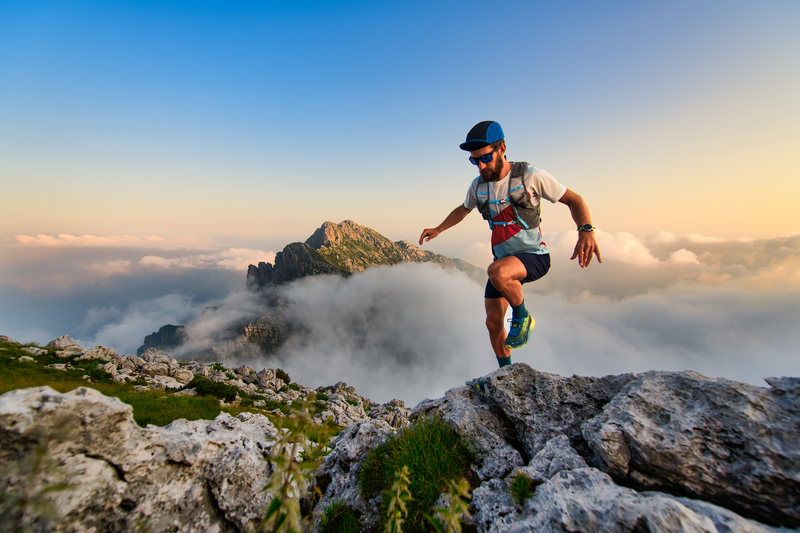
94% of researchers rate our articles as excellent or good
Learn more about the work of our research integrity team to safeguard the quality of each article we publish.
Find out more
ORIGINAL RESEARCH article
Front. Ecol. Evol. , 17 August 2016
Sec. Conservation and Restoration Ecology
Volume 4 - 2016 | https://doi.org/10.3389/fevo.2016.00098
This article is part of the Research Topic Triage in Conservation View all 11 articles
Conservation triage focuses on prioritizing species, populations or habitats based on urgency, biodiversity benefits, recovery potential as well as cost. Population Viability Analysis (PVA) is frequently used in population focused conservation prioritizations. The critical nature of many of these management decisions requires that PVA models are repeatable and reproducible to reliably rank species and/or populations quantitatively. This paper assessed the repeatability and reproducibility of a subset of previously published PVA models. We attempted to rerun baseline models from 90 publicly available PVA studies published between 2000 and 2012 using the two most common PVA modeling software programs, VORTEX and RAMAS-GIS. Forty percent (n = 36) failed, 50% (45) were both repeatable and reproducible, and 10% (9) had missing baseline models. Repeatability was not linked to taxa, IUCN category, PVA program version used, year published or the quality of publication outlet, suggesting that the problem is systemic within the discipline. Complete and systematic presentation of PVA parameters and results are needed to ensure that the scientific input into conservation planning is both robust and reliable, thereby increasing the chances of making decisions that are both beneficial and defensible. The implications for conservation triage may be far reaching if population viability models cannot be reproduced with confidence, thus undermining their intended value.
Despite concerted efforts by conservation practitioners worldwide, species extinction rates continue to increase (Butchart et al., 2010; Pimm et al., 2014). Current conservation spending remains well below that required to return rates of extinction to natural levels (Balmford et al., 2003; McCarthy et al., 2012). The persistent and often escalating threats to biodiversity, coupled with inadequate funding, make it inevitable that conservation managers apply triage in decision making (Bottrill et al., 2008, 2009; Arponen, 2012).
Conservation triage focuses on prioritizing species, populations or habitats based on urgency, biodiversity benefits, recovery potential (i.e., chance of success), and costs to achieve a desired goal (Bottrill et al., 2008). Urgency is frequently a function of extinction risk but also values associated with particular species (Farrier et al., 2007). Some argue that it is futile to spend time and scarce resources on hopeless cases or on species/populations that are likely to persist without conservation intervention (Arponen, 2012). Essentially, projects should be prioritized on species uniqueness (e.g., evolutionary distinctiveness, Jetz et al., 2014), probabilities of extinction and cost of conservation actions (McDonald-Madden et al., 2008; Reece and Noss, 2014). However, the uncertainty associated with some or all of these parameters will ultimately influence our ability to make robust conservation decisions (Beissinger and Westphal, 1998; Nicholson and Possingham, 2007). In many cases trade-offs become critical in directing limited resources optimally amongst a suite of species, whether these are a few high priority species or a greater number of lower priority species (McCarthy et al., 2008; Joseph et al., 2009; Arponen, 2012).
