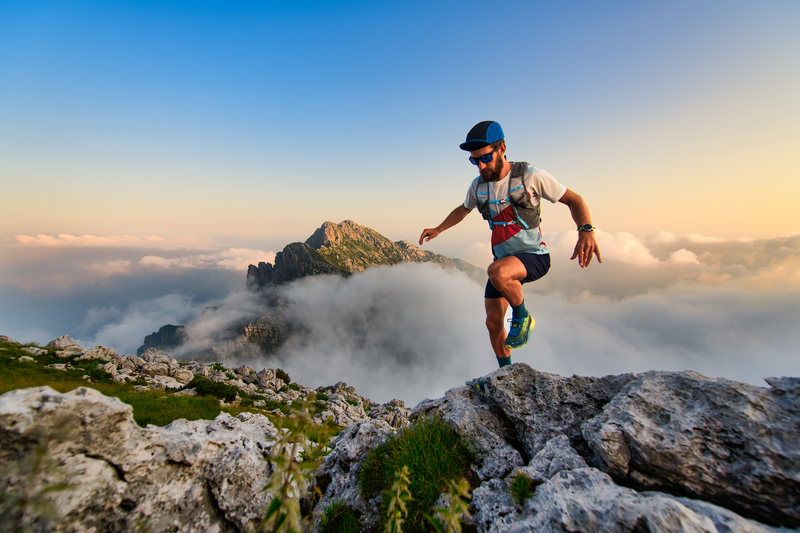
94% of researchers rate our articles as excellent or good
Learn more about the work of our research integrity team to safeguard the quality of each article we publish.
Find out more
ORIGINAL RESEARCH article
Front. Ecol. Evol. , 08 May 2019
Sec. Behavioral and Evolutionary Ecology
Volume 7 - 2019 | https://doi.org/10.3389/fevo.2019.00154
This article is part of the Research Topic Ecology and Behaviour of Free-Ranging Animals Studied by Advanced Data-Logging and Tracking Techniques View all 23 articles
Technological miniaturization is driving a biologging revolution that is producing detailed and sophisticated techniques of assessing individual behavioral responses to environmental conditions. Among the many advancements this revolution has brought is an ability to record behavioral responses of nocturnal, free-ranging species. Here, we combine captive validations of acceleration signatures with acoustic recordings from free-ranging individuals to classify behavior at two resolutions. Combining these classifications with ~2 month-long recordings, we describe winter time budgets, and responses of free-ranging snowshoe hares to changing moonlight. We successfully classified snowshoe hare behavior into four categories (not moving, foraging, hopping, and sprinting) using low frequency accelerometry, with an overall model accuracy of 88%, and acoustic recordings to three categories (silence, hopping, and chewing) with an accuracy of 94%. Broad-scale accelerometer-classified categories were composed of multiple fine-scale behavioral states with the composition varying between individuals and across the day. Time budgets revealed that hares spent ~50% of their time foraging and ~50% not moving, with most foraging and feeding occurring at night. We found that hares adjusted timing of activity in response to moon phase, with a 6% reduction in foraging and 30% reduction in traveling during the night when the moon was full. Hares compensated for this lost foraging time by extending foraging into the morning hours of the following day. Using two biologging technologies to identify behavior, we demonstrate the possibility of combining multiple devices when documenting behavior of cryptic species.
From satellites and drones to biologging devices, new technologies are providing us with the capabilities to answer questions about the natural world, and the species that live within it that could only have been dreamt about a few decades ago. Every year, the number of technologies available to ecologists expands, and the sophistication and capacity of those tools that exist improves (see reviews: Elliott, 2016; Williams et al., 2016). Although initial incorporation of devices on wildlife focused on space use, with a focus on knowing in real time the exact location of an individual, the latest phase of the biologging revolution has, in part, been behaviorally focused, with a desire to know what the individual was doing (Wilmers et al., 2015). One of the most popular devices for behavioral classification is the accelerometer, which measures 3-dimensional acceleration of a species of interest (see Figure 1; e.g., Graf et al., 2015) and for which miniaturization has reduced the weight to as little as 0.7 grams (e.g., Axy-4 without battery, Technosmart, Rome, Italy). Taking into account gravity and acceleration profiles of different movement types, these recordings can provide information on posture and orientation, energy expenditure, and activity levels, that should correspond to specific behavioral states (Wilson et al., 2006; Shepard et al., 2008; Gleiss et al., 2011; Brown et al., 2013).
Figure 1. Example of 1 Hz tri-axis accelerometer data and the orientation axis from a collar attached to a captive snowshoe hare. Arrow indicates location where accelerometer rested on the animal.
The potential for accelerometers to record behavior over long timeframes, including multi-day, cross-seasonal, and even multi-annual periods, involves a tradeoff between recording duration and the resolution of behavioral classification (Broell et al., 2013; Tatler et al., 2018). Accurate classification of detailed behavioral states requires a sampling rate that is twice the highest frequency present in the signal, referred to as the Nyquist criterion (Beutler, 1966; Chen and Bassett, 2005; Graf et al., 2015). Most classifications target rapid movements like wingbeats or steps (Shepard et al., 2008; Spivey and Bishop, 2013), but the intensive sampling needed to do so tends to limit device longevity below what is necessary for documenting wildlife responses to changes in their environment that occur at seasonal and annual timescales. To increase recording duration, sampling frequency can be lowered at the cost of only capturing behavioral categories with lower Nyquist criterion such as bouts of traveling, foraging, and resting (Campbell et al., 2013; Tatler et al., 2018; Studd et al., 2019). Such information, although less specific, is still highly useful for building activity and energy budgets (Williams et al., 2017; Studd et al., 2019).
To counteract the loss of information from using a lower sampling frequency, it may be necessary to determine the detailed behavioral composition of the broader behavioral categories through different means. In species where direct observations are difficult, this may require combining accelerometers with additional biologging technology such as video or audio recorders (Lynch et al., 2013; Pagano et al., 2018). For large terrestrial species (such as polar bears and caribou), observational data on free-ranging behavior can be obtained with video camera collars (e.g., Thompson et al., 2012; Pagano et al., 2018), while for smaller taxa where weight of monitoring devices becomes limiting, deployment of acoustic recorders may be a potential alternative (Lynch et al., 2013; Couchoux et al., 2015). Acoustic data has been incorporated into many fields within ecology providing new means of quantifying biodiversity (e.g., Depraetere et al., 2012; Gasc et al., 2013), soundscapes (Pijanowski et al., 2011), and animal communication (e.g., Reby and McComb, 2003; Fischer et al., 2004; Thiebault et al., 2016). More recently, a few studies have even revealed the potential of acoustic devices to record non-vocal behavior (e.g., flying, feeding, walking; Ilany et al., 2013; Lynch et al., 2013; Stowell et al., 2017; Wijers et al., 2018).
Here we highlight the potential of using accelerometers and acoustic recorders (attached as collars) to classify the behavior of free-ranging snowshoe hares (Lepus americanus), a cryptic small mammal (2 kg). Our primary objective was to determine if we could use low frequency acceleration to identify broad behavioral categories that could be recorded over days to months. In order to do this, we linked accelerometer recordings to observations of captive hares. Additionally, we took advantage of the ability of acoustic recorders to classify non-vocal behavior in order to determine a more detailed composition of accelerometer-based behavioral categories. Our secondary objective was to showcase how these tools, in providing detailed behavioral information over long periods of time, can then be used to investigate daily activity patterns and how aspects of the environment can influence behavior of free-ranging hares. We took a proof of concept approach whereby we explored how light conditions caused by phases of the moon and daylight influenced nocturnal hare behavior.
