Introduction
Phenotypic plasticity is the ability of an organism to change its phenotype in response to changes in the environment. Such an ability varies with genotypes (Pigliucci, 2005; El-Soda et al., 2014) and thus the environment and genotypes can interact to shape phenotypic traits (Via et al., 1995; Pigliucci and Hayden, 2001; Nussey et al., 2007). These interactions are at the basis of evolutionary processes that can, for example, drive natural populations to maladaptive evolution, when the environment is altered by anthropogenic pressures, or select for phenotypic plasticity, when the environment is unstable (Gotthard and Nylin, 1995; Pigliucci, 2005; Postma and van Noordwijk, 2005; Dunlop et al., 2009; Ingleby et al., 2010; Kuparinen and Hutchings, 2012).
Genotype by environment interactions (GEI) can also alter community structures and dynamics (Miner et al., 2005). For instance, roots forming a symbiosis with different genotypes of arbuscular mycorrhizal fungi are not affected in the same way by phosphorus availability (Ehinger et al., 2009). On the other hand, the stability of ectomycorrhizal fungal communities can be altered as a consequence of the interactions of plant genotypes with drought conditions (Gehring et al., 2014).
Assessing and modeling GEI is a key objective in evolutionary science (Gillespie and Turelli, 1989; Higginson and Reader, 2009; Ingleby et al., 2010), and in agricultural science to assist breeding in a context of global and local environmental changes (Falconer, 1952; Hammer et al., 2006). Multi-environment trials are the regular basis for the study of GEI, and the additive main effects and multiplicative interaction model is one of the most widely used analysis tools (Rodrigues et al., 2016). This has led to a widespread statistical conception of GEI, but there is a need to understand the origin of GEI when it is expressed at the level of a global performance (e.g., crop yield; Chapman, 2008). As applied ecological models, crop models have been widely designed and used by crop physiologists to decipher GEI (Yin et al., 2004; Boote et al., 2013). Paradoxically, GEI are rarely specified in genotype-dependence modeling approaches, and their occurrence is generally considered as an emerging property of simulation models, i.e., GEI reflects unexpected properties generated by complex interconnections between subsystem components and biological processes (Bertin et al., 2010).
In this paper, we specifically aim at challenging such a conception by showing that GEI can arise from simple mathematical formulations in ecological models that consider both different genotypes and environmental variation. First, we exemplify the occurrence of GEI in a model linking population dynamics with bioenergetics in passerine birds (Wiens and Innis, 1974). Then, we identify the three following basic situations that can generate GEI in ecological models, regardless of their complexity: (1) a product or quotient of a function of environment by a function of genotype, (2) a product or quotient of functions of environment and genotype, without any GEI in any of these functions; and (3) a composition of a non-linear function with a function of both environment and genotype in which there is no GEI.
An Example of GEI Occurrence in an Ecological Model
The Wiens and Innis's (1974) bioenergetics model estimates daily densities of birds at different life stages (eggs, nestlings, fledglings, juveniles, and adults) in a population when initial adult population size, vital rates, and the timings of molting and breeding are known. The consequent demographic model permits to calculate the expected daily energy demand of a population from both seasonal temperature inputs and estimates of mass- and life stage-specific metabolic rates based on existence energy requirement and seasonal costs of activity, reproduction and molting (Wiens and Innis, 1974). In this model, genotypic variation can be simulated by assuming for each genotype a different mass at the adult stage, which eventually drives the value of any mass dependent vital rate. This allows to illustrate an approach of GEI occurring at the interspecific level by considering five virtual bird species of varying adult masses in the range of 5–45 g. This was intended to generate a gradient in size in passerine species ranging from that of the Goldcrest (Regulus regulus) to that of the Red Crossbill (Loxia curvirostra). Environmental variation was simulated using five different scenarios of temperature variation obtained by translating realistic temperature series experienced by Red crossbills (Benkman et al., 2012) into a temperature delta of −2 to +2°C. The model was run for 5 × 5 combinations of bird species and temperature values. The parameter values were taken from Benkman et al. (2012), except hatching success HS that was assumed to vary with temperature whatever the species as follows: HS = HScst + 0.1 × Δθ, where Δθ is the temperature delta mentioned above and HScst is the value used by Benkman et al. (2012).
