- 1AgResearch, Ruakura Research Centre, Hamilton, New Zealand
- 2AgResearch, Grasslands Research Centre, Palmerston North, New Zealand
- 3Riddet Institute, Massey University, Palmerston North, New Zealand
- 4School of Food and Advanced Technology, Massey University, Palmerston North, New Zealand
- 5High-Value Nutrition National Science Challenge, Auckland, New Zealand
Bacterial feeding has evolved toward specific evolutionary niches and the sources of energy differ between species and strains. Although bacteria fundamentally compete for nutrients, the excreted products from one strain may be the preferred energy source or a source of essential nutrients for another strain. The large variability in feeding preferences between bacterial strains often provides for complex cross-feeding relationships between bacteria, particularly in complex environments such as the human lower gut, which impacts on the host's digestion and nutrition. Although a large amount of information is available on cross-feeding between bacterial strains, it is important to consider the evolution of cross-feeding. Adaptation to environmental stimuli is a continuous process, thus understanding the evolution of microbial cross-feeding interactions allows us to determine the resilience of microbial populations to changes to this environment, such as changes in nutrient supply, and how new interactions might emerge in the future. In this review, we provide a framework of terminology dividing bacterial cross-feeding into four forms that can be used for the classification and analysis of cross-feeding dynamics. Under the proposed framework, we discuss the evolutionary origins for the four forms of cross-feeding and factors such as spatial structure that influence their emergence and subsequent persistence. This review draws from both the theoretical and experimental evolutionary literature to provide a cross-disciplinary perspective on the evolution of different types of cross-feeding.
Introduction
All bacteria require an energy source for maintenance of cellular functions, growth, and reproduction, although these energy sources may differ between species and between strains. Bacteria compete for nutrients, but also the product of one strain's metabolism may be utilised in the nutrition of another, in a process termed metabolic cross-feeding. Due to the great variety in feeding preferences displayed by different bacterial strains, cross-feeding relationships between bacteria can be complex. While much study of cross-feeding between bacterial strains focusses on the present state of these relationships, it is important to take into account the evolutionary history of cross-feeding. With an understanding of how cross-feeding interactions have been selected for and have then propagated in a population, we may better predict how a cross-feeding population will respond to changes in nutrient availability, or how new interactions might emerge in the future, be this naturally or by synthetic means. Such an understanding necessitates interdisciplinary research between evolutionary biology, ecology, nutrition, and microbiology, therefore accurate communication across the fields of study is vital.
In any discussion of bacterial interaction, it is essential that terminology is unambiguously defined and in line with published literature. Although this can be challenging when the body of literature is large and varied, drawn as it is from many different biological fields, the importance of clarity cannot be understated. We will provide a framework of terminology that can be applied in the discussion of cross-feeding dynamics, since there are currently inconsistencies of language both within and between fields (West et al., 2007b).
Under the established framework, it will be possible to discuss sequentially how increasingly complex cross-feeding relationships may evolve. Alongside this, we must go beyond the first instance of trait evolution and consider factors influencing their subsequent persistence. Studying evolution is experimentally challenging, particularly in large and increasingly complex cross-feeding networks, such as those found in the gut. Many of the cross-feeding instances in this review involve bacteria found in the human gut. The justification for this is two-fold: firstly, study of cross-feeding by human gut microbes is abundant in the literature; and secondly, the health and nutritional impacts of the gut microbiota urgently encourage greater understanding of all aspects of this population, including its evolutionary behaviour (Macfarlane and Macfarlane, 2012; Maynard et al., 2012; Flint and Juge, 2015). Due to the difficulty in studying large systems physically, mathematical modelling is a powerful tool for inference when experimental systems are unmanageable. This review draws on both the experimental and theoretical evolutionary literature, to give a more complete perspective on the evolution of different cross-feeding types.
Classification of Bacterial Cross-Feeding
There are many forms of nutrient exchange between bacteria and the language used to classify these interactions is varied. Such variation in terminology can lead to different definitions for a single term, or multiple terms being used to describe the same interaction. This is particularly true of terminology related to metabolic cross-feeding, which is not consistent across the literature and has a number of suggested subclasses. A small number of publications studying cross-feeding have merely termed it “interaction” or “symbiotic interaction” (Helling et al., 1987; Hansen et al., 2007). “Incidental cross-feeding” has also been used to describe certain one-way interactions (Bull and Harcombe, 2009), and there are also those who only use cross-feeding if the interaction studied involves a two-way exchange of nutrients (West et al., 2006; Sun et al., 2019). In one instance, cross-feeding relationships were differentiated by the ability of the cross-feeder to utilise the sole added energy source, in addition to the excreted product of the primary degrader (Moens et al., 2016). These authors used the terms “Type one” and “Type two” cross-feeding, but this classification lacks memorability and is highly specific to their experiment. We define metabolic cross-feeding as an interaction between bacterial strains in which molecules resulting from the metabolism of one strain are further metabolised by another strain. In general, the term “metabolic cross-feeding” is used according to this definition, though not further sub-divided according to characteristics of the relationship, as we shall here (Porcher et al., 2001; Doebeli, 2002; Duncan et al., 2002; Belenguer et al., 2006, 2008; MacLean et al., 2010; Egan et al., 2014; Rivière et al., 2015; Pacheco et al., 2019).
Closely related to cross-feeding is the term “syntrophy,” which has been defined as “obligately mutualistic metabolism” (Morris et al., 2013). This definition is inclusive of behaviour such as detoxification of the environment by one strain, if that detoxification is necessary for the metabolism of the mutualist partner (Oliveira et al., 2014). Such relationships have also been referred to as reciprocal syntrophy (Kim et al., 2008) or syntrophic cooperation (Hillesland and Stahl, 2010). Alternatively, Pande and Kost (2017) defined syntrophic interactions to be those “in which two or more microorganisms obligately exchange essential metabolites,” which would not include detoxification. In a recent review, Hillesland (2018) stated that this condition of dependence on the relationship is not fixed, and that only some organisms are obligately syntrophic. Another definition of syntrophy in the literature describes the combined capabilities of two or more organisms being necessary for degradation of an otherwise unavailable substrate (Stams and Plugge, 2009), while yet another corresponds to any two-way exchange of nutrients (Gudelj et al., 2016). This evidence of variation in the use of terminology in the field encourages the formulation of an explicitly defined set of terms for the discussion of metabolic cross-feeding.
We begin to address this with experimental examples of cross-feeding in one direction only, which we separate into two distinct classes. Belenguer et al. (2006) observed a Eubacterium hallii strain utilising lactate produced by bifidobacteria in co-culture. Importantly, lactate cannot be further metabolised by these bifidobacteria. Correspondingly, we define metabolite cross-feeding as the feeding of one bacterial strain on molecules produced by another, given that these molecules cannot be further metabolised by the producing bacterium, and are therefore waste products. Our second example is the growth of bifidobacterial strains on partially degraded oligofructose or inulin, resultant from the metabolism of Bacteroides thetaiotaomicron in co-culture (Falony et al., 2009). It is believed that extracellular degradation of the carbon source by B. thetaiotaomicron results in the accumulation of shorter chain sugars in the environment, which are substrates for both B. thetaiotaomicron and the bifidobacteria. We therefore define substrate cross-feeding as the feeding of one strain on molecules produced by the metabolic actions of another, which may still be further metabolised by either strain (Figure 1). These relationships were described in the mathematical modelling work of Van Wey et al. (2016) and, since all these strains are found in the human gut, these examples of cross-feeding may be expected to occur there.
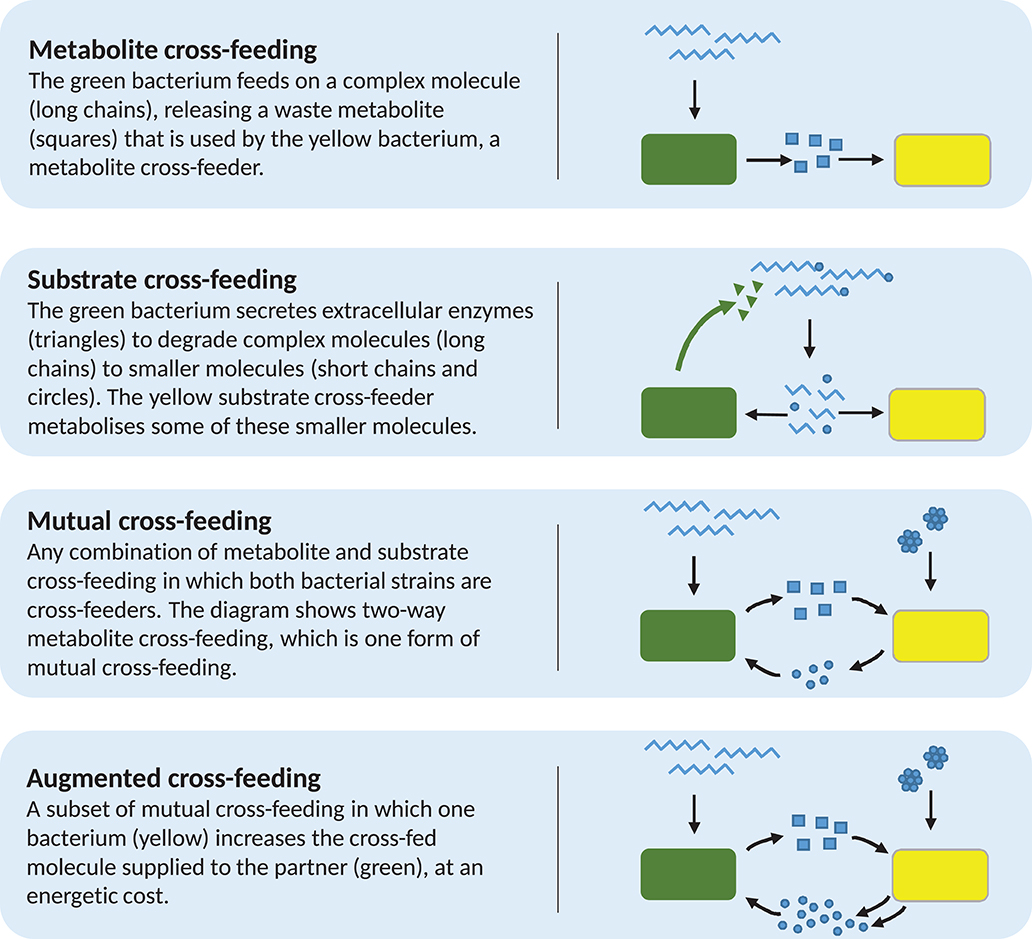
Figure 1. Diagrammatic examples of each of the four forms of cross-feeding discussed in the text. Note that the example for augmented cross-feeding is identical to that of mutual cross-feeding, but for the increased metabolite production by the yellow bacterium. This is reflective of the fact that augmented cross-feeding is mutual cross-feeding with the condition that one or both strains involved must increase the supply of metabolite to their partner, at some energetic cost.