The study took place in southwestern Yukon (61°N, 138°W) within the Shakwak trench, an area of boreal forest where snowshoe hares have been the focus of studies for the past 45 years (Krebs et al., 2018). The forest is predominantly white spruce (Picea glauca) intermixed with patches of aspen (Populus tremuloides) and balsam poplar (Populus balsamifera), and an understory of gray willow (Salix glauca) and dwarf birch (Betula pumila var. glandulifera) (Boonstra et al., 2016). Snowshoe hares exhibit 10-year population cycles, which are, at least in part driven by their primary predators Canada lynx (Lynx canadensis), coyote (Canis latrans), and great horned owls (Bubo virginianus; Rohner and Krebs, 1996; O'Donoghue et al., 1998). During this study, snowshoe hares were in the increase phase of the cycle with densities averaging 1 hare/ha (Krebs et al., 1995).
All snowshoe hares were captured using Tomahawk live-traps (Tomahawk Live Trap Co. Tomahawk, WI, USA) baited with alfalfa and rabbit chow, and set and checked overnight (Keith, 1964). Individuals were fitted with an accelerometer (model Axy3, 4 g, Technosmart, Rome, Italy) and VHF radio transmitter (Model SOM2380, Wildlife Materials Inc., USA, or Model MI-2M, Holohil, Canada, both 27 +/– 1 g) in the form of a collar (31 +/– 1 g, 2.5% of smallest hare mass). Accelerometers rested on the dorsal side of the neck and recorded acceleration on 3 dimensional axes at 1 Hz with a resolution of +/– 8 g−forces. To record observations of snowshoe hare behavior, we captured six hares (>1,200 g) in April 2015, attached collars and transferred them to outdoor enclosures (4.5 m by 4.5 m; modified from Sheriff et al., 2009; Lavergne and Boonstra pers comm). Hares were held for three days and supplied with rabbit chow, water, and willow branches collected from the surrounding area. At the completion of observational trials, collars were removed, and hares were released at point of capture. To explore the potential of accelerometers for monitoring behavior over multiple months, we live-trapped and collared 14 free-ranging snowshoe hares between October 2015 and March 2016. Once collared, hares were released at their capture site, and recaptured 1–3 months later (average = 62 days, range: 32–100 days) for collar removal and data download. This research conformed to the guidelines of the American Society of Mammalogists (Sikes et al., 2016) and was approved by the McGill University, University of Alberta, and University of Toronto Animal Care and Use Committees.
We used the observations of captive hares to cross-validate behavioral categories based on accelerometer information. For this, 2 h of video (Nikon D90 with 50 mm, Sony Handycam HDR-CX240) were recorded per day for three days capturing morning and dusk activity. During recordings, personnel left the enclosure area to minimize influence of human activity on hare behavior. Hares tended to move rapidly when humans entered the enclosures to provide food and remove droppings, so cameras also recorded during these times. The same time-keeping device was held in frame at the start of each video to sync times for all observations. Videos were watched by two observers who recorded the start and end times of each behavior, which included digging, feeding, grooming, jumping, vigilance (sitting while head moves to look in multiple directions), sprinting, shaking, sitting (motionless), standing, travel with multiple hops, or travel with one hop only. From this we selected the six most common behavioral states that represented 91.8% of all observations and combined them into three broader categories (not moving: sitting and vigilance; foraging: feeding and travel with one hop; traveling: sprinting and travel with multiple hops). This included at least one observation of each behavior per hare per day with the average number of observations of each behavior per hare per day ranging from 8 for feeding to 42 for vigilance (Table S1). Since clocks on separate devices did not run at exactly the same rate, we visually identified multiple occasions per day per hare where the animal transitioned from sitting to traveling to calculate the time divergence between each accelerometer and the camera clock to generate a time correction equation for each accelerometer (error = +/– 3 s).
We observed few instances of hares sprinting at maximum speeds in the enclosures. Thus, to capture potential high-speed chase or “fleeing” behavior (an important aspect of predator-prey interactions), we added additional behavioral data from free-ranging snowshoe hares that were chased by a simulated predator (i.e., a dog, Canis familiaris, a model for coyotes; see methods in Boudreau, 2019). For each chase, the time and whether or not the hare sprinted were recorded, and a subset of chases (n = 47) where hares were observed sprinting from the dog were used as examples of sprinting behavior.
Average static acceleration was calculated using a running medians smoothing window of 91 samples (see Supplementary Materials 1.1 for window size selection method). We removed this long duration static acceleration (general orientation of device) from total acceleration to retain only acceleration generated from the movement of the animal on which all further analyses were based. This remaining acceleration is primarily the measurement of small changes in the posture of the animal that occur during each behavior, and secondarily, measurements of the dynamic acceleration of the movement. To classify acceleration by behavioral categories we constructed a decision tree consisting of three hierarchical divisions (Studd et al., 2019): (1) not moving (no visible motion, i.e., sitting) and moving (any physical movement), (2) all moving into foraging (feeding, travel with one hop) or traveling (travel with multiple hops), and (3) all traveling into hopping (observed in enclosure) and sprinting (observed in simulated chases in the wild). For each division of the tree, observed behavioral data was split between training (70%) and testing (30%), and then subsampled to ensure equal numbers of each behavior. Over each sample window duration, determined by the average duration observed in videos of behavioral states in each division, we calculated the mean, maximum, minimum, range, standard deviation, and sum of acceleration on each of the three axes (surge, heave, and sway), along with the sum of overall acceleration (OA; similar to ODBA in Wilson et al., 2006), and the change in overall acceleration (ΔOA) across all three axes. ΔOA is the change in acceleration (Δa) for each axis from 1 s to the next summed over the time window across all three axes. Threshold values for behavioral categories at each division were determined by a two-step process. First, the percent overlap between the two behavioral categories for each summary statistic was calculated and the statistic with the lowest overlap was selected (Figure 2A). Second, the percent error of classification was calculated for every 0.1 increment of the selected statistic between the minimum and maximum values, and the threshold value was set according to the lowest classification error (Figure 2B). Using the remaining 30% of the observational data, accuracy for each division of the tree was calculated as proportion of all observations that were correctly classified by the threshold value.
Figure 2. Example illustrating method for determination of threshold values for separation of behavioral states using accelerometer data from collars attached to snowshoe hares. Histograms provide visualization of percent overlap between two behavioral categories using a given summary statistic (A). Optimization is performed by examining the accuracy of the behavioral classification between two behavioral states across a range of values and selecting the value at which the overall accuracy is the highest and where the individual accuracy of each behavior intersects (B). Dotted line represents the selected threshold value for classifying accelerometer data into forage and travel using ΔOA.
The flexibility of this method allows for different sample duration windows to be used at each division. Sample window size was 12 s for not moving and moving, and 4 s for each of foraging and traveling, and for hopping and sprinting. Different training datasets were used for each division. For the first division we used all not moving and moving events with durations of at least 12 s (91.8% of observed behavior). The second division included all foraging (51% or more feeding with no type of travel) and traveling (51% or more travel with multiple hops) events that lasted at least 4 s (89.4% of observed behavior). The final division included all hopping (low speed travel with multiple hops) and sprinting (simulated predator chases) events with summary statistics calculated over 4 s. Due to low sample size of sprinting events, overall classification accuracy was calculated on the first two divisions only.
Although we used a threshold-based classification approach, much recent accelerometer-based literature uses alternative machine learning methods. These approaches have the advantage of efficient processing for the generation of complex classifications with high accuracies, but the disadvantage of black box, non-transferrable thresholds, and non-hierarchical trees (Bidder et al., 2014; McClune et al., 2014). Given pros and cons to both approaches, we also explored the accuracy of random forest-based classification, as described more fully in the Supplementary Materials 1.2, and briefly describe the outcome of this alternative approach in the results. As the random forest provided similar results, we decided to report the more simple method in the body of the paper.
