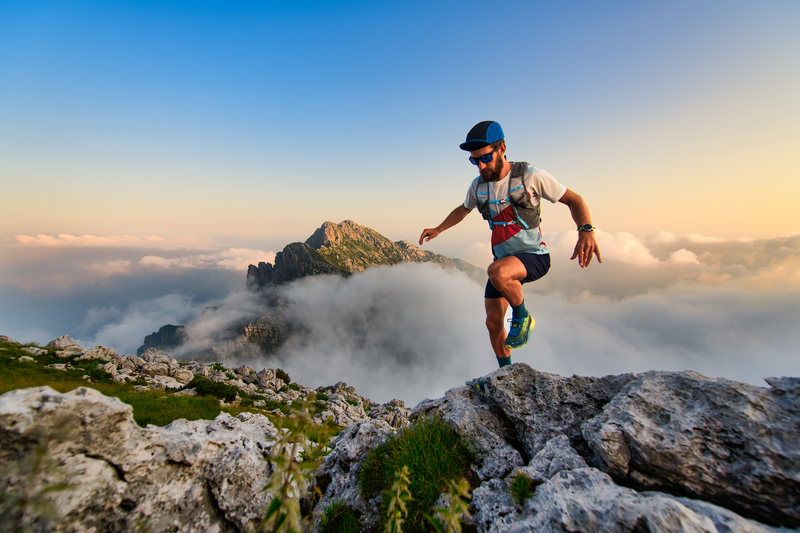
95% of researchers rate our articles as excellent or good
Learn more about the work of our research integrity team to safeguard the quality of each article we publish.
Find out more
ORIGINAL RESEARCH article
Front. Ecol. Evol. , 06 August 2019
Sec. Biogeography and Macroecology
Volume 7 - 2019 | https://doi.org/10.3389/fevo.2019.00291
Similarity of climatic niches occupied by a species in its native and invasive ranges has been recognized as one of the key factors for successful invasion. Accounting for changes in climatic niche over time may provide better understanding of the dynamic biological invasion process. We investigated these changes for an invasive alien plant Mikania micrantha in South and Southeast Asia, Australia, Oceania and parts of the USA. Based on documented occurrences over 200 years (1800–2017), we reconstructed the introduction history of the species and estimated climatic niche dynamics for the presumed invasion routes. We estimated niche conservatism and the environmental analogy between native and invasive ranges and used ecological niche modeling to identify the potential distribution of M. micrantha under current and future climate change scenarios. Our study identified six pathways through which the species was presumably introduced from its native range. Climatic conditions of the initial introduction sites were found to be similar to those in the native niche. However, the species occupied climatic niches in dry and cold areas over time, being outside of the limits of the realized climatic niche of its native range. The climatic niche dynamics also differed between the invasion pathways with variable time lag. This pattern of niche change over time was found to be consistent in future. Nearly 20% of the invasive range was found to be climatically suitable for this species and was predicted to expand toward cold and dry areas of the invasive range affecting nearly half of the global biodiversity hotspots. By analyzing M. micrantha invasion at a time scale, this study revealed multiple introduction pathways, variation in climatic niche dynamics among invasion routes, and potential range expansion of the species in its invasive range. Although further experimental and molecular studies are needed to explain these findings fully, this study highlights the need for temporally explicit approaches toward better understanding and successful management of biological invasions.
With increasing number of reports on negative impacts of invasive species on regional biota (Iacarella et al., 2015; Early et al., 2016; Bellard et al., 2017), biological invasion has become a severe problem globally and for obvious reasons, is in the spotlight of recent research trends (e.g., Bosso et al., 2017; Franco et al., 2018; Briscoe Runquist et al., 2019). Numerous studies have been conducted to identify how a minor component of native communities has successfully established itself in new and heterogeneous environment and becomes dominant in the invaded communities (Callaway and Maron, 2006).
One of the key factors for invasion success is the climate similarity between native and invasive ranges (Williamson, 2006) and recent studies have revealed that introduction of invasive species took place within climatic niches of their native range (Broennimann et al., 2007; Broennimann and Guisan, 2008). Based on the assumption that climatic niche would be conserved between native and invasive ranges (Elith et al., 2006, 2010), ecological niche models (ENMs) are frequently used to develop the potential distribution of invasive species under current and future climate change scenarios (Mainali et al., 2015; Elith, 2017). However, since biological invasion is a process along introduction-naturalization-invasion continuum (Moodley et al., 2013) and the invaded environment is also dynamic in nature, this assumption may not always hold true. Indeed, invasive species are often found to occupy novel climate conditions in the invasive range (Guisan et al., 2014) due to their ability for local adaptation (Hällfors et al., 2016; Oduor et al., 2016) and expressing phenotypic variation across a range of environmental conditions (Grewell et al., 2016; Li et al., 2016). Additionally, repeated introduction to different locations is a feature of many successful invasions (e.g., Chapman et al., 2016). Multiple introductions across space enhance the probability of encountering favorable environment (Blackburn et al., 2015) and therefore, may induce variability of climatic niche occupancy among introduced populations. Impact of climate change on biological invasions has been well-recognized, although the strength and direction of these impacts may vary depending on the species and ecosystem concerned (Bellard et al., 2013). In this context, accounting for these dynamic processes over time may provide a better understanding of the spatial and temporal aspects of successful invasions (Dietz and Edwards, 2006). Integration of climatic niche dynamics with ENM modeling approaches is therefore required to understand how ecological and evolutionary forces interact to shape the distribution of an invasive species under current and projected climate change. While a number of studies have used ENMs to investigate the climatic niche conservatism between native and invasive ranges (Atwater et al., 2018), assessment of change in climatic niche over time is rare for most of the invasive species (Broennimann et al., 2014). These research gaps are more prominent in the tropical regions where majority of biodiversity hotspots are located and invasions are least recognized and studied (Early et al., 2016). In these regions, in the coming decades, the threat of invasive alien species is estimated to be much higher.
In this study, we considered one global invasive species (species with ranges spanning more than one continent) Mikania micrantha Kunth, a native of Latin America and highly invasive in South and Southeast Asia, Oceania and Australia (Ellison and Sankaran, 2017). Due to its extensive economic and ecological impacts on natural forests, plantations and agricultural systems across its invasive range (Tripathi et al., 2012), it is considered as one of the top ten worst weeds of the world (Holm et al., 1977). The history of M. micrantha in most of its invasive range, although speculative and based on anecdotal evidence, indicates multiple introduction events separated geographically and temporally (Day et al., 2016). Previous ENM studies have predicted the potential distribution of M. micrantha at regional (Choudhury et al., 2016), national (Iyer et al., 2019), and global (Day et al., 2016) scales, however, with the assumption of climatic niche conservatism between its native and invasive ranges. M. micrantha can establish across different environmental conditions due to its ability to express phenotypic variation of traits [e.g., photosynthetic activity (Prabu et al., 2014), water and nitrogen use efficiency (Deng et al., 2004), leaf construction cost (Song et al., 2007), and vegetative and sexual reproduction Li et al., 2013; Banerjee et al., 2017a]. In this context, the modeled distribution based on the niche conservatism hypothesis may not reflect the true potential distribution of the species. In addition, these studies were also limited by the choice of algorithms and general circulation models for future predictions.
Given the invasion risk posed by M. micrantha in its invasion range, it is important to identify the introduction pathways of this species and assess its spread dynamics across its invasive range under present and future climate conditions. Based on previous reports of ecological tolerance and wide adaptability of M. micrantha across a range of environmental conditions, we hypothesized that although initial introductions of the species would occur within climate conditions similar to those of its native range, the species might occupy novel climatic conditions in its invasive range over time. We tested this hypothesis with the following specific objectives: (1) What were the possible introduction pathways of this species in the invasive range? (2) How did the species spread in its invasive range over time? and (3) How much area in the invasive range was climatically suitable for the species under current and future climate change scenarios? To achieve these objectives, we used an extensive occurrence dataset of M. micrantha covering its native and invasive distribution along with comprehensive climate data. The occurrence dataset was analyzed in the context of major historical events to reconstruct the introduction pathways and climatic niches were characterized for native and invasive ranges to analyze the spread dynamics of M. micrantha. Ensemble modeling framework was used to map potential distribution of the species under projected climate change.
Native and invasive occurrence records of M. micrantha were collected from the Global Biodiversity Information Facility (GBIF) database using search terms “Mikania micrantha Kunth.” Occurrence records lacking spatial and temporal information were removed. We consulted archival records since the eighteenth century (n = 26) and herbarium databases (n = 8) to collect the occurrence records. Each herbarium specimen was checked for possible misidentification (except for digital collections), and information from the label data (including sampling location, year, and habitat characteristics and species identities) was recorded. In addition, an extensive review of the literature (1900–2017) was carried out by consulting several databases and repositories (e.g., CABI invasive species compendium, Pacific Islands Ecosystem at Risk database), and internet search engines like Google Scholar using the keywords: “Mikania,” “invasion,” “invasive plants.” Geographic coordinates were collected from the herbarium records (n = 605) and literature reports (n = 160). We used locality descriptions and Google Maps to georeference the records lacking specific geographic coordinates at a precision level of two decimal degrees. Records that lacked geographic distribution data were not considered in our study. Details of occurrence data sources are listed in Supplementary Table 1. Furthermore, occurrence records collected during our field surveys were also used. Presence records were screened for duplicates and a total of 2,434 occurrence records were kept for native range and 2,225 records for invasive range (Supplementary Table 2).