The model simulations suggest a greater increase in adult food intake in bigger than in smaller species when temperature rises (Figure 1A). We will show further that this interaction between temperature and genotype is not concerned with the model complexity.
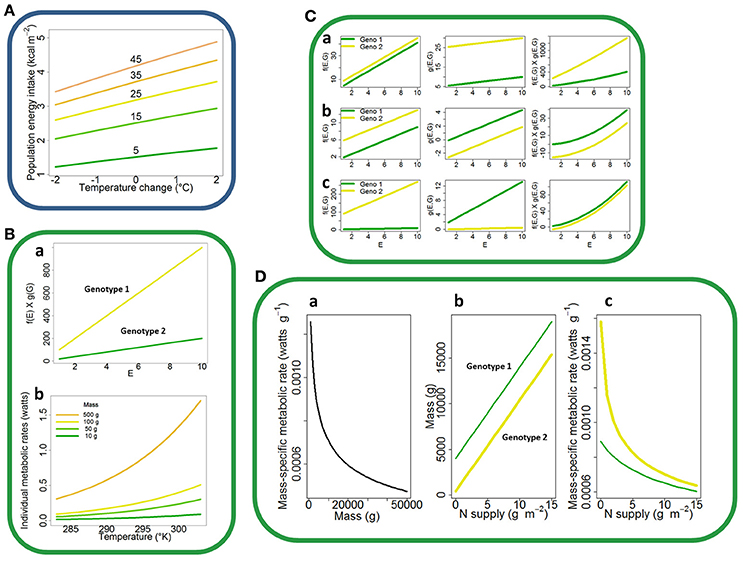
Figure 1. Genotype-by-environment interactions in ecological models. (A) Species-by-temperature interaction for population annual energy intake predicted by the Wiens and Innis's (1974) bioenergetics model. The model was run for five virtual bird species of adult masses increasing arithmetically from 5 to 45 g. (B) GEI arising from a product of functions of environment and genotype only. Variation of f(E) × g(G) with f(E) = aE where E is an environmental factor and g(G) = b1 or b2 depending on the genotype (a). Variation of individual metabolic rates predicted by MTE as a function of temperature for four species of different masses ranging from 10 to 500 g (b). (C) GEI arising or not from a product of functions of both an environmental factor E and the genotype G. Variation along E of f(E, G) = (a1E + b1), g(E, G) = (a2E + b2) and their product for two genotypes (Geno 1 and Geno 2), where ai and bi are parameters independent and dependent on the genotype, respectively; there is no GEI neither in f or g but a clear GEI arises from the product (a). Variation along E of the same functions and product as in (a), with a set of parameters (a1 × b2 + a2 × b1 = cste) such that no GEI arises from the product (b). Variation along E of the same functions and product as in (a), but where ai and bi depend on the genotype (GEI in each term of the product), with a set of parameters (chosen such as a1 × b2 + a2 × b1 = cste and a1 × a2 = cste) such that no GEI arises from the product (c). (D) GEI arising from a function composition f o g with f non-linear and g(E, G) a function of both an environmental factor E and the genotype G without interaction. Universal relationship between mass (M) and the mass-specific metabolic rate, according to the MTE (function f), with temperature T fixed (a). Variation of the mass-specific metabolic rate along a range of nitrogen supply (N supply) values for two different plant species (c), with mass being assumed to increase linearly with N (function g) without G × N interaction (b).
GEI Arising from a Product or Quotient of a Function of Environment by a Function of Genotype
Let us consider two functions f(E) and g(G), where E and G denote an environmental factor and the genotype, respectively. Functions f and g represent a given process of an organism, population, community, or ecosystem. They can be linear or non-linear. The most straightforward GEI arises from the product or quotient of f(E) by g(G), knowing that such products or quotients of functions are very common in ecological models. A very simple case shown in Figure 1Ba is f(E) × g(G), with f(E) = aE and where a is a parameter independent of the genotype that indicates the sensitivity of the process to the environment, and g(G) = bG where bG is a parameter whose value depends on the genotype G.
