- 1Department of Entomology, University of California, Riverside, Riverside, CA, United States
- 2Department of Ecology and Evolutionary Biology, University of Michigan, Ann Arbor, MI, United States
- 3Center for the Study of Complex Systems, University of Michigan, Ann Arbor, MI, United States
- 4Institute of Evolution and Ecology, Eberhard Karls Universität Tübingen, Tübingen, Germany
- 5Institute of Ecology and Environmental Sciences, Université Pierre et Marie Curie, Paris, France
- 6Department of Environmental Science, Policy, and Management, University of California, Berkeley, Berkeley, CA, United States
- 7Departamento de Ecologia, Universidade de São Paulo, São Paulo, Brazil
- 8Department of Entomology, Cornell University, Ithaca, NY, United States
- 9Department of Biology, Stanford University, Stanford, CA, United States
Species interactions are responsible for many key mechanisms that govern the dynamics of ecological communities. Variation in the way interactions are organized among species results in different network structures, which translates into a community's ability to resist collapse and change. To better understand the factors involved in dictating ongoing dynamics in a community at a given time, we must unravel how interactions affect the assembly process. Here, we build a novel, integrative conceptual model for understanding how ecological communities assemble that combines ecological networks and island biogeography theory, as well as the principles of niche theory. Through our conceptual model, we show how the rate of species turnover and gene flow within communities will influence the structure of ecological networks. We conduct a preliminary test of our predictions using plant-herbivore networks from differently-aged sites in the Hawaiian archipelago. Our approach will allow future modeling and empirical studies to develop a better understanding of the role of the assembly process in shaping patterns of biodiversity.
1. Introduction
Species interactions shape the dynamics of ecological communities. As communities assemble, species accumulate—despite prevailing biotic and abiotic conditions—either through speciation or immigration (Hutchinson, 1957; Fukami, 2015; Mittelbach and Schemske, 2015). Antagonistic, mutualistic and facilitatory interactions among species can act as gate keepers to new species, and as a consequence, eco-evolutionary feedbacks between species can determine the trajectory of community assembly (Post and Palkovacs, 2009; Gravel et al., 2011; Pillai et al., 2011; De Meester and Pantel, 2014; Fahimipour and Hein, 2014; Fukami, 2015; Mittelbach and Schemske, 2015; Cadotte and Tucker, 2017). But how do ecological networks assemble?
Since the 1970s, researchers have searched for patterns in community assembly (e.g., Cody and Diamond, 1975; Drake, 1990; Morton et al., 1996; Chase, 2003; Tilman, 2004; Fukami, 2015), though species interaction networks were not explicitly considered until a decade later (Pimm, 1979; Post and Pimm, 1983). Subsequently, how interaction-based, eco-evolutionary feedbacks shape the community emergence has become the subject of numerous modeling approaches (Loeuille and Loreau, 2005; Fussmann et al., 2007; Brännström et al., 2011; Allhoff et al., 2015; de Andreazzi et al., 2018, see Brännström et al., 2012 for a review). To test whether models reflect likely mechanisms of community assembly, authors compare the networks generated by their models to the interaction patterns observed in real-world communities. However, a non-standard suite of summary statistics of the final simulated and empirical networks are compared—often overlooking the community's trajectory to that endpoint (see, Yeakel et al., 2014). By ignoring the intrinsically dynamic nature of assembly, our understanding of the process is undermined. The likely reason investigators disregard the assembly process is 2-fold: (1) despite the plethora of network summary metrics that can be used to capture different aspects of network architecture (Newman, 2010), there are no clear guidelines on what aspects of network structure will change with assembly; and (2) few empirical studies exist for comparison because it is time-intensive and expensive to capture interaction assembly dynamics (see, Olesen et al., 2008; Albrecht et al., 2010; Ponisio et al., 2017; Tylianakis et al., 2018, reviewed in Bascompte and Stouffer, 2009), particularly over deep evolutionary timescales (Olesen et al., 2010).
New innovations in machine learning and metabarcoding—to allow identification of all members that make up a community and their interactions—will likely improve inference of long-term, higher resolution community network surveys in the near future (Kartzinel et al., 2015; Pornon et al., 2016; Freilich et al., 2018). To address the first impediment, here we propose a conceptual model to study and predict how networks change as communities assemble. Our conceptual model is anchored in niche theory including niche dynamics (niche breadth and overlap), dispersal, and eco-evolutionary feedbacks (taxon cycles, ecological release, and character displacement), from which we derive predictions about interaction network assembly. Our approach will help to guide future analytical modeling and empirical studies to develop a better understanding of the role ecological and evolutionary processes play in shaping community-level patterns.
We then focus on well-studied island systems to review how interactions change as a community assembles. Islands provide discrete, often differently-aged communities, allowing us to study how interactions change over an extended period of time (Brodie, 2017). By comparing the trajectory of community assembly on isolated islands with little species turnover or gene flow to near-shore islands with comparatively high turnover and gene flow, we are able to elucidate the importance of current and historic community characteristics in shaping interaction patterns. Habitat patches embedded within a less hospitable matrix may also function as biological islands (Gillespie and Roderick, 2002). Thus, understanding the mechanisms underlying the assembly of true island systems is applicable to a broad set of ecological questions. Lastly, we provide an approach for comparing network structure as a community assembles and apply those approaches within an existing dataset of Hawaiian plant-herbivore interactions.
2. Conceptual Model
2.1. Niche Theory: Limiting Similarity and the Benefits of Specialization
Theory concerned with predicting interaction occurrence often involves two principles thought to increase an individual's fitness. The first is limiting similarity (Macarthur and Levins, 1967; Abrams, 1983), since increasing sharing of resources or other ecological factors—referred to as niche overlap—intensifies competition. Thus, one individual will either out-compete the other, or each will modify their niche space in order to minimize competition (Macarthur and Levins, 1967; Abrams, 1983). In the original description of this principle, limiting similarity was a property of populations (Gause, 1932; Hutchinson, 1959), but since then, this principle has been expanded to capture individual plasticity in resource use (Bolnick et al., 2002; Brosi, 2016). When applied to interaction networks, the interaction niche is the identity of the possible partners with which a species can interact, and interaction niche overlap is partner sharing between species of the same trophic level (Box 1). Here we use “interaction partner” to describe any type of interaction between two species, for example a mutualistic interaction between a bee and a flower, or a consumptive interaction between a caterpillar and plant.
Box 1. Metrics Used to Describe Ecological Network Structure
Interaction Niche Overlap
Niche overlap measures the similarity in the interaction patterns among species in the same trophic level (e.g., plants or pollinators). The Similarity measures such as the Jaccard index can be used to quantify overlap:
where A and B are community samples. The closer to 1, the more similar interaction partners two species have, and the greater the niche overlap (Figure 2).
Interaction Niche Breadth
Niche breadth relates to the number of partners with which a species can interact, often quantified as a species' degree or rarefied species degree (Ponisio et al., 2017). Species that have many partners (i.e., have high degree) are defined as generalists. In contrast, species that have a small number of interaction partners (small degree) have a narrow niche breadth and are defined as specialists (Figure 2).
Nestedness
Nestedness is a network pattern in which the interactions of species with fewer interactions (specialist species) are proper subsets of the interactions of generalist species (Bascompte et al., 2003; Vázquez et al., 2007) (Figure 1). NODF is a commonly used metric:
for a matrix with m rows and n columns and Npaired is the degree of paired nestedness (Almeida-neto et al., 2008).
Modularity
Modularity is a network pattern in which species within particular subsets, or modules (also called compartments), of the network interact more commonly among themselves than with species outside that module (Newman and Girvan, 2004; Olesen et al., 2007; Stouffer and Bascompte, 2011) (Figure 1). There are many ways to calculate which nodes are assigned to what modules (i.e., module membership), each with its own pitfalls (for a review see Fortunato, 2010). The network's modularity (Q) is then calculated with respect to the membership (Newman and Girvan, 2004)
where the sum runs over all pairs of vertices ij. Here m is the number of edges, Aij is the element of the A adjacency matrix in row i and column j, Pij is the null model expectation for number of edges between vertices i and j, and δ is an indicator function with value 1 when species i and j are in the same module g and 0 otherwise.
The second principle is the benefits of specialization, often represented by the idiomatic phrase: “a jack of all trades is a master of none” (Gause, 1936; Holt, 1977; Parrish and Bazzaz, 1979; Waser et al., 1996; Chase and Leibold, 2003; Morlon et al., 2014). If resources are available and stable in the environment, species that use a narrower range of resources, or have narrower niche breadth (also referred to as niche width), have a fitness advantage because they are able to more efficiently use the available resources (i.e., specialists) (Leigh Jr, 1975; MacArthur, 1984). In contrast, specialization will be less likely to have a fitness advantage when resources are unstable (Herrera, 1988, 1996; Gómez and Zamora, 2006). When considering networks, the interaction niche breadth is the total of all possible partners with which a species can interact (Box 1).