We downloaded 19 bioclimatic variables from the WorldClim database version 1.4 (http://www.worldclim.org/) (Hijmans et al., 2005), averaged for the 1950–2000 period, at a spatial resolution of 5 arc minutes (approximately 9 km resolution at the equator). For future climate projections, we used four representative concentration pathways (RCPs) of the IPCC—RCP 2.6, 4.5, 6.0, and 8.5, for two future periods−2050 and 2070. We chose three general circulation models (GCMs) of physical climate processes for which the predicted values of each of the bioclimatic variables were available: the Beijing Climate Center Climate System Model (BCC_CSM1.1), the Community Climate System Model (CCSM4) and the Hadley Global Environment Model 2-Atmosphere Ocean (HADGEM2-AO). The selected GCMs were found to perform well in predicting the potential distribution of invasive species and projecting shifts in species distribution under future climate scenarios (Gillard et al., 2017; Ahmad et al., 2019).
We organized our study in two major steps as shown in Figure 1: (1) chronological arrangement of the occurrence datasets and characterization of climatic niche over time, (2) modeling potential distribution by—(2.1) testing model transferability between native and invasive ranges by comparing climatic niches and estimating environmental analogy, (2.2) implementing modeling frameworks and validating them using intrinsic and extrinsic datasets, and (2.3) predicting distribution of M. micrantha in its invasive range under present and future climates. In the following paragraphs, each of these steps was discussed in details.
Figure 1. Framework of the study comprising of four major steps: (i) analysis of climatic niche change over time, (ii) niche characterization and environmental analogy estimation, (iii) development of modeling framework and evaluation, and (iv) mapping potential distribution of Mikania micrantha.
Archival and herbarium reports were consulted to trace the introduction pathways of M. micrantha and to identify the probable sites of introductions in its invasive range. Occurrence records were chronologically arranged and analyzed in the context of major historical events involving importation and exportation relevant to the introduction of alien plant species. Specifically, we considered the geo-political relationships, trade routes, the role of botanical gardens, human perceptions about the species as recorded and anthropogenic movements across countries and regions.
To investigate the temporal climatic niche dynamics of M. micrantha in its invasive range, we performed a PCA on all the climatic variables covering both native and invasive ranges. The package ade4 (Dray and Dufour, 2007) in R was used to perform the PCA. Chronologically arranged occurrences were then projected in the orthogonal environmental space and their scores were predicted along the first two axes (using the predict function in R). For each year, we defined lower and upper niche limits by 2.5 and 97.5 percentiles of scores of the cumulative occurrence records. The niche limits were calculated for years having at least three occurrence records with the assumption that the species did not disappear from a site once colonized. To check climate analogy of the presumed introduction locations with native range, multivariate environmental similarity surface (MESS) (Elith et al., 2010) analysis was carried out. In MESS analysis, the environment of grid cells occupied by the species in the invasive range was compared with that of the native range, with respect to the set of selected bioclimatic variables. The grid cells having positive value indicate similar environment between two ranges whereas grid cells with the dissimilar environment for at least one variable received negative values (Broennimann et al., 2014). We used the dismo package version 1.1-4 (Hijmans et al., 2017) in R to compute the MESS analysis.
To avoid model over-fitting and ensure validity of the statistical analysis, occurrences from the native and invasive ranges were spatially rarefied (using SDMtoolbox 2.3 in ArcMap 10.2.1) by selecting single point per grid cell (cell size of 10 km) (e.g., Brown, 2014). A total of 1,201 occurrence records were kept for native range and 1,437 records for invasive range. Using ade4 package in R 3.4.1 (R Core Team, 2017), we conducted a principal component analysis (PCA) and visualized the correlation between the bioclimatic variables. Seven bioclimatic variables, namely annual mean temperature (Bio1), maximum temperature of the warmest month (Bio5), minimum temperature of the coldest month (Bio6), temperature annual range (Bio7), annual precipitation (Bio12), precipitation seasonality (Bio15) and precipitation of driest quarter (Bio17), were selected based on their non-collinearity and contribution to the overall environmental variation.
Environmental availability and analogy can affect the quantification of the realized niche of a species and thus affect the model projection (Guisan et al., 2014). Therefore, prior to modeling, environmental availability was characterized in form of climatic niches in native and invasive ranges using the COUE framework (Guisan et al., 2014), and the environmental analogy between two ranges was assessed using the ExDet (Mesgaran et al., 2014) analyses.
Following Broennimann et al. (2012), we used a modified Principal Component Analysis (PCA-env) to characterize environmental niches of the two ranges. Smoothed densities of occurrence data were plotted in the gridded environmental space (defined by the first two axes of the PCA built with the bioclimatic variables). Schoener's index of niche breadth (D) was used to estimate observed niche overlap between native and invasive ranges and was statistically evaluated using niche similarity test. Environmental spaces of native and invasive ranges were overlapped to estimate species niches in form of—unfilled niche of native range (U), overlapping niche of both the ranges (O) and expanded niche in the invaded range (E). These analyses were performed using the ecospat package version 3.0 (Broennimann et al., 2018) in R.
To check how much novel (non- analog) climate exists between the native and invasive ranges, a PCA analysis was performed on the selected bioclimatic variables for both areas and their coverage and overlap was visualized in the environmental space. We used ade4 package (Dray and Dufour, 2007) in R to perform these analyses. In addition, ExDet tool (downloaded from http://www.climond.org/exdet) was used to identify two types of novelty between native and invasive ranges for the selected bioclimatic variables. Non-analog environments were identified for individual variables (i.e., outside the range of individual variables of the native niche, type 1 novelty) as well as for novel combinations between variables (i.e., within the univariate range of the native niche but forming novel combinations between variables in the invasive range, type 2 novelty) (Mesgaran et al., 2014).
To generate an ecologically meaningful model background, we first spatially intersected the native occurrence points with the Köppen–Geiger climate layer (available from CliMond database, https://www.climond.org/Koppen.aspx) to identify climate classes where the species is currently occupying (Webber et al., 2011). We restrained the model background to include only those areas with the previously identified climate classes. Following the BAM framework proposed by Barve et al. (2011), we considered the selected model background as the abiotically suitable area where the species could occupy under unlimited dispersal.
A number of algorithms are available to model species distribution and significant variation has been observed among their individual performance (Elith et al., 2010). Therefore, to account for uncertainties in prediction of a single algorithm and to increase the prediction accuracy, we used ensemble modeling approach, implemented in the biomod2 package (Thuiller et al., 2009) in R. To build the ensemble model, we used nine different algorithms (e.g., Marx and Quillfeldt, 2018; Smeraldo et al., 2018; Srivastava et al., 2018): three regression methods [GAM: general additive model (Hastie and Tibshirani, 1990), GLM: general linear model (McCullagh and Nelder, 1989), MARS: multivariate adaptive regression splines (Friedman, 1991)]; three machine learning methods [GBM: generalized boosting model (Ridgeway, 1999), MAXENT: Maximum Entropy (Phillips et al., 2006), RF: random forest (Breiman, 2001)], two classification methods [CTA: classification tree analysis (Breiman, 1984), FDA: flexible discriminant analysis (Hastie et al., 1994)] and one envelope model [SRE: Surface Range Envelop (Busby, 1991)]. To meet the requirement of presence-absence datasets of these models, we generated an equal number of pseudo-absence (PA) points (as available presences) randomly across the model background. Models fitted with equal number of PA points were found to have higher predictive accuracy (Barbet-Massin et al., 2012). We created three sets of PA points to avoid the potential sampling bias of the PA points across the study area.
Invasive occurrence records were randomly partitioned three times to keep aside 20% data (n = 287) for external validation (Guisan et al., 2017). The models were built using complete occurrence records from native range (n = 1,201) and 80% of the invasive occurrence records (n = 1,150) as recent studies reported robust model performance when using combined occurrence records (Broennimann and Guisan, 2008). The models were calibrated using 70% of randomly selected combined occurrence records with 30% data were kept aside for intrinsic model evaluation. The modeling process was replicated four times, thus generating a total of 108 models (9 algorithms x 3 PA datasets x 4 cross-validation runs). Intrinsic validation was conducted using two metrics—true skill statistic (TSS) and the area under the curve (AUC) of receiver operating characteristics (ROC). Individual models with high predictive accuracy (TSS>0.55 and ROC>0.8) were used to build an ensemble model with three ensembling options: mean, committee averaging and weighted mean. The ensemble models were assessed using two metrics: TSS and ROC. The ensemble model projection on invasive range was externally validated for 20% invasive occurrences (n = 287) using the modified Boyce index (Hirzel et al., 2006) implemented in the ecospat package in R. Threshold-independent Boyce index assesses how much the model predictions differ from random expectation and the value varies from −1 to 1 (Boyce et al., 2002). Positive values indicate consistent predictions with the evaluation dataset, values close to zero indicate random predictions, and negative values indicate incorrect predictions (Boyce et al., 2002).