Combinations of both these forms of cross-feeding are also observed. The gut bacterium Anaerostipes caccae has been shown to utilise both fructose and lactate as energy sources (Moens et al., 2017). Separately, Lactobacillus acidophilus, when grown on oligofructose as the sole added carbon source, liberates free fructose via extracellular degradation of oligofructose, before importing this sugar for further metabolism. Lactobacillus acidophilus then produces lactate as a metabolic end-product. When in co-culture on oligofructose, A. caccae is able to use both the liberated fructose and the secreted lactate produced by L. acidophilus, displaying both substrate and metabolite cross-feeding.
Disposal of waste is a necessary action; thus, metabolite cross-feeding does not negatively affect the excretor. However, substrate cross-feeding is not commensal. As mentioned above, many bacteria secrete extracellular enzymes for the purpose of degrading a complex molecule to simpler constituents, which can subsequently be taken up by the cell for further metabolism [see, for example, Rossi et al. (2005) and Amaretti et al. (2007)]. In the intervening period between breakdown and uptake by the primary degrader, these constituents are also available to other bacteria in the immediate environment. This can create competition for the breakdown products. Thus, it may be that an extracellular enzyme secretor may show reduced growth when growing in co-culture with a substrate cross-feeder, as compared to growing in monoculture. This has been shown between two human gut-inhabiting sugar degraders. A Bifidobacterium breve strain, previously able to achieve only limited growth on gut-derived mucins, showed improved growth on mucins when in co-culture with Bifidobacterium bifidum (Egan et al., 2014). This was due to the extracellular degradation of mucin by B. bifidum, resulting in the release of galactose-containing oligosaccharides into the medium. Bifidobacterium bifidum showed reduced growth in co-culture with B. breve compared to monoculture, thought to be a result of the competitive substrate cross-feeding, though the authors simply term it cross-feeding.
Continuing the classification of interactions and avoiding the inconsistencies around the term syntrophy, we next define mutual cross-feeding to be the case in which both bacterial strains involved are engaged in cross-feeding on metabolites produced during the metabolism of the other (Figure 2; Rivière et al., 2015). Mutual cross-feeding is a subset of mutualism in which all benefits are nutritional. An example of such a feeding strategy has been shown in Escherichia coli populations that are auxotrophic (unable to synthesise critical organic compounds required for survival). Mee et al. (2014) engineered several strains, each unable to synthesise a certain essential amino acid. No strains were able to grow in monoculture, but certain co-cultures of two strains with differing auxotrophies achieved growth due to two-way exchange of the necessary amino acids, via secretion into the extracellular environment.
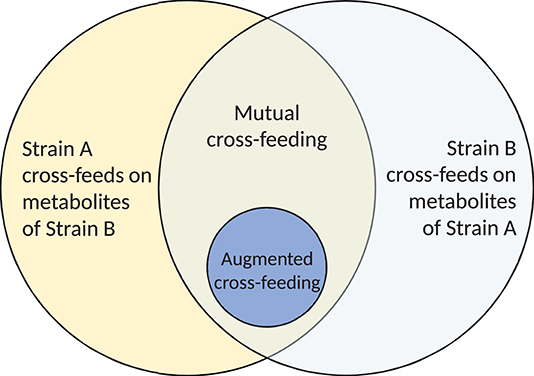
Figure 2. A Venn diagram illustrating the nature of mutual and augmented cross-feeding. Mutual cross-feeding requires that each strain is cross-feeding on products produced in the metabolism of the other. Each partner must be engaged in metabolite and/or substrate cross-feeding. Augmented cross-feeding relationships form a subset of mutual cross-feeding relationships.
Before presenting our final cross-feeding classification, we must discuss the concept of cooperation in ecology. Among the enormous quantity of ecological and biological studies mentioning cooperation, there are two widely referenced publications that define it. Sachs et al. (2004) would consider all forms of cross-feeding to be cooperation. Their definition of cooperation—action that is beneficial to the recipient regardless of its effect on the donor—is used by many (Velicer and Vos, 2009; Nadell et al., 2010; Wintermute and Silver, 2010a; Damore and Gore, 2012; Sachs and Hollowell, 2012; Momeni et al., 2013). However, West et al. (2006) have given a tighter definition, stating that selection for the beneficial trait in the donor must, at least partially, be due to its beneficial effect on the recipient. This definition has also been widely adopted in the literature (Bull and Harcombe, 2009; Estrela and Gudelj, 2010; Foster and Bell, 2012; Pande et al., 2014, 2016; Douglas et al., 2016; Germerodt et al., 2016), and would include only certain forms of mutual cross-feeding. It may be challenging to classify interactions as cooperative under this definition due to the type of information required. For example, two bacterial strains may be engaged in mutual cross-feeding, each utilising a metabolic end-product of the other; but is selection for this relationship purely due to the need to excrete waste, or is increased excretion for the strengthening of the mutualism present? Although not a naturally evolved relationship, we may still ask whether the mutual cross-feeding observed by Mee et al. (2014) is cooperative, i.e., will the exchange of amino acids be further selected for due to its benefit to the auxotrophic partner? This seems unlikely given the strains' prototrophic origin, but it would be non-trivial to prove (De Mazancourt et al., 2005). This issue is compounded by the fact that, in similar work with engineered auxotrophic E. coli strains, the secretion of amino acids by one of the strains was shown to increase over the course of mere hours in co-culture, but not in to monoculture (Hosoda et al., 2011). This increase is likely due to the observed upregulation of many genes involved in anabolic metabolism, rather than selection for individual strains that produce more amino acids. Such phenotypic plasticity makes classification of cooperative behaviour yet more difficult. Moreover, the argument could be made that the term cooperation implies some degree of communication or coordination between individuals. Bacterial communication, especially quorum sensing, has received much attention in recent years and has been implicated in bacterial metabolism [see review by Goo et al. (2015)]. However, the addition of communication to any definition of cooperation would present further challenges to its classification. For these reasons, great care must be taken with the use and interpretation of this term.
Despite the difficulties around cooperation as a classification of microbial cross-feeding interactions, there exists a further interaction, in referring to which many authors have used the term “cooperative cross-feeding” (Bull and Harcombe, 2009; Estrela and Gudelj, 2010; Pande et al., 2014, 2016; Germerodt et al., 2016). These interactions are a subset of mutual cross-feeding (Figure 2) in which one or both partners undertake an activity at energetic cost that increases the supply of the cross-fed molecule to the partner (Figure 1). Other authors have termed this by-product reciprocity (Connor, 1995; Sachs et al., 2004; West et al., 2006, 2007b), which avoids the previously stated difficulties around the term cooperation. A recent review considering the role of cross-feeding in bacterial unculturability uses both “by-product reciprocity” and “cooperative cross-feeding” to refer to mutual cross-feeding in which the interaction is energetic in one or both directions, respectively (Pande and Kost, 2017). To imply the increased energetic investment present in these interactions, we prefer the term augmented cross-feeding, defined as a mutual cross-feeding relationship in which the production of the cross-fed molecules (in one or both directions) incurs an energetic cost to the producing strain. This costly production would be disadvantageous to the producer in the absence of the mutualism, which could be tested by comparing its monoculture growth to that of an ancestor that does not perform the costly production.
To give an example of augmented cross-feeding, we again refer to genetically engineered E. coli. Comparably to Mee et al. (2014), auxotrophy for one amino acid was combined with overproduction of another in each engineered strain (Pande et al., 2014). However, in this case many more permutations of auxotrophy and over production were investigated. These strains were constructed and paired in culture in such a way that the auxotrophy of one partner corresponded to the overproduction of the other. Many of these augmented cross-feeding cultures were successful in terms of growth, despite the fact that overproduction was shown to come at a fitness cost to the bacterium when compared to its wild type ancestor. Augmented cross-feeding is the final term that we define and use in this review. Our classifications allow for easier comparison between observations of cross-feeding, without the need to delve into the biological mechanics of each individual interaction. We now discuss how the defined interactions may have evolved.
Evolution of Bacterial Cross-Feeding
Ecologists have heavily studied inter-species interactions for many decades and a well-developed theory exists for how these interactions may have evolved. Bacteria are particularly attractive for the study of evolution due to their rapid asexual reproduction, the ease with which their genetic code can be artificially altered, and our ability to control environmental variables in cultures (Elena and Lenski, 2003; West et al., 2007a; Hillesland, 2018). There is also the possibility of time-shift experiments, in which strains from different stages in evolutionary history may be co-cultured to investigate the change in relative fitness over generations. Such experiments can also facilitate study of coevolution, where the two strains involved in a cross-feeding relationship may adapt to one another [see review by Hillesland (2018)]. Alongside in vitro approaches, mathematical modelling is popular among evolutionary biologists, since the extended time periods necessary for evolution can often be simulated in silico in mere minutes to provide further insight into observed results (Widder et al., 2016). These mathematical models can be used to test theories and identify new experiments and hypotheses on the nature and evolution of microbial interactions.
An important phenomenon in ecology is the occurrence of multiple different species feeding upon a single resource. This observation should be precluded by the competitive exclusion principle, whereby, if two species are in direct competition for a single resource, the population should converge to a single species with the greatest fitness to survive on that resource (Hardin, 1960). The existence of cross-feeding interactions in which multiple species may persist in a single resource environment demonstrates a possible exception to this principle, and we now examine how such arrangements may have evolved.
Specialisation
In addition to observing the evolution of bacterial populations, it is also useful to observe how these evolutionary changes persist over time. The Long-Term Evolution Experiment (LTEE), early results of which were reported by Lenski et al. (1991), has now been conducted for more than 25 years, involving over 60,000 generations of the cultured E. coli population (Lenski, 2017). One result of this experiment was the emergence, from an originally clonal population, of two phenotypically and genotypically distinct strains, small and large (S and L, respectively), differentiable by their colony formation morphology and cell size (Rozen and Lenski, 2000). Since their first isolation, these strains have been shown to coexist for over 12,000 generations (Rozen et al., 2005). Not only are these strains visibly different, but strain L also shows a greater maximum growth rate on glucose, whereas strain S has increased relative fitness in co-culture after glucose depletion. When S was grown in monoculture on a combination of glucose and cell-free supernatant of L, it achieved a significantly higher growth rate than when grown on glucose alone. This implies cross-feeding by S on one or more molecules produced by L (Rozen and Lenski, 2000).