2.2. Evolutionary Context: Evolutionary and Phenotypic Distinctness
Phylogenetic and phenotypic distinctness will influence the interactions in which species can participate (Vermeij, 1994; Jordano, 1995; Thompson, 2005; Ives and Godfray, 2006; Petchey and Gaston, 2006; Rezende et al., 2007; Donatti et al., 2011; Schleuning et al., 2015; Coux et al., 2016; Pigot et al., 2016). Studies found that species that were distantly related to other community members were not only phenotypically distinct, but also more specialized (Coux et al., 2016; Pigot et al., 2016, but see Donatti et al., 2011; Fritschie et al., 2014). The distribution of phenotypic and/or phylogenetic distinctness in a community, thus, will affect both the average and variance in interaction niche overlap and breadth. In addition, distinct species with specialized interaction needs (either in consumer-resource, mutualistic, or facilitatory interactions) are often unsuccessful at entering a community (Holt et al., 1999; Piechnik et al., 2008; Gravel et al., 2011; Fukami, 2015). This limitation has been considered especially important in island biogeography (Wallace, 1891; Carlquist, 1974; Armbruster and Baldwin, 1998; Nobre et al., 2010).
2.3. Ecological Context: Intra- and Inter-specific Competition
The distribution of interaction niche overlaps will determine where intra- or inter-specific competition is the most limiting to population growth. When inter-specific competition is strong, a species' individuals may specialize on the resources, constricting the population-level interaction niche (Day and Young, 2004; Bolnick et al., 2010; Brosi and Briggs, 2013; Fründ et al., 2013). Similarly, release from inter-specific competition, known as competitive or ecological release, increases population niche breadth by increasing among-individual variation (Bolnick et al., 2010). On islands, the expansion of niche breadth in island taxa compared to continental relatives, driven by the absence of antagonistic interactions including competition, has long been noted as an island syndrome in evolutionary biology (Yeaton, 1974; Werner and Sherry, 1987).
Conversely, when competition within a species is strong, individuals may specialize on distinct interaction partners, broadening the population-level niche breadth (Bolnick, 2001; Bolnick et al., 2002; Svanbäck and Bolnick, 2007; Araújo et al., 2011; Brosi, 2016). Thus interaction niche breadth and overlap will change at the individual and population level based on the strength of inter- and intra-specific competition.
2.4. Eco-Evolutionary Feedbacks
The ecological context in which species are embedded will affect the evolutionary/phenotypic distinctness of species in a community. Traits that enable species interactions are shaped by reciprocal selection and, over evolutionary time scales, by patterns of speciation. Faced with intra-specific competition, individual specialization can lead to frequency-dependent disruptive selection (Bolnick, 2004; Bolnick and Lau, 2008). Similarly, with high inter-specific competition, character displacement can lead to interaction partner displacement (Grant and Grant, 2006), resulting in a species' realized niche breadth eventually becoming its fundamental niche (Rosenzweig, 1978), and ultimately greater species-level specialization. Given the hypothesized benefit of specialization and limiting similarity, consistent selection between interaction partners may favor specialization and niche partitioning among species (e.g., the taxon cycle, Ricklefs and Bermingham, 2002). Such an interaction pattern would also only result if there were no other limits to evolutionary and coevolutionary adaptions and the network is a true representation of the interacting community (Box 2).
Box 2. Other Determinants of Network Structure
Limits to Evolutionary/Coevolutionary Adaptation
Selection favoring limiting interaction overlap and breadth is limited by species' ability to restrict resource access. The ability of animals to use flowers and fruits, for example, is constrained by morphological limitations that could have evolved as a response by plants in order to control interactions with animals. Subsequently, these types of interactions are constrained and may never evolve toward high levels of interaction niche separation (Guimarães Jr. et al., 2007; Fontaine et al., 2009, 2011). Conversely, hosts can evolve specialized defenses against parasites, allowing for higher levels of specialization and interaction niche separation between parasites (Fontaine et al., 2009, 2011). The evolutionary adaptation of interactions may be constrained not only by morphological mismatches, but also by the available raw genetic material (Vanoverbeke et al., 2016). In this sense, there is a limited range of possibilities toward which interactions can evolve.
Lastly, different biotic and abiotic interactions may create conflicting selective pressures. For example, floral traits that promote pollination can accelerate attack rate by herbivores (Strauss et al., 1999). Thus, when considering how communities assemble, it is necessary to consider the interplay among ability to access resources, species' genetic diversity, and the existence of antagonistic selective pressures.
The “True” Network?
A network should reflect a community where all species have the potential to interact (i.e., then temporal and spatial ranges overlap). When different spatial and temporal scales are considered, mismatches between the spatio-temporal distribution of species will lead to “forbidden links” (Jordano et al., 2003). The existence of forbidden links, in turn, will often lead to a nested interaction pattern (Vázquez et al., 2009; Encinas-Viso et al., 2012). It is thus important to consider the spatial and temporal scale of sampling in relation to the ecology of the species in question when interpreting interaction network structure.
Conversely, continual turnover in either the environment and/or interaction partners will favor generalization (Waser et al., 1996; Hawkins, 2005; Thompson, 2005; Gómez and Zamora, 2006; Montoya et al., 2006; Loeuille and Leibold, 2008; Urban et al., 2008) because a species with a narrow interaction niche breadth will be restricted by its biotic requirements (Wilson, 1961; Ricklefs and Bermingham, 2002). In addition, gene flow between populations will dilute the selective pressure on specific, pairwise species interactions (Herrera, 1988, 1996; Waser et al., 1996; Thompson, 2005; Gómez and Zamora, 2006). Thus over evolutionary time scales, communities with high gene flow between populations and partner turnover will not favor interaction patterns in which species or groups of species are specialized on distinct sets of resources (Waser et al., 1996; Gómez and Zamora, 2006).
2.5. Networks and Interaction Niches
Niche patterns may be translated into different network architectures depending on the degree of, and variance in, interaction niche breadth and overlap (Cohen and Stephens, 1978; Araújo et al., 2011; Valdovinos et al., 2016). Two of the most used patterns to describe network architecture are modularity and nestedness. Modularity measures the extent to which species in a community form tightly interacting groups of species, or modules (Box 1). On the other hand, in nested networks, specialist species interact with a subset of the species that generalists interact with (Box 1). Modular networks can also have compartments that are nested (Figure 1C). Modularity emerges from increased niche partitioning and decreased niche breadth (Figures 1A,C,E), whereas nestedness emerges from increased niche overlap and a high variance in niche breadth (Figure 1D). Both measures of network architecture capture essential structural features affected by niche breadth and overlap, and are expected to be tightly linked with community stability (Thébault and Fontaine, 2010; Valdovinos et al., 2016). Networks dominated by generalist or specialist species with substantial interaction overlap will neither be nested nor modular (Figures 1B,F).
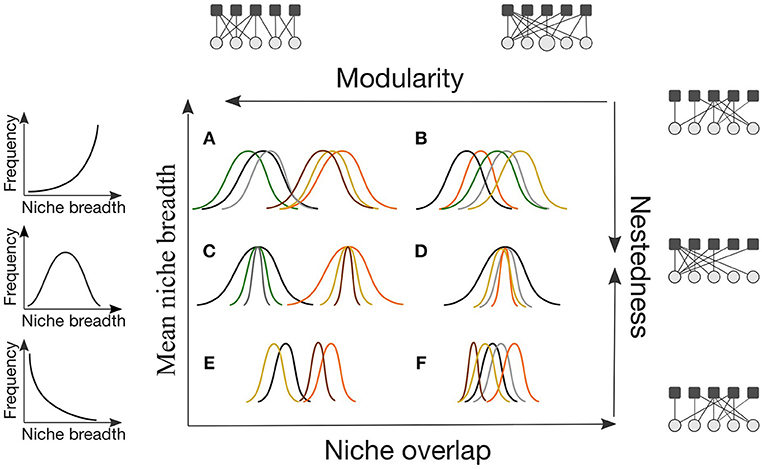
Figure 1 Conceptual figure illustrating the interplay of interaction niche overlap and interaction niche breadth in determining the arrangement of interactions in a community, and specifically nestedness and modularity (A–F). In the accompanying network figures, white circles represent one trophic level (e.g., pollinators) and dark squares represent the other trophic level (e.g., plants). Each color represents a species. Within our review, we could not find evidence of communities exhibiting a structure as depicted in (F).
