- Department of Ecology, Evolution and Behavior, University of Minnesota, St. Paul, MN, USA
Correlations between behavioral traits are widespread, but the developmental genetic architecture of such correlations is poorly characterized. Understanding the developmental mechanisms that lead to correlations between behaviors has implications for predicting how changing environments might alter the strength, direction and persistence of these associations. Here we test the idea that genetic variation in one behavioral trait can drive the development of traits related to a second behavior, resulting in correlations between them. We focus on correlations between movement and aspects of cognition, in particular accuracy of decision making and neural investment. Such syndromes have been seen across a variety of systems, from insects to birds, but the direction of the correlation often varies. We use cabbage white butterflies as a system because they are easy to rear in large numbers and show ample genetic variation in both movement and learning, facilitating a split-sibling design. We test the prediction that variation in established proxies for movement at emergence will be correlated with the development of cognitive traits later in life (in siblings). Our results suggest that genotypes (full-sibling groups) that emerge with more elongate wings explore their environment more rapidly. In addition, genotypes that emerge with relatively smaller thoraxes are more likely to learn to search for atypical host plants and subsequently develop larger brains and brain regions. Taken together, genotypes that invest less in flight are slower, better learners and develop larger brains. These data are consistent with the idea that movement can drive the development of other behavioral traits, resulting in the emergence of correlated behaviors.
Introduction
Correlations between movement and aspects of cognition are ubiquitous (Marchetti and Drent, 2000; Dugatkin and Alfieri, 2003; Sneddon, 2003; Bolhuis et al., 2004; Mery et al., 2007; Exnerova et al., 2010). In some studies, fast-moving, bold individuals are those with limited flexibility in behavior, poor long-term memory or smaller brains (Verbeek et al., 1994; Mery et al., 2007; Burns and Rodd, 2008; Exnerova et al., 2010). However, in other instances, there are positive correlations between movement and cognitive traits (Dugatkin and Alfieri, 2003; Sneddon, 2003; Guenther et al., 2014a). Understanding these correlations is important to predict patterns of coexistence and survival within species (Dall et al., 2004; Sih et al., 2004a; Bolnick et al., 2011). For instance, certain personality or movement types may be more likely to colonize new environments, influencing metapopulation dynamics (Bishop and Riechert, 1990; Hanski et al., 2004; Cote et al., 2010). Variation in movement and dispersal can also affect how organisms track environmental change (Higgins and Richardson, 1999; Thomas et al., 2001; Kotiaho et al., 2005; Poyry et al., 2009). However, how this variation in movement is correlated with cognitive abilities may affect whether a colonizing genotype survives in a new environment (Sol et al., 2002, 2005a). For instance, negative movement-cognition syndromes suggest that individuals most likely to colonize a novel environment may be the least likely to learn to use new resources in that environment.
While movement-cognition correlations have been documented across a range of systems, the developmental genetic architecture of these syndromes is unclear (Figure 1; Duckworth, 2010; Stamps and Groothuis, 2010a,b). Dissecting such proximate questions about behavioral syndromes is key to understanding how these correlations may break down or persist in the face of environmental or genetic change (Sih et al., 2004b). There are several proximate mechanisms by which correlations between behaviors might arise. In a handful of cases, correlated suites of behavior may stem from a set of genetic variants that are linked physically (e.g., in the same chromosomal region) or at the population-level due to mating patterns (e.g., “anxiety” traits in mice, Henderson et al., 2004). More commonly, behavioral syndromes emerge from pleiotropic effects of one or two genes as in the case of the foraging gene and rover-sitter Drosophila (Ben-Shahar et al., 2002; Mery et al., 2007). An idea receiving increasing attention in the literature is that of a niche-picking or niche-construction view in behavioral development (Stamps and Groothuis, 2010a; Stotz, 2010; Saltz and Nuzhdin, 2014). Exposure to different environmental conditions, such as enriched environments, high predation conditions or social stress, can influence the development of behavioral syndromes (Dirienzo et al., 2012; Edenbrow and Croft, 2013; Bengston et al., 2014). Thus, any genetic variation in traits that affect how an individual experiences the environment can result in genetic variation in behavioral syndromes. For instance, genetic variation in social preference has the potential to influence the development of personalities through the effects on a constructed social niche (Saltz, 2011; Montiglio et al., 2013).
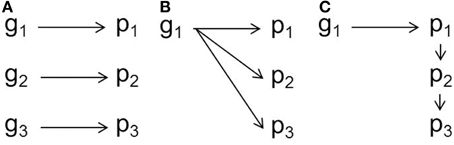
Figure 1. Schematic of developmental genetic mechanisms underlying behavioral syndromes. (A) Linked genes (g) are associated with three correlated phenotypes (p). (B) One gene has pleiotropic effects on three phenotypes. (C) One gene affects one trait, which affects the development of other phenotypes, resulting in correlated traits. This latter mechanism, the focus of this research, would be considered a special case of “developmental” pleiotropy.
In this work, we focus on the hypothesis that genetic variation in movement-related traits affects the development of movement-cognition syndromes. It is well known that sensory stimulation during development can affect neural and behavioral development: exposure to enriched environments in development, (e.g., more social interactions or a range of resources), can result in neurogenesis, synaptogenesis and increased learning abilities (Van Praag et al., 2000; Arai and Feig, 2011). In more natural settings, an increase in sensory stimulation could stem from movement through different habitats, exposure to a range of resources or a combination of the two. Indeed, both movement and exercise have significant impacts on neural development (Olson et al., 2006). Taken together, these observations suggest that movement-cognition syndromes could emerge out of developmental interactions as organisms move through and interact with their environment.
Here, we test the hypothesis that slow exploration of the environment can affect the development of cognitive abilities, thus resulting in movement-cognition syndromes in adults. The decision-making literature suggests that there are often tradeoffs between the speed and accuracy of a decision (Dickman and Meyer, 1988; Chittka et al., 2009) which likely stems from limited attention and neural processing abilities (Bernays, 2001; Dukas, 2002). Such tradeoffs are thought to explain instances where fast, bold individuals are less flexible in the face of environmental change or invest less in neural machinery (Burns and Rodd, 2008; Sih and Del Giudice, 2012). In particular, it has been suggested that many syndromes may lie on a proactive-reactive axis, where bold individuals are where bold individuals are proactive. Bold individuals quickly explore and learn in a new environment but are less sensitive to new information and less capable of adjusting their behavior to environmental change (Sih….) (Sih and Del Giudice, 2012). We predict that such correlations emerge partly out of developmental interactions: slower exploratory movements earlier in development may drive the development of greater cognitive abilities, resulting in negative movement-cognition syndromes.
Dissecting the developmental basis of behavioral syndromes is challenging because measuring a trait at one time point may prevent or bias the measurement of that trait at a later time point. Family- or sibling-level approaches are one way around this problem which can simultaneously provide data on genetic variation in suites of correlated traits (Stamps and Groothuis, 2010a). In this study, we use a family-level approach to take independent measurements on naïve individuals sacrificed early in adulthood while their siblings, which were exposed to one of several behavioral assays, were tested at a later time point. We use the cabbage white butterfly, Pieris rapae, as a study system because they are easy to rear in common garden conditions, facilitating family-level designs (Snell-Rood and Papaj, 2009). In addition, we know learning affects resource use in many butterflies (Papaj, 1986a,b; Papaj and Prokopy, 1989; Weiss and Papaj, 2003), including P. rapae, which learn motor patterns for manipulating nectar resources (Lewis, 1986; Kandori and Ohsaki, 1996) and sensory cues associated with locating host plants or rewarding flowers (Traynier, 1984, 1986; Kandori and Ohsaki, 1996; Smallegange et al., 2006). In regards to host-searching behavior, learning is particularly important for locating atypical red hosts (Snell-Rood and Papaj, 2009) and exposure to more difficult learning environments can have positive effects on neural development (Snell-Rood et al., 2009). In contrast, an innate bias to search for green colors minimizes the role of learning when typical, green-colored hosts are present. Overall, cabbage whites are an ideal system for testing how suites of cognitive traits are influenced by developmental experience.