A more realistic example, with f and g non-linear, can be provided by the Metabolic Theory of Ecology (MTE; Brown et al., 2004). This theory predicts that the individual metabolic rate (I) can be described as a function of temperature (T) and individual mass (M) as follows:
I = f(T) × g(M) = io e−AF/kT M3/4, where io is a normalization constant, AF is the activation energy, k is the Boltzmann's constant, and T is the absolute temperature in Kelvin. Assuming for the sake of demonstration that M depends only on the genotype, a strong interaction emerges between genotype and temperature for the individual metabolic rate I (Figure 1Bb). Such a type of interaction typically explains the GEI pattern observed in Figure 1A. In the bioenergetics model of Wiens and Innis (1974), clutch size is indeed a demographic parameter that depends on adult mass and therefore on genotype, and that is multiplied by hatching success (HS) to calculate the number of nestlings. When HS is a linear function of temperature, a GEI occurs and propagates to the final output of the model (Figure 1A).
GEI Arising from a Product or Quotient of Environment and Genotype Functions that do not Initially Imply GEI
Let us consider two functions f(E, G) and g(E, G). As previously, f and g are linear or non-linear and represent any process in an organism, a population, a community, or an ecosystem. E and G denote an environmental factor and the genotype, respectively. We deal with the case when there is no interaction between E and G (such interactions arising from a product or a quotient as noted previously), neither in f nor in g. A simple case is the product of f(E, G) by g(E, G) with f(E, G) = afE + bf(G) and g(E, G) = agE + bg(G), and where ai is independent of the genotype and indicates the sensitivity of the process to the environment while bi(G) varies with different genotypes. These conditions are likely to generate a significant GEI, as shown on Figure 1Ca. For example, demographic parameters such as survival probabilities of different age classes used in the Wiens and Innis's (1974) bioenergetics model are multiplied to assess global fecundity in population dynamics models, and they may be formalized as functions of temperature and species (e.g., Ramos-Jiliberto and Aranguiz-Acuna, 2007; Ullah et al., 2011).
Note that the product f(E, G) × g(E, G) does not necessarily generates GEI as its occurrence primarily relies on parameter values. Particular sets of parameters values can prevent the occurrence of GEI in f × g in the absence of initial GEI in both f and g (Figure 1Cb) or even though there is initial GEI in f and in g (Figure 1Cc).
GEI Arising from a Function Composition with Non-Linearity
Let us consider a function f composed with a function g, i.e., f o g, where f is non-linear and g(E, G) is a function of both an environmental factor E and the genotype G that do not interact. The occurrence of GEI in this case can be exampled in plants by composing the individual mass-specific metabolic rate predicted by the universal law of individual mass (, α being constant if T is fixed; Brown et al., 2004) with M = g(N) = aN + b.
Plant mass was assumed to vary positively (Ingestad and Agren, 1992) and linearly with nitrogen supply N (Xia and Wan, 2008), with parameters a and b being independent and dependent on the genotype, respectively.
Considering two different plant species (Figure 1D), a GEI clearly arises for the mass-specific metabolic rate. Many power functions of bird masses are used in the Wiens and Innis's (1974) bioenergetics model and therefore any environment-induced variation in masses would probably have a strong impact on the population energy intake predicted by this model.
Conclusions
We have shown that three main situations can generate GEI in ecological models. They are based on products or quotients or non-linearities of functions, which are commonly encountered in such models. Based on these findings, we challenge the conception according to which GEI would reflect models' unexpected properties due to interconnections between subsystem components and biological processes (Bertin et al., 2010). Our demonstration indicates that the complexity per se of a model, i.e., the degree of such interconnections and feedback regulations, is not involved in the occurrence of GEI. A high degree of complexity may however imply many products, quotients, or non-linearities and then favor the occurrence and the range of GEI. As demonstrated here, GEI may not necessarily be unexpected, but rather they can arise from simple mathematical formulations. We are confident that this contribution can help identifying GEI sources, and help formalizing GEI in ecological models.
