (n = 1). There were 8 genera represented, with the most common being Populus (n = 4) and Daphnia (n = 2). Similar to the population studies, the most frequently measured ES traits were elemental composition (n = 12) while excretion and AAA traits were each only measured in three studies.
Among the 30 studies included in our meta-analysis, 21 were based on populations of organisms that were obtained from ecosystems in North America. Of the nine remaining studies, eight were based on populations from north-western continental Europe, and one was based on a single species of freshwater snail from New Zealand. We found no suitable studies of tropical organisms to include in our meta-analyses.
How Much Intraspecific Genetic Variation Is Present in ES Traits Relative to Life History Traits?
The magnitude of genetic variation was large for both life history traits, with effect sizes of 30.9 and 84.4% for morphology and somatic growth rate across populations, and of 32.8 and 146% for the same among genotypes. Most ES traits showed a moderate or small amount of genetic variation (i.e., absolute effect size; Figure 1) relative to life history traits. Differences among genotypes in AAA traits was the only effect size that was larger than life history traits (with an effect size of 164%) and the magnitude of this effect can be partially attributed to a single study that found substantial genotypic effects, particularly for P retention (Sherman et al., 2017).
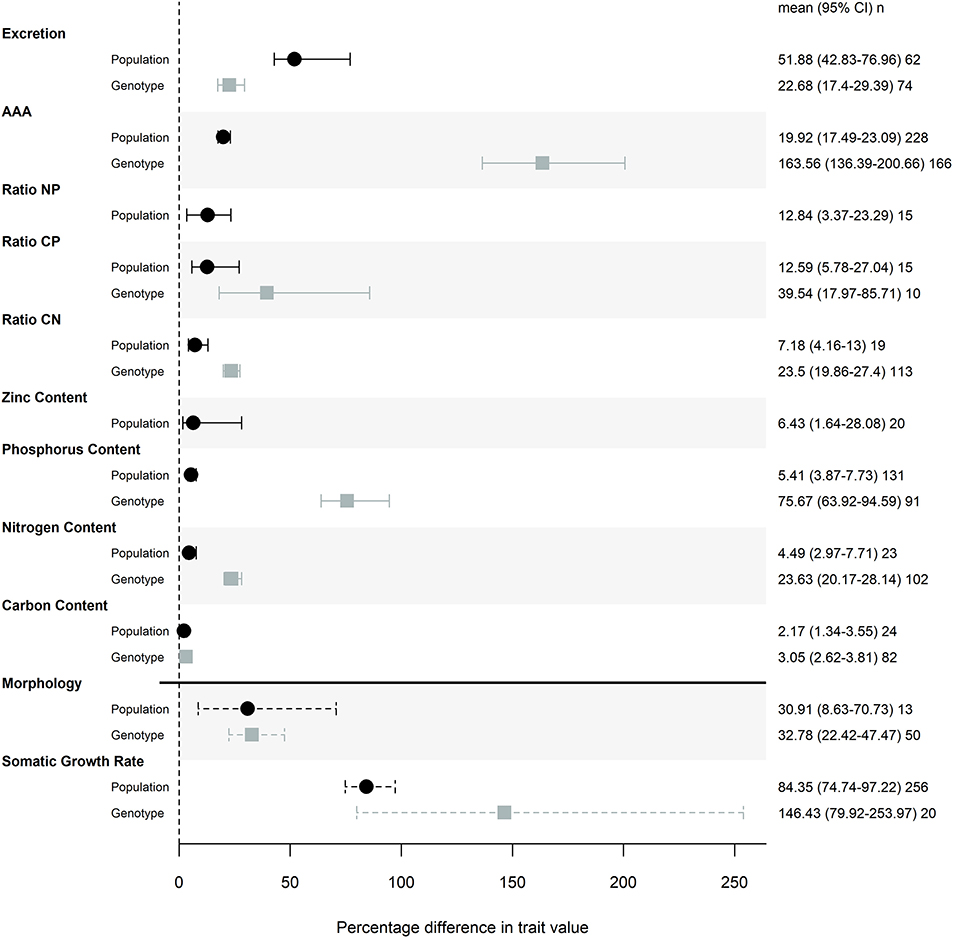
Figure 1. Magnitude of trait differences among pairs of populations (black) or genotypes (gray) grown in the same common garden. Points represent the mode of the posterior distribution and error bars are 95% credible intervals of the effect sizes. Posterior distributions of effects were taken from models that included common garden nested in study as a random effect, and the posterior distributions were transformed with a folded normal distribution to obtain the magnitude of difference between pairs of populations or genotypes. Percentage difference in trait values were calculated by back-transforming the mean effect sizes. n represents the number of pairwise comparisons used to calculate each effect size. Somatic growth rate and morphology (measures of mass and size) effect sizes are denoted with a dashed line to indicate that this meta-analysis is not a comprehensive review of common garden experiments that measured these traits.
Do Particular Classes of ES Traits Show More Trait Variation Than Others?
Of the stoichiometric traits, excretion and AAA traits showed the most variation across populations and among genotypes, while elemental composition varied less (Figure 1). Contents of P, N and C showed decreasing levels of trait variation (Figure 1), with particularly low levels of variation across populations and among genotypes in both N and C. Consequently, nutrient ratios that include P show more trait variation than C:N (Figure 1).
Is the Magnitude of Intraspecific Genetic Variation Similar Among Genotypes (i.e., Within a Population) to What Is Observed Across Populations?
We found a similar magnitude of trait variation among genotypes that came from the same population as we did when we compared distinct populations (Figure 1). For some traits, namely AAA, P content, and N content we saw more trait variation among genotypes than among populations (Table 2). To ensure that this result was not an artifact of statistical methodology we conducted a supplemental analysis. We calculated LRRs for genotype studies using a different, more conservative approach and still found that there was similar or greater variation in traits when comparing genotypes than when comparing populations (Figure S1).