We used animal-borne acoustic recorders to explore composition of broad behavioral classifications (i.e., not moving, and foraging) generated from our low frequency accelerometer recordings. In January 2018, we captured three male snowshoes hares and fitted them with an accelerometer-VHF combination collar that contained an acoustic recorder (Edic-mini Tiny+ A77, 6.6 g, total collar weight of 41 g, <3% of body mass). Once collared, each individual was released at the capture site and recaptured between 4 and 22 days later. Audio recorded continuously at 16,000 hz with μ-law compression for 3 days following capture. Prior to acoustic analysis, we listened to and recorded the sounds contained within 135–15 s audio clips (45 per recorder) that corresponded to long duration (>15 s) foraging, not moving, and traveling as determined by our accelerometer classification tree. Listeners determined that sounds that suggested chewing (33.4% of audio; see Audios 1, 2, and 3 in Supplementary Materials for example clips), hopping (24.5%), silence (23.6%), and unclassifiable noises (9.3%) could be repeatedly distinguished and were the most common. Although we could not truly validate our sound classification for each behavior, we verified that these sounds could be repeatedly associated with a specific behavior among different observers. Three independent listeners blindly classified a subset of clips into the four categories, and we calculated inter-listener agreement for each type of sound.
We manually extracted 300 s of each sound associated with chewing, hopping, and silence consisting of 20–30 independent clips from each hare. Using 70% of the clips, spectrogram analysis (window = 8,000, overlap = 50) was run on each second using the seewave package in R to determine the acoustic properties (Sueur et al., 2008). A classification algorithm consisting of upper and lower amplitude threshold values at 8 frequencies between 0 and 8 kHz for each sound (chewing, hopping, and silence) was created for each device. Thresholds were the 100% confidence intervals plus or minus 10% for that sound (Tables S4, S5, Supplementary Materials 1.3). However, if thresholds of two sounds overlapped at all frequencies, an optimization was run at the frequency where amplitudes were most distinguishable between sounds, and thresholds were adjusted to the value that generated the lowest misclassification. Any sound that did not meet all specified thresholds for chewing, hopping, or silence was classified as “other.” The accuracy of classification, unique to each recorder, was calculated using the remaining 30% of clips.
All audio files were converted to behavioral categories at a 1-s resolution and used to tabulate an acoustic-based behavioral composition of the not moving and foraging categories from accelerometer classification for each hare, and across all hares. To account for drift in the internal clocks of the devices, prior to analysis we aligned the timing between devices by identifying 30 events across the file when both acceleration and acoustic amplitude shifted from a long bout of low values to a long bout of high values (i.e., resting to moving). A linear regression of time divergence over time of recording was calculated and the coefficients were used to readjust acoustic time. However, a ~20 s error remained post-alignment so behavioral composition was calculated using not moving and foraging accelerometer bouts longer than 90 s, with the first and last 30 s removed.
From both accelerometer-classified, and acoustic-classified behavior, we determined the daily time budgets of free-ranging individuals. This was calculated as the proportion of 24 h that all hares spent expressing each behavior.
Moonlight illumination levels and daily light phase times (including moon rise and moon set times) for our study site were retrieved with suncalc package in R (Agafonkin and Thieurmel, 2017). Moonlight illumination levels were converted into a 3-level categorical variable according to the fraction of the moon that was lit: <0.33 (new), 0.33–0.66, and >0.66 (full). Eight light phases defined by the position of the sun relative to the horizon were used including: day (above horizon minus the first and last hour sunlight; ~4 h), evening (last hour above horizon; ~1 h), dusk (0–6° below horizon; ~1 h), evening twilight (6–18° below horizon; ~2 h), night (>18° below horizon; ~12 h), morning twilight (18–6° below horizon; ~2 h), dawn (6–0° below horizon; ~1 h), and morning (first hour above horizon; ~1 h). We removed all times when there was potentially cloud cover using both snowfall measures, which were collected daily from 4 locations throughout the study area, and hourly relative humidity values from the nearest weather station (Haines Junction, 40 km away; Environment Canada). We removed all nights preceding a snowfall measurement >0, and all times when the relative humidity was >85%, since cloud cover is highly correlated with relative humidity (Sandor et al., 2000). Although we did not measure moonlight illumination levels ourselves and do not know the exact values that occurred, moon phase and lunar position are commonly used in studies of the effects of moonlight on wildlife (e.g., Johnson and De León, 2015; Gigliotti and Diefenbach, 2018).
As foraging and not moving were highly correlated (Pearson's correlation coefficient = -0.99), moonlight analysis focused on foraging with the understanding that any major changes seen in foraging times are mirrored by opposite changes in not moving. We quantified accelerometer derived hare behavior in response to moonlight at three temporal scales. At a daily scale, we examined foraging time per 24 h using a generalized linear mixed effects model (GLMM) with moon phase (three level) as a fixed effect (R:lmer; Bates et al., 2015). At a within-day scale, we examined how foraging time (min/hr) was influenced by moon phase during different times of day using a GLMM with a light phase and moon phase interaction. At this scale we also tested how hopping (min/h) and sprinting (events/h) were influenced by moonlight across light phases. Hopping was examined using the same GLMM as foraging. Sprinting event data was zero-inflated so a hurdle model (Martin et al., 2005; Zuur et al., 2009) was used consisting of a binomial (logit-link) GLMM to test whether individuals sprinted or not during each light phase, and a second GLMM to test whether differences occurred in the number of sprint events during times when hares sprinted at least once. As behavioral states were analyzed separately, a Bonferroni correction (alpha = 0.02) was applied to all analyses at this temporal scale. At an hourly scale, we examined how foraging time (min/h) was influenced by the presence of moon using a GLMM with a moon phase and moon position (set or risen) interaction. This analysis only used hourly foraging values during the darkest light phase (night). All hours when the moon rose, or set were removed to reduce landscape-imposed variation in timing of rising and setting. We included the intermediate moonlight levels in this analysis as it is during this part of each month that the moon rises or sets halfway through the night and the response to moon position relative to the horizon might be most pronounced. All GLMMs included hare ID as a random factor, and for the within-day and hourly scale analysis, date was included as a random factor. Model fit was calculated using conditional R-squared values, and significance of fixed effects were assessed using Wald chi-square (χ2) tests (Bolker et al., 2009).
We found that a ΔOA threshold value of 1.15 g-forces distinguished moving from not moving with a 95.8% accuracy using a sample window duration of 12 s. Not moving was correctly classified 94.6% (159/168 events) of the time, while moving had an accuracy of 97.0% (163/168 events; Figure 3). A ΔOA threshold of 3.0 g-forces over 4 s further separated any segment classified as moving into foraging (feeding, and short travel) and traveling with an accuracy of 88.1% (traveling = 83.1%, 242/291 events; foraging = 93.1%, 271/291 events; Figure 3). Finally, traveling-classified segments were divided into sprinting and hopping (low speed travel) using a OA threshold value of 6.5 g-forces over 4 s with an accuracy of 88.4% (sprinting = 76.9%, 10/13 events; hopping = 100%, 13/13 events; Figure 3). The overall accuracy of the classification into three behavioral states (not moving, foraging, and traveling), which accounts for all misclassifications at each level of the tree was 88.0% with slightly lower divisional accuracy than what was calculated when setting the threshold values (Table 1). Accuracy varied at the individual level from 80 to 91.7%, with large variation in individual accuracy of classifying traveling and foraging (see Supplementary Materials for individual confusion matrices 1.4). Classification using a random forest algorithm generated accuracies ranging from 83.3 to 96.7% depending on the sample window chosen (see Figure S2).
Figure 3. Hierarchal decision tree used to classify snowshoe hare accelerometer data to four behavioral categories. Long duration behavioral states were classified using a 12 s window, while short duration behavior was classified using a 4 s window. The accuracy of each division is the percentage of observed behavior that were classified correctly. Histograms depict the frequency of each behavior occurring at different values of the summary statistic used for the division of the tree. Black represents behavior on left side of the decision tree while white is behavior on right side of decision tree at each split.
Table 1. Confusion matrix of accelerometer-based classification of snowshoe hare behavior to three categories: not moving, forage, and travel.
