- 1Department of Ecology and Evolutionary Biology, University of California, Los Angeles, Los Angeles, CA, United States
- 2Department of Biomathematics, David Geffen School of Medicine, University of California, Los Angeles, Los Angeles, CA, United States
- 3Santa Fe Institute, Santa Fe, NM, United States
Interactions are ubiquitous and have been extensively studied in many ecological, evolutionary, and physiological systems. A variety of measures—ANOVA, covariance, epistatic additivity, mutual information, joint cumulants, Bliss independence—exist that compute interactions across fields. However, these are not discussed and derived within a single, general framework. This missing framework likely contributes to the confusion about proper formulations and interpretations of higher-order interactions. Intriguingly, despite higher-order interactions having received little attention, they have been recently discovered to be highly prevalent and to likely impact the dynamics of complex biological systems. Here, we introduce a single, explicit mathematical framework that simultaneously encompasses all of these measures of pairwise interactions. The generality and simplicity of this framework allows us to establish a rigorous method for deriving higher-order interaction measures based on any of the pairwise interactions listed above. These generalized higher-order interaction measures enable the exploration of emergent phenomena across systems such as multiple predator effects, gene epistasis, and environmental stressors. These results provide a mechanistic basis to better account for how interactions affect biological systems. Our theoretical advance provides a foundation for understanding multi-component interactions in complex systems such as evolving populations within ecosystems or communities.
Introduction
Because of their key role in understanding the dynamics of complex biological, physical, and social systems, there is a long and rich history of studying interactions and their consequences (Wootton, 1993; Billick and Case, 1994; Darling and Côté, 2008; Mihaila et al., 2010; Hamilton, 2011; Toprak et al., 2013; Barrios-O'neill et al., 2014; Foucquier and Guedj, 2015; Palmer et al., 2015; Podgornaia and Laub, 2015; Nishikawa and Motter, 2016; Shi, 2016). These approaches have often been complemented and enhanced by network theory that has led to important advances in prediction of patterns (Segrè et al., 2005; Yeh et al., 2006; Braun and Shah, 2015). Studies of interactions and networks have heavily and almost solely focused on two-component interactions. As a result, we have a thorough understanding of pairwise interactions and the various interaction categorization measures defined across distinct subject areas such as statistical physics, gene networks, and prey-predator systems. Yet despite this focus on interactions at the pairwise level, a general and comprehensive framework for two-way interaction categorizations has not been established. Moreover, this lack of a framework makes it particularly challenging to address and incorporate higher-order interactions that must be scaled up from any specific pairwise interaction model. This also likely contributes to the paucity of studies or higher-order interactions—defined here as interactions among three or more components—in the literature. Indeed, many studies have hypothesized or either implicitly or explicitly assumed that higher-order interactions are extremely rare and/or insignificant (May, 1972; Van Belle, 2011; Wang et al., 2015; Wootton and Stouffer, 2016). In contrast, recent studies have provided evidence that there is a large amount of higher-order interactions, suggesting a critical need to include higher-order effects to better understand complex systems and alterations of ecosystem processes (Weinreich et al., 2013; Taylor and Ehrenreich, 2015a,b; Beppler et al., 2016; Tekin et al., 2016; Levine et al., 2017; Mayfield and Stouffer, 2017).
Constructing an integrated theoretical framework that encapsulates all the pairwise interaction measures and rigorously constructs higher-order interaction measures by building up from a generalized formula for pairwise interactions would be extremely valuable for gaining insights into understanding complex systems. Here, we provide a perspicuous path to this general theory of interaction measures at pairwise and higher-order levels. We aim consequently to enhance the understanding of interactions via the comparison of different interaction measures and via the search for higher-order interactions, potentially providing profound insights into complex systems research.
In Table 1 we give several of the most common choices for interaction measures that have been introduced and utilized in diverse fields. Diverse examples of interaction measures include (i) covariance for calculating the joint variability of multiple random variables (Rice, 2003), (ii) mutual information (MI) defined to quantify the information gained about one random variable through knowledge of the other random variable (Cover and Thomas, 2012), (iii) joint cumulants in statistical physics, also known as an n-point correlation or Ursell functions in quantum field theory (Kendall and Stuart, 1969), (iv) statistical measures of analysis of variance (ANOVA) for detecting the differences of means and variances among different groups (Cohen, 2008), (v) additive and multiplicative models introduced for quantifying the interactions among multiple predators in their ability to affect the survival of a prey population (Figure 1) (Sih et al., 1998), and (vi) a commonly used measure of Bliss or epistatic Independence for identification of drug-drug and gene-gene interactions (Bliss, 1939). Although these measures are all grounded on the unifying objective of quantifying the dependence or interaction between different components (referred to as objects, variables, or factors), a general theory for deriving these measures does not exist. Indeed, some of the interaction measures mentioned above are strongly associated with each other (see Similarities across different interaction measures), hence introduction of a general framework of interactions becomes much more needed.