In the present work, we make use of an existing experiment that tested for associations between genetic variation in host plant learning ability and neural investment (Snell-Rood et al., 2009). To the existing dataset, we have added several measurements, including search speed and two morphological proxies for movement. We primarily focus on relative thorax mass, which in butterflies is positively associated with investment in flight, in terms of acceleration and duration (Chai and Srygley, 1990; Dudley, 1990; Marden and Chai, 1991; Dudley and Srygley, 1994; Hill et al., 1999; Kingsolver and Srygley, 2000; Marden, 2000; Berwaerts et al., 2002, 2008; Norberg and Leimar, 2002; Berwaerts and Van Dyck, 2004). We also focus on wing elongation, which is associated with greater acceleration, flight speed and distance (Betts and Wootton, 1988; Dudley, 1990; Berwaerts et al., 2002, 2008; Berwaerts and Van Dyck, 2004; Dockx, 2007). We relate measures of movement to measures of behavioral flexibility at different time points during adulthood. We test the primary prediction that if movement affects the development of cognition syndromes, movement traits at emergence will be related to cognitive traits (brain size and host-finding ability) assayed later in development, but not earlier in development.
Methods
Measures of Flight Capability
We used two morphological proxies for flight ability. First, we focused on thorax mass relative to body size, which has been linked to aspects of flight acceleration, speed and duration in over a dozen species of butterflies, including close relatives of Pieris rapae (Chai and Srygley, 1990; Dudley, 1990; Marden and Chai, 1991; Dudley and Srygley, 1994; Hill et al., 1999; Kingsolver and Srygley, 2000; Marden, 2000; Berwaerts et al., 2002, 2008; Norberg and Leimar, 2002; Berwaerts and Van Dyck, 2004). For this measure, we used full siblings that had been sacrificed at emergence given that thorax mass changes over the lifespan of a butterfly (Stjernholm et al., 2005; Stjernholm and Karlsson, 2008; Snell-Rood et al., 2013). Individuals were stored frozen in glassine envelopes until measurement. Wings, head, abdomen and legs were removed from the thorax which was dried at 60°C in a drying oven for at least 24 h. Thoraxes were measured to the nearest 0.1 mg. We calculated the relative thorax mass of a family by running a model with family as a fixed effect and forewing area as a separate measure of body size. Least square means were taken from this model as our measure of size-corrected thoracic investment at emergence. Mean sample size per family was 5.2 individuals (range = 2–14).
Second, we used forewing “circularity” as a measure of forewing elongation. Wing elongation has been linked to acceleration, flight speed and flight distance in at least four butterfly species (Betts and Wootton, 1988; Dudley, 1990; Berwaerts et al., 2002, 2008; Berwaerts and Van Dyck, 2004; Dockx, 2007). To measure circularity, wings of individual butterflies were removed with forceps, photographed and measured in Image J (NIH). Circularity is a function of wing area and perimeter [4π(area/perimeter^2)], thus, larger values indicate a shape closer to a circle, less elongate. For family-level measures of wing elongation, we used individuals sacrificed at both emergence and after host-searching experience because experience did not affect the measurement, for instance through wing damage [F(1, 341) = 1.22, P = 0.27]. Because area is a component of this measurement, we did not correct for size. Mean sample size per family was 10.0 (range = 3–24).
Host-Finding Behavior and Neural Investment
Full description and analysis of the behavioral experiment is presented in Snell-Rood et al. (2009). Briefly, after rearing in a common garden on artificial diet, naïve butterflies were sacrificed at emergence for measures of brain size while their siblings were subjected to one of four host-searching assays (Figure 2). Mated female butterflies searched for either a green host (kale) or a red host (photic stressed Barbarea vulgaris) in either a simple or complex environment (simple = 50% hosts and 1 non-host type; complex = 20% hosts and 4 non-host types). Female butterflies sample potential host plants through landings where they “taste” chemicals in the plants with their foretarsii (Hern et al., 1996). We recorded all landings made during host searching using Noldus software that included a time stamp for each individual observation. After opportunities to search for hosts over a 2-day period (about 1–2 h of experience per individual), females were sacrificed for subsequent measures of neural investment. This host-searching assay, in a large flight cage (4 × 4 × 2 m tall), was the first opportunity for females to fly for longer distances and extended periods of time – prior to this assay they were housed in smaller 60 × 60 × 60 cm mating cages.
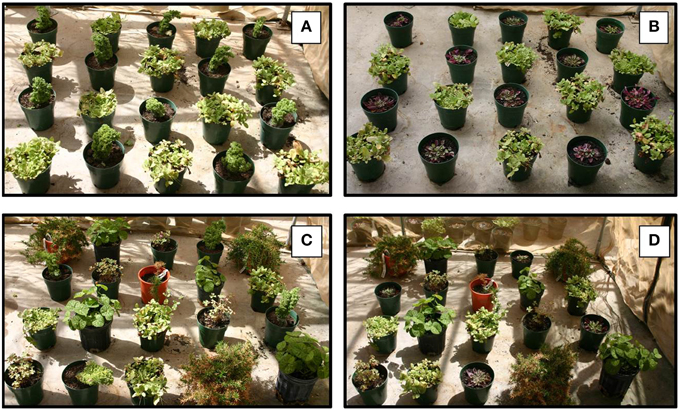
Figure 2. Experimental setup. Individual female butterflies experienced environments that varied in host color (green vs. red color) or non-host complexity, which varied in diversity and density of non-hosts. (A) Green host, simple non-host environment. (B) Red host, simple non-host environment. (C) Green host, complex non-host environment. (D) Red host, complex non-host environment.
For family-level measures of host-finding performance, we focused on the proportion of host-searching landings on hosts vs. non-hosts (arcsine-square root transformed for normality). We contrasted performance in two of the four search environments—the most “difficult” search environment (red host, complex non-host) and the simplest search environment (green host, simple non-host). “Difficulty” was assessed based on performance (host-finding efficiency) in these host-search environments: both host color and non-host complexity had independent effects on host-finding (see analyses in Snell-Rood and Papaj, 2009; Snell-Rood et al., 2009). For example, initial searching in the red host environment was close to finding hosts at random chance. Additionally, these two environments had the most pronounced differences among full-sibling groups in performance [e.g., red-complex, Family effect = F(9, 22) = 2.35, P = 0.04]. We focused on naïve individuals with at least 20 landings during their first-host-searching test period. We binned landings into a “naïve” category, landings 1–10, and an “experienced” category, landings 11–20. We took the average performance values for siblings from a family, using only families with at least 2 individuals for a given category (range 2–5, mean = 3.4 and 2.7 individuals for red-complex and green-simple environments).
For measures of search speed, we focused on the median time between host-search landings (in seconds). It is important to note that during this time measurement, female butterflies are visually inspecting plants prior to landing on them and chemically gathering information with foretarsi after landing on them (rev. Hern et al., 1996). Thus, our measure of search time combines two types of exploration into one value. We take time flying between plants and time in contact with plants as one measurement of exploration time. This measure showed significant variation among full-sibling groups for initial landings in a model that controlled for search environment [landings 1–10: Family, F(11, 183) = 2.11, P = 0.02, host color, P = 0.004, NH complexity, P = 0.97]. For later landings, time variation across families was not significant [landings 11–20: Family, F(11, 110) = 0.55, P = 0.86, host color, P = 0.02, NH complexity, P = 0.16], but we still analyzed this variable as a contrast between time periods (consistent with our brain and performance measures).
