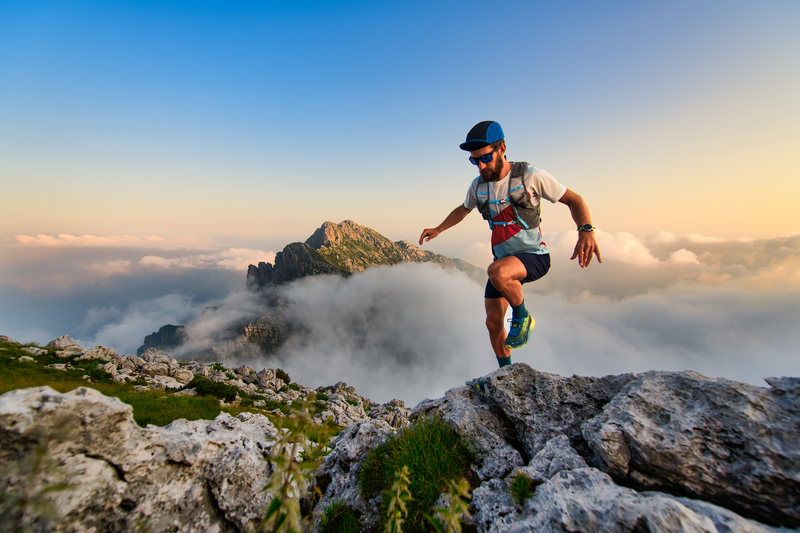
94% of researchers rate our articles as excellent or good
Learn more about the work of our research integrity team to safeguard the quality of each article we publish.
Find out more
ORIGINAL RESEARCH article
Front. Ecol. Evol. , 13 March 2018
Sec. Behavioral and Evolutionary Ecology
Volume 6 - 2018 | https://doi.org/10.3389/fevo.2018.00017
Antipredator responses may appear unsuccessful when animals are exposed to approaching vehicles, often resulting in mortality. Recent studies have addressed whether certain biological traits are associated with variation in collision risk with cars, but not with higher speed-vehicles like aircraft. Our goal was to establish the association between different species traits (i.e., body mass, eye size, brain size, wing loading, wing aspect ratio) and the frequency of bird collisions with aircraft (hereafter, bird strikes) using a comparative approach controlling for the effects of shared ancestry. We proposed directional predictions as to how each of the species traits would affect the frequency of bird strikes. Considering 39 bird species with all traits represented, the model containing wing loading had the best fit to account for the variance in bird strikes across species. In another model with 54 species exploring the fit to different polynomial models but considering only wing loading, we found that wing loading was negatively and linearly associated with the frequency of bird strikes. Counterintuitively, species with lower wing loading (hence, greater maneuverability) had a higher frequency of bird strikes. We discuss potential non-mutually exclusive explanations (e.g., high wing loading species fly faster, thus gaining some extra time to avoid the aircraft flight path; high wing loading species are hazed more intensively at airports, which could lower collisions, etc.). Ultimately, our findings uncovered that species with low wing loading get struck at a higher rate at airports, which reduces the safety risk for humans because these species tend not to cause damaging strikes, but the ecological consequences of their potentially higher local mortality are unknown.
When attacked by a predator, animals engage in antipredator behavior (e.g., crouching, dashing into a refuge, leaving a foraging patch, etc.). Different species have evolved various antipredator strategies (Caro, 2005) driven by energetic, physiological, morphological, perceptual, and cognitive species-specific constraints (Lima, 1993; Beale, 2007). For instance, birds with more pointed wings escape at greater distances than species with more rounded wings, likely because it is more difficult for the former to take off, thus escaping earlier might lower their risk of predation (Fernández-Juricic et al., 2006). Additionally, large-sized birds and lizards have been found to escape at greater distances than small-sized ones, probably because larger species have greater vulnerability, less agility to escape, etc. (Blumstein, 2006; Samia et al., 2015).
However, some of these evolved antipredator strategies have proven unsuccessful when animals encounter approaching vehicles (e.g., cars, airplanes) and attempt to evade them (Lima et al., 2015). One of the potential reasons is because the speed of the vehicles is so much higher than that of the predators with whom prey evolved (DeVault et al., 2014, 2015, 2017). The implication is that some traits that enhance the chances of survival during a predator attack may still be used by animals during a vehicle approach but may nevertheless lead to mortality. For instance, Bernhardt et al. (2010) found that birds tried to fly upwards when moving toward an aircraft and downwards when moving away from it, as they do when exposed to predators (Lima, 1993); yet they still collided with airplanes. Furthermore, birds tend to increase the escape distances on roads with higher speed limits (Legagneux and Ducatez, 2013; Husby, 2016) and increase the escape angle away from the road as the speed of the vehicles increases (Husby and Husby, 2014). Nevertheless, an increase in vehicle speed has been linked to more road kills (Farmer and Brooks, 2012). These mortality effects could pose substantial risk to populations (e.g., Bujoczek et al., 2011) and even reshape communities (e.g., Santos et al., 2016).
A central question is whether certain biological traits can increase or decrease the risk of collisions with high-speed vehicles. This question has been addressed from a comparative perspective in the context of collisions between birds and cars (Møller et al., 2011; Cook and Blumstein, 2013; Husby and Husby, 2014; Santos et al., 2016), but not between birds and aircraft. Birds and aircraft share a three dimensional space that potentially allows the former a greater degree of spatial maneuverability to engage in escape, although aircraft move much faster than cars. From 1990 through 2015, 169,856 bird-aircraft collisions (hereafter, bird strikes) were reported to the U.S. Federal Aviation Administration (FAA) (Dolbeer et al., 2016). Bird strikes are a far-reaching problem involving financial loss to commercial, civil, and military fleets worldwide and a source of mortality for birds (Anderson et al., 2015; Blackwell et al., 2016).
Our goal was to assess the association between different species traits (i.e., body mass, eye size, brain size, wing loading, wing aspect ratio) and the frequency of bird strikes. We used a comparative approach whereby we considered different species but controlled for the effects of phylogenetic relatedness between them. We collected data on the frequency of bird strikes from the FAA. We gathered information on body mass, eye size, brain size, wing loading, and wing aspect ratio from the literature based on the species with bird strike information available. In our analysis of bird strikes, we considered traits that are proxies of body size, visual acuity, cognitive ability and maneuverability, which have been previously implicated as contributing to road mortality (Møller et al., 2011; Cook and Blumstein, 2013; Husby and Husby, 2014; Santos et al., 2016).
First, we hypothesized that larger species have more mechanical and aerodynamic constraints (Norberg, 1990), which translates into higher energetic costs in taking flight (Tatner and Bryant, 1986; Møller et al., 2013), leading to longer take-off distances to escape an approaching threat (Samia et al., 2015; Husby, 2016) to reduce the likelihood of a collision (Møller et al., 2011) compared to smaller species. Additionally, large-bodied species have relatively less energetic requirements than small-bodied species (Nagy et al., 1999), which would allow the former to leave a foraging patch earlier when approached by a threat. Consequently, we predicted that species with lower body mass would have a higher frequency of bird strikes than species with higher body mass.