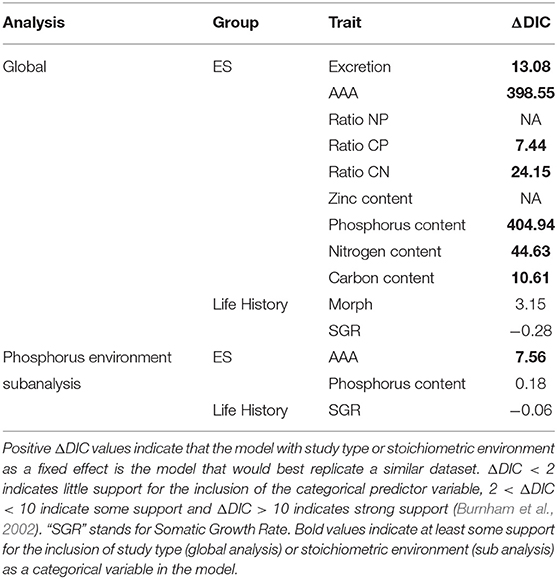
Table 2. Deviance information criteria (DIC) of models for each trait with and without study type (global analysis) or stoichiometric environment (sub analysis) as a categorical variable.
Do Populations From Different Biogeochemical Environments Show More Trait Differentiation?
Our subanalysis included a total of 17 studies; two studies that differed in resource availability, but not specifically P, were removed from this analysis. Seven studies (16 common gardens) used populations originating from environments that differed in P availability. All seven studies focused on freshwater invertebrates, and five used Daphnia spp. Populations that were from environments with different P availability did not show any more trait differentiation than those from populations without any known or measured biochemical differences (Figure 2). Somatic growth rate and P content showed nearly identical differentiation across populations that did and did not come from different P environments (somatic growth rate: 85 and 100% difference, P content: 9.4 and 4.8% difference), while AAA traits varied more in populations that were from similar environments (Table 2; Figure 2).
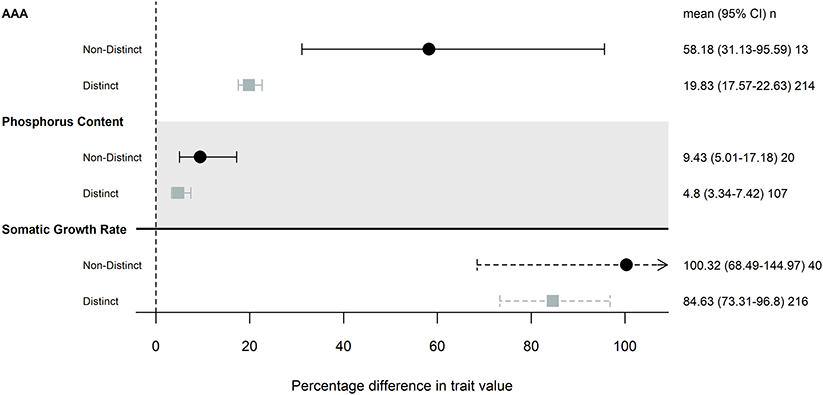
Figure 2. Magnitude of trait differences among pairs of populations that were from environments with distinct levels of P availability (gray) or not (black), that were grown in the same common garden. Only traits that had 10 pairwise comparisons were included in this analysis: somatic growth rate, acquisition, assimilation, and allocation (AAA) and phosphorus content. Points, error bars and line types as in Figure 1.
Discussion
Our meta-analysis of common garden studies uncovered substantial intraspecific genetic variation in most ES traits. However, the magnitude of genetic variation that we observed for ES traits was generally less than what was observed for key life history traits measured in these same studies. Among ES traits, acquisition, assimilation, allocation, and excretion traits tended to vary more than elemental composition traits (i.e., the elemental content and stoichiometric ratios of organism biomass), and there tended to be markedly more variation among genotypes than across populations. Taken together, these results suggest that genetic variation in ES traits, particularly AAAE traits, within and across populations may be important for ecological and evolutionary outcomes.
Evolution and the Magnitude of Genetic Variation in ES and Life History Traits
Our meta-analysis revealed substantial genetic intraspecific variation in ES traits across a range of taxa. These findings join a growing body of literature that has demonstrated that variation below the species level can be both substantial and biologically important (Schweitzer et al., 2004; Crutsinger et al., 2006; Whitham et al., 2006; Post et al., 2008; Violle et al., 2012; Des Roches et al., 2018). In the light of these findings it is increasingly clear that incorporating data on intraspecific variation is likely to increase understanding of ecological patterns in nature (Bolnick et al., 2011). Our comparison of the amount of genetic variation in ES traits and the genetic variation in life history traits demonstrated that nearly all types of ES traits showed lower genetic intraspecific variation than life history traits. One clear exception was genetic variation among genotypes in AAA traits, which was influenced by a large amount of variation in one study (Sherman et al., 2017). If this difference in variation between ES and life history traits is genuine, it could be inferred that less genetic variation in ES traits is maintained within populations and that ES trait means evolve slowly across populations, in comparison to life history traits. It is certainly plausible that life history traits should vary more widely than ES traits, because constraints on organism elemental composition may be strong relative to those on some life history traits, and because trade-offs in element allocation within an organism could decouple variation in the total elemental content of an organism from variation in organism morphology and/or growth rate (Meunier et al., 2017).
The evolution of ES traits has been explicitly assessed in various ways in a small number of prior studies. Two studies have calculated the heritability of ES traits using genotypes from wild populations (H2 = 0.61 [Barbour et al., 2015]; H2 = 0.18–0.21 [Crutsinger et al., 2014a]) suggesting that ES traits can respond to selection when genetic variation is present (but see Hansen et al., 2011). Indeed, artificial selection experiments have shown that all livestock species appear to have genetic variation for feed efficiency and this variation often has moderate heritability (Arthur and Herd, 2005). Moreover, artificial selection experiments on ES traits tend to achieve rapid ES trait evolution in agricultural species (Neely et al., 2008; de Verdal et al., 2013; Mignon-Grasteau et al., 2017). Selection on life history traits can also lead to evolution in ES traits, for example, artificial selection on body size can produce rapid evolution in P content (Gorokhova et al., 2002). We were able to locate two selection experiments where environmental conditions were manipulated and evolution of ES traits were tracked through time in laboratory conditions. In one of these experiments ES traits did not evolve over ~130 generations (Declerck et al., 2015) while the second experiment, which found that N and P content evolved in carbon-limited conditions, assessed trait evolution over 50,000 generations (Turner et al., 2017). We were only able to find a single instance of tracking the evolution of ES traits of natural or naturalistic populations (Frisch et al., 2014). In this study, the comparison of genotypes hatched from Daphnia resting eggs originating from a 700-year time interval in a single lake undergoing cultural eutrophication revealed an effect of P availability on AAA traits but not elemental composition (Frisch et al., 2014). Clearly, much more work is needed to determine whether and when ES traits evolve rapidly in natural contexts. Selection experiments conducted in nature or observational studies that track the evolution of populations through time in both ES and life history traits would provide valuable empirical data on the rate of evolution of ES phenotypes with realistic population dynamics and selective landscapes. These types of approaches could improve the understanding of whether and why ES traits tend to show less genetic variation than life history traits.