Three audio sounds (silence, chewing, and hopping) could consistently be identified by listeners (see Audios 1, 2, and 3 in Supplementary Materials for example clips; Table S3). Inter-rater reliability was 97 and 95% for chewing and silence, respectively. There was 83.3% agreement among all raters for “unclassifiable sounds”; some sound clips were suggested to be hopping or chewing, but there was no consensus across all listeners. Hopping had the lowest among-listener agreement (55.6%) with the most common alternative classification being unclassifiable sound. We are confident in our identification of these four sounds due to (1) the high inter-rater reliability scores, and (2) sounds labeled chewing, hopping, and silence primarily occurred when the hare was foraging, traveling, and not moving according to the accelerometer, respectively. That being said, we have no means to truly validate that these sounds are the behavioral states classified. As such, our acoustic results should be taken with caution as there is a potential for misclassification.
Acoustic spectral analysis of user-classified sounds indicated that silence had no peaks in amplitude at any frequency, chewing had a peak at 250–600 Hz, and hopping had a primary peak at 0–650 Hz and secondary peak at 3,650–4,000 Hz (Figure 4), but peak frequencies varied between recorders (Supplementary Materials 1.3, Figure S3). Automated classification of these three acoustic behavioral states produced an accuracy of 94.1% (Table 2) with little variance in accuracy between devices (Supplementary Materials 1.5). Only 5.7% of acoustic files did not match the properties of these three behaviors and were classified as other sounds. The majority (~60%) of these other sounds were short in duration (1 s) and may have consisted of branches or parts of the hare hitting the microphone, or the hare shifting in position. In comparison, only a small amount of the silence (4%), chewing (9.4%), and hopping (22.8%) were short duration (1 s).
Figure 4. Amplitude frequency spectral properties of hop, chew, and silent sounds from animal-borne acoustic recorders on snowshoe hares (Right) and accompanying spectrograms (Left). Shading represents the 95% CI of the amplitude for each behavior.
Table 2. Confusion matrix of acoustic-based behavioral classification of snowshoe hare behavior to three categories: silence, chew, and hop.
Acoustic refinement of accelerometer classification revealed that bouts of foraging were composed of a combination of chewing (42.5%), hopping (35.7%), silence (13.0%), and other sounds (8.8%). The composition of foraging bouts varied among individuals (Figure 5A) but, on average, was consistent throughout the day (Figure 6A). The behavioral composition of not moving was consistent among individuals and was composed of silence (~80%) chewing (~13%), other sounds (~4%), and hopping (0.3%) (Figure 5B). The composition of not moving varied with time of day switching from primarily silence during the day to primarily chewing at night (Figure 6B).
Figure 5. Acoustic determination of time spent chewing (black), hopping (white), silent (black stripe), and other (white stripe) during accelerometer-defined bouts of foraging (A) and not moving (B), across all individuals (n = 3) and for each individual free-ranging snowshoe hare.
Figure 6. Accelerometer classification of the proportion of each hour of the day spent foraging (A) and not moving (B) and the acoustic composition (chewing: black, hopping: white, silence: black stripe, or other: white stripe) of those behavioral states. All data was collected over the same 2 days from free-ranging snowshoe hares (n = 3) that were outfitted with an accelerometer and an acoustic recorder. Arrows denote sunrise and sunset times.
We calculated average daily time budgets of free-ranging snowshoe hares in winter using accelerometer data from all individuals. Hares spent almost all of their time either foraging (~49%) or not moving (~49%) with small amounts of traveling (2%; i.e., either long duration hopping or sprinting). Not moving was the predominant behavior during daylight hours, and time spent foraging increased at dusk, remained high throughout the night, and decreased at dawn (Figures 6B, 7A). Acoustic recorders revealed that snowshoe hares split their time between silence (46.3%), chewing (37.5%), and hopping (10.8%) with small amounts of other sounds (5.4%). Hares were mainly silent during daylight, while at night they were chewing and hopping (Figure 6).
Figure 7. Average time spent by snowshoe hares on each behavior during different light phases of a day and moon phase with light boxes for full moon (lunar illumination > 0.66) and dark boxes for new moon (lunar illumination < 0.33) conditions (mean ± 95% CI). Not moving (A), foraging (B), and hopping (C) are presented as time spent (min/h) expressing each behavior, while sprinting (D) is depicted as number of events per hour. Bar at top indicates the solar illumination level of each light phase and average number of hours that occur during that phase in a 24-h period.
Snowshoe hares decreased daily time spent foraging by 40 min per day during a full moon compared to during a new moon (χ2 = 11.6, df = 2, P = 0.003, model R2(c) = 0.23). Time spent foraging, hopping, and the occurrence of sprinting events during each light phase was influenced by the phase of the moon (foraging: χ2 = 230.8, df = 14, P < 0.001, model R2(c) = 0.69; hopping: χ2 = 104.5, df = 14, P < 0.001, model R2(c) = 0.37; sprinting: χ2 = 42.9, df = 14, P < 0.001, model R2(c) = 0.67; Figure 7), but the number of sprint events in a light phase was not influenced by the moon (χ2 = 15.2, df = 14, P = 0.36, model R2(c) = 0.32). Hares had the largest decrease in foraging time during the night, with an average decrease of 3 min/h or 51 min between dusk until dawn during full moons compared to new moons (Figure 7B). Hopping, but not sprinting, was also substantially reduced through the darkest phases from twilight until dawn (Figures 7C,D). However, this pattern switched during dawn and morning following a full moon when hares spent noticeably more time foraging and hopping and were more likely to sprint than after a new moon (Figures 7B–D). Within a single night, snowshoe hare responses to the moon being above or below the horizon depended on the phase of the moon (χ2 = 15.1, df = 2, P < 0.001, model R2(c) = 0.30; Figure 8). When the moon was at its brightest (>0.66 fraction is visible), snowshoe hares spent more time foraging per hour during the hours that the moon was below the horizon than when the moon had risen (t = 2.07, df = 1,443, P = 0.039; Figure 8). However, during all other phases of the moon (visible fraction is <0.66), hares decreased time spent foraging when the moon was below the horizon as compared to when it was above the horizon (fraction<0.33: t = −2.25, df = 1,436, P = 0.024; fraction>0.33: t = −3.62, df = 1,444, P < 0.001; Figure 8).
Figure 8. Average foraging time (min/h) of snowshoe hares when moon is above (risen; light gray) or below (set; black) the horizon during different moon illumination fractions (mean ± 95% CI).
By integrating two biologging technologies (accelerometers and acoustic recorders), we demonstrate the possibility of accurately classifying behavior of a nocturnal, and often difficult to observe, free-ranging mammal over multi-month durations. After achieving high overall accuracy for both accelerometer- and acoustic-based behavioral classification, we were able to explore the composition of broad accelerometer categories revealing inter- and intra-individual differences in behavior. Our demonstration of the potential for accelerometers to assess behavioral responses of hares to moonlight revealed that hares adjust their time spent foraging as light conditions change.
Despite general difficulties in observing cryptic species, we successfully recorded behavior of a nocturnal species for up to 3 months continuously using biologging technology. We found that different technologies were best suited for extracting specific behavioral states and that a combination of technologies may be necessary to understand the complex nature of a species' behavior. For example, although accelerometers could classify foraging behavior, the act of chewing in snowshoe hares did not generate a measurable amount of acceleration. In this particular study, our capacity to detect chewing was limited by low sampling regime but it is also likely that some behavioral acts, like chewing, may be difficult to detect using acceleration at any sampling frequency without adjusting the attachment of the device to a different location (such as to the jaw; Iwata et al., 2012). However, chewing was a behavior that was easily identifiable on the acoustic recorders. Traveling, on the other hand, was more accurately distinguishable with accelerometers than through acoustics. Additionally, while we were significantly below the Nyquist criterion for a hare hop (Brown et al., 2013), we show that accurate classification is possible to accuracies (88.0%) comparable to those achieved (75% to 98%) using higher frequencies (3.3 to 40 Hz; Nathan et al., 2012; Bidder et al., 2014; McClune et al., 2014; Hammond et al., 2016). At this lower frequency, the detail of behavioral categories (e.g., foraging instead of feeding and individual hops) is compromised to maintain accuracy. This compromise will not suit researchers interested in describing steps or wingbeats over the span of days, but for researchers interested in behavioral changes over the span of months this will likely be a negligible cost.