Second, eye size is a proxy of visual acuity or the ability to resolve an object visually from a certain distance (i.e., species with larger eyes have higher visual acuity; Kiltie, 2000). We hypothesized that species with smaller eyes would only be able to visually resolve the approaching aircraft from relatively closer distances (Blackwell et al., 2009), compared to species with larger eyes, leaving little time to avoid a collision given the fast aircraft speeds. Thus, we predicted that species with smaller eyes would experience higher frequency of bird strikes than species with larger eyes.
Third, we hypothesized that species with relatively larger brains would have enhanced cognitive abilities (Kotrschal et al., 2013) that would allow them to better assess the speed and distance of an approaching threat to minimize monitoring costs, maximize the benefits of foraging patch exploitation, but still escape successfully, compared to species with relatively smaller brains (Husby and Husby, 2014; Samia et al., 2015). This hypothesis predicts that species with larger brains would exhibit shorter escape distances. Actually, bird species with relatively larger brains tend to escape at closer distances from a threat (Møller and Erritzøe, 2014), wait longer to escape after becoming alert (Samia et al., 2015), and fly away from the road more often (Husby and Husby, 2014), compared to species with smaller brain size. Therefore, we predicted that species with smaller brains would have higher frequency of collisions with aircraft than species with larger brains, as has been found in the context of road mortality (Møller and Erritzøe, 2017).
Fourth, based on the fact that birds try to avoid collisions with aircraft by veering from their flight paths (Bernhardt et al., 2010), we hypothesized that species with morphological adaptations that enhance maneuverability would be at an advantage to engage in quick steering away (i.e., faster escape speed; Burns and Ydenberg, 2002; McFarlane et al., 2016) from an approaching vehicle to prevent a strike (Brown and Bomberger Brown, 2013; Santos et al., 2016). Two measures of wing morphology can be used as indices of maneuverability: (1) wing loading (i.e., ratio of body mass to wing area), which reflects the ability of a wing to turn relative to body mass (i.e., increasing with lower wing loadings; Lindhe Norberg, 2002); and (2) wing aspect ratio (i.e., ratio of wing span squared to wing area), which reflects the ability of a wing to quickly change direction (i.e., increasing with lower aspect ratios; Alexander, 2002). Therefore, we predicted that species with low maneuverability (high wing loadings, high aspect ratios) would have a higher frequency of bird strikes than species with higher maneuverability, as proposed for road mortality (Cousins et al., 2012; Brown and Bomberger Brown, 2013; Santos et al., 2016).
Identifying the biological traits associated with bird collisions with aircraft has important implications. First, we can better understand the combinations of morphological, sensory, and cognitive traits that can increase avian mortality at local levels (i.e., airfields). Second, this information can help inform practitioners about conservation priorities in areas with high traffic levels (e.g., airports, highways; Lima et al., 2015; Blackwell et al., 2016) by targeting highly vulnerable species, ultimately optimizing limited resources instead of trying to protect species at random. Third, wildlife management on and around airports that targets those species more susceptible to collisions can reduce the threat to human safety (DeVault et al., 2013).
To develop our species list, we collected data on the frequency of bird strikes from the FAA National Wildlife Strike Database (https://wildlife.faa.gov/). This FAA database represents voluntary reporting of wildlife strikes involving civil aircraft in the USA to the FAA (since 1990). Strike reports come from pilot and crew, as well as ground crew via paper and electronic versions of the FAA form 5200-7. Strike reports are also submitted to the FAA via the Air Traffic Organization (ATO) Mandatory Occurrence Reporting system; ATO personnel are required to report all bird strikes of which they become aware (Dolbeer et al., 2016). The FAA collaborates with the Smithsonian Institution to aid in species identification of collected remains from strikes, including feather and DNA analysis (Dove et al., 2008; Marra et al., 2009; https://www.faa.gov/airports/airport_safety/wildlife/smithsonian/). Although the reporting of wildlife-aircraft collisions to the FAA is not mandatory in the USA, since 2010 reporting rates have exceeded 90% for passenger-certificated airports (Dolbeer, 2015).
We collected data on bird strikes from seven major U.S. airports that spanned the Pacific, Central, Mississippi, and Atlantic migratory flyways (Olson, 2015): Seattle-Tacoma International (47.4436°N, 122.2961°W) in Washington, Los Angeles International (LAX; 33.9416°N, 118.4085°W) in California, Denver International (39.8561°N, 104.6737°W) in Colorado, Chicago O'Hare International (41.9742°N, 87.9073°W) in Illinois, John F. Kennedy International (40.6413°N, 73.7781°W) in New York, Hartsfield-Jackson Atlanta International (33.6407°N, 84.4277°W) in Georgia, and Orlando International (28.4312°N, 81.3081°W) in Florida. We chose these airports because they covered broad regions of the USA and each experienced >17 million passenger enplanements and >300,000 aircraft movements in 2015 (Federal Aviation Administration, 2017; western airports: mean annual movements = 579, 501, SE = 247,208 movements; Midwest, Chicago O'Hare International: 878,117, SD = 5,579; eastern airports: 574,545, SE = 323,051).
Strikes reported to the FAA are inherently affected by multiple factors, including local land use, landscape composition, airport wildlife management, migration corridors, annual aircraft movements, etc. In assessing whether the strike rates in the airports studied differed from the national strike rates, we performed a correlation analysis between the number of individuals for each of the 87 species struck at our 7 airports vs. the number of individuals of the same species struck at all other airports (minus the 7 airports included in this study) reported to the FAA from June through July (2010–2015). We found a significant correlation between bird strikes at the 7 studied airports and bird strikes at all other airports (r = 0.752, P < 0.001). We concluded that the bird strikes at the 7 selected airports were representative of bird strikes in the USA at least from the perspective of the studied species.
We developed a list of bird species reported as struck from June through July (2010–2015) by accessing the FAA Wildlife Strike Database (http://wildlife.faa.gov/). We selected strikes reported from June through July, as this represented the time of year when migration in northern hemisphere was generally completed and local populations were relatively stable. In addition, this period was early enough in the breeding season to have fewer hatching year birds moving within the airspace because it is unclear how hatching year birds respond to novel risk like aircraft approach. Also, there is a strong pattern of increase in strikes in the northern hemisphere associated with species population peaks in late summer following reproduction (Dolbeer and Franklin, 2013).
To maintain a perspective on possible management implications, we included only strikes identified to species that occurred ≤457 m above ground level (AGL), thus within ~8 km of the airport runways for aircraft on final approach or initial climb (Dolbeer and Begier, 2012). The FAA recommends that this 8-km zone be managed to reduce attractants to species (primarily birds) recognized as hazardous to aviation (Federal Aviation Administration, 2007). Further, 82% of strikes (1990–2015) occurred at or below 457 m AGL. We also included species whose carcasses were recovered on or within close proximity to runway surfaces and deemed by airport personnel to have been struck by an aircraft (e.g., Blackwell and Wright, 2006; DeVault et al., 2011; see also Federal Aviation Administration, 2013).
We used the total number of collisions per species over the six-year period and across all studied airports as our response variable (Supplementary Material). We collected data on 87 species, but eventually used fewer species for the statistical analyses due to the availability of information on the independent factors (body mass, brain mass, eye axial length, aspect ratio, and wing loading; see below for details).