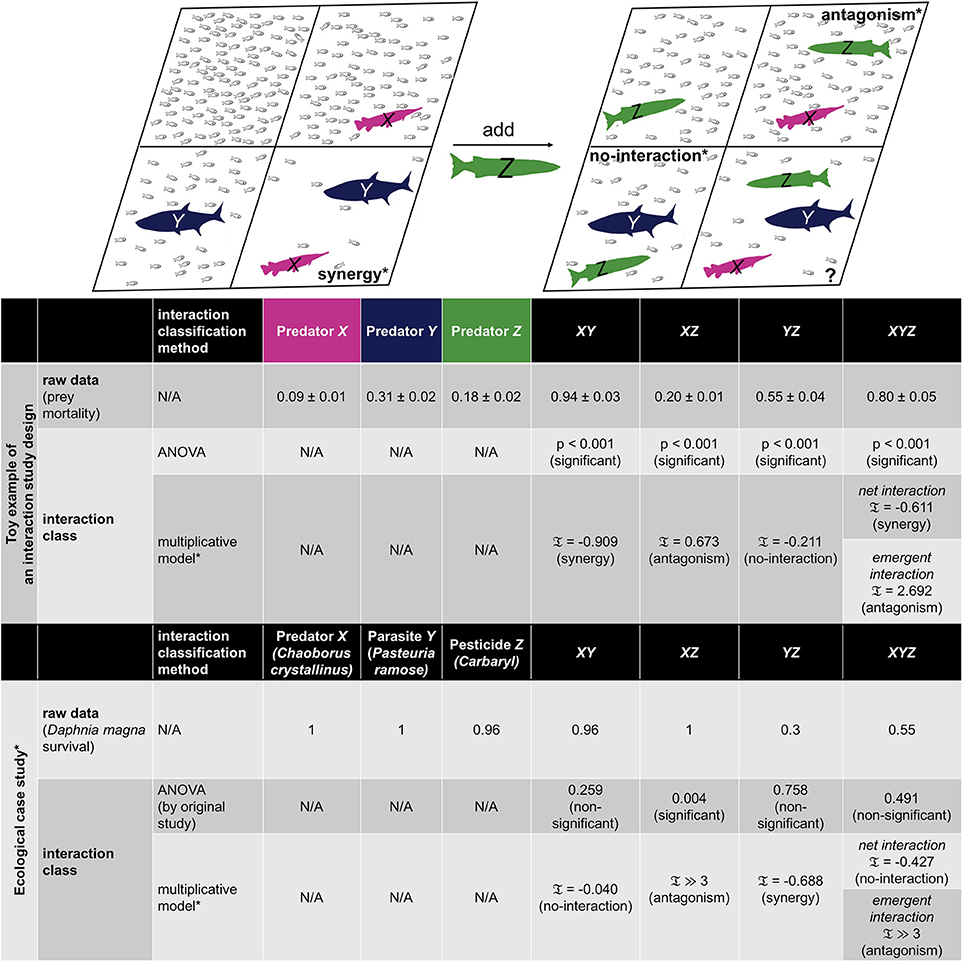
Figure 1. Example representation and ecological case study of 2-way and 3-way interactions for consumer-resource systems. A toy example of an interaction study design for multiple predator effects (MPE) is given to represent 2-way and 3-way interactions, where mortality rates of prey population (small fish) are dependent on the consuming ability of certain predator or combination of predators. Schematic of no predator, single predator, pairwise combination, and triple combination of fish predator populations are illustrated in 8 different panels, where the pairwise panels of XY, XZ, YZ are chosen to represent the three distinct types of interactions: synergy, antagonism, and no-interaction by the multiplicative model with the rescaling introduced in Segrè et al. (2005) and Tekin et al. (2016). In particular, synergistic interaction corresponds to the case when the mortality rate with two predators is sufficiently larger than the expected mortality rate of predators based on single-predator effects. Conversely, predators can act antagonistically, meaning that the mortality rate in the presence of combined predators is sufficiently less than the expected mortality rate based on single-predator effects. In the latter scenario, predators do not interact, hence expected prey mortality is the same as actual prey mortality with both predators in the environment. Classification of interactions is typically done by evaluating the effects of the combined components relative to the effects of independent single components or lower-order combination effects. Given the raw data (see table), we employ the analysis of variance (ANOVA) as well as multiplicative model of interactions and present p-values and interaction measure calculations (𝔗, see section Methods for the definition), respectively. For 3-way combinations, two distinct types of interactions are characterized by the multiplicative model, where net interaction represents the overall effect and emergent interaction represents the three-way combination effect that is beyond any pairwise interactions. The same interaction study design has been performed for an ecological case study by Coors and De Meester (2008), where stressors are a predator (X), parasite infection (Y), and pesticide exposure by carbanyl (Z) on a water flea Daphnia magna survival. A MATLAB code package that has the toy data with the implementation of the interaction measure calculations are included as part of our paper (see Data Sheet 1).
Identifying higher-order interactions is essential because the behavior of complex systems can be unpredictable due to interactions among the pairwise subsets of system components (Case and Bender, 1981; Billick and Case, 1994). However, generalizing 2-way (pairwise) interaction measures to three or more component systems is often challenging, often done incorrectly or incompletely, and requires a heuristic process of learning the complex calculations or directly implementing software packages with implicit choices and computations. Consequently, measures for higher-order interactions are often not known or not well-defined (Tekin et al., 2017). All in all, a simplified yet rigorous approach for studying pairwise and higher-order interactions is vitally important for creating a basis to explore the patterns and consequences of emergent phenomena in a wide range of systems.
One important point to consider is that proceeding from lower-order to higher-order interaction measures requires the resolution of ambiguities concerning what exactly higher-order interactions represent (Foucquier and Guedj, 2015). In this respect, two recent studies on higher-order drug interactions with a major focus on three-way interactions—in terms of data representation and analysis—shed light by distinguishing between net and emergent higher-order interactions (Beppler et al., 2016; Tekin et al., 2016). A net interaction, which is the more commonly considered type of higher-order interaction, refers to the total interaction that results from effects at all levels. In contrast, emergent interactions arise only when all of the components (or objects) or some subset of components are combined. Similarly, a more comprehensive and general approach is needed to evaluate higher-order interaction effects in a wide range of fields. From an ecological perspective, when conservation management is the goal for a prey population, understanding the actual interactions among all predators can be critical. We cannot assume that more predators will increase prey risk, and similarly, without proper emergent interactions analysis, we do not know which predator removal or decrease will ultimately increase a prey's number. Therefore, a generalized approach for quantifying higher-order interactions must directly distinguish between net and emergent interactions and incorporate effects resulting from different levels of interaction [where the level corresponds to the number of components in any given subset of component, ranging from one component (level 1) up to the whole system (level N)]. This higher-order interaction framework would require data for responses for each subsets of components—singles, pairs, triples, etc.
In this paper, we first establish a general framework for analyzing two-way interactions and show how our framework can be used to derive the many interaction measures described above. Indeed, we show how all the measures mentioned above (such as mutual information, ANOVA, multiplicative models, etc., see Table 1) fit within this framework. Next, we present a conceptual and theoretical advance for building higher-order interaction measures that starts from a general framework of pairwise interactions and the definition of no-interaction at the pairwise level. In doing so, we show how different interaction measures are generated by different choices of mathematical operations and functionals, potentially allowing exploration of all possible interaction measures via all possible choices of operations and associated identity elements. Importantly, our general theory of interactions facilitates the understanding of connections between different measures that are frequently used in different research areas and will thus be a useful guide for studies of interactions in many distinct fields.
Similarities Across Different Interaction Measures
Despite the fact that there are a wide range of choices for pairwise interaction metrics (see Table 1), interactions are defined generically based on a prediction or expectation that signifies no interaction and corresponds to each respective measure having a value of 0. Given the no-interaction expectation—equivalently referred to as additive, multiplicative, or independence expectations—the classification of interactions is evaluated based on deviations from this no-interaction case. When the combined effect is sufficiently greater or weaker than the expected effect of no-interaction, the interaction is classified as positive (synergy or cooperation) or negative (antagonism or interference), respectively (see Figure 1 for a toy example and an ecological case study). Interpreting the magnitude of interaction often requires rescaling (normalizing), using methods such as those developed by Segrè et al. (2005) and Tekin et al. (2016), and then testing the significance by various statistical analysis methods such as t-tests, bootstrapping experiments, and Bayesian statistics (Jakulin, 2005).
Importantly, some interaction measures (as briefly overviewed in the Introduction and presented in Table 1) are strongly associated with each other in terms of their construction and baseline expectation of no-interaction. For instance, the underlying additive model of ANOVA is strongly linked with the additive model of Multiple Predator Effects (MPE) studies (Sih et al., 1998), where the expected effect of combination is sum of their individual effects. On the other hand, there is a correspondence between the multiplicative model of MPEs—the expected effect of combination is product of their individual effects—and the Bliss Independence model of drug interactions and epistasis (Beppler et al., 2016). These correspondences suggest that further exploration and potential applications of interaction measures to different systems could be fruitful. Our general framework uncovers these similarities and hence proves to be very useful in understanding the interaction measures and their similarities as well as their applications to different systems.