Our use of animal-borne acoustic recorders not only provided a means of determining behavioral composition of accelerometer classification, but also demonstrated an alternative technique for collecting continuous behavioral data in circumstances when other methodologies might not be possible. Despite only recording on a few individuals (n = 3) for a short duration to test the potential of this technology, we revealed that individual and temporal variation exists in the composition of accelerometer-classified foraging and not moving categories. Although sounds like chewing were easily identifiable, other sounds could not be identified to a particular behavior with any confidence. For this reason, validating acoustic data with behavioral observations would be recommended in order to generate a more detailed classification than what we presented here. Acoustic recorders are rarely used for non-vocal behavior despite their commonality in animal communication research (e.g., Bee and Gerhardt, 2001; Reby and McComb, 2003; Fischer et al., 2004). However, successful applications of acoustics to record behavior all highlight the considerable wealth of information contained in this media form including bodily functions (e.g., heart rate; Couchoux et al., 2015), behavior (e.g., chewing, grooming, wingbeats; Ilany et al., 2013; Stowell et al., 2017; Wijers et al., 2018), and environmental noise (e.g., anthropogenic noise, wind, vocalization of other species; Lynch et al., 2015; Stowell et al., 2017).
Analyses of both accelerometer and acoustic information revealed that snowshoe hares split their time primarily between not moving and foraging-related behavior with limited time spent traveling. This is a similar pattern as seen in other hare species where vigilance, rest and feeding represented over 95% of the day (Lush et al., 2016). At least in winter, when nights are long and dark, we found that snowshoe hares are characterized by a single daily activity peak centered in the night, with elevated activity extending into dawn and dusk. The limited literature on the behavior of free-ranging snowshoe hares generally classifies the species as exhibiting either crepuscular (Murray, 2003) or nocturnal (Foresman and Pearson, 1999) activity patterns. Using the definition of Anderson and Wiens (2017) and Bartness and Albers (2000), snowshoe hares are likely best classified as a nocturnal species, but alternatively as a nocturnal species with activity that extends into the crepuscular period.
For nocturnal animals, moonlight can drastically change the landscape, and impacts the tradeoff between foraging and predation risk (Prugh and Golden, 2014; Gigliotti and Diefenbach, 2018). We found that moonlight conditions caused snowshoe hares to make substantial adjustments in behavior throughout the night, with the magnitude and direction being dependent on the light phase. During the darkest phases of the night (dusk to morning twilight), traveling was reduced by ~30% and foraging by ~6% when the moon was full. This reduction in foraging is considerably less than the average reduction found in foraging trials (13.6%) across all species where it has been tested (Prugh and Golden, 2014), but the disproportionate reduction in traveling to foraging suggests that the snowshoe hares were not moving around their environment in the same manner while foraging. These responses to moonlight confirm previous studies in snowshoe hares that have reported decreased activity and adjustments in habitat use under full moon conditions (Gilbert and Boutin, 1991; Griffin et al., 2005; Gigliotti and Diefenbach, 2018). Although we could not directly measure whether moonlight impacts predation risk, increased illumination at night has been linked to increased activity and feeding in various predators including coyotes (Kenaga et al., 2013) and Lynx spp. (Rockhill et al., 2013; Heurich et al., 2014). The reduction in activity under full moons suggests that snowshoe hares change their behavior to reduce risk of predation.
Hares appeared to behaviorally compensate for the lost foraging time associated with full moons via an extension of foraging into morning daylight hours. Such compensatory temporal shifts in behavior due to moonlight have also been shown to occur in other species (e.g., Dipodomys merriami; Daly et al., 1992). All animals have minimum energy intake requirements that must be achieved through daily foraging (Norberg, 1977) and although some species can reduce this requirement (e.g., through fat storage or use of torpor), snowshoe hares who have limited body fat stores (~ 4 days resting metabolic support; Whittaker and Thomas, 1983) must forage on a daily basis. This nutritional constraint seems to translate to drastic adjustments in temporally-explicit foraging choices rather than changes in overall foraging time as light conditions change (Gilbert and Boutin, 1991). If choice in timing of foraging is directly related to predation risk, then in hares, moonlight shifts risk of predation from relatively low to relatively high during the night to the extent that it is perceived to be safer to forage in the morning than maintain high foraging rates during the night.
A second compensation that seems to occur is that adjustments in behavior made during the night counteract any increases in predation risk that would be expected. We considered sprinting to be a measure of flightiness, as although some sprints occur from direct predator encounters, the majority are likely responses stemming from wary behavior (Vasquez et al., 2002). If the amount of flightiness is related to the amount of perceived predation risk, as would be expected, then the lack of effect of moonlight on sprinting during the night (Figure 7D) suggests that hares reduce their time spent foraging to minimize the level of predation risk, creating a constant level of risk across moonlight conditions. However, the increased sprinting during dawn and morning periods following full moon nights suggests the morning compensational foraging bouts come at a cost. This increased level of perceived predation risk may be due to the morning period being a time when all predators can be active. Predators of hares are characterized as nocturnal/crepuscular (Lynx canadensis, Canis latrans, Bubo virginianus), or diurnal/crepuscular (Accipiter gentilis), but many nocturnal species have considerable movement into and throughout the day, especially in winter, making the morning a time when both primarily nocturnal and diurnal predators may be hunting (Ozoga and Harger, 1966; Squires and Reynolds, 1997; Kolbe and Squires, 2007; Arias-Del Razo et al., 2011; Artuso et al., 2013). Despite this increased risk during the compensatory foraging, the strategy of reducing foraging during the night likely results in lower exposure to risk overall.
We additionally found that the extent of moonlight avoidance by snowshoe hares was dependent on the phase of the moon, with diminished foraging times when the moon was above the horizon on full moon nights and below the horizon on partial or new moon nights; a switching of preference that has not been observed previously. Generally in other species, individuals are found to be more active during the darkest hours when the moon is below the horizon regardless of moon phase (Morrison, 1978; Daly et al., 1992). This switching of preference for moonlight would suggest that risk is highest when light conditions are either at their brightest or darkest (Prugh and Golden, 2014). The main predators of snowshoe hares all rely on visual cues for hunting (Wells, 1978; Artuso et al., 2013). As such, a decrease in activity with increasing light may be a response to higher hunting efficiency from their predators through improved vision. Although the darkest conditions may inhibit the predators ability to hunt (Wells, 1978), these conditions will have similar effects on the hares ability to detect predators as they also rely partially on visual senses to detect danger. It may be that the loss of use of one of their senses is enough to cause the hares to select against these conditions for foraging, even if predators are similarly hindered.
Biologgers provide us with the opportunity to investigate detailed behavioral adjustments over long temporal timeframes revealing subtle and short-term responses to environmental change. Every biologging technique has strengths and weaknesses, however, combining biologging technology in complementary ways can allow a circumvention of such issues. Short duration acoustic recorders provided a method of collecting behavioral states of a free ranging nocturnal animal that were not possible with accelerometers and vice versa. This allowed for easier interpretation of the behavioral classification generated from long duration accelerometers. Such multi-faceted approaches will allow us to gain the most detailed insight yet into behavioral responses of species to environmental change.
This research conformed to the guidelines of the American Society of Mammalogists (Sikes et al., 2016) and was approved by the McGill University, University of Alberta, and University of Toronto Animal Care and Use Committees.