Differences in the Magnitude of Genetic Variation Among Stoichiometric Traits
One of the fundamental principles of ES theory is that the elemental composition of organisms is constrained by their basic requirements for the non-substitutable elemental resources that are allocated to fundamental biological structures and processes (Reiners, 1986; Sterner and Elser, 2002). Many of these life-enabling structures and processes are ancient (e.g., bone and wood; Wagner and Aspenberg, 2011; Morris et al., 2018), and some are as old as life itself (e.g., RNA synthesis; Joyce, 1989). Thus, it is perhaps not surprising that we found little genetic variation in bulk elemental composition within species, because any contemporary variation is miniscule relative to that found over the entirety of evolutionary history. In other words, the magnitude of rapid evolution in elemental composition is limited relative to what is seen across species with deep evolutionary divergence.
However, there was substantially more intraspecific genetic variation present in the AAAE traits than in the elemental composition traits, and this was the case for both genotype and population comparisons (Figure 1). This leads to questions about constraint of genetic diversity for elemental composition relative to AAAE traits. Acquisition, assimilation, allocation, and excretion are traits which actually contribute to and maintain elemental composition within the necessary range for organismal growth, maintenance, and survival (Sterner and Elser, 2002). Thus, our results suggest that there is more genetic variability in the strength of stoichiometric homeostasis than there is in the actual bulk elemental composition of organisms. This makes sense, given that genetic variation in AAAE traits is a pre-requisite for genetic variation in elemental composition (Jeyasingh et al., 2014). Indeed, elemental composition reflects the “balance” between the acquisition, assimilation, allocation, and excretion of elements at a given point during an organism's life. An evolved change in elemental composition would, therefore, presumably entail a corresponding heritable shift in the balance among AAAE traits.
Importantly, this does not mean to say that elemental composition does not evolve. Over macroevolutionary timescales evolution has generated an enormous diversity of stoichiometric phenotypes across taxa (Sterner and Elser, 2002). Yet much of this stoichiometric diversity has emerged not necessarily because ES traits are (or were) under direct selection, but because they are mechanistically correlated with functional traits that have had direct fitness implications. For example, the high C:nutrient ratio of a woody tree is a consequence of selection for height, which imparts an obvious competitive advantage to terrestrial autotrophs. Wood provides the rigid structural material necessary for this height and has a C:N ratio 200–1000 (Levi and Cowling, 1969). Thus, as entire organisms, trees have high C:N ratios relative to other organisms, but not because C:N ratio was under direct selection per se. Another example of indirect selection on ES traits with clearer implications for rapid evolution, comes from the stoichiometric growth rate hypothesis (GRH), which asserts that the functional trait of rapid growth has a P rich signature due to the high P content of ribosomal RNA (Elser et al., 2000b; Sterner and Elser, 2002). Identifying the stoichiometric patterns associated with variation in a larger suite of functional traits will be tremendously valuable to the study of evolutionary and ecological relationships (Meunier et al., 2017).
Within the elemental composition traits, we found more variation in P content than in C and N content. This is consistent with the argument that variation in organism P content will generally be greater than variation in organism C and N content, because levels of C and N tend to be similar among major biomolecules (i.e., protein and nucleic acids) while P content varies widely among biomolecules (e.g., protein contains no P, while RNA contains around 9.2% P; Sterner and Elser, 2002). Thus, changes in proportional concentrations of major biomolecules on a cellular level can scale up to drive variation in overall organism P content. Our results provide a novel genetic perspective that supports the view that P content is more variable than C and N, at least in the case of genotype comparisons.
Genetic Variation in ES Traits Within Populations and Across Population Pairs
We collected data on the amount of genetic variation by comparing across population pairs and among genotypes from the same population. The extent of variation among genotypes was generally larger than variation in population trait means, even when using a conservative methodology to calculate genotypic variation (Figure S1). When comparing across populations, we assessed shifts in the means between pairs of populations, which does not explicitly account for variation within populations. As such, our finding that genetic variation is generally greater amongst genotypes within a population than across populations reflects that the average genetic trait difference between genotypes is larger than the average trait shift between populations. Finding lower levels of variation across populations than among genotypes indicates that there is either little differentiation across populations, or large amounts of variation among genotypes. Whether this is to be expected or not depends on where genetic variation within a species is expected to be found: within or across populations. Genomic sequencing both within and across populations has uncovered support for each, including cases where the majority of genetic variation is found within populations (Pometti et al., 2015) and cases where the majority is found among them (Bakker et al., 2006). From the latter perspective this result is surprising, given that genetic variation within a population is limited by recombination amongst genotypes, which would serve to limit divergence within a population (Jain and Bradshaw, 1966; Ehrlich and Raven, 1969). There is potential for increased variation across populations because they originated from different environments, which have the potential to act as an agent of divergent selection driving local adaptation, whereas the genotypes compared mostly originated from the same environment. Genotypes may show a high amount of variation due to fluctuations in the environment, gene flow, or any other mechanism that can enhance the maintenance of trait diversity. On the other hand, trait divergence between populations may be limited if they do not come from stoichiometrically-disparate environments (e.g., little divergent selection on ES traits) or do not show substantial local adaptation due to stoichiometric constraints.
Our data provide some ability to assess the role of environmental differences, specifically P availability, in driving divergence in ES traits across populations. Populations that come from divergent P environments and those that come from similar P environments showed no differences in the amount of genetic variation in ES traits. This suggests that natural environmental differences in elemental availability may not be a sufficiently strong agent of selection to drive rapid evolution in ES traits, which could explain the largely similar levels of variation observed across genotypes and pairs of populations. Determining whether the limited variation we observed across natural populations is due to comparatively modest environmental stoichiometric differences or other factors that could limit divergence of populations (e.g., low divergence times, connectance between populations, reduced efficacy of selection) would be valuable in addressing this disparity.