Population Viability Analysis (PVA) is used to support conservation decision making by providing empirical evaluations of different management actions for the species or population in question (Burgman and Possingham, 2000; Dreschler and Burgman, 2004; IUCN, 2008). PVA modeling of the effects of demographic, environmental and genetic stochasticity, natural catastrophes, environmental spatial structure, landscape heterogeneity, and the influence of management strategies permits estimation of the extinction risk of populations (Reed et al., 2002). By predicting population persistence in the short (a few years) to medium (10s–100s years) term, PVA allows quantitative ranking of alternative management strategies that benefit populations or metapopulations (Burgman and Possingham, 2000; Reed et al., 2002; Traill et al., 2010).
The use of PVA as a decision support tool to guide threatened species management interventions is not without limitations. The decisions made by users are heavily reliant on PVAs using comprehensive, reliable, accurate, and up-to-date information (Beissinger and Westphal, 1998; Traill et al., 2010; Flather et al., 2011). The reliability and predictive capacity of PVA has been tested previously (Taylor, 1995; Brook et al., 2000) and is influenced by the availability of known historical population level data (Reed et al., 2002). While the underlying data quality (robustness) is fundamentally important in supporting conservation triage decision making, an often overlooked aspect relates to how reliable or repeatable the PVAs are themselves. This has recently been emphasized by Pe'er et al. (2013) when advocating for a standard protocol for PVA that included detailed communication criteria.
This has important implications for dynamic conservation management considering that if original PVAs cannot be repeated and reproduced, how can we reliably evaluate the effectiveness of different management strategies or prioritize species? Repeatability is important for the development of any field of research (Cassey and Blackburn, 2006; Ellison, 2010) and is a basic requirement for the assessment of management strategies. Reproducibility is desirable when extending or attempting to evaluate the results of previous research and goes someway to protecting against deliberate fraud (Cassey and Blackburn, 2006) or accidental errors.
Faced with the need to adopt a more strategic and defensible approach to threatened species management and prioritization, it can be expected that practitioners will want to reassess the extinction risk of species at some time in the future building on initial PVA predictions. These may be required for various reasons including that better data may have become available, management interventions may have changed in response to ongoing or novel threatening processes or financial and/or other resources may have changed. A first step in such revisions will be the comparison of previous predictions and models using new data. This paper explores this aspect by asking to what extent previously published PVAs are repeatable and reproducible. This is critically important in determining their effectiveness in providing accurate and reliable information for conservation management decisions.
Our evaluation of previous PVAs comprised three successive steps (Figure 1). First, we created a database of accessible PVA models published since 2000. We confined our analysis to more recent studies, i.e., post 2000, given recent advances in the computational capacities of simulation software commonly used in undertaking these analyses and the presence of older reviews of PVAs (e.g., Menges, 2000). For our purposes “PVA models” referred to those where a PVA or Population and Habitat Viability Analysis (PHVA) had been completed for primarily terrestrial fauna and flora. The quality of data used in PVA models can vary widely depending on the species or populations involved (Brook et al., 2000). We wanted to test PVA models with the best data so we focused our data collection on species that included well-known keystone species (e.g., wolves), species involved in tourism (e.g., whale sharks), or species involved in subsistence or commercial hunting (e.g., dugongs)(n = 148), to maximize the potential for repeating these models. The demographic data on these species tends to be more extensive and as a result, PVA models and the subsequent population predictions are more robust (Brook et al., 2000; Coulson et al., 2001; Gordon et al., 2004).
Figure 1. Summary of methodology used to select Population Viability Analysis (PVA) models and determine repeatability and reproducibility of PVAs.
Secondly, we eliminated PVAs that were either user-defined PVAs with unusual structures (n = 43) or PVAs incorporating both spatial and demographic data where the data were inextricably linked and the spatial data were not available (n = 15). We focused our data collection on studies that were published using two of the most common PVA modeling software tools, i.e., VORTEX or RAMAS-GIS. These two programs are repeatedly used, subject to wide scrutiny, and are frequently revised and updated (Brook et al., 2000). They have both been used in the management and conservation of endangered species. We also chose PVA studies using these programs as many PVA models are not necessarily run or constructed by modeling experts. VORTEX and RAMAS-GIS have many default values for standard analyses and can be easily run if the required data are available. Therefore, while authors of these individual studies were likely familiar with their focal species, they would not be expected to (i) be able to construct their own models, or (ii) calculate some demographic criteria from other data.