ES, AM, MH, MP, YM, and SB conceived the ideas. ES, AM, MP, YM, JS, and MB collected the data. ES analyzed the data and led the writing. All authors contributed critically to the ideas and drafts and gave final approval for publication.
The authors declare that the research was conducted in the absence of any commercial or financial relationships that could be construed as a potential conflict of interest.
The handling editor is currently co-organizing a Research Topic with two of the authors, DM and SB, and confirms the absence of any other collaboration.
We wish to thank all the field assistants who helped trap hares and Technosmart for troubleshooting devices for us. We thank Agnes MacDonald and her family for long-term access to her trapline, and the Champagne and Aishihik First Nations for supporting our work within their traditional territory. This work was supported by a Natural Sciences and Engineering Council of Canada (NSERC), the Canada Research Chairs program, Northern Studies Training Program, Wildlife Conservation Society, Canada Graduate Scholarship [to ES, MB, YM, and MP.], Vanier Graduate Scholarship [to AM], W. Garfield Weston Award for Northern Research [to ES, AM, and MP], and Ontario Graduate Scholarship [to JS and MB]; NSERC Discovery Grants [to SB, DM, and MH], and Institut Nordique du Quebec (INQ) Chair in Northern Research [to MH].
The Supplementary Material for this article can be found online at: https://www.frontiersin.org/articles/10.3389/fevo.2019.00154/full#supplementary-material
Audio 1. Example sound clip of chewing from acoustic recorders attached to snowshoe hares.
Audio 2. Example sound clip of hopping from acoustic recorders attached to snowshoe hares.
Audio 3. Example sound clip of silence from acoustic recorders attached to snowshoe hares.
Agafonkin, V., and Thieurmel, B. (2017). Suncalc: Computer Sun Position, Sunlight Phases, Moon Position and Lunar Phase. R package version 0.3.
Anderson, S. R., and Wiens, J. J. (2017). Out of the dark: 350 million years of conservatism and evolution in diel activity patterns in vertebrates. Evolution 71, 1944–1959. doi: 10.1111/evo.13284
Arias-Del Razo, I., Hernández, L., Laundré, J. W., and Myers, O. (2011). Do predator and prey foraging activity patterns match? A study of coyotes (Canis latrans), and lagomorphs (Lepus californicus and Sylvilagus audobonii). J. Arid Environ. 75, 112–118. doi: 10.1016/j.jaridenv.2010.09.008
Artuso, C., Houston, C. S., Smith, D. G., and Rohner, C. (2013). “Great Horned Owl (Bubo virginianus),” in The Birds of North America, ed A. F. Poole (Ithaca, NY: Cornell Lab of Ornithology).
Bartness, T., and Albers, H. (2000). “Activity patterns and the biological clock in mammals,” in Activity Patterns in Small Mammals: An Ecological Approach, eds S. Halle and N. Stenseth (Berlin: Springer-Verlag), 23–47. doi: 10.1007/978-3-642-18264-8_3
Bates, D., Maechler, M., Bolker, B., and Walker, S. (2015). Fitting linear mixed-effects models using lme4. J. Stat. Softw. 67, 1–48. doi: 10.18637/jss.v067.i01
Bee, M. A., and Gerhardt, H. C. (2001). Neighbour-stranger discrimination by territorial male bullfrogs (Rana catesbeiana): I. Acoustic basis. Anim. Behav. 62, 1129–1140. doi: 10.1006/anbe.2001.1851
Beutler, F. J. (1966). Error-free recovery of signals from irregularly spaced samples. SIAM Review 8, 328–335. doi: 10.1137/1008065
Bidder, O. R., Campbell, H. A., Gómez-Laich, A., Urgé, P., Walker, J., and Cai, Y., et al. (2014). Love thy neighbour: automatic animal behavioural classification of acceleration data using the K-nearest neighbour algorithm. PLoS ONE 9:e88609. doi: 10.1371/journal.pone.0088609
Bolker, B. M., Brooks, M. E., Clark, C. J., Geange, S. W., Poulsen, J. R., and Stevens, M. H. H., et al. (2009). Generalized linear mixed models: a practical guide for ecology and evolution. Trends Ecol. Evol. 24, 127–135. doi: 10.1016/j.tree.2008.10.008
Boonstra, R., Andreassen, H. P., Boutin, S., Hušek, J., Ims, R. A., and Krebs, C. J., et al. (2016). Why do the boreal forest ecosystems of Northwestern Europe differ from those of Western North America? Bioscience 66, 722–734. doi: 10.1093/biosci/biw080
Boudreau, M. R. (2019). The Cascading Effects of Risk in the Wild: How Snowshoe Hares Respond to the Threat of Predation, Ph.D dissertation. Trent University, 159.
Broell, F., Noda, T., Wright, S., Domenici, P., Steffensen, J. F., and Auclair, J. P., et al. (2013). Accelerometer tags: detecting and identifying activities in fish and the effect of sampling frequency. J. Exp. Biol. 216, 1255–1264. doi: 10.1242/jeb.077396
Brown, D. D., Kays, R., Wikelski, M., Wilson, R., and Klimley, A. (2013). Observing the unwatchable through acceleration logging of animal behavior. Anim. Biotelemetr. 1:20. doi: 10.1186/2050-3385-1-20
Campbell, H. A., Gao, L., Bidder, O. R., Hunter, J., and Franklin, C. E. (2013). Creating a behavioural classification module for acceleration data: using a captive surrogate for difficult to observe species. J. Exp. Biol. 216, 4501–4506. doi: 10.1242/jeb.089805
Chen, K. Y., and Bassett, D. R. (2005). The technology of accelerometry-based activity monitors: current and future. Med. Sci. Sports Exerc. 37, 490–500. doi: 10.1249/01.mss.0000185571.49104.82
Couchoux, C., Aubert, M., Garant, D., and Réale, D. (2015). Spying on small wildlife sounds using affordable collar-mounted miniature microphones: an innovative method to record individual daylong vocalisations in chipmunks. Sci. Rep. 5:10118. doi: 10.1038/srep10118
Daly, M., Behrends, P. R., Wilson, M. I., and Jacobs, L. F. (1992). Behavioural modulation of predation risk: moonlight avoidance and crepuscular compensation in a nocturnal desert rodent, Dipodomys merriami. Anim. Behav. 44, 1–9. doi: 10.1016/S0003-3472(05)80748-1
Depraetere, M., Pavoine, S., Jiguet, F., Gasc, A., Duvail, S., and Sueur, J. (2012). Monitoring animal diversity using acoustic indices: implementation in a temperate woodland. Ecol. Indic. 13, 46–54. doi: 10.1016/j.ecolind.2011.05.006
Elliott, K. H. (2016). Measurement of flying and diving metabolic rate in wild animals: Review and recommendations. Compar. Biochem. Physiol. Part A 202, 63–77. doi: 10.1016/j.cbpa.2016.05.025
Fischer, J., Kitchen, D. M., Seyfarth, R. M., and Cheney, D. L. (2004). Baboon loud calls advertise male quality: acoustic features and their relation to rank, age, and exhaustion. Behav. Ecol. Sociobiol. 56, 140–148. doi: 10.1007/s00265-003-0739-4
Foresman, K. R., and Pearson, D. E. (1999). Activity patterns of American martens, Martes americana, snowshoe hares, Lepus americanus, and red squirrels, Tamiasciurus hudsonicus, in westcentral Montana. Can. Field Natural. 113, 386–389.