We generated an ensemble model using complete occurrence datasets from both ranges (n = 3,426) and used the ensemble forecasting method (implemented in biomod2 package) to map the predicted distribution of M. micrantha in the invasive range. Minimum training presence (MTP) values obtained by invasive occurrences were used as thresholds to convert the continuous predictions to binary predictions (pixels identified as suitable or unsuitable). Using the pixels having values above MTP, classified suitability maps were generated based on omission percentage of occurrence points: 10% omission (low suitability), 10–25% omission (medium suitability), 25–50% (high suitability) and >50% (very high suitability) (Mukherjee et al., 2011).
To identify the range change of the species under future climate conditions, we used binary predictions generated by using the MTP values as thresholds. Raster overlay analysis was conducted with the binary predictions from three GCMs and a combined raster was produced based on prediction analogy. The combined raster was then overlain with the current binary prediction to identify range change. ArcMap 10.2.1 was used for these analyses. Risk assessment of M. micrantha was further conducted based on numbers of biodiversity hotspots (Myers et al., 2000) and terrestrial ecoregions (Olson and Dinerstein, 2002) to be affected by its predicted distribution under current and future climate change scenarios.
Analysis of archival literature and herbarium catalogs revealed multiple introduction pathways for this species (Table 1): outside its native range, M. micrantha was first recorded from Luzon province of the Philippines in 1838. From the Philippines, the species was introduced to the Malay Peninsula of undivided British India at the end of the nineteenth century, and subsequently to northeast India (route 1); introduction through botanical gardens took place in Hong Kong (route 2) and Kolkata (route 3) at the end of the nineteenth century from where the species started spreading in southern China and eastern India respectively; in early 1900, M. micrantha was recorded from Fiji from where it was introduced to Oceanic islands and in PNG in around 1960, and from PNG to Australia at the end of the Twentieth century (route 4); the species was introduced to Indonesia and Malaysia in early 1950 (route 5) and latest introduction reports in early 2010 came from the USA (route 6).
Table 1. Presumed invasion pathways of Mikania micrantha and the initial occurrence records from its invasive range.
Climatic niche dynamics of M. micrantha at a temporal scale (Figures 2A,B) showed that climate conditions at the presumed introduction sites along PCA axis 1 (lower niche limit = 0.39, upper niche limit = 5.29) matched conditions found within the realized climate niche limit of the species in its native range (range: −2.39 to 5.78). The climate conditions of the initial introduction sites along PCA axis 2 were also found to be within limits of the native climatic niche (range: −4.4 to 1.99), except the introduction location in Kolkata of eastern India (2.61). All sites of initial introductions showed positive values in the MESS analysis (Figure 2C; Supplementary Table 3). However, considerable variation in apparent lag and initial spread was observed among six introduction routes. The populations introduced through route 1, 2, and 4 remained confined to a few populations for nearly 100 years (lag phase) whereas shorter lag phase was recorded for the populations introduced via route 3 (60 years) and route 5 (40 years). From its initial introduction sites, the species started spreading to new locations (initial spread phase) and it was only after 2000 when range expansion was observed for all introduction pathways. Interestingly, the populations introduced and spread via Fiji (route 4) and Indonesia (route 5) spread outside of the realized native niche, toward cold climate conditions in Papua New Guinea (PNG) and southern India, as evident from the PCA axis 1 values for PNG in 1974 (7.08) and southern India in 1985 (7.03) (Figure 2A). On the other hand, PCA axis 2 values revealed that populations introduced through route 1 started spreading toward dry conditions in northeast India (3.96), Myanmar (2.13), Nepal (2.21) and Thailand (2.79) since 2000 (Figure 2B).
Figure 2. Climatic niche dynamics of M. micrantha since introduction along environmental (A,B) and geographical (C) space: (A) Change in the realized climatic niche along first principal component analysis (PCA) axis (temperature gradient); (B) Change in the realized niche along second PCA axis (humidity) gradient. Straight lines indicate upper and lower niche limits of the invasive occurrences. Different colors of the triangles and vertical lines indicate 6 presumed introductions and spread routes of the species in its invasive range: route 1(black), 2 (red) 3 (blue), 4 (pink), 5 (green), and 6 (yellow). Triangles show the climatic niche values at the introduction sites whereas the vertical lines denote climatic niche values of the invasive occurrence locations. The stacked bars at the left show lower and upper niche limit of the species whereas the gray areas highlight the realized climatic niche of the species in its native range. (C) Multivariate environmental similarity surface (MESS) analysis of South and Southeast Asia, Oceania and Australia (lower panel) and the USA (upper panel); dark green areas indicate similar climate conditions with native range and the light green-blue gradient indicates the degree of dissimilarity with the native climate. Blue dots denote the initial introduction sites in the invasive range.
The first two PCA axes explained maximum environmental variation between native and invasive ranges (74.3%) (Table 2). The three variables that contributed most in explaining the variation in the native occurrence data were the minimum temperature of the coldest month (Bio6), mean temperature of coldest quarter (Bio11) and mean temperature of the driest quarter (Bio9) (Table 2). The hypothesis of retained niche similarity was accepted (p = 0.5) indicating that climatic niches in the two ranges were more similar than would be expected at random. High amount of overlap (D = 0.678) was observed between native and invasive ranges. High value of niche stability (O = 0.9997) along with low expansion (E = 0.0003) and unfilling (U = 0.132) indicated that M. micrantha occupied most of its native niche in its invasive range (Figure 3A). PCA analysis of the selected bioclimatic variables revealed that the differences between the two regions are minor (Figure 3B). From the ExDet map it was evident that majority of the invasive range was inside the univariate range of climate covariates of the native niche (green in Figure 3C) whereas the NT1 component (marked red in Figure 3C) was restricted to the northern part of China, the USA and central to west India. The most influential covariate (MIC) map revealed that the novel univariate range (i.e., NT1) in northern China and the USA was driven by the temperature annual range (Bio7) whereas minimum temperature of the coldest month (Bio6) contributed to the NT1 novelty in northwest China and Alaska in the USA (Figure 3D).
Table 2. Loadings on two axes of the principal component analysis (PCA) of the bioclimatic variables.
Figure 3. Characterization of climatic niche and environmental analogy: (A) Visualization of climatic niches of M. micrantha in native and invasive ranges. The green colored areas correspond to the unfilled zone, blue areas represent the overlap zone of the two niches, and the red areas delineate the expansion zone; red arrows represent how the center of the niche has changed between the two ranges. Green and red contour lines delineate the available niche in native and invasive ranges respectively; (B) Differences in the ecological space of M. micrantha between native (red) and invasive (blue) ranges as mapped in the geographic space (left panel) and environmental space (right panel) based on selected bioclimatic variables; (C) The ExDet map of the extrapolated projection areas; green colored areas indicate similar climatic condition between native and invasive ranges whereas the Type 1 novelty (red) indicates areas in the invasive range with at least one bioclimatic variable outside the univariate range of the native range; (D) Most influential covariate (MIC) map showing the spatial distribution of the dissimilar covariates in terms of their contribution to Type 1 novelty whereas blue areas have similar environment with that of native range.
All the individual modeling algorithms had ROC values above 0.8 and TSS values above 0.5 with RF models appeared to be the most accurate on average. The ensemble models performed well as evident from high evaluation scores (>0.7) for all three metrics. Weighted mean provided better evaluation than mean and committee averaging models, so external validation with the 20% invasive occurrence data was performed on the weighted mean model forecasts. Positive Boyce index (Spearman's rank correlation coefficient >0.9) values confirmed model projection accuracy (Supplementary Table 4).
Under present climatic conditions, nearly 20% of the total invasive range was found to be climatically suitable for M. micrantha (Figure 4) covering 39 terrestrial ecoregions belonging to 8 biogeographical realms and 7 biomes along with 10 biodiversity hotspots (Supplementary Table 5). In South Asia, the Western Ghats of south India, parts of northeast India, eastern parts of Vietnam and Laos, southern China and Taiwan were predicted to be climatically suitable for M. micrantha (Figure 4A). Among Southeast Asian countries, northern Philippines, and parts of south and west Indonesia had low to high climatic suitability (Figure 4B). Most of Papua New Guinea and northeast Australia along with the Oceanic islands were climatically suitable for M. micrantha growth (Figure 4D). In the western hemisphere, the potential distribution of M. micrantha was restricted to Florida of the USA (Figure 4C).
Figure 4. Projected distribution of M. micrantha on—(A) South Asia, (B) Southeast Asia, (C) the USA, and (D) Australia and Oceania with current climate condition. Legends depict five climate suitability classes based on thresholds calculated in terms of minimum training presence of known occurrences and the ecoregions prioritized for conservation have been delineated.