Thirdly, we compiled the necessary model parameters as reported by the final selected studies (n = 90) and tried to rerun the baseline models of each to determine repeatability. We then determined the reproducibility of repeatable models. Models deemed to be reproducible were those where the confidence limits of data from our models overlapped with confidence limits of the data from the original model predictions.
We obtained publicly available, peer-reviewed species PVAs through extensive internet searches using Google Scholar, Science Direct and from websites including the IUCN Conservation Breeding Specialist Group (CBSG, http://www.cbsg.org/cbsg/). Searches were conducted between September 20 and October 23, 2012.
We found 148 species-specific PVAs on “popular” species (described earlier) published in peer-reviewed journal articles, PVA/PVHA workshop reports, and accepted post-graduate theses. The majority of PVAs were run using VORTEX (87 PVAs for 81 species) and RAMAS-GIS (18). The remaining PVAs were completed using a variety of self-built models.
We extracted baseline model input values from 81 of the 90 PVAs from the published sources and entered the data into VORTEX (version 9.99) or RAMAS-GIS (version 4.0) to run the baseline models. No baseline models were provided for the other 9 PVAs. For some PVAs the parameters were clearly defined in tables or lists; for some they were unclear and/or buried within the text; some stated that the input values could be found in supplementary data, which were not always accessible; and for several PVAs they were simply not available. For each PVA where applicable, we noted parameters with missing data and/or for which the data were ambiguous or had multiple options. These measures provided an indication of the robustness of these model data parameters.
In some instances, assumptions could be made about missing parameters for models rerun using VORTEX where these were not explicitly articulated in the respective studies. We assumed Environmental Variation (EV) concordance, catastrophes, dispersal, density dependent reproduction, future change in carrying capacity, harvesting, and supplementation were all excluded from the original baseline model if not explicitly mentioned. We left lethal equivalents, per cent due to recessive lethals, and age distribution at default values of 3.14, 50, and stable, respectively, if not specified. We left EV correlation among populations at 0.5 if a value was not provided, unless the baseline consisted of only one population. We were still able to run baseline models without some of these data.
If information was not available for parameters that were required to run the model (see Table 1 for required data for VORTEX), or for which assumptions could not be made, we recorded the PVA as missing required data and these studies were deemed non-repeatable. We assumed that the authors of the studies would not be able to calculate missing parameters based on other demographic data, e.g., “% adult females breeding” is not required if fecundity is estimated from a regression of juveniles (t) on adults (t-1).
Table 1. Criteria required for running the VORTEX Population Viability Analyses (PVAs) reviewed in this study including the number and frequency of examined PVAs missing these criteria.
We compared the baseline model outputs for our successfully run PVAs (repeatable) to the output values of the original models. This included a combination of commonly used viability measures such as growth rates, probability of extinction, extant population size, remaining genetic diversity, lambda and time to extinction in addition to the confidence limits for these data. If our baseline models did not match the original models (no overlapping confidence limits) we rechecked the input data and any parameters for which assumptions had been made (based on missing or ambiguous data), and these parameters were re-estimated. We then reran models and if these still did not match the original models we recorded the PVA as being non-reproducible. If baseline models were not provided in the original study, we recorded the PVA as missing baseline. As we wanted our analyses to be consistent and rigorous, we did not attempt to run alternative models for those studies missing baseline models.
All 90 PVAs were independently analyzed by two of the authors (CW, CM). For each original PVA, we recorded the version of VORTEX or RAMAS used the year the study was conducted, and the threat status of the species based on the IUCN Red List criteria (http://www.redlist.org). At the end of the analyses, we classified each PVA into one of four categories, (i) repeatable + reproducible = PVA ran and matched original (overlapping confidence limits), (ii) repeatable only = PVA ran but did not match original (non-overlapping confidence limits), (iii) failed = PVA could not be run due to missing data, or (iv) missing baseline models.