We must consider how cross-feeding between two differentially evolved strains may have arisen. Analysis of samples obtained periodically throughout the LTEE demonstrated that the L strain emerged first, before the subsequent emergence of the newly evolved S strain, from which point the two have coexisted (Rozen and Lenski, 2000; Rozen et al., 2005; Lenski, 2017). Importantly, the S strain appears not to have evolved from the L strain, but from a shared ancestral strain, which was outcompeted by the evolved L and S strains (Rozen et al., 2005). Similar dynamics have been discussed elsewhere (Rosenzweig et al., 1994; Treves et al., 1998), and the hypothesised evolutionary route is as follows. Strain L evolves from the ancestral strain with an increased growth rate on glucose, giving it an advantage and allowing it to persist in the ancestral population. However, the fact that the L strain did not outcompete its ancestor implies some mechanism by which the two were able to coexist. This mechanism was thought to be a trade-off between glucose uptake and the use of some unknown metabolic intermediate molecule that could be utilised by either strain: the L strain's increased glucose usage results in more of this intermediate molecule being released into the medium, which can be better utilised by the ancestral strain. This increase in concentration of the intermediate molecule provides an advantage for a second mutant, S, which attains better growth on this molecule than either the ancestor or the L strain. As a result, the S strain is also able to persist in the population, but does not dominate due to its dependency on the intermediate molecule (Lenski, 2017).
More recent follow-up experimentation has provided supportive evidence for the trade-off between growth efficiency on glucose and the unknown intermediate molecule when experimenting with historical LTEE samples (Großkopf et al., 2016). These authors gave evidence that acetate was the molecule upon which S had a growth advantage, whereas L specialised in glucose uptake, resulting in increased acetate excretion. It has been proposed that the excretion of acetate frees phosphoryl groups in the cell, which are required for glucose uptake (Rosenzweig et al., 1994). Thus, increased acetate excretion by strain L would facilitate increased glucose uptake. This has been supported by more recent experimental and modelling studies (Treves et al., 1998; Kumari et al., 2000; Großkopf et al., 2016; Enjalbert et al., 2017). If, as has been suggested (Großkopf et al., 2016; Lenski, 2017), strain L has lost the ability to metabolise acetate, then L and S have a metabolite cross-feeding relationship, although this has not been fully elucidated and continued evolution may result in this classification changing over time.
To examine abstractly how diversification such as that observed in the LTEE may occur, Doebeli (2002) presented a mathematical model for the evolution of cross-feeding on metabolic intermediates. In this model, a single-species bacterial population converts an initial molecule to an intermediate, before further converting it to a final end-product. The bacteria are constrained by a trade-off function between efficient metabolism of the initial molecule and the intermediate. Mutation in the system was restricted to changes in the maximum growth rates achieved on the initial and intermediate molecules. From these assumptions, the model exhibits branching divergence of a single population into two increasingly distinct strains. One strain metabolises the initial molecule efficiently, while being less efficient at metabolising the intermediate and thus releases increased amounts of the intermediate into the environment. The second strain more efficiently degrades the intermediate than the initial molecule, thus cross-feeds on that which is released by the first strain.
Pfeiffer and Bonhoeffer (2004) produced a similar abstract model, containing a more extended metabolic pathway for complete degradation of an initial molecule with several distinct steps, each with a catalysing enzyme. They assume that bacteria maximise ATP production—this production being possible only at specific steps of the metabolic pathway—whilst minimising concentrations of enzymes and intermediate compounds. In a homogeneous population that metabolises the initial molecule entirely, the model introduces mutation, whereby a mutant produces more of the earlier enzymes in the pathway than the original strain, and less of the later. As seen in the previous model, this results in the subsequent evolution of a cross-feeding strain, specialising on an intermediate molecule produced by the first mutant, with eventual extinction of the ancestor.
Both of these theoretical models achieve results reflective of those observed in the LTEE. A notable point on which the two models differ is the manner in which new strains are established: the first model involved gradual speciation from a single population over an extended time period, whereas the second considered the occurrence of phenotypically very distinct mutants, which quickly increase in number due to their growth advantage. The latter is more readily comparable with the long-term observations of the LTEE, in which the relative proportions of S and L strains vary widely across generations. The hypothesised cause of the generational fluctuation in population proportions is the invasion of new, fitter derivatives of each strain, which then rapidly alter the relative abundance of each strain at steady state (Rozen and Lenski, 2000; Lenski, 2017). In addition, neither model allowed for the accumulation of more than one intermediate molecule and the possible complex cross-feeding dynamics that might ensue. Is, therefore, a two-strain cross-feeding dynamic the most favourable on a single resource? If not, would it be possible to evolve a stable cross-feeding population of three or more strains utilising different intermediate molecules of the same metabolic pathway? The dynamics studied in these models could conceivably take place in already complex bacterial communities, such as the human gut, increasing the number of exchanged molecules and the diversity of the population.
A separate question posed by Pfeiffer and Bonhoeffer (2004), is whether a cross-feeding relationship is the result of mutation leading to the loss or alteration of a function, or simply due to phenotypic plasticity. An example of such plasticity is the switching between glucose and acetate metabolism as the former becomes depleted, resulting in the diauxic growth of E. coli described by Friesen et al. (2004). In an environment in which glucose concentrations is maintained at an abundant level, such a switching would not occur, but this does not imply that the bacteria have lost the ability to switch. It should always be asked, when studying any cross-feeding relationship, whether the bacteria involved have evolved complete dependence on cross-feeding or are merely behaving in the most energetically efficient manner for a particular environment. The answer to this question is likely case-dependent (Pande et al., 2014), and may qualitatively change as a population evolves, due to processes such as adaptive gene loss.
The Black Queen Hypothesis
A different, but complementary perspective for the evolution of cross-feeding dependencies is provided by the Black Queen Hypothesis (BQH; Morris et al., 2012). The hypothesis is that there are “conditions under which it is advantageous for an organism to stop performing a function,” also termed adaptive gene loss or reductive evolution, and relates to a permanent change in the phenotype. If a bacterium must expend energy to perform a function, but the end-product of this function is freely available in the environment, it would be beneficial to the bacterium to utilise the available product, and permanently desist in performing the function itself. A strongly related concept is streamlining theory, the idea that selection can favour the “minimisation of cell size and complexity” (Giovannoni et al., 2014; Mas et al., 2016). An example of such a function is the production of extracellular enzymes for the degradation of complex carbohydrates (Figure 3). Such enzymes perform a beneficial function for all bacteria in the immediate environment, since the resulting simpler sugars are available to all bacteria in the vicinity. Thus, under the BQH, it would be selectively advantageous for a bacterium in this environment to not synthesise the extracellular enzyme, whilst still maintaining the same feeding strategy on the released sugars. Any mutant unable to synthesise the extracellular enzyme could have an immediate growth advantage via such energy saving, and thus this trait would propagate in the population, provided that other members of the population continue to synthesise the enzyme. The BQH applies in any case where an individual performs a function that is to some degree “leaky” by nature, meaning that benefits of it are also available to other individuals.
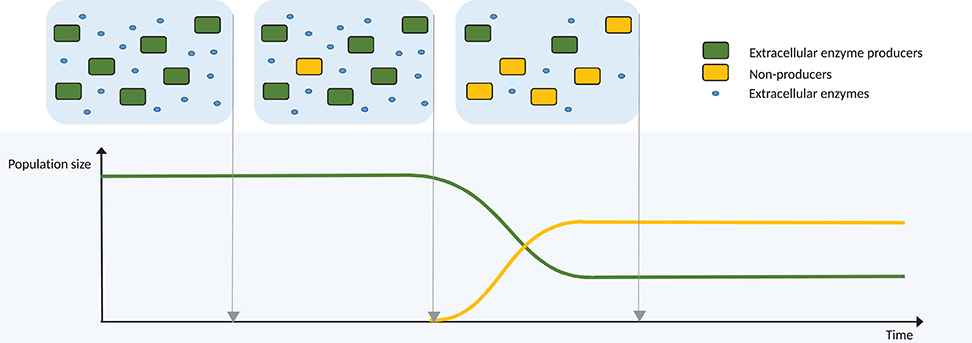
Figure 3. Diagrammatic example of evolution via the Black Queen Hypothesis, where the function lost is the production of an extracellular enzyme for the degradation of a complex molecule. Initially, all bacteria produce the extracellular enzyme, resulting in an abundance of available breakdown products. Mutation produces an individual unable to synthesise this enzyme, therefore giving it a growth advantage via energy saving, but making it entirely dependent on the ancestor. The mutant proportion of the population increases until, at some critical point, the population growth of the mutants is halted, since the reduced overall enzyme production by the population results in reduced availability of breakdown products. The enzyme producers are maintained via negative frequency dependent selection.
The potential for improved growth through reductive evolution is exemplified by studies on auxotrophic E. coli and Acinetobacter baylyi strains (D'Souza et al., 2014). Engineered auxotrophs for a specific essential nutrient were created via gene deletion. Many of these auxotrophs achieved greater growth than their prototrophic ancestor in competition experiments on growth medium containing the essential nutrient. Although the level of advantage varied between nutrients and was concentration-dependent, this shows that lack-of-function can be advantageous when the product of the function is readily available. In this study, lack-of-function was not due to loss-of-function via adaptive gene loss, but the single gene deletions necessary to achieve the observed growth advantage support the BQH. The authors did investigate the relative advantages of different gene deletions within the same biosynthetic pathway, but no investigation was carried out to ascertain whether further genome reduction would spontaneously occur once the pathway was made redundant by the artificial deletion. Moreover, in a large-scale analysis of metabolic networks and sequenced bacterial genomes, more than 60% of the gut-inhabiting bacteria tested were predicted to be auxotrophic for at least one of the amino acids, nucleosides and vitamins considered, with a median of two auxotrophies per strain (D'Souza et al., 2014). This analysis showed that the majority of the gut strains studied had lost almost all of the genes for the biosynthetic pathway corresponding to their auxotrophy. Together, these results suggest that it is possible that cross-feeding dependencies emerging from gene loss may be widespread in complex communities such as the gut, and that a single gene loss is likely followed by the loss of other genes within the same biosynthetic pathway. Longer-term studies with these E. coli auxotrophs would then be expected to show further adaptive gene loss, but this remains to be confirmed.
In much simpler populations than that of the human gut, cross-feeding via adaptive gene loss shows potential for stability. In this scenario, evolution under the BQH would not necessarily lead to the extinction of the original, prototrophic strain. If the newly evolved strain were entirely dependent upon the costly function performed by the ancestor, then extinction of the ancestor would be swiftly followed by extinction of the new strain (Mas et al., 2016). In the case of cross-feeding, if the cross-feeder is entirely dependent upon its partner for the provision of some essential molecule, then the newly-evolved cross-feeder cannot drive the ancestral strain to extinction, despite its energetic advantage (Figure 3). If repeated loss of function occurs in a population, an ancestral strain, which retains those functions and upon which all others depend, may become a “keystone” strain [see “Shooting the Moon” (Morris et al., 2012; Morris, 2015)]. Loss of the keystone strain is avoided by negative frequency-dependent selection, since the dependent strains experience reduced relative fitness as the keystone population decreases (Ze et al., 2013). Several studies have identified and discussed candidate keystone species in the gut microbiota, often Bacteroides strains with broad metabolic capabilities, but not always strains that are at high abundances in the gut (Bäckhed et al., 2005; Ze et al., 2012, 2013; Fisher and Mehta, 2014; Cockburn and Koropatkin, 2016).