We obtained body mass data from Dunning (2007). When body mass data were available for males and females in sexually dimorphic species, we used the average of the two measurements.
Eye axial length, defined as the distance between the anterior surface of the cornea and the most posterior portion of the back of the eye (Moore et al., 2012), has been shown to serve as a valid proxy of eye size (Howland et al., 2004) and visual acuity (Kiltie, 2000). We collected eye axial length measurements from a comprehensive database that contained data from Ritland (1982); Hall and Ross (2007); Hall (2008), and Hall et al. (2009). We obtained additional measurements from unpublished data by EFJ. All animal procedures were performed in accordance with the relevant guidelines and regulations and were approved by Purdue Institutional Animal Care and Use Committee (protocols 1201000567 and 1112000398). Eye size was estimated following Ullmann et al. (2012).
We collected measurements of brain mass from previously published datasets: Garamszegi et al. (2007); Sol et al. (2010); Galván and Møller (2011); Samia et al. (2015); Vincze et al. (2015); Møller and Erritzøe (2016), and Mlíkovský (1989a,b,c). Additionally, we obtained brain mass data by converting brain volume measurements (volume × density of fresh brain tissue −1.036 g/mL; Iwaniuk and Nelson, 2002) for two species (Accipiter cooperi and Chordeiles minor) from Iwaniuk and Nelson (2003). We ran the analyses with and without these two species in case the conversion might bias the dataset. The results were the same, so we decided to include these two species to increase the statistical power of our analyses.
Wing loading is traditionally measured as either the ratio of body weight to wing area (N/m2) or the ratio of body mass to wing area (kg/m2). We chose the former following Norberg and Rayner (1987) as an index of force per unit area of wing. High values of wing loading, exhibited by relatively heavy birds with relatively small wings, are associated with faster flight speeds but less maneuverability. Low values of wing loading, exhibited by relatively light birds with relatively large wings, are associated with soaring ability and increased maneuverability. Aspect ratio, defined as the ratio between the wingspan and the length of the mean wing chord, is calculated as the square of the wingspan divided by total wing area (Lindhe Norberg, 2002). Birds with high aspect ratios have relatively long and slender wings, and they are able to fly with much less drag, but at the cost of lower maneuverability. Birds with low aspect ratios have relatively short and wide wings, which induce more drag but with high maneuverability. We collected wing loading and aspect ratio data from previously published studies: Poole (1938); Nudds et al. (2007); Andrews et al. (2009), and Serrano et al. (2017).
Previous studies on road mortality have found that highly abundant species tend to collide more frequently with cars (e.g., Møller et al., 2011). However, we did not include abundance in our analysis for two reasons. First, species abundance estimates at U.S. airports are not standardized because of different survey methods that do not correct for detection bias (Blackwell et al., 2013). Therefore, across-airport species relative abundance estimates for a specified time interval, though important, are hampered by biases associated with breadth and periodicity of surveys as well as variation in detection probabilities. Second, in an different study, we estimated the role of relative airport abundance on bird strike frequency for 23 bird species across 7 US airports, and found no significant effect (Blackwell et al. unpubl. ms.).
Aircraft speed and altitude at the moment of a collision with a bird could be potential confounding factors because any association between the biological traits studied and the frequency of bird collisions could be influenced by different species being struck at different altitudes and speeds. The FAA database we used to estimate the frequency of bird collisions also provides some information about the altitude and aircraft speed related to the bird strike. However, this information has some limitations because (a) it is based on estimates from the pilots or airport biologists rather than specific measurements, and (b) it is not reported consistently. Therefore, the number of records on bird strike altitude and aircraft speed is much lower than the available strike records per species. In the specific case of aircraft altitude, for instance, a 0 value in the database can indicate that the bird was struck when the aircraft was close to touch down or on the ground (e.g., prior to rotation on takeoff, landing roll, taxiing, or at a hold position awaiting instructions). Further, if the body of the bird was found on the runway (noted in the FAA database as “carcass found”), the altitude of the strike and airspeed at the time of the strike are unknown. This lack of data on altitude of strikes and aircraft speed at the time of strike limited our ability to include these parameters in the analyses. Nevertheless, we conducted some analyses to see if there were differences in aircraft altitude and speed between species, including those with at least 4 records in the database (i.e., we excluded altitude = 0 for the aforementioned reasons). We did not find significant differences between species in aircraft altitude [F(5, 36) = 1.73, P = 0.153, 6 species that met the criteria] and aircraft speed [F(9, 101) = 1.25, P = 0.272, 10 species that met the criteria]. Despite the limitations of the dataset, we believe that the potential between-species bias in the altitude and speed of the collisions might not be substantial in our findings.
We followed a two-tiered approach. First, we used multimodel inference to determine which of the independent factors would better account for the variation in bird strikes of 38 bird species, controlling for the effects of phylogenetic relatedness. We used this reduced dataset, which was a subset of the larger dataset we collected (Supplementary Material), because we only had information for each independent variable for 38 species. Based on this first analysis, we identified a single independent factor as the main contributor to the variation in bird strikes (see Results). Second, to corroborate the role of this independent factor, we ran another model controlling for the effects of phylogenetic relatedness with a larger dataset comprising 54 bird species to maximize power in our analysis (i.e., we had information on this specific independent factor for 54 species). We also assessed the best fit function to obtain a predictive equation that could be used as a predictor of potential risk of collisions based on variations in this independent factor.
We utilized a Phylogenetic Generalized Least Squares (PGLS) approach to account for the non-independence problems that arise from analyzing multiple species which have a shared evolutionary history (Nunn, 2011). We used BirdTree.org (Jetz et al., 2012) to download 2,000 phylogenetic trees for the 38 species. BirdTree.org phylogenies provide a valid and accurate phylogenetic background for statistical analyses (Rubolini et al., 2015). According to Rubolini et al. (2015), sampling at least 1,000 trees minimizes variation for parameter estimates in a phylogenetic analysis. We then sampled 2,000 trees to enhance the precision of our phylogeny. We built a consensus tree by using the sumtrees function from the DendroPy package in Python (Sukumaran and Holder, 2010), using the 50% majority method for splits and using the first 200 trees as a burn-in set. All trees were treated as unrooted. The branch lengths of the consensus tree were assigned to be the mean of the corresponding input tree branch lengths.
We used Information Theory and Akaike's Information Criterion (AIC; Akaike, 1973) as a model fitting procedure following Garamszegi and Mundry (2014). In a regression analysis of fixed sample size, AIC values vary from model to model only as a function of model fit and number of model parameters (Burnham and Anderson, 2002). AIC values assess how well a model fits the data, and are calculated as AIC = –ln(L) + 2k, where L was the maximum likelihood estimate for the model and k was the number of parameters in the model. However, for finite samples like ours, a slightly modified version of AIC, called AICc, is recommended (Garamszegi and Mundry, 2014). We thus calculated AICc as: AICc = AIC + , where k is the number of model parameters as noted above, and n is the sample size. Smaller AICc values indicate better model fit.
