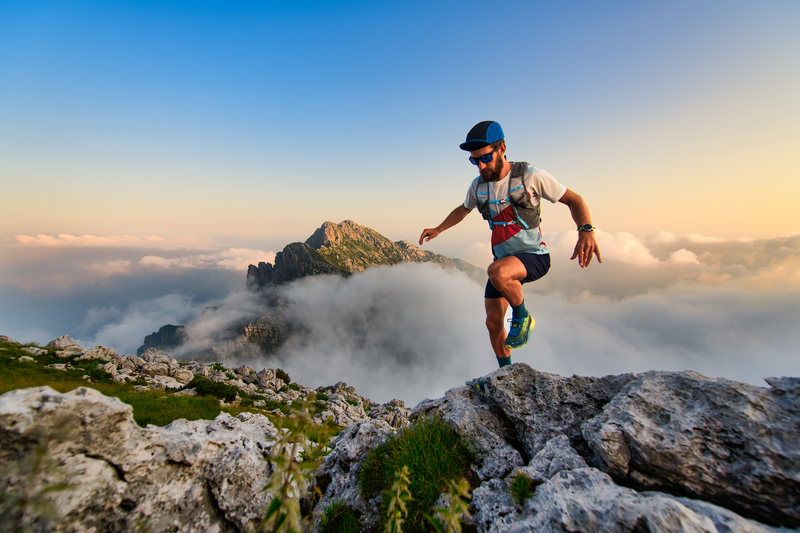
95% of researchers rate our articles as excellent or good
Learn more about the work of our research integrity team to safeguard the quality of each article we publish.
Find out more
ORIGINAL RESEARCH article
Front. Ecol. Evol. , 24 September 2018
Sec. Urban Ecology
Volume 6 - 2018 | https://doi.org/10.3389/fevo.2018.00148
This article is part of the Research Topic Partitioning the Effects of Urbanization on Biodiversity: Beyond Wildlife Behavioural Responses to a Multilevel Assessment of Community Changes in Taxonomic, Functional and Phylogenetic Diversity View all 6 articles
Urban habitats present new ecological and evolutionary challenges for animals. Noise and infrastructure often change behavior and community composition, with potential physical costs such as decreased condition. However, the underlying mechanisms driving these patterns are virtually unknown. One potential driver of condition within a species is the diversity of the gut microbiome. Here, we investigate how the urban habitat affects the gut microbiome of White-crowned Sparrows (Zonotrichia leucophrys) males using spatial analyses of land cover (impervious, scrub, grass, and trees) at the regional level and territory level in urban San Francisco, CA and nearby rural Point Reyes, California. We hypothesized that urbanization of habitats affects gut microbial composition and diversity, potentially through direct effects on diet and/or indirect environmental effects. We measured gut microbial community diversity from 16s rRNA sequences amplified from cloacal swabs. We find that the urban and rural male gut microbiomes are significantly different, such that the urban gut microbiome is more diverse than the rural gut microbiome. This relationship may be due to more variable land cover types in urban habitats as compared to rural habitats, which are mainly composed of native scrub. We do not find support for regional impervious cover affecting the gut microbiome, but the more precise territory level analyses show that higher tree cover correlates with increased alpha diversity and impervious cover correlates with relative abundances of gut microbial taxa (Unifrac beta diversity). Although some studies show that gut diversity affects physiology, our measures of body condition do not indicate a strong relationship. Our results highlight how changes in the landscape may affect the gut microbiome of animals in an ever-urbanizing world, and provide a baseline for future studies of how anthropogenic change affects communities at multiple levels.
Anthropogenic activity has changed the course of evolution and adaptation to natural phenomena—such that since the rise of the industrial revolution, animals, plants, and microbes have faced an unprecedented ecological pressure. In recent years, many studies have investigated the consequences of anthropogenic activities on individual behavior (Slabbekoorn, 2013), community dynamics (Francis et al., 2012), ecosystem features (Aronson et al., 2014; Turrini and Knop, 2015), and biodiversity management (Aronson et al., 2017). One such important feature of anthropogenic change is the physical change in landscape (Fischer and Lindenmayer, 2007).
Urban landscapes often have drastically different plant communities and available food sources for species that persist in cities. While species may persist, there are often physiological and behavioral consequences for individuals that can carry population level fitness consequences, both positive and negative (Fahrig and Rytwinski, 2009; Morelli et al., 2014). For example, urban landscapes often are correlated with increased disease transmission (Dhondt et al., 2007; Robb et al., 2008) and limited native plants (Paker et al., 2014), which can lead to decreased fitness and biodiversity. Furthermore, many behavioral changes have been noted across taxa based in urbanization gradients. Many species have acoustically adapted their vocalizations to better transmit in urban environments (Morton, 1975; Endler, 1992), singing higher pitched, narrow bandwidth songs, or changing tempo (Parris et al., 2009; Luther and Derryberry, 2012; Slabbekoorn, 2013; Derryberry et al., 2016). Noisy conditions (Phillips and Derryberry, 2018), habitat quality (Evans et al., 2010; Scales et al., 2011, 2013; Foltz et al., 2015), and introduced food sources (Theimer et al., 2015) may increase aggression among individuals competing for limited resources. These discoveries in both vocal and behavioral changes are important first steps to approaching a multilevel understanding of urbanization on community function and assemblage.