An intriguing point is what population composition is reached at steady state, if after gene loss the cross-feeder is not entirely dependent on its ancestor? In this case, negative frequency-dependent selection may not be powerful enough to preserve the ancestor, potentially leading to the loss of the original function in the population. The consequences of such an eventuality are difficult to predict. It could be assumed that the cross-feeder will persist, but, without the benefit of the cross-feeding molecule, may show reduced growth. After this point, it is interesting to consider in which direction selection pressure will drive the remaining strain, especially in the presence of some third-party competitor. Mathematical modelling could be applied to study the conditions that might lead to such a scenario, and the population dynamics that would ensue.
The two routes to cross-feeding discussed thus far, divergence due to specialisation or by adaptive gene loss, are not mutually exclusive. Combinations of both routes are entirely plausible, but difficult to identify. Even with knowledge of a bacterial population's evolutionary history, the root cause of an evolutionary shift is elusive, as shown by the LTEE. These difficulties are compounded once we consider cross-feeding relationships in which resources are exchanged in a more complex manner.
Mutual Cross-Feeding
Although the term mutualism is broad and applicable across taxonomic kingdoms, we are concerned here with the subset relating to bacterial nutrition. Mutual cross-feeding has been observed in several microbial communities [for examples, see Harcombe (2010), Rivière et al. (2015) and Marchal et al. (2017)] and it is interesting to question how these two-way interactions may have arisen in initially self-sufficient populations. The answer to this lies in the cross-feeding relationships discussed previously.
For example, let us consider a pair of bacterial strains, one of which is a metabolite cross-feeder on some waste product of the other (molecule A). If a mutation occurred in a cross-feeding bacterium that caused it to excrete, at no detriment to itself, some metabolite (molecule B) required by the primary degrader, this mutation would be favoured. By supplementing the ambient availability of molecule B for the primary degrader, the mutation in the cross-feeder may benefit the growth of the primary degrader. This may result in subsequently increased availability of molecule A for the cross-feeder, in a positive feedback mechanism that would lead to increased growth of both. This description of evolution from a nutritional one-way benefit to mutualism is consistent with the hypothesised emergence of mutualism described by Connor (1995) and widely repeated in the literature (Sachs et al., 2004; Foster and Wenseleers, 2006; Harcombe, 2010; Wintermute and Silver, 2010a; Estrela and Brown, 2013). Although not specific to nutritional mutualisms, or to bacteria, the framework of Connor (1995) is a potential route by which mutual cross-feeding may evolve.
An example of mutual cross-feeding is again provided by experimental culture using engineered auxotrophic E. coli (Harcombe, 2010). In this instance, an E. coli strain with engineered methionine auxotrophy is partnered with a Salmonella typhimurium strain, which displays cross-feeding on products of lactose metabolism released by the wild type E. coli. Initially the auxotrophic E. coli and the cross-feeder were unable to grow in co-culture on lactose, since neither was supplying its partner with the essential molecule. However, upon treatment with ethionine, a methionine antagonist, and selection of Salmonella strains displaying ethionine resistance, a novel strain evolved which excreted methionine and could enable growth of both strains in co-culture. Although this evolution involved a degree of interference from the experimenters, this did not extend to genome editing of the Salmonella strain, and the ultimate emergence of methionine excretion was via mutation. Methionine secretion was even favoured in the presence of wild-type Salmonella, which were unable to become established in a population of methionine-secreting Salmonella and auxotrophic E. coli, perhaps due to the effects of spatial structure discussed later in this review. Following the expectations of the BQH, we would expect that the wild type E. coli would not become established in culture with the mutual cross-feeding partners, since the energetic cost of producing methionine would put it at a disadvantage compared to the auxotrophic strain. As would be expected, in the absence of either E. coli, the methionine excreting Salmonella strain lost its advantage over the ancestral strain, since this advantage was entirely dependent on the mutualism. The relationship observed here shows the gain of a function to support cross-feeding, but mutual cross-feeding may also emerge from loss-of-function.
An alternative perspective to the framework of Connor (1995) is provided by the adaptive gene loss of the BQH. Consider a cross-feeder that is dependent, due to adaptive gene loss, upon a molecule produced by its prototrophic ancestor. Morris (2015) proposed a scenario in which this ancestor also undergoes adaptive gene loss and becomes dependent on the cross-feeder for provision of some other nutrient. Both strains would then be dependent on one another for a specific product, thus forming an obligate mutual cross-feeding partnership. This specialisation is supported by the biological markets model of Schwartz and Hoeksema (1998). Nations profit more by specialising in the production and export of certain products, whilst importing others, than by producing all they need internally. The model applied this principle to biology and found further support for the benefits of mutualistic interactions.
We have thus far focussed upon the evolution of one-way and mutual cross-feeding in an initially identical population or a population that has been artificially constructed to perform cross-feeding. It is also entirely possible that two strains of bacteria will engage incidentally in cross-feeding when grown together. When feeding preferences and waste production coincide, bacteria may be predisposed to such behaviour (Sachs et al., 2004). Investigations using genome-scale metabolic models found that simulations under anoxic conditions, such as those in the human gut, are more conducive to mutual cross-feeding and the secretion of central carbon intermediates (Pacheco et al., 2019). Although the evolutionary background was not included here, only molecules that are secreted at no detriment to growth were considered, ensuring that only passive relationships were studied. This form of cross-feeding does not necessarily require any evolutionary process to emerge, merely coexistence. However, further evolution via routes such as adaptive gene loss and specialisation is still possible in these more general cases, as they are based upon passive production of metabolites. Passive interactions, which require no more energetic investment from either party than would be seen in the absence of interaction, do not account for all forms of cross-feeding. We next discuss the instances in which bacteria actively invest in some action at an individual cost, to the benefit of others.
Augmented Cross-Feeding
Within the established group of mutual cross-feeding interactions, there are those that are beyond the boundaries of direct individual benefits. From an evolutionary perspective, investment in an unrelated individual should be precluded by natural selection (Darwin, 1859; Sachs et al., 2004; Penders et al., 2006). However, augmented cross-feeding is sustainable because ultimately, though not directly, the investor does receive benefit.
As discussed previously, an existing metabolite or substrate cross-feeding relationship may lead to the evolution of mutual cross-feeding (Connor, 1995; Sachs et al., 2004). In most cases, it seems reasonable to assume that augmented cross-feeding, a subset of mutual cross-feeding, will emerge when one of a mutualist pair begins to energetically increase its contribution to its partner (Pande and Kost, 2017). The example of mutual cross-feeding given in the previous section, between an E. coli auxotroph and a Salmonella strain, fits the criteria for augmented cross-feeding since methionine excretion was shown to be costly (Harcombe, 2010). More recent follow-up experimentation found a variety of levels of methionine excretion on the part of different Salmonella strains (Douglas et al., 2016, 2017). Higher production of the essential amino acid resulted in slower growth of Salmonella, but faster growth of E. coli auxotrophs, as would be expected. Although higher methionine production resulted in greater consortia growth, the Salmonella strains reached lower proportions in the population due to the high cost involved in this production.
The concept of cost and benefit is perhaps most important in the evolution of augmented cross-feeding. The cost-benefit ratio is widely used terminology in the literature and is vital to the interaction (Trivers, 1971; Yamamura et al., 2004; Foster and Wenseleers, 2006; Harcombe, 2010; Nadell et al., 2010; Wintermute and Silver, 2010b; Damore and Gore, 2012; Oliveira et al., 2014; Germerodt et al., 2016). Clearly, the benefits of investing must outweigh the costs if an investing mutant is to invade a non-investing population.
The cost-benefit ratio was studied by Wintermute and Silver (2010b) in their auxotrophic E. coli experiments. Paired co-cultures of 46 E. coli strains with differing auxotrophies showed that several of these combinations could grow successfully in media that did not support monoculture growth, as a result of mutual cross-feeding. It was found that it was most frequently molecules of low cost to produce that were involved in successful mutual cross-feeding combinations, as would be expected.
Pande et al. (2014) took a similar line of study and genetically altered auxotrophic E. coli strains to overproduce the amino acid required by their co-culture partner. Many of these combinations were shown to have a growth advantage over prototrophic monocultures, achieving higher growth rates than their ancestors. The cost of overproducing one amino acid was less than the benefit of not needing to synthesise both. While both of these studies involved genetically engineered bacteria in order that the cross-feeding mechanism could be fully controlled, they provide evidence that augmented cross-feeding on specific molecules can be a successful growth strategy.
Examples of augmented cross-feeding relationships in the less controlled and more diverse environment of the human gut are rare. Rakoff-Nahoum et al. (2016) showed the costly production of extracellular enzymes by the gut bacterium Bacteroides ovatus that were not required for its own growth, but which were beneficial to other gut bacteria. It was shown that B. ovatus received some growth advantage in return when performing this action in co-cultures, however the mechanism by which B. ovatus benefits from this relationship has not yet been established. The authors suggest cross-feeding of some other molecule and detoxification as possible mechanisms of the mutualism. It seems likely that cross-feeding occurs in both directions, which would make this an example of augmented cross-feeding by gut strains, but this remains to be proven. Furthermore, understanding the evolution of such a relationship will also require more complete knowledge of its current nature.
The experiments with synthetic consortia discussed here show that strains native to the gut are capable of such interactions, but only under strict culture conditions. Furthering the difficulty of evolving augmented cross-feeding, mathematical modelling has found that this phenotype is only favoured at intermediate population densities, due to a lack of sufficient reciprocation in sparse populations and competition for space in dense populations (Bull and Harcombe, 2009). The tight constraints on augmented cross-feeding might lead one to believe that it must be vanishingly rare. However, there are several factors that increase selection for positive interaction, foremost being the physical structure of the population.
The Influence of Spatial Structure on Cross-Feeding Evolution
In discussing mixed microbial systems, Wintermute and Silver state that: “In well-mixed systems, shared metabolites are easily lost” (Wintermute and Silver, 2010a). This is clearly true for unstructured populations, but bacteria living in such a planktonic manner are only a subset of bacterial populations, especially in the gut (Macfarlane et al., 2011). Biofilm populations abound, so spatial analysis must be applied to the study of cross-feeding and its evolution (Tolker-Nielsen and Molin, 2000; Nadell et al., 2010; Wintermute and Silver, 2010a; Macfarlane et al., 2011; Van Wey et al., 2011).
Before adding the context of cross-feeding evolution, we must qualify the terminology of structured and unstructured environments. A well-mixed culture implies little or no aggregation of cells and equal availability of nutrients to all individuals. In a biofilm however, we must allow for dynamics that are more complex. Diffusion in a biofilm is hampered by the density of cells and extra-cellular polymeric substances, as well as the three-dimensional biofilm structure (Wanner et al., 2006; Van Wey et al., 2011, 2012). Nutrient transport is slower in a biofilm than an unstructured medium, resulting from hindered diffusion, thereby decreasing the rate of resource exchange between nearby biofilm-dwelling cross-feeders.