Caveats and Limitations of the Meta-Analysis
There are several limitations in our analysis stemming from the meta-analysis framework, the nature of common gardens, and the specific designs of the studies available. First, studies that met the criteria for our meta-analysis included both plants and animals, but those that measured variation across genotypes were dominated by plants and cladocerans. Thus, inherent differences among taxonomic groups may be confounded with the genotype- and population-level comparisons, though the relative amounts of variation were similar among all taxonomic groups. Second, genotype-level variation may be overestimated relative to natural populations, because some studies included genotypes with low ecological fitness (e.g., Sherman et al., 2017) and some collected genotypes from wide geographical areas that covered a range of environments similar to the magnitude of variation we would expect across populations. Third, many of the common gardens were only conducted for a single generation, meaning that maternal and epigenetic effects could represent latent sources of trait variation (Weaver et al., 2004). Finally, a “common garden” allows for measurement of the amount of genetic variation in traits in a single environment and the chosen environmental conditions may influence the amount of trait variation displayed. For example, there was more variation in P-acquisition and assimilation under P-limited conditions relative to P-sufficient conditions (Sherman et al., 2017). However, we assumed that the common garden conditions were chosen by the original study authors to reflect ecologically-relevant environments, and that the trait variation we observed is, therefore, a reasonable representation of that found in nature.
Ecological Consequences of Intraspecific Variation in ES Traits and the Potential for Eco-Evolutionary Feedbacks
Over the past two decades there has been a growing recognition that intraspecific trait variation can have large effects on ecological patterns and processes (Schweitzer et al., 2004; Crutsinger et al., 2006; Whitham et al., 2006; Post et al., 2008; Harmon et al., 2009; Bassar et al., 2010; Violle et al., 2012; Des Roches et al., 2018). Intraspecific variation in ES traits has been identified as particularly likely to have strong ecological consequences because ES traits connect the organism with its environment. Our meta-analysis uncovered substantial differences in the amount of intraspecific variation across types of ES traits, with excretion and AAA traits showing considerably more intraspecific variation than most elemental ratios and measures of elemental composition. Although there have been few empirical tests of the ecological consequences of intraspecific variation in ES traits (but see Chowdhury and Jeyasingh, 2016), any evolution that shapes these traits is likely to have ecological consequences (Jeyasingh et al., 2014; El-Sabaawi et al., 2016). Future experimental work that measures the ecological consequences of genetic variation in ES traits in ecologically realistic contexts will be crucial to determining the importance of intraspecific variation in ES traits for community structure and ecosystem function.
Ideally, this future work will indicate whether rapid evolution of ES traits is likely to have ecological consequences, and whether these ecological consequences could lead to rapid evolution (i.e., an eco-evolutionary feedback). Using a strict definition of eco-evolutionary feedback (Schoener, 2011), two interacting processes would be required: ES traits would have to evolve rapidly in a population and the evolution of these ES traits would need to modify the environment in a way that significantly affects selection on that population. The potential for eco-evolutionary feedbacks mediated by the evolution of ES traits has been much discussed (Jeyasingh et al., 2014; Leal et al., 2017b; Rudman et al., 2019), and some studies have demonstrated that rapid evolution can shape elemental availability (Bassar et al., 2010; Rudman and Schluter, 2016). Yet, whether these differences in elemental availability feed back onto rapid evolution is wholly unknown. As such, determining the existence, magnitude, and prevalence of these eco-evolutionary feedbacks is well beyond the scope of our meta-analysis, especially as there is very little empirical evidence of eco-evolutionary feedbacks overall (Schoener, 2011; Turcotte et al., 2011; Matthews et al., 2016; Rudman et al., 2018). What little data our meta-analysis does provide to examine eco-evolutionary feedbacks based on ecological stoichiometry does not support widespread rapid evolution of ES traits, as variation across populations was similar to what was observed amongst genotypes found within populations. Moreover, we did not find evidence that divergence in P environment led to enhanced divergence in ES traits across populations, suggesting that environmental variation might need to be substantial to drive ES trait evolution. Nevertheless, our findings are mere hints at the prevalence of eco-evolutionary feedbacks mediated by ES traits and future work is certainly warranted.
Author Contributions
KL conceived of the study. KL, OB, TK, SR, and CS reviewed literature, aggregated data, and wrote and edited the manuscript. The authors were listed alphabetically after the first author.
Funding
Funding was provided by the National Science Foundation (NSF) through NSF award DEB-1840408.
Conflict of Interest Statement
The authors declare that the research was conducted in the absence of any commercial or financial relationships that could be construed as a potential conflict of interest.
The reviewer, PJ, declared a past co-authorship with one of the authors, SR, to the handling editor.
Acknowledgments
We would like to thank Jim Elser, Michelle Evans-White, and Joe Vanderwall for their efforts that made the Woodstoich IV workshop possible. We thank our Woodstoich IV mentors, Jotaro Urabe, and Jennifer Schweitzer for feedback and helpful discussions. We thank Daniel Noble and Vincent Fugère for valuable guidance on the statistical analysis.
Supplementary Material
The Supplementary Material for this article can be found online at: https://www.frontiersin.org/articles/10.3389/fevo.2019.00339/full#supplementary-material
References
Arthur, P. F., and Herd, R. M. (2005). Efficiency of feed utilisation by livestock — Implications and benefits of genetic improvement. Can. J. Anim. Sci. 85, 281–290. doi: 10.4141/A04-062
Bakker, E. G., Stahl, E. A., Toomajian, C., Nordborg, M., Kreitman, M., and Bergelson, J. (2006). Distribution of genetic variation within and among local populations of Arabidopsis thaliana over its species range: population structure of Arabidopsis. Mol. Ecol. 15, 1405–1418. doi: 10.1111/j.1365-294X.2006.02884.x
Barbour, M. A., Rodriguez-Cabal, M. A., Wu, E. T., Julkunen-Tiitto, R., Ritland, C. E., Miscampbell, A. E., et al. (2015). Multiple plant traits shape the genetic basis of herbivore community assembly. Funct. Ecol. 29, 995–1006. doi: 10.1111/1365-2435.12409
Barrett, R. D. H., and Schluter, D. (2008). Adaptation from standing genetic variation. Trends Ecol. Evol. 23, 38–44. doi: 10.1016/j.tree.2007.09.008
Bassar, R. D., Marshall, M. C., López-Sepulcre, A., Zandonà, E., Auer, S. K., Travis, J., et al. (2010). Local adaptation in Trinidadian guppies alters ecosystem processes. Proc. Natl. Acad. Sci. U.S.A. 107, 3616–3621. doi: 10.1073/pnas.0908023107
Bland, M. (2015). Estimating mean and standard deviation from the sample size, three quartiles, minimum, and maximum. Int. J. Stats. Med. Res. 4, 57–64. doi: 10.6000/1929-6029.2015.04.01.6
Bolnick, D. I., Amarasekare, P., Araújo, M. S., Bürger, R., Levine, J. M., Novak, M., et al. (2011). Why intraspecific trait variation matters in community ecology. Trends Ecol. Evol. 26, 183–192. doi: 10.1016/j.tree.2011.01.009
Burnham, K. P., Anderson, D. R., and Burnham, K. P. (2002). Model Selection and Multimodel Inference: A Practical Information-Theoretic Approach, 2nd Edn. New York, NY: Springer.