Depending on current ecological context and evolutionary history, interaction patterns will differ in their degree of niche overlap and average niche breadth, as well as the variance of both between species. As discussed above, the turnover of interaction partners and gene flow will influence an individual's ability to limit similarity and specialize. The evolutionary and phenotypic distinctness of species entering a community will also influence the distribution of niche overlap and breadth. Lastly, the strength of inter- and intra-specific competition will influence a species's realized niche overlap and breadth.
The community characteristics (species turnover, gene flow, intra- and inter-specific competition, evolutionary/phenotypic distinctness) that shape niche breadth and overlap, in turn, are a function of a community's stage of assembly and isolation (MacArthur and Wilson, 1967; Levin, 1981). Just as species richness is determined by the balance between species extinction rate and the rate at which new species enter a community (either due to colonization or in situ speciation), our conceptual model predicts that: (1) the rates of species and gene flow within communities will affect the patterns observed in interaction networks, and (2) as assembly progresses, isolated systems will support greater interaction specialization and niche partitioning (Figures 2, 3). In contrast, we predict the opposite trend in proximate environments, where high species and genetic turnover will result in communities having high levels of niche overlap and limited specialization (Figures 2, 3).
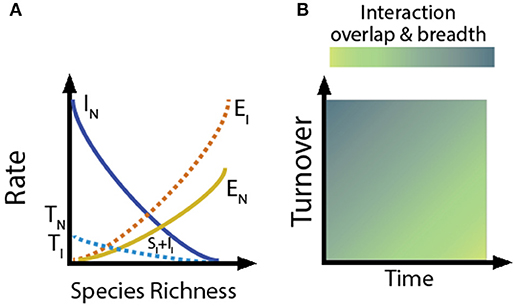
Figure 2 Extension of classical theory of island biogeography to understanding how network structure changes as communities assemble. (A) For a given area, the rate of species turnover will depend on the rates of immigration, speciation, and extinction in a community. The species extinction rate near source pools (EN) will be lower than that far from source pools (EI) because of the rescue effect. Similarly, immigration rates (II) will be lower for communities far from source pools, but speciation (SI) will be higher. Because the intersection between EN and IN (solid lines) is greater than the intersection between EI and SI + II (dashed lines), the rate of species turnover in the community near the source pool (TN) is higher than in the more isolated community (TI). (B) Low rates of species turnover and many generations of evolution/coevolution will favor niche partitioning and specialization, whereas high turnover will favor niche overlap and generalization.
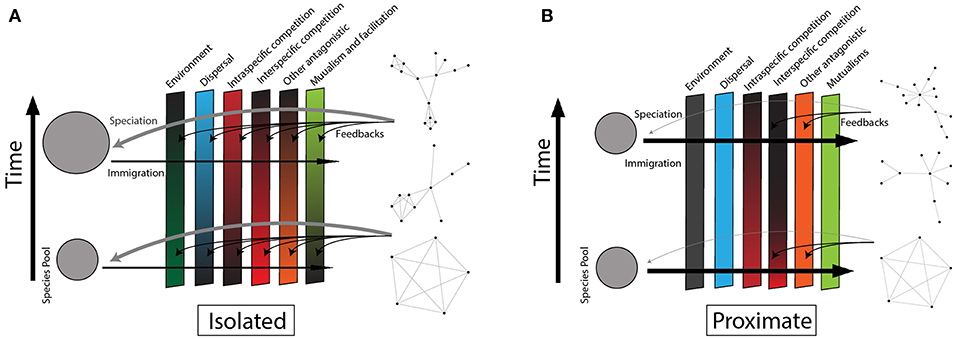
Figure 3. Adaptation of the classic community assembly conceptual model with the addition of species interaction networks. Time and assembly progress from figure bottom to figure top, and species begin in the source pool (left) and progress through the filters to join the community interaction network (right). A variable's strength in determining which species that can enter a community (“filter”) is indicated by the rectangular panel's shade (darker color is a strong filter). Interaction structure feedbacks to affect the strength of the variables as filters (black curved arrows). Black horizontal arrows indicate immigration from the source pool and gray curved arrows represent in situ speciation. (A) Represents an isolated community (e.g., Hawaii). The abiotic environment is a weak filter in comparison to dispersal. Few species disperse with their mutualists/facilitators, but these interactions accumulate as species (co)evolve. Once a few species have dispersed, intra-specific competition is high, leading at first to expanding species-level diet breadth as subsets of the population specialize, and then eventually speciate. Inter-specific competition is originally low due to the low rate of immigration and species richness, but this increases as clades diversify and contribute to the species pool. Networks assemble toward niche partitioning and specialization as evolution/coevolution shape species phenotypes to maximize the efficiency of interactions. (B) Represents a non-isolated community (e.g., nearshore mangrove islands). Dispersal is not limited, and species that are pre-adapted to the abiotic environment out-compete others. Inter-specific competition is high, while intra-specific competition is low due to the continual immigration of new species and individuals from neighboring islands. Species and genetic turnover inhibit selection for interaction efficiency, so interactions remain relatively generalized with high overlap. Networks have limited interaction niche partitioning and specialization.
2.6. A Note About Different Types of Interactions
Most of the theory on which we base our conceptual model is focused on competitive interactions. We do this because competition is widespread, has a long history of study especially as a part of adaptive radiation (Schluter, 2000), and because other types of interactions are likely to contain competitive processes within guilds or trophic levels (Ehrlich and Raven, 1964; Armbruster and Muchhala, 2009). We note, however, that other types of interactions can have different effects on selection and diversification (Yoder and Nuismer, 2010; Hembry et al., 2014), and we thus do not necessarily expect all interaction types to follow these same mechanisms and assembly rules under all circumstances. Throughout, where it is useful and illustrative, we invoke examples from non-competitive antagonistic and mutualistic interactions alongside those from competition.
3. Conceptual Model Predictions
3.1. Partner Predictability and Low Gene Flow in Isolated Systems
Island biogeography theory predicts that dispersal to isolated communities will be rare in all stages of assembly. Sporadically colonizing species, initially freed from predators, parasites, and inter-specific competition, will be confronted with an array of open niche space (Figure 3A). Species that depend on specific interaction partners are rarely early colonizers due to the low likelihood of both species simultaneously reaching a new community. Consequently, the filter is high for specialized consumer-resource interactions, mutualisms, and facilitative interactions (Figure 3A, Holt et al., 1999; Piechnik et al., 2008; Gravel et al., 2011; Fukami, 2015). Having colonized, antagonistic interactions that limit population growth and establishment in mainland systems, such as herbivory and predation, will be less important because of the low likelihood the antagonists colonize with the host/prey species (Figure 3A).
Early arrival may allow some species to adapt and monopolize resources (i.e., priority and monopolization effects, Gillespie, 2004; Fukami, 2015; Shaw and Gillespie, 2016; Vanoverbeke et al., 2016), precluding other species from establishing or persisting, a phenomenon known as a priority effect. Because priority effects are more likely in systems with resource and composition stability (Fukami, 2015), their effects can be particularly strong on isolated islands, depending on the disturbance regime. We thus expect that networks of early stage isolated communities will be composed of predominantly generalist species due to either (1) ecological release of interaction specialization subsequent to colonization; or (2) being generalist prior to colonization. The degree of niche overlap will depend on the evolutionary history and traits of the species that happen to colonize. Thus the community at very early assembly stages will be composed of generalists with either little (Figure 1A) or some niche overlap (Figure 1, between A and B), resulting in a non-nested networks that are either also non-modular (Figure 1B) or somewhat modular (Figure 1, between A and B).
As colonists' abundance increases, intra-specific competition also increases (Figure 3A, negative frequency-dependence, Chesson, 2000; HilleRisLambers et al., 2012), potentially favoring species that are able to partition resources amongst individuals by increasing individual specialization on specific interaction partners. This individual-level specialization (Roughgarden (1972)'s “within niche component") results in a broader niche at the species level, potentially increasing overlap between species (Roughgarden (1972)'s “between niche component") (Wilson, 1961; Roughgarden, 1972). The species interaction networks at this stage will continue to be generalized but also shift toward more niche overlap, resulting in low nestedness and modularity (Figure 1B).