We also calculated the AICc differences (Δi) for each model as the difference between the AICc value for a given model and that of the model with the minimum AICc of the whole set of models. We then used the Δi to calculate other inferential metrics: Akaike weights (wi) and Evidence Ratios (ER). Akaike weights (wi) were calculated as: and can be interpreted as the probability that the specified model is the best model of the set of models (Burnham and Anderson, 2002). Evidence Ratios were calculated as: , and are a measure of how much more likely the top model of the set of models is compared to a given competing model. Additionally, we computed the relative importance score of each independent factor by summing wi up the Akaike weights from each model where that factor appeared. For example, body mass appeared as an independent factor in eight of our candidate models, so its variable importance score was the sum of the Akaike weights for those eight models. We used the relative importance scores to rank and compare the independent factors in terms of the probability that each belongs in the best fit model (Symonds and Moussalli, 2011). We calculated AICc, Δi, Akaike weights, and Evidence Ratios of 32 candidate models (summarized in Table 1), which represented every possible combination of our five independent factors.
Table 1. Summary of 32 candidate models assessing the effects of body mass (BoM), eye axial length (EAL), brain mass (BrM), wing loading (WL), and wing aspect ratio (AR) on the frequency of bird strikes following different criteria: Akaike's Information Criterion (corrected for sample size, AICc), AICc difference (Δi), Akaike weight (wi), and Evidence Ratio (ER).
We log10 transformed all of the variables to meet the normality requirements of the analysis. Some of our log-transformed independent variables (brain mass, eye axial length, and wing loading) exhibited multicollinearity due to the high degree of correlation with body mass (adjusted R2 values of 0.916, 0.791, and 0.680, respectively), which could inflate the standard error and decreases t-values for parameter estimates in the PGLS analysis (Farrar and Glauber, 1967). Including unnecessary intercorrelated variables in the model may increase the variability of the regression coefficients, diminish the model's descriptive capacity, and impair the model's predictive capability (Kutner et al., 2013). Therefore, we presented three types of model calculations to assess the consistency of our results under different levels of multicollinearity: (1) 32 models including all variables irrespective of the degree of correlation between pairs of predictors, (2) 24 models removing those that contained any two predictors with a correlation ≥0.90, and (3) 12 models removing those that contained any two predictors with a correlation ≥0.70.
Given that the first phase of our analysis identified a single independent factor as the best one to fit the variation in the bird strike database, we decided to re-run the model but including only this independent factor to add 16 more species to our dataset (total sample size, 54 species) and increase the power of our test. We followed the same approach as described above to construct a consensus phylogenetic tree and control for phylogenetic effects. We did not have any a priori prediction about the shape of this relationship, but to derive a predictive equation, we fitted polynomial models of order 1, 2, 3, and 4 to the data to explore the one with the best fit. We calculated the F and P values of each of the four polynomial models and assessed their fit with the AIC approach described above, and the adjusted R2 values. Additionally, we derived an averaged estimate of the intercept and slope across the 2,000 trees for the relationship between log(Wing Loading) and log(Strikes) following the procedure outlined in Garamszegi and Mundry (2014).
All analyses were performed in the R statistical environment (R Core Team, 2017). We utilized the caper package (Orme et al., 2013) to perform PGLS analyses, the MuMIn package (Barton, 2016) to calculate AICc information, and the ggplot2 package (Wickham, 2009) to create figures.
Based on the AICc, the model containing wing loading had the best fit to the frequency of bird strikes (AICc = 87.144, Table 1), controlling for the effects of phylogenetic relatedness across the 38 species considered. The lowest Evidence Ratio was 2.926, which means that the model containing only wing loading was nearly three times more likely than any other model considered (Table 1). Five of the top six most likely models included wing loading as a parameter (Table 1). Furthermore, variable importance scores (wing loading, 0.64; body mass, 0.33; brain mass, 0.29; eye axial length, 0.26; aspect ratio, 0.23) indicated that wing loading was nearly twice as likely as any other variable. Richards (2005) warned against discounting models with Δi < 2, but we found no such model in our analysis (Table 1). The model containing only wing loading maximized the adjusted R2 (Table 1). In general, species with higher wing loading were associated with a lower frequency of bird strikes (Figure 1A), although the proportion of the variability explained was relatively low (~11%).
Figure 1. Relationship between wing loading and the frequency of bird strikes based on (A) 38 bird species, and (B) 54 bird species controlling for the effects of phylogenetic relatedness. Shown in (B) are two models that significantly fit the data: linear in black (y = ax+b) and polynomial of order 4 in blue (y = ax4+bx3+cx2+dx+e). See text for details.
These results were consistent when removing pairs of parameters that had different levels of multicollinearity. Tables 2, 3 present the scenarios in which models with pairs of variables with a correlation ≥ 0.90 and ≥ 0.70, respectively, were removed. In both cases, the model with only wing loading remained the best model because it had the highest Akaike weight, it had the lowest Evidence Ratio (about 3), which means the wing loading model was about 3 times more likely than any other model considered, it maximized the adjusted R2, and there were no models with Δi < 2. We present model averaged coefficients with confidence intervals in each of the three scenarios considered in Table 4. In all scenarios, wing loading had the highest variable importance.
Table 2. Summary of 24 candidate models (but excluding the ones in which pairs of variables had correlations ≥ 0.90) assessing the effects of body mass (BoM), eye axial length (EAL), brain mass (BrM), wing loading (WL), and wing aspect ratio (AR) on the frequency of bird strikes following different criteria: Akaike's Information Criterion (corrected for sample size, AICc), AICc difference (Δi), Akaike weight (wi), and Evidence Ratio (ER).
Table 3. Summary of 24 candidate models (but excluding the ones in which pairs of variables had correlations ≥ 0.70) assessing the effects of body mass (BoM), eye axial length (EAL), brain mass (BrM), wing loading (WL), and wing aspect ratio (AR) on the frequency of bird strikes following different criteria: Akaike's Information Criterion (corrected for sample size, AICc), AICc difference (Δi), Akaike weight (wi), and Evidence Ratio (ER).
Table 4. Model conditional average estimate of each parameter coefficient in each of the three scenarios considered: (A) 32 models including all variables irrespective of the degree of correlation between pairs of predictors, (B) 24 models removing those that contained any two predictors with a correlation ≥ 0.90, and (C) 12 models removing those that contained any two predictors with a correlation ≥ 0.70.
Based on the results from the multimodal inference approach, we ran the model again, but with wing loading as the only independent factor, which allowed us to consider 54 species, and fitted different polynomials (Table 5). The two models that were significant were the linear model (y = ax+b) and the polynomial model of order 4 (y = ax4+bx3+cx2+dx+e) (Table 5, Figure 1B). Both of these models also yielded all their parameters significant (Table 5). Based on the AICc, the linear model had a slightly better fit than the polynomial of order 4, but the Δi < 2 (Table 5). However, based on the Evidence Ratios, the linear model was twice as likely to be the best-approximating model as the polynomial of order 4 model (Table 5). Therefore, we considered that wing loading was significantly and negatively associated with the number of bird strikes in a linear fashion (Figure 1B). Across the 2,000 trees, the averaged intercept was 1.902 (95% CI: 0.474, 3.329) and the averaged slope was −0.774 (95% CI: −1.675, 0.127).
Table 5. Summary of 4 candidate models with different polynomial order assessing the effects of wing loading on the frequency of bird strikes following different criteria: F and P values, Akaike's Information Criterion (corrected for sample size, AICc), AICc difference (Δi), Akaike weight (wi), and Evidence Ratio (ER).