For all the three GCMs, increase in percentage of climatically suitable region was observed by 2050 and 2070 with respect to current climate conditions (Figure 5). However, with respect to 2050, percentage of climatically suitable region was decreased in 2070 under RCP 4.5 and 2.6 (except BCC_CSM1.1) whereas under RCP 8.5 and 6.0 (except CCSM4), percentage of climatically suitable region was increased across the three GCM scenarios. Under projected climate change, suitable climates for M. micrantha were likely to shift geographically (Figure 6), covering parts of 5 additional terrestrial ecoregions (Supplementary Table 5). Parts of central India, Myanmar, Thailand, China and Australia were found to become climatically suitable under RCP 2.6 (Figures 6A,B) and RCP 8.5 (Figures 6E,F). In the western hemisphere, novel climatic suitability for M. micrantha was observed in the coastal areas of southern and western USA under RCP 2.6 (Figures 6C,D) and RCP 8.5 (Figures 6G,H). Similar pattern of range expansion was also observed for RCP 4.5 and RCP 6.0 (Supplementary Figure 1).
Figure 5. Percentage of climatically suitable region for M. micrantha with current climatic conditions, and for 2050 and 2070 under two RCPs with the three GCM scenarios—the Beijing Climate Center Climate System Model (BCC_CSM1.1), the Community Climate System Model (CCSM4), the Hadley Global Environment Model 2-Atmosphere Ocean (HADGEM2-AO; and the combined prediction for three GCMs.
Figure 6. Overlay maps showing changes in predicted distributions of M. micrantha according to RCP 2.6 (A–D) and RCP 8.5 (E–H) with the combined GCM scenario—changes in predicted distributions in South and Southeast Asia, Australia and Oceania by 2050 (A,E) and by 2070 (B,F); and in the USA by 2050 (C,G) and by 2070 (D,H).
Amidst the speculative introduction history of M. micrantha in most of its invasive range countries, our study revealed the possibility of multiple introduction pathways of this species. Chronological analysis of the historical evidence indicates that outside of its native range, M. micrantha was first introduced into the Philippines at the end of the nineteenth century, probably through the Pacific trade routes between the European countries and Latin America with the Philippines as an important trading post (Legarda, 1999). For example, through the Manila galleon trade route, Spanish trading ships made round-trip sailing voyages from the port of Acapulco in New Spain (present-day Mexico) to Manila in the Spanish East Indies (present-day Philippines). Earlier reports also highlighted the possibility of M. micrantha introduction into the Philippines from Mexico (Cock et al., 2000), though the report could not be substantially evaluated. The species started spreading from the Philippines to the Malay Peninsula, as evident from a number of floristic records of that time from that region. Our analysis suggested that the species was introduced to northeast India through this route, which is in contradiction with previous studies suggesting that the species was introduced into northeast India during the 2nd World War (1939–1945) to camouflage airfields (Tripathi et al., 2012; Ellison and Sankaran, 2017). The earliest herbarium record of M. micrantha from this region (of 1909) was before the 2nd World War which indicates that the species was introduced in this region in around 1900.
Botanical gardens played an important role in the introduction of alien invasive plant species worldwide (van Kleunen et al., 2018). M. micrantha was possibly introduced in the botanical gardens of Kolkata and Hong Kong by the end of the nineteenth century when botanical knowledge was recognized as an integral component of the imperial expansion of the British (Cronk and Fuller, 2001). M. micrantha has been used as a folk medicine for anti-bacterial activity in Jamaica (Facey et al., 1999) from where it was introduced into the Kew botanical garden in 1886 for cultivation purpose (Supplementary Table 1). Among the three species of Mikania suggested for cultivation in the Calcutta Botanical Garden (Spry, 1839), Mikania guaco Kunth is morphologically similar to M. micrantha [as evident from that fact that one of the common names of M. micrantha was “falso guaco” (Duke et al., 2008)]. Therefore, it is likely that M. micrantha (= M. guaco) was introduced from Jamaica to Calcutta Botanical Garden through the Kew Botanical Garden. Several authors presumed that Mikania was present in the Kolkata Botanical Garden in early 1900, and its presence was also attributed to the Kew Botanical Garden (Choudhury, 1972; Cock et al., 2000).
Another introduction of M. micrantha took place in Fiji in 1900; although the introduction history in this region is still obscure (Macanawai et al., 2012). Introduction of M. micrantha took place as ground cover for rubber plantation in Indonesia and Malaysia in around 1950 (Teoh et al., 1985), from where it started spreading in Southeast Asian countries like Singapore, Sri Lanka and southwest India. In 2011, the species was introduced to the USA where the distribution of the species was recorded from disturbed areas such as roadsides, woodlots and in plant nurseries in Florida (Manrique et al., 2011). In conjunction, these findings are indicative of multiple introductions of M. micrantha in its invasive range.
The initial introductions of M. micrantha took place within the analog climatic niche of the species in its native range, thereby supporting the hypothesis that initial introductions of an invasive species took place within the similar climate conditions of its native range. However, our study revealed that the species is not at equilibrium with the current environmental conditions of its invasive range (Václavík and Meentemeyer, 2012) as it has been occupying new climatic niches over time. The shift of the realized climatic niche of M. micrantha toward cold and dry conditions in its invasive range is not surprising since the bioclimatic variables related to cold temperature (Bio6, Bio11) and temperature of the dry months (Bio9) made significant contributions in governing the distribution of this species. Previous studies have revealed that M. micrantha can establish across a range of environmental conditions due to phenotypic variation of physiological traits (Banerjee et al., 2017a). For example, experimental studies have found enhanced NAC gene expression in response to cold stress (Li et al., 2012) and phenotypic plasticity in traits in different altitudinal gradient (Prabu et al., 2014), to elevated CO2 concentration (Song et al., 2009), and to contrasting light and soil water conditions (Zhang and Wen, 2009) in M. micrantha. These properties may help the species to occupy different habitat conditions in its invasive range and probably explain the occupancy of novel climate conditions outside its native niche. The ability of successful establishment of M. micrantha across a range of environmental conditions is also evident from the high amount of niche stability between native and invasive ranges. This finding is indicative that the species has adapted to unique environmental conditions encountered in its invasive range, and in agreement with other studies which showed absence of climatic niche shift in terrestrial invaders (Petitpierre et al., 2012).
However, intraspecific variation in spread dynamics has been found among different introduction pathways of this species, as observed in other invaders like spotted knapweed in the USA (Broennimann et al., 2014). While the inherent ecological and evolutionary processes may be responsible for the time between introduction and range expansion of an invasive species, multiple factors have been identified which can influence the observed lag and spread dynamics of an invasive species (Daehler, 2009). Firstly, the range expansion of M. micrantha population introduced through Philippines and the Malay Peninsula probably started since 1960 through the tea trading routes. M. micrantha is recognized as an important weed in tea plantations in northeast India (Sankaran et al., 2008; Puzari et al., 2010) which constitute one of the largest tea growing regions of the world. Therefore, multiple tea trading routes possibly aided in its spread to adjoining states and countries like Nepal and Bhutan. However, the significantly longer lag period observed for the populations introduced through this route (i.e., more than 100 years) could also be due to detection lag (Crooks, 2011) when the species was restricted to small populations, or because of inconsistent and coarse-scale historical information (Daehler, 2009) collected during this time period. This temporal variation in sampling efforts may also explain the lag phase observed for the populations introduced through Fiji. After introduction to Fiji in 1900, the species started spreading to oceanic islands from 1930 (Day et al., 2016), probably through European settlement wave (Jupiter et al., 2014). However, the observed lag phase, spanning more than 80 years, could likely be due to lack of reliable quantitative data on species diversity and distributions and inadequate sampling effort in the island countries (Keppel et al., 2014).
Secondly, although intentionally introduced through botanical gardens (route 2 and 3), the difference in the lag period between Kolkata (60 years) and Hong Kong (100 years) populations of M. micrantha might be explained on the basis of trade activities of that time period. Kolkata, being one of the major cities of undivided British India, was well connected to adjoining states through trade and transport networks which facilitated the rapid spread of M. micrantha to the surrounding areas (Haines, 1925). On the other hand, the industrialization of Hong Kong accelerated since 1950 after the establishment of the People's Republic of China in 1949 (https://bit.ly/2JttdOH; accessed on 31.05.2019). Newly developed trade relationships and transport networks between these two regions probably aided the spread of M. micrantha from Hong Kong to its neighboring city Shenzhen in mainland China in around 1984.
Finally, the populations introduced through Indonesia and Malaysia started spreading in Southeast Asian countries through rubber trading routes operational between these countries. Introduction of a species from a region where it is already naturalized has been found to increase fitness of the introduced populations, thereby accelerating its establishment and dispersal in the introduced range. This probably explains the shortest lag period (35 years) observed for these populations which were presumably introduced from the Indonesia and Malaysia where the species had already naturalized. This kind of increased fitness for the secondarily introduced population (O'Loughlin and Green, 2017) has also been observed for other invasive species. For example, in a study evaluating the role of propagule origin (non-native vs. native) on invasion success of Centaurea solstitialis, it was found that seeds of the non-native populations from California reached higher abundance than seeds of the native population collected from Spain (Xiao et al., 2016). However, the short lag period may also be due to increased noticeability for its impacts on rubber plantations. This lag phase is within the range of lag times (50 years) observed for many tropical invasive plant species (Daehler, 2009).