As assembly continues, subsets of the population that specialize on different resources and experience distinct selection pressures may become reproductively isolated, ultimately leading to adaptive radiations via ecological speciation for some lineages (Figure 3A, Roughgarden, 1972; Rainey and Travisano, 1998; Grant, 1999; Travisano and Rainey, 2000; Ackermann and Doebeli, 2004; Day and Young, 2004). In situ specialization becomes an alternative source of new species (weakening the dispersal filter, Figure 3A, Mittelbach and Schemske, 2015). The increase in species richness will also increase and intensify inter-specific competition (Figure 3A), favoring resource partitioning and partner specialization between species (Wilson, 1961; Schluter, 2000; Ricklefs and Bermingham, 2002; Maherali and Klironomos, 2007; Knelman and Nemergut, 2014). For example, when presented with potential interactions with the Galápagos flora, Darwin's finches evolved specialized beak morphologies to consume the seeds of different plants under variable abiotic conditions (Grant, 1999; Guerrero and Tye, 2009). Similar radiations to exploit novel interaction partners have been documented in lizards on oceanic islands and Hawaiian honeycreepers (Olesen and Valido, 2003; Lerner et al., 2011). Assuming that evolutionarily similar species interact with similar partners (Coux et al., 2016; Pigot et al., 2016), we expect species-level networks to be modular, with niche overlap of closely related species within a module and limited overlap between modules (Figure 1C). Given enough time, partner and resource stability leading to speciation via niche differentiation, networks in isolated islands would approach specialized, partitioned networks (Figure 1E).
Interestingly, some of the empirical literature on oceanic island interaction networks suggests very different predictions than those derived from our conceptual model, and provides some intriguing insights into the processes that might dictate deviations from our predictions. In particular, mutualistic species interaction networks on oceanic islands often appear to be nested and sometimes dominated by super-generalist taxa which interact with nearly all potential partners in their community (Olesen et al., 2002; Traveset et al., 2015; Nogales et al., 2016) but see (Trøjelsgaard et al., 2013); this can even occur in cases where other factors like interaction intimacy might otherwise be expected to drive specialization (Hembry et al., 2018). It may be that smaller islands, like the Galápagos, Canary, and Society islands where these studies took place, even if they are isolated, do not provide sufficient area for as much diversification as we describe in our model (Losos and Schluter, 2000; Gillespie et al., 2017). Because relatively less diversification is able to take place, these communities are paused in an intermediate stage in this process of assembly where ecological release is the dominant determinant of interactions. Thus, we tend to observe many generalized and even super-generalized interactions in food webs (Piechnik et al., 2008) and mutualistic networks on oceanic islands (reviewed in Kaiser-Bunbury et al., 2010).
3.2. Metacommunity Dynamics and Gene Flow in Proximate Systems
The examples described so far take place in isolated locations with low rates of colonization and gene flow. At sites with many colonists, however, assembly will have a different trajectory. Metacommunity theory is commonly used to study the assembly of less isolated environments (Leibold et al., 2004), as illustrated through the well-known study of Florida's defaunated mangrove islands (Simberloff, 1974; Piechnik et al., 2008).
Following defaunation, the mangrove islands quickly accumulated species through dispersal. This led to high inter-specific competition, with species that were pre-adapted to the existing conditions prevailing (species sorting and “slotting in", Simberloff and Wilson, 1969; Simberloff, 1974). Because the available abiotic and biotic resources on these islands were limited, the most successful species were generalized (Piechnik et al., 2008). In these proximate islands, dispersal and intra-specific competition are weak filters, while environmental and inter-specific competition are strong ones (Figure 3B). Priority and monopolization effects are less important during assembly in these communities than in more isolated islands, because poorly-adapted early colonizers have insufficient time to adapt or reach sufficient abundances before being out-competed by pre-adapted later colonists (Fukami, 2015; Vanoverbeke et al., 2016). The networks of these early-stage proximate communities will be highly generalized with substantial niche overlap, and thus neither nested nor modular (Figure 1B).
Given the principles of limiting similarity, inter-specific competition should favor niche partitioning among colonizing species, but the lack of isolation of these communities and the presence of constant gene flow makes it less likely that existing species will adapt to local conditions (Vanoverbeke et al., 2016). In addition, the high rate of species turnover—due to high colonization rates by new species and the continual extirpation of residents—leads to unstable resource availability and dilutes the selective pressure of any one species on its partners, ultimately inhibiting the evolution of specialized interactions (Thompson, 2005; Gómez and Zamora, 2006).
However, eventually it is likely that specialist species that can exploit the available resources more efficiently than generalists will colonize from the mainland (i.e., species sorting, Leibold et al., 2004; Piechnik et al., 2008). If specialist species rely on other specialist interaction partners where there is high species turnover, they will likely go extinct. Therefore, specialists that interact with generalist species would be more likely to persist. Combined high niche overlap and asymmetric specialization of intermediate to late stage proximate island communities will generate nested interaction networks (Figure 1D). Eventually, if the environment remains stable, species particularly adapted to the habitat will predominate, increasing intra-specific competition (Figure 3B). Modules, each with a nested structure, will emerge (Figure 1C).
4. Methods for Testing Our Conceptual Model
Using a published dataset of plant-herbivore interaction networks along the chronosequence of the Hawaiian islands (Rominger et al., 2016), we demonstrate a network approach to study community assembly by examining changes in interaction patterns through extended time (Pellissier et al., 2017). We extracted qualitative data on interactions between native herbivorous Hemiptera species and native plants from published species accounts and host-plant association at four focal sites on three islands: Kauai (1 site; 5.1 Ma), Maui (1 site; 0.75 Ma) and Hawaii (2 sites; 0.15 and 0.003 myo), and used these data to assemble species interaction networks. Focal sites had a similar forest composition (dominated by Metrosideros polymorpha; Myrtaceae), elevation (1,100–1,400 m) and rainfall (mean annual precipitation 2,000–3,000 mm). This published dataset represents one of very few empirical studies of interaction networks across an evolutionary timescale (but see, Trøjelsgaard et al., 2013; Hembry et al., 2018), however, these data are very sparse (presence/absence data for interactions at only four sites on three islands). More extensive sampling to capture other stages of assembly are necessary to explore interaction assembly on these islands; however, our intention is to illustrate the possible methods for testing our framework's predictions.
We calculated the niche overlap of plants and herbivores using Jaccard's similarity index (Box 1) and the interaction niche breadth (average degree, Box 1) within each trophic level. Because the networks have different numbers of species and thus different maximum diet breadths, we divided by the total number of possible species with which to interact. We calculated network nestedness using the estimator NODF (Box 1, Almeida-neto et al., 2008). We estimate modularity using a random walk algorithm (Pons and Latapy, 2005; Gabor and Tamas, 2006). We calculated standardized z-scores so that nestedness, modularity, and niche overlap could be compared across communities (Ulrich et al., 2009; Pellissier et al., 2017). To generate z-score values, we first assembled 999 null networks by shuffling the interactions between species while keeping each species' total number of interactions constant (the quasiswap null model in the vegan package, Ulrich et al., 2009; Oksanen et al., 2017).
Overall, the majority of our predictions aligned with network analysis results from these Hawaiian plant-herbivore communities. Plant-herbivore networks tended to be significantly modular and unnested (Fontaine et al., 2011), and modularity increased through time while nestedness decreased (Figure 4). These results corroborate our prediction that over time, evolution/coevolution and in situ speciation will result in increasing modularity and decreased nestedness. Except for the oldest site (Kokee, Kauai), niche overlap of plants and herbivores decreased with substrate age (Figure 4). As expected, niche breadth of herbivores decreased with island age (Figure 4). However, niche breadth peaked at intermediate island ages in plants (Figure 4). Given that our aim is to illustrate how one might test our conceptual model's predictions, we will refrain from speculating further about the reasons certain islands depart from some of our predictions in this example.
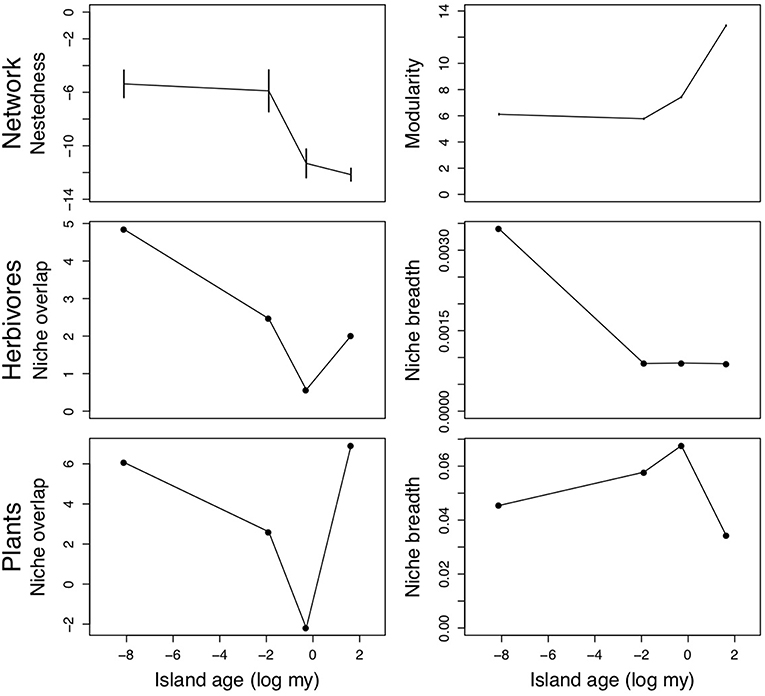
Figure 4. Network nestedness and modularity (z-scores, Top panels), niche overlap and average niche breadth of herbivores (Middle panels) and plants (Bottom panels) along the Hawaiian chronosequence (from youngest to oldest: Kilauea, Hawaii; Kohala, Hawaii; Haleakala, Maui; Kokee, Kauai). Error bars for nestedness and modularity represent the 95% confidence intervals generated by the null community simulation.