Using the estimates from the linear regression and reversing the transformations on the variables, we obtained the following approximate equation:
Our results show that of the five traits considered, only wing loading provided the best fit relative to the frequency of bird strikes. However, this association was counter to our prediction in that species with lower wing loading (hence, higher maneuverability) were actually more frequently struck. We discuss this unexpected finding and derive some applied implications.
Our analyses indicated that species with higher maneuverability collided with aircraft more often on the airfield and nearby airport property than species with lower maneuverability. However, we caution that this result although significant explained a low proportion of variability in bird strikes (~11%). From the perspective of birds trying to avoid a collision by changing flight direction very quickly and sharply (Bernhardt et al., 2010) or trying to take-off quickly and at a sharp angle from the ground or perching position (Witter et al., 1994; Kullberg et al., 1996; Lind et al., 1999; McFarlane et al., 2016), this finding might seem counterintuitive. Yet, Santos et al. (2016) also found a similar trend (i.e., positive association between bird collisions with cars and wing loading) relative to road kills.
Interestingly, when we take the perspective of the speed of the approaching aircraft (i.e., most common commercial jets exceed 240 km/h), a different interpretation is plausible. Higher wing loading is positively associated with avian flying airspeed (Alerstam et al., 2007; Serrano et al., 2017), and wing loading has been found to be a better predictor of flight speed than body mass (Greenewalt, 1975; Lighthill, 1977). Thus, the species that could achieve higher flight speeds (i.e., higher wing loading) were those that we found to have lower frequency of collisions with aircraft. Assuming that aircraft detection occurred above a certain distance threshold for all the species (i.e., the spatial margin of safety was not compromised; DeVault et al., 2015), it is reasonable to speculate that species that tended to fly at higher speeds might have had extra time to engage in evasive maneuvers (e.g., climbing, diving, steering away from the flying path horizontally). This finding suggests that the speed at which species can fly can be an indicator of vulnerability to bird strike occurrence.
We can also identify at least four other (non-mutually exclusive) alternative interpretations. First, species with higher wing loading might have started evasive maneuvers at longer distances (irrespective of flight speed), in the same way as species with higher body mass initiate escape from predators at longer flight-initiation distances (e.g., Blumstien et al., 2005). Second, species with high wing loadings have higher take-off costs (Alexander, 2002); consequently, it is possible that these species decided to stay put, avoid escaping, and thus reduced their chances of being struck. This explanation would be particularly applicable to situations in which the animal was on the ground or perching away from the flight path of the aircraft. Third, the studied airports have Wildlife Hazard Management Plans (U.S. Code of Federal Regulations Part 139.337; Cleary and Dolbeer, 2005) in place, and species that pose known strike hazards to aircraft (Dolbeer et al., 2000; DeVault et al., 2011) are targeted for hazing and, in some instances, lethal control (Dolbeer and Franklin, 2013). Upon examination of the identity of the species with high wing loading (Supplementary Material), many of those species were previously deemed to have the highest probability of causing damaging strikes (DeVault et al., 2011). Therefore, it is possible that our findings reflect the result of species with high wing loading being hazed more intensively and, hence, having lower strike frequencies. Further, as we noted earlier, the role of species abundance in strike rates remains an important question, but one for which objective data across airports are not standardized. As such, we cannot discount the role of local airport abundances on strike frequency (i.e., the likelihood of an aircraft striking birds), nor should we necessarily attribute it undue weight. For instance, higher abundance of birds at airports might also lead to a higher likelihood of detecting approaching aircraft (via collective detection) and ultimately lower chances of individual birds colliding with aircraft because of the dilution effect (Krause and Ruxton, 2002).
Fourth, species with low wing loading tended to be species with relatively higher energetic requirements (Supplementary Material; Nagy et al., 1999). Therefore, we would expect species with low wing loading to have higher activity levels and consequently spend more time in the air (i.e., looking for food patches, traveling between food patches, etc.). This enhanced activity, regardless of abundance, could increase the chances of colliding with aircraft because of the high volume of air traffic.
In our analyses, none of the other factors considered (body mass, eye size, brain size, wing aspect ratio) had a strong enough signal to fit the data with the sample size used. This does not necessarily mean that these biological traits are not relevant in making a species more or less prone to bird strikes. However, despite the classic sources of noise in a comparative analysis, wing loading explained a significant proportion of the variation (despite the relatively low amount of variability explained).
One of the implications of our findings is that high maneuverability might not be enough to reduce the chances of collisions with aircraft because of their high speeds. From a management perspective, our findings suggest that those species capable of causing damage to the aircraft (see Dolbeer et al., 2000; i.e., species with high wing loading) are involved in fewer collisions. This finding, however, does not indicate that species with high wing loading necessarily pose a lower strike risk (a metric composed of the likelihood of a strike and the likelihood of damage associated with striking a particular species; DeVault et al., in press). Further, our findings do not indicate how particular species react to aircraft approach. Such reactions themselves, despite strike frequencies, can enhance strike risk. From an ecological perspective, the higher mortality of species with low wing loading begets the question as to whether airports are suitable habitats for these species (Blackwell et al., 2013). Addressing this question is certainly important but it would require a coordinated effort to collect information on abundance, foraging ecology, and breeding success at airports compared to other areas with similar habitat properties. Even if airports are found to be population sinks, the effects might still not be relevant at the regional scale, which would also require empirical testing.
EF-J co-developed the idea for this research with BB. BB obtained species bird-aircraft strike data, and JB and EF-J collected trait data from the literature. JB conducted, in collaboration with EF-J, the statistical analyses. EF-J, JB, and BB interpreted the results and drafted the manuscript. TS assisted in data collection, interpreting results, and drafting the manuscript. TD assisted in interpreting the results and drafting the manuscript.
The authors declare that the research was conducted in the absence of any commercial or financial relationships that could be construed as a potential conflict of interest.
This study was funded by USDA APHIS and the Federal Aviation Administration.
The Supplementary Material for this article can be found online at: https://www.frontiersin.org/articles/10.3389/fevo.2018.00017/full#supplementary-material
Akaike, H. (1973). “Information theory as an extension of the maximum likelihood principle,” in Second International Symposium on Information Theory, eds B. N. Petrov and F. Csaki (Budapest: Akaemiai Kiado), 267–281.
Alerstam, T., Rosén, M., Bäckman, J., Ericson, P. G. P., and Hellgren, O. (2007). Flight speeds among bird species: allometric and phylogenetic effects. PLoS Biol. 5:e197. doi: 10.1371/journal.pbio.0050197
Alexander, D. E. (2002). Nature's Flyers: Birds, Insects, and the Biomechanics of Flight. Baltimore; London: The John Hopkins University Press.