This measure of exploration time focused only on landings butterflies made during active host searching. We focus on active host-searching because these behaviors are presumably the most relevant for fitness. However, we also considered time spent in other activities such as time spent sitting on plants and total search time (which includes time spent trying to escape and time flying around other parts of the flight cage). Time spent sitting on plants and total search time were not related to family variation in thorax mass [sitting time: F(1, 9) = 0.16, P = 0.70; total flight time: F(1, 9) = 0.59, P = 0.46].
A complete description of neural methods and analyses can be found in Snell-Rood et al. (2009). Briefly, butterfly heads were fixed in formalin and stored in cacodylate buffer until dissection. They were stained with osmium, embedded in plastic and sectioned at 15 microns. The volume of each brain region was measured using Image J (NIH). We focused on family-specific estimates of the total brain (from a model that corrected for body size using hindwing area) and the volume of individual brain regions (from a model that corrected for total brain volume). For specific brain regions, we only focused on those that showed family-level variation of experienced individuals (see Table 6, Snell-Rood et al., 2009), the central body, antennal lobes and medulla (part of the optic lobe). Measures of experienced brain size came from models that controlled for specific host-searching experience (host color, non-host complexity and total landings of an individual, N = 49 individuals from 7 families).
All statistical tests focused on family-level measures (i.e., each data point is a full-sibling family). Analyses were performed in JMP 9.0 (SAS Institute).
Results
There was significant variation across full-sibling families in both forewing circularity and thorax mass relative to body size [forewing circularity: Family: F(11, 114) = 4.84, P < 0.0001; thorax mass: Family: F(10, 41) = 19.2, P < 0.0001, forewing area: F(1, 41) = 70.9, bST = 0.02, P < 0.0001]. These two proxies for movement were not significantly correlated (Spearman's ρ = −0.07, P = 0.83).
Butterfly families with more circular wings (less dispersive) were initially slower (i.e., had longer exploration times between host landings) during host search than those with more elongate wings [Figure 3; landings 1–10, F(1, 10) = 6.33, P = 0.03]. However, this trend reversed, in a marginally significant manner, later during host search [Figure 3; landings 11–20, F(1, 10) = 3.84, P = 0.08]. There were no significant relationships between search speed and thorax mass at emergence [landings 1–10, F(1, 9) = 0.54, P = 0.47; landings 11–20: F(1, 9) = 0, P = 0.98].
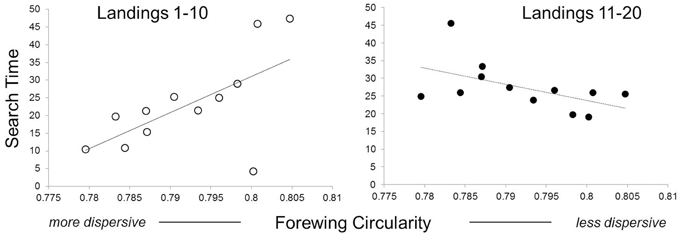
Figure 3. Effects of wing elongation on speed of host-searching. Each data point represents a full-sibling family. Families with more elongate (less circular) wings spent less time initially searching their host environment. Search time is measured as the median time between host-searching landings (in seconds) for a given butterfly family. The left panel shows behavior of naïve individuals (first 10 host-searching landings) while the right panel shows behavior of experienced individuals (second 10 host-searching landings).
Butterfly families that emerged with relatively greater thorax mass (more dispersive) had poorer performance in the more difficult host-searching environment, where butterflies searched for red hosts within a diverse and dense non-host environment. However, this relationship was not present for naïve butterflies—it emerged after host-search experience (Table 1, Figure 4) and remained significant following a Bonferroni correction for four comparisons. There was no relationship between thorax mass and family performance in the simple host-searching environment (Table 1). There were no significant relationships between forewing circularity and measures of host-finding performance, although there was a marginally significant positive relationship between forewing circularity and experienced performance (landings 11–20) in the green host, simple non-host environment (Table 2).
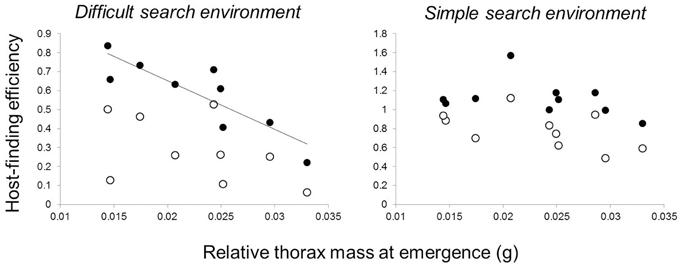
Figure 4. Effects of relative thorax mass on host-finding efficiency. Each data point represents a full-sibling family. Host-finding efficiency (the proportion of searching landings on hosts vs. non-hosts, arcsine-square-root transformed) tended to increase between naïve butterflies (open circles—first 10 landings of a completely naïve host search) and later experience (closed circles—second 10 landings in the same searching sequence). Butterflies searched in either a difficult searching environment, where a red-colored host was interspersed amongst a high density of four non-host types, or a simple search environment where a green-colored host was interspersed in a low density of one non-host type.
Butterfly families that emerged with relatively greater thorax mass had smaller brains and brain regions, but only for measurements performed on experienced individuals (Table 3, Figure 5). More specifically, sibling groups with relatively smaller thoraxes had greater total brain volume along with regions of the brain dedicated to the antennal lobes and the central bodies. However, this relationship was specific to brain measurements of experienced individuals, not naïve individuals, although there was a marginally significant relationship between total naïve brain volume and relative thorax volume (Table 3). Two of these relationships (antennal lobe and whole brain) remained significant after a Bonferroni correction that accounted for four brain regions, but not when accounting for all eight comparisons. There were no significant associations between forewing shape and neural measures (Table 4).
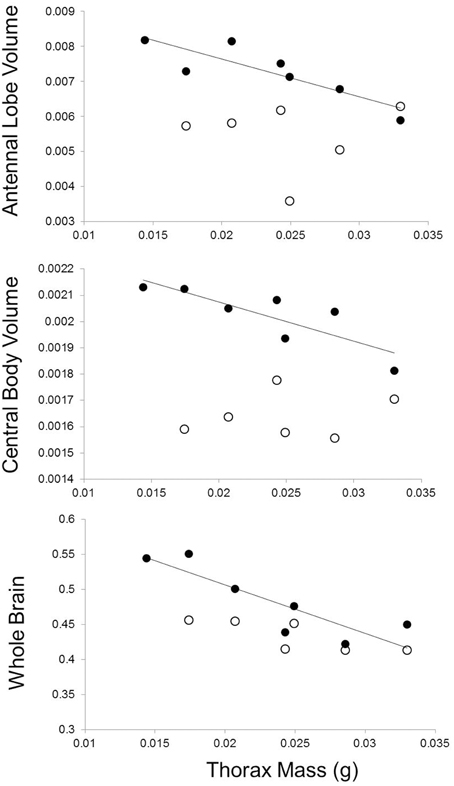
Figure 5. Effects of relative thorax mass on neural investment. Full-sibling families with relatively smaller thorax mass at emergence developed larger brains and brain regions (experienced individuals shown with closed circles). There was no significant relationship between brain measures and thorax mass for brain measures taken on individuals sacrificed at emergence (open circles). Measures of individual brain regions account for body size variation.
Discussion
Genetic Variation in Movement Drives Emergence of Behavioral Correlations
Our results support the hypothesis that genetic variation in traits related to movement and exploration of the environment can drive the development of behavioral syndromes. Across all described patterns, variation in movement traits at emergence was tied to cognitive traits, but only those behavioral traits measured in experienced individuals, not naïve individuals. We focused on two validated proxies for movement that differed significantly between full-sibling groups of cabbage white butterflies. Previous studies have found that butterflies with a larger relative thorax mass and more elongate wings fly faster and further (Betts and Wootton, 1988; Chai and Srygley, 1990; Dudley, 1990; Marden and Chai, 1991; Dudley and Srygley, 1994; Hill et al., 1999; Kingsolver and Srygley, 2000; Marden, 2000; Berwaerts et al., 2002, 2008; Norberg and Leimar, 2002; Berwaerts and Van Dyck, 2004; Dockx, 2007).