When proceeding from simpler forms of interactions (i.e., pairwise interactions) to higher-order interactions (i.e., three-way, four-way, N-way interactions), two different types of interaction classifications arise, namely net and emergent interactions (Beppler et al., 2016). Net interactions measure whether any effective interaction exists at all, whereas emergent interactions measure whether there are interactions beyond what is expected from the “sum” of the lower-order parts. Inherently, characterizing higher-order interactions necessitates data for all the system outcomes (i.e., responses) in the presence of all the subsets of components. For example, for the characterization of three-way interaction, one would need responses in the presence of each single component alone, responses under all pairwise component combinations, and responses under all three components. Overall, better understanding of how higher-order interaction measures translate into these two different types of higher-order interactions is vital to better predict dynamics of complex system cooperations.
Methods
Generalized Form of Interaction Measures
General Theory of Two-Way Interactions
Here, we introduce a mathematical framework that generalizes and unifies interaction measure formulations for two components (equivalently referred to as factors or objects). In a broad context, interactions are determined based on the evaluation of the combined effect relative to a null expectation of no-interaction based on the single-component effects. Hence, for defining a generalized formula for interactions, it is essential to introduce notation, properties of operations for combining components, and formulations for assessing the effects of combined or single components. Here, we define an algebraic operation ° (associative and commutative) that combines two components X and Y and that has identity element I, and a real-valued functional, , (a function of functions) and a second algebraic operation △ (associative and commutative) that combines this functional with different arguments (Figure 2). Then, we express the definition of no-interaction as , and define the generalized measure for quantifying interactions between two components as
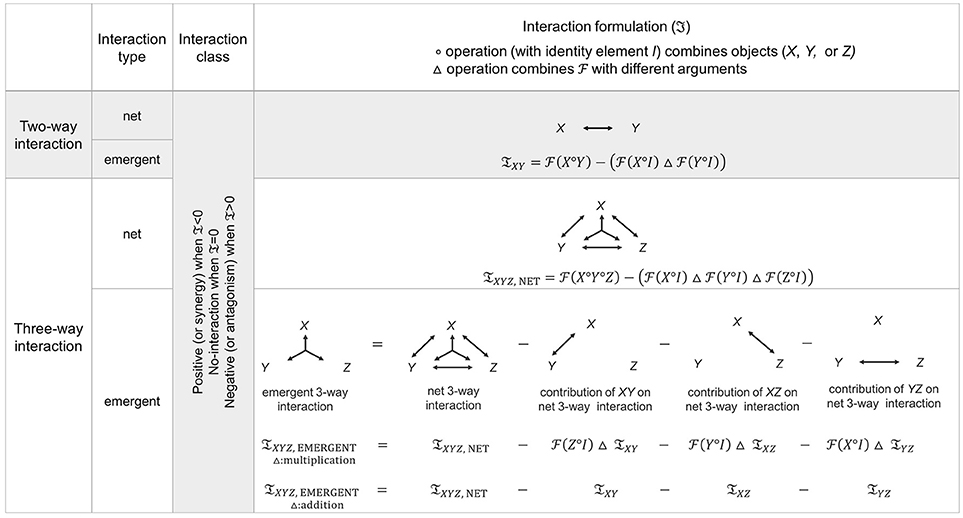
Figure 2. Generalized form of interaction diagrams and measures. Two-way and three-way interaction diagrams and measures (𝔗) over distinct interaction types, general functionals (), and operations (, △) are given. Empirically, classification of pairwise interaction requires measurements from each single component alone as well as measurements from each pairwise combination of components. On the other hand, classification of three-way interactions requires measurements from all possible subsets: measurement under each single-component alone, measurement from all pairwise subsets, and measurement from triple-component combination. Theoretically, for two-way interactions, the definitions of net and emergent interactions are identical. On the other hand, net and emergent interactions are not the same at the three-way level because the net three-way combination subsumes the pairwise combination effects of each pair (XY, XZ, YZ). Therefore, the emergent 3-way interaction (the diagram on the bottom far left) is expressed by subtracting all lower-order contributions from the net 3-way interaction. The interaction classification is obtained from the sign of the interaction measure, 𝔗, where 𝔗 = 0 represents no-interaction, 𝔗 < 0 represents synergy (positive interaction), and 𝔗 > 0 represents antagonism (negative interaction). See Figure 1 for a toy example of consumer-resource systems that depicts different interaction classes: synergy, no-interaction, and antagonism.
The identity element in the functional form of the interaction measure is provided in the generalized formula to explicitly make the point that the absence of another component should yield no interaction. In other words, combining any component (X) with the identity element (Y = I) should be equivalent to no interaction, corresponding to I = 0 for addition and to I = 1 for multiplication. Moreover, the arithmetic operations are restricted to either addition or multiplication to preserve the associativity and commutativity properties of combining more than two components. This preservation property is because there is no time component in our framework that corresponds to introducing different components to the system. In other words, the ordering of different components in the interaction definition does not matter, hence 𝔗XY = 𝔗YX for two components and 𝔗XYZ = 𝔗YXZ = 𝔗ZXY for three components (see next section). By definition, 𝔗XY = 0 generally means there is no interaction or dependence between two components, whereas deviation of 𝔗XY from zero suggests an interaction. Different choices of operations and functional correspond to different choices of interaction measures as derived below. Furthermore, the sign and magnitude of 𝔗XY indicates the type and strength of interaction after proper rescaling (Segrè et al., 2005; Tekin et al., 2016). Rescaling has a firm conceptual foundation that has been proven useful in many areas and is defined relative to important baselines, such as a maximally synergistic scenario or special cases of antagonism, to appropriately reflect the strength of any interaction (Segrè et al., 2005; Sanjuán and Elena, 2006; Tekin et al., 2016). Similarly, two-way and higher-order interaction measures need a rescaling method (a normalization procedure) to distinguish different interaction categorizations.
General Theory of Higher-Order Interactions
As we proceed from two-way to higher-order interactions, there are two distinct types of interactions: (1) net interactions and (2) emergent interactions (Figure 2). The first form measures whether an effective or net interaction exists at all, hence defined as an effect of a combination that is different than predicted from solely the effects of non-interacting single components. On the other hand, the second form measures whether there are emergent interactions beyond what is expected from the lower-order parts of the whole combination. Below, by following a similar notation as for two-way interactions, we introduce the functional forms of generalized higher-order interaction measures. For that, we first note that the ordering of the components (or objects) does not matter as we assume the absence of a time component in our framework. Therefore, the interaction measures are symmetric with respect to each component—hence X(Y°Z) is equivalent to X°Y°Z or X°Z°Y and vice versa—because algebraic operations of ° and △ hold commutativity and associativity properties.