We used χ2 tests to compare the repeatability and reproducibility of PVA models in (i) different taxonomic groups (birds, mammals, reptiles), (ii) IUCN threatened species categories (Critically Endangered, Endangered, Vulnerable, Near Threatened, Least Concern), (iii) version of software (VORTEX or RAMAS) used in the original study, and (iv) publication quality (based on current journal impact factors and/or gray literature). We also used χ2 analysis to compare missing data in species from different threat categories. A correlation analysis was used to determine if there was a relationship between year of publication and our ability to replicate/reproduce the study. Kruskall-Wallis tests were used to compare the average number of missing criteria in the different taxonomic groups and threatened species categories. Statistical analysis was completed using SPSS Ver. 22 with alpha set at 0.05.
The vast majority of the 90 PVAs modeled mammal (62 species) and bird (19) populations. The remaining PVAs assessed amphibians (1), fish (1), insects (1), and reptiles (6). No plant PVAs met all the selection criteria. Eleven species are currently listed as Critically Endangered, 27 Endangered, 18 Vulnerable, 11 Near Threatened, and 23 Least Concern. The geographic spread of species was wide ranging covering the Neotropical (25 species), Nearctic (16), Afrotropical (16), Palearctic (15), Indomalayan (12), and Australasian (6) regions. Forty-one PVAs were published in peer-reviewed journals, 48 were published in CBSG reports and one was a post-graduate thesis.
Half of the 90 PVAs (n = 45) were both repeatable and reproducible, none were repeatable only, 36 failed, and nine had no baseline model (Table S1 for details).
Bird PVAs appeared more repeatable and reproducible than those for mammals or reptiles (71% vs. 43% and 33%, respectively) but not significantly so (χ2 = 4.659, df = 2, p = 0.097). There was also no relationship between the threat status of the species and PVA repeatability (χ2 = 1.304, df = 4, p = 0.86).
There was no correlation between the year the original model was run and our ability to replicate it (r = 0.108, p = 0.29), nor was there a relationship between the version of VORTEX or RAMAS used in the original PVA and our ability to replicate the model (χ2 = 27.336, df = 27, p = 0.49). Publication quality (assessed by using current journal impact factors) had no effect on PVA repeatability (χ2 = 3.524, df = 4, p = 0.47).
VORTEX 9.99 has 65 input data criteria, 11 of which are required data (Table 1). Most of the failed PVAs were missing these data (n = 32) and/or provided a range of data values (n = 12). The required data most frequently absent from PVAs included mortality rates for males and females (missing from 9% of all reviewed PVAs), standard deviation in mortality rates (20%), mate monopolization (11%), and EV (Environmental Variation) in % breeding (22%).
Required data were missing from 12% of bird PVAs, 50% of reptile PVAs, and 36% of mammal PVAs. There was no relationship between the threat status of the species and missing data (CR = 36%, EN = 24%, VU = 33%, NT = 45%, LC = 27%; χ2 = 1.983, df = 4, p = 0.74).
The total number of input data missing from PVAs (out of 65) ranged from 0 to 43 (average = 8.67 ± 9.42). There was no difference in the average number of input data missing in bird (6.0 ± 7.80), mammal (9.26 ± 10.09), and reptile (10.17 ± 8.57) PVAs (Kruskal Wallis test: p = 0.187). There was also no difference in the average number of input data missing in CR (8.1 ± 6.67), EN (6.68 ± 7.15), VU (10.61 ± 16.54), NT (11.55 ± 7.03), and LC (8.18 ± 5.12) PVAs (Kruskal Wallis test: p = 0.197).
Of the PVAs run using RAMAS-GIS, two were both repeatable and reproducible while the third did not provide a baseline model for comparison.
Our analysis has revealed that a substantial number of current PVAs for “popular” species are not repeatable due largely to the fact that the model parameters required to repeat these analyses were poorly communicated in papers or reports. The importance of communicating all inputs and outputs of PVA models in a systematic manner to ensure that studies can be repeated was recently highlighted by Pe'er et al. (2013). Here we provide an empirical demonstration of the consequences should these model parameters not be reported. Of course this has immediate effects on whether conservation practitioners can repeat the models. More broadly, however, this also diminishes the ability of practitioners to reliably make decisions on conservation actions.