5. Conclusion
The role of species interactions in the assembly trajectory of isolated islands, such as Hawaii, and proximate islands, such as mangrove islets, implies that communities will diverge over time, even if the starting conditions are the same, resulting in different network structures. Because patterns of selection imposed by interacting species varies across space, we expect that evolution and coevolution will lead to distinct outcomes in different communities, as predicted by the geographic mosaic theory of coevolution (Thompson, 2005). Both isolated and proximate systems favor interactions that minimize inter- and intra-specific competition and maximize resource use efficiency. However, interactions will differ depending on the rate of species turnover and gene flow, both of which are higher on proximate islands. Our approach will help guide future modeling and empirical studies to improve the understanding of how communities assemble through the lens of species interaction networks.
Author Contributions
LCP, FSV, and RG planned a workshop which the other authors attended to outline this perspective. FSV and KTA designed simulations to help formulate the framework. LCP, PRG, and MPG designed the conceptual figures. LCP wrote the first version of the manuscript and all other authors contributed to revisions.
Conflict of Interest Statement
The authors declare that the research was conducted in the absence of any commercial or financial relationships that could be construed as a potential conflict of interest.
Acknowledgments
We would like to thank Rebecca Brunner for her thoughtful comments on the manuscript, as well as Daniel Gruner, Robert Holt, Helen Morlon, Eric Berlow, Neo Martinez, Elske Tielens, Anna Kuparinen, and Santiago Ramírez for their involvement in the workshop from which this article was originally inspired.
References
Abrams, P. (1983). The theory of limiting similarity. Annu. Rev. Ecol. Evol. Syst. 14, 359–376. doi: 10.1146/annurev.es.14.110183.002043
Ackermann, M., and Doebeli, M. (2004). Evolution of niche width and adaptive diversification. Evolution 58, 2599–2612. doi: 10.1111/j.0014-3820.2004.tb01614.x
Albrecht, M., Riesen, M., and Schmid, B. (2010). Plant–pollinator network assembly along the chronosequence of a glacier foreland. Oikos 119, 1610–1624. doi: 10.1111/j.1600-0706.2010.18376.x
Allhoff, K. T., Ritterskamp, D., Rall, B. C., Drossel, B., and Guill, C. (2015). Evolutionary food web model based on body masses gives realistic networks with permanent species turnover. Sci. Rep. 5:10955. doi: 10.1038/srep10955
Almeida-neto, M., Gumarães, P., Gumarães, P., Loyola, R., and Ulrich, W. (2008). A consistent metric for nestedness analysis in ecological systems: reconciling concept and measurement. Oikos 117, 1227–1239. doi: 10.1111/j.0030-1299.2008.16644.x
Araújo, M. S., Bolnick, D. I., and Layman, C. A. (2011). The ecological causes of individual specialisation. Ecol. Lett. 14, 948–958. doi: 10.1111/j.1461-0248.2011.01662.x
Armbruster, W. S., and Baldwin, B. G. (1998). Switch from specialized to generalized pollination. Nature 394:632. doi: 10.1038/29210
Armbruster, W. S., and Muchhala, N. (2009). Associations between floral specialization and species diversity: cause, effect, or correlation? Evol. Ecol. 23:159. doi: 10.1007/s10682-008-9259-z
Bascompte, J., Jordano, P., Melian, C. J., and Olesen, J. M. (2003). The nested assembly of plant-animal mutualistic networks. Proc. Natl. Acad. Sci. U.S.A. 100, 9383–9387. doi: 10.1073/pnas.1633576100
Bascompte, J., and Stouffer, D. B. (2009). The assembly and disassembly of ecological networks. Philos. Trans. R. Soc. B 364:1781. doi: 10.1098/rstb.2008.0226
Bolnick, D. I. (2001). Intraspecific competition favours niche width expansion in Drosophila melanogaster. Nature 410:463. doi: 10.1038/35068555
Bolnick, D. I. (2004). Can intraspecific competition drive disruptive selection? an experimental test in natural populations of sticklebacks. Evolution 58, 608–618. doi: 10.1111/j.0014-3820.2004.tb01683.x
Bolnick, D. I., Ingram, T., Stutz, W. E., Snowberg, L. K., Lau, O. L., and Paull, J. S. (2010). Ecological release from interspecific competition leads to decoupled changes in population and individual niche width. Proc. R. Soc. Lond. B Biol. Sci. 277, 1789–1797. doi: 10.1098/rspb.2010.0018
Bolnick, D. I., and Lau, O. L. (2008). Predictable patterns of disruptive selection in stickleback in postglacial lakes. Am. Nat. 172, 1–11. doi: 10.1086/587805
Bolnick, D. I., Svanbäck, R., Fordyce, J. A., Yang, L. H., Davis, J. M., Hulsey, C. D., et al. (2002). The ecology of individuals: incidence and implications of individual specialization. Am. Nat. 161, 1–28. doi: 10.1086/343878
Brännström, Å., Johansson, J., Loeuille, N., Kristensen, N., Troost, T. A., Lambers, R. H. R., et al. (2012). Modelling the ecology and evolution of communities: a review of past achievements, current efforts, and future promises. Evol. Ecol. Res. 14, 601–625.
Brännström, Å., Loeuille, N., Loreau, M., and Dieckmann, U. (2011). Emergence and maintenance of biodiversity in an evolutionary food-web model. Theor. Ecol. 4, 467–478. doi: 10.1007/s12080-010-0089-6
Brodie, J. F. (2017). Evolutionary cascades induced by large frugivores. Proc. Natl. Acad. Sci. U.S.A. 114, 11998–12002. doi: 10.1073/pnas.1710172114
Brosi, B. J. (2016). Pollinator specialization: from the individual to the community. New Phytol. 210, 1190–1194. doi: 10.1111/nph.13951
Brosi, B. J., and Briggs, H. M. (2013). Single pollinator species losses reduce floral fidelity and plant reproductive function. Proc. Natl. Acad. Sci. U.S.A. 110, 13044–13048. doi: 10.1073/pnas.1307438110
Cadotte, M. W., and Tucker, C. M. (2017). Should environmental filtering be abandoned? Trends Ecol. Evol. 32, 429–437. doi: 10.1016/j.tree.2017.03.004
Chase, J. M. (2003). Community assembly: when should history matter? Oecologia 136, 489–498. doi: 10.1007/s00442-003-1311-7
Chase, J. M., and Leibold, M. A. (2003). Ecological Niches: Linking Classical and Contemporary Approaches, Chicago, IL: University of Chicago Press.
Chesson, P. (2000). Mechanisms of maintenance of species diversity. Annu. Rev. Ecol. Evol. Syst. 31, 343–366. doi: 10.1146/annurev.ecolsys.31.1.343
Cody, M. L., and Diamond, J. M. (1975). Ecology and Evolution of Communities, Cambridge, MA: Harvard University Press.
Cohen, J. E., and Stephens, D. W. (1978). Food Webs and Niche Space. Princeton, NJ: Princeton University Press.