Net interactions
Net interactions are measured by a direct extension of the generalized formula for two components (Figure 2) (Sanjuán and Elena, 2006; Staude et al., 2010; Beppler et al., 2016). For example, when three components are completely independent or do not interact at any level, then the functional with the combined components as the argument is equal to the combination of functionals of single components under the operation △. Therefore, adding a third component (Z) into the generalized version of the two-way interaction measure (Equation 1), the generalized version of the three-way net interaction measure becomes
Next, we derive the net interaction formulation for a combination of an arbitrary number, N, of components. Representing the set of components by X1, X2, ⋯ , XN, and assuming there is no interaction between component X1 and the rest of the components, we have
By induction or equivalently applying the same realization repeatedly to the right side of the above equation yields the N-way version of the generalized formula as given by
Using a big △ notation to denote the application of the operation △ to a sequence of numbers—similar to sigma (or pi) notation for summation (or multiplication)—our generalized formula for quantifying net N-way interaction is given by
Emergent interactions
Emergent interactions are defined as interactions that exist even after the exclusion of all interaction effects that are due to lower-order parts and may contribute to a combined overall (net) interaction (Figure 2). In the drug interaction and epistasis terminology, emergent interactions are concretely defined in our recent work and clearly contrasted with the definition of net interaction (Beppler et al., 2016; Tekin et al., 2016). Specifically, an emergent interaction is an interaction that exists beyond the effects that are due to all lower-order parts, whereas a net interaction is any effect beyond only the individual component effects. In an article by Staude et al. (2010) on covariance, this concept is referred to as the dependence in the higher-order combination that is not embedded through the lower-order correlations. In information theory, Jakulin et al. (Jakulin, 2005) referred to this idea similarly and stated that interaction information among different attributes can be gained by the information that is not present in any subset. By definition, at the two-way level, an emergent interaction is identical to the net interaction because there is nothing from which to emerge except the single-component effects. On the other hand, at the next level, the emergent three-way interaction measure captures the interaction that does not originate from the pairwise parts, and hence emerges only in the presence of all three components. For more than three components, it matters what level of lower-order interactions needs to be excluded. There can be different choices based on the specific system or the main purpose of the interaction identification, as revealed in more detail below and in the Appendix A in Supplementary Material.
As a start, to measure the emergent three-way interaction, we calculate how much of the three-way net interaction originates from each of the isolated pairs and subtract that from the net (or total) three-way interaction. In this case, the contribution that comes solely from the pair X, Y represents the situation that only X, Y interacts within the three-way combination of X, Y, and Z. In other words, the remaining component Z within the combination does not interact with the pairwise part of X and Y or either X or Y individually. Employing the two-way interaction formula of generalized interaction measure (Equation 1) and the associative properties of operations, this is equivalent to saying that . Hence, the two-way combination of components X, Y contributes to the entire (net) three-way interaction (Equation 2) by an amount of , which is equal to when △ is multiplication and is equal to 𝔗XY when △ is addition. Similarly deriving the contribution coming solely from the interaction of components Y and Z and also from X and Z, the generalized formula of the emergent three-way interaction is given by disentangling the sum of the pairwise parts from the three-way interaction. Therefore, given the multiplication operation of △, the emergent 3-way interaction among components X, Y, and Z is
On the other hand, the weighting parameters (e.g., disappear when △ is the addition operation, so an emergent interaction is given by
These expressions mean that when some interaction exists but all the pairs are independent (or not interacting), then the emergent interaction is equal to the net interaction because any interaction can only be coming from the combined effects of all three drugs for this special case. When pairwise interactions exist and do not cancel, the net and emergent interaction measures will virtually always differ. Notice also that all interaction measures are symmetric with respect to each component. Moreover, when a single component is independent (or not interacting) with the others, the emergent three-way measure vanishes, as desired.
For N components (X1, X2,… XN), the emergent N-way interaction formula can be derived similarly by starting from the definition of the generalized formula for net N-way interactions and subtracting the appropriate lower-order effects. The derivation for generalized formula of emergent interactions with four components is described in detail in Appendix A in Supplementary Material.
Results
Construction of Specific Two-Way Interaction Measures From General Theory
We will now derive how our generalized framework of two-way interactions leads to numerous interaction measures introduced in studying generic multi-component systems (Table 1). For this, we start by defining X and Y as random variables and will show that the generalized formula reduces to the covariance formulation and mutual information by the choice of different algebraic operations of and △. Given that is the expectation operator, and both and △ are the operation of multiplication, 𝔗XY (Equation 2) becomes covariance and hence measures the joint variability between X and Y, as
Here, we note that when X and Y are independent, their covariance is zero, i.e., 𝔗XY = 0. Notably, the two-way covariance measure is analogous to concepts in theoretical physics such as joint cumulants or Ursell functions (Kendall and Stuart, 1969) and the Isserlis formula in statistics (Isserlis, 1918). However, the higher-order interaction formulations of these concepts differ from the higher-order covariance when there are more than three components, as derived in Appendix A in Supplementary Material.
Following the covariance derivation using our general theory of two-way interactions, we now show that choosing appropriate functional and algebraic operations leads to the mutual information measure of interactions. Hence, we define to be negative entropy, △ to be addition, and ° to be addition (i.e., I = 0). Here, the ° operation represents the combination of two components and hence defines joint entropy under the functional . For these choices, the generalized formula yields the mutual information
Here, associating the random variables X and Y with attributes, the interaction formulation 𝔗XY measures the correlation between the attributes based on concepts of information theory. By definition, when X and Y have no interaction, their mutual information vanishes because no information is gained about one attribute through the other attribute (Jakulin, 2005).
Proceeding to cases for which the arguments X and Y represent experimental data rather than standard random variables, we discuss how interactions are measured by the formula 𝔗XY by evaluating the effects of combined components inferred from system responses () such as growth, survival, or mortality rates (Figure 1). These experimental data types and response functions are used in many important and frequently-used measures of interactions, such as ANOVA, epistatic additivity, Bliss Independence, and multiple predator effects measures.
We begin by reviewing how ANOVA (Analysis of Variance) translates into a measure for the prediction of interactions and then deriving its correspondence with our general interaction formula. In the case of two-component systems, ANOVA interactions are quantified by a 2 by 2 factorial design with four different observations—corresponding to no components (0), component X alone, component Y alone, and components X and Y together. In this case, two-way ANOVA tests whether the presence of Y affects the impact of X according to a measure based on linear deviations. When the effects of observations are denoted by 𝔖, no interaction is described as 𝔖(X) − 𝔖(0) = 𝔖(X + Y) − 𝔖(Y). Note that this equation can be re-expressed in the form 𝔖(X + Y) − 𝔖(0) = 𝔖(X) − 𝔖(0) + 𝔖(Y) − 𝔖(0). Therefore, redefining the response measurement to be , the interaction is quantified by the significance of deviation from the additive effects of two components, i.e., . This corresponds to a general formula of interactions, 𝔗XY, with ° and △ being addition operations
Regarding the use of ANOVA for interaction classifications, work by Billick and Case (1994) and Wootton (1994) demonstrate that the results of ANOVA can be misleading if the transformation of data is not carefully done or when the empirical system does not align with the assumptions of ANOVA.