In the present study, we found three general patterns linking movement traits to behavioral traits. The first pattern suggests that individuals with different movement traits have different sampling strategies. Naïve females from families with more elongate (less circular) wings, explored their environment more rapidly when first searching for host plants (Figure 3). This suggests that more dispersive families explored visual and chemical plant cues less thoroughly than less dispersive families.
To evaluate whether these differences in movement might lead to the development of different aspects of cognition, we tested whether movement traits and cognition were correlated in naïve and experienced individuals. In both cases, we found movement-cognition correlations only in experienced individuals. First, females from families with smaller relative thorax mass (less dispersive) were more capable of finding atypical host plants in complex environments (Figure 4), but this correlation was only evident in the second 10 host landings, after initial exploration of their environment. This result suggests that families with smaller relative thorax masses can more successfully learn to navigate complex environments than families with larger thoraxes. Second, butterflies from families with smaller thoraxes (less dispersive) were also more likely to develop larger brains (Figure 5) with larger regions involved in sensation (antennal lobes) and movement (central body, Strauss, 2002; Neuser et al., 2008). However, there were no correlations between movement traits and neural investment at emergence, consistent with the idea that initial variation in movement may have affected neural development.
Taken together, these data are consistent with the idea that variation between families in movement affects sampling strategy, the ability to find atypical resources, and, over developmental time, learning and neural development. Throughout, we have assumed that the differences between sibling groups reflect genetic variation. However, it is important to note that because we used a full-sibling design as opposed to a split-sibling design, it's possible that the family-level variation stems in part from maternal effects. Either mechanism is consistent with the idea that initial variation in behavior could drive the development of behavioral syndromes, but teasing apart genetic and maternal effects may give insights into how such syndromes might evolve as the environment changes. More specifically, genetic correlations between movement and cognition that arise through gene-environment correlations have important evolutionary implications (Saltz and Nuzhdin, 2014). Niche-constructing behavior such as habitat preference or modification has the potential for generating complex evolutionary feedbacks because the selective environment potentially has a genetic component (Kerr and Feldman, 2003; Kylafis and Loreau, 2008). For instance, a decline in movement could decrease exposure to a range of environments, potentially weakening selection on learning and plasticity in certain environments (Sultan and Spencer, 2002; Scheiner et al., 2012). In some instances, by increasing the frequency of exposure to certain environments (e.g., atypical hosts), niche constructing behavior can speed up adaptation to those specific environments (Drown and Wade, 2014).
The present dataset is limited to only 12 full-sibling families. A more thorough quantitative genetic dissection of this question would consider a larger number of families. Such a design was not permissible in the present work which originally used behavioral measurements in four separate environments. However, we observed that the emergence of behavioral correlations was more pronounced in the most complex environment, similar to experiments in spiders which manipulated environmental enrichment (Bengston et al., 2014). These experiments suggest that the effects of niche construction and niche picking should be more pronounced in heterogeneous environments where behavioral variation would have more pronounced effects. From an experimental perspective, this suggests that limiting observations to one, complex environment would permit an increase in the number of families sampled, ideally using a split-sibling design to estimate maternal effects. Regardless, the limited family-level sample size for some of our comparisons (e.g., brain measures in Tables 3, 4) suggests that some of these comparisons should be treated as preliminary, informing follow-up studies.
We chose our proxies for movement based on existing research on butterfly flight patterns in over a dozen species, including those closely related to cabbage white butterflies (see citations above). Existing flight studies have considered butterflies in flight tunnels, tethered individuals or longer-distance free flight of wild individuals. These test conditions are somewhat different from the flight cage used here. However, the fact that thorax mass and wing shape tend to be important in a variety of experimental assays suggest they are also relevant for our assay. Additionally, while we were focused on short-distance flight patterns here, female cabbage whites move large distances in host-searching, sometimes 500–1000 m or more, spreading hundreds of eggs across many different host plants (Jones, 1977; Suzuki, 1978; Jones et al., 1980; Root and Kareiva, 1984). While it is likely these proxies for movement apply to host-searching in female cabbage whites, it's important that future studies validate the present patterns with measures of flight behavior in the field.
Overall, our results support the idea that variation in movement between families may result in the development of movement-cognition syndromes. For both neural measures and host-finding performance, movement traits (at emergence) were correlated with cognitive traits in experienced, but not naïve, individuals. Given the importance of exercise and enriched environments in neural development (Van Praag et al., 2000; Olson et al., 2006), it is not surprising that variation in movement between families could affect the expression of correlations between movement and cognition. Indeed, the same complex non-host and red host environments used in this experiment were previously shown to have positive effects on neural development in these butterflies (Snell-Rood et al., 2009). These results more broadly suggest that initial variation in a behavioral trait may affect the development of other traits, resulting in correlated behavior.
Insights into Movement-Cognition Syndromes Across Species
Our results linking measures of movement and cognitive behavior recall other systems where bold or dispersive genotypes are less behaviorally flexible. Such correlations within species have been seen in both birds and fish (Verbeek et al., 1994; Burns and Rodd, 2008; Exnerova et al., 2010; Guillette et al., 2011). Across species, similar correlations have been noted with respect to migratory birds—migratory species have smaller brains and are less behaviorally flexible than temperate residents that have to cope with drastic changes across seasons (Sol et al., 2005b). Similarly, resident species of parrots (relative to nomadic species) tend to explore their environment more thoroughly and carefully (Mettke-Hofmann et al., 2012). Negative movement-cognition syndromes have also been suggested within humans with respect to attention deficit hyperactivity disorder (Rosenthal and Allen, 1978; Biederman et al., 1991; Blickle, 1996; Furnham et al., 2009).
Across species, however, there are examples of both positive and negative movement-cognition syndromes (Verbeek et al., 1994; Dugatkin and Alfieri, 2003; Sneddon, 2003; Mery et al., 2007; Burns and Rodd, 2008; Exnerova et al., 2010; Guenther et al., 2014a). Taking a developmental niche construction perspective can help to clarify such variation. Increased movement and dispersal may increase the degree of environmental variation an individual experiences, increasing the benefits of behavioral plasticity and learning (Papaj, 1994; Scheiner, 2013). However, if individuals are choosing to interact with only a subset of resources or environments experienced during dispersal, they may actually be experiencing more stable, predictable conditions, which would favor the use of innate behavior. Indeed, our results hinted that more dispersive genotypes (more elongate wings) may do better with more typical, green hosts, for which these butterflies have an innate bias (Table 2).
Why might more dispersive individuals invest less in learning and cognition? It is possible that such negative correlations could result from a tradeoff between investment in costly neural tissue and flight muscle (Isler and Van Schaik, 2006; McGuire and Ratcliffe, 2011). However, because negative relationships between neural tissue and thorax mass were less pronounced or entirely absent at emergence suggests there may not be inherent tradeoffs. It is possible that less dispersive individuals are making the “best of a bad situation.” Nutritionally stressed larvae, for instance those with poor nitrogen assimilation abilities or access to a poor diet, may emerge as smaller adults, less able to fly around extensively. This idea recalls observations from other systems that early life nutritional environment may affect the development of behavioral syndromes (Andersson and Hoglund, 2012). However, it seems unlikely this explanation can account for the present results. We controlled for body size in our analyses; despite this, there were no significant relationships between family body size (a reflection of larval nutrition) and relative thorax mass or wing circularity. This idea also suggests that thorax mass and wing elongation would be more tightly correlated. A third explanation for such negative relationships between movement and cognition may be coexistence of a continuum of strategies. While more dispersive genotypes are likely to find more typical hosts spread over a broad area, less dispersive genotypes should be more likely to utilize locally common, less typical hosts. Overall, the fitness of these two strategies may well end up being identical. Movement-cognition syndromes may represent an instance where behavioral types coexist as different strategies with different routes to comparable fitness (Wolf et al., 2007, 2008).