Gasc, A., Sueur, J., Jiguet, F., Devictor, V., Grandcolas, P., and Burrow, C., et al. (2013). Assessing biodiversity with sound: do acoustic diversity indices reflect phylogenetic and functional diversities of bird communities? Ecol. Indic. 25, 279–287. doi: 10.1016/j.ecolind.2012.10.009
Gigliotti, L. C., and Diefenbach, D. R. (2018). Risky behavior and its effect on survival: snowshoe hare behavior under varying moonlight conditions. J. Zool. 305, 27–34. doi: 10.1111/jzo.12532
Gilbert, B., and Boutin, S. (1991). Effect of moonlight on winter activity of snowshoe hares. Arctic Alpine Res. 23, 61–65. doi: 10.2307/1551438
Gleiss, A. C., Wilson, R. P., and Shepard, E. L. C. (2011). Making overall dynamic body acceleration work: on the theory of acceleration as a proxy for energy expenditure. Methods Ecol. Evol. 2, 23–33. doi: 10.1111/j.2041-210X.2010.00057.x
Graf, P. M., Wilson, R. P., Qasem, L., Hackländer, K., Rosell, F., and Falcone, E. (2015). The use of acceleration to code for animal behaviours; a case study in free-ranging Eurasian beavers Castor fiber. PLoS ONE 10:e0136751. doi: 10.1371/journal.pone.0136751
Griffin, P. C., Griffin, S. C., Waroquiers, C., and Mills, L. S. (2005). Mortality by moonlight: predation risk and the snowshoe hare. Behav. Ecol. 16, 938–944. doi: 10.1093/beheco/ari074
Hammond, T. T., Springthorpe, D., Walsh, R. E., and Berg-Kirkpatrick, T. (2016). Using accelerometers to remotely and automatically characterize behavior in small animals. J. Exp. Biol. 2, 1618–1624. doi: 10.1242/jeb.136135
Heurich, M., Hilger, A., Küchenhoff, H., Andrén, H., Bufka, L., and Krofel, M., et al. (2014). Activity patterns of Eurasian lynx are modulated by light regime and individual traits over a wide latitudinal range. PLoS ONE 9:e114143. doi: 10.1371/journal.pone.0114143
Ilany, A., Barocas, A., Kam, M., Ilany, T., and Geffen, E. (2013). The energy cost of singing in wild rock hyrax males: evidence for an index signal. Anim Behav. 85, 995–1001. doi: 10.1016/j.anbehav.2013.02.023
Iwata, T., Sakamoto, K. Q., Takahashi, A., Edwards, E. W. J., Staniland, I. J., and Trathan, P. N., et al. (2012). Using a mandible accelerometer to study fine-scale foraging behavior of free-ranging Antarctic fur seals. Mar. Mamm. Sci. 28, 345–357. doi: 10.1111/j.1748-7692.2011.00482.x
Johnson, M. D., and De León, Y. L. (2015). Effect of an invasive plant and moonlight on rodent foraging behavior in a coastal dune ecosystem. PLoS ONE 10:e0117903. doi: 10.1371/journal.pone.0117903
Kenaga, B. A., Krebs, R. A., and Clapham, W. B. (2013). Coyote land use inside and outside urban parks. Am. Midl. Nat. 170, 298–310. doi: 10.1674/0003-0031-170.2.298
Kolbe, J. A., and Squires, J. R. (2007). Circadian activity patterns of Canada lynx in Western Montana. J. Wildlife Manage. 71, 1607–1611. doi: 10.2193/2005-727
Krebs, C. J., Boonstra, R., and Boutin, S. (2018). Using experimentation to understand the 10-year snowshoe hare cycle in the boreal forest of North America. J. Anim. Ecol. 87, 87–100. doi: 10.1111/1365-2656.12720
Krebs, C. J., Boutin, S., Boonstra, R., Sinclair, A. R. E., Smith, J. N. M., and Dale, M. R. T., et al. (1995). Impact of food and predation on the snowshoe hare cycle. Science 269, 1112–1115. doi: 10.1126/science.269.5227.1112
Lush, L., Ellwood, S., Markham, A., Ward, A. I., and Wheeler, P. (2016). Use of tri-axial accelerometers to assess terrestrial mammal behaviour in the wild. J. Zool. 298, 257–265. doi: 10.1111/jzo.12308
Lynch, E., Angeloni, L., Fristrup, K., Joyce, D., and Wittemyer, G. (2013). The use of on-animal acoustical recording devices for studying animal behavior. Ecol. Evol. 3, 2030–2037. doi: 10.1002/ece3.608
Lynch, E., Northrup, J. M., McKenna, M. F., Anderson, C. R., Angeloni, L., and Wittemyer, G. (2015). Landscape and anthropogenic features influence the use of auditory vigilance by mule deer. Behav. Ecol. 26, 75–82. doi: 10.1093/beheco/aru158
Martin, T. G., Wintle, B. A., Rhodes, J. R., Kuhnert, P. M., Field, S. A., and Low-Choy, S. J., et al. (2005). Zero tolerance ecology: improving ecological inference by modelling the source of zero observations. Ecol. Lett. 8, 1235–1246. doi: 10.1111/j.1461-0248.2005.00826.x
McClune, D. W., Marks, N. J., Wilson, R. P., Houghton, J. D., Montgomery, I. W., and McGowan, N. E., et al. (2014). Tri-axial accelerometers quantify behaviour in the Eurasian badger (Meles meles): towards an automated interpretation of field data. Anim. Biotelemetr. 2:5. doi: 10.1186/2050-3385-2-5
Morrison, D. W. (1978). Lunar phobia in a neotropical fruit bat, Artibevs jamaicensis (Chiroptera: Phyllostomidae). Anim. Behav. 26, 852–855. doi: 10.1016/0003-3472(78)90151-3
Murray, D. (2003). “Snowshoe hares and other hares,” in Wild Mammals of North America: Biology, Management and Conservation, 2nd Edn, eds G. Feldhamer, B. Thompson, and J. Chapman (Baltimore, MD: John Hopkins University Press), 147–157.