An observed result of this reduced mixing is increased genetic diversity. Rainey and Travisano (1998) compared the genetic variation from static or shaken monocultures of Pseudomonas fluorescens, a strain known for its rapid evolutionary capabilities. In static cultures, the P. fluorescens population diverged to multiple genetically different strains, many of which were able to coexist over several days of culture. The novel strains were direct competitors, exploiting a niche only available due to the spatial separation between them. In contrast, the mixed culture saw no genetic differentiation over the same time period.
More recent experimentation compared the success of a cross-feeding pair when grown in structured or unstructured media (Hansen et al., 2007). An Acinetobacter strain converted benzyl alcohol to benzoate, which could then be utilised by Pseudomonas putida. When grown as a biofilm, the relationship evolved from mere proximity of populations, to P. putida forming a covering layer over aggregates of Acinetobacter. P. putida achieved greater population growth in the resultant co-culture arrangement, and such adaption was not observed in the mixed chemostat environment, demonstrating that the biofilm structure was necessary for the increased productivity.
It is especially fruitful when the study of evolutionary processes is carried out in an environment where spatial structure can be varied. Long-term culture of Burkholderia cenocepacia, a pathogenic bacterium known for biofilm formation, gave rise to three morphologically and genetically different strains (Poltak and Cooper, 2011; Traverse et al., 2013). This work was carried out under a regime of daily recolonisation in a new biofilm environment, and planktonic control experiments showed markedly less diversification. Subsequent growth assays for each strain on cell-free supernatant of the others was suggestive of cross-feeding dynamics, though the most pronounced differences between the novel strains was in their biofilm formation. Imaging of these biofilm structures showed growth similar to that observed by Hansen et al. (2007), in that the different strains grew in close proximity and achieved better growth as a result. Both these observations support the authors' assertion that cross-feeding was present and beneficial to the biofilm strains.
The fact that cells remain in close proximity to one another in a biofilm is promotive of the evolution of positive interactions. Any newly arising mutant that gains a selective advantage from molecules produced by the surrounding population will only succeed if it remains in the environment that gives it that advantage. Structure, and the ability to interact continuously with a consistent group of neighbours is more conducive to cross-feeding evolution than a well-mixed environment (Trivers, 1971; Wintermute and Silver, 2010a), and it is important in mutual and augmented cross-feeding that the bacteria are able to remain in proximity to their partners (Germerodt et al., 2016). One difficulty in the evolution of augmented cross-feeding is the fact that any mutant that evolves overproduction of the focal molecule at an energetic cost should be selected against, as it is at a disadvantage compared to its ancestors (Marchal et al., 2017). In a well-mixed population, any increased mutual exchange promoted by overproduction will also benefit the non-investing ancestor. In contrast, a spatially structured environment allows the benefits of overproduction to be more readily available to the overproducing bacterium, and thus is more likely to encourage the evolution of augmented cross-feeding (Bull and Rice, 1991; Doebeli and Knowlton, 1998; Foster and Wenseleers, 2006; Kim et al., 2008).
A mathematical formulation for the impact of spatial structure can be derived from Hamilton's rule (Hamilton, 1964). It states that sustained interaction of a costly nature requires that
where c is the cost of a beneficial act, b is the benefit of the act to the receiver, and r is the relatedness of the individuals involved. Although simplistic and originally posed for genetic relatedness, Hamilton's rule is flexible and intuitive.
Nadell et al. (2010) adapted the relatedness term in their study of evolution with spatial structure. They considered a mathematical model population divided into individuals that perform the costly production of a public good, and those that do not, grown in a spatially structured environment. For the purpose of this model, Hamilton's relatedness term can simply be seen as the proportion of the benefits of producing the public good that are shared with other producers, as opposed to non-producers (Nadell et al., 2010; Damore and Gore, 2012). Thus, in terms of Hamilton's rule, the proportion of the benefit of producing the public good accrued to other producers must be greater than the cost of producing. For a wide range of parameter values favouring public good production, the model displayed the producers forming segregated colonies, excluding the non-producers.
This segregation in a structured environment has been shown experimentally between unrelated bacterial species (Pande et al., 2016). E. coli and A. baylyi were each engineered for obligate augmented cross-feeding, the focal molecules being two essential amino acids. In tri-cultures including a strain without the overproduction characteristic, segregation of the population was observed, with the overproducing pair excluding those cells that did not overproduce. Further experimentation and accompanying mathematical modelling found that the overproducing cross-feeders were well-mixed. Meanwhile, small patches of the non-producers were excluded to the edge of the much larger and more successful overproducing colony. Similar results have been published based on experimentation and modelling of yeast populations (Momeni et al., 2013), and in both cases, repeat experimentation under mixed conditions resulted in domination by the non-overproducing strain. The similarity between model simulations and experimental observations in the work of both groups is clear, and encourages the use of further modelling of spatial self-organisation in naturally occurring communities such as gut biofilms.
However, it would be incorrect to generalise completely and state that structured groups of cross-feeders always attain greater growth than heterogeneously mixed groups. Interactions between differing bacterial species are complex, as exemplified by Kim et al. (2008). They found that when an engineered three-member obligate mutualist group was grown in mixed culture, one bacterial strain dominated at the expense of the others. However, all three strains showed increased growth when kept separated using a microfluidic device, with resources—but not cells—able to pass between colonies. This increased growth was only seen at intermediate separation distances (600–1,200 μm); increasing distance between strain colonies resulted in a decline in the growth of all three populations. While this specific interaction of these three strains is not observed in nature, the results are sufficient to urge researchers to co-culture bacteria in various spatially structured media, before declaring that the combinations are unculturable.
A similar result was recently found by Shitut et al. (2019), who observed that exchange of amino acids between overproducing and auxotrophic E. coli strains was prevented when the two were separated by a membrane. Despite the fact that amino acids could diffuse through this membrane, growth of the auxotrophic strains under separation was negligible. Nanotubes [thin, membrane-based structures linking individual cells (Pande et al., 2015; Shitut et al., 2019)] were found to be facilitating the exchange of amino acids in mixed co-culture, but could not be formed through the separating membrane. Contrary to the results of Kim et al. (2008), separation was preventative of co-culture growth here, highlighting the differing outcomes of spatial structure in specific cross-feeding combinations.
Although several of the experiments and models discussed in this section are not specifically focussed on cross-feeding, the conclusions generalise to a range of bacterial interactions. We have presented routes by which cross-feeding interactions may emerge and discussed conditions that may favour their evolution. However, persistence of these relationships in a population is also important. Once cross-feeding has evolved, it must be resilient to fluctuations in levels of nutrients, competitors and inconsistent cross-feeding partners to remain sustainable.
Stability and Persistence of Cross-Feeding Relationships
Not all evidence credits cross-feeding with persistence and enhanced growth over many generations. There is the argument that adaptive gene loss and interdependence between species may lead to reduced overall growth. Oliveira et al. (2014) found in their mathematical model that a cross-feeding community is less productive than a corresponding prototrophic population, and state that a given strain which relies upon another strain for the provision of essential nutrients is vulnerable to extinction if the other strain is lost. Network modelling of bacterial community stability has been used to derive a similar conclusion: that the entire community is less stable as mutual cross-feeding increases (Coyte et al., 2015). A precarious population structure is formed, in which the loss of a single strain may cause the collapse of much or all of the population, particularly if the strain lost is a keystone strain.
Alongside the difficulties of inter-species dependency, there is also the problem of inconsistent nutrient supply. This is a factor of particular importance in the gut, where host diet plays a crucial role in determining the availability of resources for bacteria. Cross-feeding bacteria are dependent on the actions of primary degraders, who themselves require sufficient energy sources for growth if the population is to survive. Gudelj et al. (2016) presented a mathematical model simulating two E. coli strains that have the capacity for cross-feeding but are also both able to feed on the primary resource. This dynamic is comparable to that of strains L and S in the LTEE literature, however the simulation was carried out for continuous culture conditions, parameterised using data from Rosenzweig et al. (1994). The model showed that the strains maintained a stable cross-feeding relationship when the concentration of the initial molecule was high. Low or intermediate concentrations resulted in the eventual extinction of one strain, for all but a very narrow range of population densities. Which strain was lost was determined by the initial conditions. Regardless of which strain is lost, a large event such as a drop in resource availability would seriously challenge a cross-feeding relationship. Significant dietary changes have been shown in numerous cases to result in rapid changes in the gut population (for example, Walker et al., 2011; Korpela et al., 2014; Salonen et al., 2014). Though this is not necessarily due to the availability of resources for bacterial metabolism, as other factors such as gut pH are partially determined by diet (Flint et al., 2014), cross-feeding relationships in the gut must also be affected by the resultant changes in resource and strain concentrations.
By contrast, mutual cross-feeding may be favoured by low environmental resource availabilities, by selecting for greater resource exchange in the face of adverse conditions. Experimentation using two mutual cross-feeding yeast strains has observed that continued cross-feeding is determined by the level of dependency on the cross-fed molecules (Müller et al., 2014). It was found that the cross-feeders, which were homogenously mixed initially, showed increased separation as the plate culture expanded radially. An even mixing of the two strains was only observed when the environmental availability of the cross-fed molecules was low, increasing inter-dependency.
Regarding augmented cross-feeding relationships, data from a pair of E. coli strains, engaged in obligate augmented cross-feeding for essential amino acids, was used to fit a computational model (Germerodt et al., 2016). Limiting the addition of amino acids to the population was predicted by the model to select for augmented cross-feeding, as this encouraged auxotrophs to support their partners. When the focal amino acids were abundantly available, augmented cross-feeding was no longer advantageous due to the cost of overproduction and cells that did not overproduce were at an advantage. At this point, we introduce the term “cheats,” to describe such individuals. Formally, a cheat is a member of a population that benefits from a function, but does not pay the full cost of performing this function, thus decreasing the fitness of those individuals that do (Hamilton, 1964; Trivers, 1971; Axelrod and Hamilton, 1981; Ferriere et al., 2002; West et al., 2007a; Ghoul et al., 2014). Naturally, in some cases individuals may be investing to various degrees, at which point deciding what level constitutes cheating becomes difficult. Examples from the literature most frequently deal with the binary case in which cheats invest nothing into the beneficial function.
Intuitively, an individual that reaps all the benefits of augmented cross-feeding, at no individual cost, will have a fitness advantage over those paying the cost, so could be expected to thrive. Much research exists aiming to explain the persistence of mutually beneficial interactions in the presence of cheats. Most commonly, these studies assess what conditions are necessary for a cheat to invade a reciprocating population. Pande et al. (2014) introduced cheats into their co-cultures of reciprocating E. coli strains and found that, although the cheats exhibited negative frequency-dependent growth and increased their population size, augmented cross-feeding persisted. All three strains could stably coexist in these experiments. This result has also been obtained in mathematical models that emphasise the importance of the cost-benefit ratio (Yamamura et al., 2004; Estrela and Gudelj, 2010; Sun et al., 2019). The stochastic model of Yamamura et al. (2004) showed that a cheat could invade an augmented cross-feeding population only when the cost of overproduction was high relative to its benefits—the opposite of the ideal conditions for the evolution of augmented cross-feeding. Sun et al. (2019) recently published a mathematical investigation of cheat invasion and persistence, in a framework that can be easily adapted to multiple species and multiple exchanged resources. This model structure could be parameterised from experimental data to facilitate the study of cheat dynamics in more complex populations, such as the gut.