Importantly, there was no pattern among studies to suggest that some were worse than others in terms of reporting baseline parameters. Consequently, repeatability was not linked to taxa, IUCN category, PVA software version used, year published or the quality of publication outlet. Plant-focused PVAs were not represented in our analysis as these were either completed using self-constructed models, or RAMAS-GIS where there were no associated spatial data. A detailed assessment of these models was therefore beyond the scope of the current paper. This does, however, highlight the need for a more detailed review of these aspects within plant-focused PVAs, building on the previous review by Menges (2000).
While the quality and quantity of data is one primary source of uncertainty affecting the reliability of PVA predictions (Beissinger and Westphal, 1998), the implications of not being able to repeat studies has not yet been empirically evaluated. While the reliability of predictions could result in scarce resources being directed inefficiently, where predictions cannot be repeated or reproduced practitioners may be unable to evaluate whether any conservation action or spending has achieved the desired conservation objective. Our results suggest that the latter problem is systemic within the discipline, despite the fact that numerous guidelines for undertaking PVAs exist (e.g., Beissinger and Westphal, 1998; Burgman and Possingham, 2000; Ralls et al., 2002; IUCN, 2008). Given that our sample of PVAs also concentrated on species with a higher profile, we may have expected that data for these species would be more comprehensive. Nevertheless, the number of PVAs that could not be replicated was still relatively high suggesting that our assessment of repeatability and reproducibility in PVAs could be an overestimate. We therefore, echo the sentiments of Pe'er et al. (2013) who have called for the complete and systematic presentation of PVA parameters and results to ensure repeatability of these studies.
Previous reviews of the utility of PVAs consider the importance of reducing uncertainty through careful selection of model structures based on known available data (Burgman and Possingham, 2000). Pe'er et al. (2013) provide the most recent evaluation of model parameters commonly included in the application of PVAs. However, they do not suggest which of these are fundamental to being able to compile and run a simple baseline model, despite suggesting that the inclusion of density-dependent processes remains poor. From our analyses we were able to identify those parameters that should be seen as minimum requirements (in our case for studies completed using VORTEX) to enable others to repeat the models at a later stage. These parameters are similar to those listed by Ralls et al. (2002) and included aspects of mortality rates and changes in carrying capacity. Of course the suggestions provided by Pe'er et al. (2013) are still valid in that any data used in these baseline models should be accompanied by all the necessary metadata. As such, all baseline PVA models should be checked for repeatability and reproducibility during the peer review process to make sure that all necessary data is provided prior to publication. The current transition to academic publication models that require authors to submit their raw data together with manuscripts may successfully address this issue in the future.
The repeatability of PVAs is critical to improving conservation efficiencies for a number of reasons. Firstly, those that are not repeatable may bring into question the validity and predictions of the original model. This is important as there are numerous authors who have highlighted the shortcomings for conservation practice should PVA predictions not be sufficiently robust (Taylor, 1995; Burgman and Possingham, 2000; Ralls et al., 2002). Furthermore, given that improvement of PVA models is an ongoing process (Lindenmayer et al., 2000; Ralls et al., 2002), non-repeatable PVAs limit the ability of conservation practitioners to compare revised models using updated parameters to previous models. This will be the case regardless of the simulation program used, i.e., VORTEX, RAMAS, etc.
With finite resources to develop and implement conservation strategies for threatened populations, conservation managers need to prioritize strategies and options to the species and/or habitats where they produce the greatest benefit (McDonald-Madden et al., 2008; Arponen, 2012). Robust and reliable PVAs based on biology and management resources that examine the costs and benefits of different management options can aid in decision making in an objective and transparent way. In practice though, conservation prioritization is often a subjective and value-driven process (Farrier et al., 2007; Arponen, 2012) that is heavily influenced by sociopolitical factors. Given the influence of so many other factors on the conservation planning process, it is critical that the scientific input is robust, reliable, and reproducible thereby increasing the chances of making decisions that are both beneficial and justifiable.
Study design CM, JC; data collection and analysis CM, CW; manuscript preparation CM, JC, CW.
The authors declare that the research was conducted in the absence of any commercial or financial relationships that could be construed as a potential conflict of interest.
We thank Mark Burgman for feedback on an earlier version of this manuscript and Ralf Buckley for discussions on the topic. We also thank Daniel Brito and the reviewers for their useful comments and suggestions that improved the manuscript.