Coux, C., Rader, R., Bartomeus, I., and Tylianakis, J. M. (2016). Linking species functional roles to their network roles. Ecol. Lett. 19, 762–770. doi: 10.1111/ele.12612
Day, T., and Young, K. A. (2004). Competitive and facilitative evolutionary diversification. AIBS Bull. 54, 101–109. doi: 10.1641/0006-3568(2004)054[0101:CAFED]2.0.CO;2
de Andreazzi, C. S., Guimarães, P. R. Jr., and Melián, C. J. (2018). Eco-evolutionary feedbacks promote fluctuating selection and long-term stability of antagonistic networks. Proc. R. Soc. B 285:20172596. doi: 10.1098/rspb.2017.2596
De Meester, L., and Pantel, J. (2014). Eco-evolutionary dynamics in freshwater systems. J. Limn. 73, 193–200. doi: 10.4081/jlimnol.2014.831
Donatti, C. I., Guimarães, P. R. Jr., Galetti, M., Pizo, M. A., Marquitti, F., and Dirzo, R. (2011). Analysis of a hyper-diverse seed dispersal network: modularity and underlying mechanisms. Ecol. Lett. 14, 773–781. doi: 10.1111/j.1461-0248.2011.01639.x
Drake, J. A. (1990). Communities as assembled structures: do rules govern pattern? Trends Ecol. Evol. 5, 159–164. doi: 10.1016/0169-5347(90)90223-Z
Ehrlich, P. R., and Raven, P. H. (1964). Butterflies and plants: a study in coevolution. Evolution 18, 586–608. doi: 10.1111/j.1558-5646.1964.tb01674.x
Encinas-Viso, F., Revilla, T. A., and Etienne, R. S. (2012). Phenology drives mutualistic network structure and diversity. Ecol. Lett. 15, 198–208. doi: 10.1111/j.1461-0248.2011.01726.x
Fahimipour, A. K., and Hein, A. M. (2014). The dynamics of assembling food webs. Ecol. Lett. 17, 606–613. doi: 10.1111/ele.12264
Fontaine, C., Guimarães, P. R. Jr., Kéfi, S., Loeuille, N., Memmott, J., Van Der Putten, W. H., et al. (2011). The ecological and evolutionary implications of merging different types of networks. Ecol. Lett. 14, 1170–1181. doi: 10.1111/j.1461-0248.2011.01688.x
Fontaine, C., Thébault, E., and Dajoz, I. (2009). Are insect pollinators more generalist than insect herbivores? Proc. R. Soc. Lond. B Biol. Sci. 276, 3027–3033. doi: 10.1098/rspb.2009.0635
Fortunato, S. (2010). Community detection in graphs. Phys. Rep. 486, 75–174. doi: 10.1016/j.physrep.2009.11.002
Freilich, M. A., Wieters, E., Broitman, B. R., Marquet, P. A., and Navarrete, S. A. (2018). Species co-occurrence networks: can they reveal trophic and non-trophic interactions in ecological communities? Ecology 99, 690–699. doi: 10.1002/ecy.2142
Fritschie, K. J., Cardinale, B. J., Alexandrou, M. A., and Oakley, T. H. (2014). Evolutionary history and the strength of species interactions: testing the phylogenetic limiting similarity hypothesis. Ecology 95, 1407–1417. doi: 10.1890/13-0986.1
Fründ, J., Dormann, C. F., Holzschuh, A., and Tscharntke, T. (2013). Bee diversity effects on pollination depend on functional complementarity and niche shifts. Ecology 94, 2042–2054. doi: 10.1890/12-1620.1
Fukami, T. (2015). Historical contingency in community assembly: integrating niches, species pools, and priority effects. Annu. Rev. Ecol. Evol. Syst. 46, 1–23. doi: 10.1146/annurev-ecolsys-110411-160340
Fussmann, G., Loreau, M., and Abrams, P. (2007). Eco-evolutionary dynamics of communities and ecosystems. Funct. Ecol. 21, 465–477. doi: 10.1111/j.1365-2435.2007.01275.x
Gabor, C., and Tamas, N. (2006). The igraph software package for complex network research. InterJournal Complex Syst. 1695. Available online at: http://igraph.org
Gause, G. (1936). The struggle for existence. Soil Sci. 41:159. doi: 10.1097/00010694-193602000-00018
Gause, G. F. (1932). Experimental studies on the struggle for existence: I. Mixed population of two species of yeast. J. Exp. Biol. 9, 389–402.
Gillespie, R. (2004). Community assembly through adaptive radiation in hawaiian spiders. Science 303, 356–359. doi: 10.1126/science.1091875
Gillespie, R., Brewer, M., and Roderick, G. (2017). Ancient biogeography of generalist predators on remote oceanic islands. J. Biogeogr. 44, 1098–1109. doi: 10.1111/jbi.12967
Gillespie, R. G., and Roderick, G. K. (2002). Arthropods on islands: colonization, speciation, and conservation. Annu. Rev. Entomol. 47, 595–632. doi: 10.1146/annurev.ento.47.091201.145244
Gómez, J. M., and Zamora, R. (2006). “Ecological factors that promote the evolution of generalization in pollination systems,” in Plant-Pollinator Interactions, eds N. Waser and J. Ollerton (Chicago, IL: The University of Chicago Press), 145–166.
Grant, P. R. (1999). Ecology and Evolution of Darwin's Finches. Princeton, NJ: Princeton University Press.
Grant, P. R., and Grant, B. R. (2006). Evolution of character displacement in Darwin's finches. Science 313, 224–226. doi: 10.1126/science.1128374
Gravel, D., Massol, F., Canard, E., Mouillot, D., and Mouquet, N. (2011). Trophic theory of island biogeography. Ecol. Lett. 14, 1010–1016. doi: 10.1111/j.1461-0248.2011.01667.x
Guerrero, A. M., and Tye, A. (2009). Darwin's finches as seed predators and dispersers. Wilson J. Ornithol. 121, 752–764. doi: 10.1676/09-035.1
Guimarães, P. Jr., Rico-Gray, V., Oliveira, P., Izzo, T., dos Reis, S., and Thompson, J. (2007). Interaction intimacy affects structure and coevolutionary dynamics in mutualistic networks. Curr. Biol. 17, 1797–1803. doi: 10.1016/j.cub.2007.09.059
Hawkins, B. A. (2005). Pattern and Process in Host-Parasitoid Interactions, Cambridge: University Press.
Hembry, D. H., Raimundo, R. L. G., Newman, E. A., Atkinson, L., Guo, C., Guimares, P. R., et al. (2018). Does biological intimacy shape ecological network structure? A test using a brood pollination mutualism on continental and oceanic islands. J. Anim. Ecol. 87, 1160–1171. doi: 10.1111/1365-2656.12841
Hembry, D. H., Yoder, J. B., and Goodman, K. R. (2014). Coevolution and the diversification of life. Am. Nat. 184, 425–438. doi: 10.1086/677928
Herrera, C. M. (1988). Variation in mutualisms: the spatiotemporal mosaic of a pollinator assemblage. Biol. J. Linnean Soc. 35, 95–125. doi: 10.1111/j.1095-8312.1988.tb00461.x
Herrera, C. M. (1996). “Floral traits and plant adaptation to insect pollinators: a devil's advocate approach,” in Floral Biology, eds D. G. Lloyd and S. C. H. Barrett (New York, NY: Springer), 65–87.
HilleRisLambers, J., Adler, P., Harpole, W., Levine, J., and Mayfield, M. (2012). Rethinking community assembly through the lens of coexistence theory. Annu. Rev. Ecol. Evol. Syst. 43, 227–248. doi: 10.1146/annurev-ecolsys-110411-160411
Holt, R. D. (1977). Predation, apparent competition, and the structure of prey communities. Theor. Popul. Biol. 12, 197–229. doi: 10.1016/0040-5809(77)90042-9
Holt, R. D., Lawton, J. H., Polis, G. A., and Martinez, N. D. (1999). Trophic rank and the species–area relationship. Ecology 80, 1495–1504.
Hutchinson, G. E. (1959). Homage to santa rosalia or why are there so many kinds of animals? Am. Nat. 93, 145–159. doi: 10.1086/282070
Hutchinson, M. (1957). “Concluding remarks,” in Cold Spring Harbour Symposia on Quantitative Biology: Population Studies: Animal Ecology and Demography (Cold Spring Harbor, NY: Cold Spring Harbor Laboratory), 415–427.