While urban areas have widespread and diverse effects on “megafauna” behavior and biodiversity, the microfauna that may ultimately affect daily health or behavior of megafauna is vastly understudied (Barberán et al., 2016). Therefore, a gap exists in our knowledge of how microbiota health of a given system is affected by urban landscapes. Microorganisms play important roles in the function of ecosystems and community diversity (McFall-Ngai et al., 2013), and recent studies are beginning to show links between gut flora and health (Wang et al., 2011; Burcelin, 2012) or behavior in mammals (Collins et al., 2012; Sylvia et al., 2017; Sylvia and Demas, 2018). Typically, a more diverse gut microbiome indicates a healthier host (Consortium, 2012). Gut community is gained both through vertical transmission (mother to offspring) (e.g., Trevelline et al., 2018) and horizontally from the environment (e.g., Hird et al., 2014). Egg-laying animals can initially inoculate eggs in ovo, as seen in five bird species and one lizard species (Ruiz-Rodríguez et al., 2018; Trevelline et al., 2018). For birds, the egg environment in the nest is relatively sterile and inoculation at this stage depends on temperature, humidity, nest hygiene (reviewed in D'Alba and Shawkey, 2015), and ectoparasites (Tomás et al., 2018). Once chicks are hatched, the food fed by parents is likely to further inoculate the gut microbiome (Ruiz-Rodríguez et al., 2018); therefore horizontal transmission of microbes is likely to have a strong effect on gut microbiome diversity. In other words, the habitat and food source of a given organism is likely to affect the host's microbiome, which in turn affects the hosts' immunity pathways, nutrition, growth, and stress regulation (Dillon and Dillon, 2004; Ferrari et al., 2004; Henry et al., 2015; Ruokolainen et al., 2016).
Here, we use a well-studied bird of the coastal sage scrub ecosystem of California, the white-crowned sparrow (Zonotrichia leucophrys), to investigate whether gut diversity is affected by urban and rural landscape features, and whether gut diversity affects body condition. This species is abundant both in urban San Francisco and rural Point Reyes, allowing for a natural experiment for investigating (1) whether urban and rural gut microbiomes differ; (2) whether gut microbial diversity differs with regional impervious surfaces; (3) whether territorial landscape features predict gut diversity, and (4) whether gut diversity correlates with body condition. We predict that urban and rural gut microbiomes will differ, such that native rural landscape features will be correlated to higher gut diversity. We also predict that % impervious surfaces at the regional and territorial scale will be negatively related to gut diversity, while % scrub will positively relate to gut diversity. Lastly, we predict that lower gut diversity correlates with decreased body condition (Sylvia et al., 2017; Sylvia and Demas, 2018).
We mist-netted 77 Nuttall's white-crowned sparrow (Z. l. nuttalli) males, a year-round resident subspecies found in the urban bay area (N = 50) and rural Point Reyes (N = 27) of California, and took cloacal swabs and morphological measurements. Within Point Reyes, we sampled in three regions: Limantour (LIMA), Commonweal (COMW), and Abbott's Lagoon (ABLA) (Figures 1A–C). Within the urban limits, we sampled in seven regions: Fort Funston (FOFU), Fort Scott (FWSC), Battery East (BATE), Battery West (BATE), Lobos Dunes (LODU), Baker Beach (BABE), and Richmond (RICH) (Figures 1D–F). We cleaned sparrows' cloacas with an alcohol swab before sample collection, then inserted sterile swabs (Puritan 25-3316-U 6′′ Sterile Mini-Tipped Nylon Ultra Flocked Swab with Polystyrene Handle) fully into cloaca, turning for 3–5 s. We preserved swabs in RNA Later and froze all samples at the end of each day. Samples were collected during the active breeding season May–June 2016. While in the hand, we took measures of fat score (scale 0–5), mass (g), and tarsus length (mm). We calculated a scaled mass index as a measure of body condition, using tarsus measurements to correct for structural body size following Peig and Green (2009). Sampling techniques were approved by Tulane University Institutional Animal Care and Use Committee (protocol 0427-R), Bird Banding Laboratory Permit (23900), California State Collecting Permit (6799), Golden Gate National Recreation Area (GGNRA) Scientific Research and Collecting Permit (GOGA-00079), San Francisco Parks and Recreation Permit (032014), and Point Reyes National Park Scientific Research and Collecting Permit (PORE-0014).
Figure 1. (Left) National Land Cover Database impervious cover (NLCD; Homer et al., 2011) and (right) aerial views of 3 rural regions (A–C) and 7 urban regions (D–F). Maps created in ArcMap 10 (ESRI, Redlands, CA, USA) with ESRI world imagery (Sources: ESRI, DigitalGlobe, GeoEye, Earthstar Geographics, CNES/Airbus DS, USDA, USGS, AeroGRID, IGN, and the GIS User Community).
We extracted DNA from cloacal swabs using the MoBio Powersoil extraction kit and recommended protocol, with modifications recommended by Vo and Jedlicka (2014). Additionally, we combined solutions C2 and C3, which precipitate non-DNA substances to increase DNA yield. MoBio technicians recommended this modification (personal communication 2016). We chose the V4 region of the 16s rRNA gene to amplify using 515F/806R primers in 25 μL reactions. Each reaction contained: 12 μL sterile, molecular grade water, 1 uL bovine serum albumin, 10 μL 5' hot Mastermix by Thermo Fisher, 0.5 uL of each primer, and 2 μL of DNA template. Each reaction was performed in triplicate to reduce PCR bias. Water was used as a negative control for each set of reactions. Denaturation of DNA was initially performed at 94°C for 2 min, then cycling was carried out as follows: 94°C for 8 s, annealing at 50°C for 20 s, extension at 72°C for 30 s; for 35 cycles. A final elongation was performed at 72°C for 10 min. PCR success was verified with gel electrophoresis.