Author Contributions
MG and FL designed and oversaw the opinion. MG, FL, DB, and TB drafted and critically revised the manuscript. All authors read and approved the final manuscript.
Conflict of Interest Statement
The authors declare that the research was conducted in the absence of any commercial or financial relationships that could be construed as a potential conflict of interest.
References
Benkman, C. W., Fetz, T., and Talluto, M. V. (2012). Variable resource availability when resource replenishment is constant: the coupling of predators and prey. Auk 129, 115–123. doi: 10.1525/auk.2011.11069
Bertin, N., Martre, P., Génard, M., Quilot, B., and Salon, C. (2010). Under what circumstances can process-based simulation models link genotype to phenotype for complex traits? Case-study of fruit and grain quality traits. J. Exp. Bot. 61, 955–967. doi: 10.1093/jxb/erp377
Boote, K. J., Jones, J. W., White, J. W., Asseng, S., and Lizaso, J. I. (2013). Putting mechanisms into crop production models. Plant Cell Environ. 36, 1658–1672. doi: 10.1111/pce.12119
Brown, J. H., Gillooly, J. F., Allen, A. P., Savage, V. M., and West, G. B. (2004). Toward a metabolic theory of ecology. Ecology 85, 1771–1789. doi: 10.1890/03-9000
Chapman, S. C. (2008). Use of crop models to understand genotype by environment interactions for drought in real-world and simulated plant breeding trials. Euphytica 161, 195–208. doi: 10.1007/s10681-007-9623-z
Dunlop, E. S., Heino, M., and Dieckmann, U. (2009). Eco-genetic modeling of contemporary life-history evolution. Ecol. Appl. 19, 1815–1834. doi: 10.1890/08-1404.1
Ehinger, M., Koch, A. M., and Sanders, I. R. (2009). Changes in arbuscular mycorrhizal fungal phenotypes and genotypes in response to plant species identity and phosphorus concentration. New Phytol. 184, 412–423. doi: 10.1111/j.1469-8137.2009.02983.x
El-Soda, M., Malosetti, M., Zwaan, B. J., Koornneef, M., and Aarts, M. G. (2014). Genotype× environment interaction QTL mapping in plants: lessons from Arabidopsis. Trends Plant Sci. 19, 390–398. doi: 10.1016/j.tplants.2014.01.001
Falconer, D. S. (1952). The problem of environment and selection. Am. Nat. 86, 293–298. doi: 10.1086/281736
Gehring, C., Flores-Renteria, D., Sthultz, C. M., Leonard, T. M., Flores-Renteria, L., Whipple, A. V., et al. (2014). Plant genetics and interspecific competitive interactions determine ectomycorrhizal fungal community responses to climate change. Mol. Ecol. 23, 1379–1391. doi: 10.1111/mec.12503
Gillespie, J. H., and Turelli, M. (1989). Genotype-environment interactions and the maintenance of polygenic variation. Genetics 121, 129–138.