Ives, A. R., and Godfray, C. J. (2006). Phylogenetic analysis of trophic associations. Am. Nat. 168, E1–E14. doi: 10.1086/505157
Jordano, P. (1995). Angiosperm fleshy fruits and seed dispersers: a comparative analysis of adaptation and constraints in plant-animal interactions. Am. Nat. 145, 163–191. doi: 10.1086/285735
Jordano, P., Bascompte, J., and Olesen, J. M. (2003). Invariant properties in coevolutionary networks of plant-animal interactions. Ecol. Lett. 6, 69–81. doi: 10.1046/j.1461-0248.2003.00403.x
Kaiser-Bunbury, C. N., Traveset, A., and Hansen, D. M. (2010). Conservation and restoration of plant–animal mutualisms on oceanic islands. Perspect. Plant Ecol. Syst. 12, 131–143. doi: 10.1016/j.ppees.2009.10.002
Kartzinel, T. R., Chen, P. A., Coverdale, T. C., Erickson, D. L., Kress, W. J., Kuzmina, M. L., et al. (2015). Dna metabarcoding illuminates dietary niche partitioning by african large herbivores. Proc. Natl. Acad. Sci. U.S.A. 2015, 8019–8024. doi: 10.1073/pnas.1503283112
Knelman, J. E., and Nemergut, D. R. (2014). Changes in community assembly may shift the relationship between biodiversity and ecosystem function. Front. Microbiol. 5:424. doi: 10.3389/fmicb.2014.00424
Leibold, M. A., Holyoak, M., Mouquet, N., Amarasekare, P., Chase, J. M., Hoopes, M. F., et al. (2004). The metacommunity concept: a framework for multi-scale community ecology. Ecol. Lett. 7, 601–613. doi: 10.1111/j.1461-0248.2004.00608.x
Leigh, E. G. (1975). “Population fluctuations, community stability, and environmental variability,” in Ecology and Evolution of Communities, eds M. L. Cody, and J. M. Diamond (Cambridge, MA: Harvard University Press), 51–73.
Lerner, H. R., Meyer, M., James, H. F., Hofreiter, M., and Fleischer, R. C. (2011). Multilocus resolution of phylogeny and timescale in the extant adaptive radiation of hawaiian honeycreepers. Curr. Biol. 21, 1838–1844.
Levin, D. A. (1981). Dispersal versus gene flow in plants. Ann. Missouri Bot. Gard. 68, 233–253. doi: 10.2307/2398797
Loeuille, N., and Leibold, M. A. (2008). Evolution in metacommunities: on the relative importance of species sorting and monopolization in structuring communities. Am. Nat. 171, 788–799. doi: 10.1086/587745
Loeuille, N., and Loreau, M. (2005). Evolutionary emergence of size-structured food webs. Proc. Natl. Acad. Sci. U.S.A. 102, 5761–5766. doi: 10.1073/pnas.0408424102
Losos, J. B., and Schluter, D. (2000). Analysis of an evolutionary species–area relationship. Nature 408:847. doi: 10.1038/35048558
Macarthur, R., and Levins, R. (1967). The limiting similarity, convergence, and divergence of coexisting species. Am. Nat. 101, 377–385. doi: 10.1086/282505
MacArthur, R. H. (1984). Geographical Ecology: Patterns in the Distribution of Species, Princeton, NJ: Princeton University Press.
MacArthur, R. H., and Wilson, E. O. (1967). Theory of Island Biogeography. (MPB-1), Vol. 1. Princeton, NJ: Princeton University Press.
Maherali, H., and Klironomos, J. N. (2007). Influence of phylogeny on fungal community assembly and ecosystem functioning. Science 316, 1746–1748. doi: 10.1126/science.1143082
Mittelbach, G. G., and Schemske, D. W. (2015). Ecological and evolutionary perspectives on community assembly. Trends Ecol. Evol. 30, 241–247. doi: 10.1016/j.tree.2015.02.008
Montoya, J. M., Pimm, S. L., and Solé, R. V. (2006). Ecological networks and their fragility. Nature 442, 259–264. doi: 10.1038/nature04927
Morlon, H., Kefi, S., and Martinez, N. D. (2014). Effects of trophic similarity on community composition. Ecol. Lett. 17, 1495–1506. doi: 10.1111/ele.12356
Morton, R. D., Law, R., Pimm, S. L., and Drake, J. A. (1996). On models for assembling ecological communities. Oikos 75, 493–499. doi: 10.2307/3545891
Newman, M. E., and Girvan, M. (2004). Finding and evaluating community structure in networks. Phys. Rev. E 69:026113. doi: 10.1103/PhysRevE.69.026113
Nobre, T., Eggleton, P., and Aanen, D. (2010). Vertical transmission as the key to the colonization of madagascar by fungus-growing termites? Proc. R. Soc. Lond. B Biol. Sci. 277, 359–365. doi: 10.1098/rspb.2009.1373
Nogales, M., Heleno, R., Rumeu, B., González-Castro, A., Traveset, A., Vargas, P., et al. (2016). Seed-dispersal networks on theCanaries and the Galápagos archipelagos: interaction modules as biogeographical entities. Global Ecol. Biogeogr. 25, 912–922. doi: 10.1111/geb.12315
Oksanen, J., Blanchet, F. G., Friendly, M., Kindt, R., Legendre, P., McGlinn, D., et al. (2017). vegan: Community Ecology Package. R package version 2.4-4
Olesen, J., Bascompte, J., Dupont, Y., and Jordano, P. (2007). The modularity of pollination networks. Proc. Natl Acad. Sci. U.S.A. 104, 19891–19896. doi: 10.1073/pnas.0706375104
Olesen, J., Bascompte, J., Elberling, H., and Jordano, P. (2008). Temporal dynamics in a pollination network. Ecology 89:1573. doi: 10.1890/07-0451.1
Olesen, J. M., Dupont, Y. L., O'Gorman, E., Ings, T. C., Layer, K., Melián, C. J., et al. (2010). “From broadstone to Zackenberg: space, time and hierarchies in ecological networks,” in Ecological Networks, Vol. 42, Advances in Ecological Research, ed G. Woodward (Academic Press), 1–69. doi: 10.1016/B978-0-12-381363-3.00001-0
Olesen, J. M., Eskildsen, L. I., and Venkatasamy, S. (2002). Invasion of pollination networks on oceanic islands: importance of invader complexes and endemic super generalists. Divers. Distrib. 8, 181–192. doi: 10.1046/j.1472-4642.2002.00148.x
Olesen, J. M., and Valido, A. (2003). Lizards as pollinators and seed dispersers: an island phenomenon. Trends Ecol. Evol. 18, 177–181. doi: 10.1016/S0169-5347(03)00004-1
Parrish, J., and Bazzaz, F. (1979). Difference in pollination niche relationships in early and late successional plant communities. Ecology 60, 597–610. doi: 10.2307/1936080
Pellissier, L., Albouy, C., Bascompte, J., Farwig, N., Graham, C., Loreau, M., et al. (2017). Comparing species interaction networks along environmental gradients. Biol. Rev. 93, 785–800. doi: 10.1111/brv.12366
Petchey, O. L., and Gaston, K. J. (2006). Functional diversity: back to basics and looking forward. Ecol. Lett. 9, 741–758. doi: 10.1111/j.1461-0248.2006.00924.x
Piechnik, D. A., Lawler, S. P., and Martinez, N. D. (2008). Food-web assembly during a classic biogeographic study: speciestrophic breadth corresponds to colonization order. Oikos 117, 665–674. doi: 10.1111/j.0030-1299.2008.15915.x
Pigot, A. L., Bregman, T., Sheard, C., Daly, B., Etienne, R. S., and Tobias, J. A. (2016). Quantifying species contributions to ecosystem processes: a global assessment of functional trait and phylogenetic metrics across avian seed-dispersal networks. Proc. R. Soc. B 283:20161597. doi: 10.1098/rspb.2016.1597
Pillai, P., Gonzalez, A., and Loreau, M. (2011). Metacommunity theory explains the emergence of food web complexity. Proc. Natl Acad. Sci. U.S.A. 108, 19293–19298. doi: 10.1073/pnas.1106235108
Pimm, S. L. (1979). The structure of food webs. Theor. Popul. Biol. 16, 144–158. doi: 10.1016/0040-5809(79)90010-8
Ponisio, L. C., Gaiarsa, M. P., and Kremen, C. (2017). Opportunistic attachment assembles plant–pollinator networks. Ecol. Lett. 20, 1261–1272. doi: 10.1111/ele.12821
Pons, P., and Latapy, M. (2005). “Computing communities in large networks using random walks,” in Computer and Information Sciences-ISCIS 2005. ISCIS 2005, Lecture Notes in Computer Science, eds G.T. Yolum, F. Gürgen, and C. Özturan (Berlin, Heidelberg: Springer). doi: 10.1007/11569596_31
Pornon, A., Escaravage, N., Burrus, M., Holota, H., Khimoun, A., Mariette, J., et al. (2016). Using metabarcoding to reveal and quantify plant-pollinator interactions. Sci. Rep. 6:27282. doi: 10.1038/srep27282