Revisiting Germerodt et al. (2016), the observed clustering of the augmented cross-feeders in the model proved resistant to cheats, as the benefits of cross-feeding sought by the cheats were not as readily available outside these clusters. Diffusion of the essential amino acids produced during augmented cross-feeding was limited, thus encouraging cluster formation by the producers. It is conceivable that this spatial separation may also be promoting stronger mutual cross-feeding in individuals in the clusters. There is also evidence that quorum sensing among bacteria may decrease the expression of extracellular enzymes in the presence of cheats, thereby achieving maximum productivity only when cheats are absent (Allen et al., 2016). The selection pressure caused by the presence of a cheat may also drive higher levels of cross-feeding under certain conditions (Ferriere et al., 2002; MacLean et al., 2010). In yeast experimentation and accompanying mathematical modelling, the presence of cheats that did not produce the extracellular carbohydrate-degrading enzyme invertase improved population fitness, subject to strict conditions on: the imbalance between invertase production and carbohydrate availability; the trade-off between carbohydrate availability and efficiency of use, as discussed for the cross-feeding observed in the LTEE; and the now common necessity for imperfect mixing due to spatial structure (MacLean et al., 2010). The intriguing concept that cheats may positively affect cross-feeding relationships deserves further physical and theoretical study.
We see that the factors that promote the evolution of augmented cross-feeding - low cost-benefit ratio and spatial structure—also contribute to its persistence in adversity. However, the studies of stability reviewed here consider only a small number of strains with distinct phenotypes, whereas in the gut there are a multitude of different species, and wide genetic variation within species. Although the influencing factors discussed here will also likely be decisive in larger cross-feeding systems, more complex experimentation and mathematical modelling will no doubt identify further characteristics that are key to cross-feeding persistence in more complex networks. For example, we would expect the host to have a role in determining selection pressure on members of the gut microbiota. How the host immune system has coevolved with the microbiota remains unclear, but it is important to accept that members of the microbiota are not necessarily evolving in a constant environment, but rather one that also responds and adapts (Maynard et al., 2012).
Conclusion and Future Directions
Whether and in what manner other forms of nutrient exchange fit within our definitions of cross-feeding pose individual and intriguing questions. This leads to the question of how revealing the classification of cross-feeding types may be. We believe that our framework primarily provides for quick comparison of cross-feeding relationships observed in separate experiments.
Using our terminology framework as a base, we have discussed possible evolutionary origins for the four forms of bacterial cross-feeding that we defined initially and listed factors influencing their emergence and subsequent persistence. It is notable that we mainly considered consortia of only a few different strains engaged in few interactions in this review, as this is what is available in the literature. In the environment of the human gut, the great variety of the microbiota implies that far more complex networks are likely active (Pande and Kost, 2017). With ongoing evolution and changing environmental conditions, these networks are an enormous yet enticing challenge to the researcher. One area which stands out as requiring further research is the manner in which cross-feeding may continue to evolve once established, particularly in complex cross-feeding networks (Hillesland, 2018). The abstract techniques reviewed here to examine evolutionary dynamics often provide further insight when in conjunction with experimentation, and we foresee ever more widespread use of mathematical modelling in evolutionary biology to determine mechanisms of action as the level of complexity in experimental studies increases. Translating the results obtained from synthetic experiments and mathematical models to in situ bacterial metabolism will be a challenge, but rewarded by a greater understanding of important communities such as the gut microbiota.
It is also important to consider what role cross-feeding plays in the wider field of bacterial interactions. Recent genome-scale metabolic modelling of 800 bacterial communities, drawn from a variety of natural environments including the gut, analysed the metabolic relationships within each (Zelezniak et al., 2015). Competitive interactions dominated these relationships, but significant signs of metabolic dependencies and cross-feeding were also present. Evidence also exists for widespread auxotrophy across many bacterial environments (D'Souza and Kost, 2016). The implication of cross-feeding is clear, but competition should be accepted as the dominant form of interaction between bacteria in any environment (Foster and Bell, 2012; Pacheco et al., 2019). Moreover, all examples of augmented cross-feeding discussed in this review were artificially induced, not involving naturally occurring interactions. There is doubt as to the existence of naturally occurring bacterial relationships that satisfy our definition for augmented cross-feeding, and any empirical evidence for it should be rigorously examined with the aid of mathematical modelling to ascertain the true mechanism behind observed data.
Author Contributions
NS drafted the manuscript. All authors contributed to manuscript revision, read and approved the submitted version.
Conflict of Interest Statement
The authors declare that the research was conducted in the absence of any commercial or financial relationships that could be construed as a potential conflict of interest.
Acknowledgments
NS was supported by a Ph.D. fellowship from the Riddet Institute, through funding provided by the New Zealand Tertiary Education Commission. Thanks must also go to AgResearch for the resources provided to NS. The authors thank A.S. Van Wey for her contributions to cross-feeding discussions, and E. Rettedal and R. Ronimus at AgResearch for their insightful comments on the manuscript.
References
Allen, R. C., McNally, L., Popat, R., and Brown, S. P. (2016). Quorum sensing protects bacterial co-operation from exploitation by cheats. ISME J. 10, 1706–1716. doi: 10.1038/ismej.2015.232
Amaretti, A., Bernardi, T., Tamburini, E., Zanoni, S., Lomma, M., Matteuzzi, D., et al. (2007). Kinetics and metabolism of Bifidobacterium adolescentis MB 239 growing on glucose, galactose, lactose, and galactooligosaccharides. Appl. Environ. Microbiol. 73, 3637–3644. doi: 10.1128/aem.02914-06
Bäckhed, F., Ley, R. E., Sonnenburg, J. L., Peterson, D. A., and Gordon, J. I. (2005). Host-bacterial mutualism in the human intestine. Science 307, 1915–1920. doi: 10.1126/science.1104816
Belenguer, A., Duncan, S. H., Calder, A. G., Holtrop, G., Louis, P., Lobley, G. E., et al. (2006). Two routes of metabolic cross-feeding between Bifidobacterium adolescentis and butyrate-producing anaerobes from the human gut. Appl. Environ. Microbiol. 72, 3593–3599. doi: 10.1128/aem.72.5.3593-3599.2006
Belenguer, A., Duncan, S. H., Holtrop, G., Flint, H. J., and Lobley, G. E. (2008). Quantitative analysis of Microbial metabolism in the human large intestine. Curr. Nutr. Food Sci. 4, 109–126. doi: 10.2174/157340108784245957
Bull, J. J., and Harcombe, W. R. (2009). Population dynamics constrain the cooperative evolution of cross-feeding. PLoS ONE 4:e4115. doi: 10.1371/journal.pone.0004115
Bull, J. J., and Rice, W. R. (1991). Distinguishing mechanisms for the evolution of co-operation. J. Theor. Biol. 149, 63–74. doi: 10.1016/S0022-5193(05)80072-4
Cockburn, D. W., and Koropatkin, N. M. (2016). Polysaccharide degradation by the intestinal microbiota and its influence on human health and disease. J. Mol. Biol. 428, 3230–3252. doi: 10.1016/j.jmb.2016.06.021
Connor, R. C. (1995). The benefits of mutualism: a conceptual framework. Biol. Rev. 70, 427–457. doi: 10.1111/j.1469-185X.1995.tb01196.x
Coyte, K. Z., Schluter, J., and Foster, K. R. (2015). The ecology of the microbiome: networks, competition, and stability. Science 350, 663–6. doi: 10.1126/science.aad2602
Damore, J. A., and Gore, J. (2012). Understanding microbial cooperation. J. Theor. Biol. 299, 31–41. doi: 10.1016/j.jtbi.2011.03.008
De Mazancourt, C., Loreau, M., and Dieckmann, U. L. F. (2005). Understanding mutualism when there is adaptation to the partner. J. Ecol. 93, 305–314. doi: 10.1111/j.0022-0477.2004.00952.x
Doebeli, M. (2002). A model for the evolutionary dynamics of cross-feeding polymorphisms in microorganisms. Popul. Ecol. 44, 59–70. doi: 10.1007/s101440200008
Doebeli, M., and Knowlton, N. (1998). The evolution of interspecific mutualisms. Proc. Natl. Acad. Sci. U.S.A. 95, 8676–8680.
Douglas, S. M., Chubiz, L. M., Harcombe, W. R., and Marx, C. J. (2017). Identification of the potentiating mutations and synergistic epistasis that enabled the evolution of inter-species cooperation. PLoS ONE 12:e0174345. doi: 10.1371/journal.pone.0174345
Douglas, S. M., Chubiz, L. M., Harcombe, W. R., Ytreberg, F. M., and Marx, C. J. (2016). Parallel mutations result in a wide range of cooperation and community consequences in a two-species bacterial consortium. PLoS ONE 11:e0161837. doi: 10.1371/journal.pone.0161837
D'Souza, G., and Kost, C. (2016). Experimental evolution of metabolic dependency in bacteria. PLoS Genet. 12:e1006364. doi: 10.1371/journal.pgen.1006364
D'Souza, G., Waschina, S., Pande, S., Bohl, K., Kaleta, C., and Kost, C. (2014). Less is more: selective advantages can explain the prevalent loss of biosynthetic genes in bacteria. Evolution 68, 2559–2570. doi: 10.1111/evo.12468
Duncan, S. H., Barcenilla, A., Stewart, C. S., Pryde, S. E., and Flint, H. J. (2002). Acetate utilization and butyryl coenzyme A (CoA): Acetate-CoA transferase in butyrate-producing bacteria from the human large intestine. Appl. Environ. Microbiol. 68, 5186–5190. doi: 10.1128/aem.68.10.5186-5190.2002
Egan, M., O'Connell Motherway, M., Kilcoyne, M., Kane, M., Joshi, L., Ventura, M., et al. (2014). Cross-feeding by Bifidobacterium breve UCC2003 during co-cultivation with Bifidobacterium bifidum PRL2010 in a mucin-based medium. BMC Microbiol. 14:0282. doi: 10.1186/s12866-014-0282-7
Elena, S. F., and Lenski, R. E. (2003). Evolution experiments with microorganisms: the dynamics and genetic bases of adaptation. Nat. Rev. Gen. 4, 457–469. doi: 10.1038/nrg1088
Enjalbert, B., Millard, P., Dinclaux, M., Portais, J.-C., and Létisse, F. (2017). Acetate fluxes in Escherichia coli are determined by the thermodynamic control of the Pta-AckA pathway. Sci. Rep. 7:42135. doi: 10.1038/srep42135
Estrela, S., and Brown, S. P. (2013). Metabolic and demographic feedbacks shape the emergent spatial structure and function of microbial communities. PLoS Comp. Biol. 9:e1003398. doi: 10.1371/journal.pcbi.1003398
Estrela, S., and Gudelj, I. (2010). Evolution of cooperative cross-feeding could be less challenging than originally thought. PLoS ONE 5:e0014121. doi: 10.1371/journal.pone.0014121
Falony, G., Calmeyn, T., Leroy, F., and De Vuyst, L. (2009). Coculture fermentations of bifidobacterium species and bacteroides thetaiotaomicron reveal a mechanistic insight into the prebiotic effect of inulin-type fructans. Appl. Environ. Microbiol. 75, 2312–2319. doi: 10.1128/aem.02649-08
Ferriere, R., Bronstein, J. L., Rinaldi, S., Law, R., and Gauduchon, M. (2002). Cheating and the evolutionary stability of mutualisms. Proc. Biol. Sci. 269, 773–80. doi: 10.1098/rspb.2001.1900
Fisher, C. K., and Mehta, P. (2014). Identifying keystone species in the human gut microbiome from metagenomic timeseries using sparse linear regression. PLoS ONE 9:e102451. doi: 10.1371/journal.pone.0102451
Flint, H. J., Duncan, S. H., Scott, K. P., and Louis, P. (2014). Links between diet, gut microbiota composition and gut metabolism. Proc. Nutr. Soc. 74, 13–22. doi: 10.1017/s0029665114001463
Flint, H. J., and Juge, N. (2015). Role of microbes in carbohydrate digestion. Food Sci. Technol. 29, 24–26.