Given that the species is occupying new climatic niches in its invasive range, the modeled distribution combining native and invasive occurrence data provided a robust estimate of the potential distribution of M. micrantha under current and future climate conditions. The observed pattern of realized climatic niche movement toward dry and cold areas of its invasive range is also evident from the climatic suitability and occurrence of the species in the temperate broadleaf and mixed forest biome which is present but unoccupied in its native range (Banerjee et al., 2017b). This shift in biome occupancy between native and invasive ranges has been recorded in other invasive species as well (Gallagher et al., 2010). Based on a robust estimate of the potential distribution of this species under three GCMs, our study revealed predicted a potential increase of suitable habitat in dry and cold areas of central India, southern China and northern Australia. This pattern of range expansion is consistent with the studies regarding the impact of climate change (Liu et al., 2017; Akin-Fajiye and Gurevitch, 2018; Thapa et al., 2018), and on M. micrantha as well (Shrestha et al., 2018). Interestingly, the effects of climate change on species distribution was more evident in mainland countries, as oceanic barriers might hinder species movements following climate change in the Oceanic islands (Bellard et al., 2018). In addition, fresh introductions are being reported from new areas (e.g., in the USA; Manrique et al., 2011) where the species has not yet reached all of its suitable environments and management initiatives are controlling the spread of this species in certain areas. For example, our model found high climatic suitability for M. micrantha's invasion to the coastal regions of eastern Australia. We did not find records from that region, and it is likely that effective management and strong quarantine measures have reduced the spread of this species since its introduction in 1998 (Brooks et al., 2008). In this context, our study does not reflect the current distribution of the species in its invasive range, rather highlights the risk of new areas to be colonized.
We believe that the exhaustive and robust occurrence dataset used in this study ensured good precision for tracing the introduction pathways, mapping temporal spread and modeling potential distribution of M. micrantha in its invasive range. This is the first study that identifies multiple introduction pathways for M. micrantha in its invasive range, and highlights spatio-temporal variation of the spread dynamics among the invasion pathways. Our study also revealed that nearly 30% of the terrestrial ecoregions (among those which have been recognized for priority conservation) and nearly half of the global biodiversity hotspots are vulnerable for the invasion of this species. Predicted range expansion under future climate change scenarios further highlights the need for effective management of potential new suitable habitat. In this perspective, the findings of our study provide valid support for developing management strategies for M. micrantha invasion, e.g., by designing early detection and rapid response initiatives, implementing quarantine measures and devising scientifically informed site-specific management policies. Taking clues from the successful management strategies implemented so far, an inter-regional approach to research and management of M. micrantha invasion should be prioritized (Clements et al., 2019).
Although this framework is applicable for other invasion scenarios, we identified scopes of further improvements of these approaches and addressed these uncertainties for consideration in future endeavors. Firstly, herbarium and archival records on which the temporal spread has been estimated, are often suffered from multiple biases (Delisle et al., 2003). Although consultation of key herbaria across its invasive range took care of these factors to some extent, data from local herbaria might provide finer-scale information of introduction and spread of M. micrantha in its invasive range. The evidence introductions should be strengthened with future population genetics studies using both nuclear and chloroplast DNA primers (Williams et al., 2005; Oduor et al., 2015). Identification of the invasion source may also help in selecting the optimal classical biological control agent(s) (Richmond et al., 2015; Sun et al., 2017), which will aid the effective management of this species. Secondly, our study indicated that detection lag and species responsiveness to a range of environmental conditions might be responsible for the observed variation in climatic niche dynamics among the invasion pathways of M. micrantha. However, further experimental studies, e.g., common garden and reciprocal transplant involving species from multiple demes, may provide important information on local adaptation and phenotypic variation across environmental gradients (Kawecki and Ebert, 2004; Davidson et al., 2011). These findings may provide useful insights into the intraspecific variation in lag time and climatic niche change over time. Finally, climatic niche change over time. Finally, projections of species distribution are limited by the assumption of climatic variables as the only drivers of change, whereas potential distribution can be influenced by both biotic (e.g., species interactions, anthropogenic influence on spread) and other abiotic factors (e.g., land use, topography; Bellard et al., 2013). Including these predictor variables to the models might improve the spatial accuracy of the predictions. Since these variables may influence species distribution at a local scale where climate is not a limiting factor (Bellard et al., 2018), assessment of the effects of climate change at country level may provide deeper insights in the distribution pattern under future climate scenarios (e.g., Kelly et al., 2014).
All datasets generated for this study are included in the manuscript/Supplementary Files.
AB, AM, and YH conceived the idea and AB and AM designed the study. AB, WG, and YL collected the data. AB, WG, and YH analyzed the data and interpreted the results. AB, AM, and YH wrote the manuscript text. All authors reviewed the manuscript and have approved the final version of the article.
This study is supported by grants from the National Natural Science Foundation of China (Grant Nos. 41776166 and 31700178), Special Fund for Science and Technology Development of Guangdong Province (2017A030303014), the Natural Science Foundation of Guangdong Province (2017A030313159), the Science Foundation of the State Key Laboratory of Biocontrol (32-2017-A30), the Science and Technology Program of Guangzhou (Grant No. 201707020035) and the Chang Hungta Science Foundation of Sun Yat-sen University.
The authors declare that the research was conducted in the absence of any commercial or financial relationships that could be construed as a potential conflict of interest.
We would like to thank Sun Yat-sen University for necessary funding and logistical support for this research. We also acknowledge the help and support received from the following individuals in collecting occurrence data of the species: Lan Wentao from South China Agricultural University; Lin Yuting and Li Guanghe from Sun Yat-sen University (China); and Dr. K. V. Sankaran, Dr. P. Sujanapal, Sumod, and Kartik from Kerala Forest Research Institute; Prof. Anjana Dewanji, Sandip Chatterjee, Susant Mahankur, and Sandip Mondal from Indian Statistical Institute; Dr. Keshab Puzari and Dr. Pranab Dutta from Assam Agricultural University (India). The authors are thankful to the editor and two reviewers for their valuable comments and suggestions which have immensely improved the manuscript quality.
The Supplementary Material for this article can be found online at: https://www.frontiersin.org/articles/10.3389/fevo.2019.00291/full#supplementary-material
Supplementary Figure 1. Overlay maps showing changes in predicted distributions of M. micrantha according to RCP 4.5 (A–D) and RCP 6.0 (E–H) with the combined GCM scenario - changes in predicted distributions in South and Southeast Asia, Australia and Oceania by 2050 (A,E) and by 2070 (B,F); and in the USA by 2050 (C,G) and by 2070 (D,H).
Ahmad, R., Khuroo, A. A., Hamid, M., Charles, B., and Rashid, I. (2019). Predicting invasion potential and niche dynamics of Parthenium hysterophorus (Congress grass) in India under projected climate change. Biodiversity Conserv. 28, 2319–2344. doi: 10.1007/s10531-019-01775-y
Akin-Fajiye, M., and Gurevitch, J. (2018). The influence of environmental factors on the distribution and density of invasive Centaurea stoebe across Northeastern USA. Biol. Invas. 20, 3009–3023. doi: 10.1007/s10530-018-1755-7
Atwater, D. Z., Ervine, C., and Barney, J. N. (2018). Climatic niche shifts are common in introduced plants. Nat. Ecol. Evol. 2, 34–43. doi: 10.1038/s41559-017-0396-z
Banerjee, A. K., Ghosh, S., and Dewanji, A. (2017a). Do plants have a choice of traits to be modulated? Evidence from an invasive plant Mikania micrantha Kunth in different urban environments. Am. J. Plant Sci. 8, 835–855. doi: 10.4236/ajps.2017.84057
Banerjee, A. K., Mukherjee, A., and Dewanji, A. (2017b). Potential distribution of Mikania micrantha Kunth in India – evidence of climatic niche and biome shifts. Flora 234, 215–223. doi: 10.1016/j.flora.2017.08.001
Barbet-Massin, M., Jiguet, F., Albert, C. H., and Thuiller, W. (2012). Selecting pseudo-absences for species distribution models: how, where and how many? Methods Ecol. Evol. 3, 327–338. doi: 10.1111/j.2041-210X.2011.00172.x
Barve, N., Barve, V., Jiménez-Valverde, A., Lira-Noriega, A., Maher, S. P., Peterson, A. T., et al. (2011). The crucial role of the accessible area in ecological niche modeling and species distribution modeling. Ecol. Modell. 222, 1810–1819. doi: 10.1016/j.ecolmodel.2011.02.011
Bellard, C., Jeschke, J. M., Leroy, B., and Mace, G. M. (2018). Insights from modeling studies on how climate change affects invasive alien species geography. Ecol. Evol. 8, 5688–5700. doi: 10.1002/ece3.4098
Bellard, C., Rysman, J. F., Leroy, B., Claud, C., and Mace, G. M. (2017). A global picture of biological invasion threat on islands. Nat. Ecol. Evol. 1, 1862–1869. doi: 10.1038/s41559-017-0365-6
Bellard, C., Thuiller, W., Leroy, B., Genovesi, P., Bakkenes, M., and Courchamp, F. (2013). Will climate change promote future invasions? Global Change Biol. 19, 3740–3748. doi: 10.1111/gcb.12344
Blackburn, T. M., Lockwood, J. L., and Cassey, P. (2015). The influence of numbers on invasion success. Mol. Ecol. 24, 1942–1953. doi: 10.1111/mec.13075
Bosso, L., De Conno, C., and Russo, D. (2017). Modelling the risk posed by the Zebra Mussel Dreissena polymorpha: Italy as a case study. Environ. Manage. 60, 304–313. doi: 10.1007/s00267-017-0882-8
Boyce, M. S., Vernier, P. R., Nielsen, S. E., and Schmiegelow, F. K. A. (2002). Evaluating resource selection functions. Ecol. Model. 157, 281–300. doi: 10.1016/S0304-3800(02)00200-4
Briscoe Runquist, R. D., Lake, T., Tiffin, P., and Moeller, D. A. (2019). Species distribution models throughout the invasion history of Palmer amaranth predict regions at risk of future invasion and reveal challenges with modeling rapidly shifting geographic ranges. Sci. Rep. 9:2426. doi: 10.1038/s41598-018-38054-9
Broennimann, O., Cola, V. D., and Guisan, A. (2018). ecospat: Spatial Ecology Miscellaneous Methods. R package version 3.0.