Anderson, A., Carpenter, D. S., Begier, M. L., Blackwell, B. F., DeVault, T. L., and Shwiff, S. A. (2015). Modeling the cost of bird strikes to US civil aircraft using a two-part regression model. Transport. Res. Part D 38, 49–58. doi: 10.1016/j.trd.2015.04.027
Andrews, C. B., Mackenzie, S. A., and Gregory, T. R. (2009). Genome size and wing parameters in passerine birds. Proc. R. Soc. B 276, 55–61. doi: 10.1098/rspb.2008.1012
Beale, C. M. (2007). The behavioral ecology of disturbance responses. Int. J. Comp. Psychol. 20, 111–120. Available online at: https://escholarship.org/uc/item/43m7b2d5
Bernhardt, G. E., Blackwell, B. F., DeVault, T. L., and Kutschbach-Brohl, L. (2010). Fatal injuries to birds from collisions with aircraft reveal anti-predator behaviours. Ibis 152, 830–834. doi: 10.1111/j.1474-919X.2010.01043.x
Blackwell, B. F., DeVault, T. L., Fernández-Juricic, E., Gese, E., Gilbert-Norton, L., and Breck, S. (2016). No single solution: application of conservation behaviour principles in mitigating human-wildlife conflict. Anim. Behav. 120, 245–254. doi: 10.1016/j.anbehav.2016.07.013
Blackwell, B. F., Fernández-Juricic, E., Seamans, T. W., and Dolan, T. (2009). Avian visual system configuration and behavioural response to object approach. Anim. Behav. 77, 673–684. doi: 10.1016/j.anbehav.2008.11.017
Blackwell, B. F., Seamans, T. W., Schmidt, P., DeVault, T. L., Belant, J. L., Whittingham, M. J., et al. (2013). A framework for managing airport grasslands and birds amidst conflicting priorities. Ibis 155, 189–193. doi: 10.1111/ibi.12011
Blackwell, B. F., and Wright, S. E. (2006). Collisions of red-tailed hawks (Buteo jamaicensis), turkey (Cathartes aura), and black vultures (Coragyps atratus) with aircraft: implications for bird strike reduction. J. Raptor Res. 40, 76–80. doi: 10.3356/0892-1016(2006)40[76:CORHBJ]2.0.CO;2
Blumstein, D. T. (2006). Developing an evolutionary ecology of fear: how life history and natural history traits affect disturbance tolerance in birds. Anim. Behav. 71, 389–399. doi: 10.1016/j.anbehav.2005.05.010
Blumstien, D. T., Fernández-Juricic, E., Zollner, P. A., and Garity, S. C. (2005). Inter-specific variation in avian responses to human disturbance. J. Appl. Ecol. 42, 943–953. doi: 10.1111/j.1365-2664.2005.01071.x
Brown, C. R., and Bomberger Brown, M. (2013). Where has all the road kill gone? Curr. Biol. 23, R233–R234. doi: 10.1016/j.cub.2013.02.023
Bujoczek, M., Ciach, M., and Yosef, R. (2011). Road-kills affect avian population quality. Biol. Conserv. 144, 1036–1039. doi: 10.1016/j.biocon.2010.12.022
Burnham, K. P., and Anderson, D. R. (2002). Model Selection and Multimodel Inference, 2nd Edn. New York, NY: Springer.
Burns, J. G., and Ydenberg, R. C. (2002). The effects of wing loading and gender on the escape flights of least sandpipers (Calidris minutilla) and western sandpipers (Calidris mauri). Behav. Ecol. Sociobiol. 52, 128–136. doi: 10.1007/s00265-002-0494-y
Caro, T. (2005). Antipredator Defenses in Birds and Mammals. Chicago, IL: University of Chicago Press.
Cleary, E. C., and Dolbeer, R. A. (2005). Wildlife Hazard Management at Airports, 2nd Edn. Federal Aviation Administration, Office of Airport Safety and Standards, Airport Safety and Compliance Branch, Washington, DC.
Cook, T. C., and Blumstein, D. (2013). The omnivore's dilemma: diet explains variation in vulnerability to vehicle collision mortality. Biol. Conserv. 167, 310–315. doi: 10.1016/j.biocon.2013.08.016
Cousins, R. A., Battley, P. F., Gartrell, B. D., and Powlesland, R. G. (2012). Impact injuries and probability of survival in a large semiurban endemic pigeon in New Zealand, Hemiphaga Novaeseelandiae. J. Wildl. Dis. 48, 567–574. doi: 10.7589/0090-3558-48.3.567
DeVault, T. L., Belant, J. L., Blackwell, B. F., and Seamans, T. W. (2011). Interspecific variation in wildlife hazards to aircraft: implications for airport wildlife management. Wildlife Soc. B. 35, 394–402. doi: 10.1002/wsb.75
DeVault, T. L., Blackwell, B. F., and Belant, J. L. (2013). Wildlife in Airport Environments: Preventing Animal-Aircraft Collisions through Science-Based Management. Baltimore, MD: The Johns Hopkins University Press.
DeVault, T. L., Blackwell, B. F., Seamans, T. W., Begier, M. J., Kougher, J. D., Washburn, J. E., et al. (in press). Estimating interspecific economic risk of bird strikes with aircraft. Wildl. Soc. Bull. doi: 10.1002/wsb.859.
DeVault, T. L., Blackwell, B. F., Seamans, T. W., Lima, S. L., and Fernández-Juricic, E. (2014). Effects of vehicle speed on flight initiation by turkey vultures: implications for bird-vehicle collisions. PLoS ONE 9:e87944. doi: 10.1371/journal.pone.0087944
DeVault, T. L., Blackwell, B. F., Seamans, T. W., Lima, S. L., and Fernández-Juricic, E. (2015). Speed kills: ineffective avian escape responses to oncoming vehicles. Proc. R. Soc. B 282:20142188. doi: 10.1098/rspb.2014.2188
DeVault, T. L., Seamans, T. W., Blackwell, B. F., Lima, S. L., Martinez, M. A., and Fernández-Juricic, E. (2017). Can experience reduce collisions between birds and vehicles? J. Zool. 301, 17–22. doi: 10.1111/jzo.12385
Dolbeer, R. A. (2015). Trends in Reporting of Wildlife Strikes with Civil Aircraft and in Identification of Species Struck under a Primarily Voluntary Reporting System, 1990-2013. Special report submitted to the U.S. Department of Transportation, Federal Aviation.
Dolbeer, R. A., and Begier, M. J. (2012). “Comparison of wildlife data among airports to improve aviation safety,” in Proceeding 30th International Bird Strike Conference, (Stavanger), 26–29.
Dolbeer, R. A., and Franklin, A. B. (2013). “Population management to reduce the risk of wildlife-aircraft collisions,” in Wildlife in Airport Environments: Preventing Animal-Aircraft Collisions through Science-Based Management, eds T. L. DeVault, B. F. Blackwell, and J. L. Belant (Baltimore, MD: The Wildlife Society and Johns Hopkins University Press), 67–78.
Dolbeer, R. A., Wright, S. E., and Cleary, E. C. (2000). Ranking the hazard level of wildlife species to aviation. Wildlife Soc. B. 28, 372–378. doi: 10.2307/3783694
Dolbeer, R. A., Wright, S. E., Weller, J., Anderson, A. L., and Begier, M. J. (2016). Wildlife Strikes to Civil Aircraft in the United States 1990-2015. U.S. Department of Transportation, Federal Aviation Administration, Office of Airport Safety and Standards, Serial Report 22, Washington, DC.