The Supplementary Material for this article can be found online at: http://journal.frontiersin.org/article/10.3389/fevo.2016.00098
Arponen, A. (2012). Prioritizing species for conservation planning. Biol. Conserv. 21, 875–893. doi: 10.1007/s10531-012-0242-1
Balmford, A., Gaston, K. J., Blyth, S., James, A., and Kapos, V. (2003). Global variation in terrestrial conservation costs, conservation benefits, and unmet conservation needs. Proc. Natl. Acad. Sci. U.S.A. 100, 1046–1050. doi: 10.1073/pnas.0236945100
Beissinger, S. R., and Westphal, M. I. (1998). On the use of demographic models of population viability in endangered species management. J. Wild. Manage. 62, 821–841. doi: 10.2307/3802534
Bottrill, M. C., Joseph, L. N., Carawardine, J., Bode, M., Cook, C., Game, E. T., et al. (2008). Is conservation triage just smart decision making? Trends Ecol. Evol. 23, 649–654. doi: 10.1016/j.tree.2008.07.007
Bottrill, M. C., Joseph, L. N., Carawardine, J., Bode, M., Cook, C., Game, E. T., et al. (2009). Finite conservation funds mean triage is unavoidable. Trends Ecol. Evol. 24, 183–184. doi: 10.1016/j.tree.2008.11.007
Brook, B. W., O'Grady, J. J., Chapman, A. P., Burgman, M. A., Akcakaya, H. R., and Frankham, R. (2000). Predictive accuracy of population viability analysis in conservation biology. Nature 404, 385–387. doi: 10.1038/35006050
Burgman, M., and Possingham, H. P. (2000). “Population viability analysis for conservation: the good, the bad and the undescribed,” in Genetics, Demography and Viability of Fragmented Populations, eds A. G. Young and G. M. Clarke (London: Cambridge University Press), 97–112.
Butchart, S. H., Walpole, M., Collen, B., van Strien, A., Scharlemann, J. P. W., Almond, R. E. A., et al. (2010). Global biodiversity: indicators of recent declines. Science 328, 1164–1168. doi: 10.1126/science.1187512
Cassey, P., and Blackburn, T. M. (2006). Reproducibility and repeatability in ecology. Bioscience 56, 958–959. doi: 10.1641/0006-3568(2006)56[958:RARIE]2.0.CO;2
Coulson, T., Mace, G. M., Hudson, E., and Possingham, H. (2001). The use and abuse of population viability analysis. Trends Ecol. Evol. 16, 219–221. doi: 10.1016/S0169-5347(01)02137-1
Dreschler, M., and Burgman, M. A. (2004). Combining population viability analysis with decision analysis. Biol. Conserv. 13, 115–139. doi: 10.1023/B:BIOC.0000004315.09433.f6
Ellison, A. M. (2010). Repeatability and transparency in ecological research. Ecology 9, 2536–2539. doi: 10.1890/09-0032.1
Farrier, D., Whelan, R., and Mooney, C. (2007). Threatened species listing as a trigger for conservation action. Environ. Sci. Policy 10, 219–229. doi: 10.1016/j.envsci.2006.12.001
Flather, C. H., Hayward, G. D., Beissinger, S. R., and Stephens, P. A. (2011). Minimum viable populations: is there a ‘magic number’ for conservation practitioners? Trends Ecol. Evol. 26, 307–316. doi: 10.1016/j.tree.2011.03.001
Gordon, I. J., Hester, A. J., and Festa-Bianchet, M. (2004). The management of wild large herbivores to meet economic, conservation and environmental objectives. J. Appl. Ecol. 41, 1021–1031. doi: 10.1111/j.0021-8901.2004.00985.x
IUCN (2008). Strategic Planning for Species Conservation: A Handbook. Gland, Switzerland: IUCN Species Survival Commission.