Conclusions
This work contributes to a growing literature investigating the development of behavioral syndromes. A large number of studies have considered stability of behavioral correlations across ontogeny (Petelle et al., 2013; Boulton et al., 2014; Guenther et al., 2014b), which can give some insight into developmental mechanisms. A replicate genotype or quantitative genetics approach can give more insight into the developmental genetic architecture underlying a suite of correlated behaviors (Stamps and Groothuis, 2010a). The present study adds empirical weight to the idea that genetic variation in behavior such as movement or exploration can affect the development of behavioral syndromes. Such niche construction can result in gene-environment correlations and complex evolutionary feedbacks (Laland et al., 1999; Saltz and Nuzhdin, 2014). Given that most behavior affects how organisms experience the environment, and thus the subsequent development of traits, it's likely that such developmental feedbacks between traits and the environment (Figure 1) are a more general phenomenon in personality development, something that has long been recognized by psychologists studying human personality traits (Scarr and McCartney, 1983; Rutter and Silberg, 2002; Caspi et al., 2005).
Conflict of Interest Statement
The authors declare that the research was conducted in the absence of any commercial or financial relationships that could be construed as a potential conflict of interest.
Acknowledgments
Dan Papaj and Wulfila Gronenberg provided important insight and guidance on the design and analysis of the experiment that formed the basis for the present analysis. Bonnie Bell and Noel Eloriaga assisted with thorax measurements. Participants in the Santa Fe Institute's symposium on “Frontiers in Niche Construction” provided helpful comments on this dataset. ESR was supported in part by NSF IOS-1354737.
References
Andersson, M. A., and Hoglund, E. (2012). Linking personality to larval energy reserves in rainbow trout (Oncorhynchus mykiss). PLoS ONE 7:49247. doi: 10.1371/journal.pone.0049247
PubMed Abstract | Full Text | CrossRef Full Text | Google Scholar
Arai, J. A., and Feig, L. A. (2011). Long-lasting and transgenerational effects of an environmental enrichment on memory formation. Brain Res. Bull. 85, 30–35. doi: 10.1016/j.brainresbull.2010.11.003
PubMed Abstract | Full Text | CrossRef Full Text | Google Scholar
Bengston, S. E., Pruitt, J. N., and Riechert, S. E. (2014). Differences in environmental enrichment generate contrasting behavioural syndromes in a basal spider lineage. Anim. Behav. 93, 105–110. doi: 10.1016/j.anbehav.2014.04.022
Ben-Shahar, Y., Robichon, A., Sokolowski, M. B., and Robinson, G. E. (2002). Influence of gene action across different time scales on behavior. Science 296, 741–744. doi: 10.1126/science.1069911
PubMed Abstract | Full Text | CrossRef Full Text | Google Scholar
Bernays, E. A. (2001). Neural limitations in phytophagous insects: implications for diet breadth and evolution of host affiliation. Annu. Rev. Entomol. 46, 703–727. doi: 10.1146/annurev.ento.46.1.703
PubMed Abstract | Full Text | CrossRef Full Text | Google Scholar
Berwaerts, K., Matthysen, E., and Van Dyck, H. (2008). Take-off flight performance in the butterfly Pararge aegeria relative to sex and morphology: a quantitative genetic assessment. Evolution 62, 2525–2533. doi: 10.1111/j.1558-5646.2008.00456.x
PubMed Abstract | Full Text | CrossRef Full Text | Google Scholar
Berwaerts, K., and Van Dyck, H. (2004). Take-off performance under optimal and suboptimal thermal conditions in the butterfly Pararge aegeria. Oecologia 141, 536–545. doi: 10.1007/s00442-004-1661-9
PubMed Abstract | Full Text | CrossRef Full Text | Google Scholar
Berwaerts, K., Van Dyck, H., and Aerts, P. (2002). Does flight morphology relate to flight performance? An experimental test with the butterfly Pararge aegeria. Funct. Ecol. 16, 484–491. doi: 10.1046/j.1365-2435.2002.00650.x
Betts, C. R., and Wootton, R. J. (1988). Wing shape and flight behavior in butterflies (Lepidoptera, Papilionoidea and Hesperioidea)—a preliminary analysis. J. Exp. Biol. 138, 271–288.
Biederman, J., Newcorn, J., and Sprich, S. (1991). Comorbidity of attention deficit hyperactivity disorder with conduct, depressive anxiety, and other disorders. Am. J. Psychiatry 148, 564–577. doi: 10.1176/ajp.148.5.564
PubMed Abstract | Full Text | CrossRef Full Text | Google Scholar
Bishop, L., and Riechert, S. E. (1990). Spider colonization of agroecosystems—mode and source. Environ. Entomol. 19, 1738–1745. doi: 10.1093/ee/19.6.1738
Blickle, G. (1996). Personality traits, learning strategies, and performance. Eur. J. Pers. 10, 337–352.
Bolhuis, J. E., Schouten, W. G. P., De Leeuw, J. A., Schrama, J. W., and Wiegant, V. A. (2004). Individual coping characteristics, rearing conditions and behavioural flexibility in pigs. Behav. Brain Res. 152, 351–360. doi: 10.1016/j.bbr.2003.10.024
PubMed Abstract | Full Text | CrossRef Full Text | Google Scholar
Bolnick, D. I., Amarasekare, P., Araujo, M. S., Burger, R., Levine, J. M., Novak, M., et al. (2011). Why intraspecific trait variation matters in community ecology. Trends Ecol. Evol. 26, 183–192. doi: 10.1016/j.tree.2011.01.009
PubMed Abstract | Full Text | CrossRef Full Text | Google Scholar
Boulton, K., Grimmer, A. J., Rosenthal, G. G., Walling, C. A., and Wilson, A. J. (2014). How stable are personalities? A multivariate view of behavioural variation over long and short timescales in the sheepshead swordtail, Xiphophorus birchmanni. Behav. Ecol. Sociobiol. 68, 791–803. doi: 10.1007/s00265-014-1692-0
Burns, J. G., and Rodd, F. H. (2008). Hastiness, brain size and predation regime affect the performance of wild guppies in a spatial memory task. Anim. Behav. 76, 911–922. doi: 10.1016/j.anbehav.2008.02.017
Caspi, A., Roberts, B. W., and Shiner, R. L. (2005). Personality development: stability and change. Annu. Rev. Psychol. 56, 453–484. doi: 10.1146/annurev.psych.55.090902.141913
PubMed Abstract | Full Text | CrossRef Full Text | Google Scholar
Chai, P., and Srygley, R. B. (1990). Predation and the flight, morphology, and temperature of neotropical rain forest butterflies. Am. Natural. 135, 748–765. doi: 10.1086/285072
Chittka, L., Skorupski, P., and Raine, N. E. (2009). Speed-accuracy tradeoffs in animal decision making. Trends Ecol. Evol. 24, 400–407. doi: 10.1016/j.tree.2009.02.010
PubMed Abstract | Full Text | CrossRef Full Text | Google Scholar
Cote, J., Clobert, J., Brodin, T., Fogarty, S., and Sih, A. (2010). Personality-dependent dispersal: characterization, ontogeny and consequences for spatially structured populations. Philos. Trans. R. Soc. Lond. B Biol. Sci. 365, 4065–4076. doi: 10.1098/rstb.2010.0176
PubMed Abstract | Full Text | CrossRef Full Text | Google Scholar
Dall, S. R. X., Houston, A. I., and McNamara, J. M. (2004). The behavioural ecology of personality: consistent individual differences from an adaptive perspective. Ecol. Lett. 7, 734–739. doi: 10.1111/j.1461-0248.2004.00618.x
Dickman, S. J., and Meyer, D. E. (1988). Impulsivity and speed accuracy tradeoffs in information processing. J. Pers. Soc. Psychol. 54, 274–290. doi: 10.1037/0022-3514.54.2.274
PubMed Abstract | Full Text | CrossRef Full Text | Google Scholar
Dirienzo, N., Pruitt, J. N., and Hedrick, A. V. (2012). Juvenile exposure to acoustic sexual signals from conspecifics alters growth trajectory and an adult personality trait. Anim. Behav. 84, 861–868. doi: 10.1016/j.anbehav.2012.07.007
Dockx, C. (2007). Directional and stabilizing selection on wing size and shape in migrant and resident monarch butterflies, Danaus plexippus (L.), in Cuba. Biol. J. Linn. Soc. 92, 605–616. doi: 10.1111/j.1095-8312.2007.00886.x
Drown, D. M., and Wade, M. J. (2014). Runaway coevolution: adaptation to heritable and nonheritable environments. Evolution 68, 3039–3046. doi: 10.1111/evo.12470
PubMed Abstract | Full Text | CrossRef Full Text | Google Scholar
Duckworth, R. A. (2010). Evolution of personality: developmental constraints on behavioral flexibility. Auk 127, 752–758. doi: 10.1525/auk.2010.127.4.752
Dudley, R. (1990). Biomechanics of flight in neotropical butterflies—morphometrics and kinematics. J. Exp. Biol. 150, 37–53.