Chowdhury, P., and Jeyasingh, P. (2016). Differences in phosphorus use between ancient and extant Daphnia genotypes alters algal stoichiometry and abundance. Inland Waters 6, 165–172. doi: 10.5268/IW-6.2.912
Chowdhury, P. R., Lopez, J. A., Weider, L. J., Colbourne, J. K., and Jeyasingh, P. D. (2014). Functional genomics of intraspecific variation in carbon and phosphorus kinetics in Daphnia: Sources of variation in carbon and phosphorus use. J. Exp. Zool. 321, 387–398. doi: 10.1002/jez.1869
Crutsinger, G. M., Collins, M. D., Fordyce, J. A., Gompert, Z., Nice, C. C., and Sanders, N. J. (2006). Plant genotypic diversity predicts community structure and governs an ecosystem process. Science 313, 966–968. doi: 10.1126/science.1128326
Crutsinger, G. M., Rodriguez-Cabal, M. A., Roddy, A. B., Peay, K. G., Bastow, J. L., Kidder, A. G., et al. (2014a). Genetic variation within a dominant shrub structures green and brown community assemblages. Ecology 95, 387–398. doi: 10.1890/13-0316.1
Crutsinger, G. M., Rudman, S. M., Rodriguez-Cabal, M. A., McKown, A. D., Sato, T., MacDonald, A. M., et al. (2014b). Testing a ‘genes-to-ecosystems' approach to understanding aquatic-terrestrial linkages. Mol. Ecol. 23, 5888–5903. doi: 10.1111/mec.12931
Crutsinger, G. M., Sanders, N. J., and Classen, A. T. (2009). Comparing intra- and inter-specific effects on litter decomposition in an old-field ecosystem. Basic Appl. Ecol. 10, 535–543. doi: 10.1016/j.baae.2008.10.011
Dalton, C. M., El-Sabaawi, R. W., Honeyfield, D. C., Auer, S. K., Reznick, D. N., and Flecker, A. S. (2017). The influence of dietary and whole-body nutrient content on the excretion of a vertebrate consumer. PLoS ONE 12:e0187931. doi: 10.1371/journal.pone.0187931
de Verdal, H., Narcy, A., Bastianelli, D., Même, N., Urvoix, S., Collin, A., et al. (2013). Genetic variability of metabolic characteristics in chickens selected for their ability to digest wheat. J. Anim. Sci. 91, 2605–2615. doi: 10.2527/jas.2012-6182
Declerck, S. A. J., Malo, A. R., Diehl, S., Waasdorp, D., Lemmen, K. D., Proios, K., et al. (2015). Rapid adaptation of herbivore consumers to nutrient limitation: eco-evolutionary feedbacks to population demography and resource control. Ecol. Lett. 18, 553–562. doi: 10.1111/ele.12436
DeMott, W. R., Pape, B. J., and Tessier, A. J. (2004). Patterns and sources of variation in Daphnia phosphorus content in nature. Aquat. Ecol. 38, 433–440. doi: 10.1023/B:AECO.0000035183.53389.66
Des Roches, S., Post, D. M., Turley, N. E., Bailey, J. K., Hendry, A. P., Kinnison, M. T., et al. (2018). The ecological importance of intraspecific variation. Nat. Ecol. Evol. 2, 57–64. doi: 10.1038/s41559-017-0402-5
Dinh Van, K., Janssens, L., Debecker, S., De Jonge, M., Lambret, P., Nilsson-Örtman, V., et al. (2013). Susceptibility to a metal under global warming is shaped by thermal adaptation along a latitudinal gradient. Glob. Change Biol. 19, 2625–2633. doi: 10.1111/gcb.12243
Ehrlich, P. R., and Raven, P. H. (1969). Differentiation of populations. Science 165, 1228–1232. doi: 10.1126/science.165.3899.1228
El-Sabaawi, R. W., Warbanski, M. L., Rudman, S. M., and Hovel, R. others (2016). Investment in boney defensive traits alters organismal stoichiometry and excretion in fish. Oecologia 181, 1209–1220. doi: 10.1007/s00442-016-3599-0
Elser, J. (2006). Biological stoichiometry: a chemical bridge between ecosystem ecology and evolutionary biology. Am. Nat. 168(Suppl. 6), S25–S35. doi: 10.1086/509048
Elser, J. J. (2003). Biological stoichiometry: a theoretical framework connecting ecosystem ecology, evolution, and biochemistry for application in astrobiology. Int. J. Astrobiol. 2, 185–193. doi: 10.1017/S1473550403001563
Elser, J. J., Acharya, K., Kyle, M., Cotner, J., Makino, W., Markow, T., et al. (2003). Growth rate-stoichiometry couplings in diverse biota. Ecol. Lett. 6, 936–943. doi: 10.1046/j.1461-0248.2003.00518.x
Elser, J. J., Fagan, W. F., Denno, R. F., Dobberfuhl, D. R., Folarin, A., Huberty, A., et al. (2000a). Nutritional constraints in terrestrial and freshwater food webs. Nature 408, 578–580. doi: 10.1038/35046058
Elser, J. J., Kyle, M. M., Smith, M. S., and Nagy, J. D. (2007). Biological stoichiometry in human cancer. PLoS ONE 2:e1028. doi: 10.1371/journal.pone.0001028
Elser, J. J., Sterner, R. W., Gorokhova, E., Fagan, W. F., Markow, T. A., Cotner, J. B., et al. (2008). Biological stoichiometry from genes to ecosystems. Ecol. Lett. 3, 540–550. doi: 10.1111/j.1461-0248.2000.00185.x
Elser, O'Brien, Dobberfuhl, and Dowling. (2000b). The evolution of ecosystem processes: growth rate and elemental stoichiometry of a key herbivore in temperate and arctic habitats. J. Evol. Biol. 13, 845–853. doi: 10.1046/j.1420-9101.2000.00215.x
Espeset, A., Kobiela, M. E., Sikkink, K. L., Pan, T., Roy, C., and Snell-Rood, E. C. (2019). Anthropogenic increases in nutrients alter sexual selection dynamics: a case study in butterflies. Behav. Ecol. 30, 598–608. doi: 10.1093/beheco/arz004
Frisch, D., Morton, P. K., Chowdhury, P. R., Culver, B. W., Colbourne, J. K., Weider, L. J., et al. (2014). A millennial-scale chronicle of evolutionary responses to cultural eutrophication in Daphnia. Ecol. Lett. 17, 360–368. doi: 10.1111/ele.12237