We pooled each sample's amplicon triplicates and added dual-end Illumina barcodes in the style of TruSeq HT primers. We used gel electrophoresis alongside untagged PCR product to confirm successful addition of tags. We normalized concentrations of all samples using a SequalPrep normalization kit from Thermo Fisher, then pooled PCR product and purified using Agencort AmPure beads. GeneWiz, LLC sequenced our library on an Illumina MiSeq platform with v2 reagents, two by 250 base pairs.
Sequences were processed in Qiime 2 version 2018.4 (qiime2.org) (Caporaso et al., 2010). We used the Divisive Amplicon Denoising Algorith (DADA) to remove sequence errors, and trim primers from sequences (Rosen et al., 2012). We aligned sequences, then generated a phylogeny using FastTree, then rooted the tree at the midpoint (Price et al., 2010). We assigned taxonomy using GreenGenes (McDonald et al., 2012). Finally, we filtered out all mitochondrial, chloroplast, and archaeal sequences.
All diversity metrics were calculated from an OTU table rarefied to a depth of 1000 sequences. We calculated Shannon Diversity with alpha_diversity.py in Qiime, to account for differential abundances of taxa within a bird's gut microbiome. We estimated beta diversity using PCoA values from weighted and unweighted Unifrac dissimilarity matrices, to compare composition of microbial communities among birds, taking into account phylogenetic relatedness of OTUs. The first unweighted principal coordinate axis explained < 10% of the variation, whereas the first axis of the weighted principal coordinate explained more than 50% of variation. Therefore, we used weighted Unifrac distances for all further calculations.
White-crowned sparrows typically defend a 50 m radius around the center of their territory (Morton, 1992); therefore, as an approximate estimate of land cover for each bird, we analyzed a 100 m diameter circle around the center of the territory. Territory centers were approximated based on repeated visits and observations of banded birds in 2016 following Phillips and Derryberry (2017, 2018). Within each territory circle, we visually created polygons using the polygon measuring tool for each land type (impervious, tree, shrub, grass) using Google Earth Pro high resolution imagery (Google, Mountain View, California, USA). The total area for each cover type was calculated as a percentage of the total territory.
At the regional level, we assessed percent impervious cover using NCLD landsat imagery from 2006 provided to the public by the US Geological Service (Homer et al., 2011, 2012). We assessed seven urban regions and three rural regions (Figure 1). To analyze this dataset, we used ArcGIS (10.0, ESRI, Redlands, CA, USA) spatial analyst tool to extract impervious values from the .img from each region's shape file. We set a threshold of impervious surface based on visual matching of cement covered surfaces to Google Earth imagery such that grassland and scrub was not counted as impervious.
Statistical analyses were performed in R (R Development Core Team, 2015). Our diversity values were checked for normality to meet model assumptions using the “qqp” function of packages “car” and “MASS”(Venables and Ripley, 2002; Fox and Weisberg, 2011). We used linear or linear mixed effects models with Akaike's Information Criterion (AICc) to test the predictions of (1) Habitat (urban/rural) (2) Regional impervious cover, and (3) territory level land cover would explain gut microbial diversity. We also assessed the effects of alpha and beta diversity on body condition, with habitat (urban/rural) as a random effect for mixed models. All model selection was conducted using packages “AICcmodavg” and “lme4” (Bates et al., 2015; Mazerolle, 2016). For models that fell within 2 AICc of each other (Arnold, 2010), we model averaged using MuMIn (Barton, 2011) to infer fixed effect relative variable importance (RVI).
Microbial communities were composed of a few dominant genera, with much of the rest of the community made up of more uncommon (< 5%) genera. The top four genera were Mycoplasma (25.77% of urban, 28.43% of rural), Erwinia (9.03% of urban, 6.77% of rural), Campylobacter (5.4% of urban, 0.06% of rural), and Rickettsiella (0.18% of urban, 15.84% of rural). Figure 2 shows that 50% or more of the rural sites were composed of the top two taxa, whereas urban birds have more diverse communities. Five phyla dominated gut microbial communities, Proteobacteria (40.4% of urban, 34.4% of rural), Tenericutes (27% of urban, 33.3% of rural), Firmicutes (10% of urban, 15.4% of rural), Actinobacteria (3.4% of urban, 2.4% of rural), and Bacteroidetes (1.7% of urban, 1% of rural).
Figure 2. Territory cover and relative abundance of gut microbial phyla averaged by region. BABE, Baker Beach; BATE, Battery East; BATW, Battery West; FWSC, Fort Scott; LODU, Lobos Dunes; RICH, Richmond; ABLA, Abbott's Lagoon; COMW, Commonweal.
Overall, habitat (urban or rural) is a stronger predictor of gut diversity than regional impervious cover or territorial impervious cover [ER = 2.9, β = 0.81, SE = 0.38, R2 = 0.05, F(1, 75) = 4.38, p = 0.03, Table 1]. Model averaging of the top three models within 2 AICc show habitat with a RVI of 1, territory impervious with an RVI of 0.27, and the regional impervious cover with an RVI of 0.26. Urban birds have more diverse gut microbiomes than rural birds (Urban Shannon Diversity Index = 3.85, Rural Shannon Diversity Index = 3.05). For beta diversity, the interaction between habitat and territory was the top model, followed by territory impervious cover [ER = 3.43, R2 = 0.05, F(1, 75) = 4.38, p = 0.04, Tables 1, 2].