Foster, K. R., and Bell, T. (2012). Competition, not cooperation, dominates interactions among culturable microbial species. Curr. Biol. 22, 1845–1850. doi: 10.1016/j.cub.2012.08.005
Foster, K. R., and Wenseleers, T. (2006). A general model for the evolution of mutualisms. J. Evol. Biol. 19, 1283–1293. doi: 10.1111/j.1420-9101.2005.01073.x
Friesen, M. L., Saxer, G., Travisano, M., and Doebeli, M. (2004). Experimental evidence for sympatric ecological diversification due to frequency-dependent competition in Escherichia coli. Evolution 58, 245–260. doi: 10.1111/j.0014-3820.2004.tb01642.x
Germerodt, S., Bohl, K., Lück, A., Pande, S., Schröter, A., Kaleta, C., et al. (2016). Pervasive selection for cooperative cross-feeding in bacterial communities. PLoS Comp. Biol. 12:e1004986. doi: 10.1371/journal.pcbi.1004986
Ghoul, M., Griffin, A. S., and West, S. A. (2014). Toward an evolutionary definition of cheating. Evolution 68, 318–331. doi: 10.1111/evo.12266
Giovannoni, S. J., Thrash, C. J., and Temperton, B. (2014). Implications of streamlining theory for microbial ecology. ISME J. 8, 1553–1565. doi: 10.1038/ismej.2014.60
Goo, E., An, J. H., Kang, Y., and Hwang, I. (2015). Control of bacterial metabolism by quorum sensing. Trends Microbiol. 23, 567–576. doi: 10.1016/j.tim.2015.05.007
Großkopf, T., Consuegra, J., Gaffé, J., Willison, J. C., Lenski, R. E., Soyer, O. S., et al. (2016). Metabolic modelling in a dynamic evolutionary framework predicts adaptive diversification of bacteria in a long-term evolution experiment. BMC Evol. Biol. 16:163. doi: 10.1186/s12862-016-0733-x
Gudelj, I., Kinnersley, M., Rashkov, P., Schmidt, K., and Rosenzweig, F. (2016). Stability of cross-feeding polymorphisms in microbial communities. PLoS Comp. Biol. 12:e1005269. doi: 10.1371/journal.pcbi.1005269
Hamilton, W. D. (1964). The genetical evolution of social behaviour. I and II. J. Theor. Biol. 7, 1–52.
Hansen, S. K., Rainey, P. B., Haagensen, J. A. J., and Molin, S. (2007). Evolution of species interactions in a biofilm community. Nature 445, 533–536. doi: 10.1038/nature05514
Harcombe, W. (2010). Novel cooperation experimentally evolved between species. Evolution 64, 2166–2172. doi: 10.1111/j.1558-5646.2010.00959.x
Helling, R. B., Vargas, C. N., and Adams, J. (1987). Evolution of Escherichia coli during growth in a constant environment. Genetics 116, 349–358.
Hillesland, K. L. (2018). Evolution on the bright side of life: microorganisms and the evolution of mutualism. Ann. N. Y. Acad. Sci. 1422, 88–103. doi: 10.1111/nyas.13515
Hillesland, K. L., and Stahl, D. A. (2010). Rapid evolution of stability and productivity at the origin of a microbial mutualism. Proc. Natl. Acad. Sci. U.S.A. 107, 2124–2129. doi: 10.1073/pnas.0908456107
Hosoda, K., Suzuki, S., Yamauchi, Y., Shiroguchi, Y., Kashiwagi, A., Ono, N., et al. (2011). Cooperative adaptation to establishment of a synthetic bacterial mutualism. PLoS ONE 6:e17105. doi: 10.1371/journal.pone.0017105
Kim, H. J., Boedicker, J. Q., Choi, J. W., and Ismagilov, R. F. (2008). Defined spatial structure stabilizes a synthetic multispecies bacterial community. Proc. Natl. Acad. Sci. U.S.A. 105, 18188–18193. doi: 10.1073/pnas.0807935105
Korpela, K., Flint, H. J., Johnstone, A. M., Lappi, J., Poutanen, K., Dewulf, E., et al. (2014). Gut microbiota signatures predict host and microbiota responses to dietary interventions in obese individuals. PLoS ONE 9:e90702. doi: 10.1371/journal.pone.0090702
Kumari, S., Beatty, C. M., Browning, D. F., Busby, S. J. W., Simel, E. J., and Hovel-Miner, G. (2000). Regulation of acetyl coenzyme A synthetase in Escherichia coli. J. Bacteriol. 182, 4173–4179. doi: 10.1128/JB.182.15.4173-4179.2000
Lenski, R. E. (2017). Experimental evolution and the dynamics of adaptation and genome evolution in microbial populations. ISME J. 11, 2181–2194. doi: 10.1038/ismej.2017.69
Lenski, R. E., Rose, M. R., Simpson, S. C., and Tadler, S. C. (1991). Long-term experimental evolution in Escherichia coli. I. Adaptation and divergence during 2000 generations. Am. Nat. 138, 1315–1341. doi: 10.1086/285289
Macfarlane, G. T., and Macfarlane, S. (2012). Bacteria, colonic fermentation, and gastrointestinal health. J. AOAC Int. 95, 50–60. doi: 10.5740/jaoacint.SGE-Macfarlane
Macfarlane, S., Bahrami, B., and Macfarlane, G. T. (2011). Mucosal biofilm communities in the human intestinal tract. Adv. Appl. Microbiol. 75, 111–43. doi: 10.1016/B978-0-12-387046-9.00005-0
MacLean, R. C., Fuentes-Hernandez, A., Greig, D., Hurst, L. D., and Gudelj, I. (2010). A mixture of “cheats” and “co-operators” can enable maximal group benefit. PLoS Biol. 8:e1000486. doi: 10.1371/journal.pbio.1000486
Marchal, M., Goldschmidt, F., Derksen-Müller, S. N., Panke, S., Ackermann, M., and Johnson, D. R. (2017). A passive mutualistic interaction promotes the evolution of spatial structure within microbial populations. BMC Evol. Biol. 17:106. doi: 10.1186/s12862-017-0950-y
Mas, A., Jamshidi, S., Lagadeuc, Y., Eveillard, D., and Vandenkoornhuyse, P. (2016). Beyond the black queen hypothesis. ISME J. 10, 2085–2091. doi: 10.1038/ismej.2016.22
Maynard, C. L., Elson, C. O., Hatton, R. D., and Weaver, C. T. (2012). Reciprocal interactions of the intestinal microbiota and immune system. Nature 489, 231–241. doi: 10.1038/nature11551
Mee, M. T., Collins, J. J., Church, G. M., and Wang, H. H. (2014). Syntrophic exchange in synthetic microbial communities. Proc. Natl. Acad. Sci. U. S. A. 111, E2149–E2156. doi: 10.1073/pnas.1405641111
Moens, F., Verce, M., and De Vuyst, L. (2017). Lactate- and acetate-based cross-feeding interactions between selected strains of lactobacilli, bifidobacteria and colon bacteria in the presence of inulin-type fructans. Int. J. Food Microbiol. 241, 225–236. doi: 10.1016/j.ijfoodmicro.2016.10.019
Moens, F., Weckx, S., and De Vuyst, L. (2016). Bifidobacterial inulin-type fructan degradation capacity determines cross-feeding interactions between bifidobacteria and Faecalibacterium prausnitzii. Int. J. Food Microbiol. 231, 76–85. doi: 10.1016/j.ijfoodmicro.2016.05.015
Momeni, B., Waite, A. J., and Shou, W. (2013). Spatial self-organization favors heterotypic cooperation over cheating. eLife 2:e00960. doi: 10.7554/eLife.00960
Morris, B. E. L., Henneberger, R., Huber, H., and Moissl-Eichinger, C. (2013). Microbial syntrophy: interaction for the common good. FEMS Microbiol. Rev. 37, 384–406. doi: 10.1111/1574-6976.12019
Morris, J. J. (2015). Black queen evolution: the role of leakiness in structuring microbial communities. Trends Genet. 31, 475–482. doi: 10.1016/j.tig.2015.05.004
Morris, J. J., Lenski, R. E., and Zinser, E. R. (2012). The black queen hypothesis: evolution of dependencies through adaptive gene loss. mBio 3:e00036–12. doi: 10.1128/mBio.00036-12
Müller, M. J. I., Neugeboren, B. I., Nelson, D. R., and Murray, A. W. (2014). Genetic drift opposes mutualism during spatial population expansion. Proc. Natl Acad. Sci. U.S.A. 111, 1037–1042. doi: 10.1073/pnas.1313285111
Nadell, C. D., Foster, K. R., and Xavier, J. B. (2010). Emergence of spatial structure in cell groups and the evolution of cooperation. PLoS Comp. Biol. 6:e1000716. doi: 10.1371/journal.pcbi.1000716
Oliveira, N. M., Niehus, R., and Foster, K. R. (2014). Evolutionary limits to cooperation in microbial communities. Proc. Natl. Acad. Sci. U.S.A. 111, 17941–17946. doi: 10.1073/pnas.1412673111
Pacheco, A. R., Moel, M., and Segrè, D. (2019). Costless metabolic secretions as drivers of interspecies interactions in microbial ecosystems. Nat. Commun. 10:103. doi: 10.1038/s41467-018-07946-9
Pande, S., Kaftan, F., Lang, S., Svato, A., Germerodt, S., and Kost, C. (2016). Privatization of cooperative benefits stabilizes mutualistic cross-feeding interactions in spatially structured environments. ISME J. 10, 1413–1423. doi: 10.1038/ismej.2015.212
Pande, S., and Kost, C. (2017). Bacterial unculturability and the formation of intercellular metabolic networks. Trends Microbiol. 25, 349–361. doi: 10.1016/j.tim.2017.02.015
Pande, S., Merker, H., Bohl, K., Reichelt, M., Schuster, S., De Figueiredo, L. F., et al. (2014). Fitness and stability of obligate cross-feeding interactions that emerge upon gene loss in bacteria. ISME J. 8, 953–962. doi: 10.1038/ismej.2013.211
Pande, S., Shitut, S., Freund, L., Westermann, M., Bertels, F., Colesie, C., et al. (2015). Metabolic cross-feeding via intercellular nanotubes among bacteria. Nat. Commun. 6:6238. doi: 10.1038/ncomms7238
Penders, J., Thijs, C., Vink, C., Stelma, F. F., Snijders, B., Kummeling, I., et al. (2006). Factors influencing the composition of the intestinal microbiota in early infancy. Pediatrics 118, 511–521. doi: 10.1542/peds.2005-2824
Pfeiffer, T., and Bonhoeffer, S. (2004). Evolution of cross-feeding in microbial populations. Am. Nat. 163, E126–135. doi: 10.1086/383593
Poltak, S. R., and Cooper, V. S. (2011). Ecological succession in long-term experimentally evolved biofilms produces synergistic communities. ISME J. 5, 369–378. doi: 10.1038/ismej.2010.136
Porcher, E., Tenaillon, O., and Godelle, B. (2001). From metabolism to polymorphism in bacterial populations: a theoretical study. Evolution 55, 2181–2193. doi: 10.1111/j.0014-3820.2001.tb00734.x
Rainey, P. B., and Travisano, M. (1998). Adaptive radiation in a heterogeneous environment. Nature 394, 69–72.