González, A. L., Fariña, J. M., Kay, A. D., Pinto, R., and Marquet, P. A. (2011). Exploring patterns and mechanisms of interspecific and intraspecific variation in body elemental composition of desert consumers. Oikos 120, 1247–1255. doi: 10.1111/j.1600-0706.2010.19151.x
Gorokhova, E., Dowling, T. E., Weider, L. J., Crease, T. J., and Elser, J. J. (2002). Functional and ecological significance of rDNA intergenic spacer variation in a clonal organism under divergent selection for production rate. Proc. R. Soc. Lond. B 269, 2373–2379. doi: 10.1098/rspb.2002.2145
Güsewell, S., Jakobs, G., and Weber, E. (2006). Native and introduced populations of Solidago gigantea differ in shoot production but not in leaf traits or litter decomposition. Funct. Ecol. 20, 575–584. doi: 10.1111/j.1365-2435.2006.01141.x
Hadfield, J. (2010). MCMC methods for multi-response generalized linear mixed models: the MCMCglmm R package. J. Stat. Softw. 33, 1–22. doi: 10.18637/jss.v033.i02
Hansen, T. F., Pélabon, C., and Houle, D. (2011). Heritability is not evolvability. Evol. Biol. 38, 258–277. doi: 10.1007/s11692-011-9127-6
Harmon, L. J., Matthews, B., Des Roches, S., Chase, J. M., Shurin, J. B., and Schluter, D. (2009). Evolutionary diversification in stickleback affects ecosystem functioning. Nature 458, 1167–1170. doi: 10.1038/nature07974
Hedges, L. V., Gurevitch, J., and Curtis, P. S. (1999). The meta-analysis of response ratios in experimental ecology. Ecology 80, 1150–1156. doi: 10.1890/0012-9658(1999)080<1150:TMAORR>2.0.CO;2
Hereford, J., Hansen, T. F., and Houle, D. (2004). Comparing strengths of directional selection: how strong is strong? Evolution 58, 2133–2143. doi: 10.1111/j.0014-3820.2004.tb01592.x
Higgins, J. P., and Green, S. eds. (2008). Cochrane Handbook for Systematic Reviews of Interventions. Chichester: John Wiley and Sons, Ltd. doi: 10.1002/9780470712184
Hughes, A. R., Stachowicz, J. J., and Williams, S. L. (2009). Morphological and physiological variation among seagrass (Zostera marina) genotypes. Oecologia 159, 725–733. doi: 10.1007/s00442-008-1251-3
Jain, S. K., and Bradshaw, A. D. (1966). Evolutionary divergence among adjacent plant populations I. The evidence and its theoretical analysis. Heredity 21, 407–441. doi: 10.1038/hdy.1966.42
Jeyasingh, P. D., Chowdhury, P. R., Wojewodzic, M. W., Frisch, D., Hessen, D. O., and Weider, L. J. (2015). Phosphorus use and excretion varies with ploidy level in Daphnia. J. Plankton Res., fbv095. doi: 10.1093/plankt/fbv095
Jeyasingh, P. D., Cothran, R. D., and Tobler, M. (2014). Testing the ecological consequences of evolutionary change using elements. Ecol. Evol. 4, 528–538. doi: 10.1002/ece3.950
Jeyasingh, P. D., and Weider, L. J. (2007). Fundamental links between genes and elements: evolutionary implications of ecological stoichiometry. Mol. Ecol. 16, 4649–4661. doi: 10.1111/j.1365-294X.2007.03558.x
Jeyasingh, P. D., Weider, L. J., and Sterner, R. W. (2009). Genetically-based trade-offs in response to stoichiometric food quality influence competition in a keystone aquatic herbivore. Ecol. Lett. 12, 1229–1237. doi: 10.1111/j.1461-0248.2009.01368.x
Johnson, M. T. J., Agrawal, A. A., Maron, J. L., and Salminen, J.-P. (2009). Heritability, covariation and natural selection on 24 traits of common evening primrose (Oenothera biennis) from a field experiment. J. Evol. Biol. 22, 1295–1307. doi: 10.1111/j.1420-9101.2009.01747.x
Joyce, G. F. (1989). RNA evolution and the origins of life. Nature 338, 217–224. doi: 10.1038/338217a0
Kay, A. D., Ashton, I. W., Gorokhova, E., Kerkhoff, A. J., Liess, A., and Litchman, E. (2005). Toward a stoichiometric framework for evolutionary biology. Oikos 109, 6–17. doi: 10.1111/j.0030-1299.2005.14048.x
Kingsolver, J. G., Diamond, S. E., Siepielski, A. M., and Carlson, S. M. (2012). Synthetic analyses of phenotypic selection in natural populations: lessons, limitations and future directions. Evol. Ecol. 26, 1101–1118. doi: 10.1007/s10682-012-9563-5
Leal, M. C., Best, R. J., Durston, D., El-Sabaawi, R. W., and Matthews, B. (2017a). Stoichiometric traits of stickleback: Effects of genetic background, rearing environment, and ontogeny. Ecol. Evol. 7, 2617–2625. doi: 10.1002/ece3.2802
Leal, M. C., Seehausen, O., and Matthews, B. (2017b). The ecology and evolution of stoichiometric phenotypes. Trends Ecol. Evol. 32, 108–117. doi: 10.1016/j.tree.2016.11.006
LeRoy, C. J., Whitham, T. G., Wooley, S. C., and Marks, J. C. (2007). Within-species variation in foliar chemistry influences leaf-litter decomposition in a Utah river. J. North Am. Benthol. Soc. 26, 426–438. doi: 10.1899/06-113.1
LeRoy, C. J., Wooley, S. C., and Lindroth, R. L. (2012). Genotype and soil nutrient environment influence aspen litter chemistry and in-stream decomposition. Freshw. Sci. 31, 1244–1253. doi: 10.1899/12-029.1
Levi, M. P., and Cowling, E. B. (1969). Role of nitrogen in wood deterioration, VII: Physiological adaptation of wood-destroying and other fungi to substrates deficient in nitrogen. Phytopathology, 460–468.