Table 1. Habitat alone (urban/rural) is the top ranked linear model for Shannon Diversity Index based on AICc model selection, with an evidence ratio of 2.9 over the null model.
Table 2. An interaction between percent impervious territory cover and habitat (urban/rural) is the top ranked model for beta gut diversity of male white-crowned sparrows.
With habitat as a random factor, regional impervious cover was not a strong predictor of gut alpha diversity, with a null model ranked higher than regional impervious cover, territory impervious cover, and models with quadratics of each of those factors (Null model: ER = 2.59, AICc = 300.48, wi = 0.44; Territory Impervious model: AICc = 302.29, wi = 0.17; Regional Impervious model: AICc = 302.48, wi = 0.16). Territory impervious has a RVI of 0.23 while regional impervious has a RVI of 0.21. See Table 3 for percent impervious cover by region.
Regional impervious cover also does not predict beta diversity, with the territory impervious cover and a null model again ranking above models including regional impervious as a fixed factor (Territory impervious model: ER = 4.69, AICc = 60.71, wi = 0.31, RVI = 0.78, Null model: ER = 2.4, AICc = 62.03, wi = 0.16).
To assess whether a specific land cover predicted gut diversity, we tested 15 models combining the effects of tree, shrub, grass, and impervious cover with habitat as a random effect. The highest ranked model was percent tree cover as a fixed effect [ER = 25, β = 2.6, SE = 0.87, R2 = 0.11, F(1, 75) = 9.13, p = 0.003, Table 4, Figure 3]. Model averaging of the 4 top-ranked models within 2 AICc shows relative variable importance as 0.7 for trees, 0.47 for scrub, 0.3 for grass, and 0.3 for impervious.
Table 4. Model selection for land cover within territories shows tree cover as the top model for gut alpha diversity (Shannon Diversity Index).
Figure 3. Birds with more tree cover on their territories have more diverse gut microbial communities.
For beta diversity, impervious cover is the top ranked model, followed by the null and then a model with impervious + impervious2 as fixed factors (Territory impervious model: ER = 1.94, AICc = 60.71, wi = 0.22, Null model: AICc = 62.03, wi = 0.11; impervious + impervious2 model: AICc = 60.71, wi = 0.22). Model averaging within 2 AICc show impervious cover has a relative variable importance of 0.74 while impervious2 has relative importance of 0.22.
Model selection found little support for diversity measures predicting body condition, where beta diversity (PCoA1) was ranked as the best predictor, followed by the null model within 2 AICc [PCoA1: ER = 1.44, AICc = 304.57, wi = 0.41, β = −0.04, SE = 0.57, R2 < 0.01, F(1, 73) = 0.005, p = 0.94; null: AICc = 305.31, wi = 0.29]. For fat score, the model with beta diversity had the most weight, followed by alpha diversity + beta diversity [PCoA1: ER = 3.95, AICc = 195.89, wi = 0.56, β = −0.34, SE = 0.27, R2 = 0.02, F(1, 74) = 1.54, p = 0.22; PCoA1 + Shannon Diversity Index: AICc = 197.99, wi = 0.19].
Overall, we find that male birds in rural landscapes have less diverse gut microbiomes than do male birds in urban landscapes. Variation in impervious surface cover at the regional level does little to explain gut microbiome diversity, but land cover due to trees on males′ territories correlates with their gut microbiome diversity. Our findings suggest that the health of a male birds' gut is affected by the land cover on their territory and whether that territory is located in an urban or rural habitat. These results may have implications for how landscapes are managed in terms of urban species diversity and population health. That is, diverse habitats may lead to diverse gut microbiomes, which may have negative or positive consequences for population heath, depending on microbe function.
When comparing beta diversity across microbial communities, weighted rather than unweighted unifrac distances had the higher explanatory power. This indicates that differences in beta diversity were driven more by relative abundance of each taxa than by presence or absence of microbial community members. For example, although Campylobacter composed about 5% of urban bird microbiomes and Rickettsiela was present at very low levels, the reverse was true for rural birds. White-crowned sparrows across landscapes in this study system share a “core” microbiome, with some bacteria more dominant in some communities than others.
Contrary to our predictions, urban birds have a more diverse gut than rural birds. A previous study found that urban house sparrows had less diverse gut microbiomes than rural sparrows, potentially due to more homogenous environments (Teyssier et al., 2018). In our study area, the urban sites sampled tend to have a more diverse range of cover, including impervious, trees, scrub, and grass, which may explain our findings of higher gut diversity (Figure 2, Table 2). White-crowned sparrows are abundant within or adjacent to San Francisco urban parks, which tend to have a mix of native scrub, introduced shrubs, and introduced canopy such as eucalyptus groves. Mixed with buildings, roads, and anthropogenic food sources, urban birds are likely exposed to more different microbes than rural birds, who would only experience and forage in mostly native scrub. Even though scrub is the native cover for white-crowned sparrows, it is more homogenous than a city landscape near parks, and therefore may lead to lower gut diversity. Higher gut diversity in other animals often is an indicator of health (Burcelin, 2012; Consortium, 2012). If this is the case in this system, urban ecosystems might actually benefit this species. It is important to note that white-crowned sparrows tend to thrive in urban and suburban areas, and therefore a species that is already “urban adapted” may be able to benefit from novel microbiota, whereas more sensitive species that are already declining in urban areas might show opposite patterns. It is also possible that an increase in gut diversity may be an increase in pathogenic bacteria, however 16s rRNA sequencing method is unable to discern the functionality of the genera present in our samples (Sharpton, 2014). This line of research is an open area of inquiry, and can help understand why some species thrive in cities while others do not.