Rakoff-Nahoum, S., Foster, K. R., and Comstock, L. E. (2016). The evolution of cooperation within the gut microbiota. Nature 533, 255–259. doi: 10.1038/nature17626
Rivière, A., Gagnon, M., Weckx, S., Roy, D., and De Vuyst, L. (2015). Mutual cross-feeding interactions between Bifidobacterium longum subsp. longum NCC2705 and Eubacterium rectale ATCC 33656 explain the bifidogenic and butyrogenic effects of arabinoxylan oligosaccharides. Appl. Environ. Microbiol. 81, 7767–7781. doi: 10.1128/aem.02089-15
Rosenzweig, R. F., Sharp, R. R., Treves, D. S., and Adams, J. (1994). Microbial evolution in a simple unstructured environment: genetic differentiation in Escherichia coli. Genetics 137, 903–917.
Rossi, M., Corradini, C., Amaretti, A., Nicolini, M., Pompei, A., Zanoni, S., et al. (2005). Fermentation of fructooligosaccharides and inulin by bifidobacteria: a comparative study of pure and fecal cultures. Appl. Environ. Microbiol. 71, 6150–6158. doi: 10.1128/aem.71.10.6150-6158.2005.
Rozen, D. E., and Lenski, R. E. (2000). Long-term experimental evolution in Escherichia coli. VIII. dynamics of a balanced polymorphism. Am. Nat. 155, 24–35. doi: 10.1086/303299
Rozen, D. E., Schneider, D., and Lenski, R. E. (2005). Long-term experimental evolution in Escherichia coli. XIII. Phylogenetic history of a balanced polymorphism. J. Mol. Evol. 61, 171–180. doi: 10.1007/s00239-004-0322-2
Sachs, J. L., and Hollowell, A. C. (2012). The origins of cooperative bacterial communities. mBio 3, e00099–12. doi: 10.1128/mBio.00099-12
Sachs, J. L., Mueller, U. G., Wilcox, T. P., and Bull, J. J. (2004). The evolution of cooperation. Q. Rev. Biol. 79, 135–160. doi: 10.1086/383541
Salonen, A., Lahti, L., Salojärvi, J., Holtrop, G., Korpela, K., Duncan, S. H., et al. (2014). Impact of diet and individual variation on intestinal microbiota composition and fermentation products in obese men. ISME J. 8, 2218–2230. doi: 10.1038/ismej.2014.63
Schwartz, M. W., and Hoeksema, J. D. (1998). Specialization and resource trade: biological markets as a model of mutualisms. Ecology 79, 1029–1038.
Shitut, S., Ahsendorf, T., Pande, S., Egbert, M., and Kost, C. (2019). Nanotube-mediated cross-feeding couples the metabolism of interacting bacterial cells. Environ. Microbiol. 21, 1306–1320. doi: 10.1111/1462-2920.14539
Stams, A. J. M., and Plugge, C. M. (2009). Electron transfer in syntrophic communities of anaerobic bacteria and archaea. Nat. Rev. Microbiol. 7, 568–577. doi: 10.1038/nrmicro2166
Sun, Z., Koffel, T., Stump, S. M., Grimaud, G. M., and Klausmeier, C. A. (2019). Microbial cross-feeding promotes multiple stable states and species coexistence, but also susceptibility to cheaters. J. Theor. Biol. 465, 63–77. doi: 10.1016/j.jtbi.2019.01.009
Tolker-Nielsen, T., and Molin, S. (2000). Spatial organization of microbial biofilm communities. Microb. Ecol. 40, 75–84. doi: 10.1007/s002480000057
Traverse, C. C., Mayo-Smith, L. M., Poltak, S. R., and Cooper, V. S. (2013). Tangled bank of experimentally evolved Burkholderia biofilms reflects selection during chronic infections. Proc. Natl. Acad. Sci. U.S.A. 110, E250–E259. doi: 10.1073/pnas.1207025110
Treves, D. S., Manning, S., and Adams, J. (1998). Repeated evolution of an acetate-crossfeeding polymorphism in long-term populations of Escherichia coli. Mol. Biol. Evol. 15, 789–797.
Van Wey, A., Lovatt, S., Roy, N., and Shorten, P. (2016). Determination of potential metabolic pathways of human intestinal bacteria by modeling growth kinetics resulting from cross-feeding. Food Res. Int. 88, 207–216. doi: 10.1016/j.foodres.2016.02.004
Van Wey, A. S., Cookson, A. L., Roy, N. C., McNabb, W. C., Soboleva, T. K., and Shorten, P. R. (2011). Bacterial biofilms associated with food particles in the human large bowel. Mol. Nutr. Food Res. 55, 969–978. doi: 10.1002/mnfr.201000589
Van Wey, A. S., Cookson, A. L., Soboleva, T. K., Roy, N. C., McNabb, W. C., Bridier, A., et al. (2012). Anisotropic nutrient transport in three-dimensional single species bacterial biofilms. Biotechnol. Bioeng. 109, 1280–1292. doi: 10.1002/bit.24390
Velicer, G. J., and Vos, M. (2009). Sociobiology of the myxobacteria. Annu. Rev. Microbiol. 63, 599–623. doi: 10.1146/annurev.micro.091208.073158
Walker, A. W., Ince, J., Duncan, S. H., Webster, L. M., Holtrop, G., Ze, X., et al. (2011). Dominant and diet-responsive groups of bacteria within the human colonic microbiota. ISME J. 5, 220–230. doi: 10.1038/ismej.2010.118
Wanner, O., Eberl, H. J., Morgenroth, E., Noguera, D.l.R , Picioreanu, C., et al. (2006). Mathematical Modeling of Biofilms. London: IWA Publishing.
West, S. A., Diggle, S. P., Buckling, A., Gardner, A., and Griffin, A. S. (2007a). The social lives of microbes. Annu. Rev. Ecol. Evol. Syst. 38, 53–77. doi: 10.1146/annurev.ecolsys.38.091206.095740
West, S. A., Griffin, A. S., and Gardner, A. (2007b). Social semantics: altruism, cooperation, mutualism, strong reciprocity and group selection. J. Evol. Biol. 20, 415–432. doi: 10.1111/j.1420-9101.2006.01258.x
West, S. A., Griffin, A. S., Gardner, A., and Diggle, S. P. (2006). Social evolution theory for microorganisms. Nat. Rev. Microbiol. 4, 597–607. doi: 10.1038/nrmicro1461
Widder, S., Allen, R. J., Pfeiffer, T., Curtis, T. P., Wiuf, C., Sloan, W. T., et al. (2016). Challenges in microbial ecology: building predictive understanding of community function and dynamics. ISME J. 10, 2557–2568. doi: 10.1038/ismej.2016.45
Wintermute, E. H., and Silver, P. A. (2010a). Dynamics in the mixed microbial concourse. Genes Dev. 24, 2603–2614. doi: 10.1101/gad.1985210
Wintermute, E. H., and Silver, P. A. (2010b). Emergent cooperation in microbial metabolism. Mol. Syst. Biol. 6:407. doi: 10.1038/msb.2010.66
Yamamura, N., Higashi, M., Behera, N., and Yuichiro Wakano, J. (2004). Evolution of mutualism through spatial effects. J. Theor. Biol. 226, 421–428. doi: 10.1016/j.jtbi.2003.09.016
Ze, X., Duncan, S. H., Louis, P., and Flint, H. J. (2012). Ruminococcus bromii is a keystone species for the degradation of resistant starch in the human colon. ISME J. 6, 1535–1543. doi: 10.1038/ismej.2012.4
Ze, X., Le Mougen, F., Duncan, S. H., Louis, P., and Flint, H. J. (2013). Some are more equal than others: the role of “keystone” species in the degradation of recalcitrant substrates. Gut Microbes 4, 236–240. doi: 10.4161/gmic.23998
Keywords: cross-feeding, mutualism, adaptive gene loss, black queen hypothesis, bacteria, microbes, experimental evolution, mathematical modeling
Citation: Smith NW, Shorten PR, Altermann E, Roy NC and McNabb WC (2019) The Classification and Evolution of Bacterial Cross-Feeding. Front. Ecol. Evol. 7:153. doi: 10.3389/fevo.2019.00153
Received: 05 November 2018; Accepted: 18 April 2019;
Published: 14 May 2019.
Edited by:
William Harcombe, University of Minnesota Twin Cities, United StatesReviewed by:
Sara Mitri, Université de Lausanne, SwitzerlandAlexandra Lauren McCully, Stanford University, United States
Copyright © 2019 Smith, Shorten, Altermann, Roy and McNabb. This is an open-access article distributed under the terms of the Creative Commons Attribution License (CC BY). The use, distribution or reproduction in other forums is permitted, provided the original author(s) and the copyright owner(s) are credited and that the original publication in this journal is cited, in accordance with accepted academic practice. No use, distribution or reproduction is permitted which does not comply with these terms.
*Correspondence: Paul R. Shorten, paul.shorten@agresearch.co.nz