Broennimann, O., Fitzpatrick, M. C., Pearman, P. B., Petitpierre, B., Pellissier, L., Yoccoz, N. G., et al. (2012). Measuring ecological niche overlap from occurrence and spatial environmental data. Global Ecol. Biogeogr. 21, 481–497. doi: 10.1111/j.1466-8238.2011.00698.x
Broennimann, O., and Guisan, A. (2008). Predicting current and future biological invasions: both native and invaded ranges matter. Biol. Lett. 4, 585–589. doi: 10.1098/rsbl.2008.0254
Broennimann, O., Mráz, P., Petitpierre, B., Guisan, A., and Müller-Schärer, H. (2014). Contrasting spatio-temporal climatic niche dynamics during the eastern and western invasions of spotted knapweed in North America. J. Biogeogr. 41, 1126–1136. doi: 10.1111/jbi.12274
Broennimann, O., Treier, U. A., Müller-Schärer, H., Thuiller, W., Peterson, A. T., and Guisan, A. (2007). Evidence of climatic niche shift during biological invasion. Ecol. Lett. 10, 701–709. doi: 10.1111/j.1461-0248.2007.01060.x
Brooks, S. J., Panetta, F. D., and Galway, K. E. (2008). Progress towards the eradication of mikania vine (Mikania micrantha) and limnocharis (Limnocharis flava) in northern Australia. Invas. Plant Sci. Manage. 1, 296–303. doi: 10.1614/IPSM-08-067.1
Brown, J. L. (2014). SDMtoolbox: a python-based GIS toolkit for landscape genetic, biogeographic and species distribution model analyses. Methods Ecol. Evol. 5, 694–700. doi: 10.1111/2041-210X.12200
Busby, J. R. (1991). “BIOCLIM–A bioclimate analysis and prediction system,” in Nature Conservation: Cost Effective Biological Surveys and Data Analysis, eds. C. R. Margules M. P. Austin (Sydney, NSW: CSIRO, 64–68.
Callaway, R. M., and Maron, J. L. (2006). What have exotic plant invasions taught us over the past 20 years? Trends Ecol. Evol. 21, 369–374. doi: 10.1016/j.tree.2006.04.008
Chapman, D. S., Makra, L., Albertini, R., Bonini, M., Páldy, A., Rodinkova, V., et al. (2016). Modelling the introduction and spread of non-native species: international trade and climate change drive ragweed invasion. Global Change Biol. 22, 3067–3079. doi: 10.1111/gcb.13220
Choudhury, A. (1972). Controversial Mikania (Climber)-a threat to the forests and agriculture. Ind. Forester 98, 178–186.
Choudhury, M. R., Deb, P., Singha, H., Chakdar, B., and Medhi, M. (2016). Predicting the probable distribution and threat of invasive Mimosa diplotricha Suavalle and Mikania micrantha Kunth in a protected tropical grassland. Ecol. Eng. 97, 23–31. doi: 10.1016/j.ecoleng.2016.07.018
Clements, D. R., Day, M. D., Oeggerli, V., Shen, S. C., Weston, L. A., Xu, G. F., et al. (2019). Site-specific management is crucial to managing Mikania micrantha. Weed Res. 59, 155–169. doi: 10.1111/wre.12359
Cock, M. J., Ellison, C. A., Evans, H. C., and Ooi, P. A. (2000). “Can failure be turned into success for biological control of mile-a-minute weed (Mikania micrantha),” in Proceedings of the X International Symposium on Biological Control of Weeds, ed N. R. Spencer (Bozeman, MN: Montana State University).
Cronk, Q. C. B., and Fuller, J. L. (2001). Plant Invaders: The Threat to Natural Ecosystems. London: Earthscan Publications.
Crooks, J. A. (2011). “Lag times,” in Encyclopedia of Biological Invasions, eds. D. Simberloff and M. Rejmanek. (California, CA: University of California Press, 404–410.
Daehler, C. C. (2009). Short lag times for invasive tropical plants: evidence from experimental plantings in Hawai'i. PLoS ONE 4:e4462. doi: 10.1371/journal.pone.0004462
Davidson, A. M., Jennions, M., and Nicotra, A. B. (2011). Do invasive species show higher phenotypic plasticity than native species and, if so, is it adaptive? A meta-analysis. Ecol. Lett. 14, 419–431. doi: 10.1111/j.1461-0248.2011.01596.x
Day, M. D., Clements, D. R., Gile, C., Senaratne, W. K., Shen, S., Weston, L. A., et al. (2016). Biology and impacts of pacific islands invasive species. 13. Mikania micrantha Kunth (Asteraceae). Pacific Sci. 70, 257–285. doi: 10.2984/70.3.1
Delisle, F., Lavoie, C., Jean, M., and Lachance, D. (2003). Reconstructing the spread of invasive plants: taking into account biases associated with herbarium specimens. J. Biogeogr. 30, 1033–1042. doi: 10.1046/j.1365-2699.2003.00897.x
Deng, X., Ye, W.-H., Feng, H.-L., Yang, Q.-H., Hong-Ling, C., Xu, K.-Y., et al. (2004). Gas exchange characteristics of the invasive species Mikania micrantha and its indigenous congener M. cordata (Asteraceae) in South China. Bot. Bull. Acad. Sin. 45, 213–220.
Dietz, H., and Edwards, P. J. (2006). Recognition that causal processes change during plant invasion helps explain conflicts in evidence. Ecology 87, 1359–1367. doi: 10.1890/0012-9658(2006)87[1359:RTCPCD]2.0.CO;2
Dray, S., and Dufour, A. (2007). The ade4 package: implementing the duality diagram for ecologists. J. Stat. Softw. 22, 1–20. doi: 10.18637/jss.v022.i04
Duke, J. A., MJ, B.-G., and O, A. R. (2008). Duke's Handbook of Medicinal Plants of Latin America. Boca Raton, FL: CRC Press.
Early, R., Bradley, B. A., Dukes, J. S., Lawler, J. J., Olden, J. D., Blumenthal, D. M., et al. (2016). Global threats from invasive alien species in the twenty-first century and national response capacities. Nat. Commun. 7:12485. doi: 10.1038/ncomms12485
Elith, J. (2017). “Predicting distributions of invasive species,” in Invasive Species: Risk Assessment and Management, eds. A. P. Robinson, T. Walshe, M. A. Burgman and M. Nunn (Cambridge University Press), 93–129.
Elith, J., Graham, C. H., Anderson, R. P., Dudik, M., Ferrier, S., Guisan, A., et al. (2006). Novel methods improve prediction of species' distributions from occurrence data. Ecography 29, 129–151. doi: 10.1111/j.2006.0906-7590.04596.x
Elith, J., Kearney, M., and Phillips, S. (2010). The art of modelling range-shifting species. Methods Ecol. Evol. 1, 330–342. doi: 10.1111/j.2041-210X.2010.00036.x
Ellison, C. A., and Sankaran, K. V. (2017). “Profile of an invasive plant: Mikania micrantha,” in Invasive Alien Plants: Impacts on Development and Options for Management, eds C. A. Ellison, K. V. Sankaran and S. T. Murphy (Wallingford: CABI). 18–28.