Following the similar component types and response measurements, our general interaction measure produces another widely-used model of interactions, namely the multiplicative-risk model. This measure has been introduced by Soluk and Collins (1988) for quantifying multiple predator effects (MPE), and it overcomes the ambiguities of the additive prediction by ANOVA when the single components have large impacts on the response function (. In such cases, the additive expectation can never be achieved because the predicted combined effect is stronger than is feasible or possible for the two together (for example you cannot have a stronger effect than complete killing, see Appendix B in Supplementary Material for details). The multiplicative model formulates no-interaction as , based on the idea of proportional decreases in population size. It is thus much more akin to Bliss Independence discussed below. Importantly, the multiplicative model of interaction can be written as a general interaction formula, where ° and △ are addition and multiplication operations, respectively. Hence,
associates the null model of no-interaction based on the product of response measurements (, which is prey survival rates for the MPE system (Sih et al., 1998; Barrios-O'neill et al., 2014). The subscript notation (such as ) is still typically used to express response measurements under different combinations of predators, or similar component types in different settings, even though X and Y are actually being summed together in this case. Hence, the equation above takes a form . As explained in Appendix B in Supplementary Material, this multiplicative model is preferred over additive models that do not always yield plausible expectations of combination effects. As a further note, this multiplicative model of two-way interaction can be tested by merely applying two-way ANOVA on log-transformed data because testing for is equivalent to testing for by properties of logarithms. However, the multiplicative model at higher-order levels is not simply equivalent to the log-transformed ANOVA as detailed below. Note that following the same logic of additive and multiplicative models, where the interaction effect signifies the deviation from the null model of additivity or multiplicativity, alternative measures to two-way ANOVA [namely Hedge's d and log response ratio (LnRR)] have been proposed to determine interactions based on the deviation from the corresponding model expectation standardized to the effect size (Gurevitch et al., 2000; Crain et al., 2008; Côté et al., 2016). Similar correction terms for the effect sizes can be added to net and emergent higher-order interaction measures for meta-analysis studies.
Intriguingly, there is a strong correspondence between the multiplicative model introduced for MPEs and the frequently used drug-interaction model of Bliss Independence. This indicates that the Bliss measure can also be expressed by utilizing the general interaction formulation we propose here, i.e., . In drug interaction studies, response measurements, , are given as growth rates of pathogens in the presence of single or multiple drug environments relative to the no-drug environment. Relative growth is commonly referred to as relative fitness and is indeed analogous to the survival rates of prey species in predator environments (Beppler et al., 2016). Bliss Independence categorizes interactions based on deviations from a null expectation (no-interaction case) that the percent decrease of the pathogen growth rate in the presence of drug X is not affected by the presence of the other drug Y. Analytically, the deviation from no-interaction instance is measured by or , following a subscript notation as in the multiplicative model.
Building Higher-Order Specific Interaction Measures From General Theory
In this section, we discuss the widely-used pairwise interaction measures described above and summarized in Table 1, and we derive the three-way interaction measures based on the corresponding no-interaction criterions and our general theory. For clarity, we follow the same order of measures as in the section on the construction of two-way interactions. Therefore, we start by reviewing the standard interaction measures when the components are random variables. In probability theory, the extensions of probabilistic measures of moments to multi-variable systems are generally referred as higher-order cross moments. For example, adapting the generalized formula of covariance (see Table 1) to three variables yields a 3rd-order cross moment known as co-skewness that is used as a risk management measure in finance or in social problems (Cox L. A. Jr., 2009; Miller, 2013). To be more explicit, the net interaction measure (Equation 3) is given by 𝔗XYZ, NET = E(XYZ) − E(X)E(Y)E(Z) (Stratonovich, 1967; Staude et al., 2010), which vanishes when X, Y, and Z are mutually independent with each other and each pairwise product. Next, substituting the generalized formula of two-way interactions (i.e., covariance) and the net three-way interaction, the emergent interaction (Equation 4) becomes
which is equivalent to the three-way covariance [i.e., E((X − E(X))(Y − E(Y))(Z − E(Z)))] or the non-normalized formula of co-skewness as discussed above. Notably, this three-way measure is equivalent to the Isserlis formula (Isserlis, 1918), 3-point connected correlation, or equivalently the Ursell function with three random variables (Kendall and Stuart, 1969; Staude et al., 2010). However, this does not hold true for more than three components (Appendix A in Supplementary Material).
Continuing with the component types as random variables, we now show that the general formula of higher-order interactions reduces to two interaction measures of paramount importance in information theory. Defining the functional as the negative of entropy and following the algebraic operations that yield mutual information (see Table 1), the net interaction formulation coincides with the total correlation measure as given by
This measures the total dependence among three attributes X, Y, and Z (Watanabe, 1960; Jakulin, 2005). By incorporating the pairwise mutual information into the emergent interaction formulation when △ is the addition operation (Equation 5), we attain the multiple mutual information measure defined by McGill (McGill, 1954; Sun Han, 1980; Jakulin, 2005).
Thus far, we have introduced the commonly used interaction and dependence measures from probability theory. Next, we move onto the standard measure of ANOVA, which analyzes the effects of multiple components via the factorial experiment design. As in the two-way interactions, we simply use the underlying additive model of three-way ANOVA and refer to it as just an additive model (Table 1). When the response function is defined relative to the control (no component is present), the three-way net interaction formula of the additive model is given by . Now, substituting each pairwise contribution in the generalized formula of emergent interactions (Figure 2), the emergent interaction measure is identical to the underlying model of three-way ANOVA.
This correspondence is proven explicitly in the Appendix C in Supplementary Material by recognizing that three-way ANOVA determines how the two-way interaction between the components X and Y is affected by an addition of a third factor Z. This in-depth derivation of the underlying model of three-way ANOVA helps to compare the statistical measure of ANOVA with other interaction classification methods.
Due to the additive model's implausible predictions under certain cases, many MPE studies have pursued the implementation of the multiplicative model (Appendix A in Supplementary Material). Subsequently, we now derive the definition of three-way interactions under the multiplicative model of MPE studies or equivalently the Bliss Independence formula. This derivation is very similar to the foundation of the covariance for three random variables as △ is the multiplication operation in both concepts (Table 1). Defining the functional as survival rate relative to the control, the net interaction measure becomes
or using subscripts for denoting the combination of components. In this case, substituting the terms corresponding to each pairwise contribution, such as for XY combination, the emergent interaction becomes
Using a fitness definition for , 𝔗XYZ, NET and 𝔗XYZ, EMERGENT correspond to the net and emergent interaction formulas with three components in the fields of drug interactions and gene epistasis (Sanjuán and Elena, 2006; Beppler et al., 2016).
Discussion
Multi-level interactions play an important role in explaining the characteristics of systems that arise as collective behaviors or responses among different components. As an example in conservation biology, mammalian herbivory, invertebrate herbivory, and nutrient levels all interact to affect the survival, growth rates, and fecundity of rare plant species in a forest ecosystem (McGill et al., 2006; Savage et al., 2007; Webb et al., 2010; Dávalos et al., 2014; Enquist et al., 2015). Given this importance across diverse fields, many different interaction classification methodologies have been introduced. However, a unified theory behind these choices has been lacking, and as a result, higher-order interaction measures are often incorrectly derived and interpreted and thus higher-order interactions are not well understood. Moreover, the lack of a general theory behind the pairwise interaction measures makes it difficult to establish a common understanding of higher-order interactions and to design well-defined measures that go beyond pairwise interactions. In our study, we introduce a pioneering approach to resolve these uncertainties and for studying interactions in complex systems. We further propose a general theory of interaction measures to uncover interactions at pairwise and higher-order levels. Our framework provides an advance in such a way that specific cases and measures can be derived from the definition of what an interaction means—the deviation from an expectation that there is no interaction—and what response measurements are needed to evaluate for the interactions—the functional with different arguments. From that we establish a rigorous framework for extending and generalizing these concepts and measures to higher-order levels.
In doing so, we show the utility of our general framework by deriving pairwise interaction measures across different fields—covariance, mutual information, cumulants, ANOVA, Bliss independence, and epistatic additivity (Table 1). Furthermore, we demonstrate that all pairwise interaction measures can be easily extended to characterize the net interaction—an overall effect resulting from all levels—and the emergent interaction—a measurement of an overall interaction effect relative to interactions at lower-order levels. We also discuss their specific representations, correspondences, and interpretations in a diverse set of study areas. Overall, our advance is of importance for studies of diverse complex systems that rely on a large number of interactions and emerge across multiple levels.
Despite a rich focus on pairwise interactions in the literature, recent studies have shown that higher-order interactions are pervasive in drug and gene systems (Taylor and Ehrenreich, 2015a,b; Tekin et al., 2016), and that the typical approach of focusing solely on pairwise interactions is most likely inadequate for successfully attaining important insights into research on complex systems (Weinreich et al., 2013; Beppler et al., 2016; Levine et al., 2017; Mayfield and Stouffer, 2017). Therefore, higher-order interactions (i.e., among any number of components) must be incorporated to fully grasp system dynamics. In this respect, our study provides a promising framework to uncover emergent phenomena in multi-component systems such as protein and gene interaction networks, multiple predator effects, food webs, interacting agents in economics, voting behaviors in political science and the cohesion dynamics of social groups.
As shown by our previous studies (Yeh et al., 2006; Tekin et al., 2016), empirical data of drug interactions separate into a trimodal distribution—clearly separating synergy, no-interaction, and antagonism—and clearly different than would arise from a random model of interactions because stochastic effects would have smoothed out this distribution and led to a more uniform distribution. Furthermore, in our previous study (Tekin et al., 2016), we assessed the statistical significance of interactions by testing against the null hypothesis of random interactions that would on average lead to a uniform distribution that ranges from −1 to 1 across the entire interaction scale. To quantify these comparisons, we used a Silverman test (Silverman, 1981) and found the higher-order emergent interactions are significantly different from random effects. These results reveal higher-order interactions are not simply due to random effects. Also worth noting is that uncertainty and error estimation due to measurements can be dealt with using bootstrapping strategies (Cruz-Loya et al., 2018).
Throughout the text, we also point out a well-founded concept of “rescaling” in physics that has been proven to enhance the categorization of pairwise and higher-order emergent effects (Segrè et al., 2005; Tekin et al., 2016). Without employing an appropriate rescaling approach, the magnitude of interactions is often not apparent and hence can lead to misleading results and erroneous insights. Therefore, appropriately understanding and rescaling the interaction formulations is an essential part of studying and understanding multi-component interactions in any system.
We further note that many systems have additional complexities besides just many interacting components or higher-order interactions. For example, in predator-prey systems, both predators and prey can evolve, and there are direct feedbacks in the interactions. Both of these effects can lead to drastic changes in multiple predator effects (MPEs) (Barrios-O'neill et al., 2014). In addition, pathogens can evolve in response to drug combinations in ways that are almost impossible to predict due to rugose fitness landscapes. To predict and understand the dynamics of pathogen populations thus requires further theory (Palmer et al., 2015). Our general framework of interactions, with additional modifications, will help address some of the important questions about complexities in biological, physical, and social systems. Overall, understanding emergent features can help us to predict the dynamical consequences of complex interactions, including in questions of crucial significance to human and global health, such as combatting the evolution of resistance to antibiotics and mitigating detrimental impacts of climate change on the diversity and stability of food webs.
Author Contributions
ET, PY, and VS conceived of the project, designed the study, and wrote the manuscript. ET and VS developed new analytical tools. All authors gave final approval for publication.
Funding
ET, PY, and VS received funding from James S. McDonnell Complex Systems Scholar Award, US National Science Foundation (ID: 1254159, http://nsf.gov/), UCLA Faculty Career Development Award, Hellman Foundation Award, and NIH/National Center for Advancing Translational Science (NCATS) UCLA CTSI Grant Number UL1TR001881.
Conflict of Interest Statement
The authors declare that the research was conducted in the absence of any commercial or financial relationships that could be construed as a potential conflict of interest.
Acknowledgments
We thank Nina Singh and Cynthia White for comments on the manuscript.
Supplementary Material
The Supplementary Material for this article can be found online at: https://www.frontiersin.org/articles/10.3389/fevo.2018.00166/full#supplementary-material
References
Barrios-O'neill, D., Dick, J. T., Emmerson, M. C., Ricciardi, A., Macisaac, H. J., Alexander, M. E., et al. (2014). Fortune favours the bold: a higher predator reduces the impact of a native but not an invasive intermediate predator. J. Anim. Ecol. 83, 693–701. doi: 10.1111/1365-2656.12155
Beppler, C., Tekin, E., Mao, Z., White, C., Mcdiarmid, C., Vargas, E., et al. (2016). Uncovering emergent interactions in three-way combinations of stressors. J. R. Soc. Interf. 13:20160800. doi: 10.1098/rsif.2016.0800
Billick, I., and Case, T. J. (1994). Higher order interactions in ecological communities: what are they and how can they be detected? Ecology 75, 1529–1543. doi: 10.2307/1939614
Braun, R., and Shah, S. (2015). Network methods for pathway analysis of genomic data. arXiv preprint arXiv:1411.1993.
Côté, I. M., Darling, E. S., and Brown, C. J. (2016). Interactions among ecosystem stressors and their importance in conservation. Proc. R. Soc. B Biol. Sci. 283:20152592. doi: 10.1098/rspb.2015.2592
Case, T. J., and Bender, A. E. (1981). Testing for higher order interactions. Am. Nat. 118, 920–929. doi: 10.1086/283885
Chen, C. Y., Hathaway, K. M., Thompson, D. G., and Folt, C. L. (2008). Multiple stressor effects of herbicide, pH, and food on wetland zooplankton and a larval amphibian. Ecotoxicol. Environ. Saf. 71, 209–218. doi: 10.1016/j.ecoenv.2007.08.007
Coors, A., and De Meester, L. (2008). Synergistic, antagonistic and additive effects of multiple stressors: predation threat, parasitism and pesticide exposure in Daphnia magna. J. Appl. Ecol. 45, 1820–1828. doi: 10.1111/j.1365-2664.2008.01566.x
Cover, T. M., and Thomas, J. A. (2012). Elements of Information Theory. Hoboken, NJ: John Wiley & Sons.
Cox, L. A. Jr (2009). Risk Analysis of Complex and Uncertain Systems. Denver, CO: Springer Science & Business Media. doi: 10.1007/978-0-387-89014-2
Crain, C. M., Kroeker, K., and Halpern, B. S. (2008). Interactive and cumulative effects of multiple human stressors in marine systems. Ecol. Lett. 11, 1304–1315. doi: 10.1111/j.1461-0248.2008.01253.x
Cruz-Loya, M., Kang, T. M., Lozano, N. A., Watanabe, R., Tekin, E., Damoiseaux, R., et al. (2018). Stressor interaction networks suggest antibiotic resistance co-opted from stress responses to temperature. ISME J. doi: 10.1038/s41396-018-0241-7. [Epub ahead of print].
Darling, E. S., and Côté, I. M. (2008). Quantifying the evidence for ecological synergies. Ecol. Lett. 11, 1278–1286. doi: 10.1111/j.1461-0248.2008.01243.x
Dávalos, A., Nuzzo, V., and Blossey, B. (2014). Demographic responses of rare forest plants to multiple stressors: the role of deer, invasive species and nutrients. J. Ecol. 102, 1222–1233. doi: 10.1111/1365-2745.12279
Enquist, B. J., Norberg, J., Bonser, S. P., Violle, C., Webb, C. T., Henderson, A., et al. (2015). Scaling from traits to ecosystems: developing a general Trait Driver Theory via integrating trait-based and metabolic scaling theories. Adv. Ecol. Res. 52, 249–318. doi: 10.1016/bs.aecr.2015.02.001
Foucquier, J., and Guedj, M. (2015). Analysis of drug combinations: current methodological landscape. Pharmacol. Res. Perspect. 3:e00149. doi: 10.1002/prp2.149
Gurevitch, J., Morrison, J. A., and Hedges, L. V. (2000). The interaction between competition and predation: a meta-analysis of field experiments. Am. Nat. 155, 435–453. doi: 10.1086/303337
Hamilton, L. C. (2011). Education, politics and opinions about climate change evidence for interaction effects. Clim. Change 104, 231–242. doi: 10.1007/s10584-010-9957-8
Isserlis, L. (1918). On a formula for the product-moment coefficient of any order of a normal frequency distribution in any number of variables. Biometrika 12, 134–139. doi: 10.1093/biomet/12.1-2.134
Jakulin, A. (2005). Machine Learning Based on Attribute Interactions. Ph.D. dissertation, Univerza v Ljubljani.
Jakulin, A., and Bratko, I. (2003). Analyzing attribute dependencies. Knowl. Discov. Databases PKDD 2003, 229–240. doi: 10.1007/978-3-540-39804-2_22
Kohn, A., and Smith, M. A. (2005). Stimulus dependence of neuronal correlation in primary visual cortex of the Macaque. J. Neurosci. 25, 3661–3673. doi: 10.1523/JNEUROSCI.5106-04.2005
Levine, J. M., Bascompte, J., Adler, P. B., and Allesina, S. (2017). Beyond pairwise mechanisms of species coexistence in complex communities. Nature 546, 56–64. doi: 10.1038/nature22898
Margolin, A. A., Nemenman, I., Basso, K., Wiggins, C., Stolovitzky, G., Favera, R. D., et al. (2006). ARACNE: an algorithm for the reconstruction of gene regulatory networks in a mammalian cellular context. BMC Bioinformatics 7:S7. doi: 10.1186/1471-2105-7-S1-S7
Matsui, T., and Ehrenreich, I. M. (2016). Gene-environment interactions in stress response contribute additively to a genotype-environment interaction. PLoS Genet. 12:e1006158. doi: 10.1371/journal.pgen.1006158
May, R. M. (1972). Will a large complex system be stable? Nature 238, 413–414. doi: 10.1038/238413a0
Mayfield, M. M., and Stouffer, D. B. (2017). Higher-order interactions capture unexplained complexity in diverse communities. Nat. Ecol. Evol. 1:62. doi: 10.1038/s41559-016-0062
McGill, B. J., Enquist, B. J., Weiher, E., and Westoby, M. (2006). Rebuilding community ecology from functional traits. Trends Ecol. Evol. 21, 178–185. doi: 10.1016/j.tree.2006.02.002
McGill, W. J. (1954). Multivariate information transmission. Psychometrika 19, 97–116. doi: 10.1007/BF02289159
Mihaila, B., Cardenas, A., Cooper, F., and Saxena, A. (2010). Stability and dynamical properties of Cooper-Shepard-Sodano compactons. Phys. Rev. E 82:066702. doi: 10.1103/PhysRevE.82.066702
Miller, M. B. (2013). Mathematics and Statistics for Financial Risk Management. Hoboken, NJ: John Wiley & Sons. doi: 10.1002/9781118819616
Moore, J. H., Gilbert, J. C., Tsai, C.-T., Chiang, F.-T., Holden, T., Barney, N., et al. (2006). A flexible computational framework for detecting, characterizing, and interpreting statistical patterns of epistasis in genetic studies of human disease susceptibility. J. Theor. Biol. 241, 252–261. doi: 10.1016/j.jtbi.2005.11.036
Nishikawa, T., and Motter, A. E. (2016). Symmetric states requiring system asymmetry. Phys. Rev. Lett. 117:114101. doi: 10.1103/PhysRevLett.117.114101
O'gorman, E. J., and Emmerson, M. C. (2009). Perturbations to trophic interactions and the stability of complex food webs. Proc. Natl. Acad. Sci. U.S.A. 106, 13393–13398. doi: 10.1073/pnas.0903682106
Palmer, A. C., Toprak, E., Baym, M., Kim, S., Veres, A., Bershtein, S., et al. (2015). Delayed commitment to evolutionary fate in antibiotic resistance fitness landscapes. Nat. Commun. 6:7385. doi: 10.1038/ncomms8385
Pandey, B., and Sarkar, S. (2017). How much a galaxy knows about its large-scale environment?: an information theoretic perspective. Mon. Not. R. Astron. Soc. Lett. 467, L6–L10. doi: 10.1093/mnrasl/slw250
Podgornaia, A. I., and Laub, M. T. (2015). Pervasive degeneracy and epistasis in a protein-protein interface. Science 347, 673–677. doi: 10.1126/science.1257360
Sahidullah, M., and Kinnunen, T. (2016). Local spectral variability features for speaker verification. Digit. Signal Process. 50, 1–11. doi: 10.1016/j.dsp.2015.10.011
Sanjuán, R., and Elena, S. F. (2006). Epistasis correlates to genomic complexity. Proc. Natl. Acad. Sci. U.S.A. 103, 14402–14405. doi: 10.1073/pnas.0604543103
Savage, V. M., Webb, C. T., and Norberg, J. (2007). A general multi-trait-based framework for studying the effects of biodiversity on ecosystem functioning. J. Theor. Biol. 247, 213–229. doi: 10.1016/j.jtbi.2007.03.007
Segrè, D., Deluna, A., Church, G., and Kishony, R. (2005). Modular epistasis in yeast metabolism. Nat. Genet. 37, 77–83. doi: 10.1038/ng1489
Shi, S. (2016). Customer relationship and sales. J. Econ. Theory 166, 483–516. doi: 10.1016/j.jet.2016.09.012
Sigal, J., Hsu, L., Foodim, S., and Betman, J. (1988). Factors affecting perceptions of political candidates accused of sexual and financial misconduct. Polit. Psychol. 9, 273–280. doi: 10.2307/3790956
Sih, A., Englund, G., and Wooster, D. (1998). Emergent impacts of multiple predators on prey. Trends Ecol. Evol. 13, 350–355. doi: 10.1016/S0169-5347(98)01437-2
Silverman, B. W. (1981). Using kernel density estimates to investigate multimodality. J. R. Stat. Soc. Ser. B 43, 97–99.
Soluk, D. A., and Collins, N. C. (1988). Synergistic interactions between fish and stoneflies: facilitation and interference among stream predators. Oikos 52, 94–100. doi: 10.2307/3565987
Staude, B., Grün, S., and Rotter, S. (2010). Higher-order correlations and cumulants. Anal. Parallel Spike Trains 7:253–280. doi: 10.1007/978-1-4419-5675-0_12
Stratonovich, R. L. (1967). Topics in the Theory of Random Noise. New York, NY: CRC Press; Gordon and Breach.
Sun Han, T. (1980). Multiple mutual informations and multiple interactions in frequency data. Inf. Control 46, 26–45. doi: 10.1016/S0019-9958(80)90478-7
Taylor, M. B., and Ehrenreich, I. M. (2015a). Higher-order genetic interactions and their contribution to complex traits. Trends Genet. 31, 34–40. doi: 10.1016/j.tig.2014.09.001
Taylor, M. B., and Ehrenreich, I. M. (2015b). Transcriptional derepression uncovers cryptic higher-order genetic interactions. PLoS Genet. 11:e1005606. doi: 10.1371/journal.pgen.1005606
Tekin, E., Beppler, C., White, C., Mao, Z., Savage, V. M., and Yeh, P. J. (2016). Enhanced identification of synergistic and antagonistic emergent interactions among three or more drugs. J. R. Soc. Interf. 13:20160332. doi: 10.1098/rsif.2016.0332
Tekin, E., Savage, V. M., and Yeh, P. J. (2017). Measuring higher-order drug interactions: a review of recent approaches. Curr. Opin. Syst. Biol. 4, 16–23. doi: 10.1016/j.coisb.2017.05.015
Toprak, E., Palmer, A. C., Kim, S., Veres, A., Bershtein, S., and Kishony, R. (2013). Evolution of antibiotic resistance through a multi-peaked adaptive landscape. Biophys. J. 104:660a. doi: 10.1016/j.bpj.2012.11.3644
Wang, H., Lee, D.-K., Chen, K.-Y., Chen, J.-Y., Zhang, K., Silva, A., et al. (2015). Mechanism-independent optimization of combinatorial nanodiamond and unmodified drug delivery using a phenotypically driven platform technology. ACS Nano 9, 3332–3344. doi: 10.1021/acsnano.5b00638
Watanabe, S. (1960). Information theoretical analysis of multivariate correlation. IBM J. Res. Dev. 4, 66–82. doi: 10.1147/rd.41.0066
Webb, C. T., Hoeting, J. A., Ames, G. M., Pyne, M. I., and Poff, N. L. (2010). A structured and dynamic framework to advance traits-based theory and prediction in ecology. Ecol. Lett. 13, 267–283. doi: 10.1111/j.1461-0248.2010.01444.x
Weinreich, D. M., Lan, Y., Wylie, C. S., and Heckendorn, R. B. (2013). Should evolutionary geneticists worry about higher-order epistasis? Curr. Opin. Genet. Dev. 23, 700–707. doi: 10.1016/j.gde.2013.10.007
Wootton, J. T. (1993). Indirect effects and habitat use in an intertidal community: interaction chains and interaction modifications. Am. Nat. 141, 71–89. doi: 10.1086/285461
Wootton, J. T. (1994). Putting the pieces together: testing the independence of interactions among organisms. Ecology 75, 1544–1551. doi: 10.2307/1939615
Wootton, K. L., and Stouffer, D. B. (2016). Species' traits and food-web complexity interactively affect a food web's response to press disturbance. Ecosphere 7:e01518. doi: 10.1002/ecs2.1518
Keywords: complex biological systems, emergent patterns, higher-order interactions, ecological interactions, biodiversity
Citation: Tekin E, Yeh PJ and Savage VM (2018) General Form for Interaction Measures and Framework for Deriving Higher-Order Emergent Effects. Front. Ecol. Evol. 6:166. doi: 10.3389/fevo.2018.00166
Received: 11 May 2018; Accepted: 02 October 2018;
Published: 23 October 2018.
Edited by:
Mary I. O'Connor, University of British Columbia, CanadaReviewed by:
Marcelo Michel Rivadeneira, Centro de Estudios Avanzados en Zonas Áridas (CEAZA), ChileJuan Ernesto Guevara Andino, Field Museum of Natural History, United States
Copyright © 2018 Tekin, Yeh and Savage. This is an open-access article distributed under the terms of the Creative Commons Attribution License (CC BY). The use, distribution or reproduction in other forums is permitted, provided the original author(s) and the copyright owner(s) are credited and that the original publication in this journal is cited, in accordance with accepted academic practice. No use, distribution or reproduction is permitted which does not comply with these terms.
*Correspondence: Van M. Savage, vsavage@ucla.edu