Dudley, R., and Srygley, R. B. (1994). Flight physiology of neotropical butterflies—allometry of airspeeds during natural free flight. J. Exp. Biol. 191, 125–139.
Dugatkin, L. A., and Alfieri, M. S. (2003). Boldness, behavioral inhibition and learning. Ethol. Ecol. Evol. 15, 43–49. doi: 10.1080/08927014.2003.9522689
Dukas, R. (2002). Behavioural and ecological consequences of limited attention. Philos. Trans. R. Soc. Lond. B Biol. Sci. 357, 1539–1547. doi: 10.1098/rstb.2002.1063
PubMed Abstract | Full Text | CrossRef Full Text | Google Scholar
Edenbrow, M., and Croft, D. P. (2013). Environmental and genetic effects shape the development of personality traits in the mangrove killifish Kryptolebias marmoratus. Oikos 122, 667–681. doi: 10.1111/j.1600-0706.2012.20556.x
Exnerova, A., Svadova, K. H., Fucikova, E., Drent, P., and Stys, P. (2010). Personality matters: individual variation in reactions of naive bird predators to aposematic prey. Proc. R. Soc. B Biol. Sci. 277, 723–728. doi: 10.1098/rspb.2009.1673
PubMed Abstract | Full Text | CrossRef Full Text | Google Scholar
Furnham, A., Monsen, J., and Ahmetoglu, G. (2009). Typical intellectual engagement, Big Five personality traits, approaches to learning and cognitive ability predictors of academic performance. Br. J. Edu. Psychol. 79, 769–782. doi: 10.1348/978185409X412147
PubMed Abstract | Full Text | CrossRef Full Text | Google Scholar
Guenther, A., Brust, V., Dersen, M., and Trillmich, F. (2014a). Learning and personality types are related in cavies (Cavia aperea). J. Comp. Psychol. 128, 74–81. doi: 10.1037/a0033678
PubMed Abstract | Full Text | CrossRef Full Text | Google Scholar
Guenther, A., Finkemeier, M. A., and Trillmich, F. (2014b). The ontogeny of personality in the wild guinea pig. Anim. Behav. 90, 131–139. doi: 10.1016/j.anbehav.2014.01.032
Guillette, L. M., Reddon, A. R., Hoeschele, M., and Sturdy, C. B. (2011). Sometimes slower is better: slow-exploring birds are more sensitive to changes in a vocal discrimination task. Proc. R. Soc. B Biol. Sci. 278, 767–773. doi: 10.1098/rspb.2010.1669
PubMed Abstract | Full Text | CrossRef Full Text | Google Scholar
Hanski, I., Eralahti, C., Kankare, M., Ovaskainen, O., and Siren, H. (2004). Variation in migration propensity among individuals maintained by landscape structure. Ecol. Lett. 7, 958–966. doi: 10.1111/j.1461-0248.2004.00654.x
Henderson, N. D., Turri, M. G., Defries, J. C., and Flint, J. (2004). QTL analysis of multiple behavioral measures of anxiety in mice. Behav. Genet. 34, 267–293. doi: 10.1023/B:BEGE.0000017872.25069.44
PubMed Abstract | Full Text | CrossRef Full Text | Google Scholar
Hern, A., Edwardsjones, G., and McKinlay, R. G. (1996). A review of the pre-oviposition behaviour of the small cabbage white butterfly, Pieris rapae (Lepidoptera: Pieridae). Ann. Appl. Biol. 128, 349–371. doi: 10.1111/j.1744-7348.1996.tb07328.x
Higgins, S. I., and Richardson, D. M. (1999). Predicting plant migration rates in a changing world: the role of long-distance dispersal. Am. Nat. 153, 464–475. doi: 10.1086/303193
Hill, J. K., Thomas, C. D., and Blakeley, D. S. (1999). Evolution of flight morphology in a butterfly that has recently expanded its geographic range. Oecologia 121, 165–170. doi: 10.1007/s004420050918
Isler, K., and Van Schaik, C. (2006). Costs of encephalization: the energy trade-off hypothesis tested on birds. J. Hum. Evol. 51, 228–243. doi: 10.1016/j.jhevol.2006.03.006
PubMed Abstract | Full Text | CrossRef Full Text | Google Scholar
Jones, R. E. (1977). Movement patterns and egg distribution in cabbage butterflies. J. Anim. Ecol. 46, 195–212. doi: 10.2307/3956
Jones, R. E., Gilbert, N., Guppy, M., and Nealis, V. (1980). Long-distance movement of Pieris rapae. J. Anim. Ecol. 49, 629–642. doi: 10.2307/4268
Kandori, I., and Ohsaki, N. (1996). The learning abilities of the white cabbage butterfly, Pieris rapae, foraging for flowers. Res. Pop. Ecol. 38, 111–117. doi: 10.1007/BF02514977
Kerr, B., and Feldman, M. W. (2003). Carving the cognitive niche: optimal learning strategies in homogeneous and heterogeneous environments. J. Theor. Biol. 220, 169–188. doi: 10.1006/jtbi.2003.3146
PubMed Abstract | Full Text | CrossRef Full Text | Google Scholar
Kingsolver, J. G., and Srygley, R. B. (2000). Experimental analyses of body size, flight and survival in pierid butterflies. Evol. Ecol. Res. 2, 593–612. Available online at: http://www.evolutionary-ecology.com/abstracts/v02/1184.html
Kotiaho, J. S., Kaitala, V., Komonen, A., and Paivinen, J. (2005). Predicting the risk of extinction from shared ecological characteristics. Proc. Natl. Acad. Sci. U.S.A. 102, 1963–1967. doi: 10.1073/pnas.0406718102
PubMed Abstract | Full Text | CrossRef Full Text | Google Scholar
Kylafis, G., and Loreau, M. (2008). Ecological and evolutionary consequences of niche construction for its agent. Ecol. Lett. 11, 1072–1081. doi: 10.1111/j.1461-0248.2008.01220.x
PubMed Abstract | Full Text | CrossRef Full Text | Google Scholar
Laland, K. N., Odling-Smee, F. J., and Feldman, M. W. (1999). Evolutionary consequences of niche construction and their implications for ecology. Proc. Natl. Acad. Sci. U.S.A. 96, 10242–10247. doi: 10.1073/pnas.96.18.10242
PubMed Abstract | Full Text | CrossRef Full Text | Google Scholar