Nathan, R., Spiegel, O., Fortmann-Roe, S., Harel, R., Wikelski, M., and Getz, W. M. (2012). Using tri-axial acceleration data to identify behavioral modes of free-ranging animals: general concepts and tools illustrated for griffon vultures. J. Exp. Biologyxperiment. Biol. 215(Pt 6), 986–996. doi: 10.1242/jeb.058602
Norberg, R. A. (1977). An ecological theory on foraging time and energetics and choice of optimal food-searching method. J. Anim. Ecol. 46, 511–529. doi: 10.2307/3827
O'Donoghue, M., Boutin, S., Krebs, C. J., Zuleta, G., Murray, D. L., and Hofer, E. J. (1998). Functional responses of coyotes and lynx to the snowshoe hare cycle. Ecology 79, 1193–1208. doi: 10.2307/176736
Ozoga, J. J., and Harger, E. M. (1966). Winter activities and feeding habits of northern michigan coyotes. J. Wildlife Manage. 30, 809–818. doi: 10.2307/3798288
Pagano, A. M., Durner, G., Rode, K., Atwood, T., Atkinson, S., and Peacock, E., et al. (2018). High-energy, high-fat lifestyle challenges an Arctic apex predator, the polar bear. Science 359, 568–572. doi: 10.1126/science.aan8677
Pijanowski, B. C., Villanueva-Rivera, L. J., Dumyahn, S. L., Farina, A., Krause, B. L., and Napoletano, B. M., et al. (2011). Soundscape ecology: the science of sound in the landscape. Bioscience 61, 203–216. doi: 10.1525/bio.2011.61.3.6
Prugh, L. R., and Golden, C. D. (2014). Does moonlight increase predation risk? Meta-analysis reveals divergent responses of nocturnal mammals to lunar cycles. J. Anim. Ecol. 83, 504–514. doi: 10.1111/1365-2656.12148
Reby, D., and McComb, K. (2003). Anatomical constraints generate honesty: acoustic cues to age and weight in the roars of red deer stags. Anim. Behav. 65, 519–530. doi: 10.1006/anbe.2003.2078
Rockhill, A. P., DePerno, C. S., Powell, R. A., Baker, L., Warren, R., and Diefenbach, D., et al. (2013). The effect of illumination and time of day on movements of bobcats (Lynx rufus). PLoS ONE 8:e69213. doi: 10.1371/journal.pone.0069213
Rohner, C., and Krebs, C. J. (1996). Owl predation on snowshoe hares: consequences of antipredator behaviour. Oecologia 108, 303–310. doi: 10.1007/BF00334655
Sandor, B. J., Jensen, E. J., Stone, E. M., Read, W. G., Waters, J. W., and Mergenthaler, J. L. (2000). Upper tropospheric humidity and thin cirrus. Geophys. Res. Lett. 27, 2645–2648. doi: 10.1029/1999GL011194
Shepard, E., Wilson, R., Quintana, F., Gómez Laich, A., Liebsch, N., and Albareda, D., et al. (2008). Identification of animal movement patterns using tri-axial accelerometry. Endanger. Species Res. 10, 47–60. doi: 10.3354/esr00084
Sheriff, M. J., Krebs, C. J., and Boonstra, R. (2009). The sensitive hare: sublethal effects of predator stress on reproduction in snowshoe hares. J. Anim. Ecol. 78, 1249–1258. doi: 10.1111/j.1365-2656.2009.01552.x
Sikes, R. S., Mammalogists, A. C., and Animal Care Use Committee of the American Society of Mammalogists. (2016). Guidelines of the american society of mammalogists for the use of wild mammals in research. J. Mammal. 97, 663–688. doi: 10.1093/jmammal/gyw078
Spivey, R. J., and Bishop, C. M. (2013). Interpretation of body-mounted accelerometry in flying animals and estimation of biomechanical power. J. R. Soc. Interfaces 10, 20130404–20130404. doi: 10.1098/rsif.2013.0404
Squires, J. R., and Reynolds, R. T. (1997). “Northern Goshawk (Accipiter gentilis),” in The Birds of North America, eds A. F. Poole and F. B. Gill (Ithaca, NY: Cornell Lab of Ornithology). doi: 10.2173/bna.298
Stowell, D., Benetos, E., and Gill, L. F. (2017). On-bird sound recordings: automatic acoustic recognition of activities and contexts. IEEE/ACM Transac. Audio Speech Lang. Proces. 25, 1193–1206. doi: 10.1109/TASLP.2017.2690565
Studd, E. K., Landry-Cuerrier, M., Menzies, A. K., Boutin, S., Mcadam, A. G., and Lane, J. E., et al. (2019). Behavioral classification of low-frequency acceleration and temperature data from a free-ranging small mammal. Ecol. Evol. 9, 619–630. doi: 10.1002/ece3.4786
Sueur, J., Aubin, T., and Simonis, C. (2008). Seewave: a free modular tool for sound analysis and sythesis. Bioacoustics 18, 213–226. doi: 10.1080/09524622.2008.9753600
Tatler, J., Cassey, P., and Prowse, T. A. A. (2018). High accuracy at low frequency: detailed behavioural classification from accelerometer data. J. Exp. Biol. 221:jeb184085. doi: 10.1242/jeb.184085
Thiebault, A., Pistorius, P., Mullers, R., and Tremblay, Y. (2016). Seabird acoustic communication at sea: a new perspective using bio-logging devices. Sci. Rep. 6:30972. doi: 10.1038/srep30972
Thompson, I. D., Bakhtiari, M., Rodgers, A. R., Baker, J. A., Fryxell, J. M., and Iwachewski, E. (2012). Application of a high-resolution animal-borne remote video camera with global positioning for wildlife study: observations on the secret lives of woodland caribou. Wildl. Soc. Bull. 36, 365–370. doi: 10.1002/wsb.130
Vasquez, R. A., Ebensperger, L. A., and Bozinovic, F. (2002). The influence of habitat on travel speed, intermittent locomotion, and vigilance in a diurnal rodent. Behav. Ecol. 13, 182–187. doi: 10.1093/beheco/13.2.182
Wells, M. C. (1978). Coyote senses in predation: environmental influences on their relative use. Behav. Proc. 3, 149–158. doi: 10.1016/0376-6357(78)90041-4
Whittaker, M. E., and Thomas, V. G. (1983). Seasonal levels of fat and protein reserves of snowshoe hares in Ontario. Can. J. Zool. 61, 1339–1345. doi: 10.1139/z83-180
Wijers, M., Trethowan, P., Markham, A., du Preez, B., Chamaillé-Jammes, S., and Loveridge, A., et al. (2018). Listening to lions: animal-borne acoustic sensors improve bio-logger calibration and behaviour classification performance. Front. Ecol. Evol. 6:171. doi: 10.3389/fevo.2018.00171
Williams, C. T., Barnes, B. M., and Buck, C. L. (2016). Integrating physiology, behavior, and energetics: Biologging in a free-living arctic hibernator. Compar. Biochem. Physiol. Part A 202, 53–62. doi: 10.1016/j.cbpa.2016.04.020
Williams, C. T., Barnes, B. M., Yan, L., and Buck, C. L. (2017). Entraining to the polar day: circadian rhythms in arctic ground squirrels. J. Exp. Biol. 220(Pt 17), 3095–3102. doi: 10.1242/jeb.159889
Wilmers, C. C., Nickel, B., Bryce, C. M., Smith, J. A., Wheat, R. E., and Yovovich, V. (2015). The golden age of bio-logging: how animal-borne sensors are advancing the frontiers of ecology. Ecology 96, 1741–1753. doi: 10.1890/14-1401.1
Wilson, R. P., White, C. R., Quintana, F., Halsey, L. G., Liebsch, N., and Martin, G. R., et al. (2006). Moving towards acceleration for estimates of activity-specific metabolic rate in free-living animals: the case of the cormorant. J. Anim. Ecol. 75, 1081–1090. doi: 10.1111/j.1365-2656.2006.01127.x
Zuur, A. F., Ieno, E. N., Walker, N. J., Saveliev, A. A., and Smith, G. M. (eds.). (2009). “Zero-truncated and zero-Inflated models for count data,” in Mixed Effects Models and Extensions in Ecology With R. Statistics for Biology and Health (New York, NY: Springer), 261–293. doi: 10.1007/978-0-387-87458-6_11
Keywords: accelerometer, biologging, boreal forest, environmental acoustics, Lepus americanus, lunar phases, nocturnal behavior, snowshoe hare
Citation: Studd EK, Boudreau MR, Majchrzak YN, Menzies AK, Peers MJL, Seguin JL, Lavergne SG, Boonstra R, Murray DL, Boutin S and Humphries MM (2019) Use of Acceleration and Acoustics to Classify Behavior, Generate Time Budgets, and Evaluate Responses to Moonlight in Free-Ranging Snowshoe Hares. Front. Ecol. Evol. 7:154. doi: 10.3389/fevo.2019.00154
Received: 21 August 2018; Accepted: 23 April 2019;
Published: 08 May 2019.
Edited by:
Frants Havmand Jensen, Woods Hole Oceanographic Institution, United StatesReviewed by:
Ariana Strandburg-Peshkin, Universität Konstanz, GermanyCopyright © 2019 Studd, Boudreau, Majchrzak, Menzies, Peers, Seguin, Lavergne, Boonstra, Murray, Boutin and Humphries. This is an open-access article distributed under the terms of the Creative Commons Attribution License (CC BY). The use, distribution or reproduction in other forums is permitted, provided the original author(s) and the copyright owner(s) are credited and that the original publication in this journal is cited, in accordance with accepted academic practice. No use, distribution or reproduction is permitted which does not comply with these terms.
*Correspondence: Emily K. Studd, ZW1pbHkuc3R1ZGRAbWFpbC5tY2dpbGwuY2E=
Disclaimer: All claims expressed in this article are solely those of the authors and do not necessarily represent those of their affiliated organizations, or those of the publisher, the editors and the reviewers. Any product that may be evaluated in this article or claim that may be made by its manufacturer is not guaranteed or endorsed by the publisher.
Research integrity at Frontiers
Learn more about the work of our research integrity team to safeguard the quality of each article we publish.