At the more detailed territory level, we found that birds with territories that have more tree cover had more diverse microbiomes. This is an interesting result, as San Francisco's native scrub dunes of pre-western settlers have been transformed into urban forests of Eucalyptus spp., Monterey pine (Pinus radiata) and Monterey cypress (Cupressus macrocarpa) (McClintock, 2001). While many areas throughout the Golden Gate National Recreation Area are undergoing restoration to native scrub, these cultivated trees still have a large presence throughout San Francisco. The addition of these trees seems to benefit gut diversity, opposite of our prediction that scrub cover would increase gut diversity. We postulate that trees provide a layer of heterogeneity to territories, and that trees may attract a different set of insects or other food sources that could affect microbiome diversity. A recent lab study found that gut bacterial richness increased for predatory insects when they had a more diverse prey base (Tiede et al., 2017). Future studies investigating the diet differences could parse out the insect, fruit, or anthropogenic food sources that may be related to more forested areas and potentially be a cause of increased gut diversity in these birds.
In models comparing the importance of all three landscape levels (habitat, regional impervious, and territory impervious), our data suggests that impervious cover—the anthropogenic landscape effects of concrete buildings, roads, and sidewalks—may affect beta diversity. The interaction term between habitat and territory impervious cover likely indicates that urban territories are more heterogeneous and allow urban birds to encounter a wider array of environmental conditions, potentially increasing beta diversity. However, within territory cover models with habitat as a random factor, we found weak support for territory level impervious cover affecting beta diversity. While model selection ranks a model with impervious cover first, the null model is ranked second and within 2 AICc, therefore we do not conclude that territory impervious cover affects beta diversity strongly (Arnold, 2010). Future research could directly test whether microbes from impervious surfaces influence gut diversity by swabbing of the environment itself.
Furthermore, we did not find support for body condition as measured through scaled mass index or fat score having a relationship to the gut microbiome. It may be that these relatively simple indices are not connected to gut health, or are insufficient to fully understand how the gut microbiome affects physiology of free-living wild birds. In more widely studied laboratory animals or humans, there is support for gut diversity affecting health (Burcelin, 2012; Consortium, 2012; Knights et al., 2013; Walters et al., 2014). However, not all taxa seem to be affected by horizontal transmission of gut flora (Hammer et al., 2017). More research into the microbiomes of a wide array of species, including comparisons between anthropophilic and anthrophobic species can lead to important insights into human landscape effects on animal health.
Our results suggest that gut microbiomes of free-living birds are diverse in urban habitats. However, whether broad habitat level landscapes may have positive ultimate consequences for certain populations remain to be seen. Many other urban features beyond gut diversity can affect survival and reproduction. Roads tend to lead to higher mortality (Bishop and Brogan, 2013), and urban parks often attract aerial predators like corvids which leads to higher nest mortality (Thieme et al., 2015). Cities are also louder and tend to have behavioral and physiological effects on wildlife (Francis and Barber, 2013). Within this system, urban white-crowned sparrows tend to be in better body condition than rural sparrows and body condition declines with increasing noise (Phillips et al., 2018). The interaction between humans, animals, and the microbes that ultimately affect the health of animals are uniquely entwined, and we encourage future investigations beyond behavior of single systems into multilevel community analyses.
JP, MB, and ED conceived and designed study. JP and MB collected data. MB ran laboratory procedures and phylogenetic analyses. JP analyzed land cover and models. JP wrote the manuscript with input and final approval from MB and ED.
This work was funded by NSF IOS- 1354763, 1354756, and 1827290; JP was supported by a Tulane Gunning Award, Tulane One-Term Dissertation Award, Wilson Ornithological Society Grant, and an American Ornithologist's Union Van Tyne Award. MB was supported by a Tulane EEB Graduate Student Award.
The authors declare that the research was conducted in the absence of any commercial or financial relationships that could be construed as a potential conflict of interest.
We would like to thank L. Norden for field assistance, J. Paschal for help with landscape analyses, and the Kohl lab, especially B. Trevelline for help with qiime2. Site access and permits were provided by Point Blue Palomarin Field Station staff, B. Merkle, M. Chasse (Golden Gate National Recreation Area), B. Becker (Point Reyes National Seashore), D. Bell (East Bay Regional Parks), and L. Wayne (San Francisco Parks and Recreation).