Facey, P. C., Pascoe, K. O., Porter, R. B., and Jones, A. D. (1999). Investigation of plants used in Jamaican folk medicine for anti-bacterial activity. J. Pharm. Pharmacol. 51, 1455–1460. doi: 10.1211/0022357991777119
Franco, J. N., Tuya, F., Bertocci, I., Rodríguez, L., Martínez, B., Sousa-Pinto, I., et al. (2018). The ‘golden kelp’ Laminaria ochroleuca under global change: Integrating multiple eco-physiological responses with species distribution models. J. Ecol. 106, 47–58. doi: 10.1111/1365-2745.12810
Friedman, J. H. (1991). Multivariate adaptive regression splines. Ann. Stat. 19, 1–67. doi: 10.1214/aos/1176347963
Gallagher, R. V., Beaumont, L. J., Hughes, L., and Leishman, M. R. (2010). Evidence for climatic niche and biome shifts between native and novel ranges in plant species introduced to Australia. J. Ecol. 98, 790–799. doi: 10.1111/j.1365-2745.2010.01677.x
Geng, S.-L., Chen, Q., Cai, W.-L., Cao, A.-C., and Ou-Yang, C.-B. (2017). Genetic variation in the invasive weed Mikania micrantha (Asteraceae) suggests highways as corridors for its dispersal in southern China. Ann. Bot. 119, 457–464. doi: 10.1093/aob/mcw218
Gillard, M., Thiébaut, G., Deleu, C., and Leroy, B. (2017). Present and future distribution of three aquatic plants taxa across the world: decrease in native and increase in invasive ranges. Biol. Invas. 19, 2159–2170. doi: 10.1007/s10530-017-1428-y
Grewell, B. J., Castillo, J. M., Skaer Thomason, M. J., and Drenovsky, R. E. (2016). Phenotypic plasticity and population differentiation in response to salinity in the invasive cordgrass Spartina densiflora. Biol. Invas. 18, 2175–2187. doi: 10.1007/s10530-015-1041-x
Guisan, A., Petitpierre, B., Broennimann, O., Daehler, C., and Kueffer, C. (2014). Unifying niche shift studies: insights from biological invasions. Trends Ecol. Evol. 29, 260–269. doi: 10.1016/j.tree.2014.02.009
Guisan, A., Thuiller, W., and Zimmermann, N. E. (2017). Habitat Suitability and Distribution Models with Applications in R. Cambridge, UK: Cambridge University Press.
Hällfors, M. H., Liao, J., Dzurisin, J., Grundel, R., Hyvärinen, M., Towle, K., et al. (2016). Addressing potential local adaptation in species distribution models: implications for conservation under climate change. Ecol. Appl. 26, 1154–1169. doi: 10.1890/15-0926
Hastie, T., Tibshirani, R., and Buja, A. (1994). Flexible discriminant analysis by optimal scoring. J. Am. Stat. Assoc. 89, 1255–1270. doi: 10.1080/01621459.1994.10476866
Hastie, T. J., and Tibshirani, R. J. (1990). Generalized Additive Models. New York, NY: Chapman and Hall.
Hijmans, R. J., Cameron, S. E., Parra, J. L., Jones, P. G., and Jarvis, A. (2005). Very high resolution interpolated climate surfaces for global land areas. Int. J. Climatol. 25, 1965–1978. doi: 10.1002/joc.1276
Hijmans, R. J., Phillips, S., Leathwick, J., and Elith, J. (2017). dismo: Species Distribution Modeling. R package version 1.1–4.
Hirzel, A. H., Le Lay, G., Helfer, V., Randin, C., and Guisan, A. (2006). Evaluating the ability of habitat suitability models to predict species presences. Ecol. Model. 199, 142–152. doi: 10.1016/j.ecolmodel.2006.05.017
Holm, L. G., Plucknett, D. L., Pancho, J. V., and Herberger, J. P. (1977). The World's Worst Weeds. Honolulu, HI: University Press.
Iacarella, J. C., Mankiewicz, P. S., and Ricciardi, A. (2015). Negative competitive effects of invasive plants change with time since invasion. Ecosphere 6:art123. doi: 10.1890/ES15-00147.1
Iyer, S. G., Banerjee, A. K., and Bhowmick, A. R. (2019). Making choices that matter – Use of statistical regularization in species distribution modelling for identification of climatic indicators – A case study with Mikania micrantha Kunth in India. Ecol. Indicators 98, 92–103. doi: 10.1016/j.ecolind.2018.10.036
Jupiter, S., Mangubhai, S., and Kingsford, R. T. (2014). Conservation of biodiversity in the Pacific Islands of Oceania: challenges and opportunities. Pacific Conserv. Biol. 20, 206–220. doi: 10.1071/PC140206
Kawecki, T. J., and Ebert, D. (2004). Conceptual issues in local adaptation. Ecol. Lett. 7, 1225–1241. doi: 10.1111/j.1461-0248.2004.00684.x
Kelly, R., Leach, K., Cameron, A., Maggs, C. A., and Reid, N. (2014). Combining global climate and regional landscape models to improve prediction of invasion risk. Diversity Distributions 20, 884–894. doi: 10.1111/ddi.12194
Keppel, G., Morrison, C., Meyer, J.-Y., and Boehmer, H. J. (2014). Isolated and vulnerable: the history and future of Pacific Island terrestrial biodiversity. Pacific Conserv. Biol. 20, 136–145. doi: 10.1071/PC140136
Legarda, B. J. (1999). After the Galleons: Foreign Trade, Economic Change and Entrepreneurship in the Nineteenth-century Philippines. Madison: University of Wisconsin-Madison.
Li, D. M., Wang, J. H., Peng, S. L., Zhu, G. F., and Lü, F. B. (2012). Molecular cloning and characterization of two novel NAC genes from Mikania micrantha (Asteraceae). Genetics Mol. Res. 11, 4383–4401. doi: 10.4238/2012.September.19.3
Li, J., Du, L., Guan, W., Yu, F. H., and van Kleunen, M. (2016). Latitudinal and longitudinal clines of phenotypic plasticity in the invasive herb Solidago canadensis in China. Oecologia 182, 755–764. doi: 10.1007/s00442-016-3699-x
Li, X., Shen, Y., Huang, Q., Fan, Z., and Huang, D. (2013). Regeneration capacity of small clonal fragments of the invasive Mikania micrantha HBK: effects of burial depth and stolon internode length. PLoS ONE 8:e84657. doi: 10.1371/journal.pone.0084657
Liu, Y., Oduor, A. M. O., Zhang, Z., Manea, A., Tooth, I. M., Leishman, M. R., et al. (2017). Do invasive alien plants benefit more from global environmental change than native plants? Global Change Biol. 23, 3363–3370. doi: 10.1111/gcb.13579
Macanawai, A. R., Day, M. D., Tumaneng-Diete, T., and Adkins, S. W. (2012). Vegetative growth and development of Mikania micrantha in taro and cassava production in Viti Levu, Fiji. Pak. J. Weed Sci. Res. 18, 377–384.
Mainali, K. P., Warren, D. L., Dhileepan, K., McConnachie, A., Strathie, L., Hassan, G., et al. (2015). Projecting future expansion of invasive species: comparing and improving methodologies for species distribution modeling. Global Change Biol. 21, 4464–4480. doi: 10.1111/gcb.13038
Manrique, V., Diaz, R., Cuda, J. P., and Overholt, W. A. (2011). Suitability of a new plant invader as a target for biological control in Florida. Invasive Plant Sci. Manage. 4, 1–10. doi: 10.1614/IPSM-D-10-00040.1
Marx, M., and Quillfeldt, P. (2018). Species distribution models of European Turtle Doves in Germany are more reliable with presence only rather than presence absence data. Sci. Rep. 8:16898. doi: 10.1038/s41598-018-35318-2
Mesgaran, M. B., Cousens, R. D., and Webber, B. L. (2014). Here be dragons: a tool for quantifying novelty due to covariate range and correlation change when projecting species distribution models. Diversity Distributions 20, 1147–1159. doi: 10.1111/ddi.12209
Moodley, D., Geerts, S., Richardson, D. M., and Wilson, J. R. (2013). Different traits determine introduction, naturalization and invasion success in woody plants: proteaceae as a test case. PLoS ONE 8:e75078. doi: 10.1371/journal.pone.0075078
Mukherjee, A., Christman, M. C., Overholt, W. A., and Cuda, J. P. (2011). Prioritizing areas in the native range of hygrophila for surveys to collect biological control agents. Biol. Control 56, 254–262. doi: 10.1016/j.biocontrol.2010.11.006
Myers, N., Mittermeier, R. A., Mittermeier, C. G., da Fonseca, G. A., and Kent, J. (2000). Biodiversity hotspots for conservation priorities. Nature 403, 853–858. doi: 10.1038/35002501
Oduor, A. M., Leimu, R., and Kleunen, M. (2016). Invasive plant species are locally adapted just as frequently and at least as strongly as native plant species. J. Ecol. 104, 957–968. doi: 10.1111/1365-2745.12578
Oduor, A. M. O., Gómez, J. M., Herrador, M. B., and Perfectti, F. (2015). Invasion of Brassica nigra in North America: distributions and origins of chloroplast DNA haplotypes suggest multiple introductions. Biol. Invas. 17, 2447–2459. doi: 10.1007/s10530-015-0888-1
O'Loughlin, L. S., and Green, P. T. (2017). Secondary invasion: when invasion success is contingent on other invaders altering the properties of recipient ecosystems. Ecol. Evol. 7, 7628–7637. doi: 10.1002/ece3.3315
Olson, D. M., and Dinerstein, E. (2002). The Global 200: priority ecoregions for global conservation. Ann. Missouri Bot. Garden 89, 199–224. doi: 10.2307/3298564
Petitpierre, B., Kueffer, C., Broennimann, O., Randin, C., Daehler, C., and Guisan, A. (2012). Climatic niche shifts are rare among terrestrial plant invaders. Science 335, 1344–1348. doi: 10.1126/science.1215933
Phillips, S. J., Anderson, R. P., and Schapire, R. E. (2006). Maximum entropy modeling of species geographic distributions. Ecol. Modell. 190, 231–259. doi: 10.1016/j.ecolmodel.2005.03.026
Prabu, N. R., Stalin, N., and Swamy, P. S. (2014). Ecophysiological attributes of Mikania micrantha, an exotic invasive weed, at two different elevations in the tropical forest regions of the Western Ghats, South India. Weed Biol. Manage. 14, 59–67. doi: 10.1111/wbm.12033
Puzari, K., Bhuyan, R., Dutta, P., and Nath, H. (2010). Distribution of Mikania and its economic impact on tea ecosystem of Assam. Ind. J. Forestry 33, 71–76.