Gotthard, K., and Nylin, S. (1995). Adaptive plasticity and plasticity as an adaptation - a selective review of plasticity in animal morphology and life-history. Oikos 74, 3–17. doi: 10.2307/3545669
Hammer, G., Cooper, M., Tardieu, F., Welch, S., Walsh, B., Van Eeuwijk, F., et al. (2006). Models for navigating biological complexity in breeding improved crop plants. Trends Plant Sci. 11, 587–593. doi: 10.1016/j.tplants.2006.10.006
Higginson, A. D., and Reader, T. (2009). Environmental heterogeneity, genotype-by-environment interactions and the reliability of sexual traits as indicators of mate quality. Proc. R. Soc. B Biol. Sci. 276, 1153–1159. doi: 10.1098/rspb.2008.1592
Ingestad, T., and Agren, G. I. (1992). Theories and methods on plant nutrition and growth. Physiol. Plant. 84, 177–184. doi: 10.1111/j.1399-3054.1992.tb08781.x
Ingleby, F. C., Hunt, J., and Hosken, D. J. (2010). The role of genotype-by-environment interactions in sexual selection. J. Evol. Biol. 23, 2031–2045. doi: 10.1111/j.1420-9101.2010.02080.x
Kuparinen, A., and Hutchings, J. A. (2012). Consequences of fisheries-induced evolution for population productivity and recovery potential. Proc. R. Soc. B Biol. Sci. 279, 2571–2579. doi: 10.1098/rspb.2012.0120
Miner, B. G., Sultan, S. E., Morgan, S. G., Padilla, D. K., and Relyea, R. A. (2005). Ecological consequences of phenotypic plasticity. Trends Ecol. Evol. 20, 685–692. doi: 10.1016/j.tree.2005.08.002
Nussey, D. H., Wilson, A. J., and Brommer, J. E. (2007). The evolutionary ecology of individual phenotypic plasticity in wild populations. J. Evol. Biol. 20, 831–844. doi: 10.1111/j.1420-9101.2007.01300.x
Pigliucci, M. (2005). Evolution of phenotypic plasticity: where are we going now? Trends Ecol. Evol. 20, 481–486. doi: 10.1016/j.tree.2005.06.001
Pigliucci, M., and Hayden, K. (2001). Phenotypic plasticity is the major determinant of changes in phenotypic integration in Arabidopsis. New Phytol. 152, 419–430. doi: 10.1046/j.0028-646X.2001.00275.x
Postma, E., and van Noordwijk, A. J. (2005). Genetic variation for clutch size in natural populations of birds from a reaction norm perspective. Ecology 86, 2344–2357. doi: 10.1890/04-0348
Ramos-Jiliberto, R., and Aranguiz-Acuna, A. (2007). Between-species differences in demographic responses to temperature of coexisting cladocerans. Austral Ecol. 32, 766–774. doi: 10.1111/j.1442-9993.2007.01758.x
Rodrigues, P. C., Monteiro, A., and Lourenco, V. M. (2016). A robust AMMI model for the analysis of genotype-by-environment data. Bioinformatics 32, 58–66. doi: 10.1093/bioinformatics/btv533
Ullah, M. S., Moriya, D., Badii, M. H., Nachman, G., and Gotoh, T. (2011). A comparative study of development and demographic parameters of Tetranychus merganser and Tetranychus kanzawai (Acari: Tetranychidae) at different temperatures. Exp. Appl. Acarol. 54, 1–19. doi: 10.1007/s10493-010-9420-6
Via, S., Gomulkiewicz, R., Dejong, G., Scheiner, S. M., Schlichting, C. D., and Vantienderen, P. H. (1995). Adaptive phenotypic plasticity - consensus and controversy. Trends Ecol. Evol. 10, 212–217. doi: 10.1016/S0169-5347(00)89061-8
Wiens, J. A., and Innis, G. S. (1974). Estimation of energy flow in bird communities - population bioenergetics model. Ecology 55, 730–746. doi: 10.2307/1934410
Xia, J., and Wan, S. (2008). Global response patterns of terrestrial plant species to nitrogen addition. New Phytol. 179, 428–439. doi: 10.1111/j.1469-8137.2008.02488.x
Keywords: genotype, environment, interaction, phenotype, model, simulation
Citation: Génard M, Lescourret F, Bevacqua D and Boivin T (2017) Genotype-by-Environment Interactions Emerge from Simple Assemblages of Mathematical Functions in Ecological Models. Front. Ecol. Evol. 5:13. doi: 10.3389/fevo.2017.00013
Received: 06 January 2017; Accepted: 24 February 2017;
Published: 14 March 2017.
Edited by:
Lutz Becks, Max Planck Institute for Evolutionary Biology, GermanyReviewed by:
S. Lan Smith, Japan Agency for Marine-Earth Science and Technology, JapanCopyright © 2017 Génard, Lescourret, Bevacqua and Boivin. This is an open-access article distributed under the terms of the Creative Commons Attribution License (CC BY). The use, distribution or reproduction in other forums is permitted, provided the original author(s) or licensor are credited and that the original publication in this journal is cited, in accordance with accepted academic practice. No use, distribution or reproduction is permitted which does not comply with these terms.
*Correspondence: Michel Génard, bWljaGVsLmdlbmFyZEBpbnJhLmZy