Arnold, T. W. (2010). Uninformative parameters and model selection using Akaike's information criterion. J. Wildl. Manage. 74, 1175–1178. doi: 10.1111/j.1937-2817.2010.tb01236.x
Aronson, M. F., La Sorte, F. A., Nilon, C. H., Katti, M., Goddard, M. A., Lepczyk, C. A., et al. (2014). A global analysis of the impacts of urbanization on bird and plant diversity reveals key anthropogenic drivers. Proc. Biol. Sci. 281:20133330. doi: 10.1098/rspb.2013.3330
Aronson, M. F. J., Lepczyk, C. A., Evans, K. L., Goddard, M. A., Lerman, S. B., MacIvor, J. S., et al. (2017). Biodiversity in the city: key challenges for urban green space management. Front. Ecol. Environ. 15, 189–196. doi: 10.1002/fee.1480
Barberán, A., Hammer, T. J., Madden, A. A., and Fierer, N. (2016). Microbes Should Be Central to Ecological Education and Outreach. J. Microbiol. Biol. Educ. 17, 23–28. doi: 10.1128/jmbe.v17i1.984
Bates, D., Maechler, M., Bolker, B., and Walker, S. (2015). Fitting linear mixed-effects models using lme4. J. Stat. Softw. 67, 1–48. doi: 10.18637/jss.v067.i01
Bishop, C. A., and Brogan, J. M. (2013). Estimates of Avian mortality attributed to vehicle collisions in Canada. Avian Conserv. Ecol. 8, 1–23. doi: 10.5751/ACE-00604-080202
Burcelin, R. (2012). Regulation of metabolism: a cross talk between gut microbiota and its human host. Physiology 27, 300–307. doi: 10.1152/physiol.00023.2012
Caporaso, J. G., Kuczynski, J., Stombaugh, J., Bittinger, K., Bushman, F. D., Costello, E. K., et al. (2010). QIIME allows analysis of high throughput community sequencing data. Nat. Methods 7, 335–336. doi: 10.1038/nmeth.f.303
Collins, S. M., Surette, M., and Bercik, P. (2012). The interplay between the intestinal microbiota and the brain. Nat. Rev. Microbiol. 10, 735–742. doi: 10.1038/nrmicro2876
Consortium, Human Microbiome Project. (2012). Structure, function and diversity of the healthy human microbiome. Nature 486, 207–14. doi: 10.1038/nature11234
D'Alba, L., and Shawkey, M. D. (2015). Mechanisms of antimicrobial defense in avian eggs. J. Ornithol. 156, 399–408. doi: 10.1007/s10336-015-1226-1
Derryberry, E. P., Danner, R. M., Danner, J. E., Derryberry, G. E., Phillips, J. N., Lipshutz, S. E., et al. (2016). Patterns of song across natural and anthropogenic soundscapes suggest that white-crowned sparrows minimize acoustic masking and maximize signal content. PLoS ONE 11:e0154456. doi: 10.1371/journal.pone.0154456
Dhondt, A. A., Dhondt, K. V., Hawley, D. M., and Jennelle, C. S. (2007). Experimental evidence for transmission of Mycoplasma gallisepticum in house finches by fomites. Avian Pathol. 36, 205–8. doi: 10.1080/03079450701286277
Dillon, R. J., and Dillon, V. M. (2004). The gut bacteria of insects: nonpathogenic Interactions. Annu. Rev. Entomol. 49, 71–92. doi: 10.1146/annurev.ento.49.061802.123416
Endler, J. A. (1992). Signals, signal conditions, and the direction of evolution. Am. Nat. 139, S125–S153. doi: 10.1086/285308
Evans, J., Boudreau, K., and Hyman, J. (2010). Behavioural syndromes in urban and rural populations of song sparrows. Ethology 116, 588–595. doi: 10.1111/j.1439-0310.2010.01771.x
Fahrig, L., and Rytwinski, T. (2009). Effects of roads on animal abundance: an empirical review and synthesis. Ecol. Soc. 14, 1–20. doi: 10.5751/ES-02815-140121
Ferrari, J., Darby, A. C., Daniell, T. J., Godfray, C. J., and Douglas, A. E. (2004). Linking the bacterial community in pea aphids with host-plant use and natural enemy resistance. Ecol. Entomol. 29, 60–65. doi: 10.1111/j.1365-2311.2004.00574.x
Fischer, J., and Lindenmayer, D. B. (2007). Landscape modification and habitat fragmentation: a synthesis. Glob. Ecol. Biogeogr. 16, 265–280. doi: 10.1111/j.1466-8238.2007.00287.x
Foltz, S. L., Ross, A. E., Laing, B. T., Rock, R. P., Battle, K. E., and Moore, I. T. (2015). Behavioral get off my lawn : increased aggression in urban song sparrows is related to resource availability. Behav. Ecol. 26, 1548–1557. doi: 10.1093/beheco/arv111
Fox, J., and Weisberg, S. (2011). An {R} Companion to Applied Regression, 2nd edn. Thousand Oaks, CA: Sage Publishing.
Francis, C. D., and Barber, J. R. (2013). A framework for understanding noise impacts on wildlife: an urgent conservation priority. Front. Ecol. Environ. 11, 305–313. doi: 10.1890/120183
Francis, C. D., Kleist, N. J., Ortega, C. P., and Cruz, A. (2012). Noise pollution alters ecological services: enhanced pollination and disrupted seed dispersal. Proc. Biol. Sci. 279, 2727–2735. doi: 10.1098/rspb.2012.0230
Hammer, T. J., Janzen, D. H., Hallwachs, W., Jaffe, S. P., and Fierer, N. (2017). Caterpillars lack a resident gut microbiome. Proc. Natl. Acad. Sci. U. S. A. 114, 9641–9646. doi: 10.1073/pnas.1707186114
Henry, L. M., Ferrari, J., and Godfray, H. C. (2015). Insect life history and the evolution of bacterial mutualism. Ecol. Lett. 18, 516–525. doi: 10.1111/ele.12425
Hird, S. M., Carstens, B. C., Cardiff, S. W., Dittmann, D. L., and Brumfield, R. T. (2014). Sampling locality is more detectable than taxonomy or ecology in the gut microbiota of the brood-parasitic Brown-headed Cowbird (Molothrus ater). PeerJ. 2:e321. doi: 10.7717/peerj.321
Homer, C. G., Dewitz, J. A., Yang, L., Jin, S., Danielson, P., Xian, G., et al. (2011). Completion of the 2006 national land cover database for the conterminous United States. Photogramm. Eng. Remote Sens. 77, 858–566.