Post, D. M., and Palkovacs, E. P. (2009). Eco-evolutionary feedbacks in community and ecosystem ecology: interactions between the ecological theatre and the evolutionary play. Philos. Trans. R. Soc. B 364, 1629–1640. doi: 10.1098/rstb.2009.0012
Post, W., and Pimm, S. (1983). Community assembly and food web stability. Math. Biosci. 64, 169–192. doi: 10.1016/0025-5564(83)90002-0
Rainey, P. B., and Travisano, M. (1998). Adaptive radiation in a heterogeneous environment. Nature 394, 69–72. doi: 10.1038/27900
Rezende, E. L., Lavabre, J. E., Guimarães, P. R. Jr., Jordano, P., and Bascompte, J. (2007). Non-random coextinctions in phylogenetically structured mutualistic networks. Nature 448, 925–928. doi: 10.1038/nature05956
Ricklefs, R. E., and Bermingham, E. (2002). The concept of the taxon cycle in biogeography. Global Ecol. Biogeogr. 11, 353–361. doi: 10.1046/j.1466-822x.2002.00300.x
Rominger, A., Goodman, K., Lim, J., Armstrong, E., Becking, L., Bennett, G., et al. (2016). Community assembly on isolated islands: macroecology meets evolution. Global Ecol. Biogeogr. 25, 769–780. doi: 10.1111/geb.12341
Rosenzweig, M. L. (1978). Competitive speciation. Biol. J. Linnean Soc. 10, 275–289. doi: 10.1111/j.1095-8312.1978.tb00016.x
Schleuning, M., Fründ, J., and García, D. (2015). Predicting ecosystem functions from biodiversity and mutualistic networks: an extension of trait-based concepts to plant–animal interactions. Ecography 38, 380–392. doi: 10.1111/ecog.00983
Shaw, K. L., and Gillespie, R. G. (2016). Comparative phylogeography of oceanic archipelagos: hotspots for inferences of evolutionary process. Proc. Natl. Acad. Sci. U.S.A. 113, 7986–7993. doi: 10.1073/pnas.1601078113
Simberloff, D. S. (1974). Equilibrium theory of island biogeography and ecology. Annu. Rev. Ecol. Evol. Syst. 5, 161–182. doi: 10.1146/annurev.es.05.110174.001113
Simberloff, D. S., and Wilson, E. O. (1969). Experimental zoogeography of islands: the colonization of empty islands. Ecology 50, 278–296. doi: 10.2307/1934856
Stouffer, D. B., and Bascompte, J. (2011). Compartmentalization increases food-web persistence. Proc. Natl. Acad. Sci. U.S.A. 108, 3648–3652. doi: 10.1073/pnas.1014353108
Strauss, S. Y., Siemens, D. H., Decher, M. B., and Mitchell-Olds, T. (1999). Ecological costs of plant resistance to herbivores in the currency of pollination. Evolution 53, 1105–1113. doi: 10.1111/j.1558-5646.1999.tb04525.x
Svanbäck, R., and Bolnick, D. I. (2007). Intraspecific competition drives increased resource use diversity within a natural population. Proc. R. Soc. Lond. B Biol. Sci. 274, 839–844. doi: 10.1098/rspb.2006.0198
Thébault, E., and Fontaine, C. (2010). Stability of ecological communities and the architecture of mutualistic and trophic networks. Science 329, 853–856. doi: 10.1126/science.1188321
Thompson, J. (2005). The Geographic Mosaic of Coevolution. Chicago, IL: University of Chicago Press.
Tilman, D. (2004). Niche tradeoffs, neutrality, and community structure: a stochastic theory of resource competition, invasion, and community assembly. Proc. Natl. Acad. Sci. U.S.A. 101, 10854–10861. doi: 10.1073/pnas.0403458101
Traveset, A., Olesen, J. M., Nogales, M., Vargas, P., Jaramillo, P., Antolín, E., et al. (2015). Bird–flower visitation networks in the Galápagos unveil a widespread interaction release. Nat. Commun. 6:6376. doi: 10.1038/ncomms7376
Travisano, M., and Rainey, P. B. (2000). Studies of adaptive radiation using model microbial systems. Am. Nat. 156, S35–S44. doi: 10.1086/303414
Trøjelsgaard, K., Báez, M., Espadaler, X., Nogales, M., Oromí, P., Roche, F. L., et al. (2013). Island biogeography of mutualistic interaction networks. J. Biogeogr. 40, 2020–2031. doi: 10.1111/jbi.12165
Tylianakis, J. M., Martínez-García, L. B., Richardson, S. J., Peltzer, D. A., and Dickie, I. A. (2018). Symmetric assembly and disassembly processes in an ecological network. Ecol. Lett. 21, 896–904. doi: 10.1111/ele.12957
Ulrich, W., Almeida-Neto, M., and Gotelli, N. J. (2009). A consumer's guide to nestedness analysis. Oikos 118, 3–17. doi: 10.1111/j.1600-0706.2008.17053.x
Urban, M. C., Leibold, M. A., Amarasekare, P., De Meester, L., Gomulkiewicz, R., Hochberg, M. E., et al. (2008). The evolutionary ecology of metacommunities. Trends Ecol. Evol. 23, 311–317. doi: 10.1016/j.tree.2008.02.007
Valdovinos, F. S., Brosi, B. J., Briggs, H. M., Moisset de Espanés, P., Ramos-Jiliberto, R., and Martinez, N. D. (2016). Niche partitioning due to adaptive foraging reverses effects of nestedness and connectance on pollination network stability. Ecol. Lett. 19, 1277–1286. doi: 10.1111/ele.12664
Vanoverbeke, J., Urban, M. C., and De Meester, L. (2016). Community assembly is a race between immigration and adaptation: eco-evolutionary interactions across spatial scales. Ecography 39, 858–870. doi: 10.1111/ecog.01394
Vázquez, D. P., Chacoff, N. P., and Cagnolo, L. (2009). Evaluating multiple determinants of the structure of plant-animal mutualistic networks. Ecology 90, 2039–2046. doi: 10.1890/08-1837.1
Vázquez, D. P., Melián, C. J., Williams, N. M., Blüthgen, N., Krasnov, B. R., and Poulin, R. (2007). Species abundance and asymmetric interaction strength in ecological networks. Oikos 116, 1120–1127. doi: 10.1111/j.0030-1299.2007.15828.x
Vermeij, G. J. (1994). The evolutionary interaction among species: selection, escalation, and coevolution. Annu. Rev. Ecol. Evol. Syst. 25, 219–236. doi: 10.1146/annurev.es.25.110194.001251
Wallace, A. R. (1891). Natural Selection and Tropical Nature: Essays on Descriptive and Theoretical Biology. London: Macmillan and Company.
Waser, N. M., Chittka, L., Price, M. V., Williams, N. M., and Ollerton, J. (1996). Generalization in pollination systems, and why it matters. Ecology 77, 1043–1060. doi: 10.2307/2265575
Werner, T. K., and Sherry, T. W. (1987). Behavioral feeding specialization in pinaroloxias inornata, the darwin's finch of cocos island, costa rica. Proc. Natl. Acad. Sci. U.S.A. 84, 5506–5510. doi: 10.1073/pnas.84.15.5506
Wilson, E. O. (1961). The nature of the taxon cycle in the melanesian ant fauna. Am. Nat. 95, 169–193. doi: 10.1086/282174
Yeakel, J. D., Pires, M. M., Rudolf, L., Dominy, N. J., Koch, P. L., Guimarães, P. R. Jr., et al. (2014). Collapse of an ecological network in ancient egypt. Proc. Natl. Acad. Sci. U.S.A. 111, 14472–14477. doi: 10.1073/pnas.1408471111
Yeaton, R. I. (1974). An ecological analysis of chaparral and pine forest bird communities on santa cruz island and mainland california. Ecology 55, 959–973. doi: 10.2307/1940348
Keywords: evolution, coevolution, succession, turnover, island biogeography, network
Citation: Ponisio LC, Valdovinos FS, Allhoff KT, Gaiarsa MP, Barner A, Guimarães PR Jr, Hembry DH, Morrison B and Gillespie R (2019) A Network Perspective for Community Assembly. Front. Ecol. Evol. 7:103. doi: 10.3389/fevo.2019.00103
Received: 24 November 2018; Accepted: 14 March 2019;
Published: 09 April 2019.
Edited by:
Marco A. Molina-Montenegro, University of Talca, ChileReviewed by:
Pablo A. Marquet, Pontificia Universidad Catlica de Chile, ChileJianjun Wang, Nanjing Institute of Geography and Limnology (CAS), China
Copyright © 2019 Ponisio, Valdovinos, Allhoff, Gaiarsa, Barner, Guimarães, Hembry, Morrison and Gillespie. This is an open-access article distributed under the terms of the Creative Commons Attribution License (CC BY). The use, distribution or reproduction in other forums is permitted, provided the original author(s) and the copyright owner(s) are credited and that the original publication in this journal is cited, in accordance with accepted academic practice. No use, distribution or reproduction is permitted which does not comply with these terms.
*Correspondence: Lauren C. Ponisio, lponisio@gmail.com