Lewis, A. C. (1986). Memory constraints and flower choice in Pieris rapae. Science 232, 863–865. doi: 10.1126/science.232.4752.863
PubMed Abstract | Full Text | CrossRef Full Text | Google Scholar
Marchetti, C., and Drent, P. J. (2000). Individual differences in the use of social information in foraging by captive great tits. Anim. Behav. 60, 131–140. doi: 10.1006/anbe.2000.1443
PubMed Abstract | Full Text | CrossRef Full Text | Google Scholar
Marden, J. H. (2000). Variability in the size, composition, and function of insect flight muscles. Annu. Rev. Physiol. 62, 157–178. doi: 10.1146/annurev.physiol.62.1.157
PubMed Abstract | Full Text | CrossRef Full Text | Google Scholar
Marden, J. H., and Chai, P. (1991). Aerial predation and butterfly design—how palatability, mimicry, and the need for evasive flight constrain mass allocation. Am. Nat. 138, 15–36. doi: 10.1086/285202
McGuire, L. P., and Ratcliffe, J. M. (2011). Light enough to travel: migratory bats have smaller brains, but not larger hippocampi, than sedentary species. Biol. Lett. 7, 233–236. doi: 10.1098/rsbl.2010.0744
PubMed Abstract | Full Text | CrossRef Full Text | Google Scholar
Mery, F., Belay, A. T., So, A. K. C., Sokolowski, M. B., and Kawecki, T. J. (2007). Natural polymorphism affecting learning and memory in Drosophila. Proc. Natl. Acad. Sci. U.S.A. 104, 13051–13055. doi: 10.1073/pnas.0702923104
PubMed Abstract | Full Text | CrossRef Full Text | Google Scholar
Mettke-Hofmann, C., Wink, M., Braun, M., and Winkler, H. (2012). Residency and a broad feeding spectrum are related to extensive spatial exploration in parrots. Behav. Ecol. 23, 1365–1371. doi: 10.1093/beheco/ars130
Montiglio, P. O., Ferrari, C., and Reale, D. (2013). Social niche specialization under constraints: personality, social interactions and environmental heterogeneity. Philos. Trans. R. Soc. B Biol. Sci. 368:20120343. doi: 10.1098/rstb.2012.0343
PubMed Abstract | Full Text | CrossRef Full Text | Google Scholar
Neuser, K., Triphan, T., Mronz, M., Poeck, B., and Strauss, R. (2008). Analysis of a spatial orientation memory in Drosophila. Nature 453, 1244–U1244. doi: 10.1038/nature07003
PubMed Abstract | Full Text | CrossRef Full Text | Google Scholar
Norberg, U., and Leimar, O. (2002). Spatial and temporal variation in flight morphology in the butterfly Melitaea cinxia (Lepidoptera: Nymphalidae). Biol. J. Linn. Soc. 77, 445–453. doi: 10.1046/j.1095-8312.2002.00115.x
Olson, A. K., Eadie, B. D., Ernst, C., and Christie, B. R. (2006). Environmental enrichment and voluntary exercise massively increase neurogenesis in the adult hippocampus via dissociable pathways. Hippocampus 16, 250–260. doi: 10.1002/hipo.20157
PubMed Abstract | Full Text | CrossRef Full Text | Google Scholar
Papaj, D. R. (1986a). Conditioning of leaf shape discrimination by chemical cues in the butterfly Battus philenor. Anim. Behav. 34, 1281–1288. doi: 10.1016/S0003-3472(86)80199-3
Papaj, D. R. (1986b). Interpopulation differences in host preference and the evolution of learnin gin the butterfly Battus philenor. Evolution 40, 518–530. doi: 10.2307/2408574
Papaj, D. R. (1994). “Optimizing learning and its effect on evolutionary change,” in Behavioral Mechanisms in Evolutionary Ecology, ed L. A. Real (Chicago, IL: University of Chicago Press), 133–153.
Papaj, D. R., and Prokopy, R. J. (1989). Ecological and evolutionary aspects of learning in phytophagous insects. Annu. Rev. Entomol. 34, 315–350. doi: 10.1146/annurev.en.34.010189.001531
Petelle, M. B., McCoy, D. E., Alejandro, V., Martin, J. G. A., and Blumstein, D. T. (2013). Development of boldness and docility in yellow-bellied marmots. Anim. Behav. 86, 1147–1154. doi: 10.1016/j.anbehav.2013.09.016
Poyry, J., Luoto, M., Heikkinen, R. K., Kuussaari, M., and Saarinen, K. (2009). Species traits explain recent range shifts of Finnish butterflies. Glob. Change Biol. 15, 732–743. doi: 10.1111/j.1365-2486.2008.01789.x
Root, R. B., and Kareiva, P. M. (1984). The search for resources by cabbage butterflies (Pieris rapae)—ecological consequences and adaptive significance of Markovian movements in a patchy environment. Ecology 65, 147–165. doi: 10.2307/1939467
Rosenthal, R. H., and Allen, T. W. (1978). Examination of attention, arousal, and learning dysfunctions of hyperkinetic children. Psychol. Bull. 85, 689–715. doi: 10.1037/0033-2909.85.4.689
PubMed Abstract | Full Text | CrossRef Full Text | Google Scholar
Rutter, M., and Silberg, J. (2002). Gene-environment interplay in relation to emotional and behavioral disturbance. Annu. Rev. Psychol. 53, 463–490. doi: 10.1146/annurev.psych.53.100901.135223
PubMed Abstract | Full Text | CrossRef Full Text | Google Scholar
Saltz, J. B. (2011). Natural genetic variation in social environmentl choice: context-dependent gene-environment correlation in Drosophila melanogaster. Evolution 65, 2325–2334. doi: 10.1111/j.1558-5646.2011.01295.x
PubMed Abstract | Full Text | CrossRef Full Text | Google Scholar
Saltz, J. B., and Nuzhdin, S. V. (2014). Genetic variation in niche construction: implications for development and evolutionary genetics. Trends Ecol. Evol. 29, 8–14. doi: 10.1016/j.tree.2013.09.011
PubMed Abstract | Full Text | CrossRef Full Text | Google Scholar
Scarr, S., and McCartney, K. (1983). How people make their own environments—a theory of genotype-environment effects. Child Dev. 54, 424–435.