Homer, C. H., Fry, J. A., and Barnes, C. A. (2012). The National Land Cover Database, U.S. Geological Survey Fact Sheet 2012-3020, 4. doi: 10.3133/fs20123020
Knights, D., Lassen, K. G., and Xavier, R. J. (2013). Advances in inflammatory bowel disease pathogenesis: linking host genetics and the microbiome. Gut 62, 1505–1510. doi: 10.1136/gutjnl-2012-303954
Luther, D. A., and Derryberry, E. P. (2012). Birdsongs keep pace with city life: changes in song over time in an urban songbird affects communication. Anim. Behav. 83, 1059–1066. doi: 10.1016/j.anbehav.2012.01.034
Mazerolle, M. J. (2016). AICcmodavg: Model Selection and Multimodel Inference Based on (Q)AIC(c). R package version 2.0-4. 1–158. Available online at: https://cran.r-project.org/web/packages/AICcmodavg/citation.html
McClintock, E. (2001). The Trees of Golden Gate Park and San Francisco. ed. R. G. J. Turner Berkeley (Berkeley, CA: Heydey Books), 244.
McDonald, D., Price, M. N., Goodrich, J., Nawrocki, E. P., Desantis, T. Z., Probst, A., et al. (2012). An improved Greengenes taxonomy with explicit ranks for ecological and evolutionary analyses of bacteria and archaea. ISME J. 6, 610–618. doi: 10.1038/ismej.2011.139
McFall-Ngai, M., Hadfield, M. G., Bosch, T. C., Carey, H. V., Domazet-Lošo, T., Douglas, A. E., et al. (2013). Animals in a bacterial world, a new imperative for the life sciences. Proc. Natl. Acad. Sci. U. S. A. 110, 3229–3236. doi: 10.1073/pnas.1218525110
Morelli, F., Beim, M., Jerzak, L., Jones, D., and Tryjanowski, P. (2014). Can roads, railways and related structures have positive effects on birds? – a review. Transp. Res. Part D Transp. Environ. 30, 21–31. doi: 10.1016/j.trd.2014.05.006
Morton, E. S. (1975). Ecological sources of selection on avian sounds. Am. Nat. 109, 17–34. doi: 10.1086/282971
Morton, M. L. (1992). Effects of sex and birth date on premigration biology, migration schedules, return rates and natal dispersal in the mountain white-crowned sparrow. Condor 94, 117–133. doi: 10.2307/1368802
Paker, Y., Yom-Tov, Y., Alon-Mozes, T., and Barnea, A. (2014). The effect of plant richness and urban garden structure on bird species richness, diversity and community structure. Landsc. Urban Plan. 122, 186–195. doi: 10.1016/j.landurbplan.2013.10.005
Parris, K. M., Velik-Lord, M., and North, J. M. (2009). Frogs call at a higher pitch in traffic noise. Ecol. Soc. 14:25. doi: 10.5751/ES-02687-140125
Peig, J., and Green, A. J. (2009). New perspectives for estimating body condition from mass/length data: the scaled mass index as an alternative method. Oikos 118, 1883–1891. doi: 10.1111/j.1600-0706.2009.17643.x
Phillips, J. N., and Derryberry, E. P. (2017). Equivalent effects of bandwidth and trill rate: support for a performance constraint as a competitive signal. Anim. Behav. 132, 209–215. doi: 10.1016/j.anbehav.2017.08.012
Phillips, J. N., and Derryberry, E. P. (2018). Urban sparrows respond to a sexually selected trait with increased aggression in noise. Sci. Rep. 8:7505. doi: 10.1038/s41598-018-25834-6
Phillips, J. N., Gentry, K. E., Luther, D. L., and Derryberry, E. P. (2018). Surviving in the city: higher apparent survival for urban birds but worse condition on noisy territories. Ecosphere e02440.