Dove, C. J., Rotzei, N. C., Heacker, M., and Weigt, L. A. (2008). Using DNA barcodes to identify bird species involved in birdstrikes. J. Wildl. Manag. 72, 1231–1236. doi: 10.2193/2007-272
Farmer, R. G., and Brooks, R. J. (2012). Integrated risk factors for vertebrate roadkill in Southern Ontario. J. Wildl. Manag. 76, 1215–1224. doi: 10.1002/jwmg.358
Farrar, D. E., and Glauber, R. R. (1967). Multicollinearity in regression analysis: the problem revisited. Rev. Econ. Stat. 49, 92–107. doi: 10.2307/1937887
Federal Aviation Administration (2007). Advisory Circular 150/5200-33B–Hazardous Wildlife Attractants on Or Near Airports. Available online at: https://www.faa.gov/regulations_policies/advisory_circulars/index.cfm/go/document.information/documentID/22820
Federal Aviation Administration (2013). Advisory Circular 150/5200-32B–Reporting Wildlife Aircraft Strikes. Available online at: https://www.faa.gov/documentLibrary/media/Advisory_Circular/150_5200_32b.pdf
Federal Aviation Administration (2017). Terminal Area Forecast. Available online at: http://taf.faa.gov/. (Accessed April 16, 2017).
Fernández-Juricic, E., Blumstein, D. T., Abrica, G., Manriquez, L., Bandy Adams, L., Adams, R., et al. (2006). Relationships of anti-predator escape and post-escape responses with body mass and morphology: a comparative avian study. Evol. Ecol. Res. 8, 731–752.
Galván, I., and Møller, A. P. (2011). Brain size and the expression of pheomelanin-based colour in birds. J. Evol. Biol. 24, 999–1006. doi: 10.1111/j.1420-9101.2011.02232.x
Garamszegi, L. Z., Biard, C., Eens, M., Møller, A. P., Saino, N., and Surai, P. (2007). Maternal effects and the evolution of brain size in birds: overlooked developmental constraints. Neurosci. Biobehav. Rev. 31, 498–515. doi: 10.1016/j.neubiorev.2006.11.008
Garamszegi, L. Z., and Mundry, R. (2014). “Multimodel-inference in comparative analyses,” in Modern Phylogenetic Comparative Methods and Their Application in Evolutionary Biology: Theory and Practice, ed L. Z. Garamszegi (Berlin: Springer-Verlag), 305–331.
Greenewalt, C. H. (1975). The flight of birds. Trans. Am. Phil. Soc. New Series 65, 1–67. doi: 10.2307/1006161
Hall, M. I. (2008). The anatomical relationships between the avian eye, orbit and sclerotic ring: implications for inferring activity patterns in extinct birds. J. Anat. 212, 781–794. doi: 10.1111/j.1469-7580.2008.00897.x
Hall, M. I., Iwaniuk, A. N., and Gutiérrez-Ibáñez, C. (2009). Optic foramen morphology and activity pattern in birds. Anat. Rec. 292, 1827–1845. doi: 10.1002/ar.21007
Hall, M. I., and Ross, C. F. (2007). Eye shape and activity pattern in birds. J. Zool. 271, 437–444. doi: 10.1111/j.1469-7998.2006.00227.x
Howland, H. C., Merola, S., and Basarab, J. R. (2004). The allometry and scaling of the size of vertebrate eyes. Vision Res. 44, 2043–2065. doi: 10.1016/j.visres.2004.03.023
Husby, A., and Husby, M. (2014). Interspecific analysis of vehicle avoidance behavior in birds. Behav. Ecol. 25, 504–508. doi: 10.1093/beheco/aru011
Iwaniuk, A. N., and Nelson, J. E. (2002). Can endocranial volume be used as an estimate of brain size in birds? Can. J. Zool. 80, 16–23. doi: 10.1139/z01-204
Iwaniuk, A. N., and Nelson, J. E. (2003). Developmental differences are correlated with relative brain size in birds: a comparative analysis. Can. J. Zool. 81, 1913–1928. doi: 10.1139/z03-190
Jetz, W., Thomas, G. H., Joy, J. B., Hartmann, K., and Mooers, A. O. (2012). The global diversity of birds in space and time. Nature 491, 444–448. doi: 10.1038/nature11631
Kiltie, R. A. (2000). Scaling of visual acuity with body size in mammals and birds. Funct. Ecol. 14, 226–234. doi: 10.1046/j.1365-2435.2000.00404.x
Kotrschal, A., Rogell, B., Bundsen, A., Svensson, B., Zajitschek, S., Brännström, I., et al. (2013). Artificial selection on relative brain size in the guppy reveals costs and benefits of evolving a larger brain. Curr. Biol. 23, 168–171. doi: 10.1016/j.cub.2012.11.058
Kullberg, C., Fransson, T., and Jacobsson, S. (1996). Impaired predator evasion in fat blackcaps (Sylvia atricapilla). Proc. R. Soc. B 263, 1671–1675. doi: 10.1098/rspb.1996.0244
Kutner, M. H., Nachtshiem, C. J., Neter, J., and Li, W. (2013). Applied Linear Statistical Models. New Delhi: McGraw-Hill Education (India).
Legagneux, P., and Ducatez, S. (2013). European birds adjust their flight initiation distance to road speed limits. Biol. Lett. 9:20130417. doi: 10.1098/rsbl.2013.0417
Lighthill, J. (1977). “Introduction to the scaling of animal locomotion,” in Scale Effects of Animal Locomotion, ed T. J. Pedley (New York, NY: Academic Press), 365–404.
Lima, S. L. (1993). Ecological and evolutionary perspectives on escape from predatory attack: a survey of North American birds. Wilson Bull. 105, 1–215.
Lima, S. L., Blackwell, B. F., DeVault, T. L., and Fernández-Juricic, E. (2015). Animal reactions to oncoming vehicles: a conceptual review. Biol. Rev. 90, 60–76. doi: 10.1111/brv.12093
Lind, J., Fransson, T., Jacobsson, S., and Kullberg, C. (1999). Reduced take-off ability in robins due to migratory fuel load. Behav. Ecol. Sociobiol. 46, 65–70. doi: 10.1007/s002650050593
Marra, P. P., Dove, C. J., Dolbeer, R. A., Dahlan, N. F., Heacker, M., Whatton, J. F., et al. (2009). Migratory Canada geese cause crash of US Airways Flight 1549. Front. Ecol. Environ. 7, 297–301. doi: 10.1890/090066
McFarlane, L., Altringham, J. D., and Askew, G. N. (2016). Intra-specific variation in wing morphology and its impact on takeoff performance in blue tits (Cyanistes caeruleus) during escape flights. J. Exp. Biol. 219, 1369–1377. doi: 10.1242/jeb.126888
Mlíkovský J. (1989a). Brain size in birds: 1. Tinamiformes through ciconiiformes. Vest. Cs. Spolec. Zool. 53, 33–47.
Mlíkovský J. (1989b). Brain size in birds: 2. Falconiformes through gaviiformes. Vest. Cs. Spolec. Zool. 53, 200–213.
Mlíkovský J. (1989c). Brain size in birds: 3. Columbiformes through piciformes. Vest. Cs. Spolec. Zool. 53, 252–264.