Liess, A., Guo, J., Lind, M. I., and Rowe, O. (2015). Cool tadpoles from Arctic environments waste fewer nutrients - high gross growth efficiencies lead to low consumer-mediated nutrient recycling in the North. J. Anim. Ecol. 84, 1744–1756. doi: 10.1111/1365-2656.12426
Liess, A., Rowe, O., Guo, J., Thomsson, G., and Lind, M. I. (2013). Hot tadpoles from cold environments need more nutrients - life history and stoichiometry reflects latitudinal adaptation. J. Anim. Ecol. 82, 1316–1325. doi: 10.1111/1365-2656.12107
Lynch, M., and Walsh, B. (1998). Genetics and Analysis of quantitatiVe Traits. Sunderland; Mass, MI: Sinauer.
Madritch, M., Donaldson, J. R., and Lindroth, R. L. (2006). Genetic identity of Populus tremuloides litter influences decomposition and nutrient release in a mixed forest stand. Ecosystems 9, 528–537. doi: 10.1007/s10021-006-0008-2
Madritch, M. D., and Hunter, M. D. (2005). Phenotypic variation in oak litter influences short- and long-term nutrient cycling through litter chemistry. Soil Biol. Biochem. 37, 319–327. doi: 10.1016/j.soilbio.2004.08.002
Matthews, B., Aebischer, T., Sullam, K. E., Lundsgaard-Hansen, B., and Seehausen, O. (2016). Experimental evidence of an eco-evolutionary feedback during adaptive divergence. Curr. Biol. 26, 483–489. doi: 10.1016/j.cub.2015.11.070
Matthews, B., Narwani, A., Hausch, S., Nonaka, E., Peter, H., Yamamichi, M., et al. (2011). Toward an integration of evolutionary biology and ecosystem science. Ecol. Lett. 14, 690–701. doi: 10.1111/j.1461-0248.2011.01627.x
Meunier, C. L., Boersma, M., El-Sabaawi, R., Halvorson, H. M., Herstoff, E. M., Van de Waal, D. B., et al. (2017). From elements to function: toward unifying ecological stoichiometry and trait-based ecology. Front. Environ. Sci. 5:18. doi: 10.3389/fenvs.2017.00018
Mignon-Grasteau, S., Chantry-Darmon, C., Boscher, M.-Y., Sellier, N., Le Bihan-Duval, E., and Bertin, A. (2017). Genetic determinism of fearfulness, general activity and feeding behavior in chickens and its relationship with digestive efficiency. Behav. Genet. 47, 114–124. doi: 10.1007/s10519-016-9807-1
Moody, E. K., Carson, E. W., Corman, J. R., Espinosa-Pérez, H., Ramos, J., Sabo, J. L., et al. (2018). Consumption explains intraspecific variation in nutrient recycling stoichiometry in a desert fish. Ecology 99, 1552–1561. doi: 10.1002/ecy.2372
Morris, J. L., Puttick, M. N., Clark, J. W., Edwards, D., Kenrick, P., Pressel, S., et al. (2018). The timescale of early land plant evolution. Proc. Natl. Acad. Sci U.S.A. 115, E2274–E2283. doi: 10.1073/pnas.1719588115
Morrissey, M. B. (2016). Meta-analysis of magnitudes, differences and variation in evolutionary parameters. J. Evol. Biol. 29, 1882–1904. doi: 10.1111/jeb.12950
Neely, K. G., Myers, J. M., Hard, J. J., and Shearer, K. D. (2008). Comparison of growth, feed intake, and nutrient efficiency in a selected strain of coho salmon (Oncorhynchus kisutch) and its source stock. Aquaculture 283, 134–140. doi: 10.1016/j.aquaculture.2008.06.038
Neiman, M., Kay, A. D., and Krist, A. C. (2012). Sensitivity to phosphorus limitation increases with ploidy level in a New Zealand snail. Evolution. 67, 1511–1517. doi: 10.1111/evo.12026
Noble, D. (2019). metaAidR. GitHub Repository. Available online at: https://github.com/daniel1noble/metaAidR/
Noble, D. W. A., Lagisz, M., O'dea, R. E., and Nakagawa, S. (2017). Nonindependence and sensitivity analyses in ecological and evolutionary meta-analyses. Mol. Ecol. 26, 2410–2425. doi: 10.1111/mec.14031
Pometti, C. L., Bessega, C. F., Vilardi, J. C., Cialdella, A. M., and Saidman, B. O. (2015). Genetic diversity within and among two Argentinean and one Mexican species of Acacia (Fabaceae): Genetic Diversity in Three Acacia Species. Bot. J. Linn. Soc. 177, 593–606. doi: 10.1111/boj.12262
Post, D. M., Palkovacs, E. P., Schielke, E. G., and Dodson, S. I. (2008). Intraspecific variation in a predator affects community structure and cascading trophic interactions. Ecology 89, 2019–2032. doi: 10.1890/07-1216.1
Prater, C., Wagner, N. D., and Frost, P. C. (2017). Interactive effects of genotype and food quality on consumer growth rate and elemental content. Ecology 98, 1399–1408. doi: 10.1002/ecy.1795
R Core Team (2016). R: A Language and Environment for Statistical Computing. Vienna: R Foundation for Statistical Computing. Available online at: https://www.R-project.org/
Reiners, W. A. (1986). Complementary models for ecosystems. Am. Nat. 127, 59–73. doi: 10.1086/284467
Rejmánková, E. (2005). Nutrient resorption in wetland macrophytes: comparison across several regions of different nutrient status. New Phytol. 167, 471–482. doi: 10.1111/j.1469-8137.2005.01449.x
Rodriguez-Cabal, M. A., Barrios-Garcia, M. N., Rudman, S. M., McKown, A. D., Sato, T., and Crutsinger, G. M. (2017). It is about time: genetic variation in the timing of leaf-litter inputs influences aquatic ecosystems. Freshw. Biol. 62, 356–365. doi: 10.1111/fwb.12872
Rudman, S. M., Barbour, M. A., Csilléry, K., Gienapp, P., Guillaume, F., Hairston, N. G., et al. (2018). What genomic data can reveal about eco-evolutionary dynamics. Nat. Ecol. Evol. 2, 9–15. doi: 10.1038/s41559-017-0385-2