R Core Team (2017). R: A Language and Environment for Statistical Computing. Vienna: R Foundation for Statistical Computing.
Richmond, J. Q., Wood, D. A., Stanford, J. W., and Fisher, R. N. (2015). Testing for multiple invasion routes and source populations for the invasive brown treesnake (Boiga irregularis) on Guam: implications for pest management. Biol. Invas. 17, 337–349. doi: 10.1007/s10530-014-0733-y
Sankaran, K., Puzari, K., Ellison, C., Kumar, P., and Dev, U. (2008). “Field release of the rust fungus Puccinia spegazzinii to control Mikania micrantha in India: protocols and raising awareness,” in Proceedings of the XII International Symposium on Biological Control of Weeds, eds. M. H. Julien, R. Sfroza, M. C. Bon, H. C. Evans, P. E. Hatcher, H. L.Hinz and B. G. Rector (Wallingford: CAB International, 384–389.
Sapkota, L. (2007). Ecology and management issues of Mikania micrantha in Chitwan Naitonal Park, Nepal. Banko Janakari 17, 27–39. doi: 10.3126/banko.v17i2.2153
Shrestha, U. B., Sharma, K. P., Devkota, A., Siwakoti, M., and Shrestha, B. B. (2018). Potential impact of climate change on the distribution of six invasive alien plants in Nepal. Ecol. Indicators 95, 99–107. doi: 10.1016/j.ecolind.2018.07.009
Smeraldo, S., Di Febbraro, M., Bosso, L., Flaquer, C., Guixé, D., Lisón, F., et al. (2018). Ignoring seasonal changes in the ecological niche of non-migratory species may lead to biases in potential distribution models: lessons from bats. Biodiversity Conserv. 27, 2425–2441. doi: 10.1007/s10531-018-1545-7
Song, L., Wu, J., Li, C., Li, F., Peng, S., and Chen, B. (2009). Different responses of invasive and native species to elevated CO2 concentration. Acta Oecol. 35, 128–135. doi: 10.1016/j.actao.2008.09.002
Song, L.-Y., Ni, G.-Y., Chen, B.-M., and Peng, S.-L. (2007). Energetic cost of leaf construction in the invasive weed Mikania micrantha HBK and its co-occurring species: implications for invasiveness. Bot. Stud. 48, 331–338.
Spry, H. H. (1839). Suggestions Received by the Agricultural and Horticultural Society for Extending the Cultivation and Introduction of Useful and Ornamental Plants. Calcutta: Bishop's College Press.
Srivastava, V., Griess, V. C., and Padalia, H. (2018). Mapping invasion potential using ensemble modelling. A case study on Yushania maling in the Darjeeling Himalayas. Ecol. Model. 385, 35–44. doi: 10.1016/j.ecolmodel.2018.07.001
Sun, Y., Brönnimann, O., Roderick, G. K., Poltavsky, A., Lommen, S. T. E., and Müller-Schärer, H. (2017). Climatic suitability ranking of biological control candidates: a biogeographic approach for ragweed management in Europe. Ecosphere 8:e01731. doi: 10.1002/ecs2.1731
Teoh, C., Chung, G., Liau, S., Ibrahim, G., Tan, A., Lee, S., et al. (1985). Prospects for biological control of Mikania micrantha HBK in Malaysia. Planter 61, 515–530.
Thapa, S., Chitale, V., Rijal, S. J., Bisht, N., and Shrestha, B. B. (2018). Understanding the dynamics in distribution of invasive alien plant species under predicted climate change in Western Himalaya. PLoS ONE. 13:e0195752. doi: 10.1371/journal.pone.0195752
Thuiller, W., Lafourcade, B., Engler, R., and Araújo, M. B. (2009). BIOMOD – a platform for ensemble forecasting of species distributions. Ecography 32, 369–373. doi: 10.1111/j.1600-0587.2008.05742.x
Tripathi, R., Khan, M., and Yadav, A. (2012). “Biology of Mikania micrantha HBK: a review,” in Invasive Alien Plants: An Ecological Appraisal for the Indian Subcontinent, eds J. R. Bhatt, J. S. Singh, S. P. Singh, R. S. Tripathi, and R. K. Kohli (Wallingford: CAB International), 99–107.
Václavík, T., and Meentemeyer, R. K. (2012). Equilibrium or not? Modelling potential distribution of invasive species in different stages of invasion. Diversity Distributions 18, 73–83. doi: 10.1111/j.1472-4642.2011.00854.x
van Kleunen, M., Essl, F., Pergl, J., Brundu, G., Carboni, M., Dullinger, S., et al. (2018). The changing role of ornamental horticulture in alien plant invasions. Biol. Rev. 93, 1421–1437. doi: 10.1111/brv.12402
Waterhouse, B. M. (1999). “Seek and ye shall find: new weed records from Irian Jaya, Papua New Guinea and northern Queensland”, in Weeds and Environmental Impact, Proceedings (II) of The 17th Asian-Pacific Weed Science Society Conference (Bangkok: Asian-Pacific Weed Science Society), 105–111.
Webber, B. L., Yates, C. J., Le Maitre, D. C., Scott, J. K., Kriticos, D. J., Ota, N., et al. (2011). Modelling horses for novel climate courses: insights from projecting potential distributions of native and alien Australian acacias with correlative and mechanistic models. Diversity Distributions 17, 978–1000. doi: 10.1111/j.1472-4642.2011.00811.x
Williams, D. A., Overholt, W. A., Cuda, J. P., and Hughes, C. R. (2005). Chloroplast and microsatellite DNA diversities reveal the introduction history of Brazilian peppertree (Schinus terebinthifolius) in Florida. Mol. Ecol. 14, 3643–3656. doi: 10.1111/j.1365-294X.2005.02666.x
Williamson, M. (2006). Explaining and predicting the success of invading species at different stages of invasion. Biol. Invas. 8, 1561–1568. doi: 10.1007/s10530-005-5849-7
Willis, M., Zerbe, S., and Kuo, Y.-L. (2008). Distribution and ecological range of the alien plant species Mikania micrantha Kunth (Asteraceae) in Taiwan. J. Ecol. Environ. 31, 277–290. doi: 10.5141/JEFB.2008.31.4.277
Wirjahardja, S. (1975). “Autecological study of Mikania spp,” in Proceedings of 5th Asian-Pacific Weed Science Society Conference (Tokyo).
Xiao, S., Callaway, R. M., Graebner, R., Hierro, J. L., and Montesinos, D. (2016). Modeling the relative importance of ecological factors in exotic invasion: the origin of competitors matters, but disturbance in the non-native range tips the balance. Ecol. Model. 335, 39–47. doi: 10.1016/j.ecolmodel.2016.05.005
Keywords: biological invasion, climate change, global biodiversity, introduction history, niche modeling, potential distribution
Citation: Banerjee AK, Mukherjee A, Guo W, Liu Y and Huang Y (2019) Spatio-Temporal Patterns of Climatic Niche Dynamics of an Invasive Plant Mikania micrantha Kunth and Its Potential Distribution Under Projected Climate Change. Front. Ecol. Evol. 7:291. doi: 10.3389/fevo.2019.00291
Received: 11 June 2019; Accepted: 22 July 2019;
Published: 06 August 2019.
Edited by:
Luciano Bosso, University of Naples Federico II, ItalyReviewed by:
Sonia Smeraldo, University of Naples Federico II, ItalyCopyright © 2019 Banerjee, Mukherjee, Guo, Liu and Huang. This is an open-access article distributed under the terms of the Creative Commons Attribution License (CC BY). The use, distribution or reproduction in other forums is permitted, provided the original author(s) and the copyright owner(s) are credited and that the original publication in this journal is cited, in accordance with accepted academic practice. No use, distribution or reproduction is permitted which does not comply with these terms.
*Correspondence: Yelin Huang, bHNzaHlsQG1haWwuc3lzdS5lZHUuY24=
Disclaimer: All claims expressed in this article are solely those of the authors and do not necessarily represent those of their affiliated organizations, or those of the publisher, the editors and the reviewers. Any product that may be evaluated in this article or claim that may be made by its manufacturer is not guaranteed or endorsed by the publisher.
Research integrity at Frontiers
Learn more about the work of our research integrity team to safeguard the quality of each article we publish.