Jetz, W., Thomas, G. H., Joy, J. B., Redding, D. W., Hartmann, K., and Mooers, A. O. (2014). Global distribution and conservation of evolutionary distinctness in birds. Curr. Biol. 24, 919–930. doi: 10.1016/j.cub.2014.03.011
Joseph, L. N., Maloney, R. F., and Possingham, H. P. (2009). Optimal allocation of resources among threatened species: a project prioritization protocol. Conserv. Biol. 23, 328–338. doi: 10.1111/j.1523-1739.2008.01124.x
Lindenmayer, D. B., Lacy, R. C., and Pop, M. L. (2000). Testing a simulation model for population viability analysis. Ecol. Appl. 10, 580–597. doi: 10.1890/1051-0761(2000)010[0580:TASMFP]2.0.CO;2
McCarthy, D. P., Donald, P. F., Scharlemann, J. P. W., Buchanan, G. M., Balmford, A., Green, J. M. H., et al. (2012). Financial costs of meeting global biodiversity conservation targets: current spending and unmet needs. Science 338, 946–949. doi: 10.1126/science.1229803
McCarthy, M. A., Thompson, C. J., and Garnett, S. T. (2008). Optimal investment in conservation of species. J. Appl. Ecol. 45, 1428–1435. doi: 10.1111/j.1365-2664.2008.01521.x
McDonald-Madden, E., Baxter, P. W. J., and Possingham, H. P. (2008). Subpopulation triage: how to allocate conservation effort among populations. Conserv. Biol. 22, 656–665. doi: 10.1111/j.1523-1739.2008.00918.x
Menges, E. S. (2000). Population viability analyses in plants: challenges and opportunities. Trends Ecol. Evol. 15, 51–56. doi: 10.1016/S0169-5347(99)01763-2
Nicholson, E., and Possingham, H. P. (2007). Making conservation decisions under uncertainty for the persistence of multiple species. Ecol. Appl. 17, 251–265. doi: 10.1890/1051-0761(2007)017[0251:MCDUUF]2.0.CO;2
Pe'er, G., Matsinos, Y. G., Johst, K., Franz, K. W., Turlure, C., Radchuk, V., et al. (2013). A protocol for better design, application, and communication of population viability analyses. Conserv. Biol. 27, 644–656. doi: 10.1111/cobi.12076
Pimm, S. L., Jenkins, C. N., Abell, R., Brooks, T. M., Gittleman, J. L., Joppa, L. N., et al. (2014). The biodiversity of species and their rates of extinction, distribution, and protection. Science 344:1246752. doi: 10.1126/science.1246752
Ralls, K., Beissinger, S. R., and Cochrane, J. F. (2002). “Guidelines for using population viability analysis in endangered species management,” in Population Viability Analysis, eds S. R. Beissinger and D. R. McCullough (Chicago: University of Chicago Press), 521–550.
Reece, J. S., and Noss, R. F. (2014). Prioritizing species by conservation value and vulnerability: a new index applied to species threatened by sea-level rise and other risks in Florida. Nat. Area. J. 34, 31–45. doi: 10.3375/043.034.0105
Reed, J. M., Mills, L. S., Dunning, J. B., Menges, E. S., McKelvey, K. S., Frye, R., et al. (2002). Emerging issues in population viability analysis. Conserv. Biol. 16, 7–19. doi: 10.1046/j.1523-1739.2002.99419.x
Taylor, B. L. (1995). The reliability of using population viability analysis for risk classification of species. Conserv. Biol. 9, 551–558. doi: 10.1046/j.1523-1739.1995.09030551.x
Keywords: conservation triage, VORTEX, RAMAS-GIS, essential data
Citation: Morrison C, Wardle C and Castley JG (2016) Repeatability and Reproducibility of Population Viability Analysis (PVA) and the Implications for Threatened Species Management. Front. Ecol. Evol. 4:98. doi: 10.3389/fevo.2016.00098
Received: 11 May 2016; Accepted: 02 August 2016;
Published: 17 August 2016.
Edited by:
Enrico Di Minin, University of Helsinki, FinlandReviewed by:
Andrea Santangeli, University of Helsinki, FinlandCopyright © 2016 Morrison, Wardle and Castley. This is an open-access article distributed under the terms of the Creative Commons Attribution License (CC BY). The use, distribution or reproduction in other forums is permitted, provided the original author(s) or licensor are credited and that the original publication in this journal is cited, in accordance with accepted academic practice. No use, distribution or reproduction is permitted which does not comply with these terms.
*Correspondence: J. Guy Castley, Zy5jYXN0bGV5QGdyaWZmaXRoLmVkdS5hdQ==
Disclaimer: All claims expressed in this article are solely those of the authors and do not necessarily represent those of their affiliated organizations, or those of the publisher, the editors and the reviewers. Any product that may be evaluated in this article or claim that may be made by its manufacturer is not guaranteed or endorsed by the publisher.
Research integrity at Frontiers
Learn more about the work of our research integrity team to safeguard the quality of each article we publish.