Rudman, S. M., Goos, J. M., Burant, J. B., Brix, K. V., Gibbons, T. C., Brauner, C. J., et al. (2019). Ionome and elemental transport kinetics shaped by parallel evolution in threespine stickleback. Ecol. Lett. 22, 645–653. doi: 10.1111/ele.13225
Rudman, S. M., and Schluter, D. (2016). Ecological impacts of reverse speciation in threespine stickleback. Curr. Biol. 26, 490–495. doi: 10.1016/j.cub.2016.01.004
Schade, J. D., Kyle, M., Hobbie, S. E., Fagan, W. F., and Elser, J. J. (2003). Stoichiometric tracking of soil nutrients by a desert insect herbivore. Ecol. Lett. 6, 96–101. doi: 10.1046/j.1461-0248.2003.00409.x
Schoener, T. W. (2011). The newest synthesis: understanding the interplay of evolutionary and ecological dynamics. Science 331, 426–429. doi: 10.1126/science.1193954
Schweitzer, J., Bailey, J., Rehill, B., Martinsen, G., Hart, S., Lindroth, R., et al. (2004). Genetically based trait in a dominant tree affects ecosystem processes. Ecol. Lett. 7, 127–134. doi: 10.1111/j.1461-0248.2003.00562.x
Sherman, R. E., Chowdhury, P. R., Baker, K. D., Weider, L. J., and Jeyasingh, P. D. (2017). Genotype-specific relationships among phosphorus use, growth and abundance in Daphnia pulicaria. R. Soc. Open sci. 4:170770. doi: 10.1098/rsos.170770
Silfver, T., Mikola, J., Rousi, M., Roininen, H., and Oksanen, E. (2007). Leaf litter decomposition differs among genotypes in a local Betula pendula population. Oecologia 152, 707–714. doi: 10.1007/s00442-007-0695-1
Sterner, R. W., and Elser, J. J. (2002). Ecological Stoichiometry: The Biology of Elements From Molecules to the Biosphere. Princeton University Press Available online at: https://play.google.com/store/books/details?id=53NTDvppdYUC
Sterner, R. W., Hood, J. M., Kearney, M. R., Urabe, J., and Raubenheimer, D. (2015). Couples that have chemistry: when ecological theories meet. Oikos 124, 917–919. doi: 10.1111/oik.02672
Townsend, A. R., Cleveland, C. C., Asner, G. P., and Bustamante, M. M. C. (2007). Controls over foliar N:P ratios in tropical rain forests. Ecology 88, 107–118. doi: 10.1890/0012-9658(2007)88<107:COFNRI>2.0.CO;2
Trakimas, G., Krams, R., Krama, T., Kortet, R., Haque, S., Luoto, S., et al. (2019). Ecological stoichiometry: a link between developmental speed and physiological stress in an omnivorous insect. Front. Behav. Neurosci. 13:42. doi: 10.3389/fnbeh.2019.00042
Turcotte, M. M., Reznick, D. N., and Hare, J. D. (2011). The impact of rapid evolution on population dynamics in the wild: experimental test of eco-evolutionary dynamics. Ecol. Lett. 14, 1084–1092. doi: 10.1111/j.1461-0248.2011.01676.x
Turner, C. B., Wade, B. D., Meyer, J. R., Sommerfeld, B. A., and Lenski, R. E. (2017). Evolution of organismal stoichiometry in a long-term experiment with Escherichia coli. R. Soc. Open Sci. 4:170497. doi: 10.1098/rsos.170497
Violle, C., Enquist, B. J., McGill, B. J., Jiang, L., Albert, C. H., Hulshof, C., et al. (2012). The return of the variance: intraspecific variability in community ecology. Trends Ecol. Evol. 27, 244–52. doi: 10.1016/j.tree.2011.11.014
Visanuvimol, L., and Bertram, S. M. (2011). How dietary phosphorus availability during development influences condition and life history traits of the cricket, Acheta domesticas. J. Insect Sci. 11, 1–17. doi: 10.1673/031.011.6301
Wagner, D. O., and Aspenberg, P. (2011). Where did bone come from?: An overview of its evolution. Acta Orthopaed. 82, 393–398. doi: 10.3109/17453674.2011.588861
Weaver, I. C. G., Cervoni, N., Champagne, F. A., D'Alessio, A. C., Sharma, S., Seckl, J. R., et al. (2004). Epigenetic programming by maternal behavior. Nat. Neurosci. 7, 847–854. doi: 10.1038/nn1276
Whitham, T., Bailey, J., Schweitzer, J., Shuster, S., Bangert, R., Leroy, C., et al. (2006). A framework for community and ecosystem genetics: from genes to ecosystems. Nat. Rev. Genet. 7, 510–523. doi: 10.1038/nrg1877
Keywords: elemental phenotype, evolution, eco-evolutionary dynamics, phosphorus, organismal stoichiometry, nutrient excretion, intraspecific variation
Citation: Lemmen KD, Butler OM, Koffel T, Rudman SM and Symons CC (2019) Stoichiometric Traits Vary Widely Within Species: A Meta-Analysis of Common Garden Experiments. Front. Ecol. Evol. 7:339. doi: 10.3389/fevo.2019.00339
Received: 22 July 2019; Accepted: 23 August 2019;
Published: 20 September 2019.
Edited by:
Michelle Evans-White, University of Arkansas, United StatesReviewed by:
Halvor Matthew Halvorson, University of Central Arkansas, United StatesPuni Jeyasingh, Oklahoma State University, United States
Esteban Balseiro, National University of Comahue, Argentina
Copyright © 2019 Lemmen, Butler, Koffel, Rudman and Symons. This is an open-access article distributed under the terms of the Creative Commons Attribution License (CC BY). The use, distribution or reproduction in other forums is permitted, provided the original author(s) and the copyright owner(s) are credited and that the original publication in this journal is cited, in accordance with accepted academic practice. No use, distribution or reproduction is permitted which does not comply with these terms.
*Correspondence: Kimberley D. Lemmen, bGVtbWVuLmtpbWJlcmxleSYjeDAwMDQwO2dtYWlsLmNvbQ==