Møller, A. P., and Erritzøe, J. (2014). predator-prey interactions, flight initiation distance and brain size. J. Evol. Biol. 27, 34–42. doi: 10.1111/jeb.12272
Møller, A. P., Erritzøe, H., and Erritzøe, J. (2011). A behavioral ecology approach to traffic accidents: Interspecific variation in causes of traffic casualties among birds. Zool. Res. 32, 115–127. doi: 10.3724/SP.J.1141.2011.02115
Møller, A. P., and Erritzøe, J. (2016). Brain size and the risk of getting shot. Biol. Lett. 12:20160647. doi: 10.1098/rsbl.2016.0647
Møller, A. P., and Erritzøe, J. (2017). Brain size in birds is related to traffic accidents. R. Soc. Open Sci. 4:161040. doi: 10.1098/rsos.161040
Møller, A. P., Vágási, C., and Pap, P. (2013). Risk-taking and the evolution of mechanisms for rapid escape from predators. J. Evol. Biol. 26, 1143–1150. doi: 10.1111/jeb.12147
Moore, B. A., Baumhardt, P., Doppler, M., Randolet, J., Blackwell, B. F., DeVault, T. L., et al. (2012). Oblique color vision in an open-habitat bird: spectral sensitivity, photoreceptor distribution and behavioral implications. J. Exp. Biol. 215, 3442–3452. doi: 10.1242/jeb.073957
Nagy, K. A., Girard, I. A., and Brown, T. K. (1999). Energetics of free-ranging mammals, reptiles, and birds. Annu. Rev. Nutr. 19, 247–277. doi: 10.1146/annurev.nutr.19.1.247
Norberg, U. M. (1990). Vertebrate Flight: Mechanics, Physiology, Morphology, Ecology and Evolution. Berlin: Springer-Verlag.
Lindhe Norberg, U. M. (2002). Structure, form, and function of flight in engineering and the living world. J. Morphol. 252, 52–81. doi: 10.1002/jmor.10013
Norberg, U. M., and Rayner, J. M. V. (1987). Ecological morphology and flight in bats (Mammalia; Chiroptera): wing adaptations, flight performance, foraging strategy and echolocation. Phil. Trans. R. Soc. Lond. B 316, 335–427. doi: 10.1098/rstb.1987.0030
Nudds, R. L., Dyke, G. J., and Rayner, J. M. V. (2007). Avian brachial index and wing kinematics: putting movement back into bones. J. Zool. 272, 218–226. doi: 10.1111/j.1469-7998.2006.00261.x
Nunn, C. L. (2011). The Comparative Approach in Evolutionary Anthropology and Biology. Chicago, IL: University of Chicago Press.
Olson, S. M. (2015). Pacific Flyway Data Book, 2015. U.S. Department of Interior, Fish and Wildlife Service, Division of Migratory Bird Management, Vancouver, WA.
Orme, D., Freckleton, R., Thomas, G., Petzoldt, T., Fritz, S., Isaac, N., et al. (2013). Caper: Comparative Analyses of Phylogenetics and Evolution in R. R package version 0.5.2.
Poole, E. L. (1938). Weights and wing areas in North American birds. Auk 55, 511–517. doi: 10.2307/4078421
R Core Team (2017). R: A Language and Environment for Statistical Computing. R Foundation for Statistical Computing, Vienna.
Richards, S. A. (2005). Testing ecological theory using the information-theoretic approach: examples and cautionary results. Ecology 86, 2805–2814. doi: 10.1890/05-0074
Rubolini, D., Liker, A., Garamszegi, L. Z., Møller, A. P., and Saino, N. (2015). Using the BirdTree.org website to obtain robust phylogenies for avian comparative studies: a primer. Curr. Zool. 61, 959–965. doi: 10.1093/czoolo/61.6.959
Samia, D. S., Pape Møller, A., and Blumstein, D. T. (2015). Brain size as a driver of avian escape strategy. Sci. Rep. 5:11913. doi: 10.1038/srep11913
Santos, S. M., Mira, A., Salgueiro, P. A., Costa, P., Medinas, D., and Beja, P. (2016). Avian trait-mediated vulnerability to road traffic collisions. Biol. Conserv. 200, 122–130. doi: 10.1016/j.biocon.2016.06.004
Serrano, F. J., Palmqvist, P., Chiappe, L. M., and Sanz, J. L. (2017). Inferring flight parameters of Mesozoic avians through multivariate analyses of forelimb elements in their living relatives. Paleobiology 43, 144–169. doi: 10.1017/pab.2016.35
Sol, D., Garcia, N., Iwaniuk, A., Davis, K., Meade, A., Boyle, W. A., et al. (2010). Evolutionary divergence in brain size between migratory and resident birds. PLoS ONE 5:e9617. doi: 10.1371/journal.pone.0009617
Sukumaran, J., and Holder, M. T. (2010). DendroPy: a python library for phylogenetic computing. Bioinformatics 26, 1569–1571. doi: 10.1093/bioinformatics/btq228
Symonds, M. R. E., and Moussalli, A. (2011). A brief guide to model selection, multimodel inference and model averaging in behavioural ecology using Akaike's information criterion. Behav. Ecol. Sociobiol. 65, 13–21.
Tatner, P., and Bryant, D. M. (1986). Flight cost of a small passerine measured using doubly labele water: implications for energetics studies. Auk 103, 169–180.
Ullmann, J. F., Moore, B. A., Temple, S. E., Fernández-Juricic, E., and Collin, S. P. (2012). The retinal wholemount technique: a window to understanding the brain and behaviour. Brain Behav. Evol. 79, 26–44. doi: 10.1159/000332802
Vincze, O., Vágási, C. I., Pap, P. L., Osváth, G., and Møller, A. P. (2015). Brain regions associated with visual cues are important for bird migration. Biol. Lett. 11:20150678. doi: 10.1098/rsbl.2015.0678
Keywords: bird strikes, flying maneuverability, high-speed vehicles, visual acuity, wing loading
Citation: Fernández-Juricic E, Brand J, Blackwell BF, Seamans TW and DeVault TL (2018) Species With Greater Aerial Maneuverability Have Higher Frequency of Collisions With Aircraft: A Comparative Study. Front. Ecol. Evol. 6:17. doi: 10.3389/fevo.2018.00017
Received: 25 October 2017; Accepted: 12 February 2018;
Published: 13 March 2018.
Edited by:
Sasha Raoul Xola Dall, University of Exeter, United KingdomReviewed by:
Daniel Klem, Muhlenberg College, United StatesCopyright © 2018 Fernández-Juricic, Brand, Blackwell, Seamans and DeVault. This is an open-access article distributed under the terms of the Creative Commons Attribution License (CC BY). The use, distribution or reproduction in other forums is permitted, provided the original author(s) and the copyright owner are credited and that the original publication in this journal is cited, in accordance with accepted academic practice. No use, distribution or reproduction is permitted which does not comply with these terms.
*Correspondence: Esteban Fernández-Juricic, ZWZlcm5hbkBwdXJkdWUuZWR1
Disclaimer: All claims expressed in this article are solely those of the authors and do not necessarily represent those of their affiliated organizations, or those of the publisher, the editors and the reviewers. Any product that may be evaluated in this article or claim that may be made by its manufacturer is not guaranteed or endorsed by the publisher.
Research integrity at Frontiers
Learn more about the work of our research integrity team to safeguard the quality of each article we publish.