Scheiner, S. M. (2013). The genetics of phenotypic plasticity. XII. Temporal and spatial heterogeneity. Ecol. Evol. 3, 4596–4609. doi: 10.1002/ece3.792
PubMed Abstract | Full Text | CrossRef Full Text | Google Scholar
Scheiner, S. M., Barfield, M., and Holt, R. D. (2012). The genetics of phenotypic plasticity. XI. Joint evolution of plasticity and dispersal rate. Ecol. Evol. 2, 2027–2039. doi: 10.1002/ece3.327
PubMed Abstract | Full Text | CrossRef Full Text | Google Scholar
Sih, A., Bell, A., and Johnson, J. C. (2004a). Behavioral syndromes: an ecological and evolutionary overview. Trends Ecol. Evol. 19, 372–378. doi: 10.1016/j.tree.2004.04.009
PubMed Abstract | Full Text | CrossRef Full Text | Google Scholar
Sih, A., Bell, A. M., Johnson, J. C., and Ziemba, R. E. (2004b). Behavioral syndromes: an integrative overview. Q. Rev. Biol. 79, 241–277. doi: 10.1086/422893
PubMed Abstract | Full Text | CrossRef Full Text | Google Scholar
Sih, A., and Del Giudice, M. (2012). Linking behavioural syndromes and cognition: a behavioural ecology perspective. Philos. Trans. R. Soc. B Biol. Sci. 367, 2762–2772. doi: 10.1098/rstb.2012.0216
PubMed Abstract | Full Text | CrossRef Full Text | Google Scholar
Smallegange, R. C., Everaarts, T. C., and Van Loon, J. J. A. (2006). Associative learning of visual and gustatory cues in the large cabbage white butterfly, Pieris brassicae. Anim. Biol. 56, 157–172. doi: 10.1163/157075606777304159
Sneddon, L. U. (2003). The bold and the shy: individual differences in rainbow trout. J. Fish Biol. 62, 971–975. doi: 10.1046/j.1095-8649.2003.00084.x
Snell-Rood, E. C., and Papaj, D. R. (2009). Patterns of phenotypic plasticity in common and rare environments: a study of host use and color learning in the cabbage white butterfly Pieris rapae. Am. Nat. 173, 615–631. doi: 10.1086/597609
PubMed Abstract | Full Text | CrossRef Full Text | Google Scholar
Snell-Rood, E. C., Papaj, D. R., and Gronenberg, W. (2009). Brain size: a global or induced cost of learning? Brain Behav. Evol. 73, 111–128. doi: 10.1159/000213647
PubMed Abstract | Full Text | CrossRef Full Text | Google Scholar
Snell-Rood, E., Davidowtiz, G., and Papaj, D. (2013). Plasticity in learning causes immediate and trans-generational changes in allocation of resources. Integr. Comp. Biol. 53, 329–339. doi: 10.1093/icb/ict030
PubMed Abstract | Full Text | CrossRef Full Text | Google Scholar
Sol, D., Duncan, R. P., Blackburn, T. M., Cassey, P., and Lefebvre, L. (2005a). Big brains, enhanced cognition, and response of birds to novel environments. Proc. Natl. Acad. Sci. U.S.A. 102, 5460–5465. doi: 10.1073/pnas.0408145102
PubMed Abstract | Full Text | CrossRef Full Text | Google Scholar
Sol, D., Lefebvre, L., and Rodriguez-Teijeiro, J. D. (2005b). Brain size, innovative propensity and migratory behaviour in temperate Palaearctic birds. Proc. R. Soc. B Biol. Sci. 272, 1433–1441. doi: 10.1098/rspb.2005.3099
PubMed Abstract | Full Text | CrossRef Full Text | Google Scholar
Sol, D., Timmermans, S., and Lefebvre, L. (2002). Behavioural flexibility and invasion success in birds. Anim. Behav. 63, 495–502. doi: 10.1006/anbe.2001.1953
PubMed Abstract | Full Text | CrossRef Full Text | Google Scholar
Stamps, J. A., and Groothuis, T. G. G. (2010b). Developmental perspectives on personality: implications for ecological and evolutionary studies of individual differencese. Philos. Trans. R. Soc. B Biol. Sci. 365, 4029–4041. doi: 10.1098/rstb.2010.0218
PubMed Abstract | Full Text | CrossRef Full Text | Google Scholar
Stamps, J., and Groothuis, T. G. G. (2010a). The development of animal personality: relevance, concepts and perspectives. Biol. Rev. 85, 301–325. doi: 10.1111/j.1469-185X.2009.00103.x
PubMed Abstract | Full Text | CrossRef Full Text | Google Scholar
Stjernholm, F., and Karlsson, B. (2008). Flight muscle breakdown in the green-veined white butterfly, Pieris napi (Lepidoptera: Pieridae). Eur. J. Entomol. 105, 87–91. doi: 10.14411/eje.2008.012
PubMed Abstract | Full Text | CrossRef Full Text | Google Scholar
Stjernholm, F., Karlsson, B., and Boggs, C. L. (2005). Age-related changes in thoracic mass: possible reallocation of resources to reproduction in butterflies. Biol. J. Linn. Soc. 86, 363–380. doi: 10.1111/j.1095-8312.2005.00542.x
Stotz, K. (2010). Human nature and cognitive-developmental niche construction. Phenomenol. Cogn. Sci. 9, 483–501. doi: 10.1007/s11097-010-9178-7
Strauss, R. (2002). The central complex and the genetic dissection of locomotor behaviour. Curr. Opin. Neurobiol. 12, 633–638. doi: 10.1016/S0959-4388(02)00385-9
PubMed Abstract | Full Text | CrossRef Full Text | Google Scholar
Sultan, S. E., and Spencer, H. G. (2002). Metapopulation structure favors plasticity over local adaptation. Am. Nat. 160, 271–283. doi: 10.1086/341015
PubMed Abstract | Full Text | CrossRef Full Text | Google Scholar
Suzuki, Y. (1978). Adult longevity and reproductive potential of the small cabbage white, Pieris rapae erucivora Boisduval (Lepidoptera: Pieridae). Appl. Entomol. Zool. 13, 312–313.
Thomas, C. D., Bodsworth, E. J., Wilson, R. J., Simmons, A. D., Davies, Z. G., Musche, M., et al. (2001). Ecological and evolutionary processes at expanding range margins. Nature 411, 577–581. doi: 10.1038/35079066
PubMed Abstract | Full Text | CrossRef Full Text | Google Scholar
Traynier, R. M. M. (1984). Associative learning in the ovipositional behaviour of the cabbage butterfly, Pieris rapae. Physiol. Entomol. 9, 465–472. doi: 10.1111/j.1365-3032.1984.tb00789.x
Traynier, R. M. M. (1986). Visual learning in assays of sinigrin solution as an oviposition releaser for the cabbage butterflies Pieris rapae. Entomol. Exp. Appl. 40, 25–33. doi: 10.1111/j.1570-7458.1986.tb02151.x
Van Praag, H., Kempermann, G., and Gage, F. H. (2000). Neural consequences of environmental enrichment. Nat. Rev. Neurosci. 1, 191–198. doi: 10.1038/35044558
PubMed Abstract | Full Text | CrossRef Full Text | Google Scholar
Verbeek, M. E. M., Drent, P. J., and Wiepkema, P. R. (1994). Consistent individual differences in early exploratory behavior of male great tits. Anim. Behav. 48, 1113–1121. doi: 10.1006/anbe.1994.1344
PubMed Abstract | Full Text | CrossRef Full Text | Google Scholar
Weiss, M. R., and Papaj, D. R. (2003). Colour learning in two behavioural contexts: how much can a butterfly keep in mind? Anim. Behav. 65, 425–434. doi: 10.1006/anbe.2003.2084
Wolf, M., Van Doorn, G. S., Leimar, O., and Weissing, F. J. (2007). Life-history trade-offs favour the evolution of animal personalities. Nature 447, 581–584. doi: 10.1038/nature05835
PubMed Abstract | Full Text | CrossRef Full Text | Google Scholar
Wolf, M., Van Doorn, G. S., and Weissing, F. J. (2008). Evolutionary emergence of responsive and unresponsive personalities. Proc. Natl. Acad. Sci. U.S.A. 105, 15825–15830. doi: 10.1073/pnas.0805473105
PubMed Abstract | Full Text | CrossRef Full Text | Google Scholar
Keywords: flight muscle, wing shape, brain size, cognition, personality
Citation: Snell-Rood EC and Steck M (2015) Experience drives the development of movement-cognition correlations in a butterfly. Front. Ecol. Evol. 3:21. doi: 10.3389/fevo.2015.00021
Received: 19 December 2014; Accepted: 21 February 2015;
Published: 18 March 2015.
Edited by:
Ann Valerie Hedrick, University of California, Davis, USAReviewed by:
Judy Stamps, University of California, Davis, USAAndreas Modlmeier, University of Pittsburgh, USA
Copyright © 2015 Snell-Rood and Steck. This is an open-access article distributed under the terms of the Creative Commons Attribution License (CC BY). The use, distribution or reproduction in other forums is permitted, provided the original author(s) or licensor are credited and that the original publication in this journal is cited, in accordance with accepted academic practice. No use, distribution or reproduction is permitted which does not comply with these terms.
*Correspondence: Emilie C. Snell-Rood, Department of Ecology, Evolution and Behavior, University of Minnesota, 100 Ecology Building, 1987 Upper Buford Circle, St. Paul, MN 55108, USAZW1pbGllc0B1bW4uZWR1