Price, M. N., Dehal, P. S., and Arkin, A. P. (2010). FastTree 2 - approximately maximum-likelihood trees for large alignments. PLoS ONE 5:e9490. doi: 10.1371/journal.pone,.0009490
R Development Core Team (2015). R: A Language and Environment for Statistical Computing. Vienna: R Foundation for Statistical Computing. Available online at: https://www.R-project.org/
Robb, G. N., McDonald, R. A., Chamberlain, D. E., and Bearhop, S. (2008). Food for thought: supplementary feeding as a driver of ecological change in avian populations. Front. Ecol. Environ. 6, 476–484. doi: 10.1890/060152
Rosen, M. J., Callahan, B. J., Fisher, D. S., and Holmes, S. P. (2012). Denoising PCR-amplified metagenome data. BMC Bioinformatics 13:283. doi: 10.1186/1471-2105-13-283
Ruiz-Rodríguez, M., Martín-Vivaldi, M., Martínez-Bueno, M., and Soler, J. J. (2018). Gut microbiota of great spotted cuckoo nestlings is a mixture of those of their foster magpie siblings and of cuckoo adults. Genes 9:381. doi: 10.3390/genes9080381
Ruokolainen, L., Ikonen, S., Makkonen, H., and Hanski, I. (2016). Larval growth rate is associated with the composition of the gut microbiota in the Glanville fritillary butterfly. Oecologia 181, 895–903. doi: 10.1007/s00442-016-3603-8
Scales, J., Hyman, J., and Hughes, M. (2011). Behavioral syndromes break down in urban song sparrow populations. Ethology 117, 887–895. doi: 10.1111/j.1439-0310.2011.01943.x
Scales, J., Hyman, J., and Hughes, M. (2013). Fortune favours the aggressive: territory quality and behavioural syndromes in song sparrows, Melospiza melodia. Anim. Behav. 85, 441–451. doi: 10.1016/j.anbehav.2012.12.004
Sharpton, T. J. (2014). An introduction to the analysis of shotgun metagenomic data. Front. Plant Sci. 5:209. doi: 10.3389/fpls.2014.00209
Slabbekoorn, H. (2013). Songs of the city : noise-dependent spectral plasticity in the acoustic phenotype of urban birds. Anim. Behav. 85, 1089–1099. doi: 10.1016/j.anbehav.2013.01.021
Sylvia, K. E., and Demas, G. E. (2018). A gut feeling: microbiome-brain-immune interactions modulate social and affective behaviors. Horm. Behav. 99, 41–49. doi: 10.1016/j.yhbeh.2018.02.001
Sylvia, K. E., Jewell, C. P., Rendon, N. M., St. John, E. A., and Demas, G. E. (2017). Sex-specific modulation of the gut microbiome and behavior in Siberian hamsters. Brain. Behav. Immun. 60, 51–62. doi: 10.1016/j.bbi.2016.10.023
Teyssier, A., Saleh Hudin, N., Strubbe, D., Matthysen, E., Lens, L., et al. (2018). Inside the guts of the city : urban-induced alterations of the gut microbiota in a wild passerine. Sci. Total Environ. 612, 1276–1286. doi: 10.1016/j.scitotenv.2017.09.035
Theimer, T. C., Clayton, A. C., Martinez, A., Peterson, D. L., and Bergman, D. L. (2015). Visitation rate and behavior of urban mesocarnivores differs in the presence of two common anthropogenic food sources. Urban Ecosyst. 18, 895–906. doi: 10.1007/s11252-015-0436-x
Thieme, J. L., Rodewald, A. D., Brown, J., Anchor, C., and Gerht, S. D. (2015). Linking grassland and early successional bird territory density to predator activity in urban parks. Nat. Areas J. 35, 515–532. doi: 10.3375/043.035.0404
Tiede, J., Scherber, C., Mutschler, J., McMahon, K. D., and Gratton, C. (2017). Gut microbiomes of mobile predators vary with landscape context and species identity. Ecol. Evol. 7, 8545–8557. doi: 10.1002/ece3.3390
Tomás, G., Martín-Gálvez, D., Ruiz-Castellano, C., Ruiz-Rodríguez, M., Peralta-Sánchez, J. M., Martín-Vivaldi, M., et al. (2018). Ectoparasite activity during incubation increases microbial growth on avian eggs. Microb. Ecol. 76, 555–564. doi: 10.1007/s00248-017-1140-6
Trevelline, B. K., MacLeod, K. J., Knutie, S. A., Langkilde, T., and Kohl, K. D. (2018). In ovo microbial communities: a potential mechanism for the initial acquisition of gut microbiota among oviparous birds and lizards. Biol. Lett. 14:20180225. doi: 10.1098/rsbl.2018.0225
Turrini, T., and Knop, E. (2015). A landscape ecology approach identifies important drivers of urban biodiversity. Glob. Chang. Biol. 21, 1652–1667. doi: 10.1111/gcb.12825
Venables, W. N., and Ripley, B. D. (2002). Modern Applied Statistics With S-Plus. New York, NY: Springer-Verlag.
Vo, A. E., and Jedlicka, J. A. (2014). Protocols for metagenomic DNA extraction and Illumina amplicon library preparation for faecal and swab samples. Mol. Ecol. Resour. 14, 1183–1197. doi: 10.1111/1755-0998.12269
Walters, W. A., Xu, Z., and Knight, R. (2014). Meta-analyses of human gut microbes associated with obesity and IBD. FEBS Lett. 588, 4223–33. doi: 10.1016/j.febslet.2014.09.039
Keywords: gut microbiome, urbanization, white-crowned sparrow, landscape, diversity
Citation: Phillips JN, Berlow M and Derryberry EP (2018) The Effects of Landscape Urbanization on the Gut Microbiome: An Exploration Into the Gut of Urban and Rural White-Crowned Sparrows. Front. Ecol. Evol. 6:148. doi: 10.3389/fevo.2018.00148
Received: 29 June 2018; Accepted: 05 September 2018;
Published: 24 September 2018.
Edited by:
Federico Morelli, Czech University of Life Sciences Prague, CzechiaReviewed by:
Reuven Yosef, Ben-Gurion University of the Negev, IsraelCopyright © 2018 Phillips, Berlow and Derryberry. This is an open-access article distributed under the terms of the Creative Commons Attribution License (CC BY). The use, distribution or reproduction in other forums is permitted, provided the original author(s) and the copyright owner(s) are credited and that the original publication in this journal is cited, in accordance with accepted academic practice. No use, distribution or reproduction is permitted which does not comply with these terms.
*Correspondence: Jennifer N. Phillips, am5waGlsbGlAY2FscG9seS5lZHU=
Disclaimer: All claims expressed in this article are solely those of the authors and do not necessarily represent those of their affiliated organizations, or those of the publisher, the editors and the reviewers. Any product that may be evaluated in this article or claim that may be made by its manufacturer is not guaranteed or endorsed by the publisher.
Research integrity at Frontiers
Learn more about the work of our research integrity team to safeguard